- Biodemography of Aging Research Unit, Social Science Research Institute, Duke University, Durham, NC, United States
The ε4 allele of the APOE gene (APOE4) is known for its negative association with human longevity; however, the mechanism is unclear. APOE4 is also linked to changes in body weight, and the latter changes were associated with survival in some studies. Here, we explore the role of aging changes in weight in the connection between APOE4 and longevity using the causal mediation analysis (CMA) approach to uncover the mechanisms of genetic associations. Using the Health and Retirement Study (HRS) data, we tested a hypothesis of whether the association of APOE4 with reduced survival to age 85+ is mediated by key characteristics of age trajectories of weight, such as the age at reaching peak values and the slope of the decline in weight afterward. Mediation effects were evaluated by the total effect (TE), natural indirect effect, and percentage mediated. The controlled direct effect and natural direct effect are also reported. The CMA results suggest that APOE4 carriers have 19%–22% (TE p = 0.020–0.039) lower chances of surviving to age 85 and beyond, in part, because they reach peak values of weight at younger ages, and their weight declines faster afterward compared to non-carriers. This finding is in line with the idea that the detrimental effect of APOE4 on longevity is, in part, related to the accelerated physical aging of ε4 carriers.
1 Introduction
The ε4 allele of the APOE gene (APOE4) is known for its negative association with human longevity (Arbeev et al., 2011; Kulminski et al., 2011; Arbeev et al., 2012; Kulminski et al., 2014; Garatachea et al., 2015; Zeng et al., 2016; Yashin et al., 2018a; Yashin et al., 2018b; Abondio et al., 2019; Gurinovich et al., 2019; Kulminski et al., 2019; Santovito, Galli, and Ruberto, 2019; Sebastiani et al., 2019; Kulminski et al., 2022; Arbeev et al., 2023), although the mechanism is not well understood. Certain metabolic phenotypes (e.g., diabetes and serum lipids) were proposed as potential mediators; however, their mediating role in the association of APOE4 with longevity was not confirmed (Noordam et al., 2016). APOE4 was also linked to a lower weight and body mass index (BMI) and its changes with age (Bäckman et al., 2015; Bell et al., 2017; Blautzik et al., 2018; Kulminski et al., 2019; Ando et al., 2022; Ukraintseva et al., 2024). We recently demonstrated, using HRS and FHS data, that APOE4 carriers had lower weight than non-carriers starting approximately at age 65 and reached the maximum weight at younger ages compared to non-carriers, as well as declined in weight faster than non-carriers (Ukraintseva, et al., 2024).
Weight/BMI and their changes later in life were associated with survival in older ages (Lee et al., 2001; Køster-Rasmussen et al., 2016). In a study of post-menopausal women, it was found that weight loss, especially if unintentional, over a period of three to ten years, was associated with significantly lower chances of longevity (survival to ages over 90) compared to women with stable weights (Shadyab et al., 2023). Previously, we showed that entire age trajectories of weight/BMI can significantly differ between longer- and shorter-lived individuals (Yashin et al., 2013; Yashin et al., 2016); the shorter-lived individuals reached maximum values of weight/BMI and started to decline earlier in their lives compared to the longest living individuals. Trajectories of aging-related changes in weight may also differ between longer- and shorter-lived strains of laboratory rodents such that the longer-lived strains typically reach maximum values of weight later in their lives (Turturro et al., 1999). The decline in weight at older ages may reflect the physical and physiological changes in the body that occur with aging, such as the loss of muscle mass (sarcopenia) and reduction in food absorption, among others (Bales and Ritchie, 2002; Volpi, Nazemi, and Fujita, 2004; Woods, Iuliano-Burns, and Walker, 2011; Curtis et al., 2023). If an aging person reaches their peak weight at a younger age and subsequently experiences faster weight decline than their age peers, this could be an indicator of accelerated physical aging, which, in turn, may contribute to reduced chances of extreme longevity (Yashin et al., 2010; Yashin et al., 2013; Yashin et al., 2016).
This and other evidence indicate a possibility that some of the APOE4 effects on longevity might be attributed to the dynamics of aging-related changes in weight/BMI. Here, we explore the causal relationships between APOE4 carrier status, key characteristics of mean age trajectories of body weight (such as age at reaching maximum and the slope of decline), and survival to the oldest old age (85+), applying the causal mediation analysis (CMA) (Imai, Keele, and Tingley, 2010; Valeri and VanderWeele, 2013) to the Health and Retirement Study (HRS) data. We propose that APOE4 may influence age trajectories of the weight, and the negative effect of APOE4 on longevity could, in part, be because it promotes the earlier/faster decline of weight in older adults.
2 Materials and methods
2.1 Data
For this analysis, Health and Retirement Study data were used; the HRS is sponsored by the National Institute on Aging and is conducted by the University of Michigan. Beginning in 1992, the National Institute on Aging and the University of Michigan began collecting a variety of phenotypic information with a focus on aging and aging-related information on participants aged 50+ in the United States. Since then, it has grown and expanded to about 20,000 participants and is now one of the largest longitudinal studies of those aged 50+ in the United States. The participants are provided with physical informed consent information prior to each interview, and they are required to verbally consent. More details on the study design and sample selection can be found in Sonnega et al. (2014). Phenotype information was derived from the RAND HRS Longitudinal File (version 2016v1), which is an easy-to-use dataset based on the HRS core data. This file was developed at RAND with funding from the National Institute on Aging and the Social Security Administration. It contains a variety of information from the interviews of HRS participants, such as demographics, health, employment, and retirement.
For the present analysis, a subset of participants was selected: those who survived to at least age 80 and those with genotype information for APOE4. The APOE4 status was derived from imputed genotype information obtained from the database of Genotypes and Phenotypes (dbGaP) HRS data (dbGaP Study Accession: phs000428. v2. p2), which includes genotype information for 2.5 million SNPs collected using the Omni2.5 BeadChip. Further details regarding the characteristics of the participants in the entire HRS sample and the analytic sample can be found in Tables 1 and 2; comorbidity information can be found in these tables as well. Furthermore, we tested if there was a significant difference between the incidences of comorbidities among the groups in the mediators. We found there was no significant difference between occurrences of cancer nor cardiovascular disease among the groups for SlopeW and AgeMaxW.
2.2 Statistical analysis
In general, the mediation analysis concerns two main pathways: direct and indirect. Illustrated in Figure 1, the direct pathway is seen by the effect of the “treatment” (APOE4 carrier status in our case) on the outcome. The indirect path is illustrated by the indirect effect of the “treatment” on the outcome, as mediated through the mediator.
Mediation analysis measures the direct and indirect effects illustrated in Figure 1 based on an outcome model and a mediator model (Eq. 1). The general form of these models, respectively, is expressed as follows:
Specifically, the direct effect is measured by the effect of the treatment on the outcome
Additionally, there exists a measure to determine the amount of mediation, called the percentage mediated (PM), which is calculated by dividing the indirect effect by the total effect (Eq. 3):
This measures the percentage of the effect of the treatment on the outcome that can be attributed to the mediator. In order to claim “complete mediation,” the PM should be at least 80% (Kenny, 2021).
We performed causal mediation analysis, an extension of traditional mediation analysis (TMA) (Baron and David, 1986), in the HRS data analytic sample (see Table 2) using the SAS software application version 9.4. CMA utilizes a counterfactual framework to describe the direct, indirect, and total effect estimates using natural and controlled effects. In this analysis, for example, a counterfactual approach consists of evaluating the chances of survival for each individual at both “treatment” levels even though each individual can only have one “treatment” level (i.e., an individual cannot be a carrier and a non-carrier of APOE4). The potential outcome for all individuals is then averaged to calculate the overall potential outcome at each “treatment” level. A variety of potential outcomes at differing mediator and “treatment” levels is used to calculate specific CMA estimates. Additionally, CMA effects are not bound by parametric assumptions, unlike TMA effects (Rijnhart et al., 2021).
APOE4 status was used as a binary “treatment” variable in the CMA: (1) APOE4 carriers (“treatment” group) vs. (0) APOE4 non-carriers (control group). The longevity-related outcome, Survival85, was a binary variable defined as follows: (1) survival to age 85 years and above vs. (0) death before age 85. Two mediator variables characterizing weight were considered. The first variable, AgeMaxW, was defined as (1) age at the maximum weight ≥75 years vs. (0) age at the maximum weight <75 years and age at the last follow-up or death >80 years. The second variable, SlopeW, was defined as (1) relative weight change above the median (tend to have more stable weight during ages 65–80) vs. (0) relative weight change below the median (tend to be losing weight during ages 65–80). SlopeW was calculated as (mean value at ages 75–79 − mean value at ages 65–74)/ (mean value at ages 65–74) and dichotomized among individuals with the age at the last follow-up or death >80 years.
The SAS procedure PROC CAUSALMED (SAS Institute Inc, 2021) with the SAS software application version 9.4 was used for the entire analysis. As the outcome and mediators were binary variables, logistic regression models were used for the CMA. The trust-region optimization technique (“TRUREG” option) was used to obtain the maximum likelihood estimates. The codes used to generate the reported output are available upon request from the corresponding author.
For our CMA approach, we focused primarily on three causal effect estimates: total effect (TE), natural indirect effect (NIE), and percentage mediated (PM). In general, TE, a combination of both the direct and indirect effects, provides an estimate of the “treatment” effect on the outcome at the various mediator levels. Here, this estimate compares the chances of survival for APOE4 carriers vs. non-carriers while taking into account the effect of the mediator. NIE provides further details about the effects of the “treatment” through the mediator by holding the treatment constant and considering the effect of the mediator only. In this analysis, this estimate will compare the chances of survival for the allele carriers at each of the two mediator levels. PM gives the percentage of the total effect that is mediated by the mediator. Other reported estimates include the natural direct effect (NDE) and controlled direct effect (CDE). Although both of these estimates are useful, they were not the focus of this study as they hold the effect of the mediator constant, i.e., these estimates compare the chances of survival for the two “treatment” groups (carriers vs. non-carriers) without considering the effect of the mediator (SAS Institute Inc, 2021).
The CMA approach has four assumptions (Vander and Tyler, 2016): control must be made for (Assumption 1) exposure-outcome confounding, (Assumption 2) mediator-outcome confounding, and (Assumption 3) exposure-mediator confounding, as well as (Assumption 4) no mediator-outcome confounders being affected by the treatment. The following covariates were included in both the mediator and outcome models in order to control for confounding: smoking status (coded 0–never smoked and 1–ever smoked), education (coded 0–less than high school, 1–high school only, and 2–higher education), occurrence of cancer (coded 0–no cancer and 1–cancer), sex (coded 1–male and 2–female), and race (coded 1–White, 2–Black, and 3–Other). Furthermore, we followed A Guideline for Reporting Mediation Analyses (AGReMA) long-form checklist (Lee et al., 2021) (see Supplementary Table S1). See VanderWeele (2015) for additional details and methods.
In addition to reporting results for the total analytic sample, we provided stratified results by sex and race. Instead of creating sex and race subsamples, the “Evaluate” statement was used within PROC CAUSALMED. This allows users to specify covariate levels (such as sex and race) and evaluate the respective conditional causal effects. The “Evaluate” statement uses the entire analytic sample and estimates from the associated model to compute the mean of the specified covariate level(s). SAS documentation on PROC CAUSALMED provides further details (SAS Institute Inc, 2021). Additionally, we only used observations for which all variables involved in either of the models were non-missing (i.e., only those observations with information for “treatment,” outcome, mediator, and all covariates were used).
Furthermore, using the HRS data, we compared the mean trajectories of the weight for APOE4 carriers and non-carriers and the survival trajectories by different weight slopes and ages at reaching the maximum values of weight (Figure 2). These variables are proxies for the respective variables defined for BMI because height does not generally demonstrate substantial changes in individuals at ages over 80. Furthermore, a Cox proportional hazards p-value is provided on each plot as a measure of the significance of survival models. Cancer is included as a covariate in the Cox proportional hazards p-value.
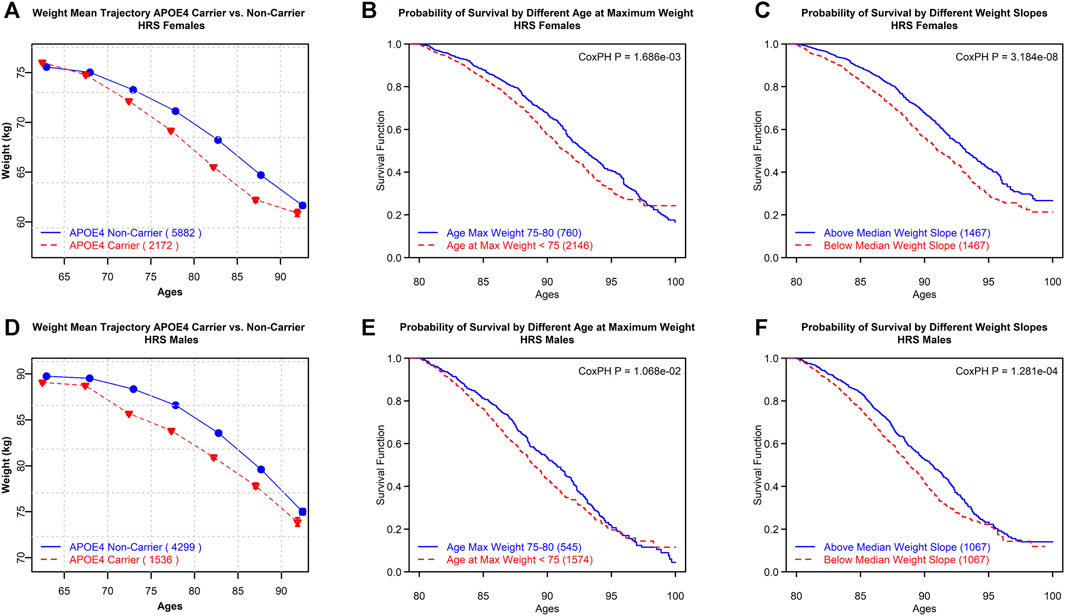
FIGURE 2. HRS females: (A) mean trajectories of body weight in APOE4 carriers vs. non-carriers. (B) Survival trajectories by different ages at reaching maximum values of weight. (C) Survival trajectories by different weight slopes. HRS males: (D) mean trajectories of body weight in APOE4 carriers vs. non-carriers. (E) Survival trajectories by different age categories at reaching maximum values of weight. (F) Survival trajectories by different weight slopes. Cox proportional hazards p-value (CoxPH P) is provided as a measure of the statistical significance of differences between survival curves in the respective groups.
Since the ε2 allele of the APOE gene is known for its beneficial effects on longevity (Wolters et al., 2019), we also conducted a sensitivity analysis with this allele removed. In other words, we compared those with the ε3/ε3 genotype (non-carriers) against those with ε3/ε4 or ε4/ε4 genotypes (carriers).
3 Results
The mean trajectories of the weight for APOE4 carriers and non-carriers, as well as survival trajectories by different weight slopes and different ages at reaching maximum values of the weight, are shown in Figure 2. These variables are proxies for similarly defined BMI variables as the height does not show substantial changes for those aged 80+. APOE4 carriers decline faster in weight with age compared to non-carriers (see Figures 2A and D). Survival was better for individuals with a less steep weight slope and older age at reaching the maximum values of weight (after age 75 vs. less than 75 years) (see Figures 2B, C, E, and F). One may notice the convergence in Figures 2B and E; this convergence is not unexpected. Although the HRS sample itself is not small, the number of people available at ages 95+ is substantially reduced. Furthermore, we tested if there was a significant difference between the incidences of comorbidities among the groups in all the plots in Figure 2. We found that there was no significant difference between the occurrences of cancer or cardiovascular disease among any of the groups.
AgeMaxW (below 75 years) was a significant mediator of the effects of the APOE alleles on survival to ages 85+ in the total sample and in samples stratified by sex and race. This was supported by the significant p-values for TE and NIE (see Table 3). TE estimates suggest that ε4 carriers are 19%–21% (p = 0.020–0.039) more likely to die before age 85 compared to non-carriers. If we hold the “treatment” constant and consider only the mediator effect, then APOE4 carriers who have a younger AgeMaxW are about 2.5% (p = 0.026–0.028) more likely to die before age 85 than the carriers who have an older AgeMaxW. The percentage of the total effect mediated by the AgeMaxW was around 14% for the overall sample and all strata, and while the PM was not statistically significant, it was marginally significant with p = 0.074–0.075.
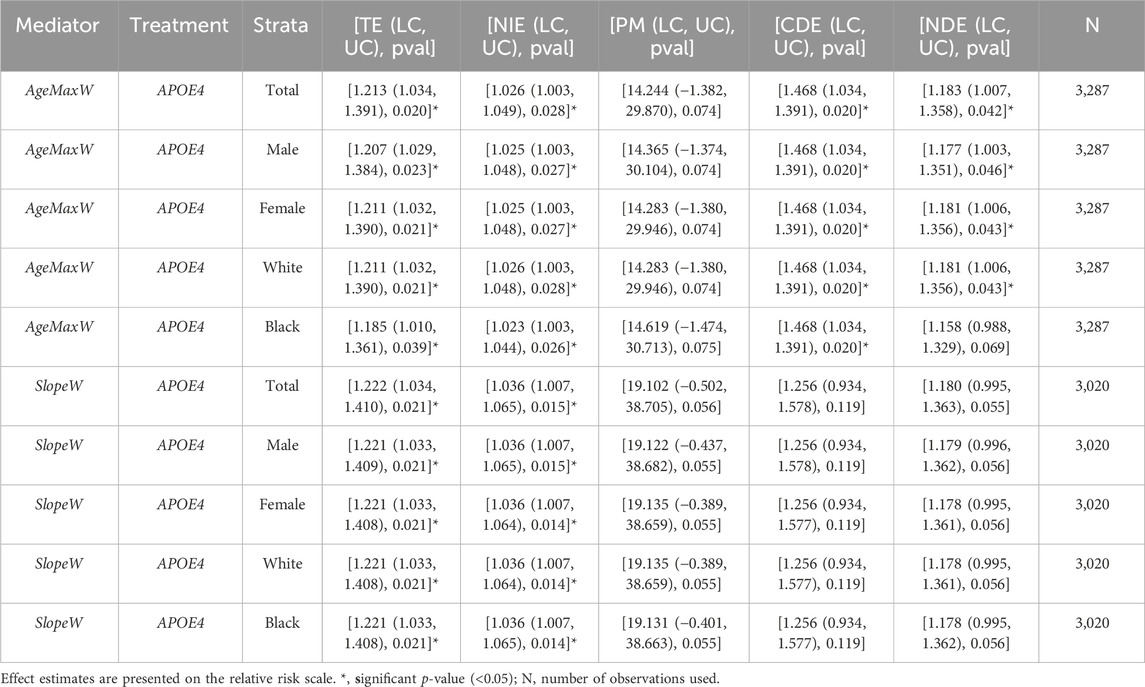
TABLE 3. Results of CMA for APOE4’s effect on survival up to age 85+ mediated by AgeMaxW and SlopeW. Results evaluated at different strata. [TE (LC, UC), pval]: [total effect estimate (lower 95% confidence interval and upper 95% confidence interval), p-value of total effect estimate], similarly for NIE (natural indirect effect), PM (percentage mediated), CDE (controlled direct effect), and NDE (natural direct effect). TE compares the chances of survival for APOE4 carriers vs. non-carriers while taking into account the effect of the mediator. NIE holds the treatment constant and considers the effect of the mediator only. PM gives the percentage of the total effect that is mediated by the mediator. NDE and CDE compare the chances of survival for the two “treatment” groups (carriers vs. non-carriers) without considering the effect of the mediator.
SlopeW was also a significant mediator of the APOE4 effects on survival for 85+ years in the total sample, with about a 4% higher (NIE: p-value = 0.014–0.015) (see Table 3) chance of death before age 85 when holding the “treatment” constant and considering the mediator’s effect only. TE estimates suggested that the chances of dying before age 85 were about 22% higher for APOE4 carriers vs. non-carriers (p-value = 0.021). A very similar effect was seen at all strata levels with similar p-values. The percentage of the total effect mediated by SlopeW, around 19%, was marginally significant (p = 0.055–0.056).
In our sensitivity analysis, which has the same framework as the initial analysis but with
4 Discussion
Findings of this CMA suggest that dynamics of aging-related changes in body weight mediates the effect of APOE4 on longevity. Specifically, APOE4 carriers have lower chances of survival to age 85+, and estimates suggest that 14%–19% of this association may be related to reaching the maximum weight at earlier ages. The APOE4 carriers were about 19%–22% more likely to die before age 85, compared to non-carriers, when considering the mediating effects of SlopeW and AgeMaxW. To the best of our knowledge, no studies have yet considered the patterns of aging-related changes in body weight over the life course as mediators of the negative association between APOE4 and longevity.
One may argue that the well-known beneficial effects of the ε2 allele of the APOE gene (Wolters et al., 2019) may contribute to the difference in survival chances seen in this CMA. To account for this, and to ensure that APOE4’s effect on longevity is indeed mediated by aging-related changes in body weight, we conducted a sensitivity analysis with the ε2 allele carriers removed from the “treatment” variable. This increased the effect size and significance of most TE and NIE estimates (Supplementary Table S2). Other estimates are relatively similar in effect size and significance, although they still vary from the original CMA results. This further suggests that the effect of APOE4 on longevity is partially mediated by aging-related changes in body weight, and the ε2 allele is not the source of the difference in survival chances seen in this CMA.
A prominent mechanism involved in many aging and longevity studies is lipids/cardiovascular disease(s). We did not consider lipids as a confounding factor as earlier studies (Kulminski et al., 2014; Tejedor et al., 2014; Loika et al., 2022; Ozen et al., 2022) do not support the connection between APOE and BMI through lipids nor cardiovascular disease. Furthermore, since cancer is a disease known to cause weight loss, we included cancer as a covariate to our analysis.
One should emphasize that the CMA that was implemented in this study has advantages over TMA. Since TMA effects are based on the coefficients of regression models, it is bound by parametric assumptions, whereas the natural effects from CMA are not. Additionally, TMA does not usually include an interaction between the treatment and mediator (Rijnhart et al., 2021). Another advantage is the use of a causal inference approach, which utilizes a counterfactual framework. Although TMA is a useful tool for detecting mediating effects and describing the total, direct, and indirect effects, CMA breaks these effects down even more, helping to better understand the underlying mechanism of the negative association between APOE4 and longevity.
Our results are in line with earlier studies that demonstrated a detrimental effect of APOE4 on longevity (Arbeev et al., 2011; Kulminski et al., 2011; Arbeev et al., 2012; Kulminski et al., 2014; Garatachea et al., 2015; Zeng et al., 2016; Yashin et al., 2018a; Yashin et al., 2018b; Abondio et al., 2019; Gurinovich et al., 2019; Kulminski et al., 2019; Santovito, Galli, and Ruberto, 2019; Sebastiani et al., 2019; Kulminski et al., 2022). They also suggested that APOE4 facilitates physical aging (manifested in an earlier and faster decline in weight) (Yashin et al., 2010), which, in turn, can causally contribute to reduced longevity. Previously, we showed that the longest-lived individuals reach peak values of weight/BMI and start to decline later in their lives compared to people with a conventional lifespan (Yashin et al., 2013; Yashin et al., 2016). The ability to grow in size and postpone weight loss until older ages may also correlate with better physical resilience to life stressors, which is essential for survival at the oldest ages (Ukraintseva et al., 2021).
A potential limitation to this study is that, while highly relevant covariates were included in both models (smoking status, education level, sex, and race), there still may exist unmeasured confounders, creating the potential for biased effect estimates. This CMA also revealed that AgeMaxW and SlopeW are factors that may explain only a part (albeit substantial) of the negative association between APOE4 and longevity because they did not meet the criteria to satisfy “complete mediation” (
In conclusion, this causal mediation analysis found that APOE4 carriers have lower chances of surviving to age 85 and beyond, in part, because they reach peak values of body weight at younger ages and decline faster afterward compared to non-carriers. Postponing and attenuating the decline in weight in APOE4 carriers could be a promising target of pro-longevity interventions.
Data availability statement
The data analyzed in this study are subject to the following licenses/restrictions: dbGaP and the University of Michigan Data Use Certification Agreement restrictions. Requests to access these datasets should be directed to the RAND HRS data provider RANDHRSHelp@rand.org. Access to the HRS genetic data is provided by the database of Genotypes and Phenotypes, dbGaP (Study accession: phs000428. v2. p2).
Ethics statement
The studies involving humans were approved by the Duke University Health System IRB. The studies were conducted in accordance with the local legislation and institutional requirements. Written informed consent for participation was not required from the participants or the participants’ legal guardians/next of kin in accordance with the national legislation and institutional requirements.
Author contributions
RH: formal analysis, visualization, writing–original draft, writing–review and editing, investigation, methodology, and software. HD: data curation, methodology, supervision, validation, and writing–review and editing. OB: data curation and writing–review and editing. DW: methodology and writing–review and editing. YL: data curation and writing–review and editing. AK: writing–review and editing. AY: data curation, funding acquisition, project administration, resources, supervision, and writing–review and editing. KA: data curation, methodology, resources, supervision, validation, writing–review and editing, and project administration. SU: conceptualization, funding acquisition, investigation, project administration, resources, supervision, writing–original draft, and writing–review and editing.
Funding
The author(s) declare that financial support was received for the research, authorship, and/or publication of this article. Research reported in this publication was supported by the National Institute on Aging of the National Institutes of Health (NIA/NIH) grants R01AG062623, R01AG070487, and RF1AG046860. The work of RH, KA, SU, HD, DW, and OB was partly supported by the NIA/NIH grant R01AG062623. The work of AY was partly supported by the NIA/NIH grants RF1AG046860 and R01AG070487. The work of AK was partly supported by the NIA/NIH grants R01AG070487 and R01AG070488. The work of YL was partly supported by the NIA/NIH grant R01AG070488. This content is solely the responsibility of the authors and does not necessarily represent the official views of the National Institutes of Health.
Acknowledgments
The Health and Retirement Study (HRS) is sponsored by the National Institute on Aging (grant number U01AG009740) and conducted by the University of Michigan. The HRS genetic data were sponsored by the National Institute on Aging (grants U01AG009740, RC2AG036495, and RC4AG039029) and collected by the University of Michigan.
Conflict of interest
The authors declare that the research was conducted in the absence of any commercial or financial relationships that could be construed as a potential conflict of interest.
Publisher’s note
All claims expressed in this article are solely those of the authors and do not necessarily represent those of their affiliated organizations, or those of the publisher, the editors, and the reviewers. Any product that may be evaluated in this article, or claim that may be made by its manufacturer, is not guaranteed or endorsed by the publisher.
Supplementary material
The Supplementary Material for this article can be found online at: https://www.frontiersin.org/articles/10.3389/fragi.2024.1359202/full#supplementary-material
References
Abondio, S., Garagnani, P., Boattini, A., Monti, D., Claudio Franceschi, , Luiselli, D., et al. (2019). The genetic variability of apoe in different human populations and its implications for longevity. Genes (Basel) 10 (3), 222. doi:10.3390/genes10030222
AndoUchida, T. K., Sugimoto, T., Kimura, A., Saji, N., Niida, S., and Sakurai, T. (2022). Apoe4 is associated with lower body mass, particularly fat mass, in older women with cognitive impairment. Nutrients 14 (3), 539. doi:10.3390/nu14030539
Arbeev, K. G., Bagley, O., Yashkin, A. P., Duan, H., Akushevich, I., Ukraintseva, S. V., et al. (2023). Understanding alzheimer's disease in the context of aging: findings from applications of stochastic process models to the health and retirement study. Mech. Ageing Dev. 211 (Apr), 111791. doi:10.1016/j.mad.2023.111791
Arbeev, K. G., Ukraintseva, S. V., Arbeeva, L. S., Akushevich, I., Kulminski, A. M., and Yashin, A. I. (2011). Evaluation of genotype-specific survival using joint analysis of genetic and non-genetic subsamples of longitudinal data. Biogerontology 12 (2), 157–166. doi:10.1007/s10522-010-9316-1
Arbeev, K. G., Ukraintseva, S. V., Kulminski, A. M., Akushevich, I., Arbeeva, L. S., Culminskaya, I. V., et al. (2012). Effect of the apoe polymorphism and age trajectories of physiological variables on mortality: application of genetic stochastic process model of aging. Sci. (Cairo) 2012, 568628. doi:10.6064/2012/568628
BäckmanJoas, K. E., Waern, M., Östling, S., Guo, X., Blennow, K., Skoog, I., et al. (2015). 37 Years of body mass index and dementia: effect modification by the apoe genotype: observations from the prospective population study of women in gothenburg, Sweden. J. Alzheimer's Dis. 48 (4), 1119–1127. doi:10.3233/JAD-150326
Bales, C. W., and Ritchie, C. S. (2002). Sarcopenia, weight loss, and nutritional frailty in the elderly. Annu. Rev. Nutr. 22, 309–323. doi:10.1146/annurev.nutr.22.010402.102715
Baron, R. M., and David, A. K. (1986). The moderator-mediator variable distinction in social psychologicalresearch: conceptual, strategic, and statistical considerations. J. Personality Soc. Psychol. 51 (6), 1173–1182. doi:10.1037//0022-3514.51.6.1173
BellDandan, S. P. L., Lauren, R. S., Avantika, A. S., Katherine, A. G., Hohman, T. J., and Jefferson, A. L. (2017). Late-life body mass index, rapid weight loss, apolipoprotein E Ε4 and the Risk of cognitive decline and incident dementia. J. Nutr. Health Aging 21 (10), 1259–1267. doi:10.1007/s12603-017-0906-3
Blanchard, J. W., Akay, L. A., Davila-Velderrain, J., von Maydell, D., Mathys, H., Davidson, S. M., et al. (2022). Apoe4 impairs myelination via cholesterol dysregulation in oligodendrocytes. Nature 611 (7937), 769–779. doi:10.1038/s41586-022-05439-w
Blautzik, J., Kotz, S., Brendel, M., Julia Sauerbeck, , Vettermann, F., Winter, Y., Bartenstein, P., and Alzheimer’s Disease Neuroimaging Initiative, (2018). Relationship between body mass index, Apoe4 status, and pet-based amyloid and neurodegeneration markers in amyloid-positive subjects with normal cognition or mild cognitive impairment. J. Alzheimers Dis. 65 (3), 781–791. doi:10.3233/JAD-170064
Curtis, M., Swan, L., Fox, R., Warters, A., and O'Sullivan, M. (2023). Associations between body mass index and probable sarcopenia in community-dwelling older adults. Nutrients 15, 1505–1506. (Mar 21). doi:10.3390/nu15061505
Garatachea, N., Marín, P. J., Santos-Lozano, A., Sanchis-Gomar, F., Emanuele, E., and Lucia, A. (2015). The apoe gene is related with exceptional longevity: a systematic review and meta-analysis. Rejuvenation Res. 18 (1), 3–13. doi:10.1089/rej.2014.1605
Gurinovich, A., Anastasia, S. L., Puca, A., Gil, A., Barzilai, N., and Paola, S. (2019). Varying effects of apoe alleles on extreme longevity in European ethnicities. J. Gerontol. A Biol. Sci. Med. Sci. 74 (Suppl. 1), S45–S51. doi:10.1093/gerona/glz179
Imai, K., Keele, L., and Tingley, D. (2010). "A general approach to causal mediation analysis." Psychol. Methods 15 (4), 309–334. doi:10.1037/a0020761
Kenny, D. A. (2021). Mediation. Form of Item https://davidakenny.net/cm/mediate.htm (Accessed July 18, 2022).
Køster-RasmussenKildevæld Simonsen, R. M., Siersma, V., Jan Erik, H., Berit Lilienthal, H., and Niels de Fine, O. (2016). Intentional weight loss and longevity in overweight patients with type 2 diabetes: a population-based cohort study. PLoS One 11 (1), e0146889. doi:10.1371/journal.pone.0146889
Kulminski, A. M., Arbeev, K. G., Culminskaya, I., Arbeeva, L., Ukraintseva, S. V., Stallard, E., et al. (2014). Age, gender, and cancer but not neurodegenerative and cardiovascular diseases strongly modulate systemic effect of the apolipoprotein E4 allele on lifespan. PLoS Genet. 10 (1), e1004141. doi:10.1371/journal.pgen.1004141
Kulminski, A. M., Culminskaya, I., Ukraintseva, S. V., Arbeev, K. G., Arbeeva, L., Wu, D., et al. (2011). Trade-off in the effects of the apolipoprotein E polymorphism on the ages at onset of cvd and cancer influences human lifespan. Aging Cell 10 (3), 533–541. doi:10.1111/j.1474-9726.2011.00689.x
Kulminski, A. M., Jain-Washburn, E., Philipp, I., He, L., Loika, Y., Loiko, E., et al. (2022). APOE ɛ4 allele and TOMM40-APOC1 variants jointly contribute to survival to older ages. Aging Cell 21 (12), e13730. doi:10.1111/acel.13730
Kulminski, A. M., Loika, Y., Culminskaya, I., Huang, J., Arbeev, K. G., Bagley, O., et al. (2019). Independent associations of Tomm40 and apoe variants with body mass index. Aging Cell 18 (1), e12869. doi:10.1111/acel.12869
Lee, H., Cashin, A. G., Lamb, S. E., Hopewell, S., Vansteelandt, S., VanderWeele, T. J., et al. (2021). A guideline for reporting mediation analyses of randomized trials and observational studies: the agrema statement. JAMA 326 (21), 1045–1056. 11. doi:10.1001/jama.2021.14075
Lee, I. M., Blair, S. N., Allison, D. B., Folsom, A. R., Harris, T. B., Manson, J. E., et al. (2001). Epidemiologic data on the relationships of caloric intake, energy balance, and weight gain over the life span with longevity and morbidity. J. Gerontol. A Biol. Sci. Med. Sci. 1 (Mar), 7–19. doi:10.1093/gerona/56.suppl_1.7
Loika, Y., Feng, F., Loiko, E., and Kulminski, A. M. (2022). Mediation of the apoe associations with alzheimer's and coronary heart diseases through body mass index and lipids. Geroscience 44 (2), 1141–1156. doi:10.1007/s11357-021-00458-3
NoordamOudt, R. C. H., Deelen, J., Slagboom, P. E., Beekman, M., and Diana van Heemst, (2016). Assessment of the contribution of apoe gene variants to metabolic phenotypes associated with familial longevity at middle age. Aging (Albany NY) 8 (8), 1790–1801. doi:10.18632/aging.101017
Ozen, E., Mihaylova, R. G., Lord, N. J., Lovegrove, J. A., and Jackson, K. G. (2022). Association between APOE genotype with body composition and cardiovascular disease Risk markers is modulated by BMI in healthy adults: findings from the BODYCON study. Int. J. Mol. Sci. 23 (29), 9766. doi:10.3390/ijms23179766
Rijnhart, V., Judith, J. M., Smyth, H. L., and MacKinnon, D. P. (2021). Statistical mediation analysis for models with a binary mediator and a binary outcome: the differences between causal and traditional mediation analysis. Prev. Sci. 24, 408–418. doi:10.1007/s11121-021-01308-6
Santovito, A., Galli, G., and Ruberto, S. (2019). Evaluation of the possible association of body mass index and four metabolic gene polymorphisms with longevity in an Italian cohort: a role for APOE, eNOS and FTO gene polymorphisms. Ann. Hum. Biol. 46 (5), 425–429. doi:10.1080/03014460.2019.1659413
SebastianiGurinovich, P. A., Nygaard, M., Sasaki, T., Sweigart, B., Bae, H., Andersen, S. L., et al. (2019). Apoe alleles and extreme human longevity. J. Gerontol. A Biol. Sci. Med. Sci. 74 (1), 44–51. doi:10.1093/gerona/gly174
Shadyab, A. H., Manson, J. E., Allison, M. A., Laddu, D., Wassertheil-Smoller, S., Van Horn, L., et al. (2023). Association of later-life weight changes with survival to ages 90, 95, and 100: the women's health initiative. J. Gerontol. A Biol. Sci. Med. Sci. 78, 2264–2273. doi:10.1093/gerona/glad177
Sonnega, A., Faul, J. D., Beth Ofstedal, M., Langa, K. M., Phillips, J. W. R., and Weir, D. R. (2014). Cohort profile: the health and retirement study (hrs). Int. J. Epidemiol. 43 (2), 576–585. doi:10.1093/ije/dyu067
Tejedor, M. T., Garcia-Sobreviela, M. P., Ledesma, M., and Arbones-Mainar, J. M. (2014). The apolipoprotein E polymorphism Rs7412 associates with body fatness independently of plasma lipids in middle aged men. PLoS One 9 (9), e108605. doi:10.1371/journal.pone.0108605
Turturro, A., Witt, W. W., Lewis, S., Hass, B. S., Lipman, R. D., and Hart, R. W. (1999). Growth curves and survival characteristics of the animals used in the biomarkers of aging program. J. Gerontol. A Biol. Sci. Med. Sci. 54 (11), B492–B501. doi:10.1093/gerona/54.11.b492
Ukraintseva, S., Duan, H., Holmes, R., Bagley, O., Wu, D., Yashkin, A., et al. (2024). "Patterns of aging changes in bodyweight may predict alzheimer’s disease." J. Alzheimer's Dis. 97 (1), 163–170. doi:10.3233/JAD-220998
Ukraintseva, S., Duan, M., Arbeev, K., Wu, D., Bagley, O., Yashkin, A. P., et al. (2021). Interactions between genes from aging pathways may influence human lifespan and improve animal to human translation. Front. Cell Dev. Biol. 9, 692020. doi:10.3389/fcell.2021.692020
Valeri, L., and VanderWeele, T. J. (2013). "Mediation analysis allowing for exposure-mediator interactions and causal interpretation: theoretical assumptions and implementation with sas and spss macros." Psychol. Methods 18 (2), 137–150. doi:10.1037/a0031034
VanderWeele, T. (2015). Explanation in causal inference: methods for mediation and interaction. Oxford University Press. https://books.google.com/books?id=K6cgBgAAQBAJ.
Vander, W., and Tyler, J. (2016). "Mediation analysis:A practitioner’s guide." Annu. Rev. Public Health 37, 17–32. doi:10.1146/annurev-publhealth-032315-021402
Volpi, E., Nazemi, R., and Fujita, S. (2004). Muscle tissue changes with aging. Curr. Opin. Clin. Nutr. Metab. Care 7 (4), 405–410. doi:10.1097/01.mco.0000134362.76653.b2
Wolters, F. J., Yang, Q., Biggs, M. L., Jakobsdottir, J., Li, S., Evans, D. S., et al. (2019). The impact of apoe genotype on survival: results of 38,537 participants from six population-based cohorts (E2-Charge). PLoS One 14 (7), e0219668. E2-CHARGE investigators. doi:10.1371/journal.pone.0219668
Woods, J. L., Iuliano-Burns, S., and Walker, K. Z. (2011). Weight loss in elderly women in low-level care and its association with transfer to high-level care and mortality. Clin. Interv. Aging 6, 311–317. doi:10.2147/CIA.S27334
Yashin, A., Arbeeva, L. S., Arbeev, K. G., Akushevich, I., Kulminski, A. M., Stallard, E., et al. (2016). “Age trajectories of physiological indices: which factors influence them?,” in The springer series on demographic methods and population analysis (Dordrecht: Springer), 40. Biodemography of Aging.
Yashin, A. I., Arbeev, K. G., Akushevich, I., Arbeeva, L., Kravchenko, J., Il'yasova, D., et al. (2010). Dynamic determinants of longevity and exceptional health. Curr. Gerontol. Geriatr. Res. 2010, 381637. doi:10.1155/2010/381637
Yashin, A. I., Arbeev, K. G., Wu, D., Arbeeva, L. S., Bagley, O., Stallard, E., et al. (2018a). Genetics of human longevity from incomplete data: new findings from the long life family study. J. Gerontol. A Biol. Sci. Med. Sci. 73 (11), 1472–1481. doi:10.1093/gerona/gly057
Yashin, A. I., Arbeev, K. G., Wu, D., Arbeeva, L. S., Kulminski, A., Akushevich, I., et al. (2013). How lifespan associated genes modulate aging changes: lessons from analysis of longitudinal data. Front. Genet. 4, 3. doi:10.3389/fgene.2013.00003
Yashin, A. I., Fang, F., Kovtun, M., Wu, D., Duan, M., Arbeev, K., et al. (2018b). Hidden heterogeneity in alzheimer's disease: insights from genetic association studies and other analyses. Exp. Gerontol. 107 (Jul 01), 148–160. doi:10.1016/j.exger.2017.10.020
Zeng, Y., Nie, C., Min, J., Liu, X., Li, M., Chen, H., et al. (2016). Novel loci and pathways significantly associated with longevity. Sci. Rep. 6 (25), 21243. doi:10.1038/srep21243
Keywords: mediation, longevity, causal inference, ApoE4, aging, weight
Citation: Holmes R, Duan H, Bagley O, Wu D, Loika Y, Kulminski A, Yashin A, Arbeev K and Ukraintseva S (2024) How are APOE4, changes in body weight, and longevity related? Insights from a causal mediation analysis. Front. Aging 5:1359202. doi: 10.3389/fragi.2024.1359202
Received: 21 December 2023; Accepted: 07 February 2024;
Published: 01 March 2024.
Edited by:
Marcella Reale, University of Studies G. d’Annunzio Chieti and Pescara, ItalyReviewed by:
Serena Dato, University of Calabria, ItalyMichael Belloy, Washington University in St. Louis, United States
Copyright © 2024 Holmes, Duan, Bagley, Wu, Loika, Kulminski, Yashin, Arbeev and Ukraintseva. This is an open-access article distributed under the terms of the Creative Commons Attribution License (CC BY). The use, distribution or reproduction in other forums is permitted, provided the original author(s) and the copyright owner(s) are credited and that the original publication in this journal is cited, in accordance with accepted academic practice. No use, distribution or reproduction is permitted which does not comply with these terms.
*Correspondence: Svetlana Ukraintseva, c3ZvQGR1a2UuZWR1