- 1Governor School of Science and Technology, Hampton, VA, United States
- 2NASA Langley Research Center, Hampton, VA, United States
A neural network nonlinear regression algorithm is developed for retrieving ocean surface wind speed from the Cloud-Aerosol Lidar and Infrared Pathfinder Satellite Observation (CALIPSO) lidar measurements. The neural network is trained with CALIPSO ocean surface and atmospheric backscatter measurements together with collocated Advanced Microwave Scanning Radiometer for EOS (AMSR-E) ocean surface wind speed. Ocean surface wind speeds are derived by applying the neural network algorithm to CALIPSO measurements between 2008 and 2020. CALIPSO wind speed measurements of 2015 are also compared with Advanced Microwave Scanning Radiometer 2 (AMSR-2) measurements on the Global Change Observation Mission–Water “Shizuku” (GCOM-W) satellite. Aerosol optical depths are then derived from CALIPSO’s ocean surface backscatter signal and theoretical ocean surface reflectance calculated from CALIPSO wind speed and Cox-Munk wind–surface slope variance relation. This CALIPSO wind speed retrieval technique is an improvement from our previous studies, as it can be applied to most clear skies with optical depths up to 1.5 without making assumptions about aerosol lidar ratio.
Introduction
Ocean surface capillary-gravity wave slopes follow Gaussian distribution. The variance of the Gaussian distribution increases linearly with the atmospheric wind speed 10 m above the ocean surface (Cox and Munk, 1954). Visible and near-infrared light scattered by the ocean surface with the wind-driven capillary-gravity waves follows Snell’s law. With a light source shining down perpendicular to the ocean surface, light reflected by the ocean surface back toward the light source reduces when wind speed increases because the probability of the ocean surface to be flat reduces when the variance of Gaussian distribution increases.
Spacebased lidar measurements, such as CALIPSO measurements, can be used for estimating the variance of each laser shot (around 70 m diameter at ocean surface) when aerosol loading is very low and uncertainty in atmospheric attenuation is relatively low (Hu et al., 2008). The error of wind speed estimates from CALIPSO increases with aerosol optical depths. It is possible to derive aerosol optical depth accurately using CALIPSO ocean surface backscatter measurements with collocated wind speed derived from collocated radar (Josset et al., 2008) and microwave radiometer measurements (e.g., AMSR-E and AMSR-2) (He et al., 2016; Tang et al., 2018). Due to uncertainty in aerosol lidar ratios, which is the ratio of extinction and backscatter cross sections, previous studies either use CALIPSO measurements to derive wind speed while assuming aerosol optical depth, or derive aerosol optical depth while assuming wind speed. CALIPSO ocean surface backscatter properties and wind speed measurements are also used for calibrations of lidar measurements of phytoplankton (Behrenfeld et al., 2013; Lu et al., 2020). Unfortunately, there are times when microwave wind speed measurements are unavailable.
Assuming marine aerosol lidar ratios of similar seasons and similar locations are similar for different years, it is possible that we can develop aerosol lidar ratio statistics from a year when there are collocated wind speed measurements. This study intends to establish an innovative method of deriving ocean surface wind speed and aerosol optical depth simultaneously using one year of CALIPSO and AMSR-E data, and apply the algorithm to derive both wind speed and aerosol optical depth in other times when wind speed is not available.
Turbulence plays an important role in the exchange of momentum, energy, and greenhouse gases between the atmosphere and ocean. High spatial resolution ocean surface atmospheric wind speed derived from this study will help to improve our understanding of vertical turbulence exchange and air-sea interaction.
Data
Lidar data from Version 4 CALIPSO level 1 data (Vaughan et al., 2019), together with collocated ocean surface wind speed data from AMSR-E measurements are used in this study. The CALIPSO data includes.
Total (parallel + perpendicular polarization) 532 nm ocean surface and subsurface integrated attenuated lidar backscatter;
Perpendicularly polarized 532 nm ocean surface and subsurface integrated attenuated lidar backscatter;
1,064 nm ocean surface and subsurface integrated attenuated lidar backscatter;
Total (parallel + perpendicular polarization) 532 nm column integrated atmospheric integrated attenuated lidar backscatter;
Perpendicular polarized 532 nm column integrated atmospheric integrated attenuated lidar backscatter;
1,064 nm column integrated atmospheric integrated attenuated lidar backscatter.
Due to low pass filter and detector after-pulsing, CALIPSO’s ocean surface backscatter spreads into three vertical range bins plus a long tail (Hu et al., 2008). Thus it is difficult to separate backscatter from ocean surface and subsurface. Thus the ocean surface and subsurface backscatter include the three surface range bins and two extra range bins below them. As ocean surface backscatter does not depolarize, the 532 nm perpendicularly polarized backscatter are contributed by the ocean subsurface theoretically. Water absorbs strongly at 1,064 nm (absorption coefficient about 50 m−1), ocean subsurface contributes very little to the 1,064 nm ocean surface and subsurface backscatter.
The column integrated atmospheric backscatter parameters include summations of all the atmospheric lidar backscatter signals between ocean 0 and 40 km above ocean surface.
The Physics Behind the Lidar Measurements of Ocean Surface Wind Speed, Neural Network Wind Speed Retrieval Algorithm, and Data Analysis
If there is nothing between a smooth ocean surface and the lidar pointing vertically to the surface, a fraction (about 2%) of the laser light will be reflected (R) by the ocean surface and the rest refracted into the water, following the Fresnel formula:
Here n is the refractive index of water (around 1.335 and 1.325 at 532 and 1,064 nm, respectively).
The ocean surface becomes rough due to wind-driven capillary-gravity waves, with wavelength around 1 cm. Ocean surface roughness increases with wind speed. For a wind-less ocean surface, the reflected light is highly focused at the backscatter angle and thus most reflected photons will be received by the lidar. Fewer photons will reach the lidar receiver when the ocean surface becomes rough since reflected light is less focused and some of the reflected light goes to other directions too. Higher wind leads to rougher surface and fewer photons received by the lidar. The lidar backscatter signal is proportional to the probability that the surface of the capillary-gravity waves is perpendicular to the line-of-sight of the laser beam. It relatively is insensitive to waves with longer wave lengths, such as swells. This theoretical ocean surface reflectance follows a Gaussian distribution (Cox and Munk, 1954):
Here V is the ocean surface wind speed. Thus, lidar backscatter signal is inversely proportional to ocean surface wind. For low wind speed (V < 7 m/s), the formula was revised (Hu et al., 2008) based on the collocated CALIPSO/AMSR-E measurements. The exponential term for nadir pointing laser (θ = 0) is 1. Recent studies (e.g., He et al., 2016; Tang et al., 2018) use the Gram--Charlier ocean surface reflectance model to relate wind-driven wave slope variances to sea surface wind speeds. As the lidar is pointed 3° off-nadir since 2008, the measurements are relatively insensitive to changes in wind directions.
To estimate ocean surface wind speed from CALIPSO lidar measurements, it is important to 1) have good calibration of the lidar measurements; 2) estimate the reduction of ocean surface lidar backscatter signal due to light attenuation (scattering and absorption) by the atmosphere. Backscatter profiles in CALIPSO’s Version 4 level 1 data are highly accurately calibrated. Atmospheric two-way transmittance can be estimated from CALIPSO’s atmospheric backscatter profiles, but it will require an assumption of the so-called lidar ratio of particulates in the atmosphere, which links atmospheric backscatter to attenuation (Hu et al., 2008). Assuming aerosol optical depth of 0.2. A 10% error in lidar ratio can cause an aerosol optical depth uncertainty of 0.02 or higher. That will result in a 5% uncertainty in the estimates of ocean surface backscatter estimates and 0.5 m/s wind speed uncertainty.
The neural network ocean surface wind speed retrieval method is an improvement of the earlier study that retrieved ocean surface wind from CALIPSO lidar measurements (Hu et al., 2008). The nonlinear neural network algorithm automatically adjusts aerosol lidar ratios that minimize wind speed retrieval errors using the ocean surface and atmospheric signals. Thus the neural network algorithm does not require assuming a predetermined lidar ratios (Hu et al., 2008) to retrieval wind speed. The neural network algorithm only requires that a relationship between wind speed and wave slope exists and it does not make an assumption about the exact relationship between wind speed and wave slope either.
The MATLAB functional fitting neural network, fitnet, is adopted for this study. The input variables of the neural network are the following CALIPSO measurements.
Total (parallel + perpendicular polarization) 532 nm ocean surface and subsurface lidar backscatter;
Perpendicularly polarized 532 nm ocean surface and subsurface lidar backscatter;
1,064 nm ocean surface and subsurface lidar backscatter;
Total (parallel + perpendicular polarization) 532 nm column integrated atmospheric lidar backscatter;
Perpendicular polarized 532 nm column integrated atmospheric lidar backscatter;
1,064 nm column integrated atmospheric lidar backscatter; and
Latitude.
Wind speed is inversely proportional to the ocean surface backscatter after correcting for atmospheric attenuations. If atmospheric attenuation can be properly corrected, wind speed can be derived from either 532 nm or 1,064 nm ocean surface backscatter. Perpendicular backscatter (#2 of the neural network input parameters listed above) carries information for removing ocean subsurface backscatter from total backscatter (#1 and #3 in the input parameter list). The ratio of 532 and 1,064 nm ocean surface backscatter (#1 and #3 in the list) carries information for fine mode aerosol optical depths. Atmospheric backscatter measurements (#4, 5, and 6 of the input parameter list) carry aerosol information. The neural network algorithm automatically adjusts lidar ratios to reduce errors in ocean surface wind speed. The algorithm selected lidar ratios change with the atmospheric backscatter (#4, 4, 6) and latitude (#7) as well as wind speed themselves.
The output variable is collocated ocean surface wind speed from AMSR-E microwave radiometer measurements. The input CALIPSO variables are averaged 40 km along-track in order to match the AMSR-E footprint size.
One limiting factor of space-based lidar measurements of ocean surface wind speed is the presence of thick clouds and aerosols. Although CALIPSO has a highly sensitive detector, accurate wind speed measurements still require more than a thousand photons to reach the telescope, in order to have a SNR of 10 and thus wind speed uncertainty of 1 m/s (Hu et al., 2008). Each CALIPSO 532 nm laser pulse has 0.11 J of laser energy (about 3 × 1017 photons). Ocean surface reflectance is roughly 0.04 sr−1 (for a 6 m/s wind speed). As the CALIPSO satellite is 705 km above the ocean surface, its 1-m diameter lidar telescope intercepts 1.6 × 10–12 sr solid angle of backscatter from ocean surface. If there are no atmospheric attenuations, there are about 20,000 photons entering the receiver. To have a thousand or more photons reaching the telescope for accurate wind speed retrievals without horizontal averaging, the maximum optical depths of the clouds and aerosols in the atmosphere should be less than 1.5 (two-way transmittance, e−2×1.5, around 0.05). This study only includes relatively “clear sky” condition with integrated attenuated backscatter of the ocean surface above 0.002 sr−1.
CALIPSO lidar backscatter measurements of January 2008 and collocated ocean surface wind speed measurements from AMSR-E instruments on Aqua satellite (75 s ahead of CALIPSO) are used for training the neural network CALIPSO ocean surface wind speed algorithms. To test the algorithm, the neural network algorithm is applied to CALIPSO measurements for the entirety of 2008 to retrieve ocean surface wind speed. The left panel of Figure 1 shows that the retrieval results are unbiased, with an average wind speed difference between CALIPSO and AMSR-E around 0.001 m/s. The standard deviation of the wind speed difference between CALIPSO and AMSR-E, which is considered as instantaneous error, is around 1.05 m/s (left panel of Figure 1). The retrieved CALIPSO wind speeds agree with AMSR-E for both low and high wind speeds (right panel of Figure 1).
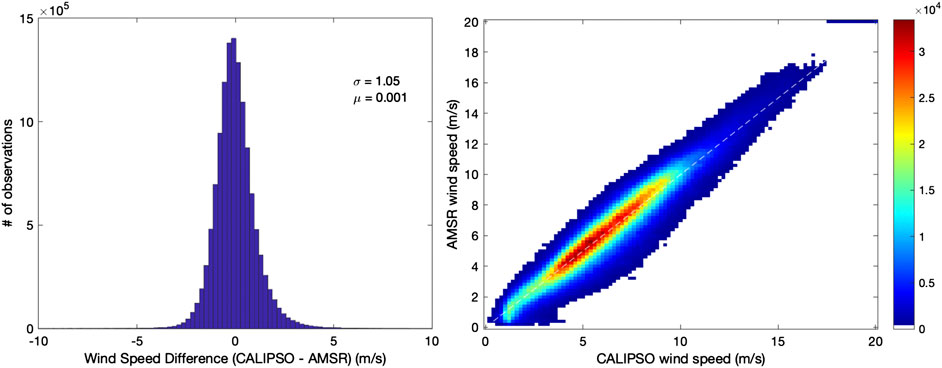
FIGURE 1. Left panel: histogram of wind speed difference between CALIPSO wind speed and AMSR wind speed; Right panel: number of observations (color) of collocated CALIPSO and MODIS wind speed.
The neural network algorithm is applied to CALIPSO measurements between 2008 and 2020, when the lidar is tilted 3° off-nadir to derive ocean surface wind speeds at 1 km resolution. While the ocean surface backscatter is at single shot resolution, input parameters of integrated attenuated backscatter of the atmosphere is averaged to 5 km along track. The SNRs for both the surface and the atmosphere are around 60.
Figure 2 shows that the spatial distributions of the annual mean CALIPSO wind speeds (upper left panel) agree with wind speed measured by AMSR-2 instrument (upper right panel of Figure 2) onboard the GCOM-W satellite, which is a few minutes ahead of Aqua satellite. CALIPSO over-estimates wind speed at coastal waters by as much as 1 m/s, especially in coastal regions with smoke and dust aerosols, heavy pollutions and the Southern Oceans near Antarctica (lower panel of Figure 2). It is possibly due to 1) the errors associated with CALIPSO’s atmospheric two-way transmittance estimates; 2) biases in AMSR-E wind speed in coastal regions. But it is more likely due to 2) and less likely due to (1). In these coastal regions with dusts and heavy pollution, aerosols lidar ratios are significantly higher than the ones in open ocean and the neural network algorithm will most-likely under-estimate the lidar ratios in these coastal regions. Under-estimates of lidar ratio can cause under-estimates of atmospheric two-way transmittance and over-estimates of the ocean surface reflectance, which will result in under-estimates of CALIPSO wind speed. The data indicate the upside of that as CALIPSO wind speed is larger. Reanalysis suggests that microwave measurements of wind speed in the Southern Oceans and coastal regions are lower than the ones from models (Zhang et al., 2018). As spatial variability of sea surface temperature is high in these regions and wind speed from AMSR-2 is sensitive to that, it is likely that AMSR-2 under-estimated wind speed in these regions.
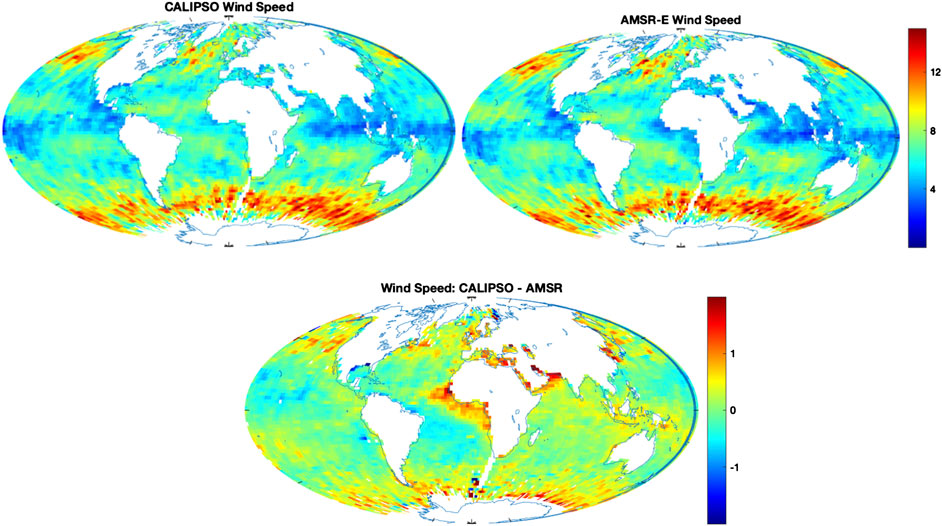
FIGURE 2. Comparisons of annual mean 2015 CALIPSO ocean surface wind speed (upper left panel) and wind speed from collocated AMSR-2 measurements *upper right panel). Lower panel: difference between CALIPSO and AMSR-2 wind speed
The seasonal statistics of the wind speeds between CALIPSO (left panels of Figure 3) also agree with AMSR-2 (right panels of Figure 3). The upper panels of Figure 3 are measurements of spring months (March, April and May) of 2014, Lower panels are fall (September, October and November) measurements.
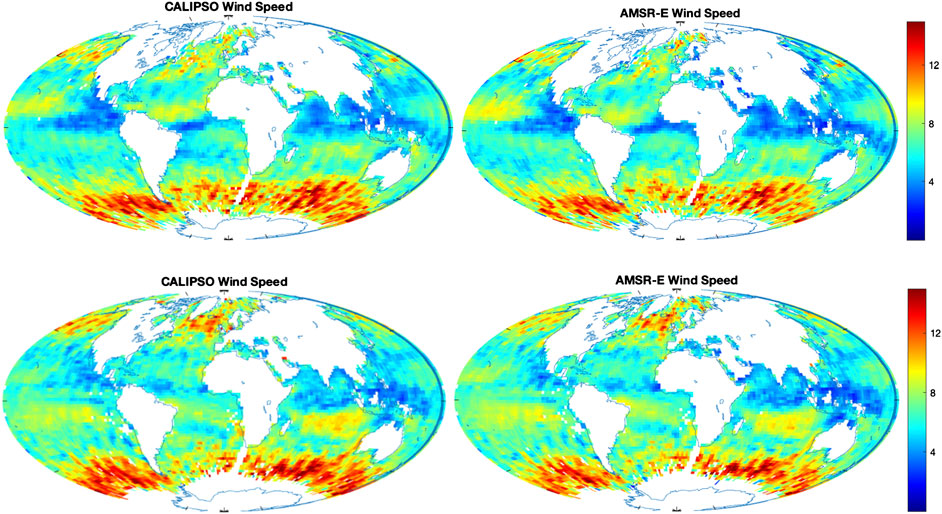
FIGURE 3. Seasonal mean (upper panel: March/April/May 2014; lower panel: September/October/November 2014) ocean surface wind speed comparisons between CALIPSO (left panels) and AMSR-2.
After the wind speed is derived from the neural network algorithm, it is straight forward to derive the aerosol optical depths (AOD), τ,
Here P(V) is the theoretical ocean surface reflectance, βsurface is CALIPSO 532 nm ocean surface integrated attenuated backscatter signal. Ocean subsurface signal is subtracted similar to Hu et al. (2008). τair is the optical depth of the 532 nm molecular scatter. And τozone is absorption optical depth of ozone.
Figure 4 shows the spatial distributions of CALIPSO aerosol optical depths of 2015 (left panel) and MODIS aerosol optical depths (right panel). The MODIS aerosol optical depth is from the MODIS Collection 6.1 monthly mean data product MOD08_M3.006 (Platnick et al., 2015). As the CALIPSO algorithm is applied to thinner aerosols (ocean surface attenuated backscatter greater than 0.002 sr−1), CALIPSO’s measurements of dust optical depths in the tropical Atlantic region are much lower than MODIS.
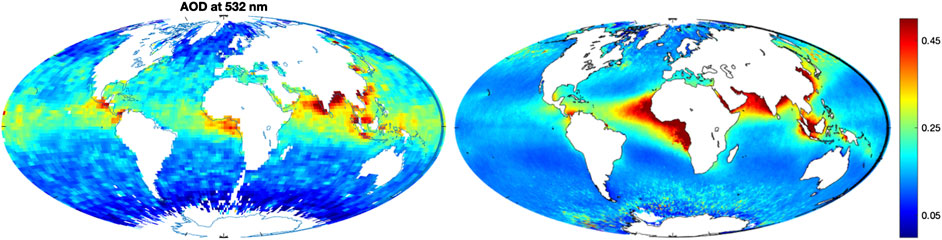
FIGURE 4. Comparison between aerosol optical depths (AOD) of year 2015 derived from CALIPSO ocean surface backscatter and wind speed (left panel) and AOD measured from MODIS instrument on Aqua satellite (right panel). Thick dusts are not considered in the CALIPSO measurements when ocean surface signal is too weak.
Figure 5 shows seasonal statistics (Left panel: March, April and May of 2008; Right panel: September, October and November of 2008) of the aerosol optical depths derived from CALIPSO ocean surface backscatter and CALIPSO wind speed from the neural network retrieval.
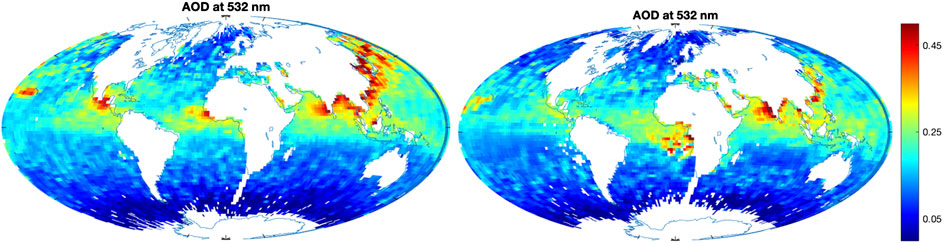
FIGURE 5. Aerosol optical depth of spring (March/April/May) 2008 (Left panel) and fall (September/October/November) 2008.
Summary
An innovative neural network retrieval algorithm is developed for retrieving ocean surface wind speed from CALIPSO lidar measurements. The neural network is trained with CALIPSO ocean surface and atmospheric backscatter measurements together with collocated AMSR-E ocean surface wind speed.
The training set includes collocated CALIPSO and AMSR-E measurements of January 2008. The algorithm is tested by applying the algorithm to all CALIPSO measurements during 2008 and comparing the 2008 CALIPSO wind speed with collocated AMSR-E measurements. The comparisons show that the CALIPSO wind speed is unbiased and the instantaneous errors (comparing with AMSR-E) is 1.05 m/s.
Ocean surface wind speeds are derived by applying the neural network algorithm to CALIPSO measurements between 2008 and 2020, when the lidar is tilted 3° off nadir. CALIPSO wind speed measurements of 2015 also compare with AMSR-2 measurements on GCOM-W satellite.
Aerosol optical depths are then derived from CALIPSO’s ocean surface backscatter signal and theoretical ocean surface reflectance calculated from CALIPSO wind speed and Cox-Munk wind–surface slope variance relation.
Previous studies (e.g., Hu et al., 2008; He et al., 2016; Tang et al., 2018) require knowing one of the two properties (wind speed, aerosol optical depth) to derive the other from CALIPSO. This innovative approach retrieves both wind speed and aerosol optical depth CALIPSO.
The neural network CALIPSO wind speed retrieval technique is an improvement from Hu et al. (2008), as it can be applied to most clear skies with optical depths up to 1.5 without making assumptions about aerosol lidar ratio.
The wind speed and aerosol optical depth data will be available to the community upon request.
Data Availability Statement
The original contributions presented in the study are included in the article/Supplementary Material, further inquiries can be directed to the corresponding author.
Author Contributions
AM lead this study, including data collection, algorithm development and data analysis, under the guidance of YH.
Funding
This study is supported by NASA’s summer intern program. CALIPSO data is provided by the CALIPSO project of NASA.
Conflict of Interest
The authors declare that the research was conducted in the absence of any commercial or financial relationships that could be construed as a potential conflict of interest.
References
Behrenfeld, M. J., Hu, Y., Hostetler, C. A., Dall'Olmo, G., Rodier, S. D., Hair, J. W., et al. (2013). Space-based lidar measurements of global ocean carbon stocks. Geophys. Res. Lett. 40, 4355–4360. doi:10.1002/grl.50816
Cox, C., and Munk, W. (1954). Measurement of the roughness of the sea surface from photographs of the Sun's glitter. J. Opt. Soc. Am. 44 (11), 838–850. doi:10.1364/josa.44.000838
He, M., Hu, Y., Huang, J. P., and Stamnes, K. (2016). Aerosol optical depth under “clear” sky conditions derived from sea surface reflection of lidar signals. Optic Express 24, A1618–A1634. doi:10.1364/OE.24.0A1618
Hu, Y., Stamnes, K., Vaughan, M., Pelon, J., Weimer, C., Wu, D., et al. (2008). Sea surface wind speed estimation from space-based lidar measurements. Atmos. Chem. Phys. 8 (13), 3593–3601. doi:10.5194/acp‐8‐3593‐2008
Josset, D., Pelon, J., Protat, A., and Flamant, C. (2008). New approach to determine aerosol optical depth from combined CALIPSO and CloudSat ocean surface echoes. Geophys. Res. Lett. 35, L10805. doi:10.1029/2008GL033442
Platnick, S., King, M., and Hubanks, P. (2015). MODIS atmosphere L3 monthly product. NASA MODIS adaptive processing system, NASA technical document, Goddard space flight center, USA. Available at: http://dx.doi.org/10.5067/MODIS/MOD08_M3.006
Stamnes, X., Hu, Y., Yang, Y., Bontempi, P., Omar, A., and Baize, R. (2020). Antarctic spring ice-edge blooms observed from space by ICESat-2. Rem. Sens. Environ. 245, 111827. doi:10.1016/j.rse.2020.111827
Tang, Q., Hu, Y., Li, W., Huang, J., and Stamnes, K. (2018). Optimizing cirrus optical depth retrievals over the ocean from collocated CALIPSO and AMSR-E observations. Appl. Optic. 57, 7472–7481. doi:10.1364/AO.57.007472
Vaughan, M., Garnier, A., Josset, D., Avery, M., Lee, K.-P., Liu, Z., et al. (2019). CALIPSO lidar calibration at 1064 nm: version 4 algorithm. Atmos. Meas. Tech. 12, 51–82. doi:10.5194/amt-12-51-2019
Keywords: Wind speed, aerosol optical depth, CALIPSO, Lidar, ocean surface, AMSR‐E
Citation: Murphy A and Hu Y (2021) Retrieving Aerosol Optical Depth and High Spatial Resolution Ocean Surface Wind Speed From CALIPSO: A Neural Network Approach. Front. Remote Sens. 1:614029. doi: 10.3389/frsen.2020.614029
Received: 05 October 2020; Accepted: 09 November 2020;
Published: 22 February 2021.
Edited by:
Feng Xu, University of Oklahoma, United StatesReviewed by:
Dong Wu, Ocean University of China, ChinaZhao-Cheng Zeng, University of California, Los Angeles, United States
Copyright © 2021 Murphy and Hu. This is an open-access article distributed under the terms of the Creative Commons Attribution License (CC BY). The use, distribution or reproduction in other forums is permitted, provided the original author(s) and the copyright owner(s) are credited and that the original publication in this journal is cited, in accordance with accepted academic practice. No use, distribution or reproduction is permitted which does not comply with these terms.
*Correspondence: Yongxiang Hu, eW9uZ3hpYW5nLmh1LTFAbmFzYS5nb3Y=