- 1Institute of Environmental Physics (IUP), Heidelberg University, Heidelberg, Germany
- 2Heidelberg Center for the Environment (HCE), Heidelberg University, Heidelberg, Germany
- 3Interdisciplinary Center for Scientific Computing (IWR), Heidelberg University, Heidelberg, Germany
- 4National Institute for Environmental Studies (NIES), Tsukuba, Japan
- 5European Centre for Medium-Range Weather Forecasts (ECMWF), Reading, United Kingdom
- 6Institute for Meteorology and Climate Research (IMK-ASF), Karlsruhe Institute of Technology, Leopoldshafen, Germany
- 7Netherlands Institute for Space Research (SRON), Utrecht, Germany
Satellite measurements of the atmospheric concentrations of carbon dioxide (CO2), methane (CH4) and carbon monoxide (CO) require careful validation. In particular for the greenhouse gases CO2 and CH4, concentration gradients are minute challenging the ultimate goal to quantify and monitor anthropogenic emissions and natural surface-atmosphere fluxes. The upcoming European Copernicus Carbon Monitoring mission (CO2M) will focus on anthropogenic CO2 emissions, but it will also be able to measure CH4. There are other missions such as the Sentinel-5 Precursor and the Sentinel-5 series that target CO which helps attribute the CO2 and CH4 variations to specific processes. Here, we review the capabilities and use cases of a mobile ground-based sun-viewing spectrometer of the type EM27/SUN. We showcase the performance of the mobile system for measuring the column-average dry-air mole fractions of CO2 (XCO2), CH4 (XCH4) and CO (XCO) during a recent deployment (Feb./Mar. 2021) in the vicinity of Japan on research vessel Mirai which adds to our previous campaigns on ships and road vehicles. The mobile EM27/SUN has the potential to contribute to the validation of 1) continental-scale background gradients along major ship routes on the open ocean, 2) regional-scale gradients due to continental outflow across the coast line, 3) urban or other localized emissions as mobile part of a regional network and 4) emissions from point sources. Thus, operationalizing the mobile EM27/SUN along these use cases can be a valuable asset to the validation activities for CO2M, in particular, and for various upcoming satellite missions in general.
1 Introduction
Various current and upcoming satellite sensors aim at measuring the atmospheric abundances of the carbon compounds carbon dioxide (CO2), methane (CH4) and carbon monoxide (CO). Most of the space-borne instruments such as the GOSAT series (Greenhouse Gas Observing Satellite) (Kuze et al., 2009; Suto et al., 2021), the OCO series (Orbiting Carbon Observatory) (Eldering et al., 2017), the S5P (Sentinel-5 Precursor) (Hu et al., 2018) and S5 series (Sentinel-5), the TanSat program (Yang et al., 2021) and others rely on spectrometric measurements of sunlight reflected by the Earth in the shortwave-infrared (SWIR) spectral range which lends sensitivity to the column-average dry-air mole fractions of the gases (commonly denoted by XCO2, XCH4, and XCO). In particular, the upcoming European Copernicus Carbon Monitoring mission (CO2M) (Kuhlmann et al., 2019; Sierk et al., 2019) and various missions that focus on localized targets and specific emission sectors (Strandgren et al., 2020; Jervis et al., 2021) will rely on the same measurement principle.
Measuring the most abundant atmospheric carbon compounds, the general goal of these missions is to contribute to a better understanding of the Earth’s contemporary carbon cycle. In terms of the required precision and accuracy, there are two main categories: Category 1 aims at constraining the natural variability of the carbon cycle on regional-to-continental spatial and on monthly-to-yearly temporal scales [e.g. (Guerlet et al., 2013; Liu et al., 2017; Crowell et al., 2019; Palmer et al., 2019; Western et al., 2021)]. Typically, these variabilities are driven by the world’s large ecosystems through variability in photosynthetic and respiratory activity, wetland dynamics, fire occurrences and other carbon cycle mechanisms. Category 2 targets at budgeting anthropogenic emissions on regional-to-local and monthly-to-instantaneous scales, where the instantaneous scale relates to the usage of individual satellite overpasses [e.g. (Hakkarainen et al., 2016; Nassar et al., 2017; Borsdorff et al., 2018; Pandey et al., 2019; Varon et al., 2019)]. Due to the larger spatial and temporal perspective, category 1 requires that satellite measurements do not show any spurious gradients or trends on the targeted larger scales. Category 2, in contrast, targets at the local-to-regional excess of the compound concentrations above the adjacent background and on direct instantaneous emission rate estimates. Thus, category 2 requires eliminating residual gradients on the local-to-regional scale e.g., within the satellite’s swath and it requires high single-shot precision since individual soundings are to be used.
In order to meet these accuracy and precision requirements – for XCO2 and XCH4 on the order of a few permille, for XCO on the order of a few percent – the satellite measurements need careful validation by ground-based instruments that are able to measure XCO2, XCH4, and XCO with similar column-average sensitivity as the satellite spectrometers. Currently, the TCCON (Total Carbon Column Observing Network) (Wunch et al., 2011) and COCCON (Collaborative Carbon Column Observing Network) (Frey et al., 2019) form the backbone of routine validation of the satellite-derived carbon compound concentrations. These networks consist of a few dozens of ground-based Fourier Transform Spectrometer (FTS) stations that are equipped with a solar tracker enabling direct-sun absorption spectroscopy. The networks’ coverage mostly serves the category 1 applications since most of these FTS are distributed throughout the world’s continents. There is a gap of validation capacities for direct verification of local-to-regional scale gradients and the respective emission estimates (category 2) and for measurements over the oceans (part of category 1). Addressing the former gap, urban networks and episodic coordinated deployments of the portable COCCON spectrometers are emerging [e.g. (Hase et al., 2015; Luther et al., 2019; Dietrich et al., 2021)] but are far from covering a representative number of cities and hotspot regions. Validation over the oceans is currently mostly limited to stations on islands or at coastal sites which implies that the satellite coincidences are not direct and that differences in topographic height often need to be carefully accounted for. Validation over the oceans should be warranted since typically the satellites observe ocean scenes under glint-view i.e., deliberately pointing towards the specular reflection at the ocean surface in order to gain sufficient signal while, over land, nadir-viewing is preferred. Basu et al. (Basu et al., 2013) reported on land-ocean biases with detrimental impacts for flux inversions that were on the order of a few tenths of a ppm for XCO2. Such land-ocean biases might be due to scattering by atmospheric particles affecting the lightpath quite differently above ocean and land (Butz et al., 2013).
Here, we review and refine plans how portable FTS of the COCCON type, further developed for mobile deployments, can be valuable assets to the existing XCO2, XCH4 and XCO validation capacities, in particular contributing directly to validating local-to-regional scale gradients and measurements over the oceans. The key innovation for making the off-the-shelf FTS suitable for deployment on mobile platforms is a solar tracker system that 1) can find the position of the Sun independent of knowledge on the instrument’s location and orientation and that, once the Sun is captured, 2) is sufficiently agile to track the Sun under (potentially fast) platform motion. Another requirement for routine and autonomous operations is a weather-proof housing. We have developed such a system and demonstrated its performance for continuous operations on ships and for stop-and-go operations on small trucks (Klappenbach et al., 2015; Butz et al., 2017; Luther et al., 2019; Knapp et al., 2021). Section 2 reviews previous campaign deployments, Section 3 summarizes the key instrument parameters and reports on the processing recipe. In Section 4, we focus our discussion on a ship deployment in March 2021 in the Western North Pacific on the research vessel (RV) Mirai which we have not reported before and which serves as an illustrative assessment of the instrument’s capabilities. Finally, Section 5 relates to potential use cases in the context of validating future satellite missions such as CO2M.
2 Field Deployments
In the past years, our mobile FTS has been deployed during five large scale field campaigns listed in Table 1. Three deployments were on ships demonstrating the performance for category 1 applications i.e., for validating the large scale constituent gradients over the oceans. Two deployments were on road vehicles in the vicinity of local emission hotspots evaluating the usefulness for category 2 applications focusing on local-scale concentration gradients and emission rates of localized sources.
Since the first deployment in spring 2014 on RV Polarstern, the setup has substantially matured in terms of solar tracker performance and its ability to be remotely operated and to resist harsh weather. Klappenbach et al. (Klappenbach et al., 2015) and Knapp et al. (Knapp et al., 2021) report on ship deployments in the Atlantic and Pacific oceans which aimed at measuring meridional and zonal cross sections of background XCO2, XCH4 and, for the latest instrument upgrade (Hase et al., 2016), XCO over the large oceans. Beside validating the satellite records from GOSAT, OCO-2, and S5P, our concentration records were also used for validating and improving a model used in the Copernicus Atmosphere Monitoring Service (CAMS) (Agusti-Panareda et al., 2017; Knapp et al., 2021). The latest ship deployment took place in the Western Pacific and along the coast of Japan from February 13 to March 24, 2021 when the mobile EM27/SUN was operated onboard the Japanese RV Mirai (framed by a collaboration of Heidelberg University and the National Institute for Environmental Studies (NIES) of Japan). Figure 1 shows the trajectory of RV Mirai and denotes the locations where weather conditions were sufficiently fair to conduct direct-sun measurements. The first part of the cruise, starting out from Shimizu port heading north-east towards the Kamtschatka peninsula, suffered from rough seas with average wave heights of up to 10 m challenging equipment and operating personnel. Weather conditions were better for the second part in the south-east and south of Japan. Therefore, the discussion here concentrates on the latter part with a particular focus on the last 3 days where we collected measurements along the southern coast of Japan observing the outflow from the island before entering Shimizu port.
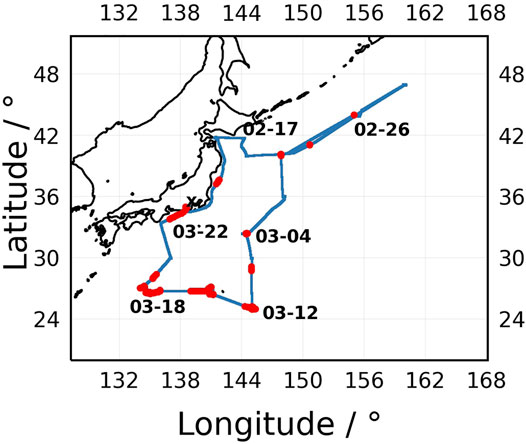
FIGURE 1. Trajectory of RV Mirai through the Western Pacific in the vicinity of Japan leaving Shimizu port towards the East. Red dots indicate valid measurement positions of the mobile EM27/SUN. For selected samples, the measurement dates are indicated.
On land, Butz et al. (Butz et al., 2017) and Luther et al. (Luther et al., 2019) measured emission plumes of CO2 emitted by the Mt. Etna volcano and plumes of CH4 from coal mine ventilation in the Upper Silesian Coal Basin (USCB), Poland, respectively. To this end, the mobile EM27/SUN was mounted on a van operated in stop-and-go patterns underneath the emission plumes of the local sources. The instrument collected cross sections of the column enhancements inside the plumes with respect to the background. Luther et al. (Luther et al., 2019) show the van setup and typical plume enhancements for XCH4 observed downwind of coal mine ventilation shafts. Together with estimates of the local wind conditions and a mass balance method, we succeeded in estimating the instantaneous CH4 emission rates from individual ventilation facilities.
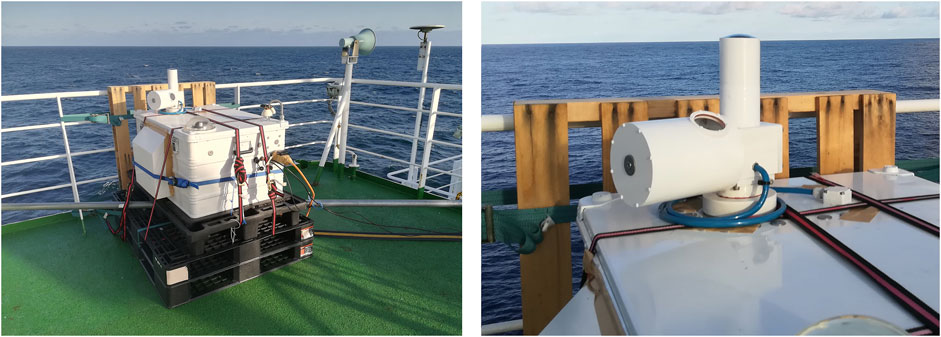
FIGURE 2. Photographs of the mobile EM27/SUN onboard RV Mirai (left) with a zoom of the outer part of the solar tracker (right) showing the lens mount of the fish-eye camera (vertical tube) and the entrance window for the solar beam.
3 Instrument Setup and Data Processing
3.1 Mobile EM27/SUN and Its Solar Tracker
The key equipment is the EM27/SUN FTS available for purchase from Bruker Optics. It covers the spectral ranges 5,500–11,000 cm−1 and 4,000–5,500 cm−1 at a spectral resolution of 0.5 cm−1 via two InGaAs detectors (Gisi et al., 2012). The custom-built solar tracker collects sunlight and feeds it into the FTS via an alt-azimuth mirror assembly. A weather-proof housing hosts the FTS and solar tracker as well as various ancillary equipment such as the computers, ventilation units, and sensors for housekeeping data. Knapp et al. (Knapp et al., 2021) describes the general setup in detail. Here, we focus on the solar tracker which is the unit enabling mobile applications and which has seen substantial improvements over the past years starting from the initial developments (Gisi et al., 2011; Klappenbach et al., 2015; Butz et al., 2017). The EM27/SUN FTS itself can be operated nominally as e.g. recommended by the COCCON protocol (Frey et al., 2019) with the exception that we recommend collecting individual interferograms (instead of automatic 10-fold co-adding) and sampling them at 20 kHz (instead of 10 kHz). Exposures under unstable solar tracking need to be screened in the processing chain and, since solar tracking is less stable under mobile than under stationary conditions, automatic co-adding and slow sampling could imply needlessly loosing data. Further, it is advised to monitor the instrument line shape (ILS) of the FTS on a regular basis. To this end, the ambient H2O absorption is measured through open-path measurements using a halogen lamp positioned at a few meters distance from the instrument (Frey et al., 2015). Overall, we believe that the configuration reported here is the one that is most suitable for operationalizing the instrument for routine, unattended deployments on carriers such as cargo ships.
The hardware setup of the solar tracker consists of a coarse-tracking camera, a fine-tracking camera and 2 mirrors assembled in alt-azimuth configuration. Figure 2 shows the outside part of the solar tracker with its protective housing. The 2-mirror assembly is very similar to the one described by Gisi et al. (Gisi et al., 2011) and is delivered with the EM27/SUN upon purchase. It uses two elliptic aluminium mirrors (2 inch aperture at 45°) mounted on two perpendicular rotation stages for the azimuth and elevation directions. The tracking software drives the mirror assembly towards the center of the Sun to feed a parallel beam of sunlight into the FTS.
The input for the driver software comes from the fine-tracking camera that consists of a 25 mm objective lens (2.33°x 1.95°field of view) mounted on a digital camera (IDS, 1.2 Megapixel) read out at a frequency of 60 (up to 125) frames-per-second. The fine-tracking camera collects images of the field stop in front of the shortwave detector where an image of the Sun occurs under nominal conditions. The driver software fits an ellipse to the image and a feedback loop drives the rotation stages such that the center of the ellipse coincides with center of the field stop. On a moving platform, the feedback loop needs to be sufficiently fast to compensate for platform motion and thus, it is essential to use a fast fine-tracking camera.
The coarse-tracking camera module consists of an F-theta fish-eye lens (Fujinon, 185°field of view) and a short-wave infrared longpass filter (Midopt, LP1000) mounted on another digital camera (IDS, 5 Megapixel) with a read-out frequency of five frames-per-second. The purpose of the coarse-tracking camera is to make the pointing independent of attitude-knowledge by identifying the rough position of the Sun in the sky and driving the mirror assembly toward the Sun. Once the Sun is in the field-of-view of the fine-tracking camera, coarse-tracking is switched off and fine-tracking takes over. If fine-tracking fails e.g. due to clouds, the coarse tracking process restarts. For the coarse tracking, it is essential to use a fish-eye lens that approximates a stereographic projection, thereby avoiding heavy compression of the lateral image scale towards low elevation angles such that the mapping from angular coordinates to camera pixel positions is well-posed even under low-sun conditions. It is beneficial to mount the coarse tracking module on the azimuthal rotation stage together with the mirror assembly in order to avoid calibration overhead by spurious tilting and misalignment between the mirror assembly and the coarse-tracking module.
The communication between the control unit (Trinamic 5072) and the cameras works via a USB-2 interface and the rotation motors are connected via RS232. The control unit runs a custom-built driver software that handles the coarse and fine-tracking processes, the feedback loops and the motor control. The feedback loop uses a PID (Proportional-Integral-Derivative) control that works on the departure of a setpoint velocity from the instantaneous velocity. We tested control loops with motor position and acceleration as control variables, but velocity control showed the most stable performance. In total, the feedback loop mechanism takes roughly 30 ms for a single cycle which is sufficient to operate the solar tracker on benign platforms such as ships or balloons. However, it does not allow for continuous operations on road vehicles. For the latter, our setup allows for quick stop-and-go patterns but the solar tracker cannot cope with shocks from ubiquitous road-bumps while driving. A gimbal mount might be required to enable measurements while driving.
Figure 3 shows the departures of the angular mirror positions from the setpoint for the measurements collected during the latest deployment on RV Mirai in the Western North Pacific. The target precision of the solar tracking is 0.05° (Gisi et al., 2011), roughly a tenth of the angular diameter of the solar disk. The solar tracker achieved the required tracking precision for more than 96% of the measurements when fine-tracking mode was operational. This is a substantial improvement over our previous deployments and is largely due to refinements of the tracking software.
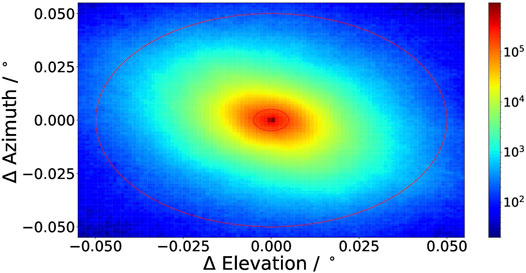
FIGURE 3. Angular departures (in perpendicular azimuth and elevation directions) of the solar tracker mirrors from the setpoint position throughout the RV Mirai cruise for all measurements where the solar tracker was in fine-tracking mode. Colors indicate (logarithmic) occurrence and red circles indicate tracking precisions of 0.05° and 0.005°.
3.2 Retrieval and Data Quality Procedures
Inferring the dry-air column-average mole-fractions XCO2, XCH4 and XCO from the mobile EM27/SUN measurements requires a range of processing and calibration steps, that we summarize here in a recipe-like fashion. Details are reported in Knapp et al. (Knapp et al., 2021).
• The DC-coupled interferograms acquired by the mobile EM27/SUN need to be Fourier-transformed to absorption spectra. We employ the preprocessor software of the COCCON-PROCEEDS project (Sha et al., 2020) with minor modifications related to the output of DC-parameters and to the hand-over of measurement coordinates that vary interferogram-by-interferogram as typical for mobile applications.
• It is important to record the interferograms with DC-part since it allows for extracting a filter criterion defined as the relative peak-to-peak DC-amplitude, i.e., the relative difference between the maximum and the minimum of the DC-part. This criterion is indicative of brightness fluctuations during the 6 s exposures. Such brightness fluctuations are typically caused by unstable pointing towards the Sun which in turn might be caused by uncompensated motion of the platform (i.e. failures of the solar tracking system) or by clouds drifting through the solar beam. If the relative DC-amplitude exceeds 5%, we exclude the interferograms from our records.
• The absorption spectra are then submitted to a radiative transfer and retrieval algorithm that is able to determine the column densities [CO2], [CH4], [CO], and [O2] and those of other interfering absorbers such as [H2O]. For our purposes, we have been using the RemoTeC algorithm (Butz et al., 2011) in its ground-based variant and with the configuration settings and window selections as detailed by Knapp et al. (Knapp et al., 2021). For mobile applications, the algorithm needs to be sufficiently flexible to deal with continuously changing positions of the observer. The retrieval of [O2] serves a two-fold purpose. Firstly, it is used to calculate the column-average dry-air mole fractions through
• The O2 quality filter relies on comparing the surface pressure measured by an in-situ pressure sensor (positioned at the outside top of our spectrometer housing) to its spectroscopic equivalent which is calculated from [O2] assuming a constant mole-fraction of 0.209 4 and from [H2O] considering its contribution to the total pressure. After accounting for a calibration offset between in-situ surface pressure and its spectroscopic counterpart, the two measurements typically agree to within fractions of a percent and the difference serves as a quick sanity check. Spectra that show differences greater than 0.3% (after calibrating the overall offset) in the two surface pressure estimates are removed from the data record and can be regarded as imperfect records despite the fact that the interferogram escaped the DC criterion.
• The retrieved XCO2, XCH4, and XCO typically show a spurious dependency on the position of the Sun which is called the airmass-dependent bias. It has been proposed to be calibrated through a cubic function of the solar zenith angle (SZA) (Wunch et al., 2010). The reasons for the bias are 1) the assumed vertical trace gas profile shape being different from the truth, 2) residual spectroscopic uncertainties, and 3) imperfect calculation of the lightpath as a function of SZA. Here, we use the correction variant implemented by Knapp et al. (Knapp et al., 2021) which reads Xgas, corrected = Xgas/(a ×SZA3 + b ×SZA + c). The coefficients a, b, c are best determined by fitting them to XCO2, XCH4, XCO background records. During our ship cruises such conditions are regularly encountered over the open oceans. Table 2 lists the coefficients for the two most recent deployments on RV Mirai and RV Sonne.
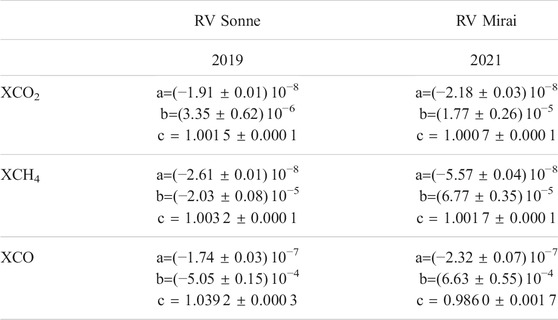
TABLE 2. Correction factors a [1/3◦], b [1/°], c for the airmass dependent correction. Errors are the standard deviations of the fits.
• The final step calibrates the mobile measurements of XCO2, XCH4, and XCO to the measurements of the TCCON and COCCON. To this end, we scale the mobile measurements by a factor that has been determined from side-by-side deployments with a TCCON instrument or a COCCON instrument that is traceable to the TCCON calibration. We conduct these side-by-side deployments typically before and after shipping our instrument into the study regions. Table 3 lists the respective calibration factors for the past years relevant for the deployment on RV Mirai. The calibration factors for XCO2 are reasonably stable over time with differences up to 0.3% which is roughly consistent with the stability reported for stationary applications (Frey et al., 2019). For XCH4, the most recent measurement at Tsukuba shows a change of 0.7% compared to the calibration before and, for XCO, there is an upward trend over time with a step of 2.4% for the measurement at Tsukuba. Currently, the origin of these findings for XCH4 and XCO is unknown and needs to be investigated e.g. through a dedicated side-by-side calibration campaign and a careful check of the instrument alignment. However, inspecting the regular ILS measurements did not reveal any indication for spurious trends in the alignment of the instrument.

TABLE 3. Calibration factors derived from the side-by-side measurements at the TCCON stations Karlsruhe and Tsukuba over the past years. The calibration factors are the average hourly ratios Xgas, TCCON/Xgas,EM27/SUN, the error bars are the standard deviations of the hourly ratios throughout the respective side-by-side deployment.
4 XCO2, XCH4, XCO in the Western Pacific and Along the Coast of Japan
Following the procedure outlined in Section 3.2, we obtain the XCO2, XCH4, and XCO time series for our recent deployment in the Western Pacific and along the coast of Japan on RV Mirai, for which we use the calibration derived from the TCCON side-by-side deployment at Karlsruhe on Oct. 31, 2020 (cf. Table 3). Figure 4 compares our mobile EM27/SUN records to XCO2, XCH4, and XCO from the CAMS near-real-time analysis product (Massart et al., 2014; Inness et al., 2015; Massart et al., 2016), and to XCO retrieved from S5P/TROPOMI measurements. Data from OCO-2 and GOSAT were too sparse to allow for a meaningful comparison. Retrievals of XCH4 over the oceans from TROPOMI are currently not available. For CAMS, we have interpolated the model fields to the locations and sampling times of our ship-borne measurements, and for TROPOMI, we have collected coincidences within a radius of 0.2° and a period of 4 h around our measurement instances. Since we use the CAMS data as a priori in our ground-based retrievals, there is no residual smoothing effects when comparing them. For comparing our data with TROPOMI XCO, we neglected smoothing effects since we consider them to be small. The ground-based and satellite XCO retrievals are performed on the same spectral bands with similar spectral resolution implying similar column sensitivities. Further, the satellite and ground-based retrievals use up-to-data CO prior profiles (from TM5 for TROPOMI) that represent the actual dynamic state of atmosphere.
Our data record is quite sparse in the first half of the campaign due to bad weather conditions but reasonably dense for the later half. We find that the campaign-average hourly standard deviations are 0.33 ppm for XCO2, 1.5 ppb for XCH4, and 1.0 ppb for XCO which we take as a measure of the precision and repeatability of the reported hourly means. These precision estimates are consistent with the previous deployments (cf. Table 1 and references therein). While the mobile EM27/SUN sampled mostly background airmasses until Mar. 18, 2021, there is a clear enhancement of a few ppm in XCO2 (ΔXCO2) and a few 10 ppb in XCO (ΔXCO) for the last days of the cruise (Mar. 19 to Mar. 22, 2021) where, on the last day, the ship’s trajectory was along the southern coast of the main island of Japan (cf. Figure 1) and the lower tropospheric winds came from North-West directions (as modelled by the HySplit online tool (Stein et al., 2015), not shown). For XCH4, variations during the campaign are on the order of 20 ppb but there is no clear enhancement pattern for the last days.
Comparing the ship records to CAMS, we find differences of CAMS XCO2, XCH4, and XCO amounting on average to 4.1 ± 0.5 ppm (mean difference±standard deviation of differences), −32.1 ± 9.7 ppb, and 0.5 ± 5.8 ppb, respectively. The CAMS data follow the observed variability well, i.e., they show an enhancement of XCO2 and XCO for Mar. 19 to Mar. 22, 2021, and they generally follow the observed day-to-day variations. But, CAMS XCO2 is overall high-biased, and CAMS XCH4 is overall low-biased while CAMS XCO fits well on average. For our previous assessment (Knapp et al., 2021) with measurements across the subtropical Pacific in 2019, we did not find these biases for XCO2 and XCH4. However, comparisons of CAMS to TCCON indicate that there might be a time dependent error in CAMS (Schulz et al., 2021) which might explain the larger differences observed here than in (Knapp et al., 2021). These time dependent differences might point to deficiencies in the anthropogenic emissions and natural fluxes (and their temporal variation) that drive the respective concentrations in the CAMS model. Comparing the mobile EM27/SUN data to TROPOMI XCO, we find a mean differences of 9.0 ± 6.6 ppb with TROPOMI showing overall greater scatter than the ship records and confirming the XCO enhancements on Mar. 19 and 22, 2021.
The period Mar. 19 to Mar. 22, 2021 (green box in Figure 4) at the end of the cruise shows correlated enhancements for XCO2 and XCO. To calculate the enhancements, we define the period Mar. 11 to Mar. 18 as a background period (red box in Figure 4). Averaging the records over the background period yields background concentrations amounting to 415.6 ± 0.4 ppm (mean ± standard deviation over period) for XCO2 and 110.4 ± 4.0 ppb for XCO. The choice of the background period causes uncertainties of less than 0.3 ppm and 2 ppb, respectively, as checked by repeating the calculations for other periods. Subtracting the background from the measurements during the enhancement period yields the enhancements ΔXCO2 and ΔXCO. Since the CAMS simulations largely follow the same pattern, we apply the same calculations for ΔXCO2 and ΔXCO and compare the enhancement ratio ΔXCO/ΔXCO2 to our measurements (see Figure 5). The enhancement ratios vary between 30 and 10 ppb/ppm with quite some intra-day and day-to-day variability. CAMS agrees reasonably well with EM27/SUN measurements pointing at a reasonable model representation of both, the transport of emission signatures toward our measurement instances and the relative partitioning of the upwind CO2 and CO emission sources contributing to the enhancement.
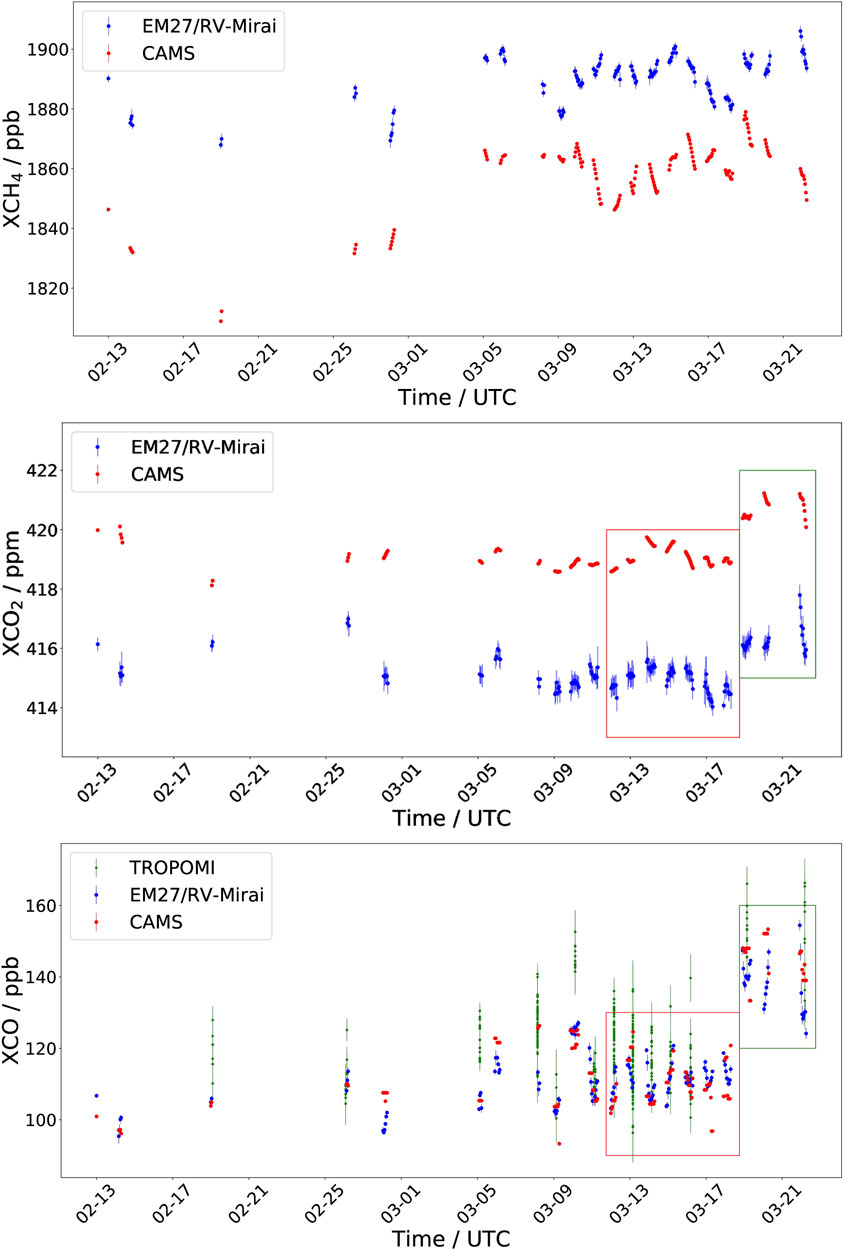
FIGURE 4. Timeseries of XCH4 (upper panel), XCO2 (middle panel), and XCO (lower panel) of the EM27/SUN on RV Mirai (blue), co-sampled CAMS data (red), and co-incident TROPOMI XCO measurements (green). The EM27/SUN records our hourly averages with hourly standard deviations shown as error bars. For TROPOMI, individual XCO data are shown with their provided error-bars. The red and green boxes (upper and lower panel) define the background and enhancement periods, respectively, as discussed in Section 4.
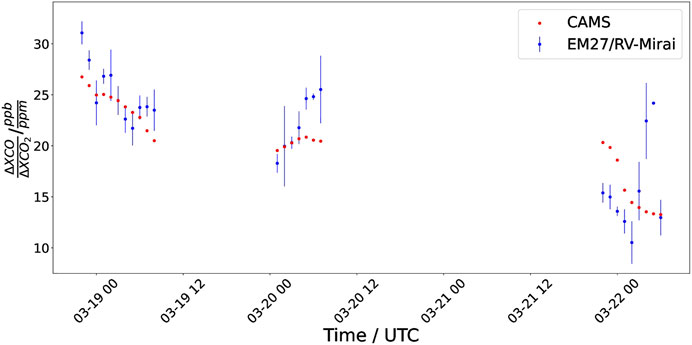
FIGURE 5. Timeseries of the enhancement ratio ΔXCO/ΔXCO2 for the mobile EM27/SUN (blue) and CAMS (red) with a focus on the last period of the RV Mirai cruise.
Overall, the deployment of our mobile EM27/SUN on RV Mirai confirms the fitness of the instrument for providing reliable XCO2, XCH4, and XCO validation measurements over the oceans. On top of sampling background airmasses, we were also able to collect a few days of measurements with XCO2 and XCO enhancements that point to local-to-regional gradients and source signatures although a confirmed attribution to specific regions using backward trajectories was not undertaken here.
5 Prospective Developments and Use Cases
The mobile EM27/SUN is a variant of the FTS operated within the COCCON, supplemented with a fast and flexible solar tracker. If put into operational network-like infrastructures, such versatile and flexible mobile instruments will add substantial value to the validation system for space-borne measurements of XCO2, XCH4, and XCO.
Ship deployments of the mobile EM27/SUN have been demonstrated through three campaigns over the past years (cf. Table 1). The implementation of an operational ship-based EM27/SUN could be realized in the near-term. Our system withstands harsh weather conditions and only minor technical updates are required to make it operate semi-automatically under remote control. The system requires access to electric power and network and it must be placed such that it can observe direct sunlight without obstructions from platform structures. Envisaging deployment on a cargo ship, our experience from previous campaigns suggests that the system would need some basic maintenance of 1–2 days duration on a bimonthly basis in the harbor i.e. collecting data, cleaning optical surfaces, conducting ILS measurements, and, occasionally under sunny conditions, gauging XCO2, XCH4, and XCO towards the TCCON and COCCON by side-by-side deployments of a transfer standard (e.g., another EM27/SUN). The operations on the ship would require some basic, low-bandwidth network interface to check whether the system works nominally and to allow for altering configuration files if needed. While we do not expect that expert personnel needs to travel along, it would be required to train a person on the ship how to clean the outside surfaces and how to operate a standard measurement e.g., in case of anomalous behaviour after power outages or stormy weather. In the context of CO2M and other satellites, we envision the following general use cases for such ship-based EM27/SUN:
1. A few of such ship-borne spectrometers could be arranged in a travelling network that covers the major ship-routes to serve category 1 validation purposes, i.e., validating the continental-to-regional scale concentration gradients across the world’s oceans which are currently practically devoid of validation systems. The meridional transects across the equator might prove particularly useful to evaluate not just satellite data but also the inter-hemispheric transport of long-lived gases in global models (Agusti-Panareda et al., 2017). Targeting at the validation of the minute background gradients, the periodic side-by-side calibration activities to gauge the records to TCCON and COCCON standards are particularly important to guarantee that the data are traceable the common scale of the World Meteorological Organization (WMO).
2. The most recent deployment of our mobile EM27/SUN on RV Mirai (Section 4) demonstrates that by sampling down-wind column curtains along the Japanese southern coast, the system is also able to evaluate the regional-scale emission signature of upwind sources i.e., serving category 2 applications that target at regional-to-local emission patterns. Thus, mobile EM27/SUN operated on ships traveling back and forth along the coastline sampling the outflow of an emission region can contribute to the validation of emission estimates from satellites such as CO2M for the upwind regions.
The installation and operations of EM27/SUN on road vehicles on land are less straight-forward than the ship-based variant. Our previous campaigns show that the solar tracker supports quick stop-and-go patterns, but it does not support measurements while driving. Typically, for any reasonable driving speed, shocks due to uneven road cover cause disturbances of the solar tracking and the mirror retardation of the interferometer inside the FTS. We expect that facilitating operations while driving requires a gimbal mount of the entire spectrometer system (mass roughly 30 kg) which is a technical development step and investment ahead. Further, measurements from a road vehicle are difficult to conduct in an automatic or remote way implying the need for personnel driving the vehicle and operating the instrument. We see the potential use cases as follows:
3. Mobile EM27/SUN operated on road vehicles can add a versatile and flexible component to stationary regional and local networks of COCCON spectrometers. Operated within urban observatory networks (Dietrich et al., 2021), for example, the mobile spectrometers would allow for measuring column curtains between the nodes of the network to better constrain the unsampled airmasses in-between the stationary instruments and to guarantee the relative calibration of the network by frequent side-by-side measurements with the stationary nodes. Thus, this use case would support category 2 applications by helping validate the XCO2 (and XCH4, XCO) gradients that occur on scales of urban agglomerations and by contributing to the validation of emission estimates for the respective region. One might envision a future development of the spectrometer system toward deployment on light-rail trains e.g., sampling urban domes along ring rail tracks. We expect that the current solar tracker would support measurements on driving trains right away in terms of compensating for shocks and vibrations, but the effect of viewing obstructions from the overhead wiring, tunnels, and buildings would need to be evaluated for any particular location. Likewise, deployment on small ships going along channels and rivers might be thinkable for some cities.
4. In the framework of intensive validation campaigns, mobile EM27/SUN on road vehicles can contribute to validating emission estimates of localized, point-like sources (category 2 application) essentially following the recipe of our demonstrator study in the USCB (Luther et al., 2019). To this end, one would select point-sources such as coal-fired power-plants, cement factories and industrial emitters for XCO2 and such as oil, gas, coal production facilities for XCH4 and repeatedly sample their plumes in a cross-sectional pattern by the mobile EM27/SUN operated in stop-and-go mode underneath the plumes. For that matter, it is essential to measure full cross-sections i.e., starting measurements outside the plume moving inside and ending outside on the other side in order to clearly constrain the background concentrations to be used for calculating the plume enhancements. Collecting such plume cross sections on the road requires tens of minutes or more than an hour depending on the local road network and the distance to the source. Thus, the mobile EM27/SUN can hardly validate the instantaneous plume enhancements recorded by the orbiting satellite in a matter of seconds on relatively coarse spatial resolution (roughly 2 × 2 km for CO2M). But, collecting an ensemble of cross-sectional plume enhancements and deriving the instantaneous emission rates can help validate the respective estimates from the satellite data. Such an application certainly requires ensembles of observations from both, the ground and the satellite, since 1) the emission plumes are turbulent i.e., it requires ensemble or time averaging for comparing measurements unless the measurements are exactly coincident, and 2) the emission rates might vary in time due to operating cycles of the facilities. Ideally, the ground-based measurements would be supported by wind profile measurements (e.g., of a mobile Doppler lidar) to drive mass balance (or related) methods for estimating the emission rates.
The use cases 1 through 4 exemplified above imply some further technical developments and some customizations that depend on the particular platform and field of application but they do not require modifications of the key hardware, the spectrometer and the solar tracker, and software. Developing the mobile system towards the ability to measure more molecular species can enhance the versatility of the setup even further:
• During the deployment at Mt. Etna, we operated a UV/visible DOAS (Differential Optical Absorption Spectroscopy) instrument together with the EM27/SUN FTS (Butz et al., 2017). The two instruments shared the solar tracker and a large part of the operational infrastructures. The UV/visible spectral range gives access to species such as sulfur dioxide (SO2) and nitrogen dioxide (NO2) co-emitted with CO2 from volcanoes and combustion processes, respectively. Due to the small background concentrations, the SO2 and NO2 plumes are easier to identify than those of CO2 and thus, they can serve as tracers for defining plume extent, shape and direction, and the constituent ratios are indicative of the emissions processes. In this spirit, CO2M will carry an NO2 spectrometer to better contour combustion plumes and to support the CO2 emission estimates from the respective sources. Thus, supplementing the mobile EM27/SUN with a UV/visible DOAS spectrometer would add the ability to validate NO2 and the NO2/CO2 ratios relevant for the category 2 use cases 2, 3, and 4. Typically, DOAS spectrometers can provide real-time information on the sampled airmasses which is particularly useful for use case 4 to inform on whether one measures inside or outside a plume.
• The spectral range of the mobile EM27/SUN covers the shortwave infrared from roughly 4,000 to 11,000 cm−1 (1.1–2.5 μm) via two detector channels. At Mt. Etna, we demonstrated that this large spectral range also allows for measuring the HCl and HF plumes of the volcano. Like, SO2 measured by the DOAS spectrometer, HCl and HF are co-emitted with CO2 and the respective concentration ratios are indicative for volcano-interior processes. Thus, for dedicated applications beyond satellite validation, developments towards standardizing the retrieval of constituents other than the carbon compounds will be useful.
6 Conclusion
The mobile EM27/SUN is a sun-viewing spectrometer that is able to measure XCO2, XCH4, and XCO from ships and road vehicles, the latter operated in stop-and-go patterns. Throughout our deployments, we find a typical precision of few tenths of a ppm for XCO2, a few ppb for XCH4 and XCO. Regular side-by-side measurements with TCCON and COCCON spectrometers enable diagnosing drifts and ensure traceability with respect to the networks’ calibration. Thus, the mobile EM27/SUN provides a versatile validation tool for CO2M and other sensors. It can be deployed on moving platforms, it can be relocated quickly, and thus, it can be used for targeted validation campaigns in the context of verifying emission estimates for localized sources. The identified uses cases for ship deployments relate to validating background concentrations over the open oceans and enhancement episodes along coast lines due to continental outflow. Through deployments on road vehicles, the mobile EM27/SUN can validate emission estimates for point sources and, it can contribute a mobile component to regional validation networks by sampling the airmasses between the network nodes and providing an internal calibration tool. Further developments will aim at covering UV/visible absorbing gases such as NO2 by co-mounting a DOAS spectrometer and exploring the retrieval of other gases such as HCl and HF that are potentially useful for attribution of emission processes. Technically, the system is ready to be operationalized as part of a satellite validation activity.
Data Availability Statement
The mobile EM27/SUN data from the cruise of RV Mirai are available from https://www.doi.org/10.1594/PANGAEA.937933; availability of previous campaign data is documented in the respective publications (cf. 424 table 1). The CAMS CO$_2$ and CH$_4$ data used in the paper is the official CAMS GHG analysis (https://www.doi.org/10.24380/654b-gm83). The data for CO$_2$ and CH$_4$ is available via request to Copernicus Service Desk by emailing toY29wZXJuaWN1cy1zdXBwb3J0QGVjbXdmLmludA==or via the CAMS enquiry portal in https://www.atmosphere.copernicus.eu/help-and-support. The CAMS CO data is from the CAMS NRT analysis available for download at https://www.doi.org/10.24380/hhra-8c27. TROPOMI CO data are available from https://www.s5phub.copernicus.eu/dhus/#/home.
Author Contributions
RK, VH, and MK developed the shipborne instrument and remotely supported the operations on RV Mirai., MF, AM, IM, and HT contributed to the observations onboard the RV Mirai; AM and MF performed the measurements, HT led the deployment, and IM provided technical guidance. AA-P provided guidance for the CAMS analyses. FH supported the technical developments. JL, FH, HT, and AB discussed the use cases. AB led the overall activity and wrote the paper. All authors read and provided comments on the paper.
Conflict of Interest
The authors declare that the research was conducted in the absence of any commercial or financial relationships that could be construed as a potential conflict of interest.
Publisher’s Note
All claims expressed in this article are solely those of the authors and do not necessarily represent those of their affiliated organizations, or those of the publisher, the editors, and the reviewers. Any product that may be evaluated in this article, or claim that may be made by its manufacturer, is not guaranteed or endorsed by the publisher.
Acknowledgments
We thank the Japan Agency for Marine-Earth Science and Technology (JAMSTEC) for allowing us to make measurements on the RV Mirai. We are grateful to the captain and crew of the RV Mirai for their support. We especially thank Fumikazu Taketani (JAMSTEC), chief scientist on the Mirai, for coordinating and leading the cruise. The development of the COCCON preprocessing tool has been supported by ESA in the framework of the COCCON-PROCEEDS project. The Copernicus Atmosphere Monitoring Service is operated by the European Centre for Medium- Range Weather Forecasts on behalf of the European Commission as part of the Copernicus program.
References
Agusti-Panareda, A., Diamantakis, M., Bayona, V., Klappenbach, F., and Butz, A. (2017). Improving the Inter-hemispheric Gradient of Total Column Atmospheric CO2 and CH4 in Simulations with the ECMWF Semi-lagrangian Atmospheric Global Model. Geosci. Model. Dev. 10, 1–18. doi:10.5194/gmd-10-1-2017
Basu, S., Guerlet, S., Butz, A., Houweling, S., Hasekamp, O., Aben, I., et al. (2013). Global CO2 Fluxes Estimated from GOSAT Retrievals of Total Column CO2. Atmos. Chem. Phys. 13, 8695–8717. doi:10.5194/acp-13-8695-2013
Borsdorff, T., aan de Brugh, J., Hu, H., Hasekamp, O., Sussmann, R., Rettinger, M., et al. (2018). Mapping Carbon Monoxide Pollution from Space Down to City Scales with Daily Global Coverage. Atmos. Meas. Tech. 11, 5507–5518. doi:10.5194/amt-11-5507-2018
Butz, A., Guerlet, S., Hasekamp, O., Schepers, D., Galli, A., Aben, I., et al. (2011). Toward Accurate CO2 and CH4 Observations from GOSAT. Geophys. Res. Lett. 38. doi:10.1029/2011gl047888
Butz, A., Dinger, A. S., Bobrowski, N., Kostinek, J., Fieber, L., Fischerkeller, C., et al. (2017). Remote Sensing of Volcanic CO2, HF, HCl, SO2, and BrO in the Downwind Plume of Mt. Etna. Atmos. Meas. Tech. 10, 1–14. doi:10.5194/amt-10-1-2017
Butz, A., Guerlet, S., Hasekamp, O. P., Kuze, A., and Suto, H. (2013). Using Ocean-Glint Scattered Sunlight as a Diagnostic Tool for Satellite Remote Sensing of Greenhouse Gases. Atmos. Meas. Tech. 6, 2509–2520. doi:10.5194/amt-6-2509-2013
Crowell, S., Baker, D., Schuh, A., Basu, S., Jacobson, A. R., Chevallier, F., et al. (2019). The 2015-2016 Carbon Cycle as Seen from OCO-2 and the Global In Situ Network. Atmos. Chem. Phys. 19, 9797–9831. doi:10.5194/acp-19-9797-2019
Dietrich, F., Chen, J., Voggenreiter, B., Aigner, P., Nachtigall, N., and Reger, B. (2021). MUCCnet: Munich Urban Carbon Column Network. Atmos. Meas. Tech. 14, 1111–1126. doi:10.5194/amt-14-1111-2021
Eldering, A., Wennberg, P. O., Crisp, D., Schimel, D. S., Gunson, M. R., Chatterjee, A., et al. (2017). The Orbiting Carbon Observatory-2 Early Science Investigations of Regional Carbon Dioxide Fluxes. Science 358. doi:10.1126/science.aam5745
Frey, M., Hase, F., Blumenstock, T., Groß, J., Kiel, M., Mengistu Tsidu, G., et al. (2015). Calibration and Instrumental Line Shape Characterization of a Set of Portable FTIR Spectrometers for Detecting Greenhouse Gas Emissions. Atmos. Meas. Tech. 8, 3047–3057. doi:10.5194/amt-8-3047-2015
Frey, M., Sha, M. K., Hase, F., Kiel, M., Blumenstock, T., Harig, R., et al. (2019). Building the COllaborative Carbon Column Observing Network (COCCON): Long-Term Stability and Ensemble Performance of the EM27/SUN Fourier Transform Spectrometer. Atmos. Meas. Tech. 12, 1513–1530. doi:10.5194/amt-12-1513-2019
Gisi, M., Hase, F., Dohe, S., and Blumenstock, T. (2011). Camtracker: a New Camera Controlled High Precision Solar Tracker System for FTIR-Spectrometers. Atmos. Meas. Tech. 4, 47–54. doi:10.5194/amt-4-47-2011
Gisi, M., Hase, F., Dohe, S., Blumenstock, T., Simon, A., and Keens, A. (2012). XCO2-measurements with a Tabletop FTS Using Solar Absorption Spectroscopy. Atmos. Meas. Tech. 5, 2969–2980. doi:10.5194/amt-5-2969-2012
Guerlet, S., Basu, S., Butz, A., Krol, M., Hahne, P., Houweling, S., et al. (2013). Reduced Carbon Uptake during the 2010 Northern Hemisphere Summer from GOSAT. Geophys. Res. Lett. 40, 2378–2383. doi:10.1002/grl.50402
Hakkarainen, J., Ialongo, I., and Tamminen, J. (2016). Direct Space‐based Observations of Anthropogenic CO2 Emission Areas from OCO-2: Direct Observations Of Anthropogenic CO2$. Geophys. Res. Lett. 43, 11,400–11,406. doi:10.1002/2016GL070885
Hase, F., Frey, M., Blumenstock, T., Groß, J., Kiel, M., Kohlhepp, R., et al. (2015). Application of Portable FTIR Spectrometers for Detecting Greenhouse Gas Emissions of the Major City Berlin. Atmos. Meas. Tech. 8, 3059–3068. doi:10.5194/amt-8-3059-2015
Hase, F., Frey, M., Kiel, M., Blumenstock, T., Harig, R., Keens, A., et al. (2016). Addition of a Channel for XCO Observations to a Portable FTIR Spectrometer for Greenhouse Gas Measurements. Atmos. Meas. Tech. 9, 2303–2313. doi:10.5194/amt-9-2303-2016
Hu, H., Landgraf, J., Detmers, R., Borsdorff, T., Aan de Brugh, J., Aben, I., et al. (2018). Toward Global Mapping of Methane with TROPOMI: First Results and Intersatellite Comparison to GOSAT. Geophys. Res. Lett. 45, 3682–3689. doi:10.1002/2018GL077259
Inness, A., Blechschmidt, A.-M., Bouarar, I., Chabrillat, S., Crepulja, M., Engelen, R. J., et al. (2015). Data Assimilation of Satellite-Retrieved Ozone, Carbon Monoxide and Nitrogen Dioxide with ECMWF's Composition-IFS. Atmos. Chem. Phys. 15, 5275–5303. doi:10.5194/acp-15-5275-2015
Jervis, D., McKeever, J., Durak, B. O. A., Sloan, J. J., Gains, D., Varon, D. J., et al. (2021). The GHGSat-D Imaging Spectrometer. Atmos. Meas. Tech. 14, 2127–2140. doi:10.5194/amt-14-2127-2021
Klappenbach, F., Bertleff, M., Kostinek, J., Hase, F., Blumenstock, T., Agusti-Panareda, A., et al. (2015). Accurate mobile Remote Sensing of XCO2 and XCH4 Latitudinal Transects from Aboard a Research Vessel. Atmos. Meas. Tech. 8, 5023–5038. doi:10.5194/amt-8-5023-2015
Knapp, M., Kleinschek, R., Hase, F., Agustí-Panareda, A., Inness, A., Barré, J., et al. (2021). Shipborne Measurements of XCO2, XCH4, and XCO above the Pacific Ocean and Comparison to CAMS Atmospheric Analyses and S5P/TROPOMI. Earth Syst. Sci. Data 13, 199–211. doi:10.5194/essd-13-199-2021
Kuhlmann, G., Broquet, G., Marshall, J., Clément, V., Löscher, A., Meijer, Y., et al. (2019). Detectability of CO2 Emission Plumes of Cities and Power Plants with the Copernicus Anthropogenic CO2 Monitoring (CO2M) mission. Atmos. Meas. Tech. 12, 6695–6719. doi:10.5194/amt-12-6695-2019
Kuze, A., Suto, H., Nakajima, M., and Hamazaki, T. (2009). Thermal and Near Infrared Sensor for Carbon Observation Fourier-Transform Spectrometer on the Greenhouse Gases Observing Satellite for Greenhouse Gases Monitoring. Appl. Opt. 48, 6716. doi:10.1364/AO.48.006716
Liu, J., Bowman, K. W., Schimel, D. S., Parazoo, N. C., Jiang, Z., Lee, M., et al. (2017). Contrasting Carbon Cycle Responses of the Tropical Continents to the 2015-2016 El Niño. Science 358, eaam5690. doi:10.1126/science.aam5690
Luther, A., Kleinschek, R., Scheidweiler, L., Defratyka, S., Stanisavljevic, M., Forstmaier, A., et al. (2019). Quantifying CH4 Emissions from Hard Coal Mines Using mobile Sun-Viewing Fourier Transform Spectrometry. Atmos. Meas. Tech. 12, 5217–5230. doi:10.5194/amt-12-5217-2019
Massart, S., Agusti-Panareda, A., Aben, I., Butz, A., Chevallier, F., Crevoisier, C., et al. (2014). Assimilation of Atmospheric Methane Products into the MACC-II System: from SCIAMACHY to TANSO and IASI. Atmos. Chem. Phys. 14, 6139–6158. doi:10.5194/acp-14-6139-2014
Massart, S., Agustí-Panareda, A., Heymann, J., Buchwitz, M., Chevallier, F., Reuter, M., et al. (2016). Ability of the 4-D-Var Analysis of the GOSAT BESD XCO2 Retrievals to Characterize Atmospheric CO2 at Large and Synoptic Scales. Atmos. Chem. Phys. 16, 1653–1671. doi:10.5194/acp-16-1653-2016
Nassar, R., Hill, T. G., McLinden, C. A., Wunch, D., Jones, D. B. A., and Crisp, D. (2017). Quantifying CO2 Emissions from Individual Power Plants from Space. Geophys. Res. Lett. 44. doi:10.1002/2017GL074702
Palmer, P. I., Feng, L., Baker, D., Chevallier, F., Bösch, H., and Somkuti, P. (2019). Net Carbon Emissions from African Biosphere Dominate Pan-Tropical Atmospheric CO2 Signal. Nat. Commun. 10, 3344. doi:10.1038/s41467-019-11097-w
Pandey, S., Gautam, R., Houweling, S., van der Gon, H. D., Sadavarte, P., Borsdorff, T., et al. (2019). Satellite Observations Reveal Extreme Methane Leakage from a Natural Gas Well Blowout. Proc. Natl. Acad. Sci. USA 116, 26376–26381. doi:10.1073/pnas.1908712116
Schulz, M., Errera, Q., Ramonet, M., Sudarchikova, N., Eskes, H., Basart, S., et al. (2021). Validation Report of the CAMS Near-Real-Time Global Atmospheric Composition Service: Period December 2020 – February 2021. Copernicus Atmosphere Monitoring Service. doi:10.24380/F540-KB09
Sha, M. K., De Mazière, M., Notholt, J., Blumenstock, T., Chen, H., Dehn, A., et al. (2020). Intercomparison of Low- and High-Resolution Infrared Spectrometers for Ground-Based Solar Remote Sensing Measurements of Total Column Concentrations of CO2, CH4, and CO. Atmos. Meas. Tech. 13, 4791–4839. doi:10.5194/amt-13-4791-2020
Sierk, B., Bezy, J.-L., Löscher, A., and Meijer, Y. (2019). “The European CO2 Monitoring Mission: Observing Anthropogenic Greenhouse Gas Emissions from Space,” in International Conference on Space Optics — ICSO 2018. Editors N Karafolas, Z Sodnik, and B Cugny (Chania, Greece: SPIE), 21. doi:10.1117/12.2535941
Stein, A. F., Draxler, R. R., Rolph, G. D., Stunder, B. J. B., Cohen, M. D., and Ngan, F. (2015). NOAA's HYSPLIT Atmospheric Transport and Dispersion Modeling System. Bull. Am. Meteorol. Soc. 96, 2059–2077. doi:10.110.1175/bams-d-14-00110.1
Strandgren, J., Krutz, D., Wilzewski, J., Paproth, C., Sebastian, I., Gurney, K. R., et al. (2020). Towards Spaceborne Monitoring of Localized CO2 Emissions: an Instrument Concept and First Performance Assessment. Atmos. Meas. Tech. 13, 2887–2904. doi:10.5194/amt-13-2887-2020
Suto, H., Kataoka, F., Kikuchi, N., Knuteson, R. O., Butz, A., Haun, M., et al. (2021). Thermal and Near-Infrared Sensor for Carbon Observation Fourier Transform Spectrometer-2 (TANSO-FTS-2) on the Greenhouse Gases Observing SATellite-2 (GOSAT-2) during its First Year in Orbit. Atmos. Meas. Tech. 14, 2013–2039. doi:10.5194/amt-14-2013-2021
Varon, D. J., McKeever, J., Jervis, D., Maasakkers, J. D., Pandey, S., Houweling, S., et al. (2019). Satellite Discovery of Anomalously Large Methane Point Sources from Oil/Gas Production. Geophys. Res. Lett. 46, 13507–13516. doi:10.1029/2019gl083798
Western, L. M., Ramsden, A. E., Ganesan, A. L., Boesch, H., Parker, R. J., Scarpelli, T. R., et al. (2021). Estimates of North African Methane Emissions from 2010 to 2017 Using GOSAT Observations. Environ. Sci. Technol. Lett. 8, 626–632. doi:10.1021/acs.estlett.1c00327
Wunch, D., Toon, G. C., Blavier, J.-F. L., Washenfelder, R. A., Notholt, J., Connor, B. J., et al. (2011). The Total Carbon Column Observing Network. Phil. Trans. R. Soc. A. 369, 2087–2112. doi:10.1098/rsta.2010.0240
Wunch, D., Toon, G. C., Wennberg, P. O., Wofsy, S. C., Stephens, B. B., Fischer, M. L., et al. (2010). Calibration of the Total Carbon Column Observing Network Using Aircraft Profile Data. Atmos. Meas. Tech. 3, 1351–1362. doi:10.5194/amt-3-1351-2010
Keywords: greenhouse gases, remote sensing, satellite validation, direct-sun spectrometers, mobile and versatile
Citation: Butz A, Hanft V, Kleinschek R, Frey MM, Müller A, Knapp M, Morino I, Agusti-Panareda A, Hase F, Landgraf J, Vardag S and Tanimoto H (2022) Versatile and Targeted Validation of Space-Borne XCO2, XCH4 and XCO Observations by Mobile Ground-Based Direct-Sun Spectrometers. Front. Remote Sens. 2:775805. doi: 10.3389/frsen.2021.775805
Received: 14 September 2021; Accepted: 02 December 2021;
Published: 05 January 2022.
Edited by:
Feng Xu, University of Oklahoma, United StatesReviewed by:
Minqiang Zhou, The Royal Belgian Institute for Space Aeronomy (BIRA-IASB), BelgiumXin Ma, Wuhan University, China
Greg Osterman, NASA Jet Propulsion Laboratory (JPL), United States
Copyright © 2022 Butz, Hanft, Kleinschek, Frey, Müller, Knapp, Morino, Agusti-Panareda, Hase, Landgraf, Vardag and Tanimoto. This is an open-access article distributed under the terms of the Creative Commons Attribution License (CC BY). The use, distribution or reproduction in other forums is permitted, provided the original author(s) and the copyright owner(s) are credited and that the original publication in this journal is cited, in accordance with accepted academic practice. No use, distribution or reproduction is permitted which does not comply with these terms.
*Correspondence: André Butz, YW5kcmUuYnV0ekBpdXAudW5pLWhlaWRlbGJlcmcuZGU=