- 1School of Information and Control Engineering, China University of Mining and Technology, Xuzhou, China
- 2School of Computer Science and Technology, Nankai University, Tianjin, China
- 3College of Computer Science and Software Engineering, Shenzhen University, Shenzhen, China
Nowadays, as more and more associations between microRNAs (miRNAs) and diseases have been discovered, miRNA has gradually become a hot topic in the biological field. Because of the high consumption of time and money on carrying out biological experiments, computational method which can help scientists choose the most likely associations between miRNAs and diseases for further experimental studies is desperately needed. In this study, we proposed a method of Graph Regression for MiRNA-Disease Association prediction (GRMDA) which combines known miRNA-disease associations, miRNA functional similarity, disease semantic similarity, and Gaussian interaction profile kernel similarity. We used Gaussian interaction profile kernel similarity to supplement the shortage of miRNA functional similarity and disease semantic similarity. Furthermore, the graph regression was synchronously performed in three latent spaces, including association space, miRNA similarity space, and disease similarity space, by using two matrix factorization approaches called Singular Value Decomposition and Partial Least-Squares to extract important related attributes and filter the noise. In the leave-one-out cross validation and five-fold cross validation, GRMDA obtained the AUCs of 0.8272 and 0.8080 ± 0.0024, respectively. Thus, its performance is better than some previous models. In the case study of Lymphoma using the recorded miRNA-disease associations in HMDD V2.0 database, 88% of top 50 predicted miRNAs were verified by experimental literatures. In order to test the performance of GRMDA on new diseases with no known related miRNAs, we took Breast Neoplasms as an example by regarding all the known related miRNAs as unknown ones. We found that 100% of top 50 predicted miRNAs were verified. Moreover, 84% of top 50 predicted miRNAs in case study for Esophageal Neoplasms based on HMDD V1.0 were verified to have known associations. In conclusion, GRMDA is an effective and practical method for miRNA-disease association prediction.
Introduction
MicroRNA (miRNA) is a small non-coding, single stranded and endogenous RNA molecule (containing 21~24 nucleotides) found in plants, animals, and some viruses, which functions in regulation of the gene expression by targeting mRNAs for cleavage or translational repression at the post-transcriptional level (Ambros, 2001, 2004; Bartel, 2004; Meister and Tuschl, 2004). The first miRNA was discovered in the early 1990s (Lee et al., 1993; Wightman et al., 1993). However, miRNAs were not recognized as a distinct class of biological regulators until the early 2000s (Pasquinelli et al., 2000; Reinhart et al., 2000; Lagos-Quintana et al., 2001; Lau et al., 2001; Lee and Ambros, 2001). Nowadays thousands of miRNAs from a wide variety of species have been found (Jopling et al., 2005; Kozomara and Griffiths-Jones, 2011). Furthermore, increasing researches have demonstrated that the miRNAs play crucial roles at multiple stages of the biological processes (Lee et al., 1993), such as early cell growth, proliferation (Cheng et al., 2005), differentiation (Miska, 2005), development (Karp and Ambros, 2005), aging (Bartel, 2009), apoptosis (Skalsky and Cullen, 2011), and so on. The dysregulation of the miRNAs has been confirmed as a main reason of aberrant cell behavior and important human complex diseases by many studies (Griffiths-Jones et al., 2006). More and more miRNAs have been verified to have associations with the development processes of many human diseases in experiments (Lynam-Lennon et al., 2009; Meola et al., 2009). For example, studies have implicated that epigenetic modulation of the miR-200 family has relevance to transition to a breast cancer stem cell-like state (Lim et al., 2013). Besides, recent study demonstrated that in human colorectal cancer cells, miR-186, miR-216b, miR-337-3p, and miR-760 could work in synergy to induce cellular senescence by targeting alpha subunit of protein kinase CKII (Kim et al., 2012). Therefore, identifying disease-related miRNAs is important and beneficial to treat, diagnose, and prevent human complex diseases. However, considering the huge amount of time and money we have to spend in carrying out experiments to verify a single miRNA-disease association, it is impossible to verify the associations one by one. Thus, it is necessary and valuable to choose the most likely associations to verify in the biological laboratory first. Therefore, considering there are some verified miRNA-disease datasets which can be treated as materials for prediction, we can develop computational models to rank and predict potential miRNA-disease associations.
In fact, scientists have already developed some computational methods in predicting miRNA-disease associations (Chen et al., 2012b, 2016c; Mork et al., 2014; Chen, 2016; Zeng et al., 2016; You et al., 2017). Many computational methods are based on a credible assumption that functionally similar miRNAs tend to have associations with phenotypically similar diseases to predict the potential associations between miRNAs and diseases. For example, Pasquier and Gardes (2016) constructed an vector space to predict miRNA-disease associations. They represented miRNA and disease distributional information with high-dimensional vectors respectively and then defined associations between miRNAs and diseases in terms of their vector similarity. Jiang et al. (2010) used a human phenome-microRNAome network to obtain the priority of miRNA-disease associations. Its weakness is that there was a high proportion of false positive and false negative samples in the miRNA-target interactions dataset on which this method extremely depended. To make up these weakness, a random walk algorithm-based model in protein-protein interaction (PPI) network was proposed (Shi et al., 2013). This method predicted potential associations between the miRNAs and diseases through combining the miRNA–target interactions, disease–gene associations, and PPIs. Mork et al. (2014) presented a miRNA-Protein-Disease Associations (miRPD) method which combined protein-disease association scores and miRNA-protein association scores to rank candidate miRNAs. Xu et al. (2014) introduced a systematic miRNA prioritization method based on known disease–gene associations and context-dependent miRNA-target interactions. Nonetheless, because of the high false positive and false negative samples existing in miRNA-target interactions and the incomplete disease-gene association network, the aforementioned methods could not provide sufficiently accurate prediction results.
Furthermore, based on the observation that miRNAs with similar functions are normally associated with similar diseases and vice versa, an effective prediction algorithm based on weighted k most similar neighbors for Human Disease MiRNAs prediction (HDMP) was proposed by Xuan et al. (2013) to predict the disease-related miRNAs using the miRNA functional similarity, disease semantic similarity, disease phenotype similarity, and the known miRNA-disease associations. However, the HDMP is not suitable to detect the association about a new disease which has no known related miRNAs. What's more, for a disease, if the number of its known related miRNAs is not enough, the prediction result will be not so satisfactory. Chen et al. (2012b) presented the first global network similarity-based computational model called Random Walk with Restart for MiRNA–Disease Association prediction (RWRMDA) by making use of the random walk algorithm based on the information of human miRNA functional similarity and known human miRNA–disease associations. RWRMDA obtained an excellent prediction performance. However, there is a non-negligible limitation that this method could not work for new diseases with no known related miRNAs. Chen et al. (2016b) proposed another model called Within and Between Score for MiRNA-Disease Association prediction (WBSMDA), which could effectively predict the potential miRNAs related to new diseases without any known related miRNAs and potential diseases related to new miRNAs without any known associated diseases. Recently, Chen et al. (2016c) developed a novel computational called Heterogeneous Graph Inference for MiRNA-Disease Association prediction (HGIMDA), using an iterative process with the initial probability vector, which can overcome the weakness that not being able to predict diseases with no known related miRNA, occurred in other methods.
Nowadays, machine learning has been applied in vast research fields and has great performance in many research problems (Chen et al., 2012a, 2016a,d; Huang et al., 2016; Zhang et al., 2017). Therefore, more and more studies have focused on using machine learning to solve problems of miRNA-disease association prediction. For instance, Xu et al. (2011) proposed a MiRNA-Target Dysregulated Network (MTDN) and constructed Support Vector Machine (SVM) classifier to identify positive miRNA-disease associations. However, since it is hard to obtain the negative miRNA-disease associations, the lack of negative samples would influence the accuracy of this method. Chen et al. (Chen and Yan, 2014) provided a method called Regularized Least Squares for MiRNA-Disease Association prediction (RLSMDA) which predicted potential disease-related miRNAs using semi-supervised learning method. RLSMDA could predict miRNAs associated with diseases without any known associated miRNAs and meanwhile it did not use negative associations between miRNAs and diseases. However, the choice of parameters for RLSMDA and the ways of combining the classifiers in different spaces together may influence prediction result to a large extent. Chen et al. (2015) further developed a computational model of Restricted Boltzmann Machine for Multiple types of MiRNA-Disease Association prediction (RBMMMDA), which can obtain both new miRNA-disease associations and their corresponding association types. Nevertheless, it is also difficult to make decision on the parameter values. Recently, Chen et al. (2017) proposed a method named Ranking-based KNN for MiRNA-Disease Association prediction (RKNNMDA) which integrated several trustable biological datasets to obtain a large data pool. However, this method may cause bias to those miRNAs that have more known associated diseases. Based on the fact that the miRNA-disease association matrix is low-rank, Li et al. (2017) presented Matrix Completion for MiRNA-Disease Association prediction (MCMDA). However, the optimal parameters of MCMDA are still in suspense.
In this study, we introduced a novel scoring method named Graph Regression for MiRNA-Disease Association prediction (GRMDA) to predict the potential miRNA-disease associations. We combined the Gaussian interaction profile kernel similarity and disease semantic similarity to get more complete integrated disease similarity. Integrated miRNA similarity was calculated in a similar way. We mapped three matrixes including miRNA-disease association matrix, integrated miRNA similarity matrix and integrated disease similarity matrix, into three graphs that were miRNA-disease association graph, miRNA similarity graph and disease similarity graph respectively. Then we synchronously applied graph regression on the three graphs, which involved three low-rank decompositions for projecting each of the three graphs into three latent spaces and two regressions between the three graphs. Finally, we got the scoring matrix by searching the minimum value of graph regression formula. Assuming that the five items of the formula are independent, we can calculate the minimum values of each item separately using Singular Value Decomposition (SVD) for low-rank decomposition and Partial Least-Squares (PLS) for graph regression. Furthermore, we used Leave-one-out cross validation (LOOCV) and five-fold cross validation to evaluate the effectiveness of GRMDA. As a result, GRMDA got an AUC of 0.8272 in LOOCV and obtained an average AUC with standard deviation of 0.8080 ± 0.0024 in five-fold cross validation. What is more, we applied three types of case studies to test the performance of GRMDA, including associated miRNA prediction for diseases based on known miRNA-disease associations from HMDD V2.0 database, for new diseases with no known related miRNAs and for the diseases based on known miRNA-disease associations from HMDD V1.0 database respectively. GRMDA performed well in the above validations and case studies, which means that GRMDA is practicable and effective in predicting potential miRNA-disease associations.
Results
Performance Evaluation
We implemented LOOCV and five-fold cross validation to evaluate the performance of GRMDA. Both of LOOCV and five-fold cross validation are implemented using the recorded miRNA-disease associations in HMDD V2.0 database. During LOOCV, each one of the known miRNA-disease associations will be left out in turn to be considered as test sample. After calculating association scores of all the miRNA-disease pairs by GRMDA, we compared the score of the test sample with all the candidate pairs including all the miRNA-disease pairs which have no known associations to observe whether the rank of the test sample was above the threshold given in advance. Moreover, we plotted the true positive rate (TPR, sensitivity) vs. the false positive rate (FPR, 1-specificity) at different thresholds to obtain the Receiver operating characteristic (ROC) curves, which were shown in Figure 1. Sensitivity means the percentage of the positive samples correctly identified among all the positives; specificity refers to the percentage of negative samples correctly identified among all the negatives. Area under the ROC curve (AUC) is calculated as an index of the prediction ability of GRMDA, the value of which is between 0 and 1. Higher the AUC is, better the prediction performance will be. If AUC is smaller than 0.5, it means that the model performs not better than random prediction. As a result, GRMDA obtained the AUC of 0.8272 in the LOOCV as shown in Figure 1. The AUCs of WBSMDA and RKNNMDA in LOOCV are 0.8030, 0.7159 respectively. Therefore, according to the LOOCV results of these methods, we can intuitively observe the improvement of predicting the miRNA-disease associations with GRMDA.
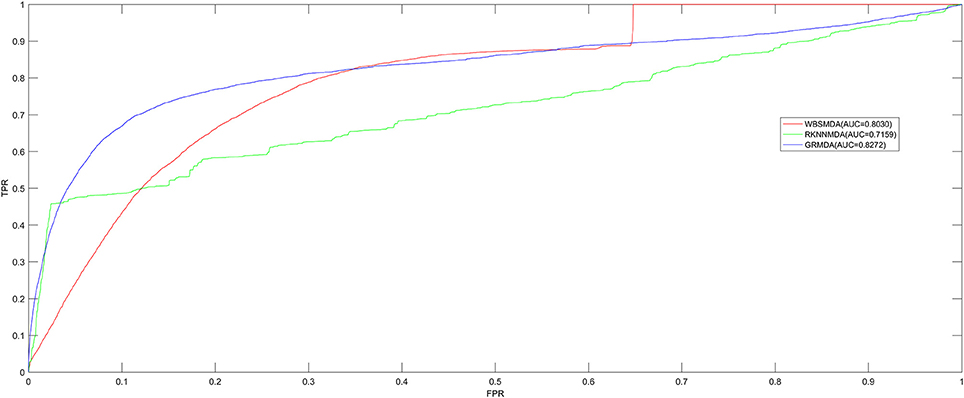
Figure 1. AUC of GRMDA in LOOCV compared with WBSMDA and RKNNMDA. As a result, GRMDA achieved AUC of 0.8272, which exceed the previous models.
During five-fold cross validation, we firstly randomly divided the known miRNA-disease associations into five parts with the same size. Then, each one of the five parts was treated as test samples in turn and the other four parts were treated as training samples. All of those miRNA-disease pairs that have no confirmed associations are candidate samples. After applying GRMDA, every score of test samples would be taken out to be compared with all the scores of candidate samples. Then we can get the rankings of test samples. In order to make the validation more accurate, we have repeated this procedure 100 times. Compared with RKNNMDA whose average AUC was 0.6723 ± 0.0027, average AUC of GRMDA in five-fold cross validation was 0.8080 ± 0.0024. The result confirmed that the GRMDA superior to RKNNMDA is able to predict miRNA-disease associations.
Case Studies
Based on two well-known miRNA-disease association databases, namely dbDEMC (Yang et al., 2010) and miR2Disease (Jiang et al., 2009), we studied Lymphoma to examine the practicability of GRMDA. In the end, we counted the number of the verified miRNAs in the top 10, top 20, and top 50 ones to evaluate the effectiveness of GRMDA.
Lymphoma is a group of blood cell tumors that develop from lymphocytes (a type of white blood cell) (Anagnostopoulos et al., 2000). According to the type of oncocyte, Lymphoma is divided into Hodgkin lymphoma (HL) and Non-Hodgkin Lymphoma (NHL) (Good and Gascoyne, 2008). About 90 percent of people who suffer Lymphoma have NHL (Alizadeh et al., 2000). Recent experimental studies showed the effect of re-expression of miRNA-150 on the formation of EBV-positive Burkitt lymphoma (Chen et al., 2013). A distinct set of five miRNAs (miR-150, miR-550, miR-124a, miR-518b, and miR-539) was shown to be differentially expressed in gastritis as opposed to MALT lymphoma (Thorns et al., 2012). After applying GRMDA method on Lymphoma, we got the result that 8 out of top 10, 17 out of top 20, and 44 out of top 50 potential miRNAs in the prediction result list for Lymphoma have been experimentally verified according to dbDEMC and miR2Disease (see Table 1). Compered with RKNNMDA and WBSMDA whose confirmed results are respectively 29 and 42 within top 50 predicted miRNAs for Lymphoma, GRMDA presents a more powerful predictive ability.
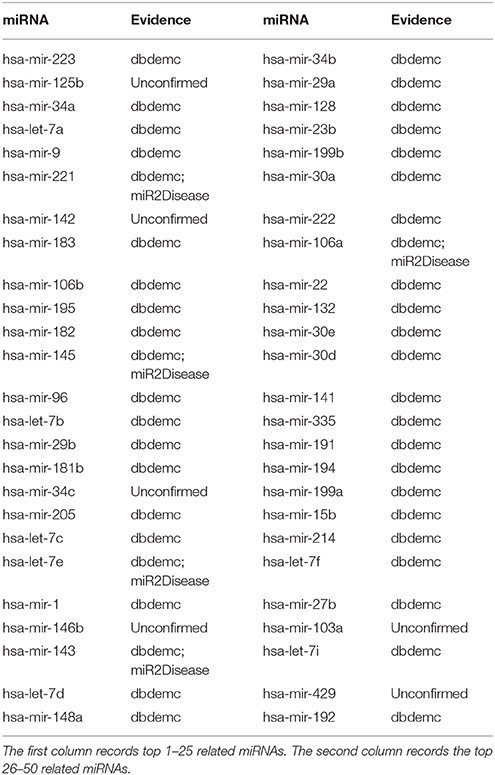
Table 1. Prediction of the top 50 predicted miRNAs associated with lymphoma based on known associations in HMDD V2.0 database.
In order to help scientists to make use of our method and predictive results more efficiently, we have provided the prediction list of the whole potential miRNAs associated with all the human diseases and their association scores predicted by GRMDA (see Supplementary Table 1).
To estimate the applicability of GRMDA on the new diseases which do not have any known associations with miRNAs, we set all of the associations which involve the test disease as unknown ones. After implementing GRMDA, we obtained the ranking of the miRNA-disease association prediction scores. We use Breast Neoplasm as an example, the predicted result of which is shown in Table 2. From the result, we can see that 10, 20, and 50 related miRNAs out of the top 10, 20, and 50 have been confirmed by at least one of the three databases HMDD, dbDEMC and miR2Disease. The result that all the top 50 associations had been confirmed means that our method has a wonderful performance in this aspect. For example, hsa-mir-302b is ranked at top 1, which exhibits high frequency genomic alternations in human Breast Neoplasm (Zhang et al., 2006).
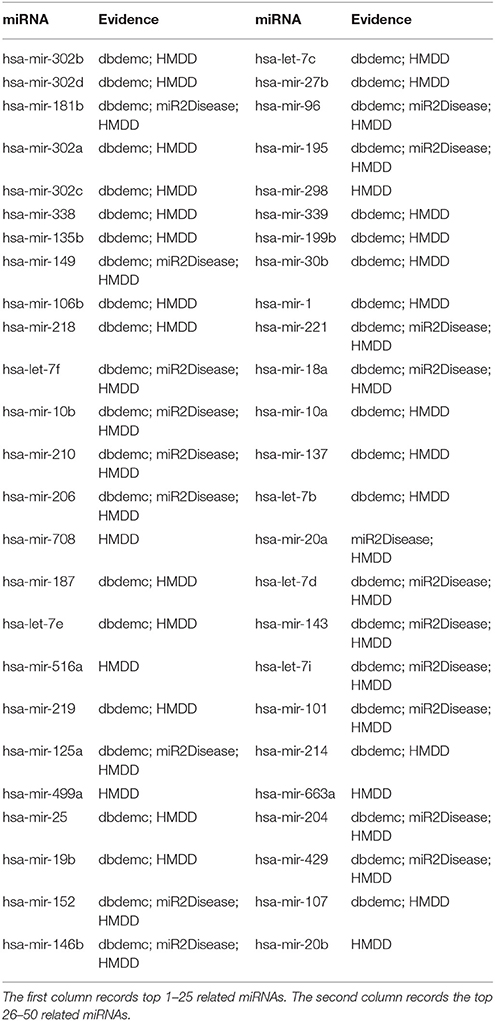
Table 2. Prediction of the top 50 predicted miRNAs associated with Breast Neoplasms based on known associations in HMDD V2.0 database by setting all of the associations which involve Breast Neoplasms as unknown ones.
Finally, we used HMDD V1.0 to test GRMDA and observe whether our method has a good robustness by observing whether our method can keep a good performance in other dataset. According to the statistical results, we can see that 7, 16, and 42 respectively out of top 10, 20, and 50 miRNAs predicted to be related to the Esophageal Neoplasms have been confirmed by three databases mentioned above (see Table 3). For example, hsa-mir-196a which ranks the second in the top 50 has been confirmed that its binding-site SNP (rs6573) can regulate RAP1A expression, which contributes to the risk and metastasis of esophageal squamous cell carcinoma (Wang et al., 2012).
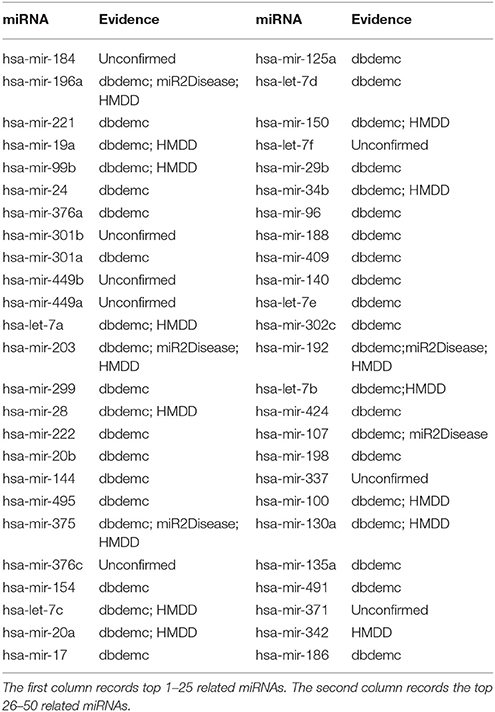
Table 3. Prediction of the top 50 predicted miRNAs associated with Esophageal Neoplasms based on known associations in HMDD V1.0 database.
In conclusion, the prediction performance of GRMDA is satisfactory. Because of that, we can foresee that with the development of experimental tools and the improvement of experimental measures, more and more miRNA-disease associations predicted by our method will be confirmed in the medical laboratory.
Discussion
We introduced GRMDA in this paper, which was based on graph regression and similarity computational methods that integrates Gaussian interaction profile kernel similarity and disease semantic similarity or miRNA functional similarity. Because we introduced Gaussian interaction profile kernel similarity, the information of the disease similarity and the miRNA similarity was fully excavated to improve the accuracy of the prediction. To verify the accuracy of the GRMDA, we used LOOCV and five-fold cross validation and three case studies of human complex diseases. GRMDA has a good performance in all the above validations and case studies.
Here are the reasons why GRMDA has better performance than some previous methods. First of all, the miRNA similarity matrix and disease similarity matrix in GRMDA can take full advantage of the information from known miRNA-disease associations by introducing the Gaussian interaction profile kernel similarity, which means that miRNA-disease association matrix also takes part in the building of the above two similarity matrixes. In that way, GRMDA makes full use of the assumption that if two miRNAs affect the same disease, they tend to be similar (It is the same way for disease). Secondly, GRMDA applies Singular Value Decomposition (SVD) and Partial Least Squares Regression (PLS) during the graph regression to decompose a series of matrixes including association matrix, miRNA similarity matrix and disease similarity matrix. SVD and PLS are two modified forms of Principle Component Analysis (PCA) to collapse multidimensional data into low-dimension, which reconstruct the information of the original dataset with reduced components represented with vectors in latent spaces (Giuliani, 2017). In that way, our method can omit the less important attributes to avoid noise and pay attention to the more important attributes. For example, we will get three matrix U, Σ, and VT after applying SVD on similarity matrix. Since Σ is a diagonal matrix in which every value represents the weight of an attribute about how significant the attribute affects the similarity between two miRNAs or two diseases, those small values can be abandoned to retain the most important attributes in the miRNA or disease similarity space. In the process of PLS, the principle components of latent association matrix and latent similarity matrix are extracted sequentially and then regressions are constructed between them with considering maximum correlation. In the end, because of our way of utilizing miRNA similarity matrix and disease similarity matrix, GRMDA could predict miRNAs for diseases with no one known related miRNA and predict diseases for miRNAs not related to any diseases, overcoming the limitations of some previous computational models.
GRMDA also has its weakness. Firstly, though current studies benefit from the increased known data, it is never a finished work to expand data which means our prediction is always under a data-lacking condition. Secondly, although GRMDA has an improvement in accuracy compared with other methods, the improvement is not enough. What's more, there are some difficulties in choosing parameters in SVD and PLS according to the size of the matrixes.
Materials and Methods
Human MiRNA-Disease Associations
We downloaded data about human miRNA-disease associations between 383 diseases and 495 miRNAs from HMDD V2.0 database, which includes up to 5430 associations. The miRNA-disease association matrix A was built, where the entity A(i,j) will be 1 if miRNA m(i) and disease d(j) compose an association, otherwise 0. Variables nm and nd denote the number of miRNAs and diseases respectively.
MiRNA Functional Similarity
The assumption that miRNAs with functional similarity tend to be associated with diseases that have phenotypical similarity is our basis to calculate the functional similarity score between miRNAs (Wang et al., 2010). Combining known miRNA-disease associations and disease similarity, the functional similarity between two miRNAs could be obtained through measuring the similarity between two disease sets that are related to the two miRNAs. The data came from http://www.cuilab.cn/files/images/cuilab/misim.zip, according to which we constructed the matrix FS to represent miRNA functional similarity, in which the entity FS(m(i), m(j)) denotes the functional similarity score between miRNAs m(i) and m(j) with value from 0 to 1.
Disease Semantic Similarity Model 1
We use Directed Acyclic Graph (DAG) whose descriptor is DAG(D) = (D,T(D),E(D)) to represent each disease, in which T(D) is the node set composed of node D itself and its ancestor nodes, E(D) is the edge set consisting of the direct edges from parent nodes to child nodes (Wang et al., 2010). The formula to calculate the semantic value of disease D is shown as below:
Where Δ is the semantic contribution factor, whose value is between 0 and 1. For example, for a certain disease D, it contributes to the semantic value of itself with a value of 1. The farther the distance is from disease D to the disease d in T(D), the less the semantic contribution of d to D will be. Moreover, contributions from diseases in the same layer to the semantic value of disease D would be equal. The way to calculate semantic similarity between disease d (i) and d (j) comes from a reliable assumption that the larger part of the sharing of DAGs of two diseases, the larger the semantic similarity between them will be. The formula shown below is the semantic similarity between disease d(i) and d(j):
Disease Semantic Similarity Model 2
In this section, we calculated the disease semantic similarity following the method given in the reference (Xuan et al., 2013). The method in Disease semantic similarity model 1 has a good performance, however, it has a weakness. For example, if different diseases d1and d2are in the same layer of DAG(D), then as a result of disease semantic similarity model 1 defined in the section above, d1and d2 have the same contribution to the semantic value of disease D. But in certain circumstances, d1 may appear in less disease DAGs than d2. If that happens, it is easy to realize that d1 is more specific than d2 and should have a higher contribution to the semantic value of D. Therefore, we defined the contribution of disease t in DAG(D) to the semantic value of disease D as follows:
The semantic value of disease D in model 2 is calculated in the similar way as in equation (2). The way to calculate semantic similarity between disease d(i) and d(j) also has same formation with method 1. The formulas are shown below:
SS2 is the disease semantic similarity matrix calculated based on model 2 and its entity SS2(d(i),d(j)) in row i column j is the disease semantic similarity between disease d(i) and d(j) based on disease semantic similarity model 2.
Gaussian Interaction Profile Kernel Similarity for Diseases
It is observed that functional similar miRNAs always tend to be associated with similar diseases. Based on this observation, we could utilize the topologic information extracted from the known miRNA-disease association network to compute the Gaussian interaction profile kernel similarity for diseases. First, we defined a binary vector IP(d(i)), the same value as the ith column of our miRNA-disease association matrix A, to represent the interaction profiles of disease d(i). The formula to calculate Gaussian interaction profile kernel similarity between disease d(i) and d(j) was shown below:
The effect of parameter γd is to control the kernel bandwidth. is usually set as 1. γd is calculated by normalizing by the average number of known miRNA-disease associations for all diseases. KD is the Gaussian interaction profile kernel similarity matrix for diseases.
Gaussian Interaction Profile Kernel Similarity for miRNAs
MiRNA Gaussian interaction profile kernel similarity matrix is calculated in a similar way with disease Gaussian interaction profile kernel similarity:
IP(m(i)) has the same value with the ith row of our miRNA-disease association matrix A to denote the interaction profiles of miRNA m(i).
Integrated Similarity for miRNAs and Diseases
In this work, the integrated disease similarity Sd were constructed based on disease semantic similarity SS and Gaussian interaction profile kernel similarity KD. Specifically, if disease d(i) and d(j) have semantic similarity, for simplicity, we assume that two types of semantic similarities between them are equally important. Then the final integrated similarity is computed directly using the average of SS1(d(i),d(j)) and SS2(d(i),d(j)), since both of two types of disease semantic similarity are calculated based on Directed Acyclic Graph (DAG) of diseases. Otherwise, if disease d(i) and d(j) do not have any semantic similarity, the integrated disease similarity equals to the Gaussian interaction profile kernel similarity which is taken as a supplement to the semantic similarity. The formula was shown as follows:
Furthermore, by supplementing the miRNA functional similarity with miRNA Gaussian interaction profile kernel similarity, we obtained the integrated miRNA similarity as follows:
GRMDA
We use a graph regression (Hu et al., 2015) among Gr, Gd, and Ga which represent graphs about miRNA similarity network, disease similarity network and miRNA-disease association network respectively, to predict unknown associations between miRNAs and diseases (see Figure 2). Because the graph regression is synchronously performed in miRNA similarity space, disease similarity space and miRNA-disease association space, we can get the following formula:
The first three items in formula (13) denote three low-rank decompositions to map Gr, Gd and Ga in three spaces respectively. The first item helps to decompose A into two parts, each part represents the information of Ga in miRNA or disease aspect. The second item is used to convert Gr to feature matrix about miRNA and the third item is used in the same way for generating feature matrix about disease. The fourth item means a regression between miRNA-disease association space and miRNA similarity space, from which we can get the regression matrix which can connect Ga and Gr. The fifth item represents a regression between miRNA-disease association space and disease similarity space, from which we can get the regression matrix which can connect Ga and Gd. To be specific, we map the miRNAs and diseases in Ga into an nm × r miRNA associating matrix Ar and a nd × r disease associating matrix Ad respectively. We map miRNAs in Gr into an nm × p miRNA latent feature matrix Fr. We map diseases in Gdinto a nd × q disease latent feature matrix Fd. In the end, the p × r matrix Br and the q × r matrix Bd are the corresponding regression coefficient matrices.
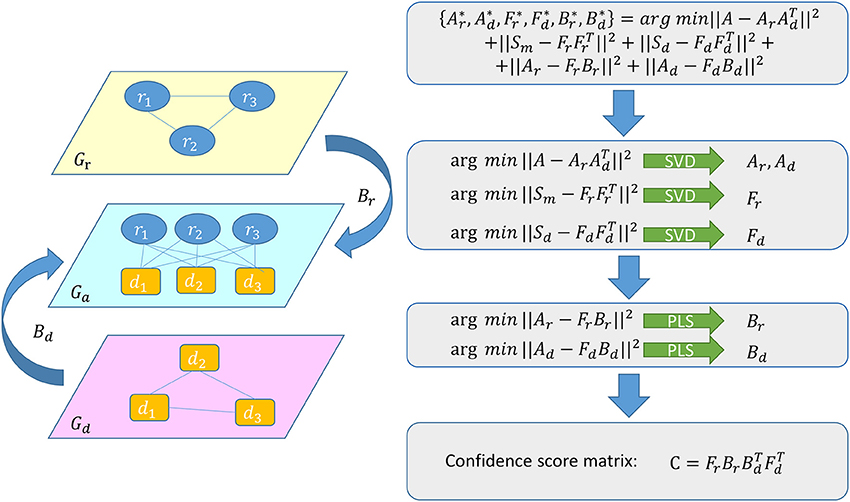
Figure 2. Flowchart of GRMDA model to predict the potential miRNA-disease associations based on the known associations in HMDD V2.0 database.
It is an intricate problem to minimize the objective function as a whole. However, since our goal is to make regression between the latent association spaces and similarity spaces, it is reasonable to divide the solution into two steps: obtaining the latent matrices and regressing between the latent matrices. To make the question easier, we assume that the five items in the formula (13) are independent. Then we can easily solve the above optimization problem by minimizing the items individually. We applied SVD for low-rank decompositions to generate Ar, Ad, Fr, and Fd respectively in the following way:
SVD is an important and widely used method to decompose matrixes. SVD can condense the size of data and extract the possible association attributes between what the column and row represent. Σ is a diagonal matrix in which each value on the diagonal represents the importance of its mapped attribute. We can omit some attributes whose value is too small in Σ. However, if too small number of attributes are selected, the subtle information may be lost (Sharma, 2016). In this work, according to the particular data structure, we have retained about 45% (Franceschini et al., 2016) components for disease similarity space and miRNA similarity space. As a result, the parameters were set as q = 170 and p = 220 respectively. And for association space, the number of selected attributes was moderately set as r = 180. We operated a canonical correlation analysis on the principal components of miRNA latent feature matrix Fr and miRNA associating matrix Ar, as well as disease latent feature matrix Fd and disease associating matrix Ad respectively to check for the mutual correlation between them. The results were shown in Supplementary Figures 1, 2. After that we utilized PLS regression on the latter two items in formula (13) to generate Br and Bd individually. For the purpose to preserve the predictive ability with small noise in PLS (Kreeger, 2013), the percentage of components to keep was set as 90% both for regression between association space and miRNA similarity space and regression between association space and disease similarity space.
According to the previous formulas, we know that Fr represents the features of miRNA, Fd represents the features of diseases, Br represents the relation between A and Fr, Bd represents the relation between A and Fd. Then, builds a bridge between the features of miRNAs, the features of diseases and the associations between them. In the end, the confidence scores of miRNA-disease pairs to be potential associations are calculated in the following formula:
where, C is the confidence score matrix and C(i,j) represents the associations core of miRNA m(i) and disease d(j). The higher the score is, the more likely the association exists.
Author Contributions
XC conceived the project, developed the prediction method, designed the experiments, analyzed the result, and wrote the paper. J-RY implemented the experiments, analyzed the result, and wrote the paper. N-NG analyzed the result and revised the paper. J-QL analyzed the result. All authors read and approved the final manuscript.
Conflict of Interest Statement
The authors declare that the research was conducted in the absence of any commercial or financial relationships that could be construed as a potential conflict of interest.
Acknowledgments
XC was supported by National Natural Science Foundation of China under Grant Nos. 61772531 and 11631014.
Supplementary Material
The Supplementary Material for this article can be found online at: https://www.frontiersin.org/articles/10.3389/fphys.2018.00092/full#supplementary-material
References
Alizadeh, A. A., Eisen, M. B., Davis, R. E., Ma, C., Lossos, I. S., Rosenwald, A., et al. (2000). Distinct types of diffuse large B-cell lymphoma identified by gene expression profiling. Nature 403, 503–511. doi: 10.1038/35000501
Ambros, V. (2001). microRNAs: tiny regulators with great potential. Cell 107, 823–826. doi: 10.1016/S0092-8674(01)00616-X
Anagnostopoulos, I., Hansmann, M. L., Franssila, K., Harris, M., Harris, N. L., Jaffe, E. S., et al. (2000). European task force on Lymphoma project on lymphocyte predominance Hodgkin disease: histologic and immunohistologic analysis of submitted cases reveals 2 types of Hodgkin disease with a nodular growth pattern and abundant lymphocytes. Blood 96, 1889–1899. Available online at: http://www.bloodjournal.org/content/96/5/1889
Bartel, D. P. (2004). MicroRNAs: genomics, biogenesis, mechanism, and function. Cell 116, 281–297. doi: 10.1016/S0092-8674(04)00045-5
Bartel, D. P. (2009). MicroRNAs: target recognition and regulatory functions. Cell 136, 215–233. doi: 10.1016/j.cell.2009.01.002
Chen, S., Wang, Z., Dai, X., Pan, J., Ge, J., Han, X., et al. (2013). Re-expression of microRNA-150 induces EBV-positive Burkitt lymphoma differentiation by modulating c-Myb in vitro. Cancer Sci. 104, 826–834. doi: 10.1111/cas.12156
Chen, X. (2016). miREFRWR: a novel disease-related microRNA-environmental factor interactions prediction method. Mol. Biosyst. 12, 624–633. doi: 10.1039/C5MB00697J
Chen, X., Liu, M. X., Cui, Q. H., and Yan, G. Y. (2012a). Prediction of disease-related interactions between microRNAs and environmental factors based on a semi-supervised classifier. PLoS ONE 7:e43425. doi: 10.1371/journal.pone.0043425
Chen, X., Liu, M. X., and Yan, G. Y. (2012b). RWRMDA: predicting novel human microRNA-disease associations. Mol. Biosyst. 8, 2792–2798. doi: 10.1039/c2mb25180a
Chen, X., Ren, B., Chen, M., Wang, Q., Zhang, L., and Yan, G. (2016a). NLLSS: predicting synergistic drug combinations based on semi-supervised learning. PLoS Comput. Biol. 12:e1004975. doi: 10.1371/journal.pcbi.1004975
Chen, X., Wu, Q. F., and Yan, G. Y. (2017). RKNNMDA: Ranking-based KNN for MiRNA-disease association prediction. RNA Biol. 14, 952–962. doi: 10.1080/15476286.2017.1312226
Chen, X., Yan, C. C., Zhang, X., Li, Z., Deng, L., Zhang, Y., et al. (2015). RBMMMDA: predicting multiple types of disease-microRNA associations. Sci. Rep. 5:13877. doi: 10.1038/srep13877
Chen, X., Yan, C. C., Zhang, X., You, Z. H., Deng, L., Liu, Y., et al. (2016b). WBSMDA: within and between score for MiRNA-disease association prediction. Sci. Rep. 6:21106. doi: 10.1038/srep21106
Chen, X., Yan, C. C., Zhang, X., You, Z. H., Huang, Y. A., and Yan, G. Y. (2016c). HGIMDA: Heterogeneous graph inference for miRNA-disease association prediction. Oncotarget 7, 65257–65269. doi: 10.18632/oncotarget.11251
Chen, X., Yan, C. C., Zhang, X., Zhang, X., Dai, F., Yin, J., et al. (2016d). Drug-target interaction prediction: databases, web servers and computational models. Brief Bioinform. 17, 696–712. doi: 10.1093/bib/bbv066
Chen, X., and Yan, G. Y. (2014). Semi-supervised learning for potential human microRNA-disease associations inference. Sci. Rep. 4:5501. doi: 10.1038/srep05501
Cheng, A. M., Byrom, M. W., Shelton, J., and Ford, L. P. (2005). Antisense inhibition of human miRNAs and indications for an involvement of miRNA in cell growth and apoptosis. Nucleic Acids Res. 33, 1290–1297. doi: 10.1093/nar/gki200
Franceschini, A., Lin, J., von Mering, C., and Jensen, L. J. (2016). SVD-phy: improved prediction of protein functional associations through singular value decomposition of phylogenetic profiles. Bioinformatics 32, 1085–1087. doi: 10.1093/bioinformatics/btv696
Giuliani, A. (2017). The application of principal component analysis to drug discovery and biomedical data. Drug Discov. Today 22, 1069–1076. doi: 10.1016/j.drudis.2017.01.005
Good, D. J., and Gascoyne, R. D. (2008). Classification of non-Hodgkin's lymphoma. Hematol. Oncol. Clin. North Am. 22, 781–805. doi: 10.1016/j.hoc.2008.07.008
Griffiths-Jones, S., Grocock, R. J., van Dongen, S., Bateman, A., and Enright, A. J. (2006). miRBase: microRNA sequences, targets and gene nomenclature. Nucleic Acids Res. 34, D140–D144. doi: 10.1093/nar/gkj112
Hu, C., Cheng, L., Sepulcre, J., Johnson, K. A., Fakhri, G. E., Lu, Y. M., et al. (2015). A spectral graph regression model for learning brain connectivity of Alzheimer's disease. PLoS ONE 10:e0128136. doi: 10.1371/journal.pone.0128136
Huang, Y. A., You, Z. H., Chen, X., Chan, K., and Luo, X. (2016). Sequence-based prediction of protein-protein interactions using weighted sparse representation model combined with global encoding. BMC Bioinformatics 17:184. doi: 10.1186/s12859-016-1035-4
Jiang, Q., Hao, Y., Wang, G., Juan, L., Zhang, T., Teng, M., et al. (2010). Prioritization of disease microRNAs through a human phenome-microRNAome network. BMC Syst. Biol. 4(Suppl. 1):S2. doi: 10.1186/1752-0509-4-S1-S2
Jiang, Q., Wang, Y., Hao, Y., Juan, L., Teng, M., Zhang, X., et al. (2009). miR2Disease: a manually curated database for microRNA deregulation in human disease. Nucleic Acids Res. 37, D98–D104. doi: 10.1093/nar/gkn714
Jopling, C. L., Yi, M., Lancaster, A. M., Lemon, S. M., and Sarnow, P. (2005). Modulation of hepatitis C virus RNA abundance by a liver-specific MicroRNA. Science 309, 1577–1581. doi: 10.1126/science.1113329
Karp, X., and Ambros, V. (2005). Developmental biology. Encountering microRNAs in cell fate signaling. Science 310, 1288–1289. doi: 10.1126/science.1121566
Kim, S. Y., Lee, Y. H., and Bae, Y. S. (2012). miR-186, miR-216b, miR-337-3p, and miR-760 cooperatively induce cellular senescence by targeting alpha subunit of protein kinase CKII in human colorectal cancer cells. Biochem. Biophys. Res. Commun. 429, 173–179. doi: 10.1016/j.bbrc.2012.10.117
Kozomara, A., and Griffiths-Jones, S. (2011). miRBase: integrating microRNA annotation and deep-sequencing data. Nucleic Acids Res. 39, D152–D157. doi: 10.1093/nar/gkq1027
Kreeger, P. K. (2013). Using partial least squares regression to analyze cellular response data. Sci. Signal. 6:tr7. doi: 10.1126/scisignal.2003849
Lagos-Quintana, M., Rauhut, R., Lendeckel, W., and Tuschl, T. (2001). Identification of novel genes coding for small expressed RNAs. Science 294, 853–858. doi: 10.1126/science.1064921
Lau, N. C., Lim, L. P., Weinstein, E. G., and Bartel, D. P. (2001). An abundant class of tiny RNAs with probable regulatory roles in Caenorhabditis elegans. Science 294, 858–862. doi: 10.1126/science.1065062
Lee, R. C., and Ambros, V. (2001). An extensive class of small RNAs in Caenorhabditis elegans. Science 294, 862–864. doi: 10.1126/science.1065329
Lee, R. C., Feinbaum, R. L., and Ambros, V. (1993). The, C. elegans heterochronic gene lin-4 encodes small RNAs with antisense complementarity to lin-14. Cell 75, 843–854. doi: 10.1016/0092-8674(93)90529-Y
Li, J. Q., Rong, Z. H., Chen, X., Yan, G. Y., and You, Z. H. (2017). MCMDA: Matrix completion for MiRNA-disease association prediction. Oncotarget 8, 21187–21199. doi: 10.18632/oncotarget.15061
Lim, Y. Y., Wright, J. A., Attema, J. L., Gregory, P. A., Bert, A. G., Smith, E., et al. (2013). Epigenetic modulation of the miR-200 family is associated with transition to a breast cancer stem-cell-like state. J. Cell. Sci. 126, 2256–2266. doi: 10.1242/jcs.122275
Lynam-Lennon, N., Maher, S. G., and Reynolds, J. V. (2009). The roles of microRNA in cancer and apoptosis. Biol. Rev. Camb. Philos. Soc. 84, 55–71. doi: 10.1111/j.1469-185X.2008.00061.x
Meister, G., and Tuschl, T. (2004). Mechanisms of gene silencing by double-stranded RNA. Nature 431, 343–349. doi: 10.1038/nature02873
Meola, N., Gennarino, V. A., and Banfi, S. (2009). microRNAs and genetic diseases. Pathogenetics 2:7. doi: 10.1186/1755-8417-2-7
Miska, E. (2005). How microRNAs control cell division, differentiation and death. Curr. Opin. Genet. Dev. 15, 563–568. doi: 10.1016/j.gde.2005.08.005
Mørk, S., Pletscher-Frankild, S., Palleja Caro, A., Gorodkin, J., and Jensen, L. J. (2014). Protein-driven inference of miRNA-disease associations. Bioinformatics 30, 392–397. doi: 10.1093/bioinformatics/btt677
Pasquier, C., and Gardès, J. (2016). Prediction of miRNA-disease associations with a vector space model. Sci. Rep. 6:27036. doi: 10.1038/srep27036
Pasquinelli, A. E., Reinhart, B. J., Slack, F., Martindale, M. Q., Kuroda, M. I., Maller, B., et al. (2000). Conservation of the sequence and temporal expression of let-7 heterochronic regulatory RNA. Nature 408, 86–89. doi: 10.1038/35040556
Reinhart, B. J., Slack, F. J., Basson, M., Pasquinelli, A. E., Bettinger, J. C., Rougvie, A. E., et al. (2000). The 21-nucleotide let-7 RNA regulates developmental timing in Caenorhabditis elegans. Nature 403, 901–906. doi: 10.1038/35002607
Sharma, L. N. (2016). Information theoretic multiscale truncated SVD for multilead electrocardiogram. Comput. Methods Programs Biomed. 129, 109–116. doi: 10.1016/j.cmpb.2016.01.010
Shi, H., Xu, J., Zhang, G., Xu, L., Li, C., Wang, L., et al. (2013). Walking the interactome to identify human miRNA-disease associations through the functional link between miRNA targets and disease genes. BMC Syst. Biol. 7:101. doi: 10.1186/1752-0509-7-101
Skalsky, R. L., and Cullen, B. R. (2011). Reduced expression of brain-enriched microRNAs in glioblastomas permits targeted regulation of a cell death gene. PLoS ONE 6:e24248. doi: 10.1371/journal.pone.0024248
Thorns, C., Kuba, J., Bernard, V., Senft, A., Szymczak, S., Feller, A. C., et al. (2012). Deregulation of a distinct set of microRNAs is associated with transformation of gastritis into MALT lymphoma. Virchows Arch. 460, 371–377. doi: 10.1007/s00428-012-1215-1
Wang, D., Wang, J., Lu, M., Song, F., and Cui, Q. (2010). Inferring the human microRNA functional similarity and functional network based on microRNA-associated diseases. Bioinformatics 26, 1644–1650. doi: 10.1093/bioinformatics/btq241
Wang, K., Li, J., Guo, H., Xu, X., Xiong, G., Guan, X., et al. (2012). MiR-196a binding-site SNP regulates RAP1A expression contributing to esophageal squamous cell carcinoma risk and metastasis. Carcinogenesis 33, 2147–2154. doi: 10.1093/carcin/bgs259
Wightman, B., Ha, I., and Ruvkun, G. (1993). Posttranscriptional regulation of the heterochronic gene lin-14 by lin-4 mediates temporal pattern formation in C. elegans. Cell 75, 855–862. doi: 10.1016/0092-8674(93)90530-4
Xu, C., Ping, Y., Li, X., Zhao, H., Wang, L., Fan, H., et al. (2014). Prioritizing candidate disease miRNAs by integrating phenotype associations of multiple diseases with matched miRNA and mRNA expression profiles. Mol. Biosyst. 10, 2800–2809. doi: 10.1039/C4MB00353E
Xu, J., Li, C. X., Lv, J. Y., Li, Y. S., Xiao, Y., Shao, T. T., et al. (2011). Prioritizing candidate disease miRNAs by topological features in the miRNA target-dysregulated network: case study of prostate cancer. Mol. Cancer Ther. 10, 1857–1866. doi: 10.1158/1535-7163.MCT-11-0055
Xuan, P., Han, K., Guo, M., Guo, Y., Li, J., Ding, J., et al. (2013). Prediction of microRNAs associated with human diseases based on weighted k most similar neighbors. PLoS ONE 8:e70204. doi: 10.1371/annotation/a076115e-dd8c-4da7-989d-c1174a8cd31e
Yang, Z., Ren, F., Liu, C., He, S., Sun, G., Gao, Q., et al. (2010). dbDEMC: a database of differentially expressed miRNAs in human cancers. BMC Genomics 11(Suppl. 4):S5. doi: 10.1186/1471-2164-11-S4-S5
You, Z. H., Huang, Z. A., Zhu, Z., Yan, G. Y., Li, Z. W., Wen, Z., et al. (2017). PBMDA: a novel and effective path-based computational model for miRNA-disease association prediction. PLoS Comput. Biol. 13:e1005455. doi: 10.1371/journal.pcbi.1005455
Zeng, X., Zhang, X., and Zou, Q. (2016). Integrative approaches for predicting microRNA function and prioritizing disease-related microRNA using biological interaction networks. Brief Bioinform. 17, 193–203. doi: 10.1093/bib/bbv033
Zhang, L., Ai, H., Chen, W., Yin, Z., Hu, H., Zhu, J., et al. (2017). CarcinoPred-EL: novel models for predicting the carcinogenicity of chemicals using molecular fingerprints and ensemble learning methods. Sci. Rep. 7:2118. doi: 10.1038/s41598-017-02365-0
Keywords: microRNA, disease, association prediction, graph regression, matrix factorization
Citation: Chen X, Yang J-R, Guan N-N and Li J-Q (2018) GRMDA: Graph Regression for MiRNA-Disease Association Prediction. Front. Physiol. 9:92. doi: 10.3389/fphys.2018.00092
Received: 07 October 2017; Accepted: 26 January 2018;
Published: 20 February 2018.
Edited by:
Jiarui Wu, Shanghai Institutes for Biological Sciences (CAS), ChinaReviewed by:
Alessandro Giuliani, Istituto Superiore di Sanità, ItalyHaoran Zheng, University of Science and Technology of China, China
Copyright © 2018 Chen, Yang, Guan and Li. This is an open-access article distributed under the terms of the Creative Commons Attribution License (CC BY). The use, distribution or reproduction in other forums is permitted, provided the original author(s) and the copyright owner are credited and that the original publication in this journal is cited, in accordance with accepted academic practice. No use, distribution or reproduction is permitted which does not comply with these terms.
*Correspondence: Xing Chen, eGluZ2NoZW5AYW1zcy5hYy5jbg==