- 1Department of Urology, Zhongnan Hospital of Wuhan University, Wuhan, China
- 2Department of Biological Repositories, Zhongnan Hospital of Wuhan University, Wuhan, China
- 3Center for Evidence-Based and Translational Medicine, Zhongnan Hospital of Wuhan University, Wuhan, China
Objective: To identify candidate biomarkers correlated with clinical prognosis of patients with bladder cancer (BC).
Methods: Weighted gene co-expression network analysis was applied to build a co-expression network to identify hub genes correlated with tumor node metastasis (TNM) staging of BC patients. Functional enrichment analysis was conducted to functionally annotate the hub genes. Protein-protein interaction network analysis of hub genes was performed to identify the interactions among the hub genes. Survival analyses were conducted to characterize the role of hub genes on the survival of BC patients. Gene set enrichment analyses were conducted to find the potential mechanisms involved in the tumor proliferation promoted by hub genes.
Results: Based on the results of topological overlap measure based clustering and the inclusion criteria, top 50 hub genes were identified. Hub genes were enriched in cell proliferation associated gene ontology terms (mitotic sister chromatid segregation, mitotic cell cycle and, cell cycle, etc.) and Kyoto Encyclopedia of Genes and Genomes (KEGG) pathways (cell cycle, Oocyte meiosis, etc.). 17 hub genes were found to interact with ≥5 of the hub genes. Survival analysis of hub genes suggested that lower expression of MMP11, COL5A2, CDC25B, TOP2A, CENPF, CDCA3, TK1, TPX2, CDCA8, AEBP1, and FOXM1were associated with better overall survival of BC patients. BC samples with higher expression of hub genes were enriched in gene sets associated with P53 pathway, apical junction, mitotic spindle, G2M checkpoint, and myogenesis, etc.
Conclusions: We identified several candidate biomarkers correlated with the TNM staging and overall survival of BC patients. Accordingly, they might be used as potential diagnostic biomarkers and therapeutic targets with clinical utility.
Introduction
Bladder cancer (BC) is the second most frequent genitourinary malignancy and the sixth most common malignancy in men. BC represents a spectrum of disease ranging from superficial, well-differentiated disease, which does not significantly affect the survival of BC patients, to highly fatal tumors for which long-term survival may be dismal (So, 2016; Ghervan et al., 2017). With the aging of population, the incidence of BC is rising year by year, and BC in elder patients will become even more frequent and evolved into a public health challenge in future. For patients with superficial BC, telescopic removal of the cancer (transurethral resection of bladder tumor, TURBT) followed by instillation of chemotherapy or vaccine-based therapy into the bladder with prolonged telescopic checking of the bladder are usually recommended, and the 5-year overall survival for these patients reaches 90%, while about 40–80% of these patients will develop disease recurrence or progression (Malmström et al., 2017). For patients with invasive BC, radical cystectomy plus pelvic lymph node dissection (PLND) followed by neo-adjuvant chemotherapy is recommended as a standard of care, and once it becomes metastatic cancer, the 5-year overall survival for patients with invasive BC is a dismal 6% (Salama et al., 2016; Sargos et al., 2016).
Biomarkers are biological substances whose detection indicates a particular disease state. So far, a variety of biomarkers have been introduced in daily clinical practice, including risk assessment, screening, differential diagnosis, determination of prognosis, prediction of response to treatment, and monitoring of progression of disease. Thus, identification of biomarkers that are associated with clinical outcomes of patients with BC might be of clinical significance (Giunchi et al., 2017).
Currently, several screening algorithms based on gene expression data, including Gene Set Enrichment Analysis (GSEA), Signalling Pathway Impact Analysis (SPIA), Topology Gene-Set Analysis, and DEGraph, and in silico Pathway Activation Network Decomposition Analysis (iPANDA), etc., have been introduced for both academic and commercial purpose, and most of these algorithms are intended to identify differentially expressed genes between groups of samples (Ozerov et al., 2016). Weighted gene co-expression network analysis (WGCNA), a systems biology algorithm, can be applied to describing the correlation patterns among genes across microarray samples, finding and summarizing modules of high related genes, and relating modules to certain clinical phenotype (Zhang and Horvath, 2005; Yip and Horvath, 2007). During the network construction, highly co-expressed genes are connected in the network and grouped into modules, thus, different modules included different functionally related genes and the most central and connected genes are treated as hub genes (Zhang and Horvath, 2005; Yip and Horvath, 2007). Correlation networks facilitate network based gene screening methods that can be used to identify candidate biomarkers or therapeutic targets. Therefore, WGCNA is usually used to facilitate the screening or identification of candidate biomarkers or therapeutic targets. Tumor node metastasis (TNM) 2009 (7th edition) was recommended for the BC, and previous studies demonstrated that patients with an infiltrative pattern had better survival than those with other pattern types (PDQ Adult Treatment Editorial Board, 2002; Yaxley, 2016). In the present study, the WGCNA algorithm was applied to identify candidate biomarkers for BC based on the TNM staging of BC patients.
Methods
Data Sources and Data Preprocessing
Gene expression profile of GSE13507 (Kim et al., 2010; Lee et al., 2010) was downloaded from Gene Expression Omnibus (GEO) database. GSE13507 is a microarray dataset containing 165 primary BC samples, 23 recurrent non-muscle invasive tumor tissues, 58 normal looking bladder mucosae surrounding cancer and 10 normal bladder mucosae, the clinical characteristics of included samples are attached as well. In the present study, only the 165 primary BC samples were included for subsequent analysis. Preprocessed gene expression profile of GSE13507 was obtained from GEO database for our WGCNA analysis. Probesets were filtered by their variance across all samples, only probes with variances ranked in top 10,000 were selected for subsequent analyses.
Co-expression Network Construction and Detection of Hub Genes
The R package “WGCNA” (Langfelder and Horvath, 2008) was used to build co-expression network for the filtered gene expression matrix. Before building a co-expression network, we applied sample networks method introduced by Oldham et al for outlier detection. We designated sample as outlying, if the Z.K value was below −2.5. The soft threshold power β was selected based on the scale-free topology criterion introduced by Zhang and Horvath (2005). We calculated Pearson's correlations between each gene pair to determine concordance of gene expression to generate a matrix of adjacencies, and then the adjacencies were transformed into topological overlap matrix (TOM) (Li and Horvath, 2009). Next, we performed average linkage hierarchical clustering based on the TOM-based dissimilarity with a minimum module size of 30 and a medium sensitivity of 2, and other parameters were designated as default. After relating modules to clinical traits and calculating the associated Gene Significance (the correlation between the genes and the trait) and Module Membership (the correlation of the module eigengene and the gene expression profile), we detected top 50 hub genes using a networkScreening function based on Gene Significance and Module Membership and genes with q.Weighetd value less than 0.001 were finally regarded as hub genes (Dong and Horvath, 2007).
Functional Annotation of Hub Genes
WebGestalt (WEB-based GEne SeT AnaLysis Toolkit) (Zhang et al., 2005; Wang et al., 2013) was used to conduct gene ontology (GO) and Kyoto Encyclopedia of Genes and Genomes (KEGG) (http://www.kegg.jp/kegg/) pathway enrichment analysis of hub genes. Search Tool for the Retrieval of Interacting Genes (STRING) database (Szklarczyk et al., 2015) was use to construct a protein-protein interaction (PPI) network, and then the PPI network was visualized using Cytoscape (Shannon et al., 2003).
Survival Analysis
Another BC microarray study GSE19915 (Lindgren et al., 2010), which included 144 BC samples and 12 normal samples and the associated clinical characteristics including gender, age, biopsy Gleason score, survival status, follow-up, etc., was regarded as a validation cohort. PROGgenesV2 (Goswami and Nakshatri, 2014) was applied to conduct log-rank based survival analyses to compare the overall survivals of particular comparing groups defined based on the medians of the expression level of hub genes. Difference with statistical significance was defined as P < 0.05.
Gene Set Enrichment Analysis (GSEA)
Bladder cancer (BC) microarray datasets GSE31684 (Riester et al., 2012, 2014) was used to conduct GSEA (Subramanian et al., 2005) analysis of hub genes. BC samples were divided into a particular hub gene high expression group and low expression group based on the median expression of this hub gene. Differences at nominal P < 0.05 and FDR (false discovery rate) less than 25% were defined as statistical significance.
Results
Results of Data Preprocessing, Co-expression Network Construction and Hub Genes Identification
Gene expression profile of GSE13507 was obtained from GEO database, and probes with variances ranked in top 10,000 were used in the subsequent analyses. After outlier detection, one sample was excluded for further analysis (Supplementary Figure 1). As shown in Figure 1A, β = 14, the lowest power for which the scale-free topology fit index reaches 0.9, was selected for the subsequent adjacencies calculation. Based on the results of TOM based clustering, we obtained 11 gene modules as shown in Figure 1B. After the modules were related to the TNM stages of BC patients, top 50 hub genes identified based on the corresponding Gene Significance and Module Membership were summarized in Supplementary Table 1.
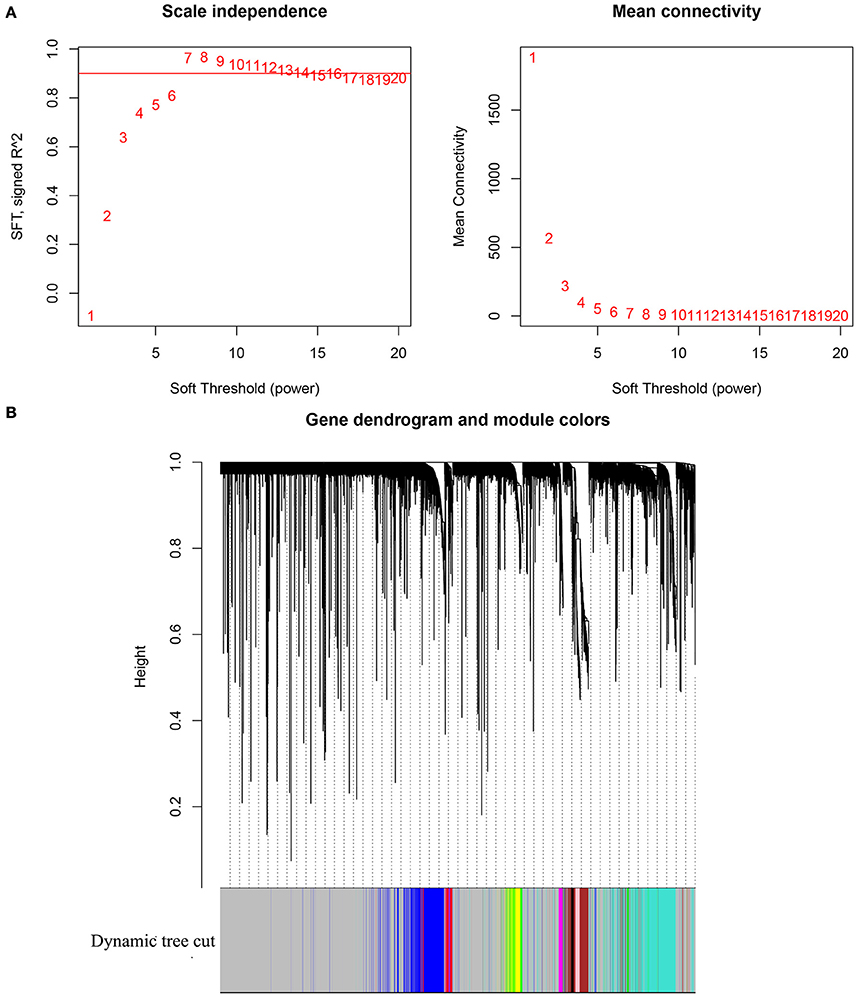
Figure 1. (A) Analysis of network topology for various soft-thresholding powers. The left panel shows the scale-free fit index (y-axis) as a function of the soft-thresholding power (x-axis). The right panel displays the mean connectivity (degree, y-axis) as a function of the soft-thresholding power (x-axis). (B) Clustering dendrogram of genes, with dissimilarity based on topological overlap, together with assigned module colors.
Gene Ontology Enrichment Analysis of Hub Genes
To get a primary understanding of the biological relevance of the hub genes, we first performed GO enrichment analysis of the hub genes. As shown in Table 1, top 10 enriched GO terms are listed. The hub genes mainly enriched in “mitotic sister chromatid segregation,” “mitotic cell cycle,” “nuclear division,” “sister chromatid segregation,” “cell cycle,” “chromosome segregation,” “mitotic nuclear division,” “cell cycle process,” “organelle fission,” and “cell division.”
KEGG Pathway Enrichment Analysis of Hub Genes
Moreover, as shown in Table 2, the KEGG pathway enrichment analysis of the hub genes indicated that these genes were mainly enriched in “Cell cycle,” “Oocyte meiosis,” “MicroRNAs in cancer,” “Protein digestion and absorption,” and “Progesterone-mediated oocyte maturation.”
PPI Network Analysis of Hub Genes
Hub genes were mapped to STRING database. As shown in Figure 2, a total number of 244 pairs of PPIs were obtained from STRING database, and 17 hub genes (“AURKB,” “TOP2A,” “PRC1,” “KIF4A,” “CENPF,” “NUSAP1,” “KIF2C,” “CEP55,” “CDCA8,” “CENPA,” “CCNB2,” “FOXM1,” “UBE2C,” “CDC45,” “KIF15,” “BUB1B,” and “CDCA3”), interacted with ≥5 of the hub genes, were at the hub of the PPI network.
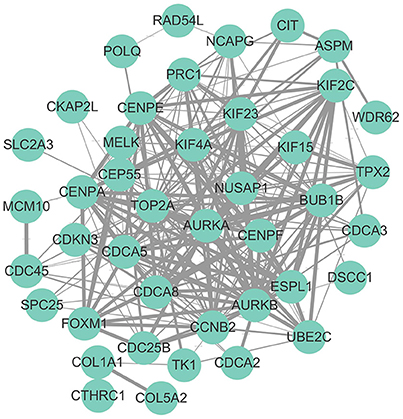
Figure 2. Protein-protein interaction network of hub genes. The edge width was proportional to the score of protein-protein interaction based on the STRING database.
Survival Analysis of Hub Genes
To perform a further validation of the hub genes and identify potential biomarkers for BC, survival analyses of the hub genes were conducted using PROGgenesV2. As shown in Table 3, lower expression of 11 hub genes (including MMP11, COL5A2, CDC25B, TOP2A, CENPF, CDCA3, TK1, TPX2, CDCA8, AEBP1, and FOXM1) were significantly associated with better overall survival of BC patients.
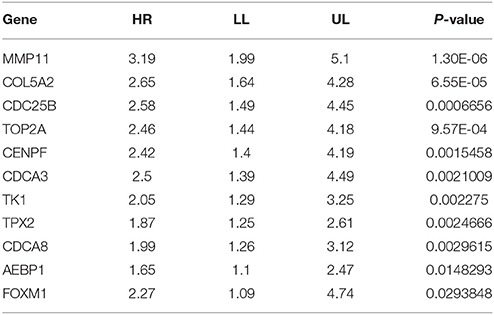
Table 3. Survival of hub genes, high expression of MMP11, COL5A2, CDC25B, TOP2A, CENPF, CDCA3, TK1, TPX2, CDCA8, AEBP1, and FOXM1 were correlated with better overall survival of patients with bladder cancer.
GSEA Analysis of Hub Genes that were Significantly Correlated with Overall Survival of BC Patients
To characterize the potential mechanisms involved in the influences on overall survival of the above 11 hub genes. GSEA was conducted based on the expression of MMP11, COL5A2, CDC25B, TOP2A, CENPF, CDCA3, TK1, TPX2, CDCA8, AEBP1, and FOXM1. As shown in Supplementary Table 2, BC samples in MMP11 high expression group were most significantly enriched in P53 pathway; BC samples in COL5A2 high expression group were most significantly enriched in apical junction (Supplementary Table 3); BC samples in CDC25B, CENPF, TPX2, CDCA8, and FOXM1 high expression groups were most significantly enriched in mitotic spindle (Supplementary Tables 4–8); BC samples in TOP2A, CDCA3 and TK1 high expression groups were most significantly enriched in G2M checkpoint (Supplementary Tables 9–11); BC samples with AEBP1 high expression were most significantly enriched in myogenesis (Supplementary Table 12).
Discussion
As mentioned above, BC is one of the most common cancers and significant progress has been made during the past decade. The prognosis of patients with BC, especially invasive BC, remains poor. The recurrence rate for superficial BC is about two-thirds, and, despite advances in management, some patients still develop stage progression. Meanwhile, approximately 30% of muscle-invasive BCs are associated with occult distant metastasis at the time of diagnosis, which led to a dismal 5-year survival of patient with muscle invasive BC (Kamat et al., 2016). Biomarker is defined as a substance found in tissue, blood, or other body fluids that might be a sign of cancer or noncancerous conditions (Mohammed et al., 2016). Previous studies suggested that biomarkers, including BC biomarkers, exhibited several features: prognostic, predictive, and pharmacodynamic. The biological functions of prognostic biomarker, predictive biomarker, and pharmacodynamic biomarker were predicting the natural course of cancers, evaluating the probable benefit of a particular treatment, and assessing the treatment effects of a drug on a tumor and determine the proper dosage of a new anticancer drug, respectively (Kojima et al., 2016; Mohammed et al., 2016; Nandagopal and Sonpavde, 2016). Nowadays, many biomarkers and their corresponding targeted agents have been determined for the diagnosis and treatment of BC. In the present study, we identified 50 hub genes that were significantly correlated with the TNM staging of BC patients using WGCNA.
As we know, TNM staging, devised by Pierre Denoix, describes the size of the original (primary) tumor and whether it has invaded nearby tissue (T), describes nearby (regional) lymph nodes that are involved (N), and describes distant metastasis (M) (Denoix, 1946). The functional enrichment analysis of hub genes that were correlated with the TNM stages indicated that these genes were enriched in cell proliferation associated GO terms (mitotic sister chromatid segregation, mitotic cell cycle and cell cycle, etc.) and KEGG pathways (cell cycle, Oocyte meiosis, etc.). Thus, we speculated that these hub genes affected the TNM staging of BC patients through promoting the proliferation of BC cells.
The PPI network analysis of the hub genes suggested that a total number of 17 hub genes interacted with ≥5 of the hub genes were at the hub of the PPI network. Previous studies demonstrated that functional partnerships and interactions that occurred between proteins were at the core of cellular processing and their systematic characterization helped to provide context in molecular systems biology. Thus, the 17 hub genes, as mentioned above, might play an important role in the biological process of BC cells.
Our survival analyses of the hub genes indicated that lower expressions of 11 hub genes were correlated with relatively shorter overall survivals of patients with BC. Meanwhile, our GSEA results indicated that BC samples with higher expression of these genes were enriched in genes sets that were correlated with biological behaviors of tumor cells (such as P53 pathway, Apical junction, mitotic spindle, G2M checkpoint, Myogenesis, etc.).
As for the 11 survival associated hub genes, we conducted a literature review of these genes. MMP11 is a member of the matrix metalloproteinase (MMP) family involved in the breakdown of extracellular matrix in normal physiological processes, such as embryonic development, reproduction, and tissue remodeling, as well as in disease processes, and several studies demonstrated that overexpression of MMP11 were correlated with many cancers (including colon cancer, laryngeal cancer, breast cancer, prostate cancer, etc.) (Deng et al., 2005; Kou et al., 2013; Roscilli et al., 2014; Li et al., 2015; Pang et al., 2016); COL5A2 encodes an alpha chain for one of the low abundance fibrillar collagens, and previous studies suggested that COL5A2 was associated with malignancy in colorectal cancer, breast cancer, osteosarcoma, etc. (Fischer et al., 2001; Vargas et al., 2012; Wu et al., 2014); CDC25B is a member of the CDC25 family of phosphatases, and previous studies suggested that elevated expression of CDC25B promoted the proliferation of gastric cancer, esophageal carcinoma, renal cell carcinoma, etc. (Yu et al., 2012; Wang et al., 2015; Leal et al., 2016); TOP2A encodes a DNA topoisomerase, and previous studies suggested that TOP2A overexpression was a poor prognostic factor in patients with breast cancer, colorectal cancer, prostate cancer, and nasopharyngeal carcinoma, etc., (de Resende et al., 2013; Lan et al., 2014; Tarpgaard et al., 2016; Zheng et al., 2016); CENPF is a component of the nuclear matrix during the G2 phase of interphase, and previous studies demonstrated that CENPF was associated with the tumor progression of patients with prostate cancer, hepatocellular carcinomas, and breast cancer, etc. (Brendle et al., 2009; Kim et al., 2012); Cell division cycle associated 3 (CDCA3) is a part of the Skp1-cullin-F-box (SCF) ubiquitin ligase and refers to a trigger of mitotic entry and mediates destruction of the mitosis inhibitory kinase, and previous studies demonstrated overexpression of CDCA3 promoted progression of several cancers (oral cancer, prostate cancer, and lung cancer, etc.) (Uchida et al., 2012; Chen et al., 2013; O'Byrne et al., 2016); TK1(thymidine kinase 1) encodes a cytosolic enzyme that catalyzes the addition of a gamma-phosphate group to thymidine, and previous studies proved that high TK1 expression is a predictor of poor survival in patients with pT1 of lung adenocarcinoma, BC, gastrointestinal cancer, etc. (Jagarlamudi et al., 2015; Du et al., 2016); Targeting protein for Xenopus kinesin-like protein 2 (TPX2) is microtubule-associated protein and impacts spindle assembly in human cells (Huang et al., 2014), and previous studies proved that TPX2 expression was associated with poor survival in gastric cancer, breast cancer, colon cancer and esophageal cancer, etc. (Wei et al., 2013; Liu et al., 2014; Yang et al., 2015; Tomii et al., 2017); The cell division cycle associated 8 (CDCA8) gene encodes a component of the chromosomal passenger complex and plays an important role in mitosis, and overexpression of CDCA8 was reported in some human cancers, demonstrating that CDCA8 was required for the growth and progression of several cancers such as breast cancer, gastric cancer, lung carcinogenesis (Hayama et al., 2007; Yan et al., 2012; Jiao et al., 2015); Several studies demonstrated the AEBP1 upregulation conferred acquired resistance to BRAF (V600E) inhibition in melanoma and was associated with bad survivals of patients with glioma (Ladha et al., 2012; Hu et al., 2013); The protein encoded by FOXM1 is phosphorylated in M phase and regulates the expression of several cell cycle genes, such as cyclin B1 and cyclin D1, and previous studies suggested that FOXM1 promoted tumor progression of multiple cancers including gastric cancer, ovarian cancer, cervical cancer, colorectal cancer and breast cancer, etc. Trichostatin A potentiates TRAIL-induced antitumor effects via inhibition of ERK/FOXM1 pathway in gastric cancer (Barger et al., 2015; Yau et al., 2015; Zheng et al., 2015; Li et al., 2016; Wang et al., 2016; Song et al., 2017). In summary, all the conclusions were consistent with the results of survival analysis and GSEA analysis that high expressions of MMP11, COL5A2, CDC25B, TOP2A, CENPF, CDCA3, TK1, TPX2, CDCA8, AEBP1, and FOXM1 were correlated with worse overall survival of BC patients and BC samples with relatively higher expression of these genes were enriched in gene sets that were associated with cell proliferation.
In conclusion, the identified 50 hub genes that were closely correlated with the TNM staging of BC patients, and 11 hub genes (MMP11, COL5A2, CDC25B, TOP2A, CENPF, CDCA3, TK1, TPX2, CDCA8, AEBP1, and FOXM1) of which were significantly correlated with the overall survival of BC, which could be candidate biomarkers for BC. Meanwhile, further in vivo and in vitro studies were needed to make clear the exact molecular mechanisms that affected the growth of BC cells.
Author Contributions
XW designed the study. SL and XL collected, analyzed and interpreted the data. XL, TL, XM, XY, CF, DH, and HW participated in revising the manuscript. XZ and YC participated in the study design and helped to draft the manuscript. All authors read and approved the final manuscript.
Funding
This work was supported by the National Key Research and Development Plan of China (Grant No. 2016YFC0106300) and the National Natural Science Foundation of China (Grant No. 81772730) and Wuhan Clinical Research Center for Male Urogenital Cancer (Grant No. 303-230100055) and the 351 Talent Project of Wuhan University (Luojia Young Scholars: SL).
Conflict of Interest Statement
The authors declare that the research was conducted in the absence of any commercial or financial relationships that could be construed as a potential conflict of interest.
Supplementary Material
The Supplementary Material for this article can be found online at: https://www.frontiersin.org/articles/10.3389/fphys.2017.00947/full#supplementary-material
Supplementary Figure 1. Cluster tree of bladder cancer samples. The leaves of the tree correspond to the bladder cancer sample. The first color band underneath the tree indicates which arrays appear to be outlying. The sixth color band represents TNM staging (red indicates high values). Similarly, the remaining color-bands color-code the numeric values of physiologic traits.
References
Barger, C. J., Zhang, W., Hillman, J., Stablewski, A. B., Higgins, M. J., Vanderhyden, B. C., et al. (2015). Genetic determinants of FOXM1 overexpression in epithelial ovarian cancer and functional contribution to cell cycle progression. Oncotarget 6, 27613–27627. doi: 10.18632/oncotarget.4546
Brendle, A., Brandt, A., Johansson, R., Enquist, K., Hallmans, G., Hemminki, K., et al. (2009). Single nucleotide polymorphisms in chromosomal instability genes and risk and clinical outcome of breast cancer: a Swedish prospective case-control study. Eur. J. Cancer 45, 435–442. doi: 10.1016/j.ejca.2008.10.001
Chen, J., Zhu, S., Jiang, N., Shang, Z., Quan, C., and Niu, Y. (2013). HoxB3 promotes prostate cancer cell progression by transactivating CDCA3. Cancer Lett. 330, 217–224. doi: 10.1016/j.canlet.2012.11.051
Deng, H., Guo, R. F., Li, W. M., Zhao, M., and Lu, Y. Y. (2005). Matrix metalloproteinase11 depletion inhibits cell proliferation in gastric cancer cells. Biochem. Biophys. Res. Commun. 326, 274–281. doi: 10.1016/j.bbrc.2004.11.027
Denoix, P. F. (1946). Enquete permanent dans les centres anticancereaux. Bull. Inst. Nat. Hyg. 1, 70–75.
de Resende, M. F., Vieira, S., Chinen, L. T., Chiappelli, F., da Fonseca, F. P., Guimarães, G. C., et al. (2013). Prognostication of prostate cancer based on TOP2A protein and gene assessment: TOP2A in prostate cancer. J. Transl. Med. 11:36. doi: 10.1186/1479-5876-11-36
Dong, J., and Horvath, S. (2007). Understanding network concepts in modules. BMC Syst. Biol. 1:24. doi: 10.1186/1752-0509-1-24
Du, Y. Y., Zhang, Q. J., and Sun, G. P. (2016). Expression and Clinical Significance of Cytokeratin-19 and Thymidine Kinase-1 in Advanced Gastrointestinal Cancer. Chin. Med. J. 129, 2168–2172. doi: 10.4103/0366-6999.189919
Fischer, H., Stenling, R., Rubio, C., and Lindblom, A. (2001). Colorectal carcinogenesis is associated with stromal expression of COL11A1 and COL5A2. Carcinogenesis. 22, 875–878.
Ghervan, L., Zaharie, A., Ene, B., and Elec, F. I. (2017). Small-cell carcinoma of the urinary bladder: where do we stand? Clujul Med. 90, 13–17. doi: 10.15386/cjmed-673
Giunchi, F., Ciccarese, C., Montironi, R., Scarpelli, M., Lopez-Beltran, A., Cheng, L., et al. (2017). Urinary biomarkers for prostate cancer. Curr. Drug Metab. 18, 723–726. doi: 10.2174/1389200218666170518161140
Goswami, C. P., and Nakshatri, H. (2014). PROGgeneV2: enhancements on the existing database. BMC Cancer 14:970. doi: 10.1186/1471-2407-14-970
Hayama, S., Daigo, Y., Yamabuki, T., Hirata, D., Kato, T., Miyamoto, M., et al. (2007). Phosphorylation and activation of cell division cycle associated 8 by aurora kinase B plays a significant role in human lung carcinogenesis. Cancer Res. 67, 4113–4122. doi: 10.1158/0008-5472.can-06-4705
Hu, W., Jin, L., Jiang, C. C., Long, G. V., Scolyer, R. A., Wu, Q., et al. (2013). AEBP1 upregulation confers acquired resistance to BRAF (V600E) inhibition in melanoma. Cell Death Dis. 4:e914. doi: 10.1038/cddis.2013.441
Huang, Y., Guo, W., and Kan, H. (2014). TPX2 is a prognostic marker and contributes to growth and metastasis of human hepatocellular carcinoma. Int. J. Mol. Sci. 15, 18148–18161. doi: 10.3390/ijms151018148
Jagarlamudi, K. K., Hansson, L. O., and Eriksson, S. (2015). Breast and prostate cancer patients differ significantly in their serum Thymidine kinase 1 (TK1) specific activities compared with those hematological malignancies and blood donors: implications of using serum TK1 as a biomarker. BMC Cancer. 15:66. doi: 10.1186/s12885-015-1073-8
Jiao, D. C., Lu, Z. D., Qiao, J. H., Yan, M., Cui, S. D., and Liu, Z. Z. (2015). Expression of CDCA8 correlates closely with FOXM1 in breast cancer: public microarray data analysis and immunohistochemical study. Neoplasma 62, 464–469. doi: 10.4149/neo_2015_055
Kamat, A. M., Hahn, N. M., Efstathiou, J. A., Lerner, S. P., Malmström, P. U., Choi, W., et al. (2016). Bladder cancer. Lancet 388, 2796–2810. doi: 10.1016/S0140-6736(16)30512-8
Kim, H. E., Kim, D. G., Lee, K. J., Son, J. G., Song, M. Y., Park, Y. M., et al. (2012). Frequent amplification of CENPF, GMNN and CDK13 genes in hepatocellular carcinomas. PLoS ONE 7:e43223. doi: 10.1371/journal.pone.0043223
Kim, W. J., Kim, E. J., Kim, S. K., Kim, Y. J., Ha, Y. S., Jeong, P., et al. (2010). Predictive value of progression-related gene classifier in primary non-muscle invasive bladder cancer. Mol. Cancer. 9:3. doi: 10.1186/1476-4598-9-3
Kojima, T., Kawai, K., Miyazaki, J., and Nishiyama, H. (2016). Biomarkers for precision medicine in bladder cancer. Int. J. Clin. Oncol. 22, 207–213. doi: 10.1007/s10147-016-1068-8
Kou, Y. B., Zhang, S. Y., Zhao, B. L., Ding, R., Liu, H., and Li, S. (2013). Knockdown of MMP11 inhibits proliferation and invasion of gastric cancer cells. Int. J. Immunopathol. Pharmacol. 26, 361–370. doi: 10.1177/039463201302600209
Ladha, J., Sinha, S., Bhat, V., Donakonda, S., and Rao, S. M. (2012). Identification of genomic targets of transcription factor AEBP1 and its role in survival of glioma cells. Mol Cancer Res. 10, 1039–1051. doi: 10.1158/1541-7786.MCR-11-0488
Lan, J., Huang, H. Y., Lee, S. W., Chen, T. J., Tai, H. C., Hsu, H. P., et al. (2014). TOP2A overexpression as a poor prognostic factor in patients with nasopharyngeal carcinoma. Tumour Biol. 35, 179–187. doi: 10.1007/s13277-013-1022-6
Langfelder, P., and Horvath, S. (2008). WGCNA: an R package for weighted correlation network analysis. BMC Bioinformatics 9:559. doi: 10.1186/1471-2105-9-559
Leal, M. F., Ribeiro, H. F., Rey, J. A., Pinto, G. R., Smith, M. C., Moreira-Nunes, C. A., et al. (2016). YWHAE silencing induces cell proliferation, invasion and migration through the up-regulation of CDC25B and MYC in gastric cancer cells: new insights about YWHAE role in the tumor development and metastasis process. Oncotarget 7, 85393–85410. doi: 10.18632/oncotarget.13381
Lee, J. S., Leem, S. H., Lee, S. Y., Kim, S. C., Park, E. S., Kim, S. B., et al. (2010). Expression signature of E2F1 and its associated genes predict superficial to invasive progression of bladder tumors. J. Clin. Oncol. 28, 2660–2667. doi: 10.120010.1186/1471-2105-9-559/JCO.2009.25.0977
Li, A., and Horvath, S. (2009). Network module detection: affinity search technique with the multi-node topological overlap measure. BMC Res. Notes 2:142. doi: 10.1186/1756-0500-2-142
Li, L., Fan, B., Zhang, L. H., Xing, X. F., Cheng, X. J., Wang, X. H., et al. (2016). Trichostatin A potentiates TRAIL-induced antitumor effects via inhibition of ERK/FOXM1 pathway in gastric cancer. Tumour Biol. 37, 10269–10278. doi: 10.1007/s13277-016-4816-5
Lindgren, D., Frigyesi, A., Gudjonsson, S., Sjödahl, G., Hallden, C., Chebil, G., et al. (2010). Combined gene expression and genomic profiling define two intrinsic molecular subtypes of urothelial carcinoma and gene signatures for molecular grading and outcome. Cancer Res. 70, 3463–3472. doi: 10.1158/0008-5472.CAN-09-4213
Liu, H. C., Zhang, G. H., Liu, Y. H., Wang, P., Ma, J. F., Su, L. S., et al. (2014). TPX2 siRNA regulates growth and invasion of esophageal cancer cells. Biomed. Pharmacother. 68, 833–839. doi: 10.1016/j.biopha.2014.08.008
Li, Z., Ding, S., Zhong, Q., Li, G., Zhang, Y., and Huang, X. C. (2015). Significance of MMP11 and P14(ARF) expressions in clinical outcomes of patients with laryngealcancer. Int. J. Clin. Exp. Med. 8, 15581–15590.
Malmström, P. U., Agrawal, S., Bläckberg, M., Boström, P. J., Malavaud, B., Zaak, D., et al. (2017). Non-muscle-invasive bladder cancer: a vision for the future. Scand. J. Urol. 51, 87–94. doi: 10.1080/21681805.2017.1283359
Mohammed, A. A., El-Tanni, H., El-Khatib, H. M., Mirza, A. A., Mirza, A. A., and Alturaifi, T. H. (2016). Urinary bladder cancer: biomarkers and target therapy, new era for more attention. Oncol. Rev. 10:320. doi: 10.4081/oncol.2016.320
Nandagopal, L., and Sonpavde, G. (2016). Circulating biomarkers in bladder cancer. Bladder Cancer. 2, 369–379. doi: 10.3233/BLC-160075
O'Byrne, K., Adams, M., Burgess, J., and Richard, D. (2016). 24P CDCA3 regulates the cell cycle and modulates cisplatin sensitivity in non-small cell lung cancer. J. Thorac. Oncol. 11:S65. doi: 10.1016/S1556-0864(16)30138-1
Ozerov, I. V., Lezhnina, K. V., Izumchenko, E., Artemov, A. V., Medintsev, S., Vanhaelen, Q., et al. (2016). In silico pathway activation network decomposition analysis (iPANDA) as a method for biomarker development. Nat. Commun. 7:13427. doi: 10.1038/ncomms13427
PDQ Adult Treatment Editorial Board (2002). Bladder Cancer Treatment (PDQ®): Health Professional Version. PDQ Cancer Information Summaries [Internet]. Bethesda, MD: National Cancer Institute, 2002–2017.
Pang, L., Wang, D. W., Zhang, N., Xu, D. H., and Meng, X. W. (2016). Elevated serum levels of MMP-11 correlate with poor prognosis in colon cancer patients. Cancer Biomark. 16, 599–607. doi: 10.3233/cbm-160601
Riester, M., Taylor, J. M., Feifer, A., Koppie, T., Rosenberg, J. E., Downey, R. J., et al. (2012). Combination of a novel gene expression signature with a clinical nomogram improves the prediction of survival in high-risk bladder cancer. Clin. Cancer Res. 18, 1323–1333. doi: 10.1158/1078-0432.CCR-11-2271
Riester, M., Werner, L., Bellmunt, J., Selvarajah, S., Guancial, E. A., Weir, B. A., et al. (2014). Integrative analysis of 1q23.3 copy-number gain in metastatic urothelial carcinoma. Clin. Cancer Res. 20, 1873–1883. doi: 10.1158/1078-0432.CCR-13-0759
Roscilli, G., Cappelletti, M., De Vitis, C., Ciliberto, G., Di Napoli, A., Ruco, L., et al. (2014). Circulating MMP11 and specific antibody immune response in breast and prostate cancer patients. J. Transl. Med. 12:54. doi: 10.1186/1479-5876-12-54
Salama, A., Abdelmaksoud, A. M., Shawki, A., Abdelbary, A., and Aboulkassem, H. (2016). Outcome of muscle-invasive urothelial bladder cancer after radical cystectomy. Clin. Genitourin. Cancer 14, e43–e47. doi: 10.1016/j.clgc.2015.07.007
Sargos, P., Baumann, B. C., Eapen, L. J., Bahl, A., Murthy, V., Roubaud, G., et al. (2016). Adjuvant radiotherapy for pathological high-risk muscle invasive bladder cancer: time to reconsider? Transl. Androl. Urol. 5, 702–710. doi: 10.21037/tau.2016.08.18
Shannon, P., Markiel, A., Ozier, O., Baliga, N. S., Wang, J. T., Ramage, D., et al. (2003). Cytoscape: a software environment for integrated models of biomolecular interaction networks. Genome Res. 13, 2498–2504. doi: 10.1101/gr.1239303
So, A. (2016). Bladder cancer, ESMO 2016. Can. Urol. Assoc. J. 10(11–12 Suppl. 6), S224–S226. doi: 10.5489/cuaj.4281
Song, X., Fiati Kenston, S. S., Zhao, J., Yang, D., and Gu, Y. (2017). Roles of FoxM1 in cell regulation and breast cancer targeting therapy. Med Oncol. 34:41. doi: 10.1007/s12032-017-0888-3
Subramanian, A., Tamayo, P., Mootha, V. K., Mukherjee, S., Ebert, B. L., Gillette, M. A., et al. (2005). Gene set enrichment analysis: a knowledge-based approach for interpreting genome-wide expression profiles. Proc. Natl. Acad. Sci. U.S.A. 102, 15545–15550. doi: 10.1073/pnas.0506580102
Szklarczyk, D., Franceschini, A., Wyder, S., Forslund, K., Heller, D., Huerta-Cepas, J., et al. (2015). STRING v10: protein-protein interaction networks, integrated over the tree of life. Nucleic Acids Res. 43, D447–D452. doi: 10.1093/nar/gku1003
Tarpgaard, L. S., Qvortrup, C., Nygård, S. B., Nielsen, S. L., Andersen, D. R., Jensen, N. F., et al. (2016). A phase II study of Epirubicin in oxaliplatin-resistant patients with metastatic colorectal cancer and TOP2A gene amplification. BMC Cancer. 16:91. doi: 10.1186/s12885-016-2124-5
Tomii, C., Inokuchi, M., Takagi, Y., Ishikawa, T., Otsuki, S., Uetake, H., et al. (2017). TPX2 expression is associated with poor survival in gastric cancer. World J. Surg. Oncol. 15, 14. doi: 10.1186/s12957-016-1095-y
Uchida, F., Uzawa, K., Kasamatsu, A., Takatori, H., Sakamoto, Y., Ogawara, K., et al. (2012). Overexpression of cell cycle regulator CDCA3 promotes oral cancer progression by enhancing cell proliferation with prevention of G1 phase arrest. BMC Cancer 12:321. doi: 10.1186/1471-2407-12-321
Vargas, A. C., McCart Reed, A. E., Waddell, N., Lane, A., Reid, L. E., Smart, C. E., et al. (2012). Gene expression profiling of tumour epithelial and stromal compartments during breast cancer progression. Breast Cancer Res. Treat. 135, 153–65. doi: 10.1007/s10549-012-2123-4
Wang, J., Duncan, D., Shi, Z., and Zhang, B. (2013). WEB-based GEne SeT AnaLysis Toolkit (WebGestalt): update. Nucleic Acids Res. 41, W77–W83. doi: 10.1093/nar/gkt439
Wang, M., Zhu, X. Y., Wang, L., and Lin, Y. (2015). Expression and significance of CDC25B, PED/PEA-15 in esophageal carcinoma. Cancer Biother. Radiopharm. 30, 139–145. doi: 10.1089/cbr.2014.1701
Wang, T., Liu, Z., Shi, F., and Wang, J. (2016). Pin1 modulates chemo-resistance by up-regulating FoxM1 and the involvements of Wnt/β-catenin signaling pathway in cervical cancer. Mol. Cell Biochem. 413, 179–187. doi: 10.1007/s11010-015-2651-4
Wei, P., Zhang, N., Xu, Y., Li, X., Shi, D., Wang, Y., et al. (2013). TPX2 is a novel prognostic marker for the growth and metastasis of colon cancer. J. Transl. Med. 11:313. doi: 10.1186/1479-5876-11-313
Wu, D., Chen, K., Bai, Y., Zhu, X., Chen, Z., Wang, C., et al. (2014). Screening of diagnostic markers for osteosarcoma. Mol. Med. Rep. 10, 2415–20. doi: 10.3892/mmr.2014.2546
Yan, G. R., Zou, F. Y., Dang, B. L., Zhang, Y., Yu, G., Liu, X., et al. (2012). Genistein-induced mitotic arrest of gastric cancer cells by downregulating KIF20A, a proteomics study. Proteomics 12, 2391–2399. doi: 10.1002/pmic.201100652
Yang, Y., Li, D. P., Shen, N., Yu, X. C., Li, J. B., Song, Q., et al. (2015). TPX2 promotes migration and invasion of human breast cancer cells. Asian Pac. J. Trop. Med. 8, 1064–1070. doi: 10.1016/j.apjtm.2015.11.007
Yau, C., Meyer, L., Benz, S., Vaske, C., Scott, G., Egan, B., et al. (2015). FOXM1 cistrome predicts breast cancer metastatic outcome better than FOXM1 expression levels or tumor proliferation index. Breast Cancer Res Treat. 154, 23–32. doi: 10.1007/s10549-015-3589-7
Yaxley, J. P. (2016). Urinary tract cancers: an overview for general practice. J. Family Med. Prim. Care. 5, 533–538. doi: 10.4103/2249-4863.197258
Yip, A. M., and Horvath, S. (2007). Gene network interconnectedness and the generalized topological overlap measure. BMC Bioinformatics 8:22. doi: 10.1186/1471-2105-8-22
Yu, X. Y., Zhang, Z., Zhang, G. J., Guo, K. F., and Kong, C. Z. (2012). Knockdown of Cdc25B in renal cell carcinoma is associated with decreased malignant features. Asian Pac. J. Cancer Prev. 13, 931–935. doi: 10.7314/APJCP.2012.13.3.931
Zhang, B., and Horvath, S. (2005). A general framework for weighted gene co-expression network analysis. Stat. Appl. Genet. Mol. Biol. 4:17. doi: 10.2202/1544-6115.1128
Zhang, B., Kirov, S., and Snoddy, J. (2005). WebGestalt: an integrated system for exploring gene sets in various biological contexts. Nucleic Acids Res. 33, W741–W748. doi: 10.1093/nar/gki475
Zheng, H., Li, X., Chen, C., Chen, J., Sun, J., Sun, S., et al. (2016). Quantum dot-based immunofluorescent imaging and quantitative detection of TOP2A and prognostic value in triple-negative breast cancer. Int. J. Nanomed. 11, 5519–5529. doi: 10.2147/ijn.s111594
Keywords: bladder cancer, biomarkers, WGCNA
Citation: Li S, Liu X, Liu T, Meng X, Yin X, Fang C, Huang D, Cao Y, Weng H, Zeng X and Wang X (2017) Identification of Biomarkers Correlated with the TNM Staging and Overall Survival of Patients with Bladder Cancer. Front. Physiol. 8:947. doi: 10.3389/fphys.2017.00947
Received: 02 May 2017; Accepted: 08 November 2017;
Published: 28 November 2017.
Edited by:
Oreste Gualillo, Servicio Gallego de Salud, SpainReviewed by:
Alex Zhavoronkov, Biogerontology Research Foundation, United KingdomMonica Catarina Botelho, Istituto Nacional de Saúde, Portugal
Copyright © 2017 Li, Liu, Liu, Meng, Yin, Fang, Huang, Cao, Weng, Zeng and Wang. This is an open-access article distributed under the terms of the Creative Commons Attribution License (CC BY). The use, distribution or reproduction in other forums is permitted, provided the original author(s) or licensor are credited and that the original publication in this journal is cited, in accordance with accepted academic practice. No use, distribution or reproduction is permitted which does not comply with these terms.
*Correspondence: Xinghuan Wang, d2FuZ3hpbmdodWFuQHdodS5lZHUuY24=
Xiantao Zeng, emVuZ3hpYW50YW8xMTI4QDE2My5jb20=
†These authors have contributed equally to this work.