- Department of Geological Oceanography, School of Oceanography, Rio de Janeiro State University, Rio de Janeiro, Brazil
Barrier islands are low-lying elongated, narrow sandy deposits, usually parallel to the coastline, separated from the continent by a lagoon. Due to their low elevation above sea level, barrier islands are environments susceptible to drastic morphological changes depending on the meteo-oceanographic conditions to which they are subjected. This work presents the morphological changes between 1985 and 2021 in “Restinga da Marambaia”—a 40 km long barrier island on Brazil’s Southeastern coast. One hundred thirty-four scenes from the Landsat collection were processed, enabling the quantification of the barrier island area. Additionally, the rates of change in the position of the shorelines facing the Atlantic Ocean, Sepetiba Bay, and Marambaia Bay were computed. The barrier island’s total area and the central sector’s width present significant seasonal variability, which is maximum during the austral fall and winter seasons. On the shores facing the Atlantic Ocean and Sepetiba Bay, it is noted that the central and far eastern sectors show an erosional trend. In contrast, the coastline is more stable on the shore facing Marambaia Bay. The seasonal variations of the barrier island area occur during a period of low rainfall and more energetic waves associated with local winds, which produce coastal currents, transporting the available sediments.
1 Introduction
Barrier islands are low-lying elongated, narrow sandy deposits, usually parallel to the coastline, separated from the continent by a lagoon (Kusky, 2005). Their formation and maintenance are related to the geological environment, sediment supply, sediment transport mechanism, wave and tidal regimes, and sea level behavior (Pilkey et al., 2009; Stutz and Pilkey, 2011; Otvos, 2012). Due to their low elevation above sea level, barrier islands are environments susceptible to drastic morphological changes depending on the meteo-oceanographic conditions to which they are subjected.
It is a central issue for coastal studies to comprehend and predict the morphological changes and the shoreline variability, as different temporal scales are involved (Turki et al., 2013; Hapke et al., 2016). Also, some uncertainties result from the short-scale natural variability and the mean sea level that are not easy to identify (Ruggiero et al., 2003; Lazarus et al., 2011).
Several tools are used in coastal studies, and remote sensing has been one of the most applied in the last decades (Zakaria et al., 2006; Batista et al., 2009; Garcia-Rubio et al., 2012; Sud et al., 2012; Cenci et al., 2015; Sánchez-García et al., 2015; Azevedo et al., 2016; Behling et al., 2018; Pardo-Pascual et al., 2018; Xu, 2018; Mitri et al., 2020). Landsat’s freely available images, spanning a few decades, make it possible to analyze the changing morphology and position of the coastline (Young et al., 2017). Additionally, satellite image processing tools have evolved considerably, especially in handling large volumes of images, improving performance, accuracy, and applicability (Gorelick et al., 2017; Obi Reddy and Singh, 2018).
Throughout the last decade, the 40 km long Marambaia barrier island has been investigated for its sedimentary dynamics and geological evolution (e.g., Borges and Nittrouer, 2016; Gomes et al., 2019; Carvalho and Guerra, 2020; Reis et al., 2020; Dadalto et al., 2022), with fewer studies quantifying the shoreline dynamics (Oliveira et al., 2008; Bahiense et al., 2014; Santos et al., 2019; Carvalho et al., 2020). Given the geomorphological importance of this barrier island and the emergency to understand the morphological behavior of coastal features due to scenarios of sea level rise (IPCC, 2022) and increased storminess (Young and Ribal, 2019; Rey et al., 2021), this work presents a contribution to the diagnostic of its morphological trends over 36 years (1985–2021), supported by Landsat imagery analysis. In contrast to the previous works, this study expands the time scale of the observation, and more images were processed. While Oliveira et al. (2008) and Bahiense et al. (2014) used, respectively, nine and five images, we used more than a hundred. Therefore, our results are robust and allow the detailed observation of the seasonal changes in shoreline position and their consequences in the barrier island area over time.
2 Methods
2.1 Study area
The 40 km long Marambaia barrier island is located on the southern coastline of Rio de Janeiro (SE Brazil), with an east-west orientation and width varying from 120 to 1800 m. In the westernmost limit, the barrier island is anchored at a pre-Cambrian massif, the Marambaia Peak. In the easternmost limit lie the tidal channels of Barra de Guaratiba (Figure 1). This barrier island may be divided into three sectors: 1) Western, including beach ridges, marshlands, inter-ridge paleo lagoons, and overland flow features; 2) Central, where the barrier island becomes strikingly narrow; and 3) Eastern, characterized by a dune field, tidal wetlands and beach ridges (Dadalto et al., 2022).
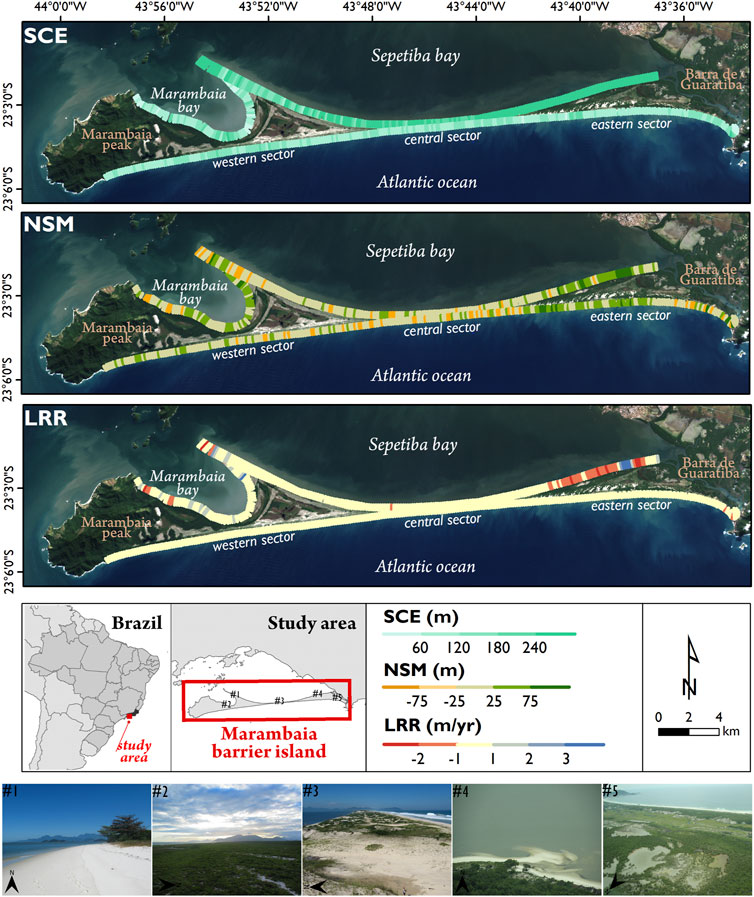
FIGURE 1. Marambaia barrier island location. SCE, shoreline change envelope; NSM, net shoreline movement; LRR, linear regression rate. Landsat 8 satellite imagery from 05/Jun/2021, 4R3G2B composition.
Based on the Köppen classification, Alvares et al. (2013) state that there are two types of climate in this region: tropical without dry season (Af) and tropical monsoon (Am), characterized by annual mean temperature between 22°C and 24°C and annual rainfall between 1,300 and 1,600 mm. The South Atlantic Subtropical Anticyclone (SASA) affects the area, which, in the face of frontal systems, causes increased cloud cover and strong winds (Dereczynski and Menezes, 2015).
The wave climate in the Rio de Janeiro littoral is characterized by fair-weather short-period waves from northeast and eastern directions and storm waves from S and SSW, with higher amplitudes and longer periods (Parente et al., 2015; Carvalho et al., 2021).
Marambaia barrier island partially isolates Sepetiba bay from the Atlantic Ocean, strongly influencing its circulation, which is affected by river discharge in its northern and eastern sectors (Fragoso, 1999). The coastal region is under a microtidal regime, with tide heights varying between 0.3 and 1.2 m (Criado-Sudau et al., 2019) and with tidal propagation from east to west (Harari and Camargo, 1994).
2.2 Landsat imagery
Landsat satellite imagery has been globally applied for environmental studies, including shoreline monitoring (Zakaria et al., 2006; Misra and Balaji, 2015; Ozturk et al., 2015; Konlechner et al., 2020; Sánchez-García et al., 2020; McAllister et al., 2022). These images are extensively used since they have global coverage and are freely distributed (Young et al., 2017). For this work, using the Google Earth Engine (GEE) platform (Gorelick et al., 2017), the TM, ETM+, and OLI sensors images were imported from the Landsat Tier 1 collection (Supplementary Figure S1) calibrated top-of-atmosphere (TOA) reflectance, encompassing the period between 1985 and 2021, with a cloud coverage of less than 10% of the scene. One hundred thirty-four scenes, with orbit/point 217/76, were used to map the Marambaia barrier island. The atmospheric correction was done using the Dark Object Subtraction (DOS) model (Chavez, 1988) to obtain surface reflectance. This model is widely used for mapping change detection, enabling reliable surface reflectance values (Kawakubo et al., 2011; Cui et al., 2014; Nazeer et al., 2014; Pacheco et al., 2015; Phan and Stive, 2022).
2.3 Shoreline detection and analysis
Shoreline delineation was performed on the GEE platform by applying the Normalized Difference Water Index (NDWI) (McFeeters, 1996) (Eq. 1), and the output rasters were converted to vector polygons.
In the Landsat 5 and 7 series, the green and near-infrared (NIR) bands correspond to bands 2 and 4, respectively, while in the Landsat 8 series, they represent bands 3 and 5, respectively.
Afterward, the quantification of the barrier island area and its central sector’s width were conducted in the QGIS 3.16 program. Marambaia peak was excluded from the computation of the barrier area. The width of the central sector was computed at a location close to photography #3, shown in Figure 1 (between coordinates 43° 44′31.43″ W, 23° 3′30.04″ S and 43° 44′30.66″ W, 23° 3′34.30″ S). With the computed values, it was possible to estimate the annual average and median barrier island area and central sector width, as well as their seasonality.
The rates of change in the position of the shorelines facing the Atlantic Ocean, Sepetiba Bay, and Marambaia Bay were calculated in the Digital Shoreline Analysis System (DSAS) program (Himmelstoss et al., 2021) for ArcMap™ 10.8. For that, the polygons were converted into polylines, representing the shorelines for each image. Five hundred and seventy-four transversal transects, equispaced 150 m, were used to compute the Shoreline Change Envelope (SCE), the Net Shoreline Movement (NSM), and the Linear Regression Rate (LRR) (Himmelstoss et al., 2021).
The SCE is obtained by calculating the largest distance among all shorelines on each transect, representing the total variation in shoreline position, and is not related to the dates of the images (Himmelstoss et al., 2021). Conversely, the NSM is the difference between the oldest and the most recent shoreline position in each transect. The LRR is obtained from a line of best fit, calculated using the least squares method, with all shoreline positions in each transect (Dolan et al., 1991), reflecting rates that indicate erosion, accretion, or stability of the coastline.
DSAS considers information on the uncertainty and horizontal accuracy of the shoreline mapping in the calculations of standard errors and confidence intervals (Ruggiero et al., 2013). In the case of using satellite imagery for determining shoreline position, these uncertainties consider data quality (pixel error, Ep), georeferencing error (Eg), high tide level uncertainty (Ev), and shoreline digitization error (Ed), compiled as a total error (Et) (Hapke et al., 2016; Nassar et al., 2019). For the mapping presented in this manuscript, the annualized Et was ±3.2 m/year, and the estimated uncertainty (UR) of the shoreline change rate was 0.2 m/year, with values similar to those reported by Carvalho et al. (2020), where the authors analyzed the Marambaia barrier island shoreline facing the Atlantic Ocean. So, when analyzing the values expressed as LRR, rates above 0.2 m/year indicate accretion, between −0.2 and 0.2 m/year indicate stability, and below −0.2 m/year indicate erosion.
3 Results
3.1 Barrier island area and central sector width variability
From 1985 to 2021, the barrier island area varied between 51.3 and 57.7 km2, averaging 53.3 ± 1.2 km2 (Figure 2A). The lowest average area was recorded in 1986 (52.1 ± 0.6 km2), while the largest was recorded in 1998 (55.0 ± 2.1 km2). The central sector width, one of the lowest regions of the barrier island, varied between 121.7 and 372.3 m, with an average of 168.4 ± 34.9 m (Figure 2B). The smallest average width was determined for 1986 (141.6 ± 16.7 m), while the largest was observed in 2020 (204.0 ± 60.2 m).
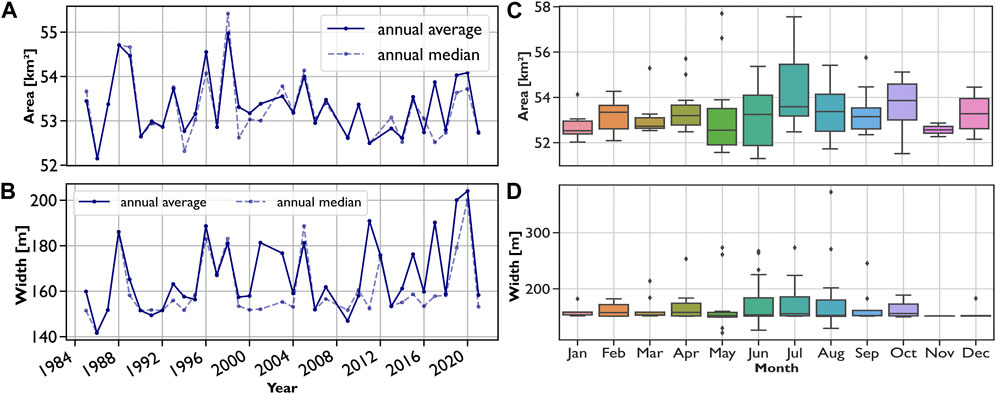
FIGURE 2. Marambaia barrier island variability (1985–2021): annual averages and medians of (A) total area and (B) central section width; and monthly variation of (C) total area and (D) central section width.
The barrier island’s total area and the central sector’s width presented significant seasonal variability, maximum between May and August, that corresponds to the austral fall and winter seasons (Figures 2C, D). November is the month when the barrier island presents the smallest average area (52.6 ± 0.4 km2), while the largest value occurred in July (54.3 ± 1.6 km2). About the width of the central area, the lowest monthly average was found in November (151.8 ± 0.2 m), whereas the highest monthly average was observed in August (178.4 ± 57.4 m).
3.2 Shoreline change metrics and rates (SCE, NSM, and LRR)
In Figure 1 are spatialized the shoreline change envelope (SCE), net shoreline movement (NSM), and the linear regression rate (LRR). Regarding the shoreline facing the open ocean, the beach envelope varied between 30 and 277 m (SCE), ranging from −62 m to +115 m (NSM), resulting in a rate of change between −1.1 and +1.0 m/year (LRR) (Figure 3A; Table 1). The eastern sector shows higher variability (maximum SCE of 277 m) and erosive tendency (maximum NSM retreat of −61.5 m and maximum LRR retreat of −1.1 m/year), representing 10% of the whole shoreline under erosion, especially near Barra de Guaratiba. The central area shows some stability (∼20% of the whole shoreline), since areas under erosion (26%) and under accretion (53%) alternate along this sector (panel NSM on Figure 1), culminating in average rates of −0.04 m/year. The western sector is the most stable, making up 34% of the shoreline with an average LRR of 0.09 m/year (Table 1).
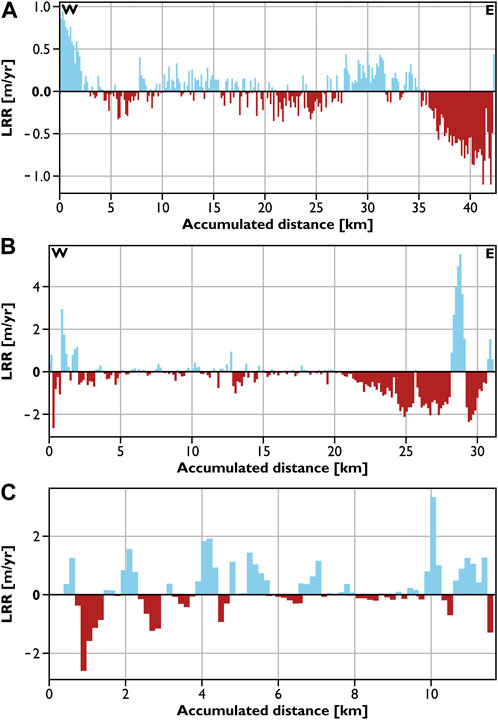
FIGURE 3. Shoreline change rates (1985–2021): (A) facing the open ocean; (B) back-barrier; (C) facing marambaia bay.
On the back-barrier shoreline, the beach envelope (SCE) varied between 95 and 894 m, ranging between −61 m and +185 m (NSM), showing a rate of change between −2.7 and +5.5 m/year (LRR) (Figure 3B; Table 1). Similarly to the coastline facing the open ocean, the eastern sector of the shoreline facing Sepetiba Bay exhibits the highest erosion rate (mean LRR of −0.6 m/year), comprising 31% of the backbarrier shoreline that is eroding. The central sector also shows erosional trends (mean LRR of −0.2 m/year), although most of this sector is stable (∼10% of the entire back-barrier coastline). The western sector is the most stable (mean LRR of 0 m/year), where almost 60% of this sector (∼6% of this shoreline) is stable (Table 1).
Finally, on the coastline facing Marambaia Bay, the beach envelope (SCE) oscillated between 0 and 320 m, ranging from −68 m to +88 m (NSM), with a rate of change between −2.6 and +3.3 m/year (LRR) (Figure 3C; Table 1). This shoreline is more stable (average NSM of 0.05 m) and exhibits the highest accretion rates on the barrier island (38%), with an average LRR of 0.2 m/year (Table 1).
4 Discussion
The Marambaia barrier island morphometric and shoreline behavior suggest that the intrannual variability is the primary driver of barrier island remodeling, intensified by interannual changes and geological control. As for the seasonal variations of the barrier island area, they occur during a period of low rainfall (Supplementary Figure S2), more energetic waves (Parente et al., 2015; Carvalho et al., 2020), and most significant mean sea level variation (Carvalho et al., 2023). This combination is conducive to sediment transport conditions that favor the maintenance of overwash zones observed in the barrier island (Photo #3 in Figure 1).
In general, the back-barrier shore presented a broader envelope of shoreline change compared to the shores facing Marambaia Bay and the open ocean. In this region are observed striking rhythmic features, classified as elongated transverse finger bars (Gomes et al., 2019). The highest average number of NE-SW oriented bars, determined by satellite imagery, occurs in August, reaching 11 bars/km (Gomes et al., 2019). Thus, it is suggested that these features influence shoreline variability. Ashton and Murray (2006); Murray et al. (2014) associated these rhythmic patterns with sediment erosion and accretion on the coastline.
The eastern sector of the back-barrier shore presents the highest shoreline change envelope (SCE >240 m) determined in the present study. This might be a consequence of its proximity to the mouth of rivers debouching into the bay and intermittent channels that drain wetlands (Photo #5 in Figure 1) present in the barrier (Dadalto et al., 2022). For example, the Piraquê river, near the eastern sector, is one of the main tributaries of Sepetiba bay, with an average discharge of 2.5 m3/s (Cunha et al., 2006). Furthermore, in the satellite images and aerial photographs is possible to observe the presence of intermittent channels that induce the formation of spur-like features (Photo #4 in Figure 1) of variable sizes.
Although the coastline is more stable along the Marambaia Bay shore, there is an erosional trend in its southwestern sector and a prograding trend in the northeastern sector (Photo #1 in Figure 1). These sediment transport trends are evidenced by morphological features and grain size trend analysis (Carvalho and Guerra, 2020). On the open-ocean shore, the Barra de Guaratiba tidal channels influence the erosional trend observed in the far eastern sector, which had been previously noted (Carvalho et al., 2020).
Regarding the width of the central sector of the barrier island, Oliveira et al. (2008), using Landsat and CBERS satellite images from 1975 to 2004, documented a variation from 158 m (in 1975) to 100 m (in 2004). In our study, which encompasses a larger temporal scale, the width increased from 151 m to 180 m. Also, between 1984 and 2004, Oliveira et al. (2008) documented a reduction of 58 m in the central sector width, while we observed a slight increase (+29 m). Using aerial photographs and GeoEye satellite images, Bahiense et al. (2014) verified that between 1975 and 2011, there was an alternation of areas of accretion and erosion on both sides of the barrier island’s central sector, with rates ranging between −0.30 and 0.15 m/yr.
The seasonality of shoreline change is a common trend observed in other sandy shorelines studied in other parts of the world. Still, the reasons for this seasonality differ regionally. For example, Bishop-Taylor et al. (2021) found that 16% of Australia’s shoreline retreated or progressed at rates greater than 0.5 m/year, indicating that these may be extreme coastal change hot spots. On the Calabrian coast (southern Italy), Foti et al. (2022) studied the evolution of the coastline at different time scales. They noted that eroding areas prevailed over accreting ones when analyzed over long and medium-term time scales, while accretion prevailed over short-term time scales. Therefore, the authors emphasize the importance of jointly analyzing human pressures and natural processes to understand shoreline dynamics. In this regard, Bamunawala et al. (2021), in assessing projections of worldwide shoreline changes near tidal inlets, emphasized that several impacts of climate change can severely modify the morphological dynamics of the shoreline. They mention, for instance, changes in mean sea level and suppression of sediment supply in coastal areas. Thus, it is apparent the importance of understanding the processes subjacent to the changes in the position of the coastline in its different temporal and spatial scales, taking into account the natural and anthropic influences.
Despite the geomorphological complexity observed on the Marambaia barrier island, using Landsat satellite images enabled us to quantify the morphological changes over the last 35 years. Remote sensing is a powerful tool in places of difficult access, such as the study area, associated with the scarcity of financial and human resources to monitor the coastline. The observed trends demonstrated the importance of the seasonality of coastal processes, reinforcing the need to fully understand these systems to cope with the changes they will undergo in scenarios of sea level rise and an increased number of storm events.
Data availability statement
The raw data supporting the conclusion of this article will be made available by the authors, without undue reservation.
Author contributions
BC designed the study, prepared and processed the satellite images and made the statistical analysis. BC and CG analyzed and interpreted the data. All authors were involved in writing or revising the manuscript.
Funding
Fundação de Amparo à Pesquisa do Estado do Rio de Janeiro (FAPERJ) financial support [E-26/010.002642/2014 and E-26/010.002208/2019].
Acknowledgments
The authors thank Fundação de Amparo à Pesquisa do Estado do Rio de Janeiro (FAPERJ) for the financial support for this research and the colleagues and institutions that supported the authors’ efforts over the last decade. They also thank the reviewers for their comments and suggestions, which helped improve this manuscript. To the editors of Women in Remote Sensing: 2022 and Rebecca Thompson, journal specialist, our thanks for their administrative efforts on this manuscript.
Conflict of interest
The authors declare that the research was conducted in the absence of any commercial or financial relationships that could be construed as a potential conflict of interest.
Publisher’s note
All claims expressed in this article are solely those of the authors and do not necessarily represent those of their affiliated organizations, or those of the publisher, the editors and the reviewers. Any product that may be evaluated in this article, or claim that may be made by its manufacturer, is not guaranteed or endorsed by the publisher.
Supplementary material
The Supplementary Material for this article can be found online at: https://www.frontiersin.org/articles/10.3389/frsen.2023.1111696/full#supplementary-material
SUPPLEMENTARY FIGURE S1 | Temporal distribution of landsat imagery (DOY: Day Of Year).
SUPPLEMENTARY FIGURE S2 | Monthly averaged air temperature and accumulated precipitation from Marambaia metereological station (2002 to 2021), located at 43° 36ʹ W and 23° 03ʹ S. Data provided by the National Institute of Meteorology (INMET).
References
Alvares, C. A., Stape, J. L., Sentelhas, P. C., Gonçalves, J. L., de, M., and Sparovek, G. (2013). Köppen's climate classification map for Brazil. Zeitschrift 22, 711–728. doi:10.1127/0941-2948/2013/0507
Ashton, A. D., and Murray, A. B. (2006). High-angle wave instability and emergent shoreline shapes: 2. Wave climate analysis and comparisons to nature. J. Geophys. Res. Earth Surf. 111, F04012–F04017. doi:10.1029/2005jf000423
Azevedo, I. F. D., Carvalho, B. C., and Guerra, J. V. (2016). Utilização de imagens de satélite LANDSAT para análise da variabilidade morfológica de pontais arenosos na planície costeira de Caravelas (NE do Brasil). Rev. Bras. Geomorfol. 17, 695–709. doi:10.20502/rbg.v17i4.843
Bahiense, F., Pereira, S. D., Geraldes, M. C., and Menezes, G. (2014). “Emprego de análise multitemporal de fotografias aéreas na evolução geomorfológica da restinga da Marambaia, Rio de Janeiro – brasil,” in Formação e Ocupação de Litorais nas Margens do Atlântico - brasil/Portugal Rio de Janeiro: Corbã, 33–52.
Bamunawala, J., Ranasinghe, R., Dastgheib, A., Nicholls, R. J., Murray, A. B., Barnard, P. L., et al. (2021). Twenty-first-century projections of shoreline change along inlet-interrupted coastlines. Sci. Rep. 11, 14038. doi:10.1038/s41598-021-93221-9
Batista, E. D. M., Souza Filho, P. W. M., and da Silveira, O. F. M. (2009). Avaliação de áreas deposicionais e erosivas em cabos lamosos da zona costeira Amazônica através da análise multitemporal de imagens de sensores remotos. Rev. Bras. Geofis. 27, 83–96.
Behling, R., Milewski, R., and Chabrillat, S. (2018). Spatiotemporal shoreline dynamics of Namibian coastal lagoons derived by a dense remote sensing time series approach. Int. J. Appl. Earth Obs. Geoinf. 68, 262–271. doi:10.1016/j.jag.2018.01.009
Bishop-Taylor, R., Nanson, R., Sagar, S., and Lymburner, L. (2021). Mapping Australia’s dynamic coastline at mean sea level using three decades of Landsat imagery. Remote Sens. Environ. 267, 112734. doi:10.1016/j.rse.2021.112734
Borges, H. V., and Nittrouer, C. A. (2016). Sediment accumulation in Sepetiba bay (Brazil) during the holocene: A reflex of the human influence. J. Sediment. Environ. 1, 90–106. doi:10.12957/jse.2016.21868
Carvalho, B. C., Araujo, T. A. A., Guerra, J. V., and Reis, A. T. D. (2023). “Mean sea level trends based on tide gauge records and their possible morphological effects on the coastline of southern Rio de Janeiro (SE Brazil),” in Appl. Geogr. Submitted.
Carvalho, B. C., Dalbosco, A. L. P., and Guerra, J. V. (2020). Shoreline position change and the relationship to annual and interannual meteo-oceanographic conditions in Southeastern Brazil. Estuar. Coast. Shelf Sci. 235, 106582. doi:10.1016/j.ecss.2020.106582
Carvalho, B. C., and Guerra, J. V. (2020). Aplicação de Modelo de Tendência Direcional de Transporte ao Longo de uma Ilha-Barreira: Restinga da Marambaia (RJ, SE Brasil). Anuário do Inst. Geociências - UFRJ 43, 101–118. doi:10.11137/2020_2_101_118
Carvalho, B. C., Lins-de-Barros, F. M., Silva, P., Pena, J. D. N., and Guerra, J. V. (2021). Morphological variability of sandy beaches due to variable oceanographic conditions: A study case of oceanic beaches of Rio de Janeiro city (Brazil). J. Coast. Conserv. 25, 28. doi:10.1007/s11852-021-00821-8
Cenci, L., Persichillo, M. G., Disperati, L., Oliveira, E. R., Alves, F. L., Pulvirenti, L., et al. (2015). “Remote sensing for coastal risk reduction purposes: Optical and microwave data fusion for shoreline evolution monitoring and modelling,” in 2015 IEEE international geoscience and remote sensing symposium (IGARSS), 1417–1420. doi:10.1109/IGARSS.2015.7326043
Chavez, P. S. (1988). An improved dark-object subtraction technique for atmospheric scattering correction of multispectral data. Remote Sens. Environ. 24, 459–479. doi:10.1016/0034-4257(88)90019-3
Criado-Sudau, F. F., Nemes, D. D., and Gallo, M. N. (2019). Rip currents dynamic of a swell dominated microtidal beach. J. Coast. Res., 121–127. doi:10.2112/SI92-014.1
Cui, L., Li, G., Ren, H., He, L., Liao, H., Ouyang, N., et al. (2014). Assessment of atmospheric correction methods for historical landsat TM images in the coastal zone: A case study in jiangsu, China. China. Eur. J. Remote Sens. 47, 701–716. doi:10.5721/eujrs20144740
Cunha, C. D. L. D. N., Rosman, P. C. C., Ferreira, A. P., and Carlos do Nascimento Monteiro, T. (2006). Hydrodynamics and water quality models applied to Sepetiba Bay. Cont. Shelf Res. 26, 1940–1953. doi:10.1016/j.csr.2006.06.010
Dadalto, T. P., Carvalho, B. C., Guerra, J. V., Reis, A. T. D., and Silva, C. G. (2022). Holocene morpho-sedimentary evolution of Marambaia barrier island (SE Brazil). Quat. Res. 105, 182–200. doi:10.1017/qua.2021.43
Dereczynski, C. P., and Menezes, W. F. (2015). “Meteorologia da Bacia de Campos,” in Meteorologia e Oceanografia. Editors R. P. Martins, and G. S. Grossmann-Matheson (Rio de Janeiro: Elsevier Editora Ltda), 1–54. doi:10.1016/B978-85-352-6208-7.50008-8
Dolan, R., Fenster, M. S., and Holme, S. J. (1991). Temporal analysis of shoreline recession and accretion. J. Coast. Res. 7, 723–744. doi:10.2307/4297888
Foti, G., Barbaro, G., Barillà, G. C., Mancuso, P., and Puntorieri, P. (2022). Shoreline evolutionary trends along calabrian coasts: Causes and classification. Front. Mar. Sci. 9. doi:10.3389/fmars.2022.846914
Fragoso, M. R. (1999). Estudo numérico da circulação marinha da região das baías de Sepetiba e Ilha Grande (RJ).
Garcia-Rubio, G., Huntley, D., and Russell, P. (2012). “Assessing shoreline change using satellite-derived shorelines in Progreso, Yucatán, México,” in Coastal engineering proceedings (santander), 1–13.
Gomes, C. L., da, S., Guerra, J. V., and Gallo, M. N. (2019). “Mapeamento de bancos transversais (tipo “finger bars”) na margem norte da restinga da Marambaia (Baía de Sepetiba, RJ),” in Anais do XIX simpósio brasileiro de Sensoriamento remoto, 96716. Available at: https://proceedings.science/sbsr-2019/papers/mapeamento-de-bancos-transversais–tipo–--finger-bars–-–na-margem-norte-da-restinga-da-marambaia–baia-de-sepetiba–r.
Gorelick, N., Hancher, M., Dixon, M., Ilyushchenko, S., Thau, D., and Moore, R. (2017). Google Earth engine: Planetary-scale geospatial analysis for everyone. Remote Sens. Environ. 202, 18–27. doi:10.1016/j.rse.2017.06.031
Hapke, C. J., Plant, N. G., Henderson, R. E., Schwab, W. C., and Nelson, T. R. (2016). Decoupling processes and scales of shoreline morphodynamics. Mar. Geol. 381, 42–53. doi:10.1016/j.margeo.2016.08.008
Harari, J., and Camargo, R. D. (1994). Simulação da propagação das nove principais componentes de maré na plataforma sudeste brasileira através de modelo numérico hidrodinâmico. Braz. J. Oceanogr. 42, 35–54. doi:10.1590/s1679-87591994000100003
Himmelstoss, E., Henderson, R., Kratzmann, M., and Farris, A. (2021). Digital shoreline analysis system (DSAS) version 5.1 user guide. doi:10.3133/ofr20211091
Intergovernmental Panel on Climate Change (IPCC) (2022). “sea level rise and implications for low-lying islands, coasts and communities,” in The Ocean and cryosphere in a changing climate (Cambridge University Press), 321–446. doi:10.1017/9781009157964.006
Kawakubo, F. S., Morato, R. G., Nader, R. S., and Luchiari, A. (2011). Mapping changes in coastline geomorphic features using landsat TM and ETM+ imagery: Examples in southeastern Brazil. Int. J. Remote Sens. 32, 2547–2562. doi:10.1080/01431161003698419
Konlechner, T. M., Kennedy, D. M., O’Grady, J. J., Leach, C., Ranasinghe, R., Carvalho, R. C., et al. (2020). Mapping spatial variability in shoreline change hotspots from satellite data; a case study in southeast Australia. Estuar. Coast. Shelf Sci. 246, 107018. doi:10.1016/j.ecss.2020.107018
Lazarus, E., Ashton, A., Tebbens, S., Murray, A. B., Tebbens, S., Burroughs, S., et al. (2011). Cumulative versus transient shoreline change: Dependencies on temporal and spatial scale. J. Geophys. Res. Earth Surf. 116, 1–10. doi:10.1029/2010jf001835
McAllister, E., Payo, A., Novellino, A., Dolphin, T., and Medina-Lopez, E. (2022). Multispectral satellite imagery and machine learning for the extraction of shoreline indicators. Coast. Eng. 174, 104102. doi:10.1016/j.coastaleng.2022.104102
McFeeters, S. K. (1996). The use of the Normalized Difference Water Index (NDWI) in the delineation of open water features. Int. J. Remote Sens. 17, 1425–1432.
Misra, A., and Balaji, R. (2015). A study on the shoreline changes and Land-use/land-cover along the south Gujarat coastline. Procedia Eng. 116, 381–389. doi:10.1016/j.proeng.2015.08.311
Mitri, G., Nader, M., Abou Dagher, M., and Gebrael, K. (2020). Investigating the performance of sentinel-2A and Landsat 8 imagery in mapping shoreline changes. J. Coast. Conserv. 24, 40. doi:10.1007/s11852-020-00758-4
Murray, A. B., Coco, G., and Goldstein, E. B. (2014). Cause and effect in geomorphic systems: Complex systems perspectives. Geomorphology 214, 1–9. doi:10.1016/j.geomorph.2014.03.001
Nassar, K., Mahmod, W. E., Fath, H., Masria, A., Nadaoka, K., and Negm, A. (2019). Shoreline change detection using DSAS technique: Case of North Sinai coast, Egypt. Mar. Georesources Geotechnol. 37, 81–95. doi:10.1080/1064119x.2018.1448912
Nazeer, M., Nichol, J. E., and Yung, Y.-K. (2014). Evaluation of atmospheric correction models and Landsat surface reflectance product in an urban coastal environment. Int. J. Remote Sens. 35, 6271–6291. doi:10.1080/01431161.2014.951742
Obi Reddy, G. P., and Singh, S. K. (2018). Geospatial technologies in land resources mapping, monitoring and management. 1st ed. New York: Springer International Publishing.
Oliveira, F. S. C., Kampel, M., and Amaral, S. (2008). Multitemporal assessment of the geomorphologic evolution of the restinga of Marambaia, Rio de Janeiro, Brazil. Int. J. Remote Sens. 29, 5585–5594. doi:10.1080/01431160802061696
Otvos, E. G. (2012). Coastal barriers - Nomenclature, processes, and classification issues. Geomorphology 139–140, 39–52. doi:10.1016/j.geomorph.2011.10.037
Ozturk, D., Beyazit, I., and Kilic, F. (2015). Spatiotemporal analysis of shoreline changes of the kizilirmak delta. J. Coast. Res. 31, 1389–1402. doi:10.2112/jcoastres-d-14-00159.1
Pacheco, A., Horta, J., Loureiro, C., and Ferreira, (2015). Retrieval of nearshore bathymetry from landsat 8 images: A tool for coastal monitoring in shallow waters. Remote Sens. Environ. 159, 102–116. doi:10.1016/j.rse.2014.12.004
Pardo-Pascual, J., Sánchez-García, E., Almonacid-Caballer, J., Palomar-Vázquez, J., Priego de los Santos, E., Fernández-Sarría, A., et al. (2018). Assessing the accuracy of automatically extracted shorelines on microtidal beaches from landsat 7, landsat 8 and sentinel-2 imagery. Remote Sens. 10, 326. doi:10.3390/rs10020326
Parente, C. E., Nogueira, I. C. M., and Ribeiro, E. O. (2015). “Climatologia de ondas,” in Meteorologia e Oceanografia. Editors R. P. Martins, and G. S. Grossmann-Matheson (Rio de Janeiro: Elsevier Editora Ltda.), 55–96. doi:10.1016/B978-85-352-6208-7.50009-X:
Phan, M. H., and Stive, M. J. F. (2022). Managing mangroves and coastal land cover in the Mekong Delta. Ocean. Coast. Manag. 219, 106013. doi:10.1016/j.ocecoaman.2021.106013
Pilkey, O. H., Cooper, J. A. G., and Lewisa, D. (2009). Global distribution and geomorphology of fetch-limited barrier islands. J. Coast. Res. 254, 819–837. doi:10.2112/08-1023.1
Reis, A. T. D., Amendola, G., Dadalto, T. P., Silva, C. G., Tardin Poço, R., Guerra, J. V., et al. (2020). Arquitetura e evolução deposicional da sucessão sedimentar pleistoceno tardio-holoceno (Últimos ∼20 Ka) da baía de sepetiba (RJ). Geosci. Geociências 39, 695–708. doi:10.5016/geociencias.v39i03.14366
Rey, W., Ruiz-Salcines, P., Salles, P., Urbano-Latorre, C. P., Escobar-Olaya, G., Osorio, A. F., et al. (2021). Hurricane flood hazard assessment for the archipelago of san andres, providencia and santa catalina, Colombia. Front. Mar. Sci. 8. doi:10.3389/fmars.2021.766258
Ruggiero, P., Kaminsky, G. M., Gelfenbaum, G., Ruggierof, P., Kaminskyt, G. M., and Gelfenbaumf, G. (2003). Linking proxy-based and datum-based shorelines on a high-energy coastline: Implications for shoreline change analyses. J. Coast. Res., 57–82.
Ruggiero, P., Kratzmann, M. G., Himmelstoss, E. A., Reid, D., Allan, J., and Kaminsky, G. (2013). National assessment of shoreline change — historical shoreline change along the pacific northwest coast: U.S. Geological survey open-file report 2012–1007. Available at: https://pubs.usgs.gov/of/2012/1007/.
Sánchez-García, E., Palomar-Vázquez, J. M., Pardo-Pascual, J. E., Almonacid-Caballer, J., Cabezas-Rabadán, C., and Gómez-Pujol, L. (2020). An efficient protocol for accurate and massive shoreline definition from mid-resolution satellite imagery. Coast. Eng. 160, 103732. doi:10.1016/j.coastaleng.2020.103732
Sánchez-García, E., Pardo-Pascual, J. E., Balaguer-Beser, A., and Almonacid-Caballer, J. (2015). “Analysis of the shoreline position extracted from landsat TM and ETM+ imagery,” in International archives of the photogrammetry, remote sensing and spatial information sciences - ISPRS archives (Berlin: International Society of Photogrammetry and Remote Sensing), 991–998. doi:10.5194/isprsarchives-XL-7-W3-991-2015
Santos, C., da, C. S., Dias, F. F., Franz, B., Alves dos Santos, P. R., Rodrigues, F., et al. (2019). Relative sea level rise effects at the Marambaia barrier island and Guaratiba mangrove: Sepetiba bay (SE Brazil). J. Sediment. Environ. 4, 249–262. doi:10.12957/jse.2019.44397
Stutz, M. L., and Pilkey, O. H. (2011). Open-Ocean barrier islands: Global influence of climatic, oceanographic, and depositional settings. J. Coast. Res. 27, 207–222. doi:10.2112/09-1190.1
Sud, B., Tohannic, C. D., Ensibs, B., and Cedex, V. (2012). “Automated mapping of coastline from high resolution satellite images using supervised segmentation,” in 4th GEOBIA, 515–517.
Turki, I., Medina, R., Gonzalez, M., and Coco, G. (2013). Natural variability of shoreline position: Observations at three pocket beaches. Mar. Geol. 338, 76–89. doi:10.1016/j.margeo.2012.10.007
Xu, N. (2018). Detecting coastline change with all available landsat data over 1986-2015: A case study for the state of Texas, USA. Atmos. (Basel) 9. doi:10.3390/atmos9030107
Young, I. R., and Ribal, A. (2019). Multiplatform evaluation of global trends in wind speed and wave height. Science 364, eaav9527. doi:10.1126/science.aav9527
Young, N. E., Anderson, R. S., Chignell, S. M., Vorster, A. G., Lawrence, R., and Evangelista, P. H. (2017). A survival guide to Landsat preprocessing. Ecology 98, 920–932. doi:10.1002/ecy.1730
Keywords: coastal dynamics, interannual changes, intra-annual variability, coastal geomorphology, remote sensing, shoreline variability, Marambaia barrier island
Citation: Carvalho BC, Gomes CLdS and Guerra JV (2023) Spatio-temporal morphological variability of a tropical barrier island derived from the Landsat collection. Front. Remote Sens. 4:1111696. doi: 10.3389/frsen.2023.1111696
Received: 30 November 2022; Accepted: 27 March 2023;
Published: 12 April 2023.
Edited by:
Hannah Victoria Herrero, The University of Tennessee, United StatesReviewed by:
Chen Wang, Ministry of Ecology and Environment, ChinaMayra Roman-Rivera, The University of Tennessee, United States
Copyright © 2023 Carvalho, Gomes and Guerra. This is an open-access article distributed under the terms of the Creative Commons Attribution License (CC BY). The use, distribution or reproduction in other forums is permitted, provided the original author(s) and the copyright owner(s) are credited and that the original publication in this journal is cited, in accordance with accepted academic practice. No use, distribution or reproduction is permitted which does not comply with these terms.
*Correspondence: Breylla Campos Carvalho, b.carvalho@alumni.usp.br
†Present address: Breylla Campos Carvalho, Center of Marine Biology, University of São Paulo, São Sebastião, Brazil