- 1Department of Surgery, University of Pittsburgh, Pittsburgh, PA, United States
- 2Center for Inflammation and Regenerative Modeling, McGowan Institute for Regenerative Medicine, University of Pittsburgh, Pittsburgh, PA, United States
- 3Pittsburgh Liver Research Center, University of Pittsburgh, Pittsburgh, PA, United States
We hypothesized that isolated primary mouse hepatic stellate cells (HSC) and hepatocytes (HC) would elaborate different inflammatory responses to hypoxia with or without reoxygenation. We further hypothesized that intracellular information processing (“thinking”) differs from extracellular information transfer (“talking”) in each of these two liver cell types. Finally, we hypothesized that the complexity of these autocrine responses might only be defined in the absence of other non-parenchymal cells or trafficking leukocytes. Accordingly, we assayed 19 inflammatory mediators in the cell culture media (CCM) and whole cell lysates (WCLs) of HSC and HC during hypoxia with and without reoxygenation. We applied a unique set of statistical and data-driven modeling techniques including Two-Way ANOVA, hierarchical clustering, Principal Component Analysis (PCA) and Network Analysis to define the inflammatory responses of these isolated cells to stress. HSC, under hypoxic and reoxygenation stresses, both expressed and secreted larger quantities of nearly all inflammatory mediators as compared to HC. These differential responses allowed for segregation of HSC from HC by hierarchical clustering. PCA suggested, and network analysis supported, the hypothesis that above a certain threshold of cellular stress, the inflammatory response becomes focused on a limited number of functions in both HSC and HC, but with distinct characteristics in each cell type. Network analysis of separate extracellular and intracellular inflammatory responses, as well as analysis of the combined data, also suggested the presence of more complex inflammatory “talking” (but not “thinking”) networks in HSC than in HC. This combined network analysis also suggested an interplay between intracellular and extracellular mediators in HSC under more conditions than that observed in HC, though both cell types exhibited a qualitatively similar phenotype under hypoxia/reoxygenation. Our results thus suggest that a stepwise series of computational and statistical analyses may help decipher how cells respond to environmental stresses, both within the cell and in its secretory products, even in the absence of cooperation from other cells in the liver.
Introduction
In settings of stress, such as liver ischemia/reperfusion, hemorrhagic shock after trauma, and drug-induced liver injury, endogenous mediators released from liver cells are known to initiate and regulate sterile liver inflammation (Peitzman et al., 1995; Vodovotz et al., 2004; Sun et al., 2017; Woolbright and Jaeschke, 2017). There are two major types of cells in the liver: parenchymal cells [hepatocytes (HC)] and non-parenchymal cells [sinusoidal endothelial cells, phagocytic Kupffer cells, and hepatic stellate cells (HSC)]. Whereas 70–85% of the normal adult murine liver volume is occupied by HC (Kmieć, 2001), Kupffer cells—which comprise approximately 35% of the non-parenchymal liver cells in normal adult mice (Bilzer et al., 2006)—play a central role in responses to early reperfusion injury following hypoxia/reoxygenation due to their macrophage-like properties (Bilzer and Gerbes, 2000). Studies over the past several decades have focused predominantly on the stress responses of HC, Kupffer cells (a heterogeneous cell population) and trafficking innate immune cells (de Groot, 1992; Peitzman et al., 1995; West and Wilson, 1996). Lesser attention has been paid to sinusoidal or vascular endothelial cells and HSC and the inherent autocrine complexity of the cellular components of the normal liver under stressful conditions have rarely been the subject of investigation.
Various studies have focused on the responses of HC cell lines to hypoxia/reoxygenation. For example, early studies showed that human HepG2 hepatoma cells express interferon-γ (IFNγ), Tumor Necrosis Factor-α (TNF-α), Transforming Growth Factor-β1 (TGFβ1), Macrophage Colony-Stimulating Factor (M-CSF), Oncostatin-M (OSM), Intercellular Adhesion Molecule (ICAM-1), Interleukin 4 (IL-4), IL-5, IL-7, IL-10, IL-11, IL-12, and IL-6 receptor (IL-6R), while the expression of IL-1β, IL-2, IL-3, IL-6, CD40 ligand and IL-2R genes was not detected (Stonans et al., 1999). Normal HC seem to have a much narrower spectrum of response: for example, primary human HC were shown to release IFNγ, IL-12p40, IL-12p70, IL-17A, and IL-10 following exposure to hepatotoxic drugs (Ogese et al., 2017). We recently used Luminex™ technology coupled with computational analyses to study the in vitro response of mouse HC to hypoxia (Ziraldo et al., 2013). We found that many inflammatory mediators were changed significantly in both normoxic and hypoxic cultures, and MCP-1 was identified as central node in the inflammatory networks of HC and as an inducer of IL-6; segregating trauma patients based on their co-expression of MCP-1 and IL-6 allowed us to suggest MCP-1 as a potential biomarker for clinical outcomes in trauma/hemorrhagic shock (Ziraldo et al., 2013).
HSC represent only 5-8% of the total number of liver cells (Geerts, 2001). The inflammatory responses of this cell type to hypoxia/reoxygenation are less well studied, though HSC are considered important in the pathogenesis of liver fibrosis (de Oliveira da Silva et al., 2017); furthermore, protection of the liver cells from ischemia/reperfusion injury in HSC-depleted mice indicates that HSC are major contributors to liver damage (Stewart et al., 2014). DNA microarray analyses have shown that hypoxia regulates the expression of genes that may alter the sensitivity of HSC to chemotaxins and other stimuli, and regulates the expression of genes important for angiogenesis and collagen synthesis (Copple et al., 2011). Furthermore, in primary HSC, bacterial lipopolysaccharide (LPS) strongly up-regulated numerous CC and CXC chemokines as well as IL-17F (Harvey et al., 2013). Other studies showed that HSC can express a number of other cytokines and chemokines such as Eotaxin, IFNγ, IL-6, IL-8, and IL-10 (Berardis et al., 2014).
Most studies of the effects of environmental stress are carried out in vivo, in which the interaction of various cell types within an organ cannot be discerned from one another. To our knowledge, no studies have utilized pure hepatic cell cultures to examine the autocrine effects, which themselves reflect complexity of response even in the absence of interaction with neighboring cell types. In addition, most studies explore the repertoire of mediator secretion (“talking”) by stressed cells, without questioning the internal reactions that take place within the cell (“thinking”). The interpretation of such complex responses require a baseline of information about the inherent responsiveness of purified cells. In addition, mere description of the secretory output of a cell under stress does not adequately address the complexity of even the simplest of experimental systems. For this reason, and given the close proximity of HSC and HC (one HSC contacting two HC on either side of the central vein), this study was designed to compare the intracellular and secretory (autocrine) responses of pure HC and HSC in culture to hypoxic stress and reoxygenation using a unique stepwise series of statistical and data driven modeling techniques previously used to define dynamic molecular networks in both experimental and clinical trauma and acute systemic inflammation (Mi et al., 2011; Ziraldo et al., 2011, 2013; Zaaqoq et al., 2014; Almahmoud et al., 2015; Abboud et al., 2016; Namas et al., 2016). These approaches, including Two-Way ANOVA, hierarchical clustering, Principal Component Analysis (PCA) and Network Analysis were chosen to unmask the complexity of even simple cellular systems. Our results suggest that intracellular inflammatory networks and principal characteristics (“thinking”) differ in several important respects from those in the conditioned medium (“talking”) of both HSC and HC. Our results further suggest that a stepwise series of computational and statistical analyses may help decipher how cells respond to inflammatory stresses.
Materials and Methods
Materials
Williams Medium E, penicillin, streptomycin, L-glutamine, and HEPES were purchased from Invitrogen (Carlsbad, CA). Insulin (Humulin®) was purchased from Eli Lilly (Indianapolis, IN), and calf serum was obtained from HyClone Laboratories (Logan, UT). Tissue culture dishes were from Corning Glass Works (Corning, NY).
Liver Cell Isolation and Culture
This study was carried out in accordance with the recommendations of the National Institutes of Health. The protocol was approved by the Institutional Animal Care and Use Committee of the University of Pittsburgh. The liver cell isolation and culture procedures were as follows:
-Primary Hepatocytes (HC) were harvested from adult C57BL/6 male mice (Charles River Laboratories, Wilmington, MA). Cells were isolated by collagenase perfusion using the method of Seglen (1976) and purified to >98% purity by repeated centrifugation at 50 g, followed by further purification over 30% Percoll. Viability at time of plating was checked by trypan blue exclusion. Highly purified HC (>98% purity and >95% viability by trypan blue exclusion) were suspended in Williams' E medium supplemented with 10% heat-inactivated calf serum, 15 mM HEPES (pH 7.4), 16 units insulin, 2 mM L-glutamine, 100 units/ml penicillin, and 100 μg/ml streptomycin. The cells were then plated on collagen-coated cell culture dishes (3 × 106 cells/6-cm dish) or plates (250,000 cells/well in 6-well plates) and cultured overnight at 37°C. The old medium was then removed and cells were further incubated with fresh media containing 5% heat-inactivated calf serum under 21% O2 (C, Control), 6 h hypoxia (H) or 6 h hypoxia followed by reoxygenation as previously described (Metukuri et al., 2009). Hypoxic conditions were obtained by placing the cells into a modular incubator chamber (Billups-Rothenburg, Del Mar, CA) flushed with a hypoxic gas mixture containing 1% O2, 5% CO2 and 94% N2. Duplicate hypoxic cultures were returned to 21% O2 for reoxygenation overnight (18 h). At the end of the experiments, both cell culture media (CCM) and whole cell lysate (WCL) were collected and stored at −80°C until analysis. Total protein isolation and determination was done using the BCA protein assay kit from Pierce (Rockford, IL) with bovine serum albumin as standard as previously described (Metukuri et al., 2009). All data were normalized and the final mediator concentrations were expressed in pg/mg total protein. For data analysis and unless otherwise indicated, the number of independent experiments (n) refers to the number of separate mice from which HC or HSC were harvested. Experimental data are presented as mean ± SEM.
-Primary Hepatic Stellate Cells (HSC) were isolated as previously described (Dangi et al., 2012). Briefly, mouse livers from C57BL/6 mice were perfused in situ through the inferior vena cava with 30–40 ml HBSS (without Ca2+), then digested with 30–40 ml HBSS (with Ca2+) containing collagenase type IV (0.25 mg/ml) (Worthington, Lakewood, NJ) and protease (0.50 mg/ml) (Sigma, St. Louis, MO). The cell suspension was filtered through 100 μm nylon mesh. Following removal of HC and cell debris by low speed centrifugation (50 g; 2 min), HSC were purified by Histodenz density gradient centrifugation, and suspended in DMEM containing 100 U/ml penicillin, 100 μg/ml streptomycin, 10% FBS and 10% horse serum. Cells were seeded in gelatin (0.1% in PBS)-coated plates at a density of 0.5 × 106/cm2 and 20 min later, loosely adherent HSC were harvested and re-seeded in new 6 or 96-well flat-bottom plates. Cell purity, as assessed by vitamin A autofluorescence, desmin and glial fibrillary acidic protein (GFAP) immunostaining (Sumpter et al., 2012), was >98%. HSC were cultured under 21% O2, 6 h hypoxia or 6 h hypoxia followed by reoxygenation for 18 h, then harvested for protein isolation and analysis as described above for HC.
Analysis of Inflammatory Mediators
Mouse inflammatory mediators were measured using a Luminex® 100 IS apparatus (Luminex, Austin, TX) and the BioSource 20-plex™mouse cytokine bead kit (BioSource-Invitrogen, San Diego, CA) as per manufacturer's specifications. The antibody bead kit included: Basic fibroblast growth factor (FGF basic), Granulocyte-Macrophage Colony-Stimulating Factor (GM-CSF), Interferon-γ (IFN-γ), Interleukin (IL)-1α, IL-1β, IL-2, IL-4, IL-5, IL-6, IL-10, IL-12 (both p40 and p70 subunits), IL-13, IL-17A, Interferon-γ-inducible Protein 10 (IP-10/CXCL10), Keratinocyte-derived Cytokine (KC/CXCL1), Monocyte Chemoattractant Protein-1 (MCP-1/CCL2), Monokine induced by Interferon-γ (MIG/CXCL9), Macrophage Inflammatory Protein-1α (MIP-1α/CCL3), Tumor Necrosis Factor-α (TNF-α), and Vascular Endothelial Growth Factor (VEGF).
Statistical and Computational Analyses
We applied a series of statistical and data-driven modeling techniques aimed at discovering principal drivers, interconnected networks, and potential key regulatory nodes of inflammation in HSC vs. HC following increasing levels of cellular stress. We applied these methods in a stepwise fashion based on our concept of the way liver cells respond to inflammatory stimuli (Figure 1, adapted from Namas et al., 2015). Importantly, we also sought to address intracellular information processing (“thinking”) as well as extracellular inflammatory mediator production (“talking”) carried out by each cell type individually, as well as inferring how these two processes may interact in each cell type. In brief, we hypothesize that low-level stresses associated with cell culture, as well as more stressful stimuli such as hypoxia with or without reoxygenation, will cause liver cells to produce a panoply of inflammatory mediators in order to restore homeostasis. We hypothesize that this dynamic production of inflammatory mediators takes the form of inflammatory networks, and thus can be elucidated using network inference algorithms. As these early inflammatory responses progress, and in the face of continued hypoxia or the onset of reoxygenation, these networks coalesce around an intermediate, core set of inflammation-associated cellular functions, which we hypothesize can be inferred via PCA and related methods. These intermediate processes evolve to form a differential set of inflammatory responses characterized by distinct mediators that characterize the autocrine response of each liver cell type. These differential responses can be discerned both by standard statistical approaches such as Two-way ANOVA as well as data-driven modeling tools such as hierarchical clustering (Figure 1). Accordingly, we followed the stepwise series of analyses detailed below:
1) Two-Way Analysis of Variance (ANOVA) was carried out to analyze the changes in inflammatory mediators in HC vs. HSC in both CCM and WCL, using SigmaPlot (Systat Software, San Jose, CA) as indicated.
2) Unsupervised Hierarchical Clustering was carried out in an attempt to differentiate the ultimate inflammatory responses of HC and HSC based on concentrations of inflammatory mediators in both CCM and WCL during each of the three experimental conditions examined: control (6 h), hypoxia (6 h) and hypoxia/reoxygenation (6 h/18 h). This analysis was performed using mediator raw values using Matlab® software (The MathWorks, Inc., Natick, MA) as previously described (Ziraldo et al., 2013). Fold change values for each inflammatory mediator are represented in heat maps, ranging from large negative to large positive values as shown in the Figure Legends.
3) Principal Component Analysis (PCA) was carried out to identify those inflammatory mediators that were the most characteristic of the intermediate, multivariate response of each cell type (HC vs. HSC) and experimental condition (control, hypoxia, and hypoxia/reoxygenation) using Matlab® software. To perform this analysis, the data were first normalized for each inflammatory mediator (i.e., a given value divided by the maximum value for a given inflammatory mediator), so that all mediator levels were converted into the same scale (from 0 to 1). In this way, any artifactual effects on variance due to the different ranges of concentration observed for different cytokines were eliminated. Only sufficient components to capture at least 70% of the variance in the data were considered. From these leading principal components, the coefficient (weight) associated with each inflammatory mediator was multiplied by the eigenvalue associated with that principal component. This product represented the contribution of a given mediator to the variance accounted for in that principal component. The overall score given to each mediator is the sum of its scores in each component, depicted as a stacked bar graph. This gives a measure of a given inflammatory mediator's contribution to the overall variance of the system. The mediators with the largest scores are the ones which contributed most to the variance of the process being studied (Mi et al., 2011).
4) Network Analysis was carried out to define the central inflammatory network nodes as a function of cell type (i.e., HC vs. HSC), experimental condition, and spatial localization (lysate vs. conditioned medium) using a modified version of our previously published algorithm for Dynamic Network Analysis (Mi et al., 2011; Ziraldo et al., 2013; Zamora et al., 2016). Connections between inflammatory mediators were created if the Pearson correlation coefficient between any two nodes (inflammatory mediators) was greater or equal to a threshold of 0.95, as indicated. The “network complexity” for each experimental condition was calculated using the following formula: Sum (N1 + N2 +…+ Nn)/n-1, where N represents the number of connections for each mediator and n is the total number of mediators analyzed. The total number of connections represents the sum of the number of connections in a given experimental sub-group.
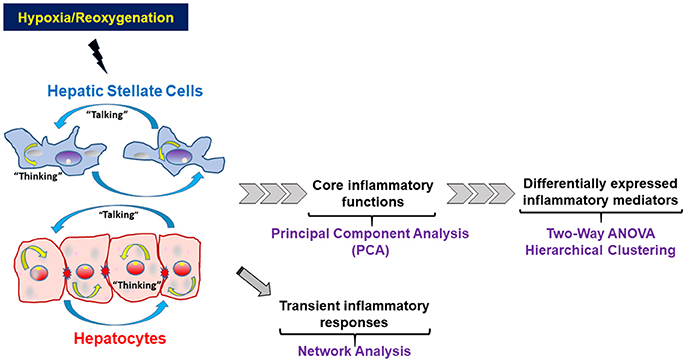
Figure 1. Schematic of the statistical and computational methods and the rationale for their use to study the way liver cells respond to inflammatory stimuli. Hepatocytes and Hepatic Stellate Cells respond to hypoxic stress and reoxygenation by both producing and releasing inflammatory mediators that form defined networks, which can be characterized using network analysis techniques. As the presence of signals and networks persists, early regulatory cytokines begin to be secreted. Some of these core mediators are present at low levels, often with high variance, and their presence and effect may be inferred, as an initial filter, using techniques such as Principal Component Analysis (PCA). At the same time, other inflammatory mediators are usually significantly elevated as defined by standard statistical analyses and thus can be defined as biomarkers of elevated inflammation.
Results
In the present study, we sought to compare, in a systematic fashion, the inflammatory responses of freshly isolated HC or HSC to hypoxia and hypoxia/reoxygenation. We reasoned that distinct statistical and computational analyses would elucidate different steps in the intracellular and extracellular responses of these cells to stress, shown schematically in Figure 1 (see Materials and Methods for details). In the context of that schematic, we worked backwards from the most directly apparent/final responses (assessed by Two-Way ANOVA and hierarchical clustering) to the intermediate responses (assessed by PCA), and then to discerning the most proximal responses to stress (assessed by network analysis). Another key goal of our studies was to utilize computational modeling to assess the interactions between intracellular information processing (“thinking”) vs. extracellular information transfer (“talking”) in both HC and HSC. For that reason, we examined both the whole-cell lysates (WCL) and the cell culture media (CCM) in each cell type.
Differential Production and Release of Inflammatory Mediators in HSC vs. HC in Response to Hypoxia and Hypoxia/Reoxygenation
The production of inflammatory mediators in both CCM and WCL under the three experimental conditions, along with the corresponding P-values for comparison of the changes in HSC vs. HC by Two-Way ANOVA, can be found in Supplementary Figure 1. Key examples of inflammatory mediators whose intracellular and extracellular levels are significantly different between HSC and HC are shown in Figure 2. In general, on a pg protein per mg total cell protein basis, HSC both produced and secreted much larger quantities of inflammatory mediators than the rather indolent response of HC. To identify those inflammatory mediators that showed similar expression or secretion behavior in each cell type, these patterns were then compared and grouped using hierarchical clustering. The resulting dendrograms showed a clear cluster separation between HC and HSC both in the CCM (Figure 3A) and WCL (Figure 3B) under the three experimental conditions studied. From this analysis, we infer that there is more heterogeneity in the intracellular (“thinking”) vs. the extracellular (“talking”) levels of inflammatory mediators in both cell types.
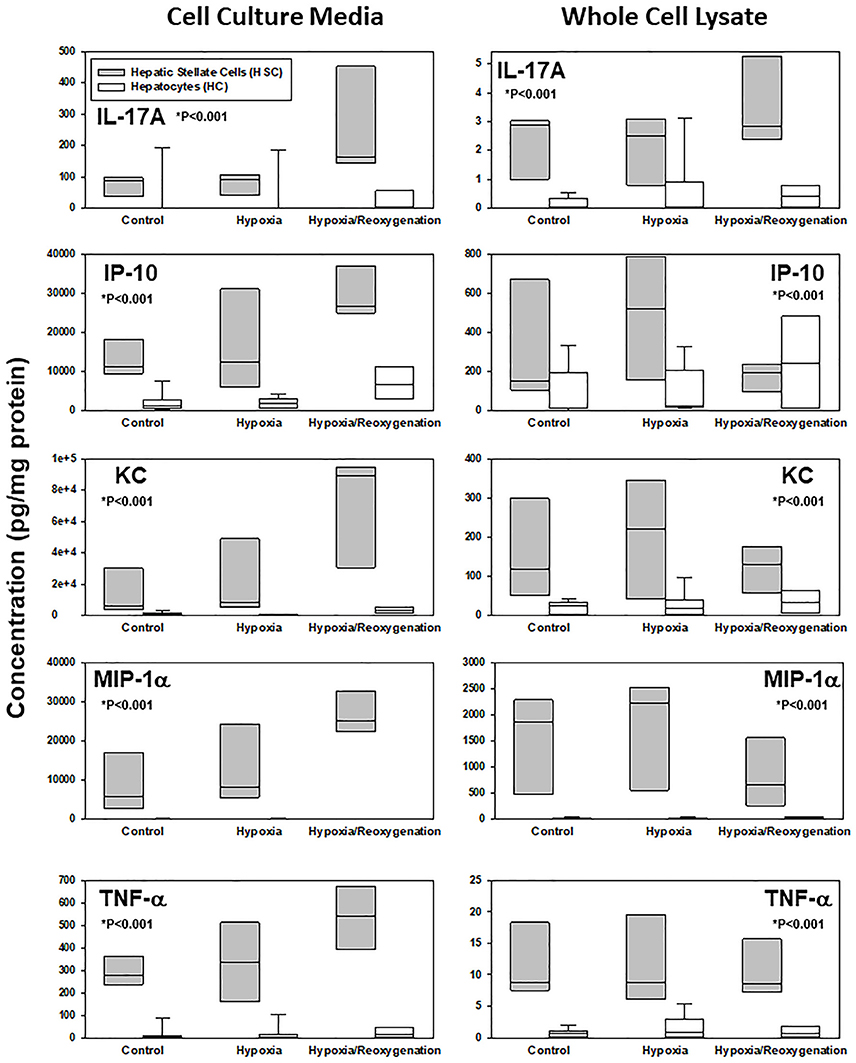
Figure 2. Differential response to hypoxia and hypoxia/reoxygenation in primary mouse hepatic stellate cells (HSC) and hepatocytes (HC). Freshly isolated HSC and HC from C57BL/6 mice were cultured under 21% O2 for 6 h (control), hypoxia (1% O2) for 6 h or hypoxia (6 h) followed by reoxygenation for 18 h. Inflammatory mediators were measured by Luminex™in both cell culture media and whole cell lysate as described in Materials and Methods. Box plots show the levels of IL-17A, IP-10, KC, MIP-1α, and TNF-α, where the line within the box marks the median and the lower and upper boundaries represent the 25th and 75th percentiles, respectively (*P < 0.001, HSC vs. HC, analyzed by Two-Way ANOVA).
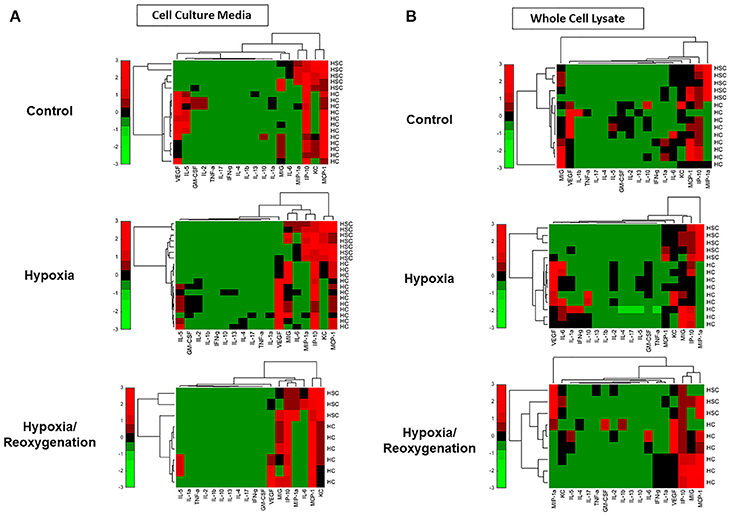
Figure 3. Hierarchical clustering of inflammatory mediators in mouse hepatic stellate cells (HSC) and hepatocytes (HC) and Freshly isolated HSC and HC from C57BL/6 mice were cultured under 21% O2 for 6 h (control), hypoxia (1% O2) for 6 h or hypoxia (6 h) followed by reoxygenation for 18 h. Inflammatory mediators were measured by Luminex™ in both cell culture media (CCM) (A) and whole cell lysate (WCL) (B) as described in Materials and Methods. Fold change values for each inflammatory mediator are represented in heat maps, ranging from large negative (green) to large positive values (red). No changes (zero values) are represented in black.
Hypoxia and Hypoxia/Reoxygenation Induce a Differential Response in Hsc vs. HC Inferred from Principal Component Analysis (PCA)
Next, we sought to define an intermediate, core set of inflammation-associated characteristics of HSC and HC in response to increasing levels of stress, which we hypothesize represent different inflammation-related functions that ultimately become manifest in the expression and secretion of the defined subset of inflammatory mediators studied. To do so, we utilized PCA (Figure 1), a technique (or variants thereof) used by multiple investigators to delineate the core characteristics of a multivariate biological response (Janes et al., 2005; Janes and Yaffe, 2006; Mi et al., 2011; Nieman et al., 2012; Azhar et al., 2013; Ziraldo et al., 2013). We assessed several properties of the inflammatory responses under control, hypoxia, and hypoxia/reoxygenation: (1) the total number of mediators contributing to the total variance (i.e., mediators with sums of eigenvalues > 0; (2) the number of inferred principal components (represented as stacked bars), which we hypothesize represent distinct characteristics or inflammation-related functions; and (3) the sums of the eigenvalues (amplitude) for each experimental condition, which we hypothesize represents the magnitude of the inflammatory response to the indicated level of stress. The number of mediators and number of principal components expressed by each cell type under each condition is shown in Figure 4. An overall comparison of the inflammatory mediators showed that the inflammatory response of HSC was clearly different from that in HC both in CCM (Figure 4A) and WCL (Figure 4B) under each experimental condition. But certain similarities were seen. The major similarity was that the amplitude of the response was greatest when both HSC and HC were subjected to hypoxia/reoxygenation. Moreover, in general, the number of mediators involved as well as the inferred functions (number of principal components) in both WCL (“thinking”) and CCM (“talking”) were lowest in both HSC and HC subjected to hypoxia/reoxygenation as compared to control or hypoxia. A notable exception was the “thinking” response in HC WCL (Figure 4B), in which all three experimental conditions were characterized by two principal components, and the overall number of mediators was least in the hypoxia setting. Together, these analyses suggest that above a certain threshold of cellular stress, the inflammatory response generally becomes focused on a limited number of functions and associated mediators at a high intensity of response.
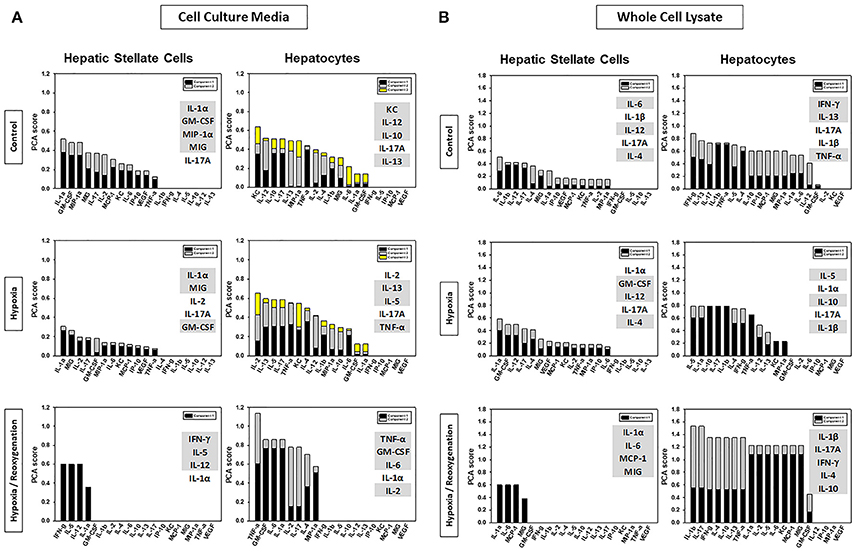
Figure 4. Comparison of HSC and HC based on Principal Component Analysis (PCA). Freshly isolated HSC and HC from C57BL/6 mice were cultured under 21% O2 for 6 h (control), hypoxia (1% O2) for 6 h or hypoxia (6 h) followed by reoxygenation for 18 h. Inflammatory mediators were measured by Luminex™ in both cell culture media (CCM) and whole cell lysate (WCL) and PCA was performed as described in Materials and Methods. Highlighted are the top five inflammatory mediators that contributed the most to the overall variance of the inflammatory response for each experimental condition in CCM (A) and WCL (B).
Network Analysis Revealed the Presence of Distinct Autocrine Inflammatory Networks in HSC vs. HC
The final analysis we carried out was aimed at discovering the initial networks of inflammation induced under control conditions, hypoxia, or hypoxia/reoxygenation (Figure 1). We have previously employed Dynamic Network Analysis (DyNA) to define the dynamic interconnections among inflammatory mediators in a mouse model of trauma/hemorrhagic shock (Mi et al., 2011), in isolated mouse HC subjected to hypoxic stress (Ziraldo et al., 2013), and to define the dynamics of systemic inflammation after trauma (Abboud et al., 2016; Namas et al., 2016) or during acute liver failure (Zamora et al., 2016). For the current analyses, we used a modified version of this method, in which data from a single experimental condition (rather than a time interval) were used.
This network analysis suggested the presence of more complex extracellular inflammatory networks in HSC than in HC under all experimental conditions (Figures 5A,B and Supplementary Figure 2A) but roughly similarly complex intracellular networks (Figures 5C,D and Supplementary Figure 2B). Despite this difference in network complexity, multiple, shared inflammatory mediators were involved in both “thinking” and “talking” in HSC and HC. Secreted mediators shared by networks in both HSC and HC under control conditions included VEGF, MCP-1, KC, and IL-17A. Intracellularly under control conditions, only MIG and IL-4 were shared by HSC and HC networks. Under hypoxia, intracellular HSC and HC networks shared the mediators TNF-α, KC, MIG, and MCP-1, while intracellular networks only shared the mediators MIP-1α and IP-10. Under hypoxia/reoxygenation, secreted HSC and HC networks shared the greatest number of mediators: IL-1α, IL-2, IL-4, IL-6, IL-17A, MIG, KC, IP-10, GM-CSF, VEGF, and TNF-α; in contrast, shared intracellular mediators included TNF-α, MIG, MCP-1, IL-1α, and IL-17A. Control experiments comparing single network analysis of both isolated HC and HSC cultured under 21% O2 for 24 h confirmed the presence of more complex extracellular inflammatory networks in HSC than in HC under both control and hypoxia/reoxygenation (Supplementary Figure 3A) and similar complex intracellular networks after hypoxia/reoxygenation (Supplementary Figure 3B).
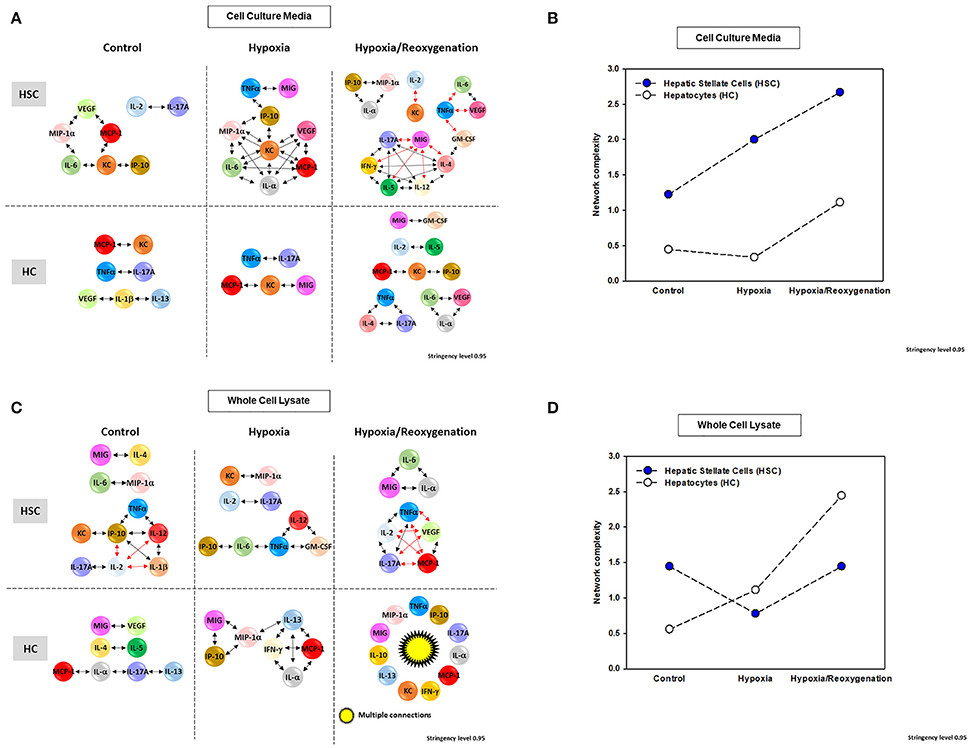
Figure 5. Network Analysis of inflammatory mediators in mouse hepatic stellate cells (HSC) and hepatocytes (HC) Freshly isolated HSC and HC from C57BL/6 mice were cultured under 21% O2 for 6 h (control), hypoxia (1% O2) for 6 h or hypoxia (6 h) followed by reoxygenation for 18 h. Inflammatory mediators released into cell culture media (CCM) and in whole cell lysate (WCL) were measured by Luminex™ and Network Analysis was performed as described in Materials and Methods. Figure shows the networks and the detailed connectivity (stringency level 0.95) for each experimental condition in both cell types in CCM (A) and WCL (C). Black and red arrows represent positive and negative connections, respectively. (B,D) Show the network complexity in HSC vs. HC for CCM and WCL, respectively.
Based on PCA (Figure 4), we hypothesized that network interactions would lead to a reduced set of principal mediators and inflammatory functions in both CCM and WCL of HSC as they transition from hypoxia to hypoxia + reoxygenation. In support of this hypothesis, the networks observed under hypoxia/reoxygenation in HSC CCM as well as WCL exhibited multiple anti-correlated nodes (indicated in red; Figures 5A,C). We interpret this finding to mean that both intracellular and extracellular HSC mediators induced in the setting of hypoxia followed by reoxygenation regulate each other in a negative fashion that leads ultimately to the evolution of a reduced number of inflammatory functions relative to hypoxia alone. In the extracellular milieu of HSC, the chemokine MIG; the cytokines TNF-α, IL-6, and IL-17A; and the hypoxia-inducible growth factor VEGF appear to coordinate some of these downregulatory responses (Figure 5A). VEGF, TNF-α, MCP-1, and IL-17A also appear to play a more limited negative intracellular role (Figure 5B).
The foregoing analyses suggested that there may be an interplay between intracellular responses (“thinking”) and extracellular responses (“talking”) in each cell type. We therefore next sought to use network analysis to gain insights into the autocrine information flow within each cell population. Accordingly, we repeated the network analysis, but this time using both CCM and WCL data together for HSC vs. HC (Figure 6). Surprisingly, this analysis suggested the presence of multiple negative interactions in control HSC. This analysis also inferred the presence of a large number of negative interactions in both HSC and HC exposed to hypoxia/reoxygenation (Figure 6A,Table 1). Network analysis also demonstrated a higher complexity of autocrine networks involving both intracellular (“thinking”) and extracellular (“talking”) in HSC as compared to HC (Figure 6B), similar to the results obtained separately in CCM and WCL (Figures 5B,D).
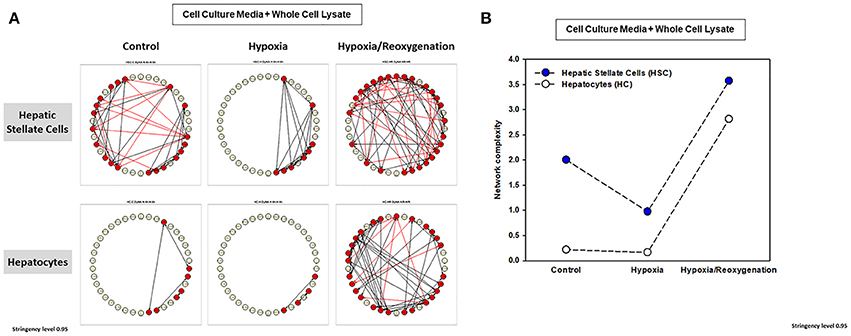
Figure 6. Network Analysis of inflammatory mediators using the combined data of both the cell culture media (CCM) and whole cell lysate (WCL) in mouse hepatic stellate cells (HSC) and hepatocytes (HC). Freshly isolated HSC and HC from C57BL/6 mice were cultured under 21% O2 for 6 h (control), hypoxia (1% O2) for 6 h or hypoxia (6 h) followed by reoxygenation for 18 h. Inflammatory mediators released into cell culture media (CCM) and in whole cell lysate (WCL) were measured by Luminex™ and Network Analysis was performed as described in Materials and Methods. The figure shows the networks and the detailed connectivity (stringency level 0.95) for each experimental condition combining the data in CCM and WCL (A). Black and red arrows represent positive and negative connections, respectively. (B) Compares the combined network complexity in HSC vs. HC.
We next assessed the flow of information from the intracellular (WCL) to extracellular milieu (CCM) in HSC vs. HC in greater detail (Figure 6 and Supplementary Table 1) Under control conditions, inflammatory networks in HC were comprised solely of extracellular mediators (IL-1β, IL-13, IL-17A, MCP-1, KC, VEGF, and TNF-α). In contrast, the combined network analysis inferred multiple positive and negative connections among a large number of intracellular and extracellular inflammatory mediators in HSC, suggesting a much more robust “thinking” and “talking” interplay in this cell type at baseline. Under hypoxia alone, both HSC and HC exhibited only positive, extracellular inflammatory connectivity, though the number of connections was higher in HSC (a large number of mediators) as compared to HC (only TNF-α, IL-17A, KC, MCP-1, and MIG). Interestingly, when subjected to hypoxia/reoxygenation, both HSC and HC exhibited a large number of positive and negative connections in the intracellular as well as extracellular compartments. This suggests a major adaptation to stress in HC, while this phenotype in HSC remained qualitatively similar to that observed under control conditions.
Discussion
We report for the first time a detailed multiplex analysis of inflammatory mediators in isolated HSC and HC cultured under hypoxic conditions with and without reoxygenation, which suggests the presence of distinct, endogenous autocrine networks in isolated HSC and HC. The other cellular and structural components of the liver (vascular and sinusoidal endothelial cells, Kupffer cells, fibroblasts, biliary ducts, etc.) were excluded deliberately from these experiments in order to study the complexity of the autocrine responses of these two cellular components without the contribution of inflammatory mediators from contiguous cell types. Our data-driven computational work flow (see conceptual summary in Figure 1) was designed to elucidate how early inflammatory networks can lead to an intermediate set of responses that manifest ultimately in a generally restricted number of inflammatory mediators/functions that distinguish the responses of HSC from those of HC. Based on this work flow, we show that both cell types respond to hypoxic stress by releasing a somewhat similar, but not identical, repertoire of cytokines and chemokines in vitro. The similarities suggest the possible presence of a mutually supportive autocrine environment in the intact liver in vivo. The hypoxic stimulus evokes a much greater change in the secreted cellular inflammatory network of HSC than HC, indicating that the secretory machine in HC might be either more limited at baseline or disrupted following stress. Furthermore, the responses of HSC to hypoxia followed by reoxygenation are more vigorous and form more interactive networks than responses to hypoxia alone.
A significant role for HSC in regulating hepatic inflammatory and immunological responses by altering expression of numerous relevant genes has already been shown in LPS-stimulated rat HSC (Harvey et al., 2013). We have also shown previously that HSC produce numerous inflammatory mediators constitutively, expression of which is increased upon stimulation by LPS (Uemura and Gandhi, 2001; Thirunavukkarasu et al., 2005) (Thirunavukkarasu et al., 2008; Jameel et al., 2010; Harvey et al., 2013; Dangi et al., 2016). Furthermore, activation of unstimulated HSC stimulate DNA synthesis in HC (Uemura and Gandhi, 2001), and the mediators produced by LPS-stimulated HSC induce autophagy in HC as a survival mechanism but also cause apoptosis of a subset of cells (Dangi et al., 2016). We also reported a comprehensive analysis of LPS-induced expression of inflammatory mediators in HSC (Harvey et al., 2013). In the setting of hypoxia, we found that HSC-depleted mice are protected from ischemia/reperfusion injury, suggesting that under ischemic/hypoxic condition HSC release mediators that are injurious to HC (Stewart et al., 2014). Our analyses in the current study showed the presence of more complex autocrine inflammatory networks in HSC as compared to HC, which supports the notion that these two cell types not only coexist but also provide a microenvironment for each other in which HSC play the “messenger” role as opposed more of a “responder” role for HC. Experimental testing of this inference is part of ongoing and future experiments that will include other liver cells as well.
Our results suggest a complex interplay among chemokines (e.g., MCP-1, KC, MIG, and IP-10), cytokines (e.g., TNF-α and IL-17A, among many others), and growth factors (e.g., VEGF) in the response to hypoxia ± reoxygenation even in the absence of the contribution of other liver cells. Some of these cytokines have been reported to be induced by stress in HC and HSC previously (Kmieć, 2001; Brenner et al., 2013; Rani et al., 2017). Our computational analysis, however, elucidate a novel dimension of information regarding how HSC and HC “think” and “talk”: as stress increases, intracellular and extracellular information transfer appears to result in a reduction of inflammation-related functions, a process we hypothesize is driven through negative network interactions among key inflammatory mediators.
Finally, we are aware of the limitations in translating these computational analyses in vivo. Under physiological conditions O2 is a major effector of metabolic zonation and plays a major role in the liver. When compared to other somatic cells, liver cells are relatively hypoxic, both in the periportal (zone 1, 9–11% O2) and the perivenous region (zone 3, 5–7% O2) (Jungermann and Kietzmann, 2000). Changes in this complex O2 gradient, as well as in the production of hormones and enzymes, affect the response of the liver in a number of pathophysiological processes. Thus, we agree that virtually all routine tissue culture experiments involving liver cells, such as the control conditions described herein are normally performed under relatively hyperoxic conditions that may not reflect in vivo physiology (Sullivan et al., 2006). Notably, the complex O2 gradient in the liver is very difficult to duplicate using isolated cells in vitro. Furthermore, it is also difficult to identify (and quantify) the presence and activity of specific inflammatory or parenchymal cells during specific time periods, as is the identification of potential crosstalk among dynamic networks in vivo. Although it is very likely, whether or not these inflammatory networks change in both composition and complexity when HC and HSC are in close proximity, as it happens in vivo, has not yet been reported and it represents another interesting element in the complex relationship between liver cells. To elucidate this and other of our hypotheses will require a more detailed analysis of data obtained from co-cultures of HC with HSC both alone and in combination with other relevant cells in the liver such as Kupffer cells and endothelial cells. Future studies will be directed to address these limitations, specifically we have ongoing studies in primary mouse HC as well as in plasma and whole liver in a mouse model of ischemia/reperfusion.
In summary, our systems-based analysis suggests the presence of an inflammatory homeostatic environment in resting isolated liver cells, which is disrupted under stress such as hypoxia and hypoxia followed by reoxygenation. The vigorous production of secreted inflammatory mediators from HSC and the relative indolent response from isolated HC suggest that HSC act as resident transformers of external stimuli or blood-borne for other less responsive cells within the complex cellular structure of the liver. The relevance of these findings in vivo warrants further investigation.
Author Contributions
YV participated in computational and statistical analysis, data interpretation, and writing. RS participated in data interpretation and writing. CG participated in data interpretation and writing. DB participated in Luminex analysis. BJ participated in cell culture experiments (HC). Fe-D participated in data analysis. QM participated in statistical and computational analysis. CH participated in cell culture experiments (HSC). TB participated in data interpretation and writing. RZ participated in study design, computational and statistical analysis, data interpretation, and writing. RN participated in cell culture experiments (HSC).
Funding
This work was supported by NIH grant RO1-GM107231.
Conflict of Interest Statement
The authors declare that the research was conducted in the absence of any commercial or financial relationships that could be construed as a potential conflict of interest.
Supplementary Material
The Supplementary Material for this article can be found online at: https://www.frontiersin.org/articles/10.3389/fphys.2017.01104/full#supplementary-material
Supplementary Figure 1. Production of inflammatory mediators in mouse hepatic stellate cells (HSC) and hepatocytes (HC). Freshly isolated HSC and HC from C57BL/6 mice were cultured under 21% O2 for 6 h (control), hypoxia (1% O2) for 6 h or hypoxia (6 h) followed by reoxygenation for 18 h. Inflammatory mediators were measured by Luminex™ in both cell culture media and whole cell lysate as described in Materials and Methods. Results shown represent mean ± SEM (*P < 0.05, HSC vs. HC, analyzed by Two-Way ANOVA).
Supplementary Figure 2. Network Analysis of inflammatory mediators in mouse hepatic stellate cells (HSC) and hepatocytes (HC). Freshly isolated HSC and HC from C57BL/6 mice were cultured under 21% O2 for 6 h (control), hypoxia (1% O2) for 6 h or hypoxia (6 h) followed by reoxygenation for 18 h. Inflammatory mediators released into cell culture media (CCM) and in whole cell lysate (WCL) were measured by Luminex™ and Network Analysis was performed as described in Materials and Methods. Figure shows the networks (stringency level 0.95) for each experimental condition in both cell types in CCM (A) and WCL (B). Black and red arrows represent positive and negative connections, respectively.
Supplementary Figure 3. Network Analysis of inflammatory mediators in mouse hepatic stellate cells (HSC) and hepatocytes (HC). Freshly isolated HSC and HC from C57BL/6 mice were cultured under 21% O2 for 24 h (control) or hypoxia (6 h) followed by reoxygenation for 18 h (as in Supplementary Table 1). Inflammatory mediators released into cell culture media (CCM) and in whole cell lysate (WCL) were measured by Luminex™ and Network Analysis was performed as described in Materials and Methods. Figure shows the networks (stringency level 0.95) for each experimental condition in both cell types in CCM (A) and WCL (B). Black and red arrows represent positive and negative connections, respectively.
Supplementary Table 1. Network Analysis of inflammatory mediators using the combined data of both the cell culture media (CCM) and whole cell lysate (WCL) in mouse hepatic stellate cells (HSC) and hepatocytes (HC). Freshly isolated HSC and HC from C57BL/6 mice were cultured under 21% O2 for 6 h (control), hypoxia (1% O2) for 6 h or hypoxia (6 h) followed by reoxygenation for 18 h. Inflammatory mediators released into cell culture media (CCM) and in whole cell lysate (WCL) were measured by Luminex® and Network Analysis was performed as described in Materials and Methods. The figure shows the detailed connectivity (stringency level 0.95) for each experimental condition combining the data in CCM and WCL.
Abbreviations
GM-CSF, Granulocyte-Macrophage Colony Stimulating Factor; IFN, Interferon; IL, interleukin; IP-10, IFN-γ-inducible Protein of 10 kDa/CXCL10; KC, Keratinocyte-derived Cytokine/CXCL1; MCP-1, Monocyte Chemotactic Protein-1/CCL2; MIG, Monokine induced by γ-interferon/CXCL9; MIP-1α, Macrophage Inflammatory Protein-1α (MIP-1α/CCL3α); TNF-α, Tumor Necrosis Factor-α; VEGF, Vascular Endothelial Growth Factor; PCA, Principal Component Analysis.
References
Abboud, A., Namas, R. A., Ramadan, M., Mi, Q., Almahmoud, K., Abdul-Malak, O., et al. (2016). Computational analysis supports an early, type 17 cell-associated divergence of blunt trauma survival and mortality. Crit. Care Med. 44, e1074–e1081. doi: 10.1097/ccm.0000000000001951
Almahmoud, K., Namas, R. A., Zaaqoq, A. M., Abdul-Malak, O., Namas, R., Zamora, R., et al. (2015). Prehospital hypotension is associated with altered inflammation dynamics and worse outcomes following blunt trauma in humans. Crit. Care Med. 43, 1395–1404. doi: 10.1097/CCM.0000000000000964
Azhar, N., Ziraldo, C., Barclay, D., Rudnick, D. A., Squires, R. H., Vodovotz, Y., et al. (2013). Analysis of serum inflammatory mediators identifies unique dynamic networks associated with death and spontaneous survival in pediatric acute liver failure. PLoS ONE 8:e78202. doi: 10.1371/journal.pone.0078202
Berardis, S., Lombard, C., Evraerts, J., El Taghdouini, A., Rosseels, V., Sancho-Bru, P., et al. (2014). Gene expression profiling and secretome analysis differentiate adult-derived human liver stem/progenitor cells and human hepatic stellate cells. PLoS ONE 9:e86137. doi: 10.1371/journal.pone.0086137
Bilzer, M., and Gerbes, A. L. (2000). Preservation injury of the liver: mechanisms and novel therapeutic strategies. J. Hepatol. 32, 508–515. doi: 10.1016/S0168-8278(00)80404-3
Bilzer, M., Roggel, F., and Gerbes, A. L. (2006). Role of Kupffer cells in host defense and liver disease. Liver Int. 26, 1175–1186. doi: 10.1111/j.1478-3231.2006.01342.x
Brenner, C., Galluzzi, L., Kepp, O., and Kroemer, G. (2013). Decoding cell death signals in liver inflammation. J. Hepatol. 59, 583–594. doi: 10.1016/j.jhep.2013.03.033
Copple, B. L., Bai, S., Burgoon, L. D., and Moon, J. O. (2011). Hypoxia-inducible factor-1alpha regulates the expression of genes in hypoxic hepatic stellate cells important for collagen deposition and angiogenesis. Liver Int. 31, 230–244. doi: 10.1111/j.1478-3231.2010.02347.x
Dangi, A., Huang, C., Tandon, A., Stolz, D., Wu, T., and Gandhi, C. R. (2016). Endotoxin-stimulated rat hepatic stellate cells induce autophagy in hepatocytes as a survival mechanism. J. Cell. Physiol. 231, 94–105. doi: 10.1002/jcp.25055
Dangi, A., Sumpter, T. L., Kimura, S., Stolz, D. B., Murase, N., Raimondi, G., et al. (2012). Selective expansion of allogeneic regulatory T cells by hepatic stellate cells: role of endotoxin and implications for allograft tolerance. J. Immunol. 188, 3667–3677. doi: 10.4049/jimmunol.1102460
de Groot, H. (1992). Isolated cells in the study of the molecular mechanisms of reperfusion injury. Toxicol. Lett. 63, 111–125
de Oliveira da Silva, B., Ramos, L. F., and Moraes, K. C. M. (2017). Molecular interplays in hepatic stellate cells: apoptosis, senescence, and phenotype reversion as cellular connections that modulate liver fibrosis. Cell Biol. Int. 41, 946–959. doi: 10.1002/cbin.10790
Geerts, A. (2001). History, heterogeneity, developmental biology, and functions of quiescent hepatic stellate cells. Semin. Liver Dis. 21, 311–335. doi: 10.1055/s-2001-17550
Harvey, S. A., Dangi, A., Tandon, A., and Gandhi, C. R. (2013). The transcriptomic response of rat hepatic stellate cells to endotoxin: implications for hepatic inflammation and immune regulation. PLoS ONE 8:e82159. doi: 10.1371/journal.pone.0082159
Jameel, N. M., Thirunavukkarasu, C., Murase, N., Cascio, M., Prelich, J., Yang, S., et al. (2010). Constitutive release of powerful antioxidant-scavenging activity by hepatic stellate cells: protection of hepatocytes from ischemia/reperfusion injury. Liver Transpl. 16, 1400–1409. doi: 10.1002/lt.22172
Janes, K. A., Albeck, J. G., Gaudet, S., Sorger, P. K., Lauffenburger, D. A., and Yaffe, M. B. (2005). A systems model of signaling identifies a molecular basis set for cytokine-induced apoptosis. Science 310, 1646–1653. doi: 10.1126/science.1116598
Janes, K. A., and Yaffe, M. B. (2006). Data-driven modelling of signal-transduction networks. Nat. Rev. Mol. Cell Biol. 7, 820–828. doi: 10.1038/nrm2041
Jungermann, K., and Kietzmann, T. (2000). Oxygen: modulator of metabolic zonation and disease of the liver. Hepatology 31, 255–260. doi: 10.1002/hep.510310201
Kmieć, Z. (2001). Cooperation of liver cells in health and disease. Adv Anat Embryol Cell Biol 161, Iii–xiii, 1–151.
Metukuri, M. R., Beer-Stolz, D., Namas, R. A., Dhupar, R., Torres, A., Loughran, P. A., et al. (2009). Expression and subcellular localization of BNIP3 in hypoxic hepatocytes and liver stress. Am. J. Physiol. Gastrointest. Liver Physiol. 296, G499–G509. doi: 10.1152/ajpgi.90526.2008
Mi, Q., Constantine, G., Ziraldo, C., Solovyev, A., Torres, A., Namas, R., et al. (2011). A dynamic view of trauma/hemorrhage-induced inflammation in mice: principal drivers and networks. PLoS ONE 6:e19424. doi: 10.1371/journal.pone.0019424
Namas, R. A., Mi, Q., Namas, R., Almahmoud, K., Zaaqoq, A. M., Abdul-Malak, O., et al. (2015). Insights into the role of chemokines, damage-associated molecular patterns, and lymphocyte-derived mediators from computational models of trauma-induced inflammation. Antioxid. Redox Signal. 23, 1370–1387. doi: 10.1089/ars.2015.6398
Namas, R. A., Vodovotz, Y., Almahmoud, K., Abdul-Malak, O., Zaaqoq, A., Namas, R., et al. (2016). Temporal patterns of circulating inflammation biomarker networks differentiate susceptibility to nosocomial infection following blunt trauma in humans. Ann. Surg. 263, 191–198. doi: 10.1097/sla.0000000000001001
Nieman, G., Brown, D., Sarkar, J., Kubiak, B., Ziraldo, C., Dutta-Moscato, J., et al. (2012). A two-compartment mathematical model of endotoxin-induced inflammatory and physiologic alterations in swine. Crit. Care Med. 40, 1052–1063. doi: 10.1097/CCM.0b013e31823e986a
Ogese, M. O., Faulkner, L., Jenkins, R. E., French, N. S., Copple, I. M., Antoine, D. J., et al. (2017). Characterisation of drug-specific signalling between primary human hepatocytes and immune cells. Toxicol. Sci. 158, 76–89. doi: 10.1093/toxsci/kfx069
Peitzman, A. B., Billiar, T. R., Harbrecht, B. G., Kelly, E., Udekwu, A. O., and Simmons, R. L. (1995). Hemorrhagic shock. Curr. Probl. Surg. 32, 925–1002.
Rani, R., Tandon, A., Wang, J., Kumar, S., and Gandhi, C. R. (2017). Stellate cells orchestrate concanavalin a-induced acute liver damage. Am. J. Pathol. 187, 2008–2019. doi: 10.1016/j.ajpath.2017.05.015
Stewart, R. K., Dangi, A., Huang, C., Murase, N., Kimura, S., Stolz, D. B., et al. (2014). A novel mouse model of depletion of stellate cells clarifies their role in ischemia/reperfusion- and endotoxin-induced acute liver injury. J. Hepatol. 60, 298–305. doi: 10.1016/j.jhep.2013.09.013
Stonans, I., Stonane, E., Russwurm, S., Deigner, H. P., Böhm, K. J., Wiederhold, M., et al. (1999). HepG2 human hepatoma cells express multiple cytokine genes. Cytokine 11, 151–156. doi: 10.1006/cyto.1998.0366
Sullivan, M., Galea, P., and Latif, S. (2006). What is the appropriate oxygen tension for in vitro culture? Mol. Hum. Reprod. 12, 653. doi: 10.1093/molehr/gal081
Sumpter, T. L., Dangi, A., Matta, B. M., Huang, C., Stolz, D. B., Vodovotz, Y., et al. (2012). Hepatic stellate cells undermine the allostimulatory function of liver myeloid dendritic cells via STAT3-dependent induction of IDO. J. Immunol. 189, 3848–3858. doi: 10.4049/jimmunol.1200819
Sun, Q., Loughran, P., Shapiro, R., Shrivastava, I. H., Antoine, D. J., Li, T., et al. (2017). Redox-dependent regulation of hepatocyte absent in melanoma 2 inflammasome activation in sterile liver injury in mice. Hepatology 65, 253–268. doi: 10.1002/hep.28893
Thirunavukkarasu, C., Uemura, T., Wang, L. F., Watkins, S. C., and Gandhi, C. R. (2005). Normal rat hepatic stellate cells respond to endotoxin in LBP-independent manner to produce inhibitor(s) of DNA synthesis in hepatocytes. J. Cell. Physiol. 204, 654–665. doi: 10.1002/jcp.20366
Thirunavukkarasu, C., Wang, L. F., Harvey, S. A., Watkins, S. C., Chaillet, J. R., Prelich, J., et al. (2008). Augmenter of liver regeneration: an important intracellular survival factor for hepatocytes. J. Hepatol. 48, 578–588. doi: 10.1016/j.jhep.2007.12.010
Uemura, T., and Gandhi, C. R. (2001). Inhibition of DNA synthesis in cultured hepatocytes by endotoxin-conditioned medium of activated stellate cells is transforming growth factor-beta and nitric oxide-independent. Br. J. Pharmacol. 133, 1125–1133. doi: 10.1038/sj.bjp.0704151
Vodovotz, Y., Kim, P. K., Bagci, E. Z., Ermentrout, G. B., Chow, C. C., Bahar, I., et al. (2004). Inflammatory modulation of hepatocyte apoptosis by nitric oxide: in vivo, in vitro, and in silico studies. Curr. Mol. Med. 4, 753–762.
West, M. A., and Wilson, C. (1996). Hypoxic alterations in cellular signal transduction in shock and sepsis. New Horiz. 4, 168–178.
Woolbright, B. L., and Jaeschke, H. (2017). The impact of sterile inflammation in acute liver injury. J. Clin. Transl. Res. 3(Suppl. 1), 170–188. doi: 10.18053/jctres.03.2017S1.003
Zaaqoq, A. M., Namas, R., Almahmoud, K., Azhar, N., Mi, Q., Zamora, R., et al. (2014). Inducible protein-10, a potential driver of neurally controlled interleukin-10 and morbidity in human blunt trauma. Crit. Care Med. 42, 1487–1497. doi: 10.1097/ccm.0000000000000248
Zamora, R., Vodovotz, Y., Mi, Q., Barclay, D., Yin, J., Horslen, S., et al. (2016). Data-driven modeling for precision medicine in pediatric acute liver failure. Mol. Med. 22, 821–829. doi: 10.2119/molmed.2016.00183
Ziraldo, C., Ghuma, A., Namas, R., Ochoa, J., Billiar, T. R., Zamora, R., et al. (2011). Early prediction of length of intensive care unit stay from inflammatory mediators in trauma patients. J. Crit. Care 26, e5–e6. doi: 10.1016/j.jcrc.2010.12.027
Keywords: liver, hepatocyte, stellate cell, cytokines, systems biology, hypoxia
Citation: Vodovotz Y, Simmons RL, Gandhi CR, Barclay D, Jefferson BS, Huang C, Namas R, el-Dehaibi F, Mi Q, Billiar TR and Zamora R (2017) “Thinking” vs. “Talking”: Differential Autocrine Inflammatory Networks in Isolated Primary Hepatic Stellate Cells and Hepatocytes under Hypoxic Stress. Front. Physiol. 8:1104. doi: 10.3389/fphys.2017.01104
Received: 11 September 2017; Accepted: 14 December 2017;
Published: 22 December 2017.
Edited by:
Enrique Hernandez-Lemus, National Institute of Genomic Medicine, MexicoReviewed by:
Savneet Kaur, Institute of Liver and Biliary Sciences, IndiaDavid Sharkey, University of Adelaide, Australia
Copyright © 2017 Vodovotz, Simmons, Gandhi, Barclay, Jefferson, Huang, Namas, el-Dehaibi, Mi, Billiar and Zamora. This is an open-access article distributed under the terms of the Creative Commons Attribution License (CC BY). The use, distribution or reproduction in other forums is permitted, provided the original author(s) or licensor are credited and that the original publication in this journal is cited, in accordance with accepted academic practice. No use, distribution or reproduction is permitted which does not comply with these terms.
*Correspondence: Ruben Zamora, emFtb3JhckBwaXR0LmVkdQ==
†Present Address: Chandrashekhar R. Gandhi, Department of Surgery, University of Cincinnati, OH, United States