Proteomics reveals pathways linked to septoria canker resistance and susceptibility in Populus trichocarpa
- 1Botany and Plant Pathology, Oregon State University, Corvallis, OR, United States
- 2U.S. Department of Energy, Office of Science, Office of Workforce Development for Teachers and Scientists, Office of Science Graduate Student Research, Oak Ridge, TN, United States
- 3Biosciences Division, Oak Ridge National Laboratory, Oak Ridge, TN, United States
- 4Genome Science and Technology, University of Tennessee-Knoxville, Knoxville, TN, United States
- 5Invaio Sciences, Cambridge, MA, United States
- 6Forest Resources, Engineering and Management Department, Oregon State University, Corvallis, OR, United States
A major threat to forest ecosystems and plantation forestry is the introduction of a non-native pathogen. Among non-domesticated populations with relatively high levels of genetic diversity, a measurable range of susceptibility to resistance can be expected. Identifying genetic determinants of resistant and susceptible individuals can inform the development of new strategies to engineer disease resistance. Here we describe pathogen-induced changes in the proteome of Populus trichocarpa stem tissue in response to Sphaerulia musiva (Septoria canker). This hemibiotrophic fungal pathogen causes stem canker disease in susceptible poplar genotypes. Proteomics analyses were performed on stem tissue harvested across 0-, 12-, 24- and 48-h post-inoculation with Septoria from three genotypes including one resistant (BESC-22) and two susceptible [BESC-801; Nisqually-1 (NQ-1)]. In total, 11,897 Populus proteins at FDR <0.01 were identified across all time points and genotypes. Analysis of protein abundances between genotypes revealed that the resistant poplar genotype (BESC-22) mounts a rapid and sustained defense response involving pattern recognition receptors, calcium signaling proteins, SAR inducers, transcriptional regulators, resistance proteins, and proteins involved with the hypersensitive response. One susceptible genotype (BESC-801) had a downregulated and delayed defense response whereas the second susceptible genotype (NQ-1) lacked a distinct pattern. Overall, the proteome-wide and protein-specific trends suggest that responses to the Septoria canker infection are genotype-specific for the naïve host, Populus trichocarpa.
1 Introduction
Plants have constitutive defenses such as cuticle, bark, cell walls, and preformed bioactive compounds that inhibit most microbes. If a pathogen overcomes these barriers, a resistant plant needs to perceive the invasion promptly in order to best regulate the appropriate defense responses (Couto and Zipfel, 2016; Pruitt et al., 2021). In pattern-triggered immunity (PTI), perception of pathogen-associated molecular patterns (PAMPs) is commonly achieved using cell surface receptors and receptor kinases that activate a response. This perception and signaling can lead to an influx of calcium ions, a burst of reactive oxygen species (ROS), transcriptional reprogramming, phytohormone signaling [e.g., salicylic acid (SA), jasmonic acid (JA), ethylene], and the synthesis of other secondary metabolites which are involved with plant defense (Bigeard et al., 2015). Understanding these signaling pathways in forest trees and how they differ among genotypes in response to pathogens is an important step towards advancing sustainable agroforestry and preserving natural ecosystems.
Poplars are an important source of pulpwood, lumber, and bioenergy due to their rapid growth, ease of propagation, and adaptability to a range of edaphic conditions (Bryant et al., 2020). The biomass yield and sustainability of poplars as a bioenergy feedstock is limited due to pests and pathogens such as Sphaerulina musiva (syn. = Septoria musiva) which causes Septoria leaf spot and stem canker disease (Bier, 1939; Waterman, 1955; Feau et al., 2010). Four genes in Populus trichocarpa are hypothesized to mediate Septoria canker disease resistance and/or susceptibility (Muchero et al., 2018). Co-expression of these resistance genes with others known to be involved with PTI peaked at 24 h post-inoculation (hpi) in the resistant genotype BESC-22 (Jones and Dangl, 2006; Muchero et al., 2018). In two other studies, genes encoding pathogenesis-related (PR) proteins, chitinases, and proteins involved with cell wall remodeling were upregulated in Septoria canker-resistant poplars within the first 48hpi (Foster et al., 2015; Abraham et al., 2019). This accumulated evidence led to the hypothesis that resistant genotypes are able to rapidly recognize the pathogen and mount a sustained defense response.
In contrast to the resistant genotypes, susceptible poplar trees did not upregulate the disease-resistance marker genes, described above (Muchero et al., 2018). The susceptible genotype, BESC-801 upregulated a G-type lectin receptor kinase (G-type LecRLK) linked to susceptibility (Muchero et al., 2018). The lectin domain of the G-type LecRLK protein was found to specifically interact with a carbohydrate originating from the fungal cell wall of S. musiva correlating a fungal-specific response with downregulation of resistance genes. This apparent suppression of the disease response may explain the delayed/partial production of physical barriers initiated as part of the host response (Weiland and Stanosz, 2007; Qin and LeBoldus, 2014). This observation is supported by a metabolomics study which noted metabolites involved with systemic acquired resistance (SAR), cell cycle regulation, and secondary cell wall biosynthesis were significantly upregulated in a resistant genotype but were absent in the susceptible genotype. In addition to these patterns, S. musiva produced metabolites hypothesized to be capable of suppressing defense mechanisms (Lenz et al., 2021).
Typically, co-evolved plant-microbe interactions are mediated by a diversity of mechanisms in the host, whereas non-co-evolved interactions involve narrow and exapted mechanisms mediated by a small number of extracellular receptors and PTI (Potts et al., 2016; Ayliffe and Sørensen, 2019; Bartholomé et al., 2020). The P. trichocarpa—S. musiva interaction is non-co-evolved because S. musiva is endemic to southeastern United States and co-evolved with P. deltoides. Recently, S. musiva has been detected beyond its native range into the Pacific Northwest raising concerns for its potential impact on poplars in that region (Callan et al., 2007; Sondreli et al., 2020). Populus trichocarpa contributes genes leading to dominant susceptibility in hybrids with the resistant poplar, P. deltoides (Newcombe and Bradshaw Jr, 1996; Newcombe and Ostry, 2001). Non-host immunity in most P. trichocarpa genotypes must be suppressed by S. musiva leading to colonization and degradation of the stem tissue (Dhillon et al., 2015). The diversity of responses within genotypes sharing the same phenotype have yet to be described. Herein, a quantitative proteomics approach was used to characterize similarities and differences in protein-level responses among one resistant and two susceptible P. trichocarpa genotypes to S. musiva inoculation.
Quantitative proteomics has been used to study the dynamic regulation and accumulation of proteins involved with abiotic stress responses as well as growth and development in poplar trees (Abraham et al., 2018; Abril et al., 2011; Cheng et al., 2015; Y. Li Y et al., 2019; Rampitsch and Bykova, 2012; Trupiano et al., 2014). Given the potential involvement of SAR and PTI in Septoria canker resistance, we hypothesized that proteins linked to these signaling pathways would be prominent during the early interaction with S. musiva in the resistant genotype and that the abundance of these proteins would be reduced in the susceptible lines. To this end, we employed a bottom-up proteomics approach to examine the molecular and temporal dynamics in Septoria canker-resistant and susceptible poplars at 0, 12, 24, and 48 hpi. Our objectives were to: 1) identify the proteome-wide changes within each genotype; 2) compare the changes between resistant and susceptible genotypes; and 3) develop a conceptual model describing the responses, at the proteome level, of the resistant and susceptible genotypes.
2 Materials and methods
2.1 Plant propagation
Three P. trichocarpa genotypes with varying levels of disease resistance to Septoria canker were micropropagated for this experiment. These included a resistant genotype, BESC-22, and two susceptible genotypes, BESC-801 and Nisqually-1 (NQ-1) (Tuskan et al., 2006; Muchero et al., 2018). These three genotypes were micro-propagated in propagation media (PM) composed of Murashige and Skoog (MS) media with vitamins (Caisson Labs Inc.) supplemented with 2.5% sucrose, 2.5 g/L charcoal, 3 g/L Gelzan, 0.005 mg/L 6-BA, adjusted to a final pH of 5.7. Sterile plantlets were micro-propagated in 4-week increments by removing the shoot tips and transferring them to fresh media until enough plantlets were produced for the experiment.
2.2 Isolate propagation and inoculation
Frozen plugs of the Sphaerulina musiva isolates MN-14, MN-5, MN-19, and MN-20 (Dunnell and LeBoldus, 2017) were poured onto Petri plates containing sterilized KV-8 media [2 g calcium carbonate, 20 g agar, 820 ml deionized water, and 180 ml V-8 vegetable juice (Campbell Soup Company, Camden, NJ, United States)] with 240 mg/L chloramphenicol (Chloramphenicol USP, Amresco, OH, United States) and 100 mg/L streptomycin (Streptomycin Sulfate USP, Amresco, OH, United States) (Stanosz and Stanosz, 2002). Plugs of sporulating mycelia were subcultured to fresh KV-8 plates. Approximately 7 days after subculture, conidia were dislodged from the plates using a sterile inoculation loop and 1 ml of sterile distilled water. The spore concentration for each isolate was measured using an automated cell counter (Countess 3, Thermo Fisher Scientific). All isolate suspensions were adjusted to a concentration of 1 × 106 spores per mL before mixing them in equal volumes to create the final bulk spore suspension for inoculations (LeBoldus et al., 2010).
2.3 Experimental design
Three Populus trichocarpa genotypes with varying levels of disease resistance to Septoria stem canker (causal agent, Sphaerulina musiva) were grown together in a completely randomized arrangement within 32 oz PhytoCon (115 mm × 135 mm) plant tissue culture vessels (PhytoTech Labs, Lenexa, KS). A total of 18 tissue culture vessels were organized as a completely random design for the different treatments. The plants in 4 vessels were sprayed with sterile water (∼0.5 ml per tree) for a mock inoculation control. The remaining trees in the other 14 vessels were sprayed with 0.5 ml per tree of S. musiva spore suspension. A total of 3 vessels with one tree from each genotype were harvested at 0 h post-inoculation (hpi) by separating leaf and stem tissue for each genotype before flash freezing in liquid nitrogen and storing at −80°C. Another group of 3 vessels were harvested in the same way at 12 hpi and flash frozen. At 24 hpi, 4 mock-inoculated and 4 spore-inoculated vessels were harvested. The last group of plants in 4 vessels were harvested at 48 hpi.
2.4 Protein extraction and processing
2.4.1 Homogenization and cell lysis
All samples were subjected to the same protein extraction method consisting of four major steps: cryogenic tissue homogenization/pulverization, cell lysis, chloroform methanol extraction (CME), and trypsin digestion. For the cryogenic pulverization, approximately 100 mg of the frozen stem tissue was placed in pre-chilled 5 ml Geno/Grinder tubes. The samples were pulverized at 1000 rpm for 60 s in a Geno/Grinder 2010 (Spex® SamplePrep). Subsequently, 1.5 ml of SDS lysis buffer (4% SDS, 10 mM dithiothreitol (DTT), 100 mM Ammonium bicarbonate (ABC), and 1X Halt™ Protease Inhibitor Cocktail (Thermo Scientific, Waltham, MA)) was added to the tubes before incubating at 85°C for 10 min. Cell membranes were disrupted in the Geno-grinder set to 1000 rpm for 60 s. The samples were then incubated for 5 more minutes at 85°C. The cell lysates were transferred to 2 ml microcentrifuge tubes and then spun down at 12,000 rpm for 5 min. 1 ml of supernatant for each sample was transferred to fresh 15 ml tubes before adding 0.5 M Iodoacetamide (IAA) (MilliporeSigma) to a final concentration of 30 mM. The solution was vortexed briefly and then incubated in the dark for 15 min at room temperature. The proteins were then isolated from the lysates using CME (Jiang et al., 2004). The pellet was washed twice with methanol and then air dried before dissolving in 1 ml of trypsin digest buffer (100 mM ABC buffer with 2% sodium deoxycholate (SDC)) using a vortex. The dissolved proteins were quantified using the SCOPES method on a NanoDrop ONE and then diluted to a concentration of 0.5 ug/ul. A total of 200 ug (400 uL) was transferred to a fresh 2 ml tube for trypsin digestion.
2.4.2 Trypsin digestion
Lyophilized trypsin protease (Pierce™ Trypsin Protease, MS Grade) was resuspended in 50 mM acetic acid to 0.5 ug/uL. A total of 2.85 ug of trypsin was added to each sample at a 1:70 ratio and left for 3 h on a shaker incubator set at 37°C and 1000 rpm. A second trypsin digest was conducted overnight after doubling the volume of each sample using ABC buffer without SDC and another 2.85 ug of trypsin. After each set of incubations, formic acid (FA) was added at a 1% v:v to halt the proteolysis and precipitate the SDC. The samples were centrifuged at the maximum speed for 5 min and the supernatant was transferred to a new 2 ml tube. The supernatant was then mixed with 1 ml water-saturated ethyl acetate to remove the remaining SDC. Samples were centrifuged at the maximum speed for 5 min. The peptide layer was aspirated using a 1 ml tip attached to a 10uL tip and transferred to a new 2 ml tube. The peptide mixture was dried using a Speedvac centrifuge. The dried pellet was resuspended in 0.1% FA water and quantified before transferring to autosampler vials for LC-MS.
2.4.3 LC-MS/MS
Samples were analyzed using two-dimensional (2D) liquid chromatography (LC) on an Ultimate 3000 RSLCnano system (Thermo Fischer Sci., United States) coupled with a Q Exactive Plus mass spectrometer (Thermo Fischer Sci., United States). For each sample, 5 ug of tryptic peptides were injected across an in-house built strong cation exchange (SCX) Luna trap column (5 μm, 150 µm × 50 mm; Phenomenex, United States) followed by a nanoEase symmetry reverse phase (RP) C18 trap column (5 μm, 300 μm × 50 mm; Waters, United States) and washed with an aqueous solvent. Peptide mixtures were separated and analyzed across three successive SCX fractions of increasing concentrations of ammonium acetate (35mM, 50mM, and 500 mM), each followed by a 100-min organic gradient (25 nL/min flow rate) to separate peptides across an in-house pulled nanospray emitter analytical column (75 μm × 350 mm) packed with Kinetex RP C18 resin (1.7 µm; Phenomenex, United States). All MS data were acquired with Thermo Xcalibur (version 4.2.47) using the topN method where N could be up to 10. Target values for the full scan MS spectra were 3 × 106 charges in the 300—1,500 m/z range with a maximum injection time of 25 ms. Transient times corresponding to a resolution of 70,000 at m/z 200 were chosen. A 1.6 m/z isolation window and fragmentation of precursor ions were performed by higher-energy C-trap dissociation (HCD) with a normalized collision energy of 27 eV. MS/MS scans were performed at a resolution of 17,500 at m/z 200 with an ion target value of 1 × 105 and a maximum injection time of 50 ms. Dynamic exclusion was set to 20 s to avoid repeated sequencing of peptides. All MS raw data files were analyzed using the Proteome Discover software (version 2.4, Thermo Fischer Sci., United States). Each MS raw data file was processed by the SEQUEST HT database search algorithm (Eng et al., 1994) and confidence in peptide-to-spectrum (PSM) matching was evaluated by Percolator (Käll et al., 2007). Peptide and PSMs were considered identified from the Populus trichocarpa v3.1 proteome (Tuskan et al., 2006) or the Sphaerulina musiva proteome (Ohm et al., 2012; Dhillon et al., 2015) at q < 0.01. Protein relative abundance values were calculated by summing together peptide extracted ion chromatograms. Proteins with false discovery rate <1% and at least 2 peptides of evidence were exported from Proteome Discoverer.
2.5 Data filtering and normalization
Protein data for each treatment group were first filtered to remove common contaminant proteins. Proteins belonging to alternative transcripts that lacked unique peptides were considered ambiguous, removed, and instead represented by only the primary transcript. Replicate 3 of the mock inoculation treatment for BESC-22 was not used due to the poor growth of the starting plant material which correlated with a reduced number of proteins identified compared to the other 3 replicates.
Each genotype file was uploaded to InfernoRDN (Polpitiya et al., 2008) for log2 transformation and normalization (Supplementary Figure S1). Log2 values were normalized across treatment group replicates using Locally Estimated Scatterplot Smoothing (LOESS), with a window width span value of 0.2 (Karpievitch et al., 2012; Vu et al., 2018), and mean-centered across the experimental conditions. Normalized protein data tables were further processed by the software Perseus (Tyanova et al., 2016) to identify differentially expressed proteins within each genotype group. Proteins identified in a single replicate were filtered out before imputing the missing data. Imputation was done by replacing missing values from a normal distribution 2.5 standard deviations down from the total data matrix mean and 0.3 of its width (Tyanova et al., 2016). This set of data matrices was used to compare the mock controls to the different timepoints. Temporal changes in protein abundance across the four time points were also compared. For this analysis, mock inoculation data and proteins that did not have at least 2 values across timepoints in the pre-imputed dataset were discarded.
2.6 Statistical analysis
2.6.1 Comparisons to the mock 24 hpi control
Principal component analysis (PCA) was conducted to characterize the overall variation and identify clusters based on treatment group within genotype (Supplementary Figure S2). In addition, a Pearson correlation analysis was conducted using the default parameters in Perseus to calculate correlation coefficients between replicates of the same timepoints and between replicates sampled at different timepoints. Subsequently, the relative protein abundances were calculated by subtracting the mock control sample mean (log2) from the 24 hpi sample mean (log2). Two-tailed t-tests were used to compare protein abundance and significance was assessed at an alpha of 0.05. The relative change in abundance of protein products of the four genes significantly associated with Septoria canker disease resistance and/or susceptibility (Potri.005G012100, Potri.003G036300, Potri.009G028200, Potri.005G018000) (Muchero et al., 2018) evaluated for consistency with previous gene expression results.
2.6.2 Differentially abundant proteins—ANOVA and hierarchical clustering
One-way ANOVA was used to determine differentially abundant proteins across the 0, 12, 24 and 48 hpi timepoints. Multiple comparisons correction was done using a permutation-based false discovery rate (FDR) of 0.05. The proteins with a q-value < 0.05 and a minimum absolute log2 fold-change of 1.0 were used in the subsequent analysis. These values were normalized using a z-score before hierarchical clustering, with a Ward distance metric, to group the proteins with similar trends across timepoints. Optimal clusters were determined using cubic clustering criterion (CCC). Averages from each cluster were calculated and overlayed onto parallel plots to depict the relative protein accumulation or depletion trends across the timepoints. Each cluster was given a trend category using 0 hpi as the baseline value. For example, any protein cluster that had relative increase in protein abundance at 24 hpi while all other timepoints were at the baseline levels, the cluster was given a trend name of “Up 24 hpi” (Supplementary Figures S3–S5).
2.6.3 Gene ontology enrichment
Whole-genome gene ontology (GO) annotation and enrichment analysis was performed using the ClueGOv2.5.8 application within Cytoscape (Bindea et al., 2009). ClueGO processed the GO terms for each protein cluster using a medium network specific selection and a Bonferroni step-down p-value correction. The selected GO tree levels were between 3 and 12 with a minimum of 3% associated proteins. GO term redundancy was reduced using the GO term fusion feature. GO terms were considered significantly enriched if the adjusted p-value was less than or equal to 0.05. The general pattern of up or downregulation of the Biological Processes GO terms were compared before examining the fluctuation across individual timepoints. All proteins with an increase in relative abundance were analyzed simultaneously with the proteins that decreased in relative abundance using the parameters described above. The default cut-off of 60% was used to determine which group (i.e., up or down-regulated) held the majority of the GO-term-associated proteins. This indicated which biological processes were up or downregulated for each genotype. ClueGO was used to contrast the regulation of biological processes in the resistant and susceptible poplars by analyzing the proteins that increased in abundance in BESC-22 and remained the same or decreased in abundance in the susceptible lines (BESC-801 and NQ-1). The proteins that increased in abundance in the susceptible lines but remained the same or decreased in abundance in the resistant line were also analyzed for GO enrichment. This revealed the changes in protein abundance that were correlated with biological processes that are specifically associated with resistance or susceptibility. The number of proteins with changes in abundance at each timepoint were also tabulated.
2.6.4 ANOVA simultaneous component analysis
Additional comparisons among genotypes were made using an ANOVA simultaneous component analysis (ASCA) (Smilde et al., 2005; Bertinetto et al., 2020). Proteins were normalized by centering each protein abundance mean to zero for each genotype. Using the ASCA module in MetaboAnalyst 5.0 (Pang et al., 2021), the proteins contributing most to the main effects of timepoint, genotype, and the genotype by timepoint interaction were identified. ASCA partitions the overall variance of the data into the individual variance components of timepoint, genotype, and their interactions. The number of variance components that explain the majority of the variation were depicted on scree-plots. Proteins that had significant difference in abundance among the components and fit the multivariate model were listed as proteins associated with the main effects and/or the interaction effects using the default parameters in MetaboAnalyst 5.0. This analysis provides a list of proteins with the strongest association to the treatment effects.
3 Results
3.1 Qualitative assessment of proteomic analysis
3.1.1 Protein extraction and abundance
The proteomes of three P. trichocarpa genotypes were measured across the timepoint treatments and the number of total proteins identified was relatively consistent between the measurements (Figure 1A). Moreover, variability in the number of proteins identified between biological replicates for each treatment group was low, and correlation coefficients between biological replicates was generally higher than between samples from different conditions. For each genotype, the average Pearson correlation coefficient (r) was 0.95 for the replicates sampled together and ranged from 0.92–0.97 for BESC-22 and BESC-801 and was 0.91–0.98 for NQ-1. Overall, 11,897 proteins were identified across all measurements with ∼1% of the total being encoded by S. musiva. The susceptible genotype, BESC-801, had a notable decrease in proteins identified at 12hpi (Figure 1A) but also had the most uniquely identified proteins not observed in the other two genotypes (Figure 1B).
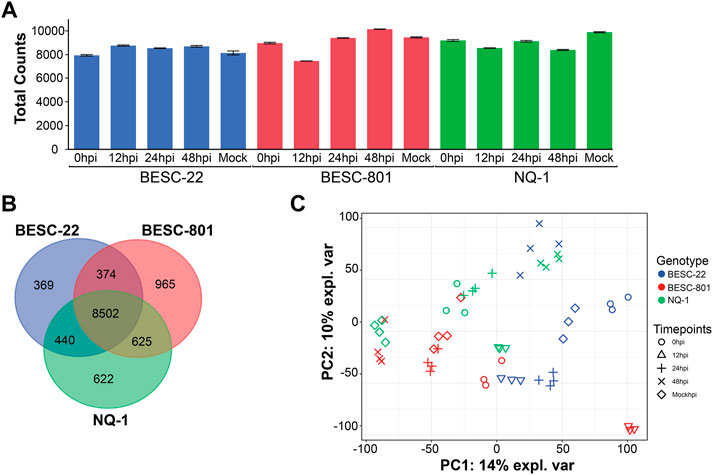
FIGURE 1. Qualitative assessment of the protein extraction for each treatment group. (A) Bar chart depicts the average number of proteins identified in each treatment group (i.e., 0, 12, 24, and 48hpi and mock control) for each genotype, and error bars show the relatively low level of variation across treatment replications. (B) Venn diagram showing the overlap of identified proteins for each genotype and the number of proteins uniquely identified for each genotype. (C) Principal component analysis and the relatedness of each treatment group cluster. Genotypes: BESC-22 is in blue, BESC-801 in red, and NQ-1 in green.
A principal component analysis (PCA) was conducted on the 8,502 proteins identified across all three genotypes to assess similarity across treatments. In general, measurements grouped together by genotype, with timepoint groupings varying for each genotype (Figure 1C). Distinct groupings were observed, such as the 12hpi timepoint in BESC-801. Other treatment groups overlapped in the PCA plot, such as the mock inoculation and 24 hpi treatments in BESC-801. In contrast, the mock groups were more distinct in BESC-22 and NQ-1. Overall, 18% of the variation in the experiment was explained by genotype (p < 0.001), 22% by the timepoint (p < 0.001), and 40% by the genotype and timepoint interaction (p < 0.001) according to a permutational multivariate analysis of variance (PERMANOVA) test.
3.1.2 Susceptibility protein accumulates as expected in BESC-801
In a previous genome-wide association study (GWAS) published by Muchero et al. (2018), a G-type LecRLK was strongly associated to Septoria canker susceptibility. Therefore, the abundance pattern of this protein was assessed and compared with the transcriptomic data described in the previous study, where it was observed to have relatively high levels of expression at 24 hpi. The relative abundance of G-type LecRLK (Potri.005G018000.1.p) was identified with high confidence and accumulated with relatively high abundance at 24hpi in BESC-801 (Figure 2). Consistent with previous observations, the G-type LecRLK abundance did not change in response to the fungal inoculation for the resistant line, BESC-22, nor the other susceptible line, NQ-1.
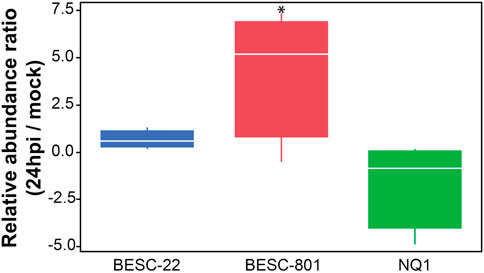
FIGURE 2. Relative accumulation or depletion of the G-type lectin receptor kinase (PtLecRK-G.1) between S. musiva inoculated and mock inoculated plants grown for 24 h post-inoculation. The * denotes a significant change (p < 0.05) in the mean at 24 hpi relative to mock control.
3.2 Differentially abundant proteins for each genotype
3.2.1 Resistant poplar (BESC-22)
ANOVA was used to characterize proteome-wide changes in relative protein abundance that occurred in stem tissue post-inoculation with S. musiva. Among the 9,430 proteins identified in BESC-22, 4,404 had significant changes in abundance (FDR <0.05, log2 fold-change > 1) across timepoints. One-way hierarchical clustering and the cubic clustering criterion (CCC) revealed 14 major clusters of proteins with similar temporal regulation patterns (Figure 3A). The changes in protein abundance for each cluster were made relative to the protein abundance for that genotype at 0hpi (Figure 3; Supplementary Figures S3–S5). The three clusters with the most proteins were cluster 4 (Up 48 hpi), cluster 9 (Up 12-24 hpi), and cluster 11 (Up 12-48 hpi) with 519, 532, and 517 proteins respectively (Supplementary Material).
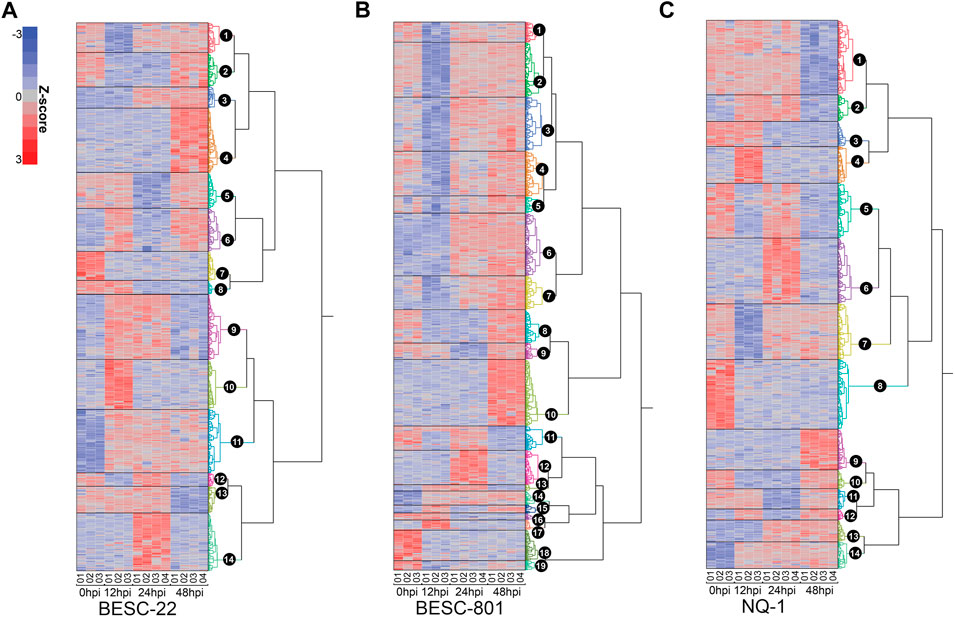
FIGURE 3. Hierarchical clustering of the significantly regulated proteins from 0 to 48hpi in genotypes: (A) BESC-22, (B) BESC-801, and (C) Nisqually-1. Cubic clustering criterion was used to identify the optimal number of major clusters per dendrogram. Each color represents a cluster of proteins regulated with similar trends (e.g., up or down at various timepoints relative to the 0hpi timepoint). Relative abundance of each row/protein is on a Z-score scale with green as low and red as high.
3.2.2 Susceptible poplar (BESC-801)
For the BESC-801 genotype, a total of 6,426 of 10,140 proteins observed had significant changes in their relative protein abundances across the timepoints (FDR <0.05, log2 fold-change > 1.0). Using one-way hierarchical clustering, 19 major clusters were identified by CCC (Figure 3B). Five clusters had a similar trend of reduced protein abundances at 12 hpi (Down 12 hpi) and as a result, were merged into one cluster. In addition, two clusters with similar trends of reduced relative protein abundances at 12 hpi and 48 hpi (Down 12-48 hpi) were collapsed into a single cluster. Similarly, two clusters with increases in relative protein abundance at 24 hpi and 48 hpi (Up 24–48 hpi) were merged. As a result, there were 13 different protein abundance patterns identified for BESC-801 (Supplementary Figure S4). Overall, the three most prominent abundance patterns were those with decreases in protein abundance at 12 hpi (clusters 1 –5), increases at 48 hpi (cluster 10), and increases in abundance at 24–48 hpi (cluster 6) (Supplemental Material).
3.2.3 Susceptible poplar (NQ-1)
For the NQ-1 genotype, 4,250 of the 9,678 proteins identified had significant changes in their protein abundance across timepoints (FDR <0.05, log2 fold-change > 1.0). In total, 14 clusters were identified using hierarchical clustering and CCC (Figure 3C). The largest clusters for NQ-1 were cluster 1 with 576 proteins, cluster 6 with 510 proteins, and cluster 8 with 540 proteins. These corresponded to the trends of Down 48 hpi, Up 24 hpi, and Down 12–48 hpi, respectively (Supplementary Figure S5).
3.3 Gene ontology enrichment of differentially abundant proteins in each genotype
Gene ontology (GO) enrichment was used to summarize the regulation of biological processes with associated changes in protein abundance patterns. In general, many GO terms were significantly enriched across multiple protein clusters suggesting non-specific changes in protein abundance. As a result, clusters generally categorized as up- or down-regulated across the timepoints were collapsed together. These condensed clusters were analyzed using GO enrichment for each genotype. For each enriched GO term, the total number of proteins and how they were distributed across timepoints was tabulated to summarize regulation patterns within each genotype. The temporal patterns were used to assess when the biological process GO term may be regulated during S. musiva—poplar infection.
3.3.1 Resistant poplar (BESC-22)
Biological processes related to growth and development were enriched in the genotype BESC-22. For example, there was an enrichment of primary cell wall biosynthesis, gene expression, and tissue differentiation GO terms (Supplementary Table S1) in the cluster with increasing protein abundance. Conversely, lignin biosynthesis, an example of secondary tissue differentiation, was enriched in the clusters with decreasing relative abundance. GO terms involved with disease signaling were enriched from the proteins with increasing abundance patterns. This included the proteins correlated with induction of SAR. A total of 56 salicylic acid (SA) response proteins increased in abundance. In addition, the GO term for the metabolism of glycerol-3-phosphate, another major SAR-inducer, was enriched in the proteins with increased abundance. There were no clear trends in proteins regulating Jasmonic acid (JA) biosynthesis. The GO terms for brassinosteroid stimulus were enriched in the proteins with increased abundance. The auxin response GO term was also enriched in this group of proteins whereas the auxin production, influx, and cytokinin signaling GO terms were enriched in the proteins with decreases in relative abundance.
Among other potential defense signaling pathways that were enriched in the proteins with increasing relative abundance involved protein kinase activity and chitinase activity (Supplementary Table S1). Calcium signaling and calcium ion import were also enriched in the clusters with increasing abundance. Conversely, antioxidant production was enriched in the clusters with decreasing protein abundance. This was accompanied by the apoptosis pathways and hydrogen peroxide transmembrane transport in the clusters with increasing relative protein abundance. The hyperosmotic response was also enriched in the clusters with increased abundance with mannitol potentially accumulating due to the reduced abundance of the mannitol dehydrogenase pathway. There were also a group of volatiles and secondary metabolite processes enriched in the clusters with increasing abundance.
3.3.2 Susceptible poplar (BESC-801)
The biological processes enriched from the differentially abundant proteins in BESC-801 were in clusters with decreasing abundance. Most of the reduction took place at 12 hpi; however, many of these GO terms were enriched from proteins with reduced relative abundance across all timepoints. Notable disease-related trends in GO enrichment involved cell wall structure, oxidative stress, cell signaling cascades, growth and development, secondary metabolism, and defense response protein pathways (Supplementary Table S2). For cell wall structure, GO terms for both secondary cell wall biosynthesis (lignin and pectin biosynthesis) and primary cell-wall biosynthesis (beta-glucan and cellulose biosynthesis) were associated with clusters with reduced protein abundance. Oxidative stress responses and antioxidant biosynthesis were also enriched from downregulated clusters. GO terms involved with growth and development, such as gene expression, translation, cell cycle transition, the TCA cycle, and plant organ morphogenesis, were all enriched in the clusters with reduced relative protein abundance in BESC-801 following inoculation. In contrast, proteins associated with the nicotinate metabolic process, which impede cell cycle progression, were enriched in the clusters with increased protein abundance.
GO terms including the activation of cell surface receptors, pattern recognition receptors, MAPKs, and calcium ion channels were all enriched in the clusters with reduced protein abundance. In contrast, specific responses to ethylene and abscisic acid were enriched in the clusters with increased protein abundance (Supplementary Table S2). Proteins associated with auxin influx were also enriched in the clusters with increasing trends in relative protein abundance. In contrast, proteins associated with the cytokinin response pathway were enriched in the reduced abundance clusters. This correlates with reduced kynurenine metabolic process which inhibits the ethylene-directed production of auxin. Secondary metabolism of small molecules involved with plant disease were also enriched in BESC-801. The enzyme involved with the production of N-hydroxypipecolic acid, a key SAR-inducer, had increased abundance; however, SAR-antagonistic processes also increased in abundance. For example, there was enrichment of 1-ACC oxidase activity which promotes ethylene production, and the metabolic process for allantoin biosynthesis, another SAR-antagonist, was promoted. There was also a reduction in the abundance of proteins associated with the cellular response to brassinosteroids, a JA signaling antagonist.
3.3.3 Susceptible poplar (NQ-1)
The susceptible genotype Nisqually-1 has different patterns of relative protein abundance compared to BESC-801. These contrasting patterns were similar to the resistant line but the changes in abundance occurred at later timepoints, and there was a lack of coordination within defense response pathways. For example, the GO terms with discordant regulation, where some pathway-associated proteins increased in abundance and others decreased, include JA biosynthesis, alkaloid production, chitinase activity, general stress responses, response to brassinosteroid, antioxidant activity, and lignin biosynthesis (Supplementary Table S3). Proteins associated with clusters with decreasing relative abundance were associated with calcium ion import, protein kinase activity, ethylene production, response to auxin, and the regulation of cell differentiation. Proteins associated with transcription and translation decreased in abundance along with those involved in cell cycle progression. Proteins associated with the ROS/oxidative stress response also had reduced relative abundance. The reduced relative abundance of proteins associated with hydrogen peroxide transmembrane transport and the apoptotic process involved with development was in direct contrast to the upregulation of these pathways in the resistant line.
3.3.4 Gene ontology enrichment of the contrasting protein abundance patterns between the resistant and susceptible genotypes
The GO enrichment revealed stark contrasts in defense pathway regulation between BESC-22 and the susceptible lines (Supplementary Table S4). The major biological processes with increases in relative protein abundance in BESC-22 but decreases in both susceptible lines were calcium-dependent kinase signaling, sucrose transport, translation, quercetin production (a natural auxin transport inhibitor) (Singh et al., 2021), vitamin biosynthesis, chitin catabolism, plant primary cell wall biogenesis, cell growth and regeneration, brassinosteroid stimulus, vesicle-mediated transport, SNARE binding, and mitosis. In contrast, auxin signaling, auxin efflux, and sucrose biosynthesis had reduced relative abundance in BESC-22. These pathways increased in relative protein abundance in the susceptible genotypes. The increase in relative abundance of proteins associated with the cellular response to SA was specific to the resistant genotype with 83 identified proteins associated with this pathway (Supplementary Table S1).
Salicylic acid is the primary signal involved with priming neighboring cells for defense in SAR (Klessig et al., 2018); therefore GO enrichment of upregulated SA-response proteins prompted an examination of changes in the abundance of SAR-associated proteins. A total of 42 SAR-associated proteins increased in abundance in BESC-22 (Figure 4A), with many SAR proteins with increased abundance at 24 and 48 hpi with 15 and 16 proteins increasing in abundance, respectively. As expected, most of the identified proteins associated with SAR did not change in abundance in the susceptible genotypes but rather decreased in abundance. Most SAR proteins decreased in relative abundance in BESC-801. Similar to the global changes in protein abundance noted for NQ-1 above, there was an approximately equal number of proteins with increasing and decreasing abundance. As such, this observation highlights disparate regulation of SAR among poplar genotypes with the same disease phenotype.
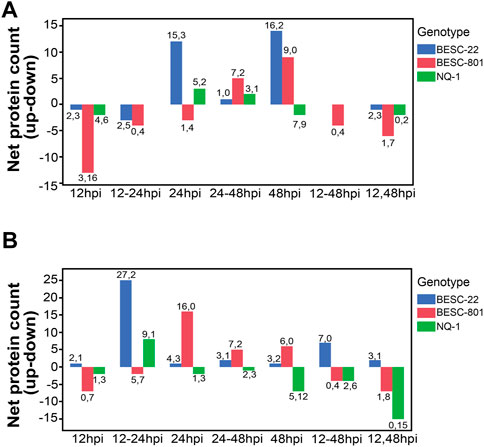
FIGURE 4. The net protein counts for (A) systemic acquired resistance (SAR, GO:0009627) and (B) the most significant receptor proteins (e.g., LRR-RKs, Lectin RKs, LysM receptor kinases, cysteine rich RLKs, etc.) identified by ASCA. Each regulatory trend is separated by genotype (BESC-22 in blue, BESC-801 in red, NQ-1 in green). The values on the y-axis represent the net total of proteins upregulated (positive bar) or downregulated (negative bar) at different timepoint clusters. The values above or below each bar indicates the number of up and downregulated proteins at that timepoint (up, down).
3.4 ANOVA simultaneous component analysis of the differentially abundant proteins across timepoints and genotypes
3.4.1 Receptor-like proteins
ANOVA simultaneous component analysis (ASCA) was used to partition the variance to identify proteins with the greatest fluctuations across timepoints and among genotypes (Supplementary Table S5). A total of 68 of the 387 (17.5%) proteins contributing most to the variation in the experiment were receptor-like or membrane-associated proteins (Supplementary Table S5). There was a net increase in abundance of receptor proteins for the resistant genotype with a peak of 27 proteins increasing in abundance and two proteins decreasing in abundance at 12–24 hpi (Figure 4B). The susceptible lines also had a net increase of receptor protein abundance at 24 hpi with 16 and nine for BESC-801 and NQ-1, respectively. The susceptible lines had a net decrease in abundance of other receptors at 12 and 12–48 hpi (Figure 4B).
3.4.2 Defense-related proteins
Proteins identified from ASCA with increased abundance in BESC-22 were linked to the brassinosteroid response, gene expression, calcium import and signaling, SAR, primary cell wall biosynthesis, and the fungal PAMP response. These pathways all had decreases in the abundance of associated proteins in the susceptible lines, supporting the GO-term enrichment analysis detailed above. Proteins that uniquely increased in abundance in BESC-22 included potential PRRs (e.g. CERK1: Potri.002G226600.1. p, LRR-RLK: Potri.016G011400.4. p, cysteine-rich RLK10: Potri.011G027800.1. p) (Couto and Zipfel, 2016), R-proteins (e.g., NB-ARC-LRR: Potri.001G427600.1. p) (Tan et al., 2007), PTI signaling proteins (e.g., GSK3: Potri.002G190500.3. p) (Stampfl et al., 2016), and a protein that links the calcium response with phytohormone signaling (CIPK3: Potri.001G222600.6. p) (Sanyal et al., 2017) (Figure 5). A broader look at the calcium-mediated cellular processes during the fungal interaction suggests that calcium-mediated signaling and downstream calcium responses were impacted in BESC-22 and BESC-801. Proteins associated with these processes were upregulated earlier (12–24 hpi vs. 24–48 hpi) in BESC-22 relative to BESC-801 (Supplementary Table S6). In NQ-1, the majority of proteins associated with calcium import, signaling, and disease response proteins decreased in relative abundance (Supplementary Table S6).
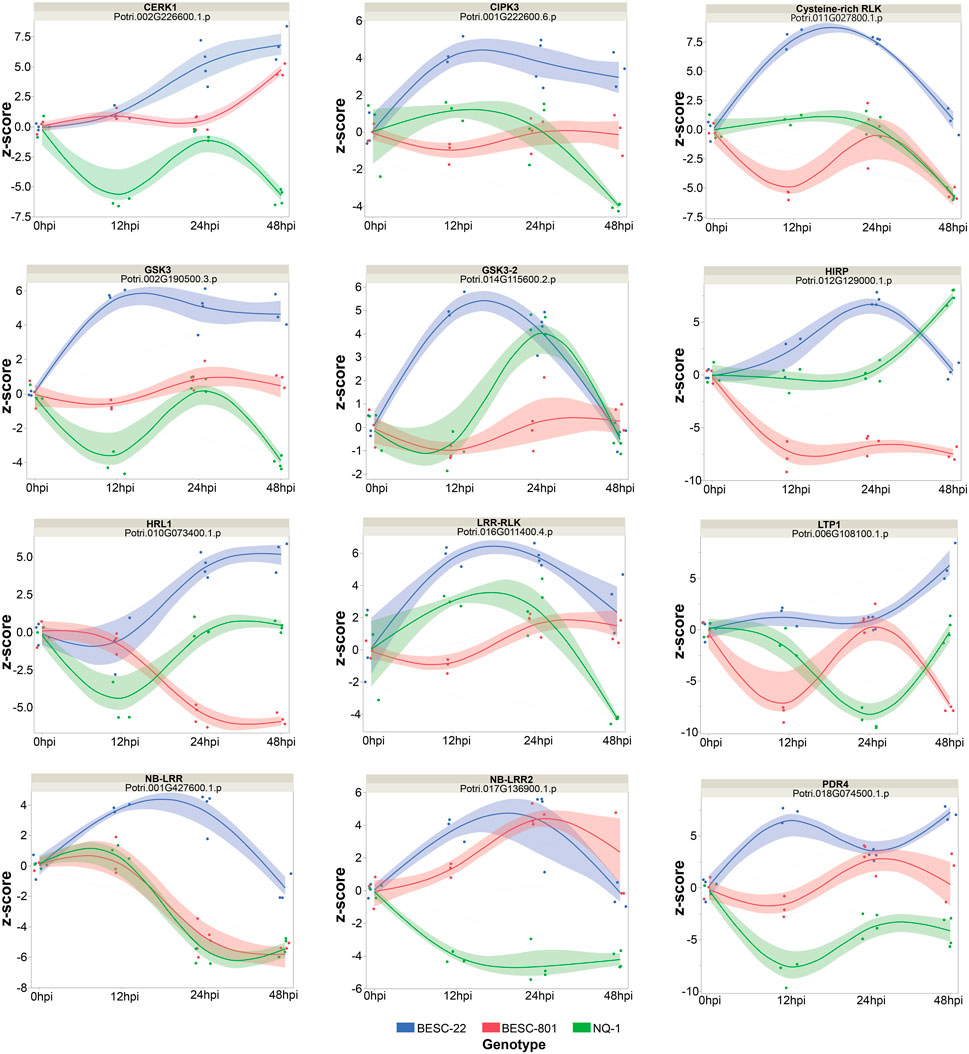
FIGURE 5. Panel of selected disease-related proteins from the ASCA list of the top proteins influenced by the experimental effects. The relative log2 abundance (y-axis) values for each protein are shown using the 0hpi timepoint as the baseline level. The relative abundance trends were compared for each genotype (BESC-22 in red, BESC-801 in blue, NQ-1 in green).
3.4.3 Pattern-triggered immunity
Together, the GO-term enrichment and ASCA analyses implicate proteins associated with PTI in the response of the resistant genotype. Other disease-response proteins with contrasting patterns between the resistant and susceptible genotype include the immunity inducer receptor/co-receptor proteins, SOBIR1 and BAK1 (van der Burgh et al., 2019). Three paralogs of these proteins (SOBIR1: Potri.013G117200.1. p, Potri.019G087700.1. p, Potri.005G083300.1. p; BAK1: Potri.001G206700.1. p, Potri.003G023000.1. p, Potri.001G206700.1. p) were identified and all six increased in relative abundance in BESC-22 from 12 to 24 hpi. Three proteins of the MOS4-associated complex (MAC) (Palma et al., 2007), including CDC5 (Potri.019G018400.2. p), MOS4 (Potri.015G041800.1. p) and PRL1 (Potri.010G012800.1. p), increased in relative abundance in BESC-22 from 12 to 48 hpi. In contrast, all three decreased in relative abundance at 12 hpi in BESC-801. CDC5 and MOS4 did not change in abundance in NQ-1 but PRL1 did increase from 12 to 24 hpi (Supplementary Material). Another gene regulation protein identified was a zinc finger protein (ZFP1: Potri.013G086800.1. p) which is part of a class of transcription factors associated with hormone signal regulation and stress responses (Sakamoto et al., 2000; Bogamuwa and Jang, 2014). This protein increased in abundance from 12 to 48 hpi in BESC-22 and decreased in abundance at 12 hpi and 48 hpi in BESC-801 and NQ-1, respectively. Finally, npr1-1 constitutive 4 (SNC4: Potri.017G009000.1. p), which is an RLK that leads to the production of PR proteins (Zhang et al., 2014), was uniquely identified in BESC-22 and increased in abundance at 24 hpi (Supplementary Material). Overall, the evidence shows many PTI-related proteins increase in abundance early in the susceptible lines and decreased in abundance in the resistant line.
3.4.4 Systemic acquired resistance and hypersensitive response proteins
Many of the individual proteins that increased in abundance in the resistant genotype included those associated with SAR and the HR (Figure 4A). For example, the SAR response modulator, RNA-binding protein-defense related 1 (RBP-DR1: Potri.002G214000.1. p) (Qi et al., 2010) increased in abundance in at 12–24 hpi in the resistant genotype but either decreased in abundance or remained the same in the susceptible genotypes. The SAR-response protein, pathogenesis-related 4 (PR4, HEL: Potri.005G054000.1. p) (Bertini et al., 2012; Naidoo et al., 2013) increased in abundance in BESC-22, decreased in abundance in BESC-801, and did not change in NQ-1. Two other defense response proteins linked to SAR can initiate a hypersensitive response (HR) (HIRP: Potri.012G129000.1. p and HRLI: Potri.010G073400.1. p) (Lv et al., 2017; Wu et al., 2021). These proteins increased in abundance in BESC-22 within 24 hpi and decreased in abundance in BESC-801 across all timepoints (Figure 5). In contrast, NQ-1 had a delayed increase in the abundance of HIRP at 48 hpi and a decrease in abundance of HRLI at 12 hpi. An ABC transporter involved with HR and cell wall defense in nonhost resistance interactions (Johansson et al., 2014; Campe et al., 2016), annotated as PEN3: Potri.003G049600.1. p (syn. PDR8, ABCG36), was increased in abundance in BESC-22 at 12 and 48 hpi. This protein decreased in abundance at the same time points in BESC-801 and NQ-1 (Supplementary Material). Two other ABC transporters had increased abundance, PDR4: Potri.018G074500.1. p and the pathogenesis-related PR-14 family protein, LTP1: Potri.006G108100.1. p (Figure 5), in BESC-22 (Buhot et al., 2004; Bessire et al., 2011). SAR also contributes to the production of chitinases (Kombrink and Schmelzer, 2001). For example, chitinase A (Potri.015G024000.1. p) and chitinase C (Potri.006G188300.1. p) had increased abundance in BESC-22 (Supplementary Material). Analyzing the temporal regulation of other chitin catabolism proteins revealed 10 proteins with increased abundance at 24 and 48 hpi in BESC-22, while these proteins decreased in abundance within 12 hpi in both susceptible lines (Supplementary Material). Chitin catabolism can lead to the release of fungal PAMPs. Accordingly, distinct patterns were found for proteins involved with the fungal PAMP response (GO:0002238). Seven proteins involved with the fungal response had increased abundance in BESC-22 at 12 hpi or 12–48 hpi. In contrast, the susceptible lines had a reduction in the abundance of 12 (BESC-801) and 15 (NQ-1) fungal response proteins at 12 hpi, 12–24 hpi, or 12–48 hpi (Supplementary Material). Collectively, this suggests that plant immunity proteins associated with PTI, SAR, and HR are accumulating within 12hpi in the resistant genotype. These same proteins had conflicting patterns of relative abundance in the susceptible lines.
4 Discussion
4.1 Plant G-type lectins mediate symbiosis in plant-microbe interactions
Like other biotrophs and hemibiotrophs, it is hypothesized that S. musiva actively suppresses and/or evades the plant host immune response immediately following inoculation (Horbach et al., 2011; Ohm et al., 2012; Muchero et al., 2018). This is corroborated by morphological changes observed in resistant and susceptible genotypes post-inoculation (Weiland and Stanosz, 2007; Qin and LeBoldus, 2014). The observed proteome differences among resistant and susceptible P. trichocarpa genotypes, described above, support this hypothesis, and provide new insights into the P. trichocarpa - S. musiva interaction. Based on patterns of differential protein abundance, BESC-801 had a delayed defense response to S. musiva and a reduced protein abundance across a diversity of biological processes at 12 hpi. There are several possible explanations for this phenomenon: 1) the absence of specific receptors able to recognize the pathogen; 2) effectors from the fungus that inhibit pathways leading to resistance; or 3) a combination of the two (Plett and Martin, 2018; Gourion and Ratet, 2021).
It is important to note that the abundance of the G-type LecRLK (Potri.005G01800.1. p), a protein associated with Septoria canker susceptibility in BESC-801, peaked at 24 hpi (Figure 2) matching the patterns in transcript abundance previously described (Muchero et al., 2018). This protein is expected to bind specific cell-wall derived components of S. musiva and may mediate the downregulation of immune responses (Muchero et al., 2018). The abundance of this susceptibility factor is negatively correlated with defense response proteins and pathways. In contrast, the resistant line (BESC-22) had a reduced abundance of the G-type LecRLK and significantly increased abundance of defense response proteins such as BAK1 (Supplemental Material) consistent with differential expression reported in the literature (Muchero et al., 2018). Another G-type lectin receptor kinase, denoted PtLecRLK1 (Potri.T022200), mediates the symbiotic interaction between P. trichocarpa and the ectomycorrhizal fungus Laccaria bicolor by downregulating similar resistance pathways (Labbé et al., 2019). Moreover, transgenic expression of PtLecRLK1 in non-ectomycorrhizal hosts led to colonization and symbiosis with similar transcriptional reprogramming and alterations to protein abundance observed in the cognate interaction (Qiao et al., 2021).
Unlike the symbiotic interaction described above, the effect of the G-type LecRLK on susceptibility appears to function in a genotype-specific manner. For example, in the susceptible genotype NQ-1, there was no change in protein abundance of the G-type LecRLK observed in BESC-22 or BESC-801. Rather, the inoculation of NQ-1 with S. musiva appears to elicit an uncoordinated defense response. The signaling pathways, measured in terms of protein abundance, do not exhibit specific patterns. Instead, an equal number of SAR-related proteins were up and downregulated, there was a lack of calcium signaling, and discordant phytohormone signaling involving JA and brassinosteroid associated proteins was observed (Supplementary Table S3). A second possible explanation for the lack of a coordinated defense response may be related to the speed at which it is activated. For example, some of the changes in defense-related protein abundance observed in the resistant genotype BESC-22 also occurred in NQ-1 but at a later timepoint. Suggesting, as hypothesized by Qin and LeBoldus (2014), that susceptibility is the result of a delayed resistant response.
4.2 Sphaerulina musiva perception and pattern-triggered immunity signaling in BESC-22
The resistant genotype BESC-22 had an increase in the abundance of defense -associated proteins within 12–24 hpi. This change in protein abundance included SAR, calcium signaling, and components of PTI resulting in the promotion of cellular growth, cell wall production, and chitinase activity (Supplementary Table S1; Figures 5, 6). Plant defense mediated by PTI is initiated by PRRs that perceive molecular patterns associated with disease including PAMPs, MAMPs, and/or DAMPs. Perception drives a signaling cascade via protein kinases reprograming the cell for defense (Couto and Zipfel, 2016). Biological processes and individual proteins involved with PTI and receptor-mediated signaling were identified via GO enrichment and ASCA. The GO enrichment of fungal-PAMP recognition proteins increased in BESC-22. This was complemented with the identification of proteins in the ASCA which included candidate PRRs with increased abundance in the resistant genotype relative to the susceptible genotypes (Figure 4B). Many of these receptor proteins were in the LRR-RLK family, a protein family that is rich in PTI-associated resistance proteins described in other plant-microbe interactions (Petre et al., 2014). For example, CERK1 (Potri.002G226600.1. p) was identified and this protein recognizes chitin from the fungal cell wall and can coordinate signaling with other LRR-RLKs initiating resistance (Couto and Zipfel, 2016).
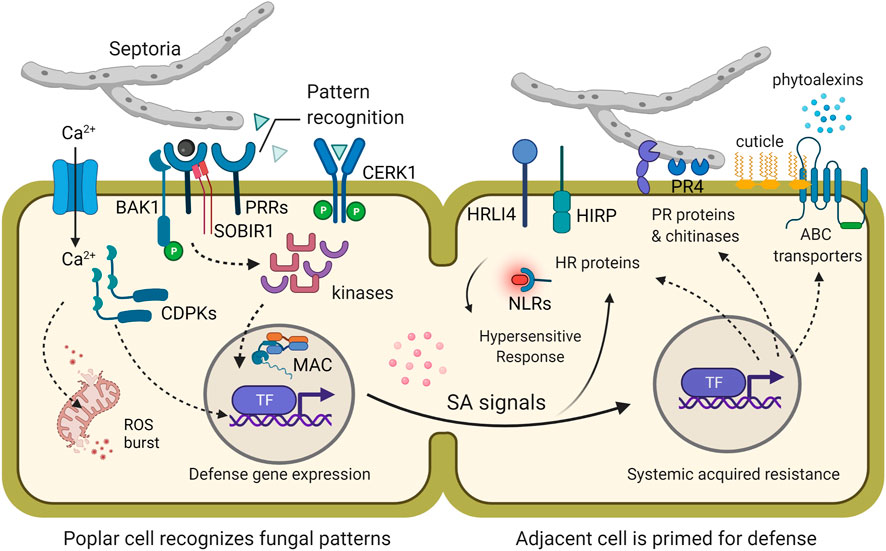
FIGURE 6. Conceptual model of cellular defense responses during Septoria canker resistance. During the first encounter with the fungal signals, pattern recognition receptors (PRRs) (e.g., CERK1) and any necessary co-receptors (e.g., BAK1 and SOBIR1) initiate a downstream signal, likely via various protein kinases leading to pattern-triggered immunity (PTI). This cascade is accompanied by an influx of calcium ions which involves another signaling cascade with calcium-dependent protein kinases. Calcium can also trigger a response in the mitochondria leading to reactive oxygen species (ROS) bursts. There are also key transcription factors and other gene expression regulators (e.g., MAC) involved with reprograming the cell and surrounding tissue for defense. One of the key signals that is sent to adjacent and distal plant cells is salicylic acid (SA). SA signaling induces systemic acquired resistance which has pathways unique and complementary to PTI. Major hallmarks of SAR can include the production of pathogenesis-related proteins (e.g., PR4), the regulation of R-proteins (e.g., NB-LRRs/NLRs), and promotion of receptors (e.g., HRLI4 and HIRP) that can trigger a hypersensitive response (HR). At the same time, the production of ABC transporters enables the plant cell to exude critical anti-microbial products (e.g., phytoalexins) or cuticle-building fatty acids. Illustration created with BioRender.com under an academic license.
An outcome of PRR activity is downstream transcriptional and/or translational regulation of genes associated with a plant defense response. Notably, a defense related protein complex involved with transcriptional regulation called the MOS4-associated complex (MAC) had increased abundance in BESC-22 and had no changes in the susceptible genotypes. All three proteins from this complex (CDC5: Potri.019G018400.2. p, MOS4: Potri.015G041800.1. p, and PRL1: Potri.010G012800.1. p) are essential for plant innate immunity and function by aggregating in the nucleus to regulate gene expression (Palma et al., 2007). Biological processes downstream of pathogen perception are responsible for proliferating defense signals priming neighboring cells to respond to pathogen exposure.
One of the earliest responses of plant cells following pathogen perception is an influx of calcium ions (Thor, 2019). The increase in abundance in calcium ion channels in BESC-22 at 12 hpi is likely correlated with this process (Supplementary Table S1). This change in abundance was correlated with an increase in the abundance of calcium response and calcium signaling proteins; for example, the CBL-CIPK3-AB1 protein complex. This complex monitors calcium ion concentrations in the cytosol and regulates abscisic acid (ABA) response proteins (Sanyal et al., 2017). Upon calcium-dependent activation, CIPK3 activates AB1 which in turn reduces the production of ABA-response proteins (Sanyal et al., 2017). A CIPK3 homolog (Potri.001G222600.6. p) was up-regulated in BESC-22, yet this pattern was not apparent in the susceptible genotypes (Figure 5). The abundance of this protein supports the hypothesis that ABA is antagonistic to Septoria canker resistance (Lenz et al., 2021). It is well-documented that ABA signaling is antagonistic to SA and SAR, which are necessary for resistance to S. musiva (Lenz et al., 2021; Derksen et al., 2013; N. Li N et al., 2019; Pieterse et al., 2009). In general, we see that PTI-related proteins increase in abundance in the resistant genotype while these signals are absent in the proteome of the susceptible genotypes.
4.3 Systemic acquired resistance is elicited by BESC-22 during the interaction with S. musiva
Activation of SAR provides a plant with broad-spectrum resistance to a range of different pathogens (Sticher et al., 1997). At the proteome level, an increased number of SAR and SA-response proteins were observed in the resistant genotype at 48 hpi compared to the susceptible genotypes (Figure 4A; Supplementary Table S4). This corresponds with previous studies suggesting that an early defense response mediated by SA is necessary for resistance before the pathogen transitions to the necrotrophic stage of its life-cycle (Abraham et al., 2019; Lenz et al., 2021). At the protein level, many SAR-associated ABC transporters increased in abundance relative to 0hpi in BESC-22 and these proteins had reduced abundance in the susceptible lines. Among these proteins was an ABC transporter G family 36 (ABCG36, synonymous PEN3 or PDR8: Potri.003G049600.1. p), which acts as central component in cell wall-based defense against the powdery mildew pathogen, Blumeria graminis sp. hordei (Johansson et al., 2014) and is linked to the calcium ion signaling pathway (Campe et al., 2016). Plant PEN/PDR/ABCG36 proteins are often stimulated by microbial infection and defense phytohormones, such as SA and JA (Lu et al., 2015). Interestingly, a loss of function mutation in this gene caused a decrease in hypersensitive cell death triggered by recognition of effectors from oomycete and bacterial pathogens in Arabidopsis (Johansson et al., 2014).
SAR-related proteins including ABC transporter proteins linked to stress are often regulated by phytohormones such as SA (Nuruzzaman et al., 2014). These lipid transfer proteins can transport antimicrobial secondary metabolites including common phytoalexins to the cell surface (Krattinger et al., 2009; Khare et al., 2017). The ASCA revealed a few ABC transporter proteins that are involved with plant defense and cuticle formation. Two of these proteins, Potri.018G074500.1 and Potri.006G108100.1 annotated as pleiotropic drug resistance 4 (PDR4) and lipid transfer protein 1 (LTP1), represent a PR-14 class of SAR-induced pathogenesis-related proteins. Both proteins are involved with development and maintenance of the plant cuticle (a waxy layer that can impede pathogen infection) (Buhot et al., 2004; Bessire et al., 2011). PDR4 increased in abundance at both 12 and 48hpi in the resistant line but was downregulated at 12 hpi in both susceptible lines. The other cuticle and defense-related protein, LTP1, was upregulated at 48hpi for the resistant line while it was downregulated in the susceptible lines (Supplementary Table S6). Some LTP proteins have strong antimicrobial activity against an extensive range of plant pathogens, which are particularly effective against necrotrophic plant pathogens (Schmitt et al., 2018).
SAR-response proteins enriched in this analysis include other pathogenesis-related (PR) proteins. For example, pathogenesis-related 4 (PR4, HEL) (Potri.005G054000.1. p) increased in abundance in BESC-22, decreased in abundance in BESC-801, and had no change in NQ-1 (Figure 5). Previous studies have demonstrated that this particular protein is induced in response to various pathogens and is known to directly interact with fungi via chitin binding and degradation (Sels et al., 2008). In addition, this protein may transcend the hyphal cell membrane to bind fungal lectin and/or degrade RNA (Bertini et al., 2012). PR4 is typically induced in response to JA signaling, but it is also induced via SA and is involved in SAR (Naidoo et al., 2013).
4.4 A hypersensitive response follows S. musiva perception.
In addition to PR protein chitinases and ABC transporters, the resistant genotype increased production of several SAR-associated proteins involved with HR. For instance, RNA-binding protein-defense related 1 (RBP-DR1) (Potri.002G214000.1. p) increased in abundance from 12 to 24 hpi in the resistant genotype but decreased in abundance or remained the same in the susceptible genotypes. The homolog of this protein in Arabidopsis (At4G03110.1) is involved in resistance to the hemibiotrophic pathogen, Pseudomonas syringae pv. tomato (DC3000) and modulates SA levels in the plant leading to HR (Qi et al., 2010). Additional HR-associated proteins identified included the hypersensitive induced response protein (HIRP) and HR-like lesion-inducing protein (HRLI) that were enriched in BESC-22 (Figure 5). Transgenic Arabidopsis overexpressing HIRP had increased resistance to P. syringae pv. tomato (S. Li S et al., 2019). The endogenous HIRPs in Arabidopsis are induced by PTI and may act as membrane scaffolds for other defense-response proteins (Lv et al., 2017). HRLI proteins mediate cell death and are involved with SA defense signaling (Wu et al., 2021). As such, it is likely that the P. trichocarpa—S. musiva interaction begins with pathogen recognition that subsequently leads to PTI and SAR, which in turn primes adjacent plant cells for defense and HR. This HR would need to be contained at the infection sites to reduce extensive cell death.
4.5 Enrichment of growth and development related proteins are correlated with resistance and promote recovery from infection.
Growth and development related proteins also strongly correlated with resistance to S. musiva in this study. GO enrichment analysis revealed proteins involved in gene expression, plant tissue regeneration, and primary cell wall biosynthesis were enriched at various timepoints in the resistant genotype. Many of these proteins decreased in abundance in both susceptible lines (Supplementary Table S4). This pattern could be the precursor leading to a cell-wall reinforcement surrounding the infection site and HR (Underwood, 2012). Following pathogen perception changes in protein abundance associated with signaling cascades, small molecule crosstalk, cell growth, and cell wall restructuring were enriched in the Septoria canker resistant genotype (Figure 6). Ultimately, the combination of these changes in protein abundance inhibit the colonization of host tissue by this pathogen supporting previous work (Liang et al., 2014; Qin and LeBoldus, 2014; Foster et al., 2015; Muchero et al., 2018; Abraham et al., 2019).
5 Conclusion
Resistant poplar trees perceive S. musiva shortly after pathogen inoculation and mount a rapid and sustained defense response initiated by membrane proteins, calcium ion signaling, and transcriptional reprogramming. This contrasts with susceptible genotype BESC-801 which has a delayed defense and decreased protein abundance at 12 hpi. NQ-1 responded differently with an uncoordinated defense response, involving proteins associated with multiple plant hormone signaling pathways that did not appear to initiate SAR. Both the susceptible poplar genotypes failed to produce a robust defense response involving proteins associated with SAR, calcium signaling, or the activation of membrane-bound receptors that could induce other defense pathways. This biological framework can be leveraged to study the impact of various proteins involved with this host-pathogen interaction in poplar.
Data availability statement
All proteomics raw data were deposited at the ProteomeXchange Consortium via the MASSIVE repository (https://massive.ucsd.edu/). The MASSIVE identifier is MSV000089584.
Author contributions
RL designed the experiments, performed the proteome extractions, and wrote the primary draft of the manuscript. HS and PA assisted with proteome measurements and data analysis. AC assisted with plant maintenance and inoculations. JL, RH, PA, and JL oversaw the research approach. All authors contributed to the writing of the manuscript.
Funding
The manuscript is based upon work supported by the U.S. Department of Energy (DOE), Office of Science, Office of Workforce Development for Teachers and Scientists, Office of Science Graduate Student Research (SCGSR) program. The SCGSR program is administered by the Oak Ridge Institute for Science and Education for the DOE under contract number DE-SC0014664. This study was also supported by the DOE Office of Science, Office of Biological and Environmental Research (BER) grant number DE-SC0018196. The work is further supported by the Secure Ecosystem Engineering and Design (SEED) project funded by the Genomic Science Program of the DOE, Office of Science, BER as part of the Secure Biosystems Design Science Focus Area (SFA). Oak Ridge National Laboratory is managed by UT-Battelle, LLC for the U.S. Department of Energy under Contract Number DE-AC05-00OR22725.
Conflict of interest
The authors declare that the research was conducted in the absence of any commercial or financial relationships that could be construed as a potential conflict of interest.
Publisher’s note
All claims expressed in this article are solely those of the authors and do not necessarily represent those of their affiliated organizations, or those of the publisher, the editors and the reviewers. Any product that may be evaluated in this article, or claim that may be made by its manufacturer, is not guaranteed or endorsed by the publisher.
Supplementary material
The Supplementary Material for this article can be found online at: https://www.frontiersin.org/articles/10.3389/frans.2022.1020111/full#supplementary-material
References
Abraham, N., Chitrampalam, P., Nelson, B., Sharma Poudel, R., Chittem, K., Borowicz, P., et al. (2019). Microscopic, biochemical, and molecular comparisons of moderately resistant and susceptible populus genotypes inoculated with Sphaerulina musiva. PhytopathologyTM 109, 2074–2086. doi:10.1094/PHYTO-03-19-0105-R
Abraham, P. E., Garcia, B. J., Gunter, L. E., Jawdy, S. S., Engle, N., Yang, X., et al. (2018). Quantitative proteome profile of water deficit stress responses in eastern cottonwood (Populus deltoides) leaves. PLOS ONE 13, e0190019. doi:10.1371/journal.pone.0190019
Abril, N., Gion, J.-M., Kerner, R., Müller-Starck, G., Cerrillo, R. M. N., Plomion, C., et al. (2011). Proteomics research on forest trees, the most recalcitrant and orphan plant species. Phytochemistry 72, 1219–1242. doi:10.1016/j.phytochem.2011.01.005
Ayliffe, M., and Sørensen, C. K. (2019). Plant nonhost resistance: Paradigms and new environments. Curr. Opin. Plant Biol. 50, 104–113. doi:10.1016/j.pbi.2019.03.011
Bartholomé, J., Brachi, B., Marçais, B., Mougou-Hamdane, A., Bodénès, C., Plomion, C., et al. (2020). The genetics of exapted resistance to two exotic pathogens in pedunculate oak. New Phytol. 226, 1088–1103. doi:10.1111/nph.16319
Bertinetto, C., Engel, J., and Jansen, J. (2020). ANOVA simultaneous component analysis: A tutorial review. Anal. Chim. Acta. X 6, 100061. doi:10.1016/j.acax.2020.100061
Bertini, L., Proietti, S., Aleandri, M. P., Mondello, F., Sandini, S., Caporale, C., et al. (2012). Modular structure of HEL protein from Arabidopsis reveals new potential functions for PR-4 proteins. Biol. Chem. 393, 1533–1546. doi:10.1515/hsz-2012-0225
Bessire, M., Borel, S., Fabre, G., Carraça, L., Efremova, N., Yephremov, A., et al. (2011). A member of the PLEIOTROPIC DRUG RESISTANCE family of ATP binding cassette transporters is required for the formation of a functional cuticle in Arabidopsis. Plant Cell 23, 1958–1970. doi:10.1105/tpc.111.083121
Bier, J. E. (1939). Septoria canker of introduced and native hybrid poplars. Can. J. Res. 17c, 195–204. doi:10.1139/cjr39c-019
Bigeard, J., Colcombet, J., and Hirt, H. (2015). Signaling mechanisms in pattern-triggered immunity (PTI). Mol. Plant 8 (4), 521–539. doi:10.1016/j.molp.2014.12.022
Bindea, G., Mlecnik, B., Hackl, H., Charoentong, P., Tosolini, M., Kirilovsky, A., et al. (2009). ClueGO: A Cytoscape plug-in to decipher functionally grouped gene ontology and pathway annotation networks. Bioinformatics 25, 1091–1093. doi:10.1093/bioinformatics/btp101
Bogamuwa, S. P., and Jang, J.-C. (2014). Tandem CCCH zinc finger proteins in plant growth, development and stress response. Plant Cell Physiol. 55, 1367–1375. doi:10.1093/pcp/pcu074
Bryant, N. D., Pu, Y., Tschaplinski, T. J., Tuskan, G. A., Muchero, W., Kalluri, U. C., et al. (2020). Transgenic poplar designed for biofuels. Trends Plant Sci. 25, 881–896. doi:10.1016/j.tplants.2020.03.008
Buhot, N., Gomès, E., Milat, M.-L., Ponchet, M., Marion, D., Lequeu, J., et al. (2004). Modulation of the biological activity of a tobacco LTP1 by lipid complexation. Mol. Biol. Cell 15, 5047–5052. doi:10.1091/mbc.E04-07-0575
Callan, B. E., Leal, I., Foord, B., Dennis, J. J., and Oosten, C. V. (2007). Septoria musiva isolated from cankered stems in hybrid poplar stool beds, Fraser Valley, British Columbia. N. Am. Fungi 2, 1–9. doi:10.2509/pnwf.2007.002.007
Campe, R., Langenbach, C., Leissing, F., Popescu, G. V., Popescu, S. C., Goellner, K., et al. (2016). ABC transporter PEN3/PDR8/ABCG36 interacts with calmodulin that, like PEN3, is required for Arabidopsis nonhost resistance. New Phytol. 209, 294–306. doi:10.1111/nph.13582
Cheng, T., Chen, J., Ef, A., Wang, P., Wang, G., Hu, X., et al. (2015). Quantitative proteomics analysis reveals that S-nitrosoglutathione reductase (GSNOR) and nitric oxide signaling enhance poplar defense against chilling stress. Planta 242, 1361–1390. doi:10.1007/s00425-015-2374-5
Couto, D., and Zipfel, C. (2016). Regulation of pattern recognition receptor signalling in plants. Nat. Rev. Immunol. 16, 537–552. doi:10.1038/nri.2016.77
Derksen, H., Rampitsch, C., and Daayf, F. (2013). Signaling cross-talk in plant disease resistance. Plant Sci. 207, 79–87. doi:10.1016/j.plantsci.2013.03.004
Dhillon, B., Feau, N., Aerts, A. L., Beauseigle, S., Bernier, L., Copeland, A., et al. (2015). Horizontal gene transfer and gene dosage drives adaptation to wood colonization in a tree pathogen. Proc. Natl. Acad. Sci. U. S. A. 112, 3451–3456. doi:10.1073/pnas.1424293112
Dunnell, K. L., and LeBoldus, J. M. (2017). The correlation between septoria leaf spot and stem canker resistance in hybrid poplar. Plant Dis. 101, 464–469. doi:10.1094/PDIS-06-16-0903-RE
Eng, J. K., McCormack, A. L., and Yates, J. R. (1994). An approach to correlate tandem mass spectral data of peptides with amino acid sequences in a protein database. J. Am. Soc. Mass Spectrom. 5, 976–989. doi:10.1016/1044-0305(94)80016-2
Feau, N., Mottet, M.-J., Périnet, P., Hamelin, R. C., and Bernier, L. (2010). Recent advances related to poplar leaf spot and canker caused by Septoria musiva. Can. J. Plant Pathology 32, 122–134. doi:10.1080/07060661003740009
Foster, A. J., Pelletier, G., Tanguay, P., and Séguin, A. (2015). Transcriptome analysis of poplar during leaf spot infection with Sphaerulina spp. PLoS ONE 10, e0138162. doi:10.1371/journal.pone.0138162
Gourion, B., and Ratet, P. (2021). Avoidance of detrimental defense responses in beneficial plant–microbe interactions. Curr. Opin. Biotechnol. 70, 266–272. doi:10.1016/j.copbio.2021.06.008
Horbach, R., Navarro-Quesada, A. R., Knogge, W., and Deising, H. B. (2011). When and how to kill a plant cell: Infection strategies of plant pathogenic fungi. J. Plant Physiol. 168, 51–62. doi:10.1016/j.jplph.2010.06.014
Jiang, L., He, L., and Fountoulakis, M. (2004). Comparison of protein precipitation methods for sample preparation prior to proteomic analysis. J. Chromatogr. A 1023, 317–320. doi:10.1016/j.chroma.2003.10.029
Johansson, O. N., Fantozzi, E., Fahlberg, P., Nilsson, A. K., Buhot, N., Tör, M., et al. (2014). Role of the penetration-resistance genes PEN1, PEN2 and PEN3 in the hypersensitive response and race-specific resistance in Arabidopsis thaliana. Plant J. 79, 466–476. doi:10.1111/tpj.12571
Jones, J. D. G., and Dangl, J. L. (2006). The plant immune system. Nature 444, 323–329. doi:10.1038/nature05286
Käll, L., Canterbury, J. D., Weston, J., Noble, W. S., and MacCoss, M. J. (2007). Semi-supervised learning for peptide identification from shotgun proteomics datasets. Nat. Methods 4, 923–925. doi:10.1038/nmeth1113
Karpievitch, Y. V., Dabney, A. R., and Smith, R. D. (2012). Normalization and missing value imputation for label-free LC-MS analysis. BMC Bioinforma. 13, S5. doi:10.1186/1471-2105-13-S16-S5
Khare, D., Choi, H., Huh, S. U., Bassin, B., Kim, J., Martinoia, E., et al. (2017). Arabidopsis ABCG34 contributes to defense against necrotrophic pathogens by mediating the secretion of camalexin. Proc. Natl. Acad. Sci. U. S. A. 114, E5712–E5720. doi:10.1073/pnas.1702259114
Klessig, D. F., Choi, H. W., and Dempsey, D. A. (2018). Systemic acquired resistance and salicylic acid: Past, present, and future. Mol. Plant. Microbe. Interact. 31, 871–888. doi:10.1094/MPMI-03-18-0067-CR
Kombrink, E., and Schmelzer, E. (2001). The hypersensitive response and its role in local and systemic disease resistance. Eur. J. Plant Pathol. 107, 69–78. doi:10.1023/A:1008736629717
Krattinger, S. G., Lagudah, E. S., Spielmeyer, W., Singh, R. P., Huerta-Espino, J., McFadden, H., et al. (2009). A putative ABC transporter confers durable resistance to multiple fungal pathogens in wheat. Science 323, 1360–1363. doi:10.1126/science.1166453
Labbé, J., Muchero, W., Czarnecki, O., Wang, J., Wang, X., Bryan, A. C., et al. (2019). Mediation of plant–mycorrhizal interaction by a lectin receptor-like kinase. Nat. Plants 5, 676–680. doi:10.1038/s41477-019-0469-x
LeBoldus, J. M., Blenis, P. V., and Thomas, B. R. (2010). A method to induce stem cankers by inoculating nonwounded populus clones with septoria musiva spore suspensions. Plant Dis. 94, 1238–1242. doi:10.1094/PDIS-02-10-0094
Lenz, R., Louie, K., Sondreli, K., Galanie, S., Chen, J.-G., Muchero, W., et al. (2021). Metabolomic patterns of septoria canker resistant and susceptible Populus trichocarpa genotypes 24 hours postinoculation. Phytopathology® 111, 2052–2066. doi:10.1094/PHYTO-02-21-0053-R
Li, N., Han, X., Feng, D., Yuan, D., and Huang, L.-J. (2019). Signaling crosstalk between salicylic acid and ethylene/jasmonate in plant defense: Do we understand what they are whispering? Int. J. Mol. Sci. 20, 671. doi:10.3390/ijms20030671
Li, S., Zhao, J., Zhai, Y., Yuan, Q., Zhang, H., Wu, X., et al. (2019). The hypersensitive induced reaction 3 (HIR3) gene contributes to plant basal resistance via an EDS1 and salicylic acid-dependent pathway. Plant J. 98, 783–797. doi:10.1111/tpj.14271
Li, Y., Feng, Y., Lü, Q., Yan, D., Liu, Z., and Zhang, X. (2019). Comparative proteomic analysis of plant–pathogen interactions in resistant and susceptible poplar ecotypes infected with botryosphaeria dothidea. Phytopathology® 109, 2009–2021. doi:10.1094/PHYTO-12-18-0452-R
Liang, H., Staton, M., Xu, Y., Xu, T., and LeBoldus, J. (2014). Comparative expression analysis of resistant and susceptible Populus clones inoculated with Septoria musiva. Plant Sci. 223, 69–78. doi:10.1016/j.plantsci.2014.03.004
Lu, X., Dittgen, J., Piślewska-Bednarek, M., Molina, A., Schneider, B., Svato¡, A., et al. (2015). Mutant allele-specific uncoupling of PENETRATION3 functions reveals engagement of the ATP-binding cassette transporter in distinct tryptophan metabolic pathways. Plant Physiol. 168, 814–827. doi:10.1104/pp.15.00182
Lv, X., Jing, Y., Xiao, J., Zhang, Y., Zhu, Y., Julian, R., et al. (2017). Membrane microdomains and the cytoskeleton constrain AtHIR1 dynamics and facilitate the formation of an AtHIR1-associated immune complex. Plant J. 90, 3–16. doi:10.1111/tpj.13480
Muchero, W., Sondreli, K. L., Chen, J.-G., Urbanowicz, B. R., Zhang, J., Singan, V., et al. (2018). Association mapping, transcriptomics, and transient expression identify candidate genes mediating plant–pathogen interactions in a tree. Proc. Natl. Acad. Sci. U. S. A. 115, 11573–11578. doi:10.1073/pnas.1804428115
Naidoo, R., Ferreira, L., Berger, D., Myburg, A., and Naidoo, S. (2013). The identification and differential expression of Eucalyptus grandis pathogenesis-related genes in response to salicylic acid and methyl jasmonate. Front. Plant Sci. 4, 43. doi:10.3389/fpls.2013.00043
Newcombe, G., and Bradshaw, H. d. (1996). Quantitative trait loci conferring resistance in hybrid poplar to Septoriapopulicola, the cause of leaf spot. Can. J. For. Res. 26, 1943–1950. doi:10.1139/x26-219
Newcombe, G., and Ostry, M. (2001). Recessive resistance to septoria stem canker of hybrid poplar. Phytopathology 91, 1081–1084. doi:10.1094/PHYTO.2001.91.11.1081
Nuruzzaman, M., Zhang, R., Cao, H.-Z., and Luo, Z.-Y. (2014). Plant pleiotropic drug resistance transporters: Transport mechanism, gene expression, and function. J. Integr. Plant Biol. 56, 729–740. doi:10.1111/jipb.12196
Ohm, R. A., Feau, N., Henrissat, B., Schoch, C. L., Horwitz, B. A., Barry, K. W., et al. (2012). Diverse lifestyles and strategies of plant pathogenesis encoded in the genomes of eighteen dothideomycetes fungi. PLoS Pathog. 8, e1003037. doi:10.1371/journal.ppat.1003037
Palma, K., Zhao, Q., Cheng, Y. T., Bi, D., Monaghan, J., Cheng, W., et al. (2007). Regulation of plant innate immunity by three proteins in a complex conserved across the plant and animal kingdoms. Genes Dev. 21, 1484–1493. doi:10.1101/gad.1559607
Pang, Z., Chong, J., Zhou, G., de Lima Morais, D. A., Chang, L., Barrette, M., et al. (2021). MetaboAnalyst 5.0: Narrowing the gap between raw spectra and functional insights. Nucleic Acids Res. 49 (W1), W388–W396. doi:10.1093/nar/gkab382
Petre, B., Hacquard, S., Duplessis, S., and Rouhier, N. (2014). Genome analysis of poplar LRR-RLP gene clusters reveals RISP, a defense-related gene coding a candidate endogenous peptide elicitor. Front. Plant Sci. 5, 111. doi:10.3389/fpls.2014.00111
Pieterse, C. M. J., Leon-Reyes, A., Van der Ent, S., and Van Wees, S. C. M. (2009). Networking by small-molecule hormones in plant immunity. Nat. Chem. Biol. 5, 308–316. doi:10.1038/nchembio.164
Plett, J. M., and Martin, F. M. (2018). Know your enemy, embrace your friend: Using omics to understand how plants respond differently to pathogenic and mutualistic microorganisms. Plant J. 93, 729–746. doi:10.1111/tpj.13802
Polpitiya, A. D., Qian, W.-J., Jaitly, N., Petyuk, V. A., Adkins, J. N., Camp, D. G., et al. (2008). DAnTE: A statistical tool for quantitative analysis of -omics data. Bioinformatics 24, 1556–1558. doi:10.1093/bioinformatics/btn217
Potts, B. M., Sandhu, K. S., Wardlaw, T., Freeman, J., Li, H., Tilyard, P., et al. (2016). Evolutionary history shapes the susceptibility of an island tree flora to an exotic pathogen. For. Ecol. Manag. 368, 183–193. doi:10.1016/j.foreco.2016.02.027
Pruitt, R. N., Gust, A. A., and Nürnberger, T. (2021). Plant immunity unified. Nat. Plants 7, 382–383. doi:10.1038/s41477-021-00903-3
Qi, Y., Tsuda, K., Joe, A., Sato, M., Nguyen, L. V., Glazebrook, J., et al. (2010). A putative RNA-binding protein positively regulates salicylic acid–mediated immunity in Arabidopsis. Mol. Plant. Microbe. Interact. 23, 1573–1583. doi:10.1094/MPMI-05-10-0106
Qiao, Z., Yates, T. B., Shrestha, H. K., Engle, N. L., Flanagan, A., Morrell-Falvey, J. L., et al. (2021). Towards engineering ectomycorrhization into switchgrass bioenergy crops via a lectin receptor-like kinase. Plant Biotechnol. J. 19, 2454–2468. doi:10.1111/pbi.13671
Qin, R., and LeBoldus, J. M. (2014). The infection biology of Sphaerulina musiva: Clues to understanding a forest pathogen. PLOS ONE 9, e103477. doi:10.1371/journal.pone.0103477
Rampitsch, C., and Bykova, N. V. (2012). Proteomics and plant disease: Advances in combating a major threat to the global food supply. PROTEOMICS 12, 673–690. doi:10.1002/pmic.201100359
Sakamoto, H., Araki, T., Meshi, T., and Iwabuchi, M. (2000). Expression of a subset of the Arabidopsis Cys2/His2-type zinc-finger protein gene family under water stress. Gene 248, 23–32. doi:10.1016/S0378-1119(00)00133-5
Sanyal, S. K., Kanwar, P., Yadav, A. K., Sharma, C., Kumar, A., and Pandey, G. K. (2017). Arabidopsis CBL interacting protein kinase 3 interacts with ABR1, an APETALA2 domain transcription factor, to regulate ABA responses. Plant Sci. 254, 48–59. doi:10.1016/j.plantsci.2016.11.004
Schmitt, A. J., Sathoff, A. E., Holl, C., Bauer, B., Samac, D. A., and Carter, C. J. (2018). The major nectar protein of Brassica rapa is a non-specific lipid transfer protein, BrLTP2.1, with strong antifungal activity. J. Exp. Bot. 69, 5587–5597. doi:10.1093/jxb/ery319
Sels, J., Mathys, J., De Coninck, B. M. A., Cammue, B. P. A., and De Bolle, M. F. C. (2008). Plant pathogenesis-related (PR) proteins: A focus on PR peptides. Plant Physiol. Biochem. 46 (11), 941–950. doi:10.1016/j.plaphy.2008.06.011
Singh, P., Arif, Y., Bajguz, A., and Hayat, S. (2021). The role of quercetin in plants. Plant Physiol. biochem. 166, 10–19. doi:10.1016/j.plaphy.2021.05.023
Smilde, A. K., Jansen, J. J., Hoefsloot, H. C. J., Lamers, R.-J. A. N., van der Greef, J., and Timmerman, M. E. (2005). ANOVA-Simultaneous component analysis (ASCA): A new tool for analyzing designed metabolomics data. Bioinformatics 21, 3043–3048. doi:10.1093/bioinformatics/bti476
Sondreli, K. L., Keriö, S., Frost, K., Muchero, W., Chen, J.-G., Haiby, K., et al. (2020). Outbreak of septoria canker caused by Sphaerulina musiva on Populus trichocarpa in eastern Oregon. Plant Dis. 104, 3266. doi:10.1094/PDIS-03-20-0494-PDN
Stampfl, H., Fritz, M., Dal Santo, S., and Jonak, C. (2016). The GSK3/shaggy-like kinase ASKα contributes to pattern-triggered immunity. Plant Physiol. 171, 1366–1377. doi:10.1104/pp.15.01741
Stanosz, J. C., and Stanosz, G. R. (2002). A medium to enhance identification of Septoria musiva from poplar cankers. For. Pathol. 32, 145–152. doi:10.1046/j.1439-0329.2002.00278.x
Sticher, L., Mauch-Mani, B., and Métraux, J. P. (1997). Systemic acquired resistance. Annu. Rev. Phytopathol. 35, 235–270. doi:10.1146/annurev.phyto.35.1.235
Tan, X., Meyers, B. C., Kozik, A., West, M. A., Morgante, M., St Clair, D. A., et al. (2007). Global expression analysis of nucleotide binding site-leucine rich repeat-encoding and related genes in Arabidopsis. BMC Plant Biol. 7, 56. doi:10.1186/1471-2229-7-56
Thor, K. (2019). Calcium—nutrient and messenger. Front. Plant Sci. 10, 440. doi:10.3389/fpls.2019.00440
Trupiano, D., Rocco, M., Renzone, G., Scaloni, A., Rossi, M., Viscosi, V., et al. (2014). Temporal analysis of poplar woody root response to bending stress. Physiol. Plant. 150, 174–193. doi:10.1111/ppl.12072
Tuskan, G. A., DiFazio, S., Jansson, S., Bohlmann, J., Grigoriev, I., Hellsten, U., et al. (2006). The genome of black cottonwood, Populus trichocarpa (torr. & gray). Science 313, 1596–1604. doi:10.1126/science.1128691
Tyanova, S., Temu, T., Sinitcyn, P., Carlson, A., Hein, M. Y., Geiger, T., et al. (2016). The Perseus computational platform for comprehensive analysis of (prote)omics data. Nat. Methods 13, 731–740. doi:10.1038/nmeth.3901
Underwood, W. (2012). The plant cell wall: A dynamic barrier against pathogen invasion. Front. Plant Sci. 3, 85. doi:10.3389/fpls.2012.00085
van der Burgh, A. M., Postma, J., Robatzek, S., and Joosten, M. H. A. J. (2019). Kinase activity of SOBIR1 and BAK1 is required for immune signalling. Mol. Plant Pathol. 20, 410–422. doi:10.1111/mpp.12767
Vu, T., Riekeberg, E., Qiu, Y., and Powers, R. (2018). Comparing normalization methods and the impact of noise. Metabolomics 14, 108. doi:10.1007/s11306-018-1400-6
Waterman, A. M. (1955). Septoria canker of poplars in the United States. Washington, DC: US Dep Agric Circ, 947.
Weiland, J. E., and Stanosz, G. R. (2007). The histology of hybrid poplar clones inoculated with septoria musiva. Plant Dis. 91, 1524–1530. doi:10.1094/PDIS-91-12-1524
Wu, X., Lai, Y., Rao, S., Lv, L., Ji, M., Han, K., et al. (2021). Genome-wide identification reveals that nicotiana benthamiana hypersensitive response (HR)-Like lesion inducing protein 4 (NbHRLI4) mediates cell death and salicylic acid-dependent defense responses to turnip mosaic virus. Front. Plant Sci. 12, 627315. doi:10.3389/fpls.2021.627315
Keywords: proteome, septoria, resistance, susceptability, populus
Citation: Lenz RR, Shrestha HK, Carrell AA, Labbé J, Hettich RL, Abraham PE and LeBoldus JM (2022) Proteomics reveals pathways linked to septoria canker resistance and susceptibility in Populus trichocarpa. Front. Anal. Sci. 2:1020111. doi: 10.3389/frans.2022.1020111
Received: 15 August 2022; Accepted: 29 November 2022;
Published: 21 December 2022.
Edited by:
Ljiljana Pasa-Tolic, Pacific Northwest National Laboratory (DOE), United StatesReviewed by:
Guanghui Han, BGI Americas, United StatesMicaela Peppino, Universidad Nacional de Córdoba, Argentina
Copyright © 2022 Lenz, Shrestha, Carrell, Labbé, Hettich, Abraham and LeBoldus. This is an open-access article distributed under the terms of the Creative Commons Attribution License (CC BY). The use, distribution or reproduction in other forums is permitted, provided the original author(s) and the copyright owner(s) are credited and that the original publication in this journal is cited, in accordance with accepted academic practice. No use, distribution or reproduction is permitted which does not comply with these terms.
*Correspondence: Paul E. Abraham, abrahampe@ornl.gov; Jared M. LeBoldus, jared.leboldus@oregonstate.edu