- 1NASA Jet Propulsion Laboratory, California Institute of Technology, Pasadena, CA, United States
- 2NASA Goddard Space Flight Center, Greenbelt, MD, United States
We argue that the Earth Polychromatic Imaging Camera (EPIC) on the Deep Space Climate ObserVatoRy (DSCOVR) platform has blazed new pathways in observational technology, starting with its ∼ 1.5 × 106 km stand-off distance, but also in remote sensing science. We focus here on EPIC’s two oxygen absorption channels that 1) are unique in their spectral sampling and 2) have stimulated deep innovation in cloud remote sensing using Differential Oxygen Absorption Spectroscopy (DO2AS). Although first formulated 6 decades ago, DO2AS-based cloud probing from overhead assets is still an emerging observational technique. It is indeed somewhat paradoxical that one should use absorption by a gas to assay scattering by particles. After surveying the history of space-based DO2AS, and looking into its future, we see that EPIC/DSCOVR marks an inflection point in this important development. EPIC’s unique DO2AS capability motivated a notable sequence of papers revisited here. This research indeed spawned a rare occurrence of information content analysis coming from radically different—yet complementary—perspectives. First, we adopted the increasingly popular machinery of optimal estimation (OE) that is grounded in Bayesian statistics and uses a somehow linearized radiative transfer (RT) model. Nonetheless, OE feels like a black-box algorithm that outputs a number of “degrees of freedom” (a.k.a. independent pieces of information about clouds under observation). However, the very same conclusions are reached using fully transparent physics-based modeling for the RT, with a few approximations that enable closed-form analytical formulation. Lastly, we preview a novel DO2AS technique for regaining shortwave sensitivity to cloud optical thickness past the threshold where cloud reflectivity flattens off.
1 Introduction and Outline
Since the beginning of operational satellite meteorology, NASA’s TIROS-1 (launched 1960), we have been accustomed to seeing clouds as a dynamical 2D map projected onto the Earth’s surface. However, meteorologists and atmospheric scientists in general yearn for knowledge of clouds in the vertical dimension where complex processes in cloud physics unfold, from nucleation to precipitation. This unsatiable thirst for knowledge of the vertical distribution of clouds persists to this day. In fact it permeates NASA’s 2017 Decadal Survey (National Academies of Sciences, Engineering, and Medicine, 2018) not only in the Designated Observables from the Aerosol/Cloud-Convection-Precipitation (ACCP) arena but also in the more experimental Planetary Boundary Layer (PBL) Incubator program.
The earliest known publications on the potential use of molecular absorption to determine cloud height are in a discussion initiated by Hanel (1961), just a year after TIROS-1’s launch. He was indeed promptly engaged by Yamamoto and Wark (1961) and Chapman (1962) who suggest using the oxygen A-band (759–769 nm) because O2 is a dominant constituent with a well-characterized pressure profile. Thus started the idea of using differential Oxygen absorption spectroscopy (DO2AS), a special case of differential optical absorption spectroscopy (DOAS), to probe clouds from space.
In the following Section 2, we survey the history and geography of DO2AS-based sensing of scattering particulates in the Earth’s atmosphere from space. We focus on the period ending in 2010 because that is more-or-less when theory-dominated research is superseded by data-driven work since, by then, several satellites with O2 A-band coverage were in orbit. To balance the theory-heavy literature survey, we describe more-or-less chronologically the core technological aspects of satellite missions so far with DO2AS capability, underscoring EPIC/DSCOVR’s uniqueness. Finally, we gaze into what lies ahead for O2 absorption in satellite missions to be launched in the foreseeable future.
This leads to the lessons-learned from investigating the cloud information content of EPIC’s (764 ± 0.2 nm) and B-band (687.75 ± 0.2 nm) channels using both physics and statistics in Section 3. From there, we connect the implicit dependence of EPIC’s O2 absorption channel responses on the mean pathlength of sunlight in the cloudy medium to recent advances in statistical physics. In turn, that deep dive into the fundamental physics of O2 absorption in scattering media such as clouds reveals a new path toward the inference of cloud optical thickness (COT) for very opaque clouds from DO2AS, not just through radiance levels in continuum channels that are soon saturated as COT increases.
We summarize in Section 4, and contemplate the future of DO2AS observation of clouds from space.
2 Brief History of Space-Based DO2AS, a Look Into the Near-Future and the Special Role of Epic/DSCOVR
Soon after the first suggestion of using DO2AS in cloud sensing (Yamamoto and Wark, 1961; Chapman, 1962), the mathematical connection between the distribution of light paths in scattering media and the detailed shape of the absorption spectrum was rigorously established (Irvine, 1964) This key development was followed by the physically-correct analogy with non-stationary radiation transport (Katsev, 1969; Katsev and Zege, 1974). Astrophysical theoreticians made important early contributions (e.g., Ivanov and Sabashvili, 1972; Nagirner, 1974). The earliest known observations of clouds from space in the O2 A-band are from 1965, using a handheld camera operated aboard Gemini-5 (Saiedy et al., 1965; Saiedy et al., 1967; Wu, 1985). It seems that the first non-astronaut counterparts were performed by a sensor aboard Kosmos 320 in 1970 (Gorodetskiy et al., 1971; Syachinov and Kozlov, 1974), and possibly as early as 1967 with the near-identical Kosmos 149 (Malkevich, 1974).
At any rate, a considerable amount of research on cloud remote sensing using overhead DO2AS was performed in the Former Soviet Union in the 1970s (Dianov-Klokov et al., 1970; Dianov-Klokov and Krasnokutskaya, 1972; Kargin et al., 1972; Malkevich et al., 1975; Dianov-Klokov, 1976; Grechko et al., 1976; Dianov-Klokov et al., 1977; Grechko, 1978), including observations from an aircraft (Grechko et al., 1973) and a satellite (Gorodetskiy et al., 1971; Syachinov and Kozlov, 1974), and into the 1980s (Badayev and Kozlov, 1980; Grechko et al., 1982; Romanova and Ustinov, 1982; Skorinov and Titov, 1984; Gusev and Dvoryashin, 1990). In the West, there was a fast-growing interest in O2 absorption as a means of probing clouds during the 1990s (Fisher et al., 1991; Fisher and Grassl, 1991; O’Brien and Mitchell, 1992; Kuze and Chance, 1994; Asano et al., 1995; Hayazaka et al., 1995; O’Brien et al., 1999) and into the 2000s (Heidinger and Stephens, 2000; Partain et al., 2000; Stephens and Heidinger, 2000; Kokhanovsky et al., 2004; Kokhanovsky and Rozanov, 2004; Rozanov and Kokhanovsky, 2004), with an increasing emphasis on 3D RT signatures (e.g., Heidinger and Stephens, 2002; Kokhanovsky et al., 2007; Davis et al., 2009).
The above extensive but non-exhaustive literature survey of cloud-focused space-based DO2AS ends in 2010. Indeed, by the end of the first decade of the 21st century, there were already several satellites in orbit collecting real DO2AS data on clouds, as we will document in the following (Table 1). We therefore view 2010, somewhat arbitrarily, as the end of an era of theory-dominated research on space-based cloud remote sensing using DO2AS and the beginning of data-driven research. Since then, activity in this field has of course continued to grow steadily. In view of this sustained growth, a Workshop on “Remote sensing in the O2 A-band” was convened at KNMI in de Bilt, Nederlands, in 2016. A Second Workshop on “Remote Sensing in Oxygen Absorption Bands” was planned to happen in Berlin, Germany, in 2020, but has been postponed because of the COVID-19 pandemic to a future date in 2022. At any rate, this shows that there is a well-defined scientific community engaged in DO2AS, for clouds and from space in particular.
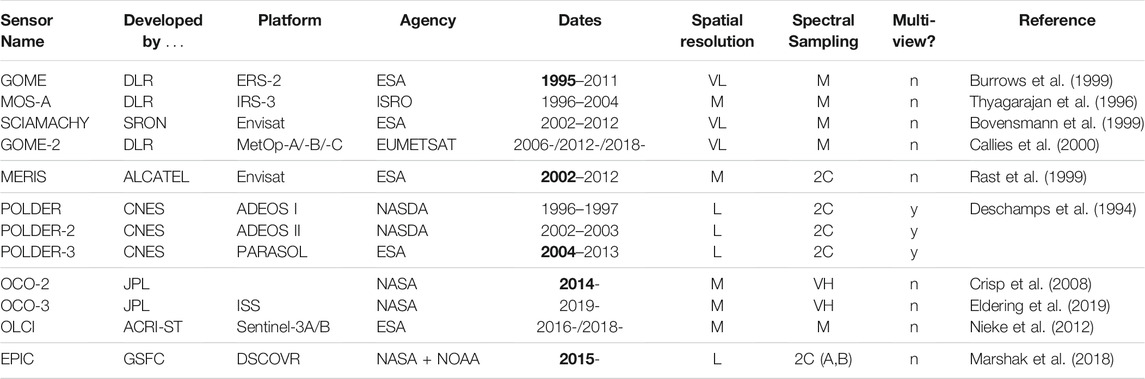
TABLE 1. Compendium of satellite missions with DOAS capability ordered chronologically and clustering sensors with similar characteristics. To the best of our knowledge, SCIAMACHY is the first instrument with an operational DO2AS-based cloud product (Kokhanovsky et al., 2005), followed by the POLDER series (Buriez et al., 1997; Vanbauce et al., 1998) and EPIC (Yang et al., 2019). Research cloud property retrievals have been developed for these missions, e.g., MOS-A (Preusker et al., 2007), POLDER-3 (Ferlay et al., 2010), and others, most recently, OCO-2/3 (Richardson et al., 2017; Richardson and Stephens, 2018; Richardson et al., 2019; Richardson et al., 2020). Such experimental retrievals can and have been transitioned into fully operational elements in the data processing pipeline.
We can now take a more-or-less chronological stroll through satellite missions with imaging DO2AS capability, whether or not implemented with clouds in mind.1 We see five clusters emerge, with spectral sampling and spatial resolution being distinguishing factors. We distinguish between moderate and low spatial resolution based on the implicit definition of “moderate” (M) resolution used by MODIS (MODerate resolution Imaging Spectro-radiometer), namely, pixels that are on the order of 1 km in scale. By this standard, sensors with “low” (L) resolution have pixels on the order of 10 km in scale, and “very low” (VL) resolution sensors have pixels that are several 10s of km. We also distinguish “low,” “moderate” and “very high” (VH) spectral resolutions: respectively, ∼2-to-5, ∼10s, ∼1000s of spectral samples across the (∼10 nm wide) A-band, all of which are useful. Alternatively, there is the “two-channel” (2C) strategy, typically in-band and out-of-band channels from which a single DOAS ratio can be formed; however, it can also be implemented with a narrow/in and broad/in-and-out pair of channels, as was done for POLDER (POLarization and Directionality of the Earth’s Reflectances). Either way, in the 2C scenario, it is important to know if there are single or multiple views. Table 1 displays satellite missions with DOAS capability that we have identified over the past two-and-a-half decades,2 with some key defining characteristics and a reference for more information. Five clusters emerge.
EPIC’s DO2AS capability is new and unique in at least two respects in the realm of technology. First, it has an extreme standoff distance of ∼1.5 106 km to the Lagrange-1 point. From there, the sensor sees almost all of the sunlit hemisphere all the time, i.e., there is no down-time. Additionally, EPIC uses a special spectral sampling strategy based on both the A- and B-bands of the di-oxygen molecule. The advantage in this is not, as we will see in the next section, that these bands have different absorption strengths. Rather, the surface albedo is low, hence less confounding for cloud probing, in at least one of these bands: over water, in both; over vegetated land, in the B-band.
The future of DO2AS in space is bright, especially in Low-Earth Orbit (LEO). There will be two more OLCI/Sentinel-3 launches in the late 2020s, followed by ESA’s TROPOMI/Sentinel-5 series (Veefkind et al., 2012)—with a precursor mission already launched in 2017. EUMETSAT will have a multi-angle/multi-spectral/multi-polarization imager (3MI) (Manolis et al., 2013), with POLDER (hence A-band) legacy, on all of its future MetOp second-generation satellites, starting in 2024. Moreover, EUMETSAT’s Sentinel-4 series (Meteosat Third Generation, MTG), due to be launched in 2023 and 2030, will carry the S4 UVN Multispectral Spectrometer (Riedl et al., 2019) to Geostationary orbit (GEO), with the O2 A-band covered at 0.12 nm resolution. Back in LEO, NASA/JPL’s Multi-Angle Imager for Aerosols (MAIA) mission (Diner et al., 2018) will have a 2C/multi-angle take on the A-band at moderate spatial resolution. NASA’s Plankton, Aerosol, Cloud ocean Ecosystem (PACE) mission (Werdell et al., 2019) will cover the A-band with two of its three sensors at relatively low spectral resolution:
• GSFC’s Ocean Color Instrument (OCI) (Meister et al., 2019), with a moderate spatial resolution, and
• SRON’s SPEXone (Rietjens et al., 2019), with a somewhat lower spatial resolution but offering multiple views and polarization across all wavelengths.
MAIA and PACE are scheduled to launch in the October 2024 – March 2025 timeframe. Last but not least, as part of NASA’s next generation of Earth observing satellites, the Atmospheric Observing System (AOS) implements the 2017 Decadal Survey’s ACCP element; it will include a UV-VIS imaging spectrometer in polar orbit that covers the O2 A-band at low spectral and moderate spatial resolutions, with a launch date in the late 2020s.
3 Information Content of the Pathlength Distribution
We contend that EPIC not only blazed a new path into the observational technology of DO2AS from space, but also in the associated remote sensing science, which we view as enabling Earth system science via remote sensing. To make this point, we briefly revisit a series of papers motivated by EPIC’s two pairs of DOAS channels for the A- and B-bands.
Even before the DSCOVR launch, Yang et al. (2013) devised a method for extracting two cloud properties from EPIC’s two DO2AS ratios, namely, cloud top height (CTH) and cloud geometric thickness (CGT). Specifically, the authors used the sum and difference apparent cloud heights from both ratios, where “apparent” refers to the fact that in-cloud propagation and scattering are not accounted for. However, the fact that they are different is precisely because of the finite pathlength cumulated inside the cloud and the different strengths of the A- and B-bands. Two-entry (CTH,CGT) look-up tables (LUTs) were therefore generated to retrieve the two cloud properties, much like how the Nakajima and King (1990) algorithm delivers cloud optical thickness (COT) and cloud particle effective radius given two reflected radiances, one VIS (with dominant sensitivity to COT) and one SWIR (with dominant sensitivity to particle size).
After the DSCOVR launch and EPIC’s first light, it became clear to the cloud product team that it is important to factor into their algorithms the sensor’s finite radiometric signal-to-noise ratio (SNR). Davis et al. (2018b) therefore followed the well-beaten path of optimal estimation (OE) theory (Rodgers, 2000) to do that. OE is, in essence, a formalism grounded in probabilistic information theory and linear algebra that relates measurement (Level 1) error and any prior/Baysian knowledge to retrieval (Level 2) error. OE has, at its core, a forward RT model that is either linearized or run at sufficient numerical precision to compute accurate Jacobian matrices by finite differencing. However, once implemented in code, the mathematical expressions of OE feel like a “black box” procedure that just has to be trusted. The authors concluded from their formal OE-based cloud information content analysis of EPIC’s two DO2AS ratios that CHT can be inferred with useful accuracy, but not CGT.
It is rare to have a second opinion on the assessment of geophysical information content of some set of measurements that is more transparent in nature, but this did occur for EPIC’s two DO2AS ratios. Indeed, Davis et al. (2018a) derived from first principles a model simple enough to be expressed in closed form, yet realistic enough to capture the main radiative processes unfolding from source to sensor. The authors used this physics-based approach to assess the sensitivities of EPICs DO2AS ratios to CTH and CGT, bearing in mind the finite amplitude of the sensor noise, and they again found a strong response to CTH and a weak one to CGT.
In hindsight, the series of three papers published in the Journal of Quantitative Spectroscopy and Radiative Transfer weave a story about adjusting expectations to sensor and algorithm realities. In the case, it is about EPIC’s ability to probe clouds: cloud top from O2 absorption channel ratios and COT from the radiometrically-calibrated continuum channels, assuming either liquid or ice particles (cf. Yang et al., 2019), but unfortunately not cloud base height via CTH.
That is not however the end of EPIC’s influence on the remote sensing science of O2 absorption observations in application to cloud profiling. By happenstance, EPIC’s DO2AS research team was alerted by N. Ferlay, an expert in POLDER’s A-band information content, about a powerful invariance property of mean pathlength ⟨L⟩ cumulated inside a scattering optical medium of arbitrary shape and internal structure: ⟨L⟩ = 4V/S, where V is the volume of the medium and S is its surface (Blanco and Fournier, 2003). This remarkable result is predicated on uniform and isotropic illumination of the medium, which clashes with the cloud-illuminated-by-the-sun scenario, and integration over all possible escape positions and directions, which conflicts with single direction sampled in remote sensing. There is nonetheless a strong message: once reduced to just in-cloud paths, ⟨L⟩ informs us directly about the size of the medium. For plane-parallel media, where V and S are infinite, ⟨L⟩ = 2H, with H being the geometrical thickness of the slab.3
This begs the question about what pathlength variance Var[L] = ⟨(L − ⟨L⟩)2⟩ = ⟨L2⟩ − ⟨L⟩2 brings to the table in terms of cloud information. Blanco and Fournier (2006) show that, unlike the mean, higher-order statistical moments of L depend on the opacity of the medium: if σ is the mean extinction coefficient, then ⟨Lq⟩ ∝ ⟨L⟩/σq−1, q = 1,2,3,⋯ , as σ → ∞ (i.e., RT diffusion limit). The cloud remote sensing implication is that knowledge of both ⟨L⟩ and Var[L] for in-cloud pathlength L can be used to infer both the bulk size and mean opacity of the cloud;. In plane-parallel cloud geometry, that translates to both CGT H and COT τ = σH, irrespective of the value of the latter. In other words, we are no longer limited to the range of COT where there is enough sensitivity in (continuum) reflected radiance to distinguish a change in COT from a fluctuation in the noise, i.e., up to a few 10s.4 Moreover, while the use of reflected radiance calls for absolute radiometric calibration, inference of moments of L, being based on DO2AS, only requires a relative calibration across spectral channels.
Figure 1 is adapted from a forthcoming paper by Davis et al. (2021) where a new derivation of the invariance law for ⟨L⟩ is presented along with a specific prediction for Var[L](τ) in the diffusion limit for plane-parallel geometry. Numerical validation of the diffusion-theoretical predictions for ⟨L⟩ and Var[L](τ) is performed. Figure 1 shows both moments as a function of τ for both isotropic and Henyey and Greenstein (1941) phase functions, assuming that asymmetry factor g = 0.85 in the latter case. As anticipated, the agreement is exact for ⟨L⟩ across all COTs, and the diffusion-based prediction for Var[L](τ) becomes excellent as the scaled COT τt = (1 − g)τ exceeds ∼4 (τ ≳ 25). At any rate, given ⟨L⟩ and Var[L](τ), one can infer H and τ at any value above ∼ 1/(1 − g), which is precisely when cloud reflectivity in the continuum starts to loose sensitivity to τ.
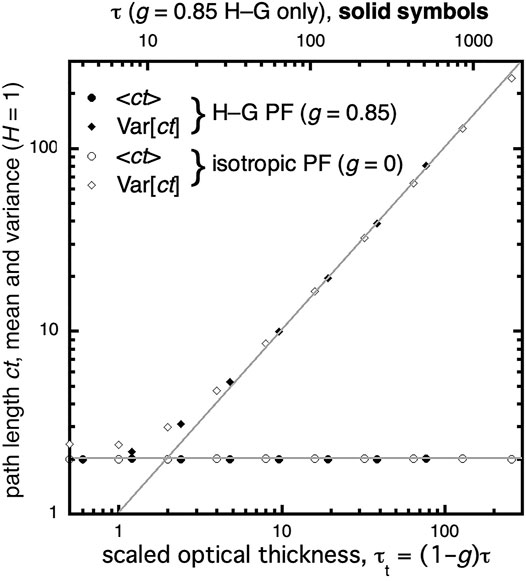
FIGURE 1. Pathlength moments ⟨L⟩ and Var[L](τ) are plotted versus τ in log-log axes. Symbols mark the Monte Carlo simulation results, while diffusion model outcomes are solid lines. The invariance of ⟨L⟩ is verified exactly. Furthermore, the diffusion-theoretical prediction that Var[L](τ) = (1 − g)τ/2χ (with extrapolation scale factor χ set to 2/3) becomes very accurate at τt = (1 − g)τ ∼4. Two phase functions were investigated: g = 0 (isotropic scattering) and g = 0.85 (forward-peaked Henyey and Greenstein (1941) scattering). Adapted from Davis et al. (2021), where ⟨L⟩ is denoted ⟨ct⟩.
In-cloud pathlength L is a random variable, and its moments are emerging here as key intermediate quantities in DO2AS that can be inferred from spectroscopic data at sufficiently high resolution (Davis et al., 2021). Figure 1 indeed shows that, given ⟨L⟩(H) and Var[L](H, (1 − g)τ), we can infer H and τ, knowing that g hardly deviates from 0.85 in liquid clouds. MUltiple Scattering Cloud Lidar (MUSCL) (Davis et al., 1999a; Davis A. B. et al., 1999b; Davis et al., 2009) is another emerging technology in cloud remote sensing from above or below (Cahalan et al., 2005; Polonsky et al., 2005; Davis, 2008) where the whole distribution of in-cloud pathlengths is measured directly. This is done by temporal binning the return times (i.e., L/c) of photons injected into a cloud using a pulsed laser beam. Now, the signal in each time bin can be noisy, but the statistical moments ⟨L⟩ and Var[L] are robust. As different as are their instrumental implementations, it is clear that DOxAs and MUSCL share the same fundamental signal physics grounded in time-dependent RT. Interestingly, DO2AS is an inherently daytime observation while MUSCL operates strictly at nighttime since the steady sunlight diffusely reflected or transmitted by the cloud would overwhelm the laser light in every time-bin (Davis, 2008). DO2AS and MUSCL are therefore the ideal pair of instruments for a satellite mission for pathlength-based cloud observation that would deliver CTH, CGT, COT and possibly a measure of internal variability from turbulence (Davis et al., 2009).
4 Summary and Discussion
In this PERSPECTIVE article, we above all celebrate space-based remote sensing using O2 absorption to track clouds in the vertical dimension above every pixel. To that effect, we survey the relevant literature emphasizing theory up to 2010, which is roughly when there were enough space assets delivering O2 A-band observations of clouds to see the research become predominantly data-driven. We hope to see others write the important literature review about post-2010 studies of clouds from space-borne O2 absorption observations. Another worthwhile review would focus on ground-based cloud studies with O2 absorption spectroscopy, and yet another should focus on using O2 absorption spectroscopy from above or below to locate aerosol layers in the vertical dimension.
Building on our limited-scope literature survey, we support the viewpoint that EPIC/DSCOVR has been a pathfinder in O2 absorption-based cloud remote sensing. Several other satellites carry sensors with O2 absorption capability, EPIC however has by far the largest standoff distance and is also unique in its spectral sampling strategy: “in-band” and “continuum” pairs of channels each for the A- and B-bands. Another hallmark of EPIC’s use of O2 absorption to probe clouds is the impetus it has generated for progress in the associated remote sensing science that is centered on the concept of pathlength cumulated by sunlight, from source to sensor, between every scattering event along the way. To substantiate this claim, we revisited three papers in the Journal of Quantitative Spectroscopy and Radiative Transfer that directly address EPIC’s characterization of clouds using O2 absorption, and previewed a key result from a forthcoming one.
Determination of cloud structure in the third dimension is a goal shared by O2 absorption spectroscopy and other emerging techniques in cloud remote sensing, for instance, 3D computational cloud tomography (CCT). CCT has, so far, been demonstrated on data with small pixel scales that are readily achievable with airborne multi-view sensors, whether imaging (Levis et al., 2015; Levis et al., 2017; Levis et al., 2020; Levis et al., 2021) or not (Alexandrov et al., 2021). When dealing with such fine pixels, in the 10s of meters, there are necessarily significant radiative fluxes crossing pixel boundaries, thus requiring 3D RT forward modeling. There has been recent progress toward 3D CCT from space using moderate (∼100s of meters) resolution multi-angle data from the likes of MODIS and MISR/Terra. On top of the 3D RT effects, this effort has to deal with complications from the larger pixels (optically thick, potentially with high internal heterogeneity) and accordingly larger clouds (Forster et al., 2021).
Looking ahead, we know that O2 absorption observation is typically implemented in spectroscopy, and the more channels in the absorption band the better since the diversity in absorption coefficient ensures probing different depths into the cloud. However, this key ability can also be obtained using a single absorption channel, as for either of EPIC’s O2 absorption bands, in a multi-view angle collection. POLDER (2004–2013) pioneered the multi-view O2 absorption observation strategy, and that path will be followed in short order by MAIA, SPEXone/PACE and 3MI. Spectroscopy-based O2 absorption is also heading into a bright future, starting with OCI/PACE and NASA’s upcoming Atmospheric Observing System (AOS). Someday, we may see the deployment of DSCOVR follow-on missions at Lagrange-1 and Lagrange-2 (Valero et al., 2021). At any rate, it will be interesting to see how future synergistic retrievals will blend O2 absorption spectroscopy with other passive sensing modalities, such as multi-view imaging and CCT, thus enabling robust 3D cloud property retrievals on a global scale.
Data Availability Statement
Inquiries about data used in Figure 1 can be directed to the corresponding author.
Author Contributions
AD proposed and wrote this article for the Special Issue on “DSCOVR EPIC/NISTAR: Five Years of Observing Earth from the First Lagrangian Point.” YY is the DSCOVR Science Team PI responsible for operational EPIC cloud products, including cloud heights from O2 A- and B-band channels; AM is Deputy Project Scientist for the DSCOVR mission. They both provided substantive feedback on the manuscript.
Funding
YY acknowledges sustained funding from the DSCOVR Science Team program. AD is supported by NASA’s SMD/ESD DSCOVR mission (EPIC CLOUD ALGORITHMS, Project: 105357, Task: 239702.04.32.01.13, HQs PM Richard S. Eckman). This work was carried out at the Jet Propulsion Laboratory, California Institute of Technology, under a contract with the National Aeronautics and Space Administration (80NM0018D0004).
Conflict of Interest
The authors declare that the research was conducted in the absence of any commercial or financial relationships that could be construed as a potential conflict of interest.
Publisher’s Note
All claims expressed in this article are solely those of the authors and do not necessarily represent those of their affiliated organizations, or those of the publisher, the editors and the reviewers. Any product that may be evaluated in this article, or claim that may be made by its manufacturer, is not guaranteed or endorsed by the publisher.
Acknowledgments
We thank Mark Richardson, Quentin Libois, Nicolas Ferlay, Qilong Min, Jay Hermann, Dennis O’Brien, and Andy Sayer for many fruitful discussions about the science and history of probing clouds from space with DO2AS techniques.
Footnotes
1We pass on the Sea-viewing Wide Field-of-view Sensor (SeaWiFS) on the OrbView-2 (a.k.a. SeaStar) satellite mission, from 1997 to 2010. Its channel 7 (745–785 nm) covers the O2 A-band but it was never exploited for atmospheric scattering. In fact, the O2 absorption was a minor impediment for the targeted ocean color sensitivity that was eventually “corrected” out of the signal (Wang, 1999).
2At first glance, Table 1 seems to show that NASA was the last space agency to develop and launch satellite missions with DO2AS capability as late as the mid-2010s. That is, however, far from true. Both NASA/JPL’s CloudSat and the joint NASA/LaRC - CNES CALIPSO (co-launched into the A-train in 2006) were originally planned to have A-band imagers that were later descoped. With their inherent sensitivity to CTH, these A-band cameras would have extended at least CTH detection from the actively-probed sub-track “curtain” into the across-track direction.
3Picture a finite cylinder with radius R and thickness H: V = H×(π R2) and S = 2×π R2 + H×(2πR). As R ˗ ∞, 〈L〉 = 4V/S ˗ 2H.
4Indeed, an opaque cloud's reflectivity R(τ) can be approximated by
References
Alexandrov, M. D., Emde, C., Van Diedenhoven, B., and Cairns, B. (2021). Application of Radon Transform to Multi-Angle Measurements Made by the Research Scanning Polarimeter: A New Approach to Cloud Tomography. Part I: Theory and Tests on Simulated Data. Front. Remote Sens. 2, 791130. doi:10.3389/frsen.2021.791130
Asano, S., Shiobara, M., and Uchiyama, A. (1995). Estimation of Cloud Physical Parameters from Airborne Solar Spectral Reflectance Measurements for Stratocumulus Clouds. J. Atmos. Sci. 52, 3556–3576. doi:10.1175/1520-0469(1995)052<3556:eocppf>2.0.co;2
Badayev, V., and Kozlov, Y. (1980). On a Determination of the Optical Parameters of the Atmosphere from Reflected Radiation Measurements in the 0.76 μm Oxygen Absorption Band. Izv. Acad. Sci. Ussr, Atmos. Oceanic Phys. 16, 375–377.
Blanco, S., and Fournier, R. (2003). An Invariance Property of Diffusive Random Walks. Europhys. Lett. 61, 168–173. doi:10.1209/epl/i2003-00208-x
Blanco, S., and Fournier, R. (2006). Short-Path Statistics and the Diffusion Approximation. Phys. Rev. Lett. 97 (23), 230604. doi:10.1103/physrevlett.97.230604
Bovensmann, H., Burrows, J. P., Buchwitz, M., Frerick, J., Noël, S., Rozanov, V. V., et al. (1999). SCIAMACHY: Mission Objectives and Measurement Modes. J. Atmos. Sci. 56 (2), 127–150. doi:10.1175/1520-0469(1999)056<0127:smoamm>2.0.co;2
Buriez, J. C., Vanbauce, C., Parol, F., Goloub, P., Herman, M., Bonnel, B., et al. (1997). Cloud Detection and Derivation of Cloud Properties from POLDER. Int. J. Remote Sensing 18 (13), 2785–2813. doi:10.1080/014311697217332
Burrows, J. P., Weber, M., Buchwitz, M., Rozanov, V., Ladstätter-Weißenmayer, A., Richter, A., et al. (1999). The Global Ozone Monitoring Experiment (GOME): Mission Concept and First Scientific Results. J. Atmos. Sci. 56 (2), 151–175. doi:10.1175/1520-0469(1999)056<0151:tgomeg>2.0.co;2
Cahalan, R. F., McGill, M., Kolasinski, J., Várnai, T., and Yetzer, K. (2005). THOR-Cloud Thickness from Offbeam Lidar Returns. J. Atmos. Oceanic Tech. 22 (6), 605–627. doi:10.1175/jtech1740.1
Callies, J., Corpaccioli, E., Eisinger, M., Hahne, A., and Lefebvre, A. (2000). GOME-2-Metop’s Second-Generation Sensor for Operational Ozone Monitoring. ESA Bull. 102, 28–36.
Chapman, R. M. (1962). Cloud Distributions and Altitude Profiles from a Satellite. Planet. Space Sci. 9, 70–71. doi:10.1016/0032-0633(62)90074-0
Crisp, D., Miller, C. E., and DeCola, P. L. (2008). NASA Orbiting Carbon Observatory: Measuring the Column Averaged Carbon Dioxide Mole Fraction from Space. J. Appl. Remote Sens. 2 (1), 023508. doi:10.1117/1.2898457
Davis, A. B., Cahalan, R. F., Spinehirne, J. D., McGill, M. J., and Love, S. P. (1999a). Off-Beam Lidar: An Emerging Technique in Cloud Remote Sensing Based on Radiative Green-Function Theory in the Diffusion Domain (Erratum). Phys. Chem. Earth B24, 757–765. doi:10.1016/S1464-1909(99)00077-5
Davis, A. B., Cahalan, R. F., Spinehirne, D., McGill, M. J., and Love, S. P. (1999b). Off-beam Lidar: An Emerging Technique in Cloud Remote Sensing Based on Radiative Green-Function Theory in the Diffusion Domain. Phys. Chem. Earth, B: Hydrol. Oceans Atmosphere 24, 177–185. doi:10.1016/s1464-1909(98)00034-3
Davis, A. B., Ferlay, N., Libois, Q., Marshak, A., Yang, Y., and Min, Q. (2018a). Cloud Information Content in EPIC/DSCOVR's Oxygen A- and B-Band Channels: A Physics-Based Approach. J. Quantitative Spectrosc. Radiative Transfer 220, 84–96. doi:10.1016/j.jqsrt.2018.09.006
Davis, A. B., Libois, Q., Ferlay, N., and Marshak, A. (2021). Moments of Sunlight Pathlength in Water and Aerosol Clouds from O2 Spectroscopy: Exploitable Parameter Sensitivities. J. Quant. Spectrosc. Rad. Transf. (in preparation). Available at: https://arxiv.org/abs/2110.07231.
Davis, A. B., Merlin, G., Cornet, C., Labonnote, L. C., Riédi, J., Ferlay, N., et al. (2018b). Cloud Information Content in EPIC/DSCOVR's Oxygen A- and B-Band Channels: An Optimal Estimation Approach. J. Quantitative Spectrosc. Radiative Transfer 216, 6–16. doi:10.1016/j.jqsrt.2018.05.007
Davis, A. B. (2008). Multiple-Scattering Lidar from Both Sides of the Clouds: Addressing Internal Structure. J. Geophys. Res. 113, D14S10–14S25. doi:10.1029/2007jd009666
Davis, A. B., Polonsky, I. N., and Marshak, A. (2009). “Space-Time Green Functions for Diffusive Radiation Transport, in Application to Active and Passive Cloud Probing,” in Light Scattering Reviews. Editor A. Kokhanovsky (Heidelberg (Germany): Springer-Praxis), 4, 169–292. doi:10.1007/978-3-540-74276-0_5
Deschamps, P.-Y., Bréon, F.-M., Leroy, M., Podaire, A., Bricaud, A., Buriez, J.-C., et al. (1994). The POLDER Mission: Instrument Characteristics and Scientific Objectives. IEEE Trans. Geosci. Remote Sensing 32, 598–615. doi:10.1109/36.297978
Dianov-Klokov, V. (1976). Determination of Effective Photon Path Lengths from the Spectral Brightness of Clouds. Izv. Acad. Sci. Ussr, Atmos. Oceanic Phys. 12, 221–224.
Dianov-Klokov, V., and Krasnokutskaya, L. (1972). Comparison of Observed and Calculated Effective Photon Path Length in Clouds. Izv. Acad. Sci. Ussr, Atmos. Oceanic Phys. 8, 487–492.
Dianov-Klokov, V., Kropotkina, E., Malkov, I., and Matveyeva, O. (1970). Absorption-band Deformation and the Effective Path Length of Light in Clouds. Izv. Acad. Sci. Ussr, Atmos. Oceanic Phys. 6, 458–463.
Dianov-Klokov, V., Yevstratov, N., and Ozerenskiy, A. (1977). Calculation of Radiant Energy Density and Equivalent Photon Paths for Certain Cloud Models. Izv. Acad. Sci. Ussr, Atmos. Oceanic Phys. 13, 217–221.
Diner, D. J., Boland, S. W., Brauer, M., Bruegge, C., Burke, K. A., Chipman, R., et al. (2018). Advances in Multiangle Satellite Remote Sensing of Speciated Airborne Particulate Matter and Association with Adverse Health Effects: From MISR to MAIA. J. Appl. Remote Sensing 12, 042603. doi:10.1117/1.jrs.12.042603
Eldering, A., Taylor, T. E., O'Dell, C. W., and Pavlick, R. (2019). The OCO-3 Mission: Measurement Objectives and Expected Performance Based on 1 Year of Simulated Data. Atmos. Meas. Tech. 12, 2341–2370. doi:10.5194/amt-12-2341-2019
Ferlay, N., Thieuleux, F., Cornet, C., Davis, A. B., Dubuisson, P., Ducos, F., et al. (2010). Toward New Inferences about Cloud Structures from Multidirectional Measurements in the Oxygen A Band: Middle-Of-Cloud Pressure and Cloud Geometrical Thickness from POLDER-3/PARASOL. J. Appl. Meteorol. Climatology 49, 2492–2507. doi:10.1175/2010jamc2550.1
Fisher, J., Cordes, W., Schmitz-Pfeiffer, A., Renger, W., and Mörl, P. (1991). Detection of Cloud-Top Height from Backscattered Radiances within the Oxygen A Band, 2, Measurements. J. Appl. Meteorol. 30, 1245–1259.
Fisher, J., and Grassl, H. (1991). Detection of Cloud-Top Height from Backscattered Radiances within the Oxygen A Band, 1, Theory. J. Appl. Meteorol. 30, 1260–1267.
Forster, L., Davis, A. B., Diner, D. J., and Mayer, B. (2021). Toward Cloud Tomography from Space Using MISR and MODIS: Locating the “Veiled Core” in Opaque Convective Clouds. J. Atmos. Sci. 78, 155–166. doi:10.1175/jas-d-19-0262.1
Gorodetskiy, A., Malkevich, M., and Syachinov, V. (1971). Determination of Cloud Height from Radiation Measurements from the “Cosmos-320” Artificial Earth Satellite. Dokl. Akad. Nauk SSSR 200 (3), 588–590. (Available in Russian at http://mi.mathnet.ru/dan36441.
Grechko, Y., Dianov-Klokov, V., and Malkov, I. (1973). Aircraft Measurements of Photon Paths in Reflection and Transmission of Light by Clouds in the 0.76 μm Oxygen Band. Izv. Acad. Sci. Ussr, Atmos. Oceanic Phys. 9, 262–269.
Grechko, Y., Dianov-Klokov, V., Yevstratov, N., and Ozerenskiy, A. (1976). Calculation of Mean and Effective Photon Paths for a Two-Layer Cloud Model with Consideration of Reflection from the Underlying Surface. Izv. Acad. Sci. Ussr, Atmos. Oceanic Phys. 12, 20–24.
Grechko, Y., Dvoryashin, S., and Red’ko, A. (1982). Evaluation of Effect of Scattering Indicatrix on the Mean Photon Ranges in Clouds. Izv. Acad. Sci. Ussr, Atmos. Oceanic Phys. 18, 854–856.
Grechko, Y. (1978). Measuring the Difference in Effective Paths at Two Wavelenghts in the Presence of Reflection of Light by Clouds. Izv. Acad. Sci. Ussr, Atmos. Oceanic Phys. 14, 479–481.
Gusev, I., and Dvoryashin, S. (1990). Recovery of the Effective Photon Path Length in Cloud from its Spectral Brightness. Izv. Acad. Sci. Ussr, Atmos. Oceanic Phys. 26, 536–539.
Hanel, R. A. (1961). Determination of Cloud Altitude from a Satellite. J. Geophys. Res. 66, 1300. doi:10.1029/jz066i004p01300
Hayazaka, A., Nakajima, T., Fujiyoshi, Y., Ishikaza, Y., Takeda, T., and Tanaka, M. (1995). Geometrical Thickness, Liquid Water Content, and Radiative Properties of Stratocumulus Over the Western North Pacific. J. Appl. Meteorol. 34, 460–470.
Heidinger, A. K., and Stephens, G. L. (2000). Molecular Line Absorption in a Scattering Atmosphere. Part II: Application to Remote Sensing in the O2A Band. J. Atmos. Sci. 57, 1615–1634. doi:10.1175/1520-0469(2000)057<1615:mlaias>2.0.co;2
Heidinger, A. K., and Stephens, G. L. (2002). Molecular Line Absorption in a Scattering Atmosphere. Part III: Pathlength Characteristics and Effects of Spatially Heterogeneous Clouds. J. Atmos. Sci. 59, 1641–1654. doi:10.1175/1520-0469(2002)059<1641:mlaias>2.0.co;2
Henyey, L. C., and Greenstein, J. L. (1941). Diffuse Radiation in the Galaxy. ApJ 93, 70–83. doi:10.1086/144246
Irvine, W. (1964). The Formation of Absorption Bands and the Distribution of Photon Optical Paths in a Scattering Atmosphere. Bull. Astron. Inst. Neth. 17, 226–279.
Ivanov, V. V., and Sabashvili, S. A. (1972). Transfer of Resonance Radiation and Photon Random Walks. Astrophys Space Sci. 17, 13–22. doi:10.1007/bf00642537
Kargin, B., Krasnokutskaya, L., and Feygel’son, Y. M. (1972). Reflection and Absorption of Solar Radiant Energy by Cloud Layers. Izv. Acad. Sci. Ussr, Atmos. Oceanic Phys. 8, 287–293.
Katsev, I. (1969). The Study of Some Characteristics of a Transient Field of Light. Zhurnal Prikladnoi Spektroskopii 11, 85–91. [in Russian]. doi:10.1007/bf00612535
Katsev, I., and Zege, E. (1974). On the Connection between Nonstationary Radiation fields in Absorbing and Nonabsorbing media. Astrofizika 10, 219–225. [in Russian].
Kokhanovsky, A. A., Mayer, B., Rozanov, V. V., Wapler, K., Burrows, J. P., and Schumann, U. (2007). The Influence of Broken Cloudiness on Cloud Top Height Retrievals Using Nadir Observations of Backscattered Solar Radiation in the Oxygen A-Band. J. Quantitative Spectrosc. Radiative Transfer 103, 460–477. doi:10.1016/j.jqsrt.2006.06.003
Kokhanovsky, A. A., Rozanov, V. V., Burrows, J. P., Eichmann, K.-U., Lotz, W., and Vountas, M. (2005). The SCIAMACHY Cloud Products: Algorithms and Examples from ENVISAT. Adv. Space Res. 36, 789–799. doi:10.1016/j.asr.2005.03.026
Kokhanovsky, A. A., and Rozanov, V. V. (2004). The Physical Parameterization of the Top-Of-Atmosphere Reflection Function for a Cloudy Atmosphere-Underlying Surface System: the Oxygen A-Band Case Study. J. Quantitative Spectrosc. Radiative Transfer 85, 35–55. doi:10.1016/s0022-4073(03)00193-6
Kokhanovsky, A. A., Rozanov, V. V., von Hoyningen-Huene, W., Bovensmann, H., Burrows, J. P., and Baltink, H. K. (2004). The Determination of Cloud Altitudes Using SCIAMACHY Onboard ENVISAT. IEEE Geosci. Remote Sensing Lett. 1, 211–214. doi:10.1109/lgrs.2004.830123
Kuze, A., and Chance, K. (1994). Analysis of Cloud Top Height and Cloud Coverage from the O2 A and B Bands. J. Geophys. Res. 99 (14), 481–491. doi:10.1029/94jd01152
Levis, A., Davis, A. B., Loveridge, J. R., and Schechner, Y. Y. (2021). “3d Cloud Tomography and Droplet Size Retrieval from Multi-Angle Polarimetric Imaging of Scattered Sunlight from above,” in Polarization Science and Remote Sensing X (Bellingham, WA: International Society for Optics and Photonics), 11833–05. doi:10.1117/12.2593369
Levis, A., Schechner, Y. Y., Aides, A., and Davis, A. B. (2015). “Airborne Three-Dimensional Cloud Tomography,” in Proceedings of the IEEE International Conference on Computer Vision, Cambridge, MA, USA, 20-23 June 1995, 3379–3387. doi:10.1109/iccv.2015.386
Levis, A., Schechner, Y. Y., Davis, A. B., and Loveridge, J. (2020). Multi-View Polarimetric Scattering Cloud Tomography and Retrieval of Droplet Size. Remote Sensing 12, 2831. doi:10.3390/rs12172831
Levis, A., Schechner, Y. Y., and Davis, A. B. (2017). “Multiple-Scattering Microphysics Tomography,” in Proceedings of the IEEE Conference on Computer Vision and Pattern Recognition, San Juan, PR, USA, 17-19 June 1997, 6740–6749. doi:10.1109/cvpr.2017.614
Malkevich, M., Chagar, L., and Shukurov, A. (1975). Corrections for Scattering of Radiation in Clouds in Photometric Cloud-Height Determination. Izv. Acad. Sci. Ussr, Atmos. Oceanic Phys. 11, 561–564.
Malkevich, M. (1974). “Optical Investigations of the Atmosphere Using Artificial Satellites. NASA Technical Translation NASA TT F-15186, National Aeronautics and Space Administration, Washington, D.C,” in Translation of “Opticheskiye Issledovaniya Atmosfery So Sputnikov,” (Moscow: Nauka Press) 1973, 303 pp.
Manolis, I., Grabarnik, S., Caron, J., Bézy, J.-L., Loiselet, M., Betto, M., et al. (2013). “The MetOp Second Generation 3MI Instrument,” in Sensors, Systems, and Next-Generation Satellites XVII (Bellingham, WA: International Society for Optics and Photonics), 88890J. doi:10.1117/12.2028662
Marshak, A., Herman, J., Adam, S., Karin, B., Carn, S., Cede, A., et al. (2018). Earth Observations from DSCOVR EPIC Instrument. Bull. Am. Meteorol. Soc. 99, 1829–1850. doi:10.1175/bams-d-17-0223.1
Meister, G., Knuble, J. J., Cook, W. B., Gorman, E. T., and Werdell, P. J. (2019). “Calibration Plan for the Ocean Color Instrument (Oci) Engineering Test Unit,” in Sensors, Systems, and Next-Generation Satellites XXIII (Bellingham, WA: International Society for Optics and Photonics), 11151, 111511W. doi:10.1117/12.2550820
Nagirner, D. (1974). Theory of Nonstationary Transfer of Radiation. Astrofizika (Engl. Transl.: Astrophysics) 10, 274–289.
Nakajima, T., and King, M. D. (1990). Determination of the Optical Thickness and Effective Particle Radius of Clouds from Reflected Solar Radiation Measurements. Part I: Theory. J. Atmos. Sci. 47, 1878–1893. doi:10.1175/1520-0469(1990)047<1878:dotota>2.0.co;2
National Academies of Sciences, Engineering, and Medicine (2018). Thriving on Our Changing Planet, A Decadal Strategy for Earth Observation from Space. Washington (DC): The National Academies Press.
Nieke, J., Borde, F., Mavrocordatos, C., Berruti, B., Delclaud, Y., Riti, J. B., et al. (2012). “The Ocean and Land Colour Imager (OLCI) for the Sentinel 3 GMES Mission: Status and First Test Results,” in Earth Observing Missions and Sensors: Development, Implementation, and Characterization II (Bellingham, WA: International Society for Optics and Photonics), 8528, 85280C. doi:10.1117/12.977247
O’Brien, D., Mitchell, R., English, S., and Da Costa, G. (1999). Airborne Measurements of Air Mass from O2 A-Band Absorption Spectra. J. Atmos. Oceanic Tech. 15, 1272–1286.
O’Brien, D., and Mitchell, R. (1992). Error Estimates for the Retrieval of Cloud Top Pressure Using Absorption in the A-Band of Oxygen. J. Appl. Meteorol. 31, 1179–1192.
Partain, P. T., Heidinger, A. K., and Stephens, G. L. (2000). High Spectral Resolution Atmospheric Radiative Transfer: Application of the Equivalence Theorem. J. Geophys. Res. 105, 2163–2177. doi:10.1029/1999jd900328
Polonsky, I. N., Love, S. P., and Davis, A. B. (2005). Wide-angle Imaging Lidar Deployment at the Arm Southern Great plains Site: Intercomparison of Cloud Property Retrievals. J. Atmos. Oceanic Tech. 22, 628–648. doi:10.1175/jtech1741.1
Preusker, R., Fischer, J., Albert, P., Bennartz, R., and Schüller, L. (2007). Cloud‐Top Pressure Retrieval Using the Oxygen A‐Band in the IRS‐3 MOS Instrument. Int. J. Remote Sensing 28, 1957–1967. doi:10.1080/01431160600641632
Rast, M., Bezy, J. L., and Bruzzi, S. (1999). The ESA Medium Resolution Imaging Spectrometer MERIS a Review of the Instrument and its mission. Int. J. Remote Sensing 20, 1681–1702. doi:10.1080/014311699212416
Richardson, M., Lebsock, M. D., McDuffie, J., and Stephens, G. L. (2020). A New Orbiting Carbon Observatory 2 Cloud Flagging Method and Rapid Retrieval of marine Boundary Layer Cloud Properties. Atmos. Meas. Tech. 13, 4947–4961. doi:10.5194/amt-13-4947-2020
Richardson, M., Leinonen, J., Cronk, H. Q., McDuffie, J., Lebsock, M. D., and Stephens, G. L. (2019). Marine Liquid Cloud Geometric Thickness Retrieved from OCO-2's Oxygen A-Band Spectrometer. Atmos. Meas. Techn. 12, 1717–1737. doi:10.5194/amt-12-1717-2019
Richardson, M., McDuffie, J., Stephens, G. L., Cronk, H. Q., and Taylor, T. E. (2017). The OCO-2 Oxygen A-Band Response to Liquid marine Cloud Properties from CALIPSO and MODIS. J. Geophys. Res. Atmos. 122, 8255–8275. doi:10.1002/2017jd026561
Richardson, M., and Stephens, G. L. (2018). Information Content of OCO-2 Oxygen A-Band Channels for Retrieving marine Liquid Cloud Properties. Atmos. Meas. Tech. 11, 1515–1528. doi:10.5194/amt-11-1515-2018
Riedl, S., Harlander, M., Schlosser, C., Kolm, M., Maurer, R., Courrèges-Lacoste, G. B., et al. (2019). “Sentinel 4 UVN, a Geostationary Imaging UVN Spectrometer for Air Quality Monitoring: Performance, Measurement Modes and Model Philosophy,” in International Conference on Space Optics—ICSO 2018, Chania, Greece (Bellingham, WA: International Society for Optics and Photonics) 11180–11205. doi:10.1117/12.2535924
Rietjens, J., Campo, J., Chanumolu, A., Smit, M., Nalla, R., Fernandez, C., et al. (2019). “Expected Performance and Error Analysis for SPEXone, a Multi-Angle Channeled Spectropolarimeter for the NASA PACE mission,” in Polarization Science and Remote Sensing IX (International Society for Optics and Photonics), 11132–08. doi:10.1117/12.2530729
Rodgers, C. D. (2000). Inverse Methods for Atmospheric Sounding: Theory and Practice. Singapore: World Scientific.
Romanova, L., and Ustinov, Y. (1982). The Generalized Transfer Equation for the Distribution of Photon Paths and the Problem of Vertically Inhomogeneous Gas Absorption in the Atmosphere. Izv. Acad. Sci. Ussr, Atmos. Oceanic Phys. 18, 186–192.
Rozanov, V., and Kokhanovsky, A. (2004). Semi-analytical Cloud Retrieval Algorithm as Applied to the Cloud Top Altitude and the Cloud Geometrical Thickness Determination from Top-Of-Atmosphere Reflectance Measurements in the Oxygen A-Band. J. Geophys. Res. D109, 5202. doi:10.1029/2003jd004104
Saiedy, F., Hilleary, D. T., and Morgan, W. A. (1965). Cloud-Top Altitude Measurements from Satellites. Appl. Opt. 4, 495–500. doi:10.1364/ao.4.000495
Saiedy, F., Jacobowitz, H., and Wark, D. Q. (1967). On Cloud-Top Determination from Gemini-5. J. Atmos. Sci. 24, 63–69. doi:10.1175/1520-0469(1967)024<0063:octdfg>2.0.co;2
Skorinov, V., and Titov, G. (1984). Mean Photon Path Lengths for Broken Clouds. Izv. Acad. Sci. Ussr, Atmos. Oceanic Phys. 20, 377–381.
Stephens, G. L., and Heidinger, A. (2000). Molecular Line Absorption in a Scattering Atmosphere. Part I: Theory. J. Atmos. Sci. 57, 1599–1614. doi:10.1175/1520-0469(2000)057<1599:mlaias>2.0.co;2
Syachinov, V., and Kozlov, Y. (1974). Determination of Cloud-Top Altitude from the Cosmos-320 Satellite. Izv. Acad. Sci. Ussr, Atmos. Oceanic Phys. 10, 582–586.
Thyagarajan, K., Neumann, A., and Zimmermann, G. (1996). The IRS-P3 Remote Sensing Mission. Acta Astronautica 39, 711–716. doi:10.1016/s0094-5765(97)00053-2
Valero, F. P. J., Marshak, A., and Minnis, P. (2021). Lagrange Point Missions: The Key to Next Generation Integrated Earth Observations. DSCOVR Innovation. Front. Remote Sens. 2. doi:10.3389/frsen.2021.745938
Vanbauce, C., Buriez, J. C., Parol, F., Bonnel, B., Sèze, G., and Couvert, P. (1998). Apparent Pressure Derived from ADEOS-POLDER Observations in the Oxygen A-Band over Ocean. Geophys. Res. Lett. 25, 3159–3162. doi:10.1029/98gl02324
Veefkind, J. P., Aben, I., McMullan, K., Förster, H., De Vries, J., Otter, G., et al. (2012). TROPOMI on the ESA Sentinel-5 Precursor: A GMES Mission for Global Observations of the Atmospheric Composition for Climate, Air Quality and Ozone Layer Applications. Remote Sensing Environ. 120, 70–83. doi:10.1016/j.rse.2011.09.027
Wang, M. (1999). Validation Study of the SeaWiFS Oxygen A-Band Absorption Correction: Comparing the Retrieved Cloud Optical Thicknesses from SeaWiFS Measurements. Appl. Opt. 38, 937–944. doi:10.1364/ao.38.000937
Werdell, P. J., Behrenfeld, M. J., Bontempi, P. S., Boss, E., Cairns, B., Davis, G. T., et al. (2019). The Plankton, Aerosol, Cloud, Ocean Ecosystem mission: Status, Science, Advances. Bull. Am. Meteorol. Soc. 100, 1775–1794. doi:10.1175/bams-d-18-0056.1
Wu, M.-L. C. (1985). Remote Sensing of Cloud-Top Pressure Using Reflected Solar Radiation in the Oxygen A-Band. J. Clim. Appl. Meteorol. 24, 539–546. doi:10.1175/1520-0450(1985)024<0539:rsoctp>2.0.co;2
Yamamoto, G., and Wark, D. Q. (1961). Discussion of the Letter by R. A. Hanel, “Determination of Cloud Altitude from a Satellite”. J. Geophys. Res. 66, 3596. doi:10.1029/jz066i010p03596
Yang, Y., Marshak, A., Mao, J., Lyapustin, A., and Herman, J. (2013). A Method of Retrieving Cloud Top Height and Cloud Geometrical Thickness with Oxygen A and B Bands for the Deep Space Climate Observatory (DSCOVR) Mission: Radiative Transfer Simulations. J. Quantitative Spectrosc. Radiative Transfer 122, 141–149. doi:10.1016/j.jqsrt.2012.09.017
Keywords: DSCOVR, EPIC, oxygen A-band, oxygen B-band, clouds, pathlength distribution, pathlength moments
Citation: Davis AB, Yang Y and Marshak A (2022) EPIC/DSCOVR as a Pathfinder in Cloud Remote Sensing Using Differential Oxygen Absorption Spectroscopy. Front. Remote Sens. 3:796273. doi: 10.3389/frsen.2022.796273
Received: 16 October 2021; Accepted: 19 January 2022;
Published: 11 March 2022.
Edited by:
Yongxiang Hu, National Aeronautics and Space Administration (NASA), United StatesReviewed by:
Chao Liu, Nanjing University of Information Science and Technology, ChinaCopyright © 2022 Davis, Yang and Marshak. This is an open-access article distributed under the terms of the Creative Commons Attribution License (CC BY). The use, distribution or reproduction in other forums is permitted, provided the original author(s) and the copyright owner(s) are credited and that the original publication in this journal is cited, in accordance with accepted academic practice. No use, distribution or reproduction is permitted which does not comply with these terms.
*Correspondence: Anthony B. Davis, QW50aG9ueS5CLkRhdmlzQGpwbC5uYXNhLmdvdg==