- 1Institute of Environmental Medicine, Karolinska Institutet, Stockholm, Sweden
- 2Division Heart and Lungs, Department of Cardiology, University Medical Center Utrecht, Utrecht University, Utrecht, Netherlands
- 3Division of Cardiovascular Medicine, Department of Medicine, Danderyd University Hospital, Karolinska Institutet, Stockholm, Sweden
- 4Department of Clinical Sciences, Danderyd University Hospital, Karolinska Institutet, Stockholm, Sweden
- 5Cardiovascular Division, John T. Milliken Department of Internal Medicine, Washington University School of Medicine, St. Louis, MO, United States
- 6Department of Medicine, Christchurch Heart Institute, University of Otago, Christchurch, New Zealand
- 7Cardiovascular Research Institute, National University of Singapore, Singapore, Singapore
- 8Institute for Biomedicine, Eurac Research, Bolzano, Italy
- 9Heart Health Research Group, The University of Auckland, Auckland, New Zealand
- 10Finnish Cardiovascular Research Center - Tampere, Department of Cardio-Thoracic Surgery, Faculty of Medicine and Health Technology, Tampere University, Tampere, Finland
- 11Department of Biochemistry and Molecular Medicine, Keck School of Medicine, University of Southern California, Los Angeles, CA, United States
- 12Department of Population and Public Health Sciences, Keck School of Medicine, University of Southern California, Los Angeles, CA, United States
- 13Department of Cardiovascular and Metabolic Sciences and Center for Microbiome and Human Health, Lerner Research Institute, Cleveland Clinic Ohio, Cleveland, OH, United States
- 14Department of Cardiovascular Medicine, Heart, Vascular and Thoracic Institute, Cleveland Clinic Ohio, Cleveland, OH, United States
- 15Department of Clinical Chemistry, Fimlab Laboratories Ltd., Tampere, Finland
- 16Finnish Cardiovascular Research Center - Tampere, Department of Clinical Chemistry, Faculty of Medicine and Health Technology, Tampere University, Tampere, Finland
- 17Finnish Cardiovascular Research Center - Tampere, Department of Cardiology, Faculty of Medicine and Health Technology, Tampere University, Tampere, Finland
- 18Heart Center, Department of Cardiology, Tampere University Hospital, Tampere, Finland
- 19Heart Center, Department of Thoracic Surgery, Tampere University Hospital, Tampere, Finland
- 20Division of Population Health and Genomics, School of Medicine, University of Dundee, Dundee, United Kingdom
- 21Division of Molecular and Clinical Medicine, School of Medicine, University of Dundee, Dundee, United Kingdom
- 22Department of Internal Medicine, Leiden University Medical Center, Leiden, Netherlands
- 23Section of Gerontology and Geriatrics, and Department of Cardiology, Leiden University Medical Center, Leiden, Netherlands
- 24Laboratory of Experimental Cardiology, University Medical Center Utrecht, Utrecht University, Utrecht, Netherlands
- 25Department of Cardiology, Radboud University Nijmegen Medical Centre, Nijmegen, Netherlands
- 26Amsterdam University Medical Centers, Department of Respiratory Medicine, University of Amsterdam, Amsterdam, Netherlands
- 27Vorarlberg Institute for Vascular Investigation and Treatment (VIVIT), Feldkirch, Austria
- 28Private University in the Principality of Liechtenstein, Triesen, Liechtenstein
- 29Academic Teaching Hospital Feldkirch, Feldkirch, Austria
- 30Center for Pharmacogenomics and Precision Medicine, Department of Pharmacotherapy and Translational Research, University of Florida, Gainesville, FL, United States
- 31Division of Cardiovascular Medicine, College of Medicine, University of Florida, Gainesville, FL, United States
- 32Azienda Ospedaliero Universitaria, Sassari, Italy
- 33Department of Medicine and Intensive Care, County Hospital Bregenz, Bregenz, Austria
- 34Intermountain Medical Center Heart Institute, Salt Lake City, UT, United States
- 35Division of Cardiovascular Medicine, Stanford University, Stanford, CA, United States
- 36Central Diagnostics Laboratory, Division Laboratories, Pharmacy, and Biomedical Genetics, University Medical Center Utrecht, Utrecht, Netherlands
- 37Medicine Laboratory Unit, ASST Rhodense (Rho-Milano), Lombardy, Italy
- 38Department of Medicine and Health Sciences, University of Molise, Campobasso, Italy
- 39Heart and Lung Center, Helsinki University Hospital, University of Helsinki, Helsinki, Finland
- 40Finnish Cardiovascular Research Center - Tampere, Department of Clinical Physiology, Faculty of Medicine and Health Technology, Department of Clinical Physiology, Tampere University, Tampere, Finland
- 41Institute of Clinical Chemistry and Laboratory Medicine, University Hospital Regensburg, Regensburg, Germany
- 42LIFE Research Center for Civilization Diseases, Leipzig University, Leipzig, Germany
- 43LIFE Research Center for Civilization Diseases, Institute for Medical Informatics, Statistics and Epidemiology, Leipzig University, Leipzig, Germany
- 44Department of Cardiology, Leiden University Medical Center, Leiden, Netherlands
- 45Netherlands Heart Institute, Utrecht, Netherlands
- 46Uppsala Clinical Research Center, Uppsala University, Uppsala, Sweden
- 47Department of Medical Sciences, Cardiology, Uppsala University, Uppsala, Sweden
- 48Saint Luke´s Mid America Heart Institute, University of Missouri-Kansas City, Kansas City, MO, United States
- 49Department of Neurology and Neurosurgery, Brain Centre Rudolf Magnus and Julius Center for Health Sciences and Primary Care, University Medical Center Utrecht, Utrecht, Netherlands
- 50Faculty of Population Health Sciences, Institute of Cardiovascular Science and Institute of Health Informatics, University College London, London, United Kingdom
- 51Bart’s Heart Centre, St Bartholomew’s Hospital, London, United Kingdom
Background: The knowledge of factors influencing disease progression in patients with established coronary heart disease (CHD) is still relatively limited. One potential pathway is related to peroxisome proliferator–activated receptor gamma coactivator-1 alpha (PPARGC1A), a transcription factor linked to energy metabolism which may play a role in the heart function. Thus, its associations with subsequent CHD events remain unclear. We aimed to investigate the effect of three different SNPs in the PPARGC1A gene on the risk of subsequent CHD in a population with established CHD.
Methods: We employed an individual-level meta-analysis using 23 studies from the GENetIcs of sUbSequent Coronary Heart Disease (GENIUS-CHD) consortium, which included participants (n = 80,900) with either acute coronary syndrome, stable CHD, or a mixture of both at baseline. Three variants in the PPARGC1A gene (rs8192678, G482S; rs7672915, intron 2; and rs3755863, T528T) were tested for their associations with subsequent events during the follow-up using a Cox proportional hazards model adjusted for age and sex. The primary outcome was subsequent CHD death or myocardial infarction (CHD death/myocardial infarction). Stratified analyses of the participant or study characteristics as well as additional analyses for secondary outcomes of specific cardiovascular disease diagnoses and all-cause death were also performed.
Results: Meta-analysis revealed no significant association between any of the three variants in the PPARGC1A gene and the primary outcome of CHD death/myocardial infarction among those with established CHD at baseline: rs8192678, hazard ratio (HR): 1.01, 95% confidence interval (CI) 0.98–1.05 and rs7672915, HR: 0.97, 95% CI 0.94–1.00; rs3755863, HR: 1.02, 95% CI 0.99–1.06. Similarly, no significant associations were observed for any of the secondary outcomes. The results from stratified analyses showed null results, except for significant inverse associations between rs7672915 (intron 2) and the primary outcome among 1) individuals aged ≥65, 2) individuals with renal impairment, and 3) antiplatelet users.
Conclusion: We found no clear associations between polymorphisms in the PPARGC1A gene and subsequent CHD events in patients with established CHD at baseline.
Introduction
Coronary heart disease (CHD) is a multifactorial disease caused by a complex interplay between genetic, behavioral, and environmental factors, with atherosclerosis as the main underlying component (Tiret, 2002). Several processes important for atherosclerosis, such as lipid homeostasis (Zhang et al., 2004; Lin et al., 2005), endothelial function, and inflammation, are potentially modulated by the peroxisome proliferator–activated receptor gamma coactivator-1 alpha (PPARGC1A), encoded by the PPARGC1A gene (Kadlec et al., 2016). PPARGC1A co-activates several transcription factors involved in energy metabolism and oxidative stress including peroxisome proliferator–activated receptors (PPARs) and nuclear respiratory factors (NRFs) (Liang and Ward, 2006).
Animal studies have shown the evidence of PPARGC1A involvement in cardiac energy metabolism (Arany et al., 2005; Rowe et al., 2010) during development (Lai et al., 2008a) and aging (Whitehead et al., 2018). Furthermore, PPARGC1A is dysregulated in heart failure (Sihag et al., 2009; Oka et al., 2020) and plays a role in endothelial regulation (Craige et al., 2016), atherosclerotic lesions (Kadlec et al., 2016), and may be involved in endogenous protective mechanisms (i.e., ROS and mitochondrial biogenesis) (Chen et al., 2011). Human studies have shown associations between a non-synonymous coding variant single nucleotide polymorphism (SNP) in PPARGC1A (G482S; rs8192678) and metabolic outcomes (Vandenbeek et al., 2017) such as adiposity, insulin resistance (Franks et al., 2014), type 2 diabetes (T2D) (Ek et al., 2001), and hypertension (Andersen et al., 2005). Other SNPs in this gene are less studied in relation to cardiometabolic outcomes, but there are a number of associations reported, for example, between rs7672915 (intron variant) and left-ventricular diastolic function (Juang et al., 2010) and between rs3755863 (T528T) and waist circumference and cholesterol levels (Brito et al., 2009; Mirzaei et al., 2012). Although not found associated with CHD in genome-wide association studies (Peden et al., 2011; Schunkert et al., 2011; Deloukas et al., 2013; Nikpay et al., 2015; van der Harst and Verweij, 2018), PPARGC1A polymorphisms have been associated with the risk of the first-time CHD event and severity in candidate gene association studies (Zhang et al., 2008; Yongsakulchai et al., 2016; Maciejewska-Skrendo et al., 2019). CHD is increasingly described to be a chronic disease with a dynamic nature (Knuuti et al., 2019) and different genetic components could be involved in its progression in different phases. To the best of our knowledge, associations between PPARGC1A and subsequent CHD outcomes have not been investigated.
The GENetIcs of sUbSequent Coronary Heart Disease (GENIUS-CHD) consortium has been established to investigate the genetic determinants of disease progression, following an index CHD event, as many patients are living with CHD due to increased survival rates after a CHD event, and little is known about the risk factors influencing disease progression (Patel et al., 2019a). We conducted an individual-level meta-analysis using data from 23 cohort studies within the GENIUS-CHD consortium (Patel et al., 2019a) to investigate the effect of three different SNPs in the PPARGC1A gene on the risk of subsequent CHD. We could not analyze more than three SNPs due to restrictions within the consortium. The SNP rs8192678 was selected based on extensive previously reported associations with cardiometabolic phenotypes (Arya et al., 2004; Andersen et al., 2005; Barroso et al., 2006; Ridderstråle et al., 2006; Povel et al., 2010). We also included rs7672915 and rs3755863 because, although less studied, they have been suggested to be involved in myocardial metabolism or metabolic traits (Brito et al., 2009; Juang et al., 2010; Mirzaei et al., 2012). In addition, we examined a number of secondary cardiovascular disease endpoints and all-cause mortality, as well as the possible effect modification by age, sex, co-morbidities, and medication use.
Methods
The Consortium
The GENIUS-CHD consortium is an international collaboration, established in 2014 to investigate the impact of genetics on secondary CHD events (http://www.genius-chd.com/). Details about the consortium and inclusion criteria are published elsewhere (Patel et al., 2019a; Patel et al., 2019b). In brief, it mainly includes prospective cohort studies where participants with established CHD at baseline were followed for secondary CHD events. The cases are defined as those experiencing a subsequent CHD event. Participating studies received the local institutional review board approval and included patients who had/provided the informed consent at the time of enrollment.
Inclusion and Exclusion Criteria
Studies were included in the GENIUS-CHD consortium according to the following criteria: First, recruitment of participants with established CHD, defined as acute coronary syndrome or coronary artery disease (any revascularization procedures such as percutaneous coronary intervention, coronary bypass surgery, or a significant (50%) coronary artery plaque at angiography that affects any major epicardial vessel) at baseline or with a history thereof; second, availability of prospective follow-up and ascertainment of at least one clinical cardiovascular outcome (including all-cause mortality); and third, availability of samples, biomarkers, or in silico genotyping data. In the present study, we only included studies if SNP data in the PPARGC1A gene were available (Figure 1, flow-chart).
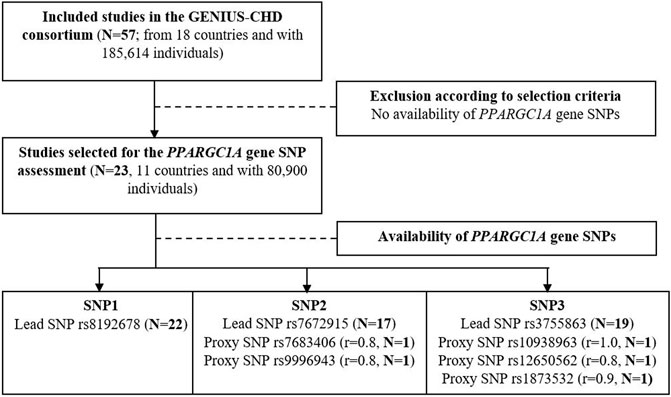
FIGURE 1. Flow chart of study selection criteria and available SNPs for the primary outcome. Correlation between lead and proxy SNP is indicated by r (source: LDproxy Tool; ldlink.nci.nih.gov in European).
Data Extraction and Quality Assessment
We examined three lead SNPs: rs8192678, rs7672915, and rs3755863. If those variants were not available, proxies in high linkage disequilibrium (r2 > 0.8) were considered: rs7683406, rs9996943, rs1873532, rs10938963, and rs12650562 (Figure 1, flow-chart). All proxy SNPs are intronic variants.
The quality control of the genotype data was performed by each study prior to analysis. Minor allele frequencies (MAFs) and Hardy–Weinberg equilibrium (HWE) were examined by each study.
Outcomes
The primary outcome was defined as myocardial infarction (MI) or CHD death during follow-up. Secondary outcomes were MI, coronary revascularization, heart failure, ischemic stroke, any stroke, any CVD (including MI, stroke, coronary revascularization, and CVD death), CHD death, CVD death, and all-cause death.
Statistical Analysis
The associations between SNPs and cardiovascular outcomes were evaluated in individual studies assuming an additive genetic model and using time-to-event Cox proportional hazards models adjusted for age and sex. Analyses were performed using shared statistical scripts and harmonized datasets across the consortium (Patel et al., 2019a; Patel et al., 2019b).
The study-level effect estimates and their corresponding standard errors were entered in an inverse variance weighted fixed-effect meta-analysis model. The χ2 test for heterogeneity and the I2 statistic were used to quantify heterogeneity. Stratified analyses were performed for CHD subtypes at baseline: acute coronary syndrome (ACS) and coronary artery disease (CAD) with prior MI and CAD without prior MI. Stratification was also performed for the baseline patient-level characteristics of age (< or ≥65 years), sex, hypertension (physician-diagnosed or under treatment), T2D (physician-diagnosed or under treatment), body mass index (BMI) (18.5–24.9; 25–29.9; ≥30 kg/m2), statin use, antiplatelet use, renal impairment (eGFR<60 ml/kg/min), and left-ventricular impairment (left-ventricular ejection fraction<45% or diagnosed heart failure with impaired systolic function). Furthermore, sensitivity analyses were performed by stratifying two study-specific factors: European ancestry (a European study where >95% of the participants were of European ancestry versus non-European) and duration of the follow-up (< versus ≥5 years). In addition, we repeated the main analysis excluding cohorts departing from HWE (p < 0.05).
Effect sizes and confidence intervals (CI) were calculated using the two-sided α of 0.05, and results are presented as hazard ratios (HRs). Analyses at the coordinating centers were conducted by R software (version 3.4.1) (R Development Core Team), and the meta-analysis was performed using the EpiSheet tool (K. Rothman, www.krothman.org).
Results
Study Characteristics
In total, 23 studies from the GENIUS-CHD consortium with established CHD and available SNP data in the PPARGC1A gene were selected, with the lead SNPs available in 22, 17, and 19 studies and highly correlated (r2 > 0.8 in Europeans) proxies available in 0, 2, and 2 studies for rs8192678 (non-synonymous variant G482S), rs7672915 (single nucleotide variant in intron 2), and rs3755863 (synonymous variant T528T), respectively (Figure 1). The participant characteristics and genotyping details (MAF and HWE p-value) of the SNPs under investigation are presented in Tables 1, 2.
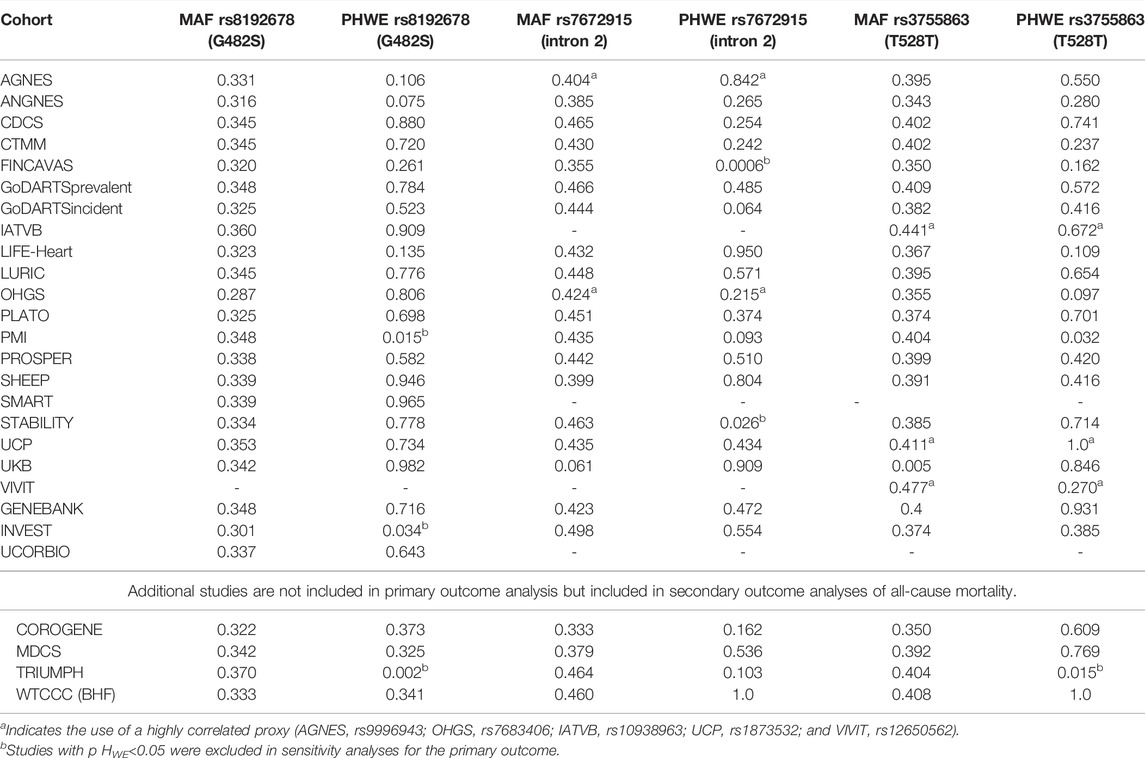
TABLE 2. Minor allele frequencies (MAFs) and p-values for Hardy–Weinberg equilibrium (PHWE) for the three SNPs in the studies included in the meta-analysis.
Meta-Analysis Results
We found null associations for all three SNPs with the primary outcome of CHD death or MI (Figure 2). Similarly, null associations were found for all three SNPs with all secondary outcomes (Figure 3).
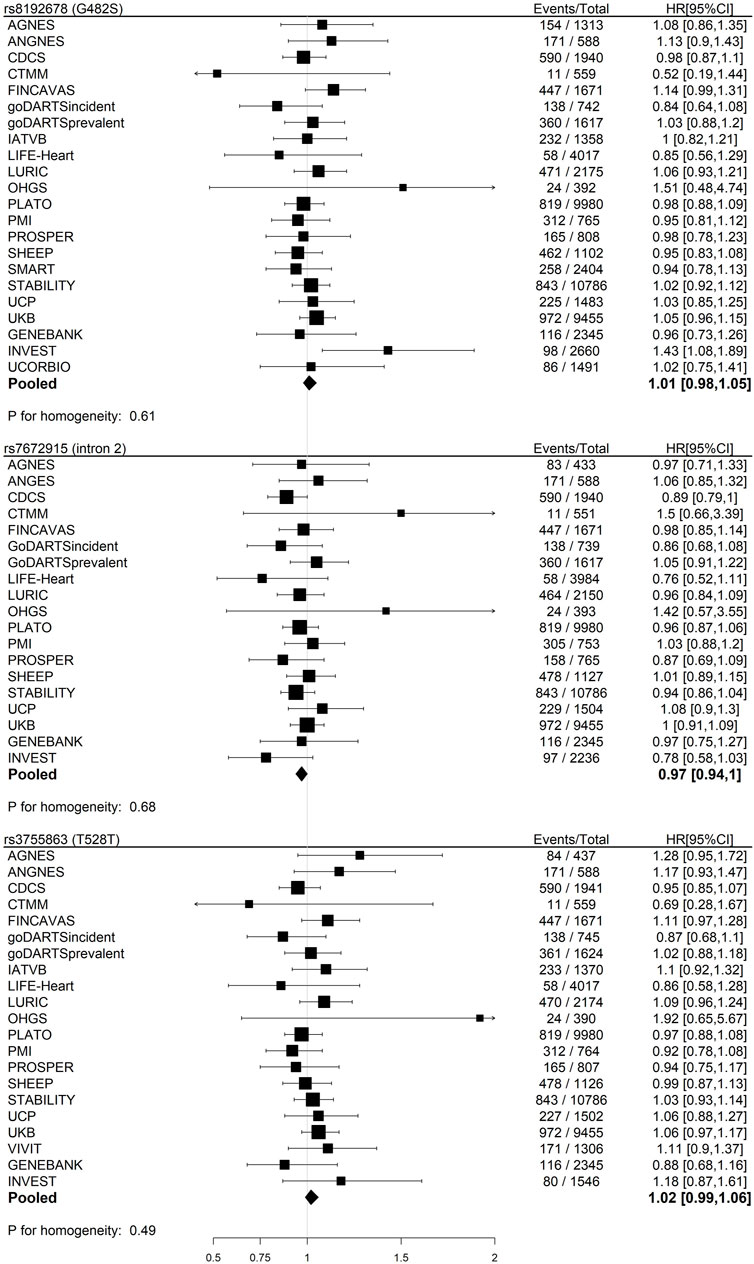
FIGURE 2. Meta-analyses of the associations between three SNPs in the PPARGC1A gene and primary outcome (CHD death or myocardial infarction) in participants with baseline CHD within GENIUS-CHD using an additive, fixed-effect model adjusted for age and sex.
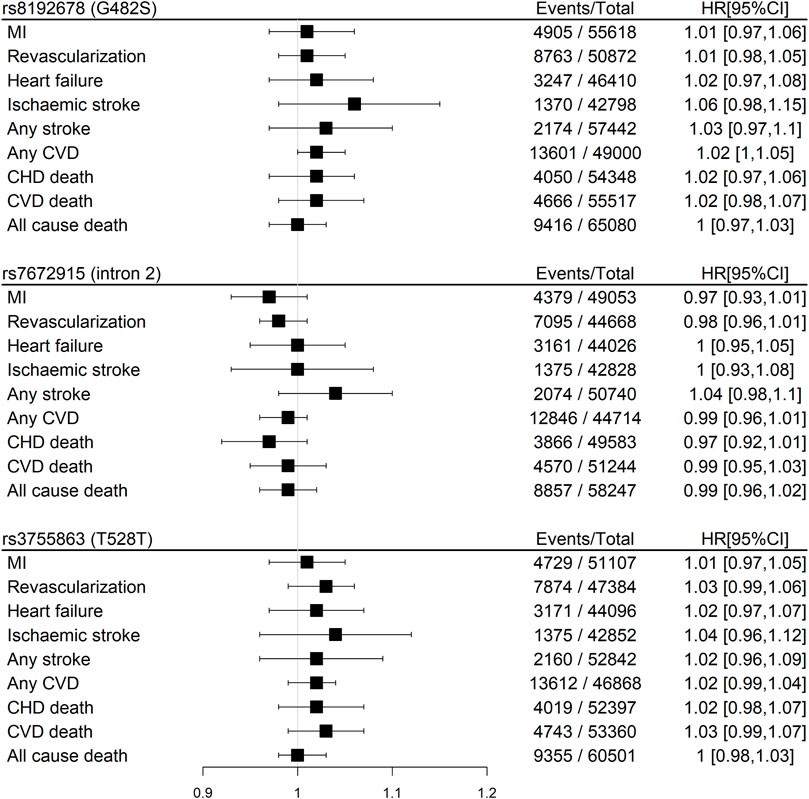
FIGURE 3. Meta-analyses pooled results of the associations between three SNPs in the PPARGC1A gene and secondary outcomes in participants with baseline CHD within GENIUS-CHD using an additive, fixed-effect model stratified for age and sex (phomogeneity>0.05 for all outcomes). Abbreviations: CHD, coronary heart disease; CVD, cardiovascular disease; MI, myocardial infarction.
Stratified Meta-Analysis
Stratification by the CHD subtype at baseline resulted in borderline significant direct associations for rs8192678 (G482S) with the primary outcome for baseline CAD without MI and borderline inverse associations for rs7672915 (intron 2) for baseline ACS (Figure 4). A significant inverse association was found for rs7672915 (intron 2) with the primary outcome among the ≥65 years of age category as well as for renal impairment and antiplatelet use (Figure 5B). There were no significant associations between any of the SNPs and the primary outcome in the models stratified by sex, hypertension, T2D, BMI, statin use, and left-ventricular impairment (Figures 5A–C).
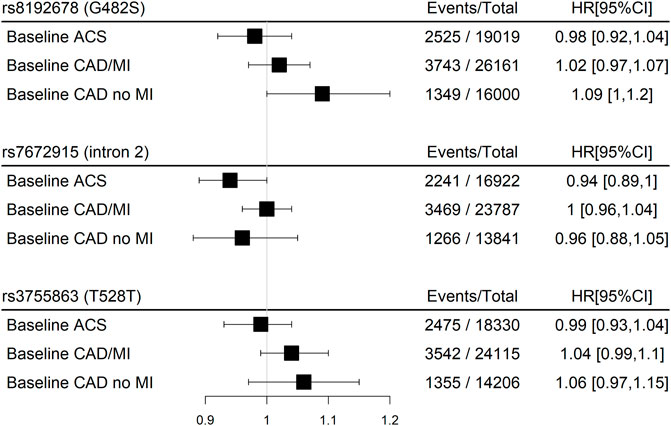
FIGURE 4. Meta-analyses pooled results of the associations between three SNPs in the PPARGC1A gene and the main outcome (CHD death or myocardial infarction) in participants with baseline CHD within GENIUS-CHD using an additive, fixed-effect model stratified for the baseline CHD subtype (phomogeneity>0.05 for all outcomes).Abbreviations: ACS, Acute Coronary Syndrome; CAD, Coronary Artery Disease; MI, Myocardial Infarction.
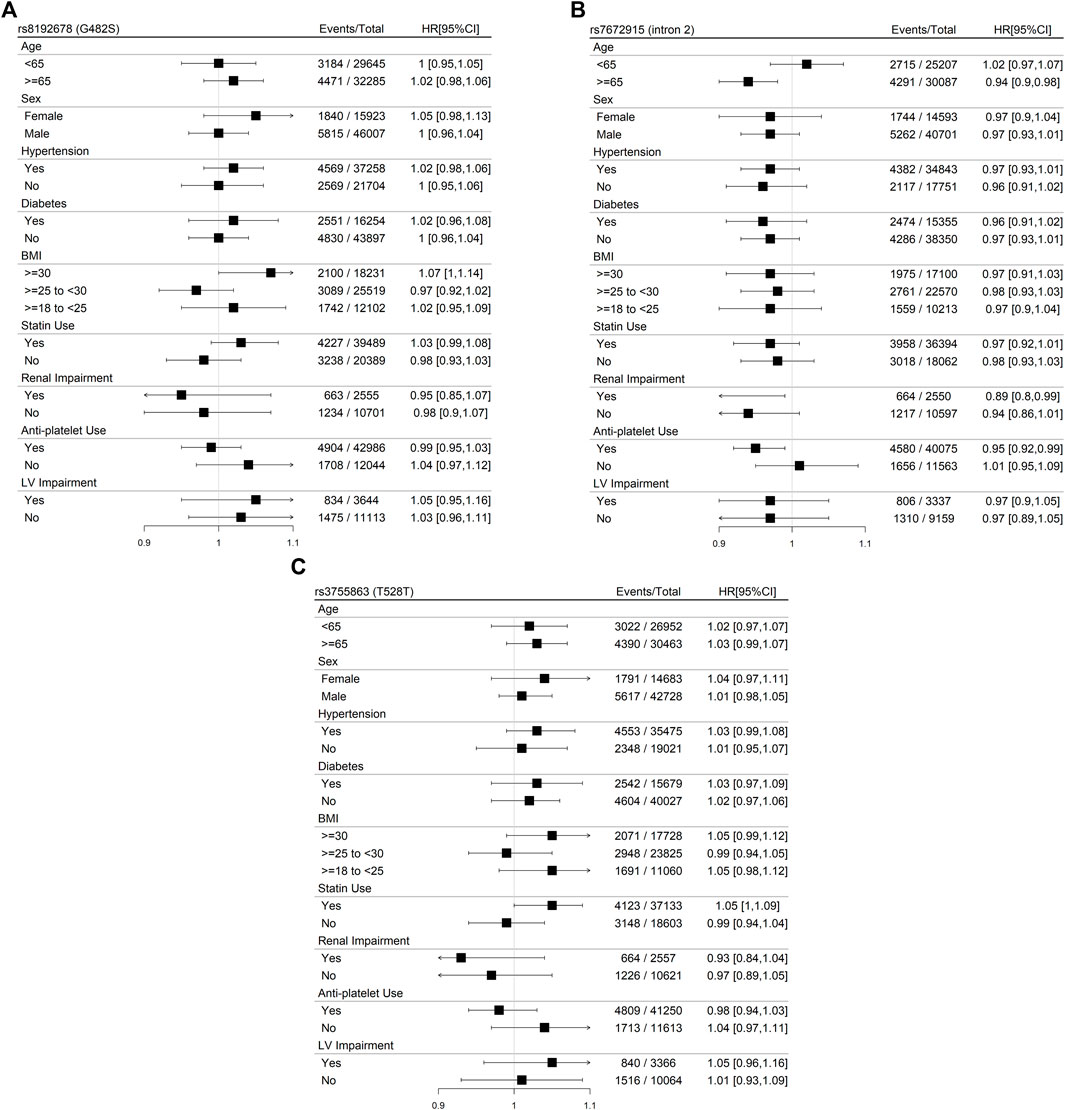
FIGURE 5. Panel (A): Meta-analyses of the associations between rs8192673 (G482S) in the PPARGC1A gene and the primary outcome (CHD death or myocardial infarction) in participants with baseline CHD within GENIUS-CHD using an additive, fixed-effect model stratified for patient-level characteristics. LV, left ventricular. Panel (B): Meta-analyses of the associations between rs7672915 (intron 2) in the PPARGC1A gene and the primary outcome (CHD death or myocardial infarction) in participants with baseline CHD within GENIUS-CHD using an additive, fixed-effect model stratified for patient-level characteristics. LV, left ventricular. Panel (C): Meta-analyses of the associations between rs3755863 (T528T) in the PPARGC1A gene and the primary outcome (CHD death or myocardial infarction) in participants with baseline CHD within GENIUS-CHD using an additive, fixed-effect model stratified for patient-level characteristics. LV, left ventricular.
Sensitivity analyses only indicated marginal differences. Neither exclusion of cohorts deviating from HWE nor stratification by European ancestry changed associations with the primary outcome (data not shown). Stratification by follow-up time also resulted in null associations, except for significant inverse associations for rs7672915 (intron 2) and the primary outcome in the stratum with follow-up <5 years (HR: 0.93, 95% CI 0.88–0.99).
Discussion
This meta-analysis resulted in overall null associations between three polymorphisms in the PPARGC1A gene studied in relation to the risk of subsequent CHD events (primary outcome) in a population with established CHD. The polymorphism rs7672915 (intron 2) was, however, observed to be borderline inversely associated with subsequent CHD events.
The results were generally consistent across the strata of CHD subtypes at baseline as well as of patient- and study-level characteristics. However, inverse associations with the primary outcome were seen for rs7672915 (intron 2) amongst those with age ≥65, renal impairment, and use of antiplatelets. In addition, inverse associations with the primary outcome were seen for rs7672915 (intron 2) among studies having a follow-up <5 years.
Human Studies on Polymorphisms in the PPARGC1A Gene
Our results suggested that the PPARGC1A gene in patients with established CHD does not play an important role in disease progression, leading to subsequent CHD events. Previous research study has not investigated this relationship. However, there are studies that indicate that the PPARGC1A gene is important for the development of CAD (Zhang et al., 2008; Yongsakulchai et al., 2016; Maciejewska-Skrendo et al., 2019) and cardiometabolic disease phenotypes (Ek et al., 2001; Barroso et al., 2006; Xie et al., 2007; Lai et al., 2008b; Vimaleswaran et al., 2008; Yang et al., 2011; Franks et al., 2014; Jemaa et al., 2015; Kruzliak et al., 2015). The PPARGC1A gene (rs8192678; G482S) was associated with an increased risk of CAD in a Chinese population (Zhang et al., 2008). Moreover, the PPARGC1A gene (rs8192678; G482S), alone as well as in combination with polymorphisms in PPARG and liver X receptor α (LXRA), associated with an increased risk and severity of CAD in a Thai population (Yongsakulchai et al., 2016). The PPARGC1A gene (rs8192678; G482S) was further associated with T2D in a Tunisian population (Jemaa et al., 2015), with waist circumference among Slovenian participants with T2D (Kruzliak et al., 2015) and with severe hypertension in a Chinese population (Xie et al., 2007). The PPARGC1A gene was also identified in a search for protein-level interactions with transcripts mapped nearest to T2D susceptibility loci (Morris et al., 2012). Although the rs8192678; G482S Ser482 allele appears to be associated with increased obesity and T2D susceptibility (Vandenbeek et al., 2017) as well as a poorer therapeutic efficacy of rosiglitazone (Zhang et al., 2010), its carriers also appear to respond better to caloric restriction (Goyenechea et al., 2008) and bariatric surgery (Geloneze et al., 2012). In the Boston Puerto Rican Health Study, they found associations between polymorphisms in the PPARGC1A gene and DNA damage, T2D, and CVD (Lai et al., 2008b). However, meta-analysis only indicated modest roles within specific ethnicity and age groups for polymorphisms in the PPARGC1A gene (rs8192678; G482S) with T2D (Barroso et al., 2006; Yang et al., 2011) and hypertension (Vimaleswaran et al., 2008). The Ser482 allele carried an increased risk for hypertrophic cardiomyopathy in a community-based cross-sectional study in China (Wang et al., 2007). However, another study in a Russian population did not find evidence for such an association (Nikitin et al., 2010). The two other SNPs under investigation were less studied but have been shown to associate with metabolic traits (Brito et al., 2009; Juang et al., 2010; Mirzaei et al., 2012): rs7672915 (intron 2) associated with left-ventricular diastolic function in Caucasians (Juang et al., 2010) whereas rs3755863 (T528T) was found to be associated with waist circumference in European children (Brito et al., 2009) and with LDL cholesterol in an adult population consisting mostly of obese women (Mirzaei et al., 2012). Furthermore, rs3755863 (T528T) seemed to decrease PPARGC1A expression levels in cellular models (Mirzaei et al., 2012). Although results from subgroup analyses always should be interpreted with caution, it is possible that the significant association between rs7672915 (intron 2) and the primary outcome seen only in the subgroup of individuals aged 65 years or older in our study population may be relevant; age-related risk factors could interact with genetics and increase vulnerability to subsequent events. Based on similar reasoning, the significant associations we observed in subgroups with impaired renal function and users of antiplatelets, respectively, could be relevant. Also, the fact that rs7672915 (intron 2) was significantly associated with our primary outcome when the basis for the analysis was limited to including cohort data with less than 5 years of follow-up may indicate that the PPARGC1A gene plays a role in CHD progression, possibly in repair and recovery after an initial event in the short term.
Mechanistic Studies on PPARGC1A and Cardiometabolic Health
The PPARGC1A gene, located on chromosome 4, encodes for a protein consisting of 798 amino acids in humans. It is highly expressed in tissues abundant in mitochondria such as the liver (in fasting states) (Yoon et al., 2001), kidney, brown adipose tissue, skeletal muscle, brain, and heart (Puigserver et al., 1998). PPARGC1A activates transcription factors by inducing a conformational change after binding to them, which increases the affinity of the transcription complex to other coactivators that have histone acetyltransferase activity. This increased affinity will then lead to the acetylation of histone proteins and conformational alterations that allow the increased accessibility of DNA to the transcription complex (Puigserver et al., 1999). Several pathways involving PPARGC1A and energy metabolism have been described, for example, mitochondria biogenesis, glucose/fatty acid metabolism, remodeling of fiber muscle composition, and adaptive thermogenesis (Liang and Ward, 2006). The effect of PPARGC1A on the mitochondrial metabolism, especially, can have implications for cardiac health by regulating the fuel availability and the amount of reactive oxygen species in the heart (Di et al., 2018). PPARGC1A target genes that are thought to play a role in cardiac health are estrogen-related receptors (ERRs; i.e., ERRα, ERRβ, and ERRγ) and nuclear respiratory factor-1, which activate many mitochondrial genes, as well as PPARs (i.e., PPARα, PPARβ, PPARγ, and PPARδ), which play important roles in the fatty acid uptake and oxidation in the heart (Di et al., 2018). Any dysregulation in PPARGC1A may be detrimental; studies have shown that its downregulation increased vascular stress (Kadlec et al., 2016), oxidative stress and inflammation (Waldman et al., 2018; Rius-Pérez et al., 2020), impaired mitochondrial function, and reduced antiapoptotic and angiogenic responses (Mahmood et al., 2019), whereas its upregulation induced pathological changes in mitochondrial biogenesis, contributing to cardiac disease (Lehman et al., 2000; Le Chen and Knowlton, 2011; Caravia et al., 2018). One of the SNPs (rs8192678; G482S) was recently shown to decrease the stability, impact structural conformation, and catalytic function of the PPARGC1A protein, which could be detrimental for CAD (Taghvaei et al., 2021). However, this is not reflected in our findings of null associations for the three tested SNPs in the PPARGC1A gene with subsequent CHD events. Our findings of inverse associations of rs7672915, intron 2 in subgroups (older age, with renal impairment, and antiplatelet users) could be random findings or due to the role of PPARGC1A in mitochondria subsequently affecting the aging process (Wenz, 2011), kidney disease (Lynch et al., 2018), and platelet function (Melchinger et al., 2019).
Limitations and Strengths
There are several limitations which may have attenuated or diluted the effect estimates. First, when studying cohorts of patients, as in this study, there is always a possibility that the index event bias may influence the results (Dahabreh and Kent, 2011; Patel et al., 2019a). Our study population consists of CHD survivors subjected to varying types of preventive actions, including lifestyle changes and drug treatments, which may have impacted risks for recurrent events and death. In addition, there is a possibility that individuals who died early with the disease have a more severe phenotype and that the degree of severity is linked to the presence of the genetic variants we studied. However, in the previously published genome-wide studies of genetic variants in relation to the risk of first-time events of CHD, the current genetic variants were not included among the significant association findings (Peden et al., 2011; Schunkert et al., 2011; Deloukas et al., 2013; Nikpay et al., 2015; van der Harst and Verweij, 2018). Furthermore, if the index event is a consequence of a strong risk factor, there may be lower levels of exposure to other—individually weaker—independent risk factors in the selected population, which could have attenuated associations between genetic variants and the risk of subsequent events in our study (Patel et al., 2019b). Second, we had no information on the age of onset of the index CHD event or on whether revascularization procedures were late-staged (belonging to the index event) or unplanned and symptom-driven (true secondary event). Third, the variability in the follow-up between studies could impact the findings through outcome misclassification. Fourth, it is possible that other SNPs, outside our selected three, in the PPARGC1A gene play a key role. Finally, in the present study, we only investigated single SNP associations within a single gene whereas it could be relevant to also address gene–gene interactions and polygenetic scores. The major strength of this study, however, is the large number of studies and individuals included. This allowed us to make reasonably conclusive inferences.
Conclusion
The findings from this large individual-level meta-analysis do not indicate the involvement of the PPARGC1A gene in the progression to secondary CHD events amongst people who experienced an index CHD event. However, future research studies on the potential role of PPARGC1A in subgroups of patients with established CHD, in relation to the risk of recurrence, may be warranted.
Data Availability Statement
The data analyzed in this study are subjected to the following licenses/restrictions: Individual participant-level data for each participating study were not collected for the present project and will, therefore, not be made available. Further details and contact information are available at http://krothman.hostbyet2.com/. Requests to access these datasets should be directed to www.genius-chd.org.
Ethics Statement
The studies involving human participants were reviewed and approved by the local institutional review board approval and included patients who had provided informed consent at the time of enrollment. Written informed consent for participation was not required for this study in accordance with the national legislation and the institutional requirements.
Author Contributions
KL, RP, and FA: conceptualization. KL, TS, BM, FL, MV, SC, and BG: preparation of the analysis plan. TS: original manuscript draft preparation. VT and MV: data analysis. All authors contributed to data collection, either within the framework of a participatory study or at a more comprehensive level in terms of collecting results from participatory studies. All authors contributed to the editing of the manuscript and approved the submitted version.
Funding
The funders of the study had no role in study design, data collection, data analysis, data interpretation, or writing of the report. Within GENIUS-CHD, all participating investigators and sponsors who contributed to data and analyses are acknowledged irrespective of academic or industry affiliations. The GENIUS-CHD was supported by the National Institute for Health Research University College London Hospitals Biomedical Research Centre. The UCORBIO are thankful for the support of the Netherlands CardioVascular Research Initiative of the Netherlands Heart Foundation [CVON 2011/B019 and CVON 2017–20: Generating the best evidence-based pharmaceutical targets for atherosclerosis (GENIUS I&II)], the ERA-CVD program “druggable-MI-targets” (grant number: 01 KL 1802), and the Leducq Fondation “PlaqOmics.” The CDCS and PMI studies were funded by the Health Research Council and Heart Foundation of New Zealand; the AGNES study was supported by research grants from the Netherlands Heart Foundation [2001D019, 2003T302, and 2007B202 and the PREDICT project (CVON 2012–10)], the Leducq Foundation (grant 05-CVD) and the Center for Translational Molecular Medicine (CTMM COHFAR); the Cleveland Clinic Genebank Study was supported in part by NIH grants R01HL103866, R01DK106000, P01HL147823, P01HL098055, P01HL076491, R01HL133169, and R01HL148110; the Corogene study was supported by grants from the Aarno Koskelo Foundation, Helsinki University Central Hospital special government funds (EVO #TYH7215, #TKK2012005, #TYH2012209, and #TYH2014312), and the Finnish Foundation for Cardiovascular research; the Wellcome Trust United Kingdom Type 2 Diabetes Case Control Collection (supporting GoDARTS) was funded by the Wellcome Trust (072960/Z/03/Z, 084726/Z/08/Z, 084727/Z/08/Z, 085475/Z/08/Z, and 085475/B/08/Z) and as part of the EU IMI-SUMMIT programme. The IATVB was supported by Epidemiologia e Genetica della Morte Improvvisa in Sardegna; INVEST-GENES was supported by the National Institute of Health Pharmacogenomics Research Network grants U01-GM074492, NIH R01 HL074730, University of Florida Opportunity Fund, BASF Pharma and Abbott Laboratories; LIFE-Heart was funded by the Leipzig Research Center for Civilization Diseases (LIFE). LIFE is an organizational unit affiliated to the Medical Faculty of the University of Leipzig and funded by means of the European Union, by the European Regional Development Fund (ERDF) and by funds of the Free State of Saxony within the framework of the excellence initiative; the LURIC study was supported by the Seventh Framework Program (AtheroRemo, grant agreement number 201668 and RiskyCAD, grant agreement number 305739) of the European Union; the OHGS was funded in part by a Heart and Stroke Foundation grant; the PROSPER study was supported by an investigator-initiated grant obtained from Bristol Myers Squibb. Support for genotyping was provided by the seventh framework program of the European commission (grant 223004) and by the Netherlands Genomics Initiative (Netherlands Consortium for Healthy Aging grant 050–060-810); the SHEEP study was supported by grants from the Swedish Council for Work Life and Social Research, and the Stockholm County Council; the TRIUMPH study was sponsored by the National Institutes of Health: Washington University School of Medicine SCCOR Grant P50 HL077113; the UCP studies were funded by the Netherlands Heart Foundation and the Dutch Top Institute Pharma Mondriaan Project. RP was funded by a British Heart Foundation Intermediate Fellowship (FS/14/76/30933); FA was supported by UCL Hospitals NIHR Biomedical Research Centre; TS was supported by the Swedish Research Council no 2017-00822; KL was supported, in part, by the Swedish Heart-Lung Foundation (grant number 20180540); SC was supported, in part, by the National Institutes of Health (Cresci R01 NR013396); SL was funded through EU H2020 TO_AITION (grant number: 848146). AP was funded by the Health Research Council of New Zealand and the Christchurch Heart Institute Trust; JJ is an established clinical investigator of the Netherlands Heart Foundation (grant 2001 D 032).
Conflict of Interest
SL has received Roche funding for unrelated work; SJ has received grants from AstraZeneca, The Medicines Company; CH declares advisory board and speaker’s bureau for AstraZeneca, institutional research grants from Bristol Myers Squibb, Merck &Co, GlaxoSmithKline, Roche Diagnostics, and advisory board for Bayer and Boehringer Ingelheim; EH declares being an expert committee member, lecture fees, and institutional research grant from Sanofi and Amgen, institutional research grants from AstraZeneca and GlaxoSmithKline, as well as an expert committee member and lecture fees for Novo Nordisk and Behringer; BH has an institutional research grant from AstraZeneca for unrelated work and is an advisory board member for Opsis Health and Lab Me Analytics, and Intermountain Healthcare has licensed his intellectual property to CareCentra and Alluceo; NE declares institutional research grants from AstraZeneca and Pfizer. AXA has received institutional research grant and speaker fee from AstraZeneca and an institutional research grant from Roche Diagnostics; AXA has received an institutional research grant and speaker fee from AstraZeneca and an institutional research grant from Roche Diagnostics; BH has an institutional research grant from AstraZeneca for unrelated work and is an advisory board member for Opsis Health and Lab Me Analytics, and Intermountain Healthcare has licensed his intellectual property to CareCentra and Alluceo.
The remaining authors declare that the research was conducted in the absence of any commercial or financial relationships that could be construed as a potential conflict of interest.
Publisher’s Note
All claims expressed in this article are solely those of the authors and do not necessarily represent those of their affiliated organizations, or those of the publisher, the editors, and the reviewers. Any product that may be evaluated in this article, or claim that may be made by its manufacturer, is not guaranteed or endorsed by the publisher.
Acknowledgments
The GENIUS-CHD collaborators would like to express their immense gratitude to all patients who participated in each of the individual studies and the many personnel who helped with recruitment, collection, curation, management, and processing of the samples and data. In addition to this, the GoDARTS would like to express gratitude to the Scottish School of Primary Care for their help in recruiting the participants, to the Health Informatics Centre, University of Dundee, for managing and supplying the anonymized data, and to the NHS Tayside, the original data owner.
References
Andersen G., Wegner L., Jensen D. P., Glümer C., Tarnow L., Drivsholm T., et al. (2005). PGC-1α Gly482Ser Polymorphism Associates with Hypertension Among Danish Whites. Hypertension 45, 565–570. doi:10.1161/01.HYP.0000158946.53289.24
Arany Z., He H., Lin J., Hoyer K., Handschin C., Toka O., et al. (2005). Transcriptional Coactivator PGC-1α Controls the Energy State and Contractile Function of Cardiac Muscle. Cell. Metab. 1, 259–271. doi:10.1016/j.cmet.2005.03.002
Arya R., Duggirala R., Jenkinson C. P., Almasy L., Blangero J., O’Connell P., et al. (2004). Evidence of a Novel Quantitative-Trait Locus for Obesity on Chromosome 4p in Mexican Americans. Am. J. Hum. Genet. 74, 272–282. doi:10.1086/381717
Barroso I., Luan J., Sandhu M. S., Franks P. W., Crowley V., Schafer A. J., et al. (2006). Meta-analysis of the Gly482Ser Variant in PPARGC1A in Type 2 Diabetes and Related Phenotypes. Diabetologia 49, 501–505. doi:10.1007/s00125-005-0130-2
Brito E. C., Vimaleswaran K. S., Brage S., Andersen L. B., Sardinha L. B., Wareham N. J., et al. (2009). PPARGC1A Sequence Variation and Cardiovascular Risk-Factor Levels: a Study of the Main Genetic Effects and Gene × Environment Interactions in Children from the European Youth Heart Study. Diabetologia 52, 609–613. doi:10.1007/s00125-009-1269-z
Caravia X. M., Fanjul V., Oliver E., Roiz-Valle D., Morán-Álvarez A., Desdín-Micó G., et al. (2018). The microRNA-29/PGC1α Regulatory axis Is Critical for Metabolic Control of Cardiac Function. PLoS Biol. 16, e2006247. doi:10.1371/journal.pbio.2006247
Chen S.-D., Yang D.-I., Lin T.-K., Shaw F.-Z., Liou C.-W., Chuang Y.-C. (2011). Roles of Oxidative Stress, Apoptosis, PGC-1α and Mitochondrial Biogenesis in Cerebral Ischemia. Int. J. Mol. Sci. 12, 7199–7215. doi:10.3390/ijms12107199
Craige S. M., Kröller-Schön S., Li C., Kant S., Cai S., Chen K., et al. (2016). PGC-1α Dictates Endothelial Function through Regulation of eNOS Expression. Sci. Rep. 6, 38210. doi:10.1038/srep38210
Dahabreh I. J., Kent D. M. (2011). Index Event Bias as an Explanation for the Paradoxes of Recurrence Risk Research. JAMA 305, 822–823. doi:10.1001/jama.2011.163
Deloukas P., Deloukas P., Kanoni S., Willenborg C., Farrall M., Assimes T. L., et al. (2013). Large-scale Association Analysis Identifies New Risk Loci for Coronary Artery Disease. Nat. Genet. 45, 25–33. doi:10.1038/ng.2480
Di W., Lv J., Jiang S., Lu C., Yang Z., Ma Z., et al. (2018). PGC-1: The Energetic Regulator in Cardiac Metabolism. Curr. Issues Mol. Biol. 28, 29–46. doi:10.21775/cimb.028.029
Ek J., Andersen G., Urhammer S. A., Gæde P. H., Drivsholm T., Borch-Johnsen K., et al. (2001). Mutation Analysis of Peroxisome Proliferator-Activated Receptor-γ Coactivator-1 (PGC-1) and Relationships of Identified Amino Acid Polymorphisms to Type II Diabetes Mellitus. Diabetologia 44, 2220–2226. doi:10.1007/s001250100032
Franks P. W., Christophi C. A., Christophi C. A., Jablonski K. A., Billings L. K., Delahanty L. M., et al. (2014). Common Variation at PPARGC1A/B and Change in Body Composition and Metabolic Traits Following Preventive Interventions: the Diabetes Prevention Program. Diabetologia 57, 485–490. doi:10.1007/s00125-013-3133-4
Geloneze S. R., Geloneze B., Morari J., Matos-Souza J. R., Lima M. M., Chaim E. A., et al. (2012). PGC1α Gene Gly482Ser Polymorphism Predicts Improved Metabolic, Inflammatory and Vascular Outcomes Following Bariatric Surgery. Int. J. Obes. 36, 363–368. doi:10.1038/ijo.2011.176
Goyenechea E., Crujeiras A. B., Abete I., Parra D., Martínez J. A. (2008). Enhanced Short-Term Improvement of Insulin Response to a Low-Caloric Diet in Obese Carriers the Gly482Ser Variant of the PGC-1α Gene. Diabetes Res. Clin. Pract. 82, 190–196. doi:10.1016/j.diabres.2008.08.011
Jemaa Z., Kallel A., Sleimi C., Mahjoubi I., Feki M., Ftouhi B., et al. (2015). The Gly482Ser Polymorphism of the Peroxisome Proliferator-Activated Receptor-γ Coactivator-1α (PGC-1α) Is Associated with Type 2 Diabetes in Tunisian Population. Diabetes & Metabolic Syndrome Clin. Res. Rev. 9, 316–319. doi:10.1016/j.dsx.2013.10.011
Juang J.-M. J., de las Fuentes L., Waggoner A. D., Gu C. C., Dávila-Román V. G. (2010). Association and Interaction of PPAR-Complex Gene Variants with Latent Traits of Left Ventricular Diastolic Function. BMC Med. Genet. 11, 65. doi:10.1186/1471-2350-11-65
Kadlec A. O., Chabowski D. S., Ait-Aissa K., Gutterman D. D. (2016). Role of PGC-1α in Vascular Regulation: Implications for Atherosclerosis. Atvb 36, 1467–1474. doi:10.1161/ATVBAHA.116.307123
Knuuti J., Wijns W., Saraste A., Capodanno D., Barbato E., Funck-Brentano C., et al. (2019). 2019 ESC Guidelines for the Diagnosis and Management of Chronic Coronary Syndromes. Eur. Heart J. 41, 407–477. oi:. doi:10.1093/eurheartj/ehz425
Kruzliak P., Haley A. P., Starcevic J. N., Gaspar L., Petrovic D. (2015). Polymorphisms of the Peroxisome Proliferator-Activated Receptor-γ (Rs1801282) and its Coactivator-1 (Rs8192673) Are Associated with Obesity Indexes in Subjects with Type 2 Diabetes Mellitus. Cardiovasc. Diabetol. 14, 42. doi:10.1186/s12933-015-0197-0
Lai C.-Q., Tucker K. L., Parnell L. D., Adiconis X., García-Bailo B., Griffith J., et al. (2008). PPARGC1A Variation Associated with DNA Damage, Diabetes, and Cardiovascular Diseases. Diabetes 57, 809–816. doi:10.2337/db07-1238
Lai L., Leone T. C., Zechner C., Schaeffer P. J., Kelly S. M., Flanagan D. P., et al. (2008). Transcriptional Coactivators PGC-1α and PGC-Lβ Control Overlapping Programs Required for Perinatal Maturation of the Heart. Genes. Dev. 22, 1948–1961. doi:10.1101/gad.1661708
Le Chen L., Knowlton A. A. (2011). Mitochondrial Dynamics in Heart Failure. Congest. Heart Fail. 17, 257–261. doi:10.1111/j.1751-7133.2011.00255.x
Lehman J. J., Barger P. M., Kovacs A., Saffitz J. E., Medeiros D. M., Kelly D. P. (2000). Peroxisome Proliferator-Activated Receptor γ Coactivator-1 Promotes Cardiac Mitochondrial Biogenesis. J. Clin. Investig. 106, 847–856. doi:10.1172/JCI10268
Liang H., Ward W. F. (2006). PGC-1α: a Key Regulator of Energy Metabolism. Adv. Physiology Educ. 30, 145–151. doi:10.1152/advan.00052.2006
Lin J., Handschin C., Spiegelman B. M. (2005). Metabolic Control through the PGC-1 Family of Transcription Coactivators. Cell. Metab. Metab. 1, 361–370. doi:10.1016/j.cmet.2005.05.004
Lynch M. R., Tran M. T., Parikh S. M. (2018). PGC1α in the Kidney. Am. J. Physiology-Renal Physiology 314, F1–f8. doi:10.1152/ajprenal.00263.2017
Maciejewska-Skrendo A., Pawlik A., Sawczuk M., Rać M., Kusak A., Safranow K., et al. (2019). PPARA, PPARD and PPARG Gene Polymorphisms in Patients with Unstable Angina. Gene 711, 143947. doi:10.1016/j.gene.2019.143947
Mahmood E., Jeganathan J., Feng R., Saraf M., Khabbaz K., Mahmood F., et al. (2019). Decreased PGC-1α Post-Cardiopulmonary Bypass Leads to Impaired Oxidative Stress in Diabetic Patients. Ann. Thorac. Surg. 107, 467–476. doi:10.1016/j.athoracsur.2018.08.009
Melchinger H., Jain K., Tyagi T., Hwa J. (2019). Role of Platelet Mitochondria: Life in a Nucleus-free Zone. Front. Cardiovasc. Med. 6, 153. doi:10.3389/fcvm.2019.00153
Mirzaei K., Hossein-nezhad A., Emamgholipour S., Ansar H., Khosrofar M., Tootee A., et al. (2012). An ExonicPeroxisome Proliferator-Activated Receptor-?Coactivator-1a Variation May Mediate the Resting Energy Expenditure through a Potential Regulatory Role on Important Gene Expression in This Pathway. J. Nutr. Nutr. 5, 59–71. doi:10.1159/000337352
Morris A. P., Voight B. F., Teslovich T. M., et al. (2012). Large-scale Association Analysis Provides Insights into the Genetic Architecture and Pathophysiology of Type 2 Diabetes. Nat. Genet. 44, 981–990. doi:10.1038/ng.2383
Nikitin A. G., Chistiakov D. A., Minushkina L. O., Zateyshchikov D. A., Nosikov V. V. (2010). Association of the CYBA, PPARGC1A, PPARG3, and PPARD Gene Variants with Coronary Artery Disease and Metabolic Risk Factors of Coronary Atherosclerosis in a Russian Population. Heart Vessels 25, 229–236. doi:10.1007/s00380-009-1159-9
Nikpay M., Goel A., Won H. H., Hall L. M., Willenborg C., Kanoni S., et al. (2015). A Comprehensive 1,000 Genomes-Based Genome-wide Association Meta-Analysis of Coronary Artery Disease. Nat. Genet. 47 (10), 1121–1130. doi:10.1038/ng.3396
Oka S.-i., Sabry A. D., Cawley K. M., Warren J. S. (2020). Multiple Levels of PGC-1α Dysregulation in Heart Failure. Front. Cardiovasc. Med. 7, 2. doi:10.3389/fcvm.2020.00002
Patel R. S., Schmidt A. F., Tragante V., McCubrey R. O., Holmes M. V., Howe L. J., et al. (2019). Association of Chromosome 9p21 with Subsequent Coronary Heart Disease Events. Circ. Genom Precis. Med. 12, e002471. doi:10.1161/CIRCGEN.119.002471
Patel R. S., Tragante V., Schmidt A. F., McCubrey R. O., Holmes M. V., Howe L. J., et al. (2019). Subsequent Event Risk in Individuals with Established Coronary Heart Disease. Circ. Genom Precis. Med. 12, e002470. doi:10.1161/CIRCGEN.119.002470
Peden J. F., Hopewell J. C., Saleheen D., et al. (2011). A Genome-wide Association Study in Europeans and South Asians Identifies Five New Loci for Coronary Artery Disease. Nat. Genet. 43, 339–344. doi:10.1038/ng.782
Povel C. M., Feskens E. J. M., Imholz S., Blaak E. E., Boer J. M. A., Dollé M. E. T. (2010). Glucose Levels and Genetic Variants across Transcriptional Pathways: Interaction Effects with BMI. Int. J. Obes. 34, 840–845. doi:10.1038/ijo.2009.302
Puigserver P., Adelmant G., Wu Z., Fan M., Xu J., O'Malley B., et al. (1999). Activation of PPARγ Coactivator-1 through Transcription Factor Docking. Science 286, 1368–1371. doi:10.1126/science.286.5443.1368
Puigserver P., Wu Z., Park C. W., Graves R., Wright M., Spiegelman B. M. (1998). A Cold-Inducible Coactivator of Nuclear Receptors Linked to Adaptive Thermogenesis. Cell. 92, 829–839. doi:10.1016/s0092-8674(00)81410-5
Ridderstråle M., Johansson L. E., Rastam L., Lindblad U. (2006). Increased Risk of Obesity Associated with the Variant Allele of the PPARGC1A Gly482Ser Polymorphism in Physically Inactive Elderly Men. Diabetologia 49, 496–500. doi:10.1007/s00125-005-0129-8
Rius-Pérez S., Torres-Cuevas I., Millán I., Ortega Á. L., Pérez S. (2020). PGC-1α, Inflammation, and Oxidative Stress: An Integrative View in Metabolism. Oxidative Med. Cell. Longev. 2020, 1–20. doi:10.1155/2020/1452696
Rowe G. C., Jiang A., Arany Z. (2010). PGC-1 Coactivators in Cardiac Development and Disease. Circ. Res. 107, 825–838. doi:10.1161/CIRCRESAHA.110.223818
Schunkert H., König I. R., Kathiresan S., Reilly M. P., Assimes T. L., Holm H., et al. (2011). Large-scale Association Analysis Identifies 13 New Susceptibility Loci for Coronary Artery Disease. Nat. Genet. 43, 333–8. doi:10.1038/ng.784
Sihag S., Cresci S., Li A. Y., Sucharov C. C., Lehman J. J. (2009). PGC-1α and ERRα Target Gene Downregulation Is a Signature of the Failing Human Heart. J. Mol. Cell. Cardiol. 46, 201–212. doi:10.1016/j.yjmcc.2008.10.025
Taghvaei S., Saremi L., Babaniamansour S. (2021). Computational Analysis of Gly482Ser Single-Nucleotide Polymorphism in PPARGC1A Gene Associated with CAD, NAFLD, T2DM, Obesity, Hypertension, and Metabolic Diseases. PPAR Res. 2021, 1–12. doi:10.1155/2021/5544233
Tiret L. (2002). Gene-environment Interaction: a Central Concept in Multifactorial Diseases. Proc. Nutr. Soc. 61, 457–463. doi:10.1079/pns2002178
van der Harst P., Verweij N. (2018). Identification of 64 Novel Genetic Loci Provides an Expanded View on the Genetic Architecture of Coronary Artery Disease. Circ. Res. 122 (3), 433–443. doi:10.1161/circresaha.117.312086
Vandenbeek R., Khan N. P., Estall J. L. (2017). Linking Metabolic Disease with the PGC-1α Gly482Ser Polymorphism. Endocrinology 159, 853–865. doi:10.1210/en.2017-00872
Vimaleswaran K. S., Luan J. a., Andersen G., Muller Y. L., Wheeler E., Brito E. C., et al. (2008). The Gly482Ser Genotype at the PPARGC1A Gene and Elevated Blood Pressure: a Meta-Analysis Involving 13,949 Individuals. J. Appl. Physiology 105, 1352–1358. doi:10.1152/japplphysiol.90423.2008
Waldman M., Cohen K., Yadin D., Nudelman V., Gorfil D., Laniado-Schwartzman M., et al. (2018). Regulation of Diabetic Cardiomyopathy by Caloric Restriction Is Mediated by Intracellular Signaling Pathways Involving 'SIRT1 and PGC-1α'. Cardiovasc. Diabetol. 17, 111. doi:10.1186/s12933-018-0754-4
Wang S., Fu C., Wang H., Shi Y., Xu X., Chen J., et al. (2007). Polymorphisms of the Peroxisome Proliferator-Activated Receptor-γ Coactivator-1α Gene Are Associated with Hypertrophic Cardiomyopathy and Not with Hypertension Hypertrophy. Clin. Chem. Lab. Med. 45, 962–967. doi:10.1515/CCLM.2007.189
Wenz T. (2011). Mitochondria and PGC-1α in Aging and Age-Associated Diseases. J. Aging Res. 2011, 1–12. doi:10.4061/2011/810619
Whitehead N., Gill J. F., Brink M., Handschin C. (2018). Moderate Modulation of Cardiac PGC-1α Expression Partially Affects Age-Associated Transcriptional Remodeling of the Heart. Front. Physiol. 9, 242. doi:10.3389/fphys.2018.00242
Xie G., Guo D., Li Y., Liang S., Wu Y. (2007). The Impact of Severity of Hypertension on Association of PGC-1αGene with Blood Pressure and Risk of Hypertension. BMC Cardiovasc. Disord. 7, 33. doi:10.1186/1471-2261-7-33
Yang Y., Mo X., Chen S., Lu X., Gu D. (2011). Association of Peroxisome Proliferator-Activated Receptor Gamma Coactivator 1 Alpha (PPARGC1A) Gene Polymorphisms and Type 2 Diabetes Mellitus: a Meta-Analysis. Diabetes Metab. Res. Rev. 27, 177–184. doi:10.1002/dmrr.1158
Yongsakulchai P., Settasatian C., Settasatian N., Komanasin N., Kukongwiriyapan U., Cote M. L., et al. (2016). Association of Combined Genetic Variations in PPARγ , PGC-1α , and LXRα with Coronary Artery Disease and Severity in Thai Population. Atherosclerosis 248, 140–148. doi:10.1016/j.atherosclerosis.2016.03.005
Yoon J. C., Puigserver P., Chen G., Donovan J., Wu Z., Rhee J., et al. (2001). Control of Hepatic Gluconeogenesis through the Transcriptional Coactivator PGC-1. Nature 413, 131–138. doi:10.1038/35093050
Zhang K.-H., Huang Q., Dai X.-P., Yin J.-Y., Zhang W., Zhou G., et al. (2010). Effects of the Peroxisome Proliferator Activated Receptor-γ Coactivator-1α(PGC-1α) Thr394ThrandGly482SerPolymorphisms on Rosiglitazone Response in Chinese Patients with Type 2 Diabetes Mellitus. J. Clin. Pharmacol. 50, 1022–1030. doi:10.1177/0091270009355159
Zhang Y., Castellani L. W., Sinal C. J., Gonzalez F. J., Edwards P. A. (2004). Peroxisome Proliferator-Activated Receptor-γ Coactivator 1α (PGC-1α) Regulates Triglyceride Metabolism by Activation of the Nuclear Receptor FXR. Genes. Dev. 18, 157–169. doi:10.1101/gad.1138104
Keywords: polymorphisms, PPARGC1A, meta-analysis, SNPs, coronary heart disease, cohort studies
Citation: Schillemans T, Tragante V, Maitusong B, Gigante B, Cresci S, Laguzzi F, Vikström M, Richards M, Pilbrow A, Cameron V, Foco L, Doughty RN, Kuukasjärvi P, Allayee H, Hartiala JA, Tang WHW, Lyytikäinen L-P, Nikus K, Laurikka JO, Srinivasan S, Mordi IR, Trompet S, Kraaijeveld A, van Setten J, Gijsberts CM, Maitland-van der Zee AH, Saely CH, Gong Y, Johnson JA, Cooper-DeHoff RM, Pepine CJ, Casu G, Leiherer A, Drexel H, Horne BD, van der Laan SW, Marziliano N, Hazen SL, Sinisalo J, Kähönen M, Lehtimäki T, Lang CC, Burkhardt R, Scholz M, Jukema JW, Eriksson N, Åkerblom A, James S, Held C, Hagström E, Spertus JA, Algra A, de Faire U, Åkesson A, Asselbergs FW, Patel RS and Leander K (2022) Associations of Polymorphisms in the Peroxisome Proliferator-Activated Receptor Gamma Coactivator-1 Alpha Gene With Subsequent Coronary Heart Disease: An Individual-Level Meta-Analysis. Front. Physiol. 13:909870. doi: 10.3389/fphys.2022.909870
Received: 31 March 2022; Accepted: 02 May 2022;
Published: 23 June 2022.
Edited by:
Prasanth Puthanveetil, Midwestern University, United StatesReviewed by:
Petr Stastny, Charles University, CzechiaMichinari Nakamura, Rutgers University, Newark, United States
Copyright © 2022 Schillemans, Tragante, Maitusong, Gigante, Cresci, Laguzzi, Vikström, Richards, Pilbrow, Cameron, Foco, Doughty, Kuukasjärvi, Allayee, Hartiala, Tang, Lyytikäinen, Nikus, Laurikka, Srinivasan, Mordi, Trompet, Kraaijeveld, van Setten, Gijsberts, Maitland-van der Zee, Saely, Gong, Johnson, Cooper-DeHoff, Pepine, Casu, Leiherer, Drexel, Horne, van der Laan, Marziliano, Hazen, Sinisalo, Kähönen, Lehtimäki, Lang, Burkhardt, Scholz, Jukema, Eriksson, Åkerblom, James, Held, Hagström, Spertus, Algra, de Faire, Åkesson, Asselbergs, Patel and Leander. This is an open-access article distributed under the terms of the Creative Commons Attribution License (CC BY). The use, distribution or reproduction in other forums is permitted, provided the original author(s) and the copyright owner(s) are credited and that the original publication in this journal is cited, in accordance with accepted academic practice. No use, distribution or reproduction is permitted which does not comply with these terms.
*Correspondence: Karin Leander, S2FyaW4uTGVhbmRlckBraS5zZQ==