- 1Laboratory of Genetics, The Rockefeller University, New York, NY, United States
- 2Department of Biology, Center for Biological Clocks Research, Texas A&M University, College Station, United States
Genome-wide profiling of rhythmic gene expression has offered new avenues for studying the contribution of circadian clock to diverse biological processes. Sleep has been considered one of the most important physiological processes that are regulated by the circadian clock, however, the effects of chronic sleep loss on rhythmic gene expression remain poorly understood. In the present study, we exploited Drosophila sleep mutants insomniac1 (inc1) and wide awakeD2 (wakeD2) as models for chronic sleep loss. We profiled the transcriptomes of head tissues collected from 4-week-old wild type flies, inc1 and wakeD2 at timepoints around the clock. Analysis of gene oscillation revealed a substantial loss of rhythmicity in inc1 and wakeD2 compared to wild type flies, with most of the affected genes common to both mutants. The disruption of gene oscillation was not due to changes in average gene expression levels. We also identified a subset of genes whose loss of rhythmicity was shared among animals with chronic sleep loss and old flies, suggesting a contribution of aging to chronic, sleep-loss-induced disruption of gene oscillation.
Introduction
Many genes exhibit rhythmic expression patterns and are regulated by an internal timing system, the circadian clock. In animals, the circadian clock, regulated by a transcription-translation feedback loop, controls networks of genes oscillating with ∼24-hour periodicity. These in turn influence rhythmic aspects of animal physiology and behavior, most prominently, sleep (Rijo-Ferreira and Takahashi, 2019).
Chronic disruptions of sleep and circadian rhythm are intertwined processes: Sleep, which is considered to be regulated by both a homeostatic and a circadian process (Borbely, 1982), is directly influenced by the circadian clock. However, sleep deprivation not only changes subsequent depth of sleep, but also alters light responsiveness and phase shifting property of the circadian clock (Challet et al., 2001; Mistlberger et al., 2002; Burgess, 2010; van Diepen et al., 2014; Jha et al., 2017). Only a handful of investigations have asked how sleep affects the circadian clock. These studies have demonstrated correlations between the electrical activity of neurons composing the suprachiasmatic nucleus (SCN), the mammalian central clock, and depth of sleep or sleep pressure (Deboer et al., 2003; Deboer et al., 2007; Schmidt et al., 2009). Sleep pressure was also found to attenuate phase shifts induced by light in mice, hamsters and humans (Challet et al., 2001; Mistlberger et al., 2002; Burgess, 2010). These physiological and behavioral effects of sleep on the circadian clock were mostly examined using sleep restriction, caffeine treatment or acute sleep deprivation experiments. How chronic sleep disruption affects rhythmicity remains poorly documented, particularly, on the level of oscillating gene expression.
In mice and primates, respectively ∼40% and ∼80% of all protein-coding genes exhibit daily rhythmic expression (Zhang et al., 2014; Mure et al., 2018). In Drosophila, micro-array and RNA-sequencing methods have identified several hundred transcripts whose oscillating properties are regulated by period, the first identified clock gene (Claridge-Chang et al., 2001; McDonald and Rosbash, 2001; Hughes et al., 2012). Drosophila has been established as an exceptional model for studying the genetic basis of sleep regulation (Shafer and Keene, 2021). Similar to the mammalian brain, spontaneous sleep, spontaneous wakefulness and sleep deprivation in Drosophila all directly modulate gene expression (Cirelli et al., 2005). Drosophila sleep mutants can serve as an efficient and inexpensive method to achieve chronic sleep disruption in a large number of animals for profiling rhythmic gene expression. Here we report a substantial loss of rhythmicity in oscillating gene expression in young adult animals that harbor mutations that chronically disrupt sleep. A subset of these genes similarly lost rhythmicity in aged flies.
Materials and methods
Drosophila strains and culture
Drosophila melanogaster stocks were raised on standard media (cornmeal/yeast/molasses/agar) at 25 °C under 12-hour light/12-hour dark (LD) cycles. Wild type isogenic strain Canton-S w1118 (iso1CJ) (Li et al., 2013a), referred to as WT, was used as the control for behavioral experiments and RNA-seq experiments. Sleep mutant strain inc1 (Stavropoulos and Young, 2011) was a standing stock of the laboratory. Sleep mutant strain wakeD2 (Liu et al., 2014) was kindly provided by Dr. Mark Wu. Both mutant strains were backcrossed to the wild type strain Canton-S w1118 (iso1CJ) for at least 5 generations.
Sleep measurement and analysis
Locomotor activity of flies was collected using the Drosophila Activity Monitor (DAM) system (TriKinetics, Waltham, MA). Flies were singly housed in glass tubes containing fly culture food and assayed at 25°C under LD cycles. Activity counts were collected at 1-min bins for 5 LD cycles immediately after the loading day. DAM monitor files were processed with pySolo software (Gilestro and Cirelli, 2009). Sleep was determined as at least 5 min of inactivity. Sleep duration and sleep bouts were analyzed with pySolo software.
For sleep measurements across the lifespan of control and mutant flies, flies were housed and aged in food bottles, with males and females housed together in each bottle. Flies were flipped every week into fresh food bottles. Batches of male flies were loaded into the DAM system every 5 days at designated ages and sleep was measured over 5 consecutive days. All flies used in Figure 1 were born around the same time and aged the same way. Each data point was collected from flies raised and aged in the same bottles.
Longevity assay
Parent flies were raised on standard media for 2–3 days and then removed. Offspring were collected within 24 h after eclosion and were raised in LD cycles at 25°C for 2 days. Subsequently, 15 male and 15 female animals were placed into each individual food vial, and the vials were placed in LD cycles at 25 °C. Animals were transferred to new food vials every 1–3 days and the number of dead animals was recorded. dLife software was used to analyze longevity data (Linford et al., 2013). Log-rank tests were performed to determine statistical significance. Flies that escaped during transfer were included in the analysis as right-censored events.
RNA extraction
Heads of about 200 flies of mixed sexes were collected at designated age and time. For RNA-seq experiments, four groups of samples were collected, WT at Day 27 as control group, inc1 at Day 27, wakeD2 at Day 27, and WT-old at Day 49. Samples were collected every 4 h over 2 days of LD cycles, yielding 12 timepoints per genotype. Total RNA was extracted using TRIzol (Invitrogen) reagents and homogenized using a BeadBug microtube homogenizer (Benchmark Scientific). Samples were further extracted using chloroform and the aqueous phase containing nucleic acids was harvested. RNeasy Mini Kit (Qiagen) was used to remove DNA with DNase and further purify the samples, according to the manufacturer’s protocol.
RNA sequencing
RNA-seq was conducted at the Genomics Resource Center of the Rockefeller University. Sequencing libraries were prepared with the Illumina TruSeq stranded mRNA LT kit. 100 ng of total RNA for each sample was used. Libraries were multiplexed and sequenced on the Illumina NextSeq 500 sequencer using high output V2 reagents and NextSeq Control Software v1.4 to generate 75bp single reads, following manufacturer’s protocol. The sequencing depth was about 25 million reads per sample. Reads were aligned to the D. melanogaster reference genome assembly (Release 6.13) with STAR and read counts were generated with featureCounts (dos Santos et al., 2015; Dobin et al., 2013; Liao et al., 2014). Genes with cpm values less than 1 in more than 28 samples in the entire dataset were considered as low expression and were removed from further analysis. Pearson’s correlations between samples were calculated using package in R. PCA was performed using module implemented in DESeq2 (Love et al., 2014).
Differential gene expression analysis
DGE analysis was performed with DESeq2 (Love et al., 2014) and edgeR (Robinson et al., 2010; McCarthy et al., 2012) with recommended parameter settings for time series analysis. Specifically, likelihood ratio test (LRT) was used in DESeq2 and generalized linear model (GLM) was used in edgeR. Differentially expressed genes were selected with significance cutoffs of BH adjusted p < 0.05 for DESeq2 and p < 0.05 for edgeR. Gene ontology (GO) analysis was performed using the Database for Annotation, Visualization, and Integrated Discovery (DAVID) (Huang da et al., 2009a; Huang da et al., 2009b).
Circadian analysis
Rhythmically expressed genes were identified using ARSER (Yang and Su, 2010), JTK cycle (Hughes et al., 2010), and GeneCycle (Wichert et al., 2004; Ahdesmaki et al., 2005; Ahdesmaki et al., 2007). For all three programs, input data was formatted as a series of two consecutive cycles. Genes whose cycling expression was deemed significant by at least two programs were considered as oscillating (Koike et al., 2012). For phase distribution, peak expression times calculated by JTK cycle were used. For change of rhythmicity analysis, highly rhythmic genes were defined as BH adjusted p < 0.01 and highly arrhythmic genes as BH adjusted p > 0.5, with BH adjusted p values calculated by JTK cycle.
Statistical analysis
Statistical analysis for sleep experiments was performed using Prism 8 (GraphPad) with specific statistical tests described in figure legends. Statistical analysis for longevity experiments was performed using dLife. RNA-seq experiments were analyzed in R.
Results
Sleep loss throughout lifetime in sleep mutants
To achieve chronic and consistent sleep loss, we monitored the sleep behavior of sleep mutants insomniac1 (inc1) and wide awakeD2 (wakeD2) over their entire lifespans (Figure 1). inc1 and wakeD2 were selected because 1) both inc1 and wakeD2 demonstrate robust sleep loss phenotypes; 2) both inc and wake function in the brain to regulate sleep; and 3) inc and wake are involved in distinct biological pathways; 4) both mutants exhibit a reduction in lifespan, indicating suboptimal levels of sleep (Stavropoulos and Young, 2011; Liu et al., 2014) (Supplementary Figure S1). The mutants were backcrossed to the same genetic background using an isogenized wild type strain iso1CJ (Li et al., 2013a), hereafter referred to as WT. It has been reported that compared to closely related w1118 strain, WT flies show deeper sleep especially at night (Faville et al., 2015).
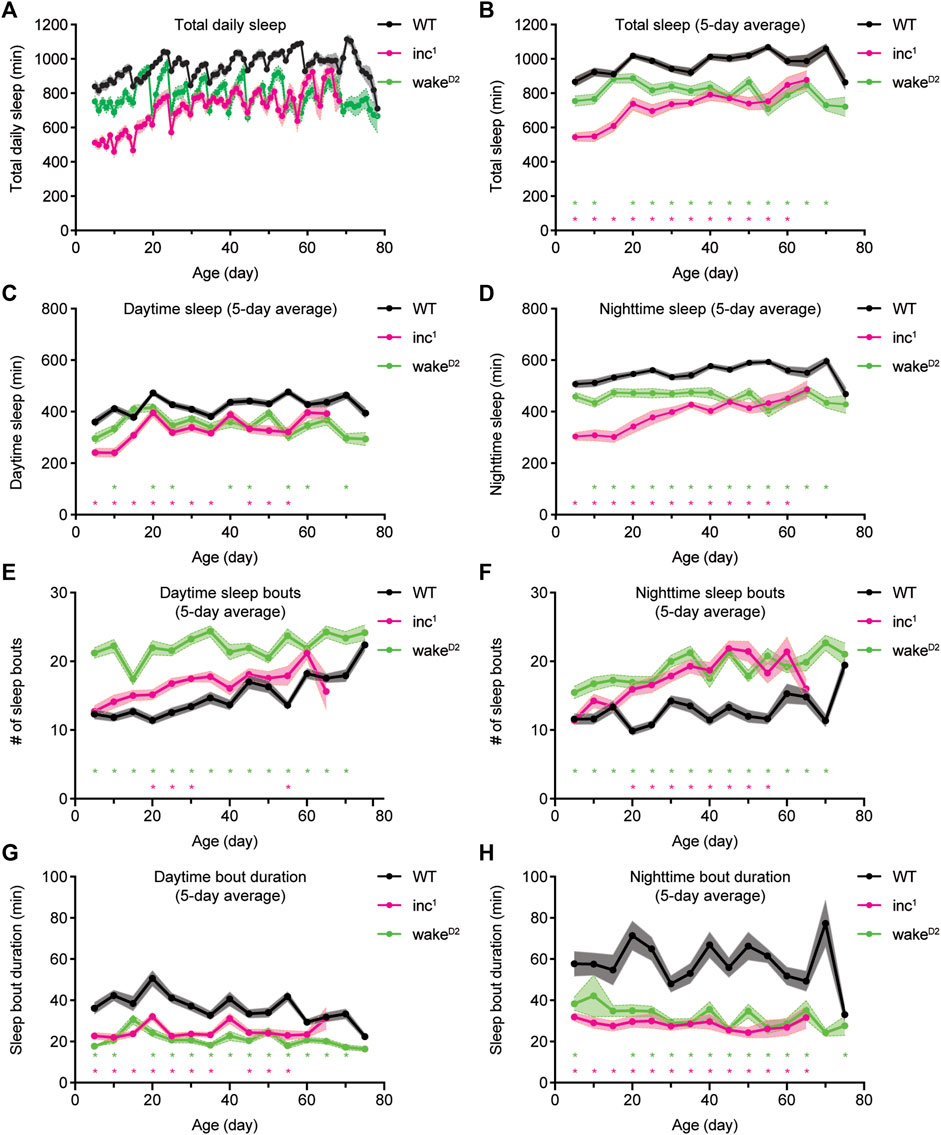
FIGURE 1. Chronic and consistent sleep loss in sleep mutants inc1 and wakeD2. (A) Average total sleep per day for WT (black), inc1 (magenta), and wakeD2 (green) male flies over lifetime. (B–D) 5-day average total sleep per day (B), average daytime sleep (C), and average nighttime sleep (D) for WT (black), inc1 (magenta), and wakeD2 (green) male flies over lifetime. Each data point represents flies of corresponding age that were loaded and measured for sleep over 5 consecutive days. Data collected from different loadings are connected by solid line. (E,F) Average number of daytime sleep bouts (E) and nighttime sleep bouts (F) for WT (black), inc1 (magenta), and wakeD2 (green) male flies over lifetime. (G, H) Average duration of daytime sleep bouts (G) and nighttime sleep bouts (H) for WT (black), inc1 (magenta), and wakeD2 (green) male flies over lifetime. Mean ± SEM is shown. n = 26–32 for WT, n = 4–32 for inc1, n = 11–32 for wakeD2. *p < 0.05; multiple t tests with Holm-Sidak correction against same-age WT flies.
To quantify sleep behavior over the entire lifespan, batches of control and mutant flies were loaded into the Drosophila Activity Monitor (DAM) system every 5 days at specific ages and tested for sleep over 5 consecutive days. Sleep mutants inc1 and wakeD2 consistently showed decreased amounts of total sleep, daytime sleep, and nighttime sleep than wild type animals (Figures 1A–D). Variations in total sleep, daytime sleep, and nighttime sleep were observed within each genotype. Measurements of sleep duration mildly increased in old WT flies. The daily sleep time, daytime sleep, and nighttime sleep of wakeD2 animals fluctuated around fixed levels. However, total sleep of inc1 flies increased substantially in old animals, most of which could be attributed to increased nighttime sleep (Figures 1C,D). Analysis of sleep bouts showed that over the entire course of lifelong sleep measurement, both inc1 and wakeD2 mutant animals showed higher numbers of sleep bouts and bouts of shorter duration compared to control animals (Figures 1E–H). The improvement of sleep with age observed for inc1 could be due to survivorship bias; inc1 flies that managed to get better sleep throughout life might be more likely to live longer. Furthermore, inc1 and wakeD2 animals showed significantly shortened lifespans, suggesting that the mutant flies suffered from physiological defects due to chronic sleep loss (Supplementary Figure S1). Taken together, sleep mutants inc1 and wakeD2 offer exceptional genetic materials to study the consequences of chronic sleep loss.
Transcriptome scale changes of rhythmic gene expression following chronic sleep loss
To study the effects of chronic sleep loss on gene oscillation, we performed transcriptome profiling using RNA-sequencing (RNA-seq) to investigate the changes on gene expression in inc1 and wakeD2 animals. Because inc and wake have diverse physiological functions, an intersectional approach using both mutants can enrich the gene expression changes that result from chronic sleep loss.
The age for sample collection was determined based on the longevity profiles of the mutants and WT animals (Supplementary Figure S1B). Both male and female flies of the same genotype were housed together, as social environment has been reported to affect sleep in flies (Li et al., 2021). Within-group comparison between male flies and female flies did not show significant differences for WT (p > 0.05) or wakeD2 (p > 0.05). inc1 flies displayed a moderate sex-dependent difference in survivorship (p < 0.05) which was more evident after Day 50 (Supplementary Figure S1B). For both males and females, same-sex comparison of sleep mutants against control animals all showed significantly reduced longevity in sleep mutants (p < 0.0001) (Supplementary Figure S1B). We collected heads from 4-week-old animals, before the rapid descent of their longevity curves. Samples were collected every 4 h over 2 days of 12-hour light/12-hour dark (LD) cycles, yielding 12 timepoints per genotype. RNA-seq results showed high within-group correlations (Supplementary Figure S2A). Principal component analysis (PCA) showed clear separations among genotypes (Supplementary Figure S2B).
We first inspected the core circadian clock genes, period (per), timeless (tim), Clock (Clk), and cryptochrome (cry) (Figures 2A–D). It was clear that the Reads Per Kilobase of transcript, per Million mapped reads (RPKM) from the RNA-seq dataset for all 4 core clock genes oscillated with a 24-hour period in all 3 groups. However, some deviations from the control group were observed (Figures 2A–D). The amplitudes of oscillation in inc1 flies were significantly lower for per (Benjamini-Hochberg (BH) adjusted p < 0.0001), tim (BH adjusted p < 0.0001), and cry (BH adjusted p < 0.0001). In wakeD2, oscillation of cry was dampened compared to WT (BH adjusted p < 0.0001). These results suggested oscillation amplitudes of core clock genes were altered in sleep mutants, albeit the core clock genes remain robustly rhythmic.
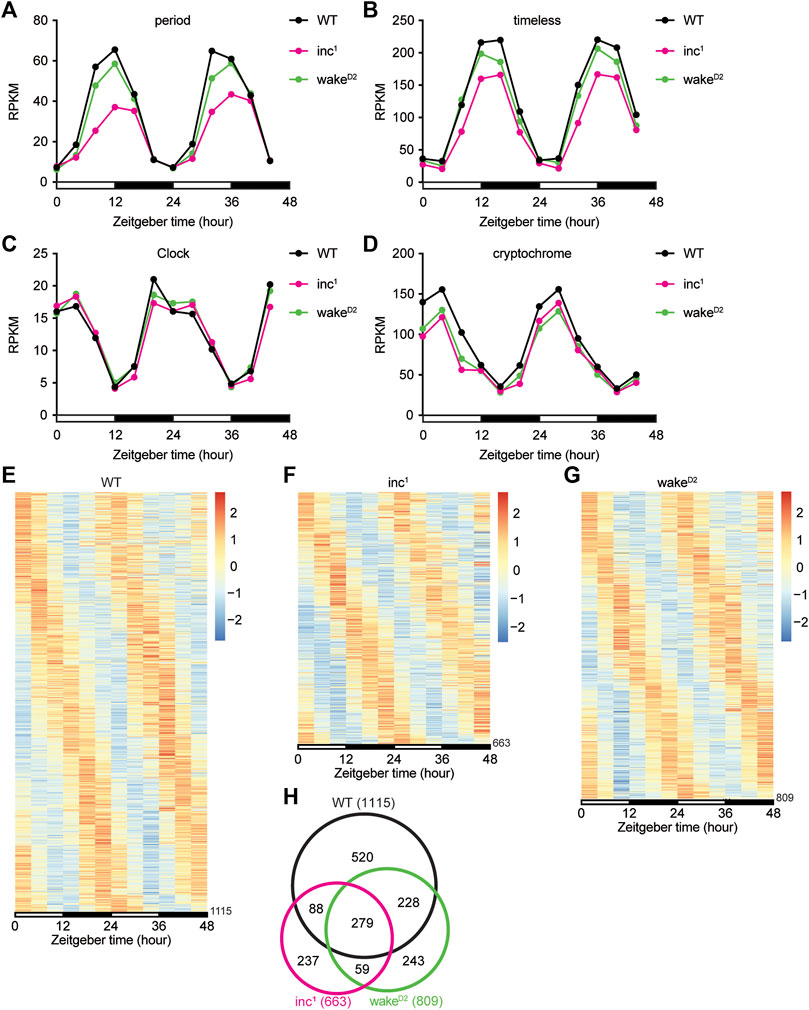
FIGURE 2. Chronic sleep loss by inc or wake mutations disrupts circadian gene oscillation. (A–D) Reads Per Kilobase of transcript, per Million mapped reads (RPKM) of period (A), timeless (B), Clock (C), and cryptochrome (D) for WT (black), inc1 (magenta), and wakeD2 (green). x axis represents two 12-hour light/12-hour dark (LD) cycles with white bars representing daytime and black bars representing nighttime. For period, **** inc1 and n.s. wakeD2. For timeless, **** inc1 and n.s. wakeD2. For Clock, n.s. inc1 and wakeD2. For cryptochrome, **** inc1 and wakeD2. All comparisons are against WT, using Benjamini-Hochberg adjusted p values calculated by DESeq2 for time-series analysis. ****p < 0.0001; n.s., not significant. (E–G) Heatmaps of rhythmic genes in WT (E), inc1 (F), wakeD2 (G) groups. Each row represents one gene, and each column represents one timepoint. Expression levels are normalized to each gene itself and data are aligned according to the peak expression time calculated by JTK cycle. The blocked horizontal bars below the panel correspond to the light-dark cycle. (H) Intersection of rhythmic genes in WT (black), inc1 (magenta), and wakeD2 (green).
Oscillating genes in whole transcriptome were selected using a combinatorial approach of multiple bioinformatic algorithms, including ARSER (Yang and Su, 2010), JTK cycle (Hughes et al., 2010), and GeneCycle (Wichert et al., 2004; Ahdesmaki et al., 2005; Ahdesmaki et al., 2007). Genes whose cycling expression was deemed significant by at least two programs were considered as oscillating (Koike et al., 2012). Using this criterion, we found that 1115 genes exhibited robust oscillation in the control WT flies (Figures 2E, Supplementary File S1), which was comparable to previous studies (Hughes et al., 2012; Kuintzle et al., 2017). We performed the same analysis to identify oscillating transcripts in sleep mutants inc1 and wakeD2 (Figures 2F,G). Compared to control WT flies, a smaller number of genes were identified as rhythmic in the two sleep mutants, 663 in inc1 (Supplementary File S2) and 809 in wakeD2 (Supplementary File S3), suggesting a strong disruption of transcriptome-level gene oscillation compared to WT. About 50% of the oscillating genes identified in inc1 and wakeD2 mutants were present in the set of oscillating genes identified in WT (Figure 2H). The intersection of oscillating genes in all 3 groups resulted in a set of 279 genes (Khan and Mathelier, 2017), suggesting that a significant portion of WT program of rhythmic gene expression is not abolished after chronic sleep loss (Figure 2H).
Surprisingly, in comparison to WT flies, a number of genes gained rhythmic patterns of expression in inc1 and wakeD2 mutants. However, as described in more detail below, these newly rhythmic genes were less often common to both sleep mutants (Figure 2H).
Since the numbers of oscillating genes dramatically decreased in sleep mutants, we evaluated the impact on the phase distributions of rhythmic gene expression in the two sleep mutants. As demonstrated by the circular histograms representing the distribution of peak expression time of oscillating genes, phase distributions among the reduced sets of oscillating genes resembled those of WT (Supplementary Figure S3).
Alteration in rhythmicity induced by chronic sleep loss
To further investigate alterations in rhythmicity after chronic sleep loss, we compared the list of rhythmic genes in each sleep mutant with that of the WT control group, focusing on genes that were found to be highly rhythmic in one group but highly arrhythmic in the other. Interestingly, when the two sleep mutants were individually compared with WT, the scale of rhythmicity change was comparable (Figure 3A,B). Compared to WT, 161 and 153 genes lost rhythmicity in inc1 and wakeD2, respectively; the gain of rhythmicity groups were much smaller, 53 genes for inc1 and 56 genes for wakeD2 (Supplementary File S4,S5). These results confirmed that in both sleep mutants, circadian gene oscillation was affected.
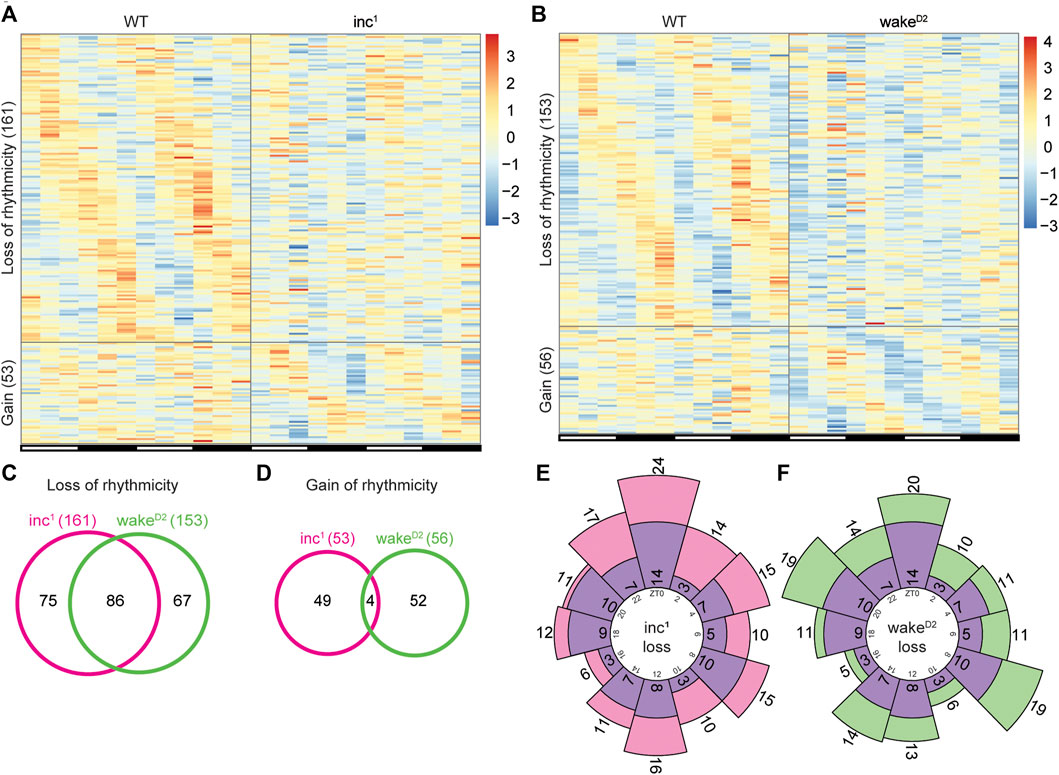
FIGURE 3. Identification of rhythmicity changes in chronically sleep deprived flies. (A,B) Genes with rhythmicity change in inc1 (A) and wakeD2 (B) flies. “Loss of rhythmicity” represents genes that are highly rhythmic in WT and highly arrhythmic in the experimental group, and vice versa for “Gain”. Highly rhythmic genes are defined as Benjamini-Hochberg adjusted p < 0.01 and high arrhythmic genes as Benjamini-Hochberg adjusted p > 0.5, calculated by JTK cycle. Each row represents one gene, and each column represents one timepoint. Expression levels are normalized to each gene itself and data are aligned according to the peak expression time in the highly rhythmic group calculated by JTK cycle. The blocked horizontal bars below the panel correspond to the light-dark cycle. (C,D) Intersection of loss of rhythmicity genes (C) and gain of rhythmicity genes (D) in inc1 (magenta) and wakeD2 (green). (E,F) Circular histogram for the distribution of WT peak expression time for loss of rhythmicity genes in inc1 (E) and wakeD2 (F). Purple bars represent the distribution of WT peak expression time for the 86 intersected genes in (C). Peak expression times are calculated from the WT samples using JTK cycle. Numbers in the inner circle represent Zeitgeber time (ZT) and numbers in or adjacent to the bars represent number of genes in the corresponding bar.
Furthermore, more than 50% (86 genes) of the loss of rhythmicity genes were shared between inc1 and wakeD2, but less than 10% (4 genes) of the genes that gained rhythmicity were shared between inc1 and wakeD2 (Figure 3C,D). These data suggested that the majority of the rhythmicity loss might be linked to chronic sleep disruption, while the gain of rhythmic gene activity appeared to be independent of sleep phenotype.
To assess whether loss of rhythmicity was enriched for a specific timepoint(s), we examined the phase distribution of loss of rhythmicity genes using peak expression time in the WT group (Figures 3E,F). We did not observe timepoint specific enrichment of the peak expression time. Furthermore, there was no significant difference between the phase distributions for the loss of rhythmicity genes in inc1 and wakeD2 (p > 0.05, Chi-square test), nor between the sleep mutants and the 86 intersected genes (p > 0.05, Chi-square test).
The expression patterns of the 86 genes that lost rhythmicity in both sleep mutants are shown in Figure 4. Although gene ontology analysis using the Database for Annotation, Visualization, and Integrated Discovery (DAVID) (Huang da et al., 2009a; Huang da et al., 2009b) did not reveal significantly enriched biological processes, these genes provided valuable information regarding the impact of chronic sleep loss on gene oscillation. Presenilin enhancer (pen-2) is believed to be required for Notch pathway signaling and activity of γ-secretase, a critical player in the development of Alzheimer’s disease (Francis et al., 2002). Pyrokinin 1 receptor (PK1-R) encodes a G-protein coupled receptor (GPCR) and is thought to regulate the production and release of insulin (Alfa et al., 2015). TNF-receptor-associated factor 6 (Traf6) and easter (ea) are both involved in Toll related receptor signaling, an essential component of innate immunity (Cha et al., 2003; Jang et al., 2006). Overexpression of reactive oxygen species regulator Superoxide dismutase 2 (Sod2) has been shown to extend lifespan in Drosophila (Curtis et al., 2007). Non-SMC element 4 (Nse4) and MAGE encode components of the SMC5/6 protein complex, which is crucial for homologous DNA recombination-based DNA repair (Gaudet et al., 2011; Li et al., 2013b). Abnormalities in the rhythmicity of these genes might translate into severe physiological defects affecting lifespan, which remains to be investigated in closer detail.
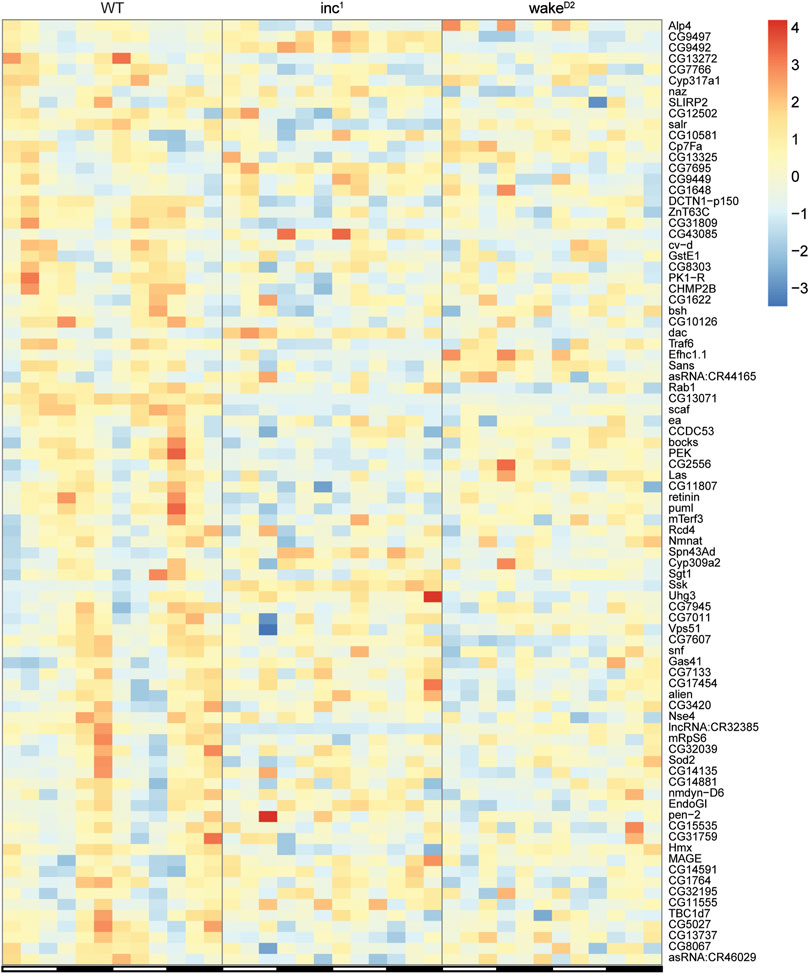
FIGURE 4. Expression of the loss of rhythmicity genes in chronically sleep deprived flies. Each row represents one gene, and each column represents one timepoint. Expression levels are normalized to each gene itself and data are aligned according to the peak expression time in WT group calculated by JTK cycle. The blocked horizontal bars below the panel correspond to the light-dark cycle.
Differential gene expression in chronically sleep deprived animals
A previous microarray study identified many transcripts with altered gene expression level after 1-week of sleep deprivation in rats using the disk-over-water deprivation method (Cirelli et al., 2006). To understand whether the losses of rhythmicity observed in inc1 and wakeD2 were due to similar changes in average expression level, we performed differential gene expression (DGE) analysis to identify genes that exhibited significantly elevated or dampened expression relative to their expression levels in control WT flies. Figure 5A illustrates the workflow of the DGE analysis (see Materials and methods). Two algorithms, DESeq2 (Love et al., 2014) and edgeR (Robinson et al., 2010; McCarthy et al., 2012), were used to identify genes whose expression levels in the experimental group were significantly different from those of the control group independent of timepoints. When compared individually against WT samples, the results from the two sleep mutants differed substantially (Figure 5B,C). Over 2,000 genes were found to be differentially expressed in inc1, while the algorithms identified about 1,000 differentially expressed genes in wakeD2.
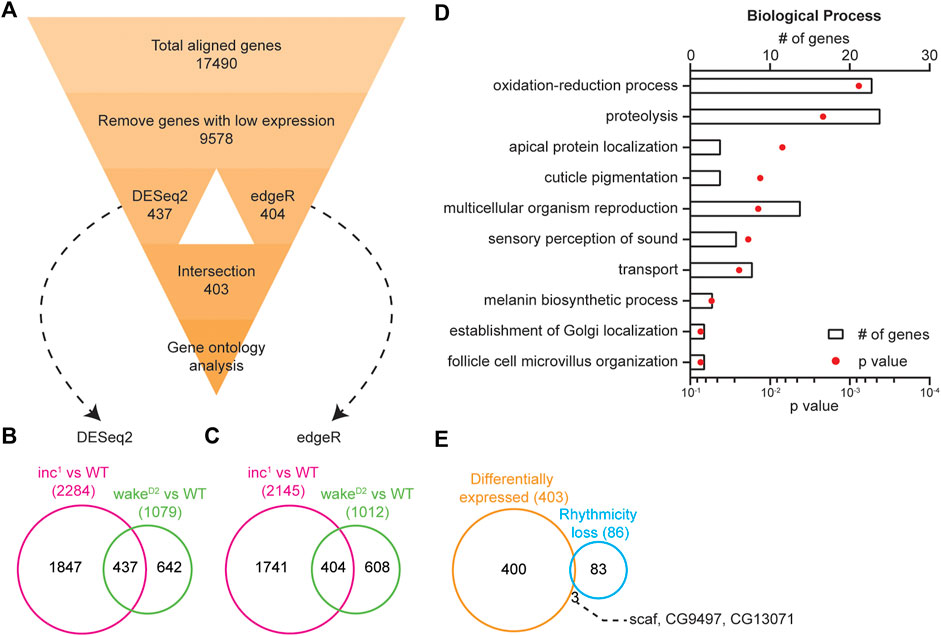
FIGURE 5. Loss of rhythmicity is not caused by differential expression. (A) Flowchart showing the pipeline for differential gene expression (DGE) analysis. Number in each box represents number of genes. (B) Intersection of differentially expressed genes identified by DESeq2 (Benjamini-Hochberg adjusted p < 0.05) in inc1 (magenta) and wakeD2 (green). (C) Intersection of differentially expressed genes identified by edgeR (p < 0.05) in inc1 (magenta) and wakeD2 (green). (D) Enriched biological processes revealed by gene ontology analysis using 403 differentially expressed genes. Top 10 enriched biological pathways are shown. Bars represent number of genes and dots represent p-value. (E) Intersection of differentially expressed genes (orange) and loss of rhythmicity genes (cyan) in both inc1 and wakeD2 animals. The 3 intersected genes are listed.
The results from both comparisons were subsequently intersected. DESeq2 detected 437 genes and edgeR 404 genes. Further intersection of the two algorithms resulted in a list consisting of 403 genes (Supplementary File S6). These analyses demonstrated the power of the intersection approach with two sleep mutants, whereas the choice of bioinformatic programs for DGE did not impose a great influence on the results. With the 403 differentially expressed genes, we conducted gene ontology analysis to examine the enriched biological processes (Huang da et al., 2009a; Huang da et al., 2009b). The top enriched biological processes include oxidation-reduction process, proteolysis, apical protein localization, cuticle pigmentation, and multicellular organism reproduction (Figure 5D).
The list of differentially expressed genes showed little intersection with that of the loss of rhythmicity genes (Figure 5E). Only 3 genes, scarface (scaf), CG9497, and CG13071, were found to be present in both lists, indicating that the changes in circadian oscillation in sleep mutants were not due to changes in timepoint averaged expression levels.
Shared rhythmicity changes across chronically sleep deprived flies and old flies
Alterations in circadian gene rhythmicity have recently been reported in aged flies (Kuintzle et al., 2017). To examine the possibility that genes with altered rhythmicity in sleep mutants exhibit rhythmicity changes in old flies, we performed transcriptome profiling using heads collected from 7-week-old WT flies, referred to as WT-old. The core clock genes remain robustly oscillating in WT-old, suggesting the integrity of the master clock in aged flies (Supplementary Figure S4). However, we discovered 111 genes that lost rhythmicity and 203 genes that gained rhythmicity in WT-old compared to control animals (Figure 6A, Supplementary File S7). The higher abundance of gain of rhythmicity genes in old flies was consistent with findings reported by Kuintzle, et al. (Kuintzle et al., 2017). Additionally, 20 out of 111 loss-of-rhythmicity genes and 61 out of 203 gain-of-rhythmic genes were found to be differentially expressed in WT-old compared to WT (Supplementary File S8).
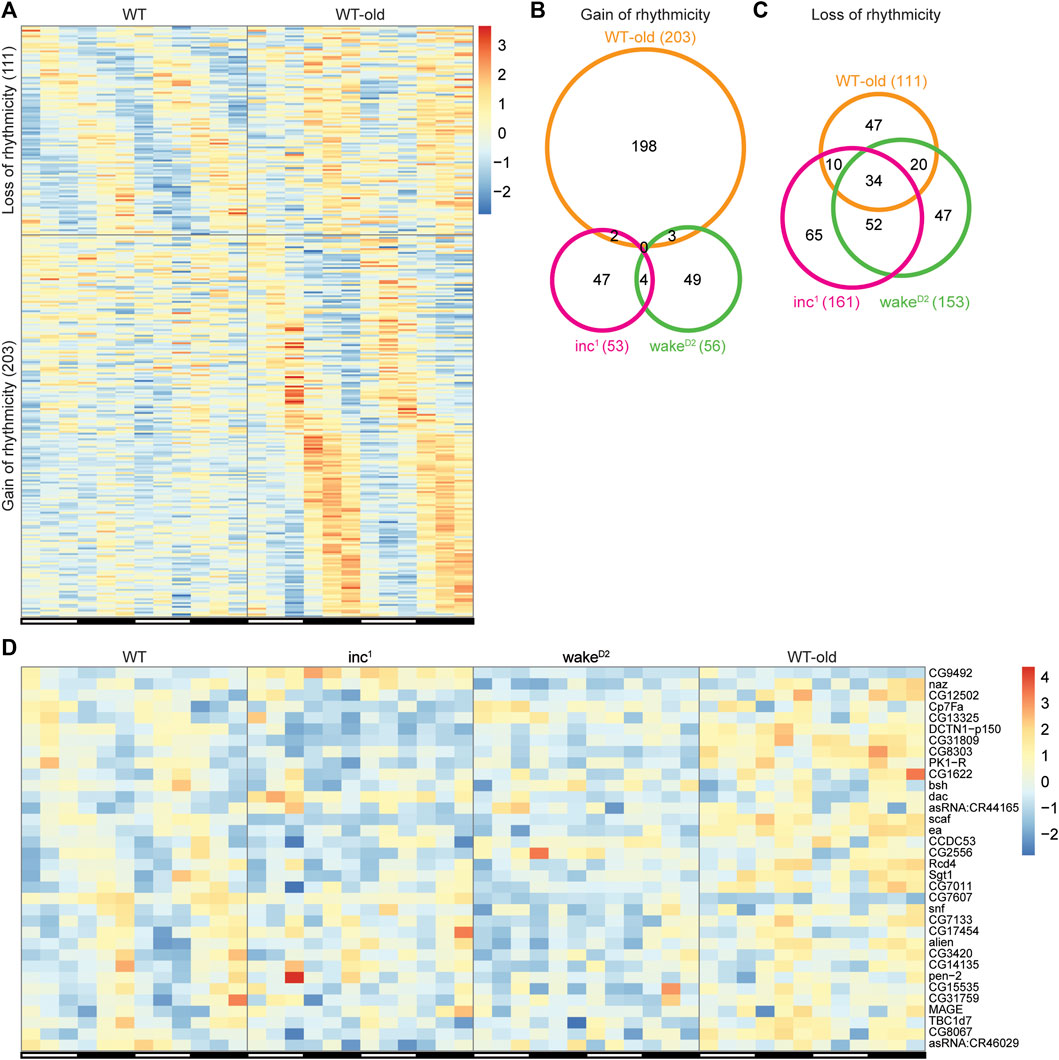
FIGURE 6. Shared rhythmicity changes across chronically sleep deprived flies and old flies. (A) Genes with rhythmicity change in WT-old. Each row represents one gene, and each column represents one timepoint. Expression levels are normalized to each gene itself and data are aligned according to the peak expression time in the highly rhythmic group calculated by JTK cycle. The blocked horizontal bars below the panel correspond to the light-dark cycle. (B,C) Intersection of gain of rhythmicity genes (B) and loss of rhythmicity genes (C) in inc1 (magenta), wakeD2 (green), and WT-old (orange). (D) Heatmap of the 34 intersected genes in (C). Each row represents one gene, and each column represents one timepoint. Expression levels are normalized to each gene itself and data are aligned according to the peak expression time in WT group calculated by JTK cycle. The blocked horizontal bars below the panel correspond to the light-dark cycle.
The results from both sleep mutants and WT-old were intersected to detect genes with similar rhythmicity alterations among experimental groups. None of the gain of rhythmicity genes overlapped across the three experimental groups (Figure 6B), suggesting that the de novo gain of rhythmicity is context specific. Although there were fewer genes in the loss of rhythmicity group than genes in the gain of rhythmicity group in WT-old, the intersection of genes with rhythmicity loss across the experimental groups yielded a fairly large subset of overlapping genes (34 intersecting genes; Figure 6C,D, Supplementary File S9). Out of the 34 genes that lost rhythmicity in all three experimental groups, only 1 gene, scaf, was found to be differentially expressed in all three groups, further supporting the conclusion that changes in circadian oscillation were not due to changes in timepoint averaged expression levels (Supplementary Figure S5)). These results indicate that the disruption of circadian gene oscillation in sleep mutants shares some biological pathways with aging or longevity regulation.
Discussion
Using sleep mutants inc1 and wakeD2 as models for chronic sleep loss, we observed significant changes in circadian gene oscillation. Rhythmicity changes in sleep mutants inc1 and wakeD2 were predominantly due to loss of rhythmicity, rather than changes in average expression level. Although we observed gain of rhythmicity in some genes, we did not identify any overlapping pathways or gene sets across both sleep mutants, suggesting that such changes are more likely to be related to the molecular function of inc or wake, rather than chronic sleep loss. Most interestingly, more than 50% of the genes with altered rhythmicity overlapped between the two sleep mutants. The circadian clock is believed to be intact in inc mutants at least within days after eclosion (Stavropoulos and Young, 2011). WAKE proteins have been shown to be rhythmically expressed in clock neurons and act downstream of CLK to regulate timing of sleep onset (Liu et al., 2014). However, we observed that the oscillation amplitudes of the components of the core circadian clock were dampened, which is likely to contribute to the disruption of circadian clock-controlled gene oscillation observed in inc1 and wakeD2. It was previously reported that a short sleep deprivation is sufficient to perturb the core molecular circadian clock in the mouse brain even long after the mice resumed normal sleep, consistent with our data that chronic sleep deprivation dampened the core molecular clock and circadian rhythmicity profoundly (Hor et al., 2019). Furthermore, we showed that the loss of rhythmicity in inc1 and wakeD2 was not due to changes in average expression levels of the affected genes. Since sleep-wake cycle has been shown to regulate gene oscillation independent of core molecular clock, it remains to be investigated whether the change of rhythmicity was the result of dampened oscillation of core clock genes or a direct effect of chronic sleep disruption (Maret et al., 2007). The collection of genes that lost rhythmic expression in this study of sleep mutants provides promising material for such investigations.
Large scale changes in circadian gene oscillation have been reported in aged flies (Kuintzle et al., 2017), thus we asked whether aging and chronic sleep-loss have an impact on a common set of rhythmically expressed genes. De novo gain of rhythmicity in aged flies was confirmed in our results. However, we observed no gene in the gain-of-rhythmicity groups that was common to inc1, wakeD2, and old flies. In contrast, 34 genes were shared in the loss-of-rhythmicity groups by such a comparison. These results suggest that the mechanisms underlying the observed gain-of-rhythmicity are likely to be independent of the sleep phenotypes of the mutants. In contrast, certain mechanisms for loss of rhythmicity are likely promoted by both chronic sleep loss and aging.
Data availability statement
The datasets presented in this study can be found in online repositories. The RNA-Seq data generated in this study have been deposited in NCBI’s Gene Expression Omnibus (GEO) under accession code GSE148136. The raw sequence data are available for samples of all time points except for WT-ZT28 and WT-ZT40. The counts data generated using STAR and featureCounts for all RNA-seq samples are available. The code used for RNA-seq analysis and intermediary results are available at https://github.com/wangzikun329/Wang2022. RNA-seq expression plots for all genes are available at https://zikunwang.shinyapps.io/rna_seq_gene_plot/.
Author contributions
ZW, Conceptualization, Data curation, Formal analysis, Validation, Investigation, Visualization, Methodology, Writing—original draft, Writing—review and editing; WL, Conceptualization, Data curation, Visualization, Methodology, Writing—original draft, Writing—review and editing. SL and ADN, Investigation, Methodology; MWY, Conceptualization, Supervision, Funding acquisition, Writing—review and editing.
Funding
This work was supported by the NIH grants NS053087, GM054339, and GM136237 to MWY and WL was supported by fellowships from the Leon Levy Foundation, the Jane Coffin Childs Memorial Fund, and the Grass Foundation. WL is currently supported by the Cancer Prevention and Research Institute of Texas.
Acknowledgments
We thank Mark Wu and Jeffrey Friedman for sharing fly stocks and equipment. We thank Leslie Vosshall, Cori Bargmann and Mimi Shirasu-Hiza for insightful discussions. We thank the Genomics Resource Center and the Bioinformatics Resource Center at the Rockefeller University for technical support. We thank Alina Patke, Kai Liu, Sofia Axelrod, Philip Kidd, Deniz Top, and Daniel Cabrera for technical advice and helpful discussions.
Conflict of interest
The authors declare that the research was conducted in the absence of any commercial or financial relationships that could be construed as a potential conflict of interest.
Publisher’s note
All claims expressed in this article are solely those of the authors and do not necessarily represent those of their affiliated organizations, or those of the publisher, the editors and the reviewers. Any product that may be evaluated in this article, or claim that may be made by its manufacturer, is not guaranteed or endorsed by the publisher.
Supplementary material
The Supplementary Material for this article can be found online at: https://www.frontiersin.org/articles/10.3389/fphys.2022.1048751/full#supplementary-material
Supplementary Figure S1 | Longevity phenotypes of sleep mutants inc1 and wakeD2. (A) Longevity of WT (black), inc1 (magenta), and wakeD2 (green) male flies. n = 145−150 for all groups. **** p < 0.0001, log-rank test against WT control animals. (B) Longevity of WT (black), inc1 (magenta), and wakeD2 (green) male (dot) and female (triangle) flies. n = 140−150 for all groups. For log-rank test between male and female of the same genotype, p > 0.05 for WT and wakeD2, p < 0.05 for inc1. For cross genotype log-rank test, p < 0.0001 for inc1 and wakeD2 against WT for both males and females.
Supplementary Figure S2 | Quality check for RNA sequencing results. (A) Heatmap showing gene expression Pearson’s correlation for all RNA-seq samples. (B) Principal component analysis for all RNA-seq samples.
Supplementary Figure S3 | Distribution of peak expression time for oscillating genes. (A–C) Circular histogram for the distribution of peak expression time for rhythmic genes in WT (A), inc1 (B), and wakeD2 (C). Peak expression times are calculated using JTK cycle. Numbers in the inner circle represent Zeitgeber time (ZT) and numbers in the bars represent number of genes in the corresponding bar. Total number of oscillating genes are labeled in parentheses.
Supplementary Figure S4 | Core circadian clock genes maintain robust rhythmicity in aged WT flies. (A–D) Reads Per Kilobase of transcript, per Million mapped reads (RPKM) of period (A), timeless (B), Clock (C), and cryptochrome (D) for WT (black) and WT-old (orange). x axis represents two 12-hour light/12-hour dark (LD) cycles with white bars representing daytime and black bars representing nighttime. No significance was observed between WT and WT-old, as determined by Benjamini-Hochberg adjusted p values calculated by DESeq2 for time-series analysis.
Supplementary Figure S5 | Identification of differentially expressed genes across chronically sleep deprived flies and old flies. (A) Flowchart showing the pipeline for differential gene expression (DGE) analysis. Number in each box represents number of genes. (B) Intersection of differentially expressed genes identified by DESeq2 (Benjamini-Hochberg adjusted p < 0.05) in inc1 (magenta), wakeD2 (green), and WT-old (orange). (C) Intersection of differentially expressed genes identified by edgeR (p < 0.05) in inc1 (magenta), wakeD2 (green), and WT-old (orange). (D) Enriched biological processes revealed by gene ontology analysis using 114 differentially expressed genes. Bars represent number of genes and dots represent p value. (E) Intersection of differentially expressed genes (purple) and loss of rhythmicity genes (blue) across inc1, wakeD2, and WT-old flies. The intersected gene is labeled.
Supplementary file S1 | Oscillating genes in 4-week-old wild type flies with results calculated using JTK cycle.
Supplementary file S2 | Oscillating genes in 4-week-old inc1 flies with results calculated using JTK cycle.
Supplementary file S3 | Oscillating genes in 4-week-old wakeD2 flies with results calculated using JTK cycle.
Supplementary file S4 | Genes with rhythmicity change in inc1 flies.
Supplementary file S5 | Genes with rhythmicity change in wakeD2 flies.
Supplementary file S6 | Intersection of differentially expressed genes in inc1 and wakeD2 with results calculated using DESeq2 and edgeR.
Supplementary file S7 | Genes with rhythmicity change in WT-old flies.
Supplementary file S8 | Differentially expressed genes in WT-old with results calculated using DESeq2 and edgeR.
Supplementary file S9 | ntersection of genes with rhythmicity change in inc1, wakeD2, and WT-old.
References
Ahdesmaki M., Lahdesmaki H., Gracey A., Shmulevich L., Yli-Harja O. (2007). Robust regression for periodicity detection in non-uniformly sampled time-course gene expression data. BMC Bioinforma. 8, 233. doi:10.1186/1471-2105-8-233
Ahdesmaki M., Lahdesmaki H., Pearson R., Huttunen H., Yli-Harja O. (2005). Robust detection of periodic time series measured from biological systems. BMC Bioinforma. 6, 117. doi:10.1186/1471-2105-6-117
Alfa R. W., Park S., Skelly K. R., Poffenberger G., Jain N., Gu X., et al. (2015). Suppression of insulin production and secretion by a decretin hormone. Cell Metab. 21, 323–334. doi:10.1016/j.cmet.2015.01.006
Burgess H. J. (2010). Partial sleep deprivation reduces phase advances to light in humans. J. Biol. Rhythms 25, 460–468. doi:10.1177/0748730410385544
Cha G. H., Cho K. S., Lee J. H., Kim M., Kim E., Park J., et al. (2003). Discrete functions of TRAF1 and TRAF2 in Drosophila melanogaster mediated by c-Jun N-terminal kinase and NF-kappaB-dependent signaling pathways. Mol. Cell. Biol. 23, 7982–7991. doi:10.1128/mcb.23.22.7982-7991.2003
Challet E., Turek F. W., Laute M., Van Reeth O. (2001). Sleep deprivation decreases phase-shift responses of circadian rhythms to light in the mouse: Role of serotonergic and metabolic signals. Brain Res. 909, 81–91. doi:10.1016/s0006-8993(01)02625-7
Cirelli C., Faraguna U., Tononi G. (2006). Changes in brain gene expression after long-term sleep deprivation. J. Neurochem. 98, 1632–1645. doi:10.1111/j.1471-4159.2006.04058.x
Cirelli C., LaVaute T. M., Tononi G. (2005). Sleep and wakefulness modulate gene expression in Drosophila. J. Neurochem. 94, 1411–1419. doi:10.1111/j.1471-4159.2005.03291.x
Claridge-Chang A., NaeFF. , Boothroyd C., RajewskyN. , Young M. W. (2001). Circadian regulation of gene expression systems in the Drosophila head. Neuron 32, 657–671. doi:10.1016/s0896-6273(01)00515-3
Curtis C., Landis G. N., Folk D., Wehr N. B., Hoe N., Waskar M., et al. (2007). Transcriptional profiling of MnSOD-mediated lifespan extension in Drosophila reveals a species-general network of aging and metabolic genes. Genome Biol. 8, R262. doi:10.1186/gb-2007-8-12-r262
Deboer T., Detari L., Meijer J. H. (2007). Long term effects of sleep deprivation on the mammalian circadian pacemaker. Sleep 30, 257–262. doi:10.1093/sleep/30.3.257
Deboer T., Vansteensel M. J., Detari L., Meijer J. H. (2003). Sleep states alter activity of suprachiasmatic nucleus neurons. Nat. Neurosci. 6, 1086–1090. doi:10.1038/nn1122
Dobin A., Davis C. A., Schlesinger F., Drenkow J., Zaleski C., Jha S., et al. (2013). Star: Ultrafast universal RNA-seq aligner. Bioinformatics 29, 15–21. doi:10.1093/bioinformatics/bts635
dos Santos G., Schroeder A. J., Goodman J. L., Strelets V. B., Crosby M. A., Thurmond J., et al. (2015). FlyBase: Introduction of the Drosophila melanogaster release 6 reference genome assembly and large-scale migration of genome annotations. Nucleic Acids Res. 43, D690–D697. doi:10.1093/nar/gku1099
Edgar R., Domrachev M., Lash A. E. (2002). Gene expression Omnibus: NCBI gene expression and hybridization array data repository. Nucleic Acids Res. 30, 207–210. doi:10.1093/nar/30.1.207
Faville R., Kottler B., Goodhill G. J., Shaw P. J., van Swinderen B. (2015). How deeply does your mutant sleep? Probing arousal to better understand sleep defects in Drosophila. Sci. Rep. 5, 8454. doi:10.1038/srep08454
Francis R., McGrath G., Zhang J., Ruddy D. A., Sym M., Apfeld J., et al. (2002). aph-1 and pen-2 are required for Notch pathway signaling, gamma-secretase cleavage of betaAPP, and presenilin protein accumulation. Dev. Cell 3, 85–97. doi:10.1016/s1534-5807(02)00189-2
Gaudet P., Livstone M. S., Lewis S. E., Thomas P. D. (2011). Phylogenetic-based propagation of functional annotations within the Gene Ontology consortium. Brief. Bioinform. 12, 449–462. doi:10.1093/bib/bbr042
Gilestro G. F., Cirelli C. (2009). pySolo: a complete suite for sleep analysis in Drosophila. Bioinformatics 25, 1466–1467. doi:10.1093/bioinformatics/btp237
Hor C. N., Yeung J., Jan M., Emmenegger Y., Hubbard J., Xenarios I., et al. (2019). Sleep–wake-driven and circadian contributions to daily rhythms in gene expression and chromatin accessibility in the murine cortex. Proc. Natl. Acad. Sci. U. S. A. 116, 25773–25783. doi:10.1073/pnas.1910590116
Huang da W., Sherman B. T., Lempicki R. A. (2009). Bioinformatics enrichment tools: Paths toward the comprehensive functional analysis of large gene lists. Nucleic Acids Res. 37, 1–13. doi:10.1093/nar/gkn923
Huang da W., Sherman B. T., Lempicki R. A. (2009). Systematic and integrative analysis of large gene lists using DAVID bioinformatics resources. Nat. Protoc. 4, 44–57. doi:10.1038/nprot.2008.211
Hughes M. E., Grant G. R., Paquin C., Qian J., Nitabach M. N. (2012). Deep sequencing the circadian and diurnal transcriptome of Drosophila brain. Genome Res. 22, 1266–1281. doi:10.1101/gr.128876.111
Hughes M. E., Hogenesch J. B., Kornacker K. (2010). JTK_CYCLE: An efficient nonparametric algorithm for detecting rhythmic components in genome-scale data sets. J. Biol. Rhythms 25, 372–380. doi:10.1177/0748730410379711
Jang I. H., Chosa N., Kim S. H., Nam H. J., Lemaitre B., Ochiai M., et al. (2006). A Spatzle-processing enzyme required for toll signaling activation in Drosophila innate immunity. Dev. Cell 10, 45–55. doi:10.1016/j.devcel.2005.11.013
Jha P. K., Bouaouda H., Gourmelen S., Dumont S., Fuchs F., Goumon Y., et al. (2017). Sleep deprivation and caffeine treatment potentiate photic resetting of the master circadian clock in a diurnal rodent. J. Neurosci. 37, 4343–4358. doi:10.1523/JNEUROSCI.3241-16.2017
Khan A., Mathelier A. (2017). Intervene: A tool for intersection and visualization of multiple gene or genomic region sets. BMC Bioinforma. 18, 287. doi:10.1186/s12859-017-1708-7
Koike N., Yoo S. H., Huang H. C., Kumar V., Lee C., Kim T. K., et al. (2012). Transcriptional architecture and chromatin landscape of the core circadian clock in mammals. Science 338, 349–354. doi:10.1126/science.1226339
Kuintzle R. C., Chow E. S., Westby T. N., Gvakharia B. O., Giebultowicz J. M., Hendrix D. A. (2017). Circadian deep sequencing reveals stress-response genes that adopt robust rhythmic expression during aging. Nat. Commun. 8, 14529. doi:10.1038/ncomms14529
Li W., Prazak L., Chatterjee N., Gruninger S., Krug L., Theodorou D., et al. (2013). Activation of transposable elements during aging and neuronal decline in Drosophila. Nat. Neurosci. 16, 529–531. doi:10.1038/nn.3368
Li W., Wang Z., Syed S., Lyu C., Lincoln S., O'Neil J., et al. (2021). Chronic social isolation signals starvation and reduces sleep in Drosophila. Nature 597, 239–244. doi:10.1038/s41586-021-03837-0
Li X., Zhuo R., Tiong S., Di Cara F., King-Jones K., Hughes S. C., et al. (2013). The Smc5/Smc6/MAGE complex confers resistance to caffeine and genotoxic stress in Drosophila melanogaster. PLoS One 8, e59866. doi:10.1371/journal.pone.0059866
Liao Y., Smyth G. K., Shi W. (2014). featureCounts: an efficient general purpose program for assigning sequence reads to genomic features. Bioinformatics 30, 923–930. doi:10.1093/bioinformatics/btt656
Linford N. J., Bilgir C., Ro J., Pletcher S. D. (2013). Measurement of Lifespan in <em>Drosophila melanogaster</em>. J. Vis. Exp. 10, 50068. doi:10.3791/50068
Liu S., Lamaze A., Liu Q., Tabuchi M., Yang Y., Fowler M., et al. (2014). WIDE AWAKE mediates the circadian timing of sleep onset. Neuron 82, 151–166. doi:10.1016/j.neuron.2014.01.040
Love M. I., Huber W., Anders S. (2014). Moderated estimation of fold change and dispersion for RNA-seq data with DESeq2. Genome Biol. 15, 550. doi:10.1186/s13059-014-0550-8
Maret S., Dorsaz S., Gurcel L., Pradervand S., Petit B., Pfister C., et al. (2007). Homer1a is a core brain molecular correlate of sleep loss. Proc. Natl. Acad. Sci. U. S. A. 104, 20090–20095. doi:10.1073/pnas.0710131104
McCarthy D. J., Chen Y., Smyth G. K. (2012). Differential expression analysis of multifactor RNA-Seq experiments with respect to biological variation. Nucleic Acids Res. 40, 4288–4297. doi:10.1093/nar/gks042
McDonald M. J., Rosbash M. (2001). Microarray analysis and organization of circadian gene expression in Drosophila. Cell 107, 567–578. doi:10.1016/s0092-8674(01)00545-1
Mistlberger R. E., Belcourt J., Antle M. C. (2002). Circadian clock resetting by sleep deprivation without exercise in Syrian hamsters: Dark pulses revisited. J. Biol. Rhythms 17, 227–237. doi:10.1177/07430402017003006
Mure L. S., Le H. D., Benegiamo G., Chang M. W., Rios L., Jillani N., et al. (2018). Diurnal transcriptome atlas of a primate across major neural and peripheral tissues. Science 359, eaao0318. doi:10.1126/science.aao0318
Rijo-Ferreira F., Takahashi J. S. (2019). Genomics of circadian rhythms in health and disease. Genome Med. 11, 82. doi:10.1186/s13073-019-0704-0
Robinson M. D., McCarthy D. J., Smyth G. K. (2010). edgeR: a Bioconductor package for differential expression analysis of digital gene expression data. Bioinformatics 26, 139–140. doi:10.1093/bioinformatics/btp616
Schmidt C., Collette F., Leclercq Y., Sterpenich V., Vandewalle G., Berthomier P., et al. (2009). Homeostatic sleep pressure and responses to sustained attention in the suprachiasmatic area. Science 324, 516–519. doi:10.1126/science.1167337
Shafer O. T., Keene A. C. (2021). The regulation of Drosophila sleep. Curr. Biol. 31, R38–R49. doi:10.1016/j.cub.2020.10.082
Stavropoulos N., Young M. W. (2011). Insomniac and Cullin-3 regulate sleep and wakefulness in Drosophila. Neuron 72, 964–976. doi:10.1016/j.neuron.2011.12.003
van Diepen H. C., Lucassen E. A., Yasenkov R., Groenen I., Ijzerman A. P., Meijer J. H., et al. (2014). Caffeine increases light responsiveness of the mouse circadian pacemaker. Eur. J. Neurosci. 40, 3504–3511. doi:10.1111/ejn.12715
Wichert S., Fokianos K., Strimmer K. (2004). Identifying periodically expressed transcripts in microarray time series data. Bioinformatics 20, 5–20. doi:10.1093/bioinformatics/btg364
Yang R., Su Z. (2010). Analyzing circadian expression data by harmonic regression based on autoregressive spectral estimation. Bioinformatics 26, i168–i174. doi:10.1093/bioinformatics/btq189
Keywords: chronic sleep loss, rhythmic gene expression, transcriptome profiling, sleep, circadian rhythm
Citation: Wang Z, Lincoln S, Nguyen AD, Li W and Young MW (2022) Chronic sleep loss disrupts rhythmic gene expression in Drosophila. Front. Physiol. 13:1048751. doi: 10.3389/fphys.2022.1048751
Received: 19 September 2022; Accepted: 01 November 2022;
Published: 18 November 2022.
Edited by:
Joanna C. Chiu, University of California, Davis, United StatesReviewed by:
Stephane Dissel, University of Missouri–Kansas City, United StatesSwathi Yadlapalli, University of Michigan, United States
Copyright © 2022 Wang, Lincoln, Nguyen, Li and Young. This is an open-access article distributed under the terms of the Creative Commons Attribution License (CC BY). The use, distribution or reproduction in other forums is permitted, provided the original author(s) and the copyright owner(s) are credited and that the original publication in this journal is cited, in accordance with accepted academic practice. No use, distribution or reproduction is permitted which does not comply with these terms.
*Correspondence: Wanhe Li, d2xpMDFAdGFtdS5lZHU=; Michael W. Young, eW91bmdAcm9ja2VmZWxsZXIuZWR1
†Present Address: Zikun Wang, Prevail Therapeutics, New York, NY, United StatesSamantha Lincoln, College of Medicine, Drexel University, Philadelphia, PA, United StatesAndrew D. Nguyen, Neuroscience Graduate Group, University of Pennsylvania, Philadelphia, PA, United States