- 1Periodontal Research Group, Dentistry, School of Health Sciences, University of Birmingham and Birmingham Dental Hospital (Birmingham Community Healthcare Trust), Birmingham, United Kingdom
- 2School of Health Sciences, University of Birmingham, Birmingham, United Kingdom
- 3Epidermolysis Bullosa Unit, Department of Dermatology, University Hospitals Birmingham, National Health Service Foundation Trust, Birmingham, United Kingdom
- 4Faculty of Medicine, Suez Canal University, Ismailia, Egypt
- 5School of Science and Technology, Nottingham Trent University, Nottingham, United Kingdom
- 6National Institute for Health and Care Research (NIHR) Birmingham Biomedical Research Centre, University of Birmingham, Birmingham, United Kingdom
- 7Department of Periodontology, Operative and Preventive Dentistry, University of Bonn, Bonn, Germany
Introduction: The cutaneous microbiome plays an essential role in guarding against invasive pathogens and maintaining healthy skin homeostasis. Several studies have demonstrated the importance of a healthy skin microbiome through its alteration in several diseases. Differing skin characteristics across the body (temperature, pH, humidity) create distinct ecological niches inhabited by diverse microbial communities. The study of cutaneous microbiota is further complicated by numerous variables at all stages of investigation, including study design, skin sampling method, sample storage, sample processing, sequencing, and data analysis. Utilisation of standardised approaches is critical for reproducibility and comparison between skin microbiome studies. However, there is a notable lack of standardisation of sampling methodologies in the literature. Studies have employed differing sampling strategies and conditions which may affect microbiota characterisation.
Methods: Antecubital fossa was sampled from sixteen individuals using sterile dry cotton swabs or eSwabs. Sterile phosphate buffered saline, or 0.9% sterile saline were used as moistening solutions. Samples were then either stored at room temperature for 30 minutes or stored at -80°C for at least 24 hours before processing. Cutaneous microbiome was identified using 16S sequencing.
Results: Comparative analysis determined whether the type of swab (cotton/eSwab), moistening solution (saline solution/phosphate buffered saline), duration of swabbing (30 sec/1 min), and sample storage temperature (room temperature/-80°C) affect sampling and identification of skin microbial communities. Comparison of the total DNA yield extracted using different conditions showed that while moistening solution, duration of swabbing, and storage conditions did not affect the total DNA amount, using eSwabs yielded higher biomass.
Discussion: Sampling approaches are critical for the success of sequencing. The conditions investigated in this study did not influence microbiome profiling allowing consistent sampling of the microbiota. However, data clustering was affected more by individual subject than by the conditions investigated, suggesting the importance of recognizing inter-individual variability as an important factor in real-life skin microbiome studies.
1 Introduction
Over the last 25 years, the study of skin microbes and their relationships with human hosts has attracted growing interest, aided by advances in sequencing technologies and bioinformatic tools. The scientific community has investigated the cutaneous microbiome in health and disease, including atopic dermatitis, acne vulgaris, psoriasis, hidradenitis suppurativa, seborrheic dermatitis, rosacea, lichen sclerosis, and alopecia areata (Langan et al., 2020; Chattopadhyay et al., 2021; Carmona-Cruz et al., 2022; Condrò et al., 2022; Sánchez-Pellicer et al., 2022). Besides the technological advances, uniform study design, standardised methods, methodological validation, and reproducible data analysis are important features for accurate interpretation and comparison of microbiome studies (Kong et al., 2017). The rigour necessary for reproducible sampling and analysis can render skin microbiome research an arduous undertaking. Indeed, the assiduousness required has prompted this dynamic to be referred to as ‘a method to the madness’ (Kong et al., 2017).
Studies are further complicated by the diversity of the ecological niches that microbes inhabit within specific sites of the human body; the cutaneous microbial communities not only vary between individuals but also between body sites within the same individual according to local skin properties (eg. oily, moist, dry, pressure bearing area), as reviewed in Byrd et al., 2018. Moreover, metagenomic analyses of human skin microbiomes are associated with very low microbial biomass (generally in the pg and ng range), high risk of contamination, and complexity in isolating enough DNA for sequencing and downstream analyses (Kong et al., 2017; Byrd et al., 2018; Bjerre et al., 2019; Bay et al., 2020). Optimised methodologies have been developed; nevertheless, they are affected by numerous variables in all stages, including study design, but also skin sampling method, sample storage, sample processing, sequencing method, and data analysis (Kong et al., 2017).
Employing standardised approaches is critical for ensuring reliable, reproducible, and comparable skin microbiome studies. Sampling techniques such as skin biopsies, tape stripping, skin scrapes, and skin swabs differ in biomass yield, human DNA contamination, discomfort, and ultimately microbial communities identified (Kong et al., 2017). Commercially available kits for skin microbiome DNA extraction have been developed with different strategies to disrupt cells, such as thermal lysis and enzymatic or mechanical disruption. Bjerre et al., 2019 compared 12 commercially available kits highlighting different characteristics, success rates, and isolation of microbial communities. The study concluded that choice of DNA extraction strategy affects the microbial profile, but no more than inter-individual variation.
Despite a “manual of procedures” being published in 2012 (Methé et al., 2012), we found marked heterogeneity in the scientific literature on the type of swabs employed for microbiota sampling, such as catch-all swabs (Aagaard et al., 2013; Chng et al., 2016; Naik et al., 2020; Moitinho-Silva et al., 2022), eSwabs (Riverain-Gillet et al., 2020), or cotton swabs (Schowalter et al., 2010; Ogai et al., 2018; Schneider et al., 2022; Zhou et al., 2022). In addition, we found variability in the moistening solutions employed and in the duration of swabbing (ranging from 20 seconds to 1 minute and from 10 times to 100 times) (Table 1).
Identifying optimal conditions prior to sampling and subsequent DNA extraction is challenging, time-consuming, and critical for successful microbiome metagenomic analysis. In this study we performed a comparative analysis to determine whether a subset of conditions not previously systematically reported in the scientific literature impact upon skin microbiome analysis.
To our knowledge, this is the first study to compare the effects of type of swabs, moistening conditions, duration of swabbing, and sample storage temperature upon the collection and identification of skin microbial communities using 16S sequencing. We determined that the reported skin swabbing strategies do not affect microbiota sampling under the conditions investigated. This is an important finding that suggests generalisability of findings from the existing cutaneous microbiome literature.
2 Results
2.1 Type of swab affects total biomass yield
Sixteen healthy volunteers (12 females, 4 males) aged 18 to 46 years were included in the study. In total, 48 samples were collected. We utilised different conditions to sample microbial communities from the antecubital fossa: cotton swabs/flocked nylon swabs (eSwabs); pre-moistened with saline solution/PBS; swabbing for 30 seconds/1 minute; and storage at RT/-80°C (Figure 1a). Total DNA was extracted from the swabs and quantified using a Qubit, as described in the methods section. The DNA yield extracted from the swabs ranged between 1.87 ng and 30.25 ng, in agreement with (Bjerre et al., 2019, whose study isolated DNA between 0.3 and 29.7 ng. Significant differences in concentrations of isolated total DNA were observed using different conditions (Figure 1b). Cotton swabs yielded an average of 5 ng in total (range 1.87 to 10.95 ng). In contrast, eSwabs yielded an average of 22.48 ng (range 12.8 to 30.25 ng) (Figure 1c). The moistening solution employed (Figure 1d), duration of swabbing (Figure 1e), and storage conditions (Figure 1f), did not affect average total DNA yield.
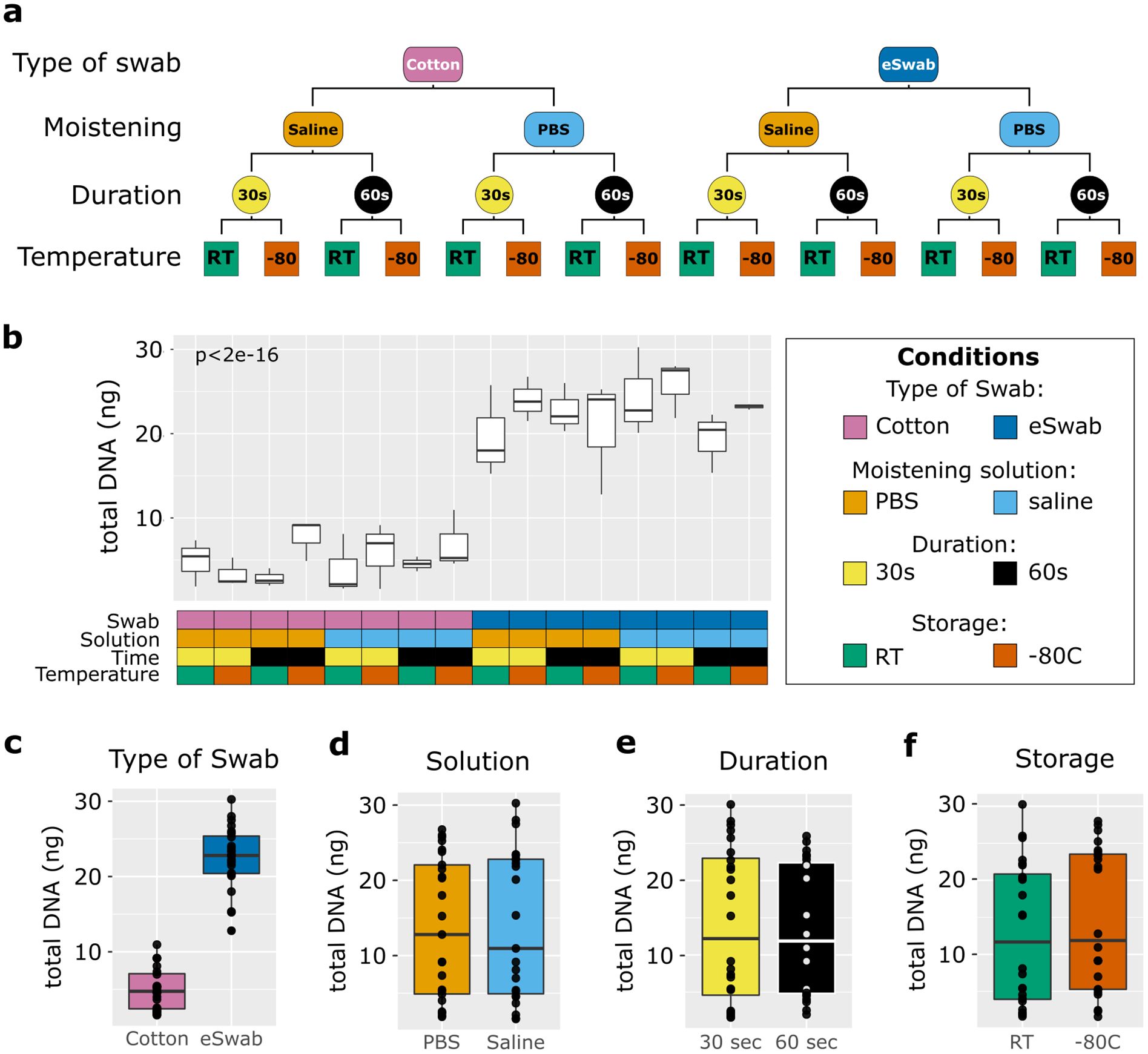
Figure 1. Study design and total biomass extracted. (a) The scheme shows the study design. Four conditions were investigated: type of swab (cotton vs eSwab), moistening solution (0.9% saline vs Phosphate buffered saline-PBS), duration of swabbing (30 seconds vs 1 minute), and storage condition (Room temperature vs -80°C). (b) Total biomass yield expressed as total DNA (ng) extracted was measured for each of the conditions investigated, which are summarised in the grid below and colour-coded. The effects on the total DNA yield were also investigated by condition separately such as (c) type of swab, (d) moistening solution used, (e) duration of swabbing, (f) storage conditions.
Microbial communities were identified with 16S rRNA gene sequencing (BioProject PRJNA940670). Library preparation failed for four samples, not correlated with the conditions used. In fact, among these four, one sample was collected with cotton swabs using PBS for 1 minute and directly processed at RT, one sample was collected using eSwab and PBS for 30 seconds and processed at RT, one sample was collected using eSwab and saline solution for 30 seconds and stored at -80°C, and one sample was collected with eSwab using saline solution for one minute and processed at RT (Supplementary Table 1). In total 11,488,003 reads were generated for the 44 samples. The minimal number of reads in a sample was 37,506, the maximum 457,005, and with a median of 270,318.
2.2 Skin swab strategies captured similar microbial communities
We investigated the microbial communities from samples collected under different conditions without identifying any major differences across groups. Independently of the conditions employed to take samples, the most abundant operational taxonomic units (OTUs) were Cutibacterium, Staphylococcus, Corynebacterium, Propionibacterium, Bacillaceae (undefined group) and Streptococcus (Figure 2a), with a relatively minor impact on Shannon-alpha Diversity (Figure 2b), and Chao1 richness (Figure 2c) indices (Supplementary Data TS1). Linear regression analysis of Shannon and Simpson indices did not highlight any significant difference. Furthermore, to identify the impact of the investigated conditions on the sampling of selected genera of bacterial residents commonly found on the skin, we compared their relative abundance sampled using different conditions (Figure 3). We investigated the relative abundances of Cutibacterium (Figure 3a), Corynebacterium (Figure 3b), Propionibacterium (Figure 3c), Staphylococcus (Figure 3d), and Streptococcus (Figure 3e). While we could detect inter-individual variability of the selected genera, no significant difference between conditions was observed. Evaluation of beta-diversity using Bray-Curtis and Jaccard dissimilarity did not reveal any clustering by conditions (Supplementary Figures S1, S2).
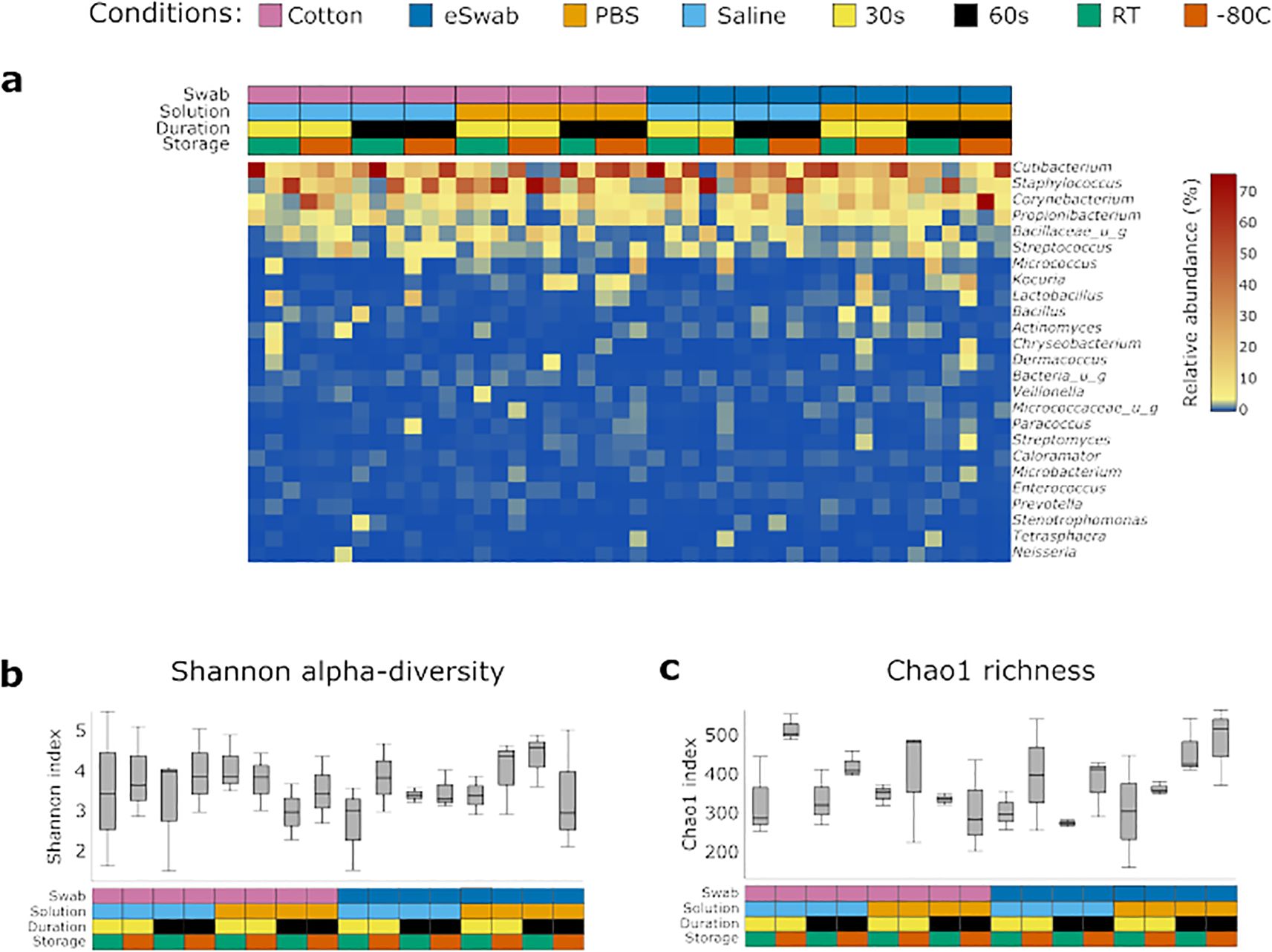
Figure 2. Identification of bacterial communities. (a) Bacterial profiles of each sample were obtained by 16S sequencing. The heatmap shows the relative abundance of the most 25 abundant genera. The conditions used to collect the samples are summarised in the grid above and are colour-coded. Diversity indexes were calculated for the microbial profile collected using the investigated conditions: (b) Shannon alpha-diversity index, (c) Chao1 richness index. The conditions used to collect the samples are summarised in the grid below and are colour coded.
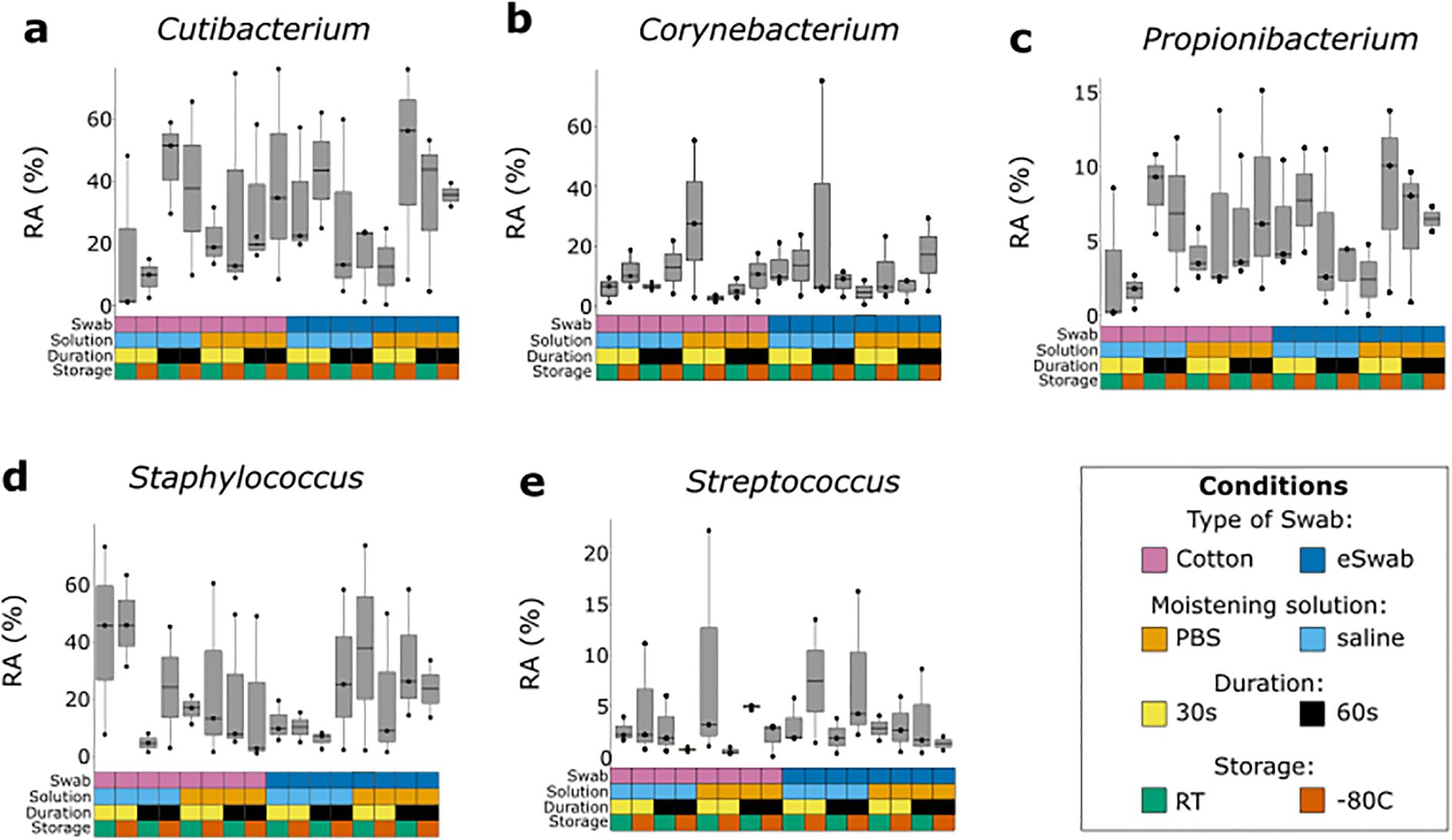
Figure 3. Relative abundance of most common bacterial genera sampled with different conditions. Each genus was investigated using different sampling conditions. Each box and whisker plot represents the relative abundance (RA) of a specific genus sampled from three different individuals using a specific condition, indicated by the grid below and colour-coded according to the legend. (a) Relative abundance of Cutibacterium. (b) Relative abundance of Corynebacterium. (c) Relative abundance of Propionibacterium. (d) Relative abundance of Staphylococcus. (e) Relative abundance of Streptococcus.
2.3 Inter-individual variability
Inter-individual variability was considered in the proposed studies. We clustered the samples by similarity (Supplementary Figure S3) and performed PCA (Supplementary Figure S4), observing that samples clustered by patient ID regardless of the condition used. We investigated the relative abundance of the 10 most abundant genera for each sample and grouped by individual to visualise inter-individual variability among samples (Figure 4a). In addition, Shannon and Chao1 diversity indices showed consistency of the individual microbiome profile (Figures 4b, c). We observed differences between individuals not dependent upon type of swab, moistening solution, duration, nor storage. Evaluation of beta-diversity using Bray-Curtis and Jaccard dissimilarity supported grouping by patient ID (Supplementary Figures S5, S6).
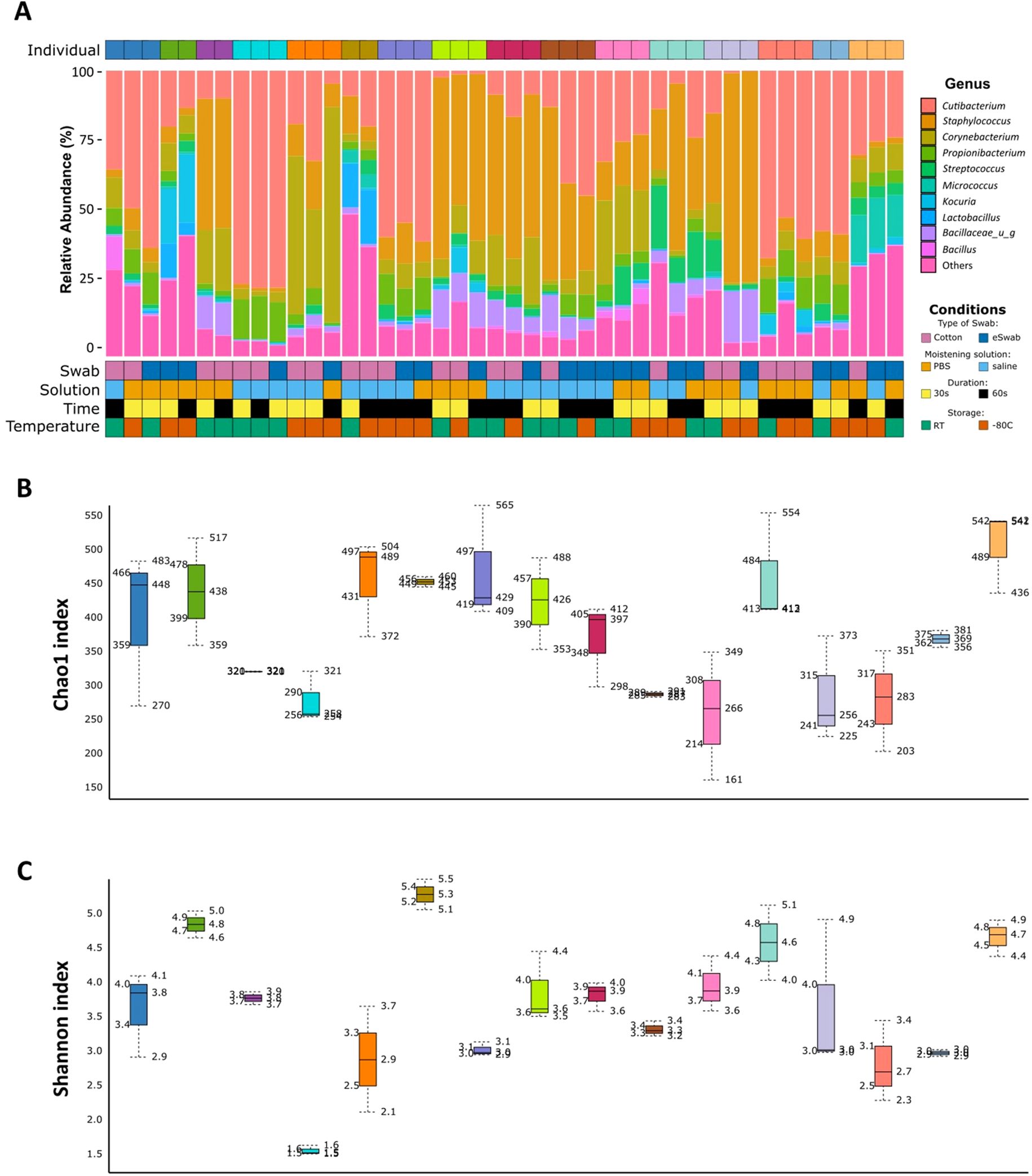
Figure 4. Inter-individual variability. (a) Relative abundance (%) of the 10 most abundant identified genera for each sample. Each individual is identified by a different colour in the top grid. Conditions used for sampling are identified for each sample and shown in the bottom grid. (b) Chao1 diversity index is shown for each patient. Each box represents an individual and is indicated by a different colour. (c) Shannon diversity index is shown for each patient. Each box represents an individual and is indicated by a different colour.
3 Discussion
Human skin is inhabited by a complex variety of microorganisms, including bacteria, fungi, and viruses. The cutaneous microbiome plays a pivotal role in maintenance of skin homeostasis and immune responses. Variations of the skin microbiome are observed between different body sites according to their skin properties (eg. oily, moist, dry, and foot) (Byrd et al., 2018; Nathan et al., 2023). Characterisation of the cutaneous microbiome at an eubiotic state is essential to understand the changes observed in disease states. Nonetheless, different sampling and sequencing approaches can affect microbiome profiling, making the use of standardised operative procedures necessary. However, regardless of the availability of a “manual of procedures” provided by the microbiome consortium (Methé et al., 2012), studies have used heterogeneous sampling approaches.
For this reason, we compared a subset of the conditions used for sampling the cutaneous microbiome utilising swabs with subsequent 16S ribosomal RNA gene sequencing. Previously Bjerre et al., 2019 extensively studied the effect of sampling strategies and DNA extraction methods, comparing eSwabs and skin scrapes, body sites, and several DNA extraction kits. This study found that data collected using eSwabs were more consistent. In addition, Chng et al., 2016 compared tape stripping, Catch-All Sample Collection Swab, and cup scrub sampling methods, while Grice et al., 2008 compared cotton swabs, scrapes, and punch biopsies. Swabs are the least invasive technique and therefore may be the preferred method for certain indications, acceptability to participants, or disease states where risks of overt infection, skin fragility or delayed healing may be considerations. Klymiuk et al., 2016 compared the effects of storage periods (24h, 90 days, and 365 days at -80°C) of skin swab samples in 8 healthy individuals in three body sites. The study found no differences in richness nor Shannon indices among the groups. Interestingly, differences in the ratios of the most abundant taxa at different time points and different body sites were observed. However, the authors state that while these results may indicate biological differences, they could also represent technical artifacts caused by different DNA isolation kit batches used over time.
In our study, we investigated whether the type of swab used, moistening solution, duration of swabbing, and storage temperature affect microbiome sampling (Figure 1). Notably, other conditions such as body site and pressure of swabbing may cause different biomass yields, and further studies are required to determine their impact. However, to limit the impact of these factors in our study, we sampled a single body site (antecubital fossa), and only two researchers collected the samples.
We compared the total DNA yield extracted using different conditions and found that while moistening solution, duration of swabbing, and storage conditions did not affect the total DNA amount (Figure 1), using eSwabs yielded higher biomass (Figure 1c). Importantly, the total DNA yield included human DNA, an essential factor to consider as contamination by host DNA may interfere with the downstream analysis, especially when performing metagenomic sequencing (Pereira-Marques et al., 2019). Pereira-Marques et al. have demonstrated that high levels of host DNA decrease the sensitivity of whole genome sequencing for microbial profiling, in particular affecting the detection of low-abundant organisms. Whilst host DNA depletion methods can be effective to improve microbial profiling, they also pose disadvantages, such as the requirement of fresh samples, intact living bacteria, high molecular weight intact DNA, and introduce a bias favouring high CpG methylated bacteria (Shi et al., 2022). Computational filtering of human genome-mapped reads is commonly used to remove host contamination; however, increased computational power is required and sequencing sensitivity will be reduced. This is an important factor to consider when selecting the sampling methodology and sequencing approach, especially when investigating fragile skin diseases, where a higher percentage of host DNA compared to healthy individuals is expected. Limiting the collection of human skin using cotton swabs rather than eSwabs may benefit the overall investigation. However, further investigations, such as 16S copy number quantification, are required to determine the relative proportions of human and microbial DNA collected via sampling by cotton swabs and eSwabs respectively. We hypothesise that eSwabs, being rougher on the surface, harvest more human cells than cotton swabs, increasing the total DNA yield. However, in our study, we did not find any correlation between the yield of total DNA (including host DNA) and number of microbial reads, richness and evenness indexes.
In this study, microbial communities were investigated using 16S sequencing. We did not identify any significant differences in diversity indices using different conditions (Figure 2). Lauber et al., 2010 have previously investigated the effect of storage temperature and duration of storage on microbial profiling assessed using barcoded pyrosequencing of 16S rRNA without identifying any differences between these parameters. This is in keeping with our findings obtained using Illumina technologies, which are now routinely employed in sequencing studies. In addition, as the data presented in this study were collected from several patients, we performed an inter-individual analysis to investigate the variability of the samples among individuals (Figure 4). In fact, studies have shown inter-individual differences in the cutaneous microbiome despite matching for body site and age (Kong et al., 2017). The analysis highlighted not only variability between individuals, but also intra-individual variability. While we investigated robustness of sampling methods in a cohort with several individuals to ensure our data was generalisable to real-life studies, a study design evaluating the effects of sampling methods on the skin microbiome within the same individuals would provide further important insights. We observed heterogeneity of skin microbiome composition between individuals; therefore, our statistical analyses were performed using a mixed model for multiple comparisons to account for samples obtained from the same or different individuals. We acknowledge that our study has limitations such as the inclusion of a cotton swab, without skin contact, moistened with saline solution and frozen at -80°C as a negative control and a lack of negative controls for all the remaining conditions, no standardisation of skin preparation, the use of buffers without detergents due to the concern of the effects of detergents on some patient populations, and small sample size. Notably, library preparation of four samples failed; no clear cause was identified. In addition, our results are specific to the antecubital fossa, and the variables investigated may have a higher impact upon other body sites. It therefore remains fundamental to include a detailed description of the sampling strategy in all cutaneous microbiome investigations.
4 Conclusions
The conditions investigated in this pilot study can be used interchangeably to study the skin microbiome. Cotton swabs may be preferable in studies that involve fragile skin diseases as they yielded less total biomass thereby limiting host contamination without affecting downstream sequencing and bioinformatics analyses. Sampling approaches are critical for the success of sequencing, and other conditions such as DNA extraction method, study design, and bioinformatic pipeline used add substantial variation to skin microbiome studies. Data clustering was affected more by individual subject than by the conditions investigated, suggesting that, whilst the conditions investigated do not impact microbiota sampling, it is important to recognise inter-individual variability as an important factor in real-life skin microbiome studies.
5 Materials and methods
5.1 Study design
Sixteen individuals aged between 18 to 46 years with no history of inflammatory disorders (including skin disease), not taking systemic medications nor applying prescribed topical antimicrobials or topical steroids were selected via verbal screening and medical history taking. Ethical approval (Reference 19/SW/0198) and written informed consent from participants were obtained. Individuals were screened during the middle of the working day/lunchtime so that no recent showering or bathing would have taken place. Each individual was asked to donate 3 swabs with 3 different conditions randomly assigned using R, for a total of 48 samples, to ensure collection in triplicates for each condition and inclusion of interpersonal variability. Swabs were collected from the antecubital fossa from non-overlapping areas of about 1 cm2. Each of the three samples donated by the same individual were 1 cm apart on the skin surface. We used sterile dry cotton swabs (MWE, Cat. MW102) and eSwabs (COPAN, Cat. 480C). Sterile phosphate buffered saline, or 0.9% sterile saline were used as moistening solutions; samples were stored at room temperature for 30 minutes, then processed or stored at -80°C for at least 24 hours (Figure 1a). To limit pressure variability and technical differences, swabbing was performed only by authors DLB and AB, and according to a standard operating protocol.
The negative control included in the study was obtained using cotton swabs from the same batch of swabs, without skin contact, moistened with saline solution and frozen at -80°C (Supplementary Figure S3).
5.2 DNA extraction
DNA was extracted from skin swabs using the QIAmp DNA Investigator kit (Qiagen, Cat. 56504) and QIAshredders homogenisers (Qiagen, Cat. 79656). DNA was quantified using DNA HS kit (ThermoFisher, Massachusetts, USA) and Qubit (ThermoFisher, Massachusetts, USA). All kits were used according to the manufacturers’ instructions.
5.3 16S sequencing and taxonomic profile
Isolated DNA was shipped to CosmosID (Germantown, MD, USA) for library preparation and sequencing. Vendor optimised protocol was used. Briefly, genomic DNA was amplified via PCR with primers 27F (AGAGTTTGATCCTGGCTCAG) and 534R (ATTACCGCGGCTGCTGG) covering hypervariable regions V1 and V3. Final libraries’ quantity and quality were assessed by Qubit 2.0 (ThermoFisher, Massachusetts, USA) and TapeStation D1000 ScreenTape (Agilent Technologies Inc., California, USA) respectively. Sequencing was performed on Illumina® Miseq (Illumina, California, USA) with a read length per sample of 500K in each direction.
For taxonomic profiling based on amplicon data, the CosmosID 16S data analysis was used (CosmosID Inc, 2022). Briefly, raw reads were first trimmed to remove adapters and bases of low quality and forward and reverse overlapping pairs were joined. OUTs were assigned using the CosmosID curated 16S database using a close-reference OUT picker and 97% sequence similarity through the QIIME framework. Data exploration and visualisation, and comparative analyses were performed using the CosmosID-Hub(CosmosID Inc [no date]).
5.4 Statistical analyses
Linear regression using mixed model multiple comparisons was performed in R using the lm4, lmerTest and multcom packages using Tukey’s Honest Significant Difference (HDS) test. Microbiome statistical analyses were performed by using the CosmosID comparative analyses tool (CosmosID Inc). Alpha-diversity and richness were calculated using Shannon index, Simpson index, and Chao1 richness. Beta diversity was estimated as Jaccard and Bray-Curtis divergence and compared by permutational multivariate analysis of variance (PERMANOVA). For all analyses, p ≤ 0.05 was considered statistically significant.
Data availability statement
The original contributions presented in the study are publicly available. This data can be found here: NCBI BioProject, accession PRJNA940670.
Ethics statement
The studies involving humans were approved by University of Birmingham (Reference 19/SW/0198). The studies were conducted in accordance with the local legislation and institutional requirements. The participants provided their written informed consent to participate in this study.
Author contributions
DB: Conceptualization, Data curation, Formal analysis, Investigation, Methodology, Software, Visualization, Writing – original draft, Writing – review & editing. AB: Data curation, Formal analysis, Investigation, Writing – original draft, Writing – review & editing. HI: Investigation, Writing – original draft, Writing – review & editing. SK: Conceptualization, Funding acquisition, Investigation, Methodology, Project administration, Resources, Supervision, Writing – original draft, Writing – review & editing. MG: Conceptualization, Funding acquisition, Investigation, Methodology, Project administration, Resources, Supervision, Writing – original draft, Writing – review & editing. JH: Conceptualization, Funding acquisition, Investigation, Methodology, Project administration, Resources, Supervision, Writing – original draft, Writing – review & editing. AH: Conceptualization, Funding acquisition, Investigation, Methodology, Project administration, Resources, Supervision, Writing – original draft, Writing – review & editing. IC: Conceptualization, Funding acquisition, Investigation, Methodology, Project administration, Resources, Supervision, Writing – original draft, Writing – review & editing.
Funding
The author(s) declare that financial support was received for the research and/or publication of this article. This work was supported by DEBRA UK.
Conflict of interest
The authors declare that the research was conducted in the absence of any commercial or financial relationships that could be construed as a potential conflict of interest.
Generative AI statement
The author(s) declare that no Generative AI was used in the creation of this manuscript.
Publisher’s note
All claims expressed in this article are solely those of the authors and do not necessarily represent those of their affiliated organizations, or those of the publisher, the editors and the reviewers. Any product that may be evaluated in this article, or claim that may be made by its manufacturer, is not guaranteed or endorsed by the publisher.
Supplementary material
The Supplementary Material for this article can be found online at: https://www.frontiersin.org/articles/10.3389/frmbi.2025.1559981/full#supplementary-material
References
Aagaard K., Petrosino J., Keitel W., Watson M., Katancik J., Garcia N., et al. (2013). The Human Microbiome Project strategy for comprehensive sampling of the human microbiome and why it matters. FASEB J. 27 (3), 1012–1022. doi: 10.1096/fj.12-220806
Ahle C. M., Stødkilde K., Afshar M., Poehlein A., Ogilvie L. A., Söderquist B., et al. (2020). Staphylococcus saccharolyticus: an overlooked human skin colonizer. Microorganisms 8 (8), 1–12. doi: 10.3390/microorganisms8081105
Ahle C. M., Stødkilde-Jørgensen K., Poehlein A., Streit W. R., Hüpeden J., Brüggemann H. (2021). Comparison of three amplicon sequencing approaches to determine staphylococcal populations on human skin. BMC Microbiol. 21 (1), 221. doi: 10.1186/S12866-021-02284-1
Ahluwalia J., Borok J., Haddock E. S., Ahluwalia R. S., Schwartz E. W., Hosseini D., et al. (2019). The microbiome in preadolescent acne: Assessment and prospective analysis of the influence of benzoyl peroxide. Pediatr. Dermatol. 36, 200–206. doi: 10.1111/PDE.13741
Akaza N., Takasaki K., Nishiyama E., Usui A., Miura S., Yokoi , et al. (2022). The microbiome in comedonal contents of inflammatory acne vulgaris is composed of an overgrowth of cutibacterium spp. and other cutaneous microorganisms. Clinical Cosmetic Investigational Dermatol. 15, 2003. doi: 10.2147/CCID.S379609
Assarsson M., Duvetorp A., Dienus O., Söderman J., Seifert O. (2018). Significant changes in the skin microbiome in patients with chronic plaque psoriasis after treatment with narrowband ultraviolet B. Acta Dermato-Venereologica 98, 428–436. doi: 10.2340/00015555-2859/
Bay L., Barnes C. J., Fritz B. G., Thorsen J., Restrup M. E. M., Rasmussen L., et al. (2020). Universal dermal microbiome in human skin. mBio 11, 1–13. doi: 10.1128/mBio.02945-19
Bayal N., Nagpal S., Haque M. M., Patole M. S., Valluri V., Suryavanshi R., et al. (2019). 16S rDNA based skin microbiome data of healthy individuals and leprosy patients from India. Sci. Data 6, 1–9. doi: 10.1038/s41597-019-0232-1
Bjerre R. D., Hugerth L. W., Boulund F., Seifert M., Johansen J. D., Engstrand L. (2019). Effects of sampling strategy and DNA extraction on human skin microbiome investigations. Sci. Rep. 9, 17287. doi: 10.1038/s41598-019-53599-z
Burnham C. A. D., Hogan P. G., Wallace M. A., Deych E., Shannon W., Warren D. K., et al. (2016). Topical decolonization does not eradicate the skin microbiota of community-dwelling or hospitalized adults. Antimicrobial. Agents Chemother. 60, 7303–7312. doi: 10.1128/AAC.01289-16/SUPPL_FILE/ZAC012165753SO1.PDF
Byrd A. L., Belkaid Y., Segre J. A. (2018). The human skin microbiome. Nat. Rev. Microbiol. 16, 143–155. doi: 10.1038/nrmicro.2017.157
Capone K. A., Friscia D. L., Nikolovski J., Telofski L. S., Stamatas G. N. (2022). A randomized clinical study on the effects of emollient use on the developing infant skin microbiome and metabolome. Exp. Dermatol. 32 (1), 75–77. doi: 10.1111/EXD.14684
Carmona-Cruz S., Orozco-Covarrubias L., Sáez-de-Ocariz M. (2022). The human skin microbiome in selected cutaneous diseases. Front. Cell. Infection Microbiol. 12. doi: 10.3389/FCIMB.2022.834135/BIBTEX
Chattopadhyay S., Arnold J. D., Malayil L., Hittle L., Mongodin E. F., Marathe K. S., et al. (2021). Potential role of the skin and gut microbiota in premenarchal vulvar lichen sclerosus: A pilot case-control study. PloS One 16 (1), e0245243. doi: 10.1371/JOURNAL.PONE.0245243
Chng K. R., Tay A. S., Li C., Ng A. H., Wang J., Suri B. K., et al. (2016). Whole metagenome profiling reveals skin microbiome-dependent susceptibility to atopic dermatitis flare. Nat Microbiol. 1 (9), 16106. doi: 10.1038/nmicrobiol.2016.106
Condrò G., Guerini M., Castello M., Perugini P. (2022). Acne vulgaris, atopic dermatitis and rosacea: the role of the skin microbiota-A review. Biomedicines 10 (10), 2523. doi: 10.3390/BIOMEDICINES10102523
CosmosID Inc CosmosID Metagenomics Cloud. (2022). Available online at: www.cosmosid.com (Accessed October 10, 2022).
Dekio I., Hayashi H., Sakamoto M., Kitahara M., Nishikawa T., Suematsu M., et al. (2005). Detection of potentially novel bacterial components of the human skin microbiota using culture-independent molecular profiling. J. Med. Microbiol. 54, 1231–1238. doi: 10.1099/JMM.0.46075-0/CITE/REFWORKS
Dekio I., Sakamoto M., Hayashi H., Amagai M., Suematsu M., Benno Y. (2007). Characterization of skin microbiota in patients with atopic dermatitis and in normal subjects using 16S rRNA gene-based comprehensive analysis. J. Med. Microbiol. 56, 1675–1683. doi: 10.1099/JMM.0.47268-0/CITE/REFWORKS
Dimitriu P. A., Iker B., Malik K., Leung H., Mohn W. W., Hillebrand G. G. (2019). New insights into the intrinsic and extrinsic factors that shape the human skin microbiome. mBio 10 (4), e00839–19. doi: 10.1128/MBIO.00839-19
Findley K., Oh J., Yang J., Conlan S., Deming C., Meyer J. A., et al. (2013). Topographic diversity of fungal and bacterial communities in human skin. Nature 498 (7454), 367–370. doi: 10.1038/nature12171
Grice E. A., Kong H. H., Renaud G., Young A. C., NISC Comparative Sequencing Program, Bouffard G. G., et al. (2008). A diversity profile of the human skin microbiota. Genome Res. 18, 1043–1050. doi: 10.1101/GR.075549.107
Hall J. B., Cong Z., Imamura-Kawasawa Y., Kidd B. A., Dudley J. T., Thiboutot D. M., et al. (2018). Isolation and identification of the follicular microbiome: implications for acne research. J. Invest. Dermatol. 138, 2033–2040. doi: 10.1016/j.jid.2018.02.038
Han S. H., Cheon H. I., Hur M. S., Kim M. J., Jung W. H., Lee Y. W., et al. (2018). Analysis of the skin mycobiome in adult patients with atopic dermatitis. Exp. Dermatol. 27, 366–373. doi: 10.1111/EXD.13500
Hsieh P., Chang C., Chen K., Cho Y., Chu C., Chen K. (2022). Temporal shifts of the microbiome associated with antibiotic treatment of purpuric drug eruptions related to epidermal growth factor receptor inhibitors. J. Eur. Acad. Dermatol. Venereol. 37 (2), 382–389. doi: 10.1111/JDV.18640
Huang Y., Xiao Z., Cao Y., Gao F., Fu Y., Zou M., et al. (2022). Rapid microbiological diagnosis based on 16S rRNA gene sequencing: A comparison of bacterial composition in diabetic foot infections and contralateral intact skin. Front. Microbiol. 13, 1021955. doi: 10.3389/FMICB.2022.1021955
Hwang B. K., Lee S., Myoung J., Hwang S. J., Lim J. M., Jeong E. T., et al. (2021). Effect of the skincare product on facial skin microbial structure and biophysical parameters: A pilot study. MicrobiologyOpen 10 (5), e1236. doi: 10.1002/MBO3.1236
Jugé R., Rouaud-Tinguely P., Breugnot J., Servaes K., Grimaldi C., Roth M. P., et al. (2018). Shift in skin microbiota of Western European women across aging. J. Appl. Microbiol. 125, 907–916. doi: 10.1111/JAM.13929
Kang Y., Ji X., Guo L., Xia H., Yang X., Xie Z, et al. (2021). Cerebrospinal fluid from healthy pregnant women does not harbor a detectable microbial community. Microbiol. Spectr. 9 (3), e0076921. doi: 10.1128/SPECTRUM.00769-21
Kelhälä H. L., Aho V. T. E., Fyhrquist N., Pereira P. A. B., Kubin M. E., Paulin , et al. (2018). Isotretinoin and lymecycline treatments modify the skin microbiota in acne. Exp. Dermatol. 27, 30–36. doi: 10.1111/EXD.13397
Khayyira A. S., Rosdina A. E., Irianti M. I., Malik A. (2020). Simultaneous profiling and cultivation of the skin microbiome of healthy young adult skin for the development of therapeutic agents. Heliyon 6, e03700. doi: 10.1016/J.HELIYON.2020.E03700
Klymiuk I., Bambach I., Patra V., Trajanoski S., Wolf P. (2016). 16S based microbiome analysis from healthy subjects’ skin swabs stored for different storage periods reveal phylum to genus level changes. Front. Microbiol. 7. doi: 10.3389/fmicb.2016.02012
Koike Y., Kuwatsuka S., Nishimoto K., Motooka D., Murota H. (2020). Skin mycobiome of psoriasis patients is retained during treatment with TNF and IL-17 inhibitors. Int. J. Mol. Sci. 21, 3892. doi: 10.3390/IJMS21113892
Kong H. H., Andersson B., Clavel T., Common J. E., Jackson S. A., Olson , et al. (2017). Performing skin microbiome research: A method to the madness. J Investig Dermatol. 137 (3), 561–568. doi: 10.1016/j.jid.2016.10.033
Kurosaki Y., Tsurumachi M., Kamata Y., Tominaga M., Suga Y., Takamori K. (2020). Effects of 308 nm excimer light treatment on the skin microbiome of atopic dermatitis patients. Photodermatol. Photoimmunol. Photomed. 36, 185–191. doi: 10.1111/PHPP.12531
Langan E. A., Recke A., Bokor-billmann T., Billmann F., Kahle B. K., Zillikens D. (2020). The role of the cutaneous microbiome in hidradenitis suppurativa—light at the end of the microbiological tunnel. Int. J. Mol. Sci. 21, 1–14. doi: 10.3390/ijms21041205
Lauber C. L., Zhou N., Gordon J. I., Knight R., Fierer N. (2010). Effect of storage conditions on the assessment of bacterial community structure in soil and human-associated samples. FEMS Microbiol. Lett. 307, 80–86. doi: 10.1111/J.1574-6968.2010.01965.X
Li M., Budding A. E., van-der-Lugt-Degen M., Du-Thumm L., Vandeven M., Fan A. (2019b). The influence of age, gender and race/ethnicity on the composition of the human axillary microbiome. Int. J. Cosmetic Sci. 41, 371–377. doi: 10.1111/ICS.12549
Li H., Wang Y., Yu Q., Feng T., Zhou R., Shao L., et al. (2019a). Elevation is associated with human skin microbiomes. Microorganisms 7, 1–23. doi: 10.3390/microorganisms7120611
Liang K., Leong C., Loh J. M., Chan N., Lim L., Lam Y. I., et al. (2022). A 3D-printed transepidermal microprojection array for human skin microbiome sampling. Proc. Natl. Acad. Sci. United States America 119, e2203556119. doi: 10.1073/PNAS.2203556119/SUPPL_FILE/PNAS.2203556119.SAPP.PDF
Liu Y., Wang S., Dai W., Liang Y., Shen C., Li Y., et al. (2020). Distinct skin microbiota imbalance and responses to clinical treatment in children with atopic dermatitis. Front. Cell. Infection Microbiol. 10, 336. doi: 10.3389/FCIMB.2020.00336/FULL
Loesche M. A., Farahi K., Capone K., Fakharzadeh S., Blauvelt A., Duffin K. C., et al. (2018). Longitudinal study of the psoriasis-associated skin microbiome during therapy with ustekinumab in a randomized phase 3b clinical trial. J. Invest. Dermatol. 138, 1973–1981. doi: 10.1016/j.jid.2018.03.1501
Maruyama S., Sano H., Wakui A., Kawachi M., Kaku N., Takahashi N., et al. (2022). Microbiota profiles on the surface of non-woven fabric masks after wearing. J. Oral. Biosci. 64, 376–379. doi: 10.1016/J.JOB.2022.07.002
Methé B. A., Nelson K. E., Pop M., Creasy H. H., Giglio M. G., Huttenhower C., et al. (2012). A framework for human microbiome research. Nature 486, 215–221. doi: 10.1038/nature11209
Mills J. G., Selway C. A., Thomas T., Weyrich L. S., Lowe A. J. (2022). Schoolyard biodiversity determines short-term recovery of disturbed skin microbiota in children. Microbial. Ecol. 1, 1. doi: 10.1007/S00248-022-02052-2
Moitinho-Silva L., Degenhardt F., Rodriguez E., Emmert H., Juzenas S., Möbus L., et al. (2022). Host genetic factors related to innate immunity, environmental sensing and cellular functions are associated with human skin microbiota. Nat. Commun. 13, 6204. doi: 10.1038/s41467-022-33906-5
Mougeot J.-L. C., Beckman M. F., Mougeot F. B., Horton J. M. (2022). Cutaneous microbiome profiles following chlorhexidine treatment in a 72-hour daily follow-up paired design: a pilot study. Microbiol. Spectr. 10 (3), e0175321. doi: 10.1128/SPECTRUM.01753-21
Naik H. B., Jo J. H., Paul M., Kong H. H. (2020). Skin microbiota perturbations are distinct and disease severity–dependent in hidradenitis suppurativa. J. Invest. Dermatol. 140, 922–925.e3. doi: 10.1016/j.jid.2019.08.445
Nathan M. K., Jasni A. S., Zakariah S. Z., Jamaluddin T. Z. M. T., Isa M. M., Ibrahim R. (2023). Hidden bugs in a newly opened hospital: the distribution of skin microbiota among healthcare workers in a newly opened teaching hospital. J. Med. Microbiol. 72 (7), 10.1099/jmm.0.001732. doi: 10.1099/jmm.0.001732
Oates A., Bowling F. L., Boulton A. J. M., McBaina A. J. (2012). Molecular and culture-based assessment of the microbial diversity of diabetic chronic foot wounds and contralateral skin sites. J. Clin. Microbiol. 50, 2263–2271. doi: 10.1128/JCM.06599-11/ASSET/C1DD55C7-1FCB-4FCC-99A8-070E053D5377/ASSETS/GRAPHIC/ZJM9990917830006.JPEG
Ogai K., Nagase S., Mukai K., Iuchi T., Mori Y., et al. (2018). A comparison of techniques for collecting skin microbiome samples: Swabbing versus tape-stripping. Front. Microbiol. 9, 2362. doi: 10.3389/FMICB.2018.02362/FULL
Oh J., Byrd A. L., Deming C., Conlan S., NISC Comparative Sequencing Program, Kong H. H., et al. (2014). Biogeography and individuality shape function in the human skin metagenome. Nature 514, 59–64. doi: 10.1038/nature13786
Peng M., Biswas D. (2020). Environmental influences of high-density agricultural animal operation on human forearm skin microflora. Microorganisms 8, 1–15. doi: 10.3390/MICROORGANISMS8101481
Pereira-Marques J., Hout A., Ferreira R. M., Weber M., Pinto-Ribeiro I., van Doorn L. J., et al. (2019). Impact of host DNA and sequencing depth on the taxonomic resolution of whole metagenome sequencing for microbiome analysis. Front. Microbiol. 10, 1277. doi: 10.3389/FMICB.2019.01277/BIBTEX
Potbhare R., RaviKumar A., Munukka E., Lahti L., Ashma R. (2022). Skin microbiota diversity among genetically unrelated individuals of Indian origin. PeerJ 10, e13075. doi: 10.7717/PEERJ.13075/SUPP-2
Proctor D. M., Dangana T., Sexton D. J., Fukuda C., Yelin R. D., Stanley M., et al. (2021). Integrated genomic, epidemiologic investigation of Candida auris skin colonization in a skilled nursing facility. Nat. Med. 27, 1401–1409. doi: 10.1038/s41591-021-01383-w
Redel H., Gao Z., Li H., Alekseyenko A. V., Zhou Y., Perez-Perez G. I., et al. (2013). Quantitation and composition of cutaneous microbiota in diabetic and nondiabetic men. J. Infect. Dis. 207, 1105. doi: 10.1093/INFDIS/JIT005
Ring H. C., Sigsgaard V., Thorsen J., Fuursted K., Fabricius S., Saunte D. M., et al. (2019). The microbiome of tunnels in hidradenitis suppurativa patients. J. Eur. Acad. Dermatol. Venereol . 33, 1775–1780. doi: 10.1111/JDV.15597
Riverain-Gillet É., Guet-Revillet H., Jais J. P., Ungeheuer M. N., Duchatelet S., Delage M., et al. (2020). The surface microbiome of clinically unaffected skinfolds in hidradenitis suppurativa: A cross-sectional culture-based and 16S rRNA gene amplicon sequencing study in 60 patients. J. Invest. Dermatol. 140, 1847–1855.e6. doi: 10.1016/j.jid.2020.02.046
Salava A., Pereira P., Aho V., Väkevä L., Paulin L., Auvinen P., et al. (2017). Skin microbiome in small- and large-plaque parapsoriasis. Acta Dermato-Venereologica 97, 685–691. doi: 10.2340/00015555-2631/
Sánchez-Pellicer P., Navarro-Moratalla L., Núñez-Delegido E., Agüera-Santos J., Navarro-López V. (2022). How our microbiome influences the pathogenesis of alopecia areata. Genes 13 (10), 1860. doi: 10.3390/GENES13101860
Schneider A. M., Nolan Z. T., Banerjee K., Paine A. R., Cong Z., Gettle S. L., et al. (2022). Evolution of the facial skin microbiome during puberty in normal and acne skin. J. Eur. Acad. Dermatol. Venereol. 37 (1), 166–175. doi: 10.1111/JDV.18616
Schowalter R. M., Pastrana D. V., Pumphrey K. A., Moyer A. L., Buck C. B. (2010). Merkel cell polyomavirus and two novel polyomaviruses are chronically shed from human skin. Cell Host Microbe 7, 509. doi: 10.1016/J.CHOM.2010.05.006
Selway C. A., Mills J. G., Weinstein P., Skelly C., Yadav S., Lowe A., et al. (2020). Transfer of environmental microbes to the skin and respiratory tract of humans after urban green space exposure. Environ. Int. 145, 106084. doi: 10.1016/J.ENVINT.2020.106084
Shi Y., Wang G., Lau H. C. H., Yu J. (2022). Metagenomic sequencing for microbial DNA in human samples: emerging technological advances. Int. J. Mol. Sci. 23 (4), 2181. doi: 10.3390/IJMS23042181
Staudinger T., Pipal A., Redl B. (2011). Molecular analysis of the prevalent microbiota of human male and female forehead skin compared to forearm skin and the influence of make-up. J. Appl. Microbiol. 110, 1381–1389. doi: 10.1111/J.1365-2672.2011.04991.X
Susic D., Davis G., O' Sullivan A. J., McGovern E., Harris K., Roberts L. M., et al. (2020). Protocol: Microbiome Understanding in Maternity Study (MUMS), an Australian prospective longitudinal cohort study of maternal and infant microbiota: study protocol. BMJ Open 10, e040189. doi: 10.1136/BMJOPEN-2020-040189
Tian Y., Gu C., Yan F., Gu Y., Feng Y., Chen J., et al. (2022). Alteration of skin microbiome in CKD patients is associated with pruritus and renal function. Front. Cell. Infection Microbiol. 12, 923581. doi: 10.3389/FCIMB.2022.923581/FULL
Verbanic S., Deacon J. M., Chen I. A. (2022). The chronic wound phageome: phage diversity and associations with wounds and healing outcomes. Microbiol. Spectr. 10 (3), e0277721. doi: 10.1128/SPECTRUM.02777-21/SUPPL_FILE/SPECTRUM.02777-21-S002.XLSX
Wetzels S. U., Strachan C. R., Conrady B., Wagner M., Burgener I. A., Virányi Z., et al. (2021). Wolves, dogs and humans in regular contact can mutually impact each other’s skin microbiota. Sci. Rep. 11, 17106. doi: 10.1038/S41598-021-96160-7
Woo Y. R., Lee S. H., Cho S. H., Lee J. D., Kim H. S. (2020). Characterization and analysis of the skin microbiota in rosacea: impact of systemic antibiotics. J. Clin. Med. 9, 185. doi: 10.3390/jcm9010185
Yu J. J., Manus M. B., Mueller O., Windsor S. C., Horvath J. E., Nunn C. L. (2018). Antibacterial soap use impacts skin microbial communities in rural Madagascar. PloS one 13 (8), e0199899. doi: 10.1371/journal.pone.0199899
Keywords: skin microbiome, microbiome, swab, skin sampling, 16S
Citation: Balacco DL, Bardhan A, Ibrahim H, Kuehne SA, Grant MM, Hirschfeld J, Heagerty AHM and Chapple IL (2025) Optimisation of cutaneous microbiota sampling methodology. Front. Microbiomes 4:1559981. doi: 10.3389/frmbi.2025.1559981
Received: 13 January 2025; Accepted: 17 March 2025;
Published: 22 April 2025.
Edited by:
Jesús Muñoz-Rojas, Meritorious Autonomous University of Puebla, MexicoReviewed by:
Georgina Hernandez-Montes, National Autonomous University of Mexico, MexicoGabriela Ibanez-Cervantes, Escuela Superior de Medicina (IPN), Mexico
Copyright © 2025 Balacco, Bardhan, Ibrahim, Kuehne, Grant, Hirschfeld, Heagerty and Chapple. This is an open-access article distributed under the terms of the Creative Commons Attribution License (CC BY). The use, distribution or reproduction in other forums is permitted, provided the original author(s) and the copyright owner(s) are credited and that the original publication in this journal is cited, in accordance with accepted academic practice. No use, distribution or reproduction is permitted which does not comply with these terms.
*Correspondence: Iain L. Chapple, aS5sLmMuY2hhcHBsZUBiaGFtLmFjLnVr