- 1Department of Soils and Agri-Food Engineering, Laval University, Quebec, QC, Canada
- 2Centre Eau-Terre-Environnement, Institut National de Recherche Scientifique, Quebec, QC, Canada
- 3Department of Civil, Environmental and Architectural Engineering, University of Padova, Padua, Italy
It is increasingly recognized that water scarcity, rather than a lack of arable land, will be the major constraint to increase agricultural production over the next few decades. Therefore, water represents a unique agricultural asset to drive agricultural sustainability. However, its planning, management and usage are often influenced by a mix of interdependent economic, engineering, social, hydrologic, environmental, and even political factors. Such a complex interdependency suggests that a sociotechnical approach to water resources management, a subject of the field of Hydroinformatics, represents a viable path forward to achieve sustainable agriculture. Thus, this paper presents an overview of the intersection between hydroinformatics and agriculture to introduce a new research field called agricultural hydroinformatics. In addition, it proposes a general conceptual framework taking into account the distinctive features associated with the sociotechnical dimension of hydroinformatics when applied in agriculture. The framework is designed to serve as a stepping-stone to achieve, not only integrated water resources management, but also agricultural sustainability transitions in general. Using examples from agricultural water development to horticultural and livestock farming, the paper highlights facets of the framework applicability as a new paradigm on data flows/sources consideration, and information and simulation models engineering as well as integration for a holistic approach to water resources management in agriculture. Finally, it discusses opportunities and challenges associated with the implementation of agricultural hydroinformatics and the development of new research areas needed to achieve the full potential of this emerging framework. These areas include, for example, sensor deployment and development, signal processing, information modeling and storage, artificial intelligence, and new kind of simulation model development approaches.
1. Introduction
The ever-growing world population and the rapidly approaching planetary boundaries have led to widespread discussion and consensus on the urgent need for the field of agriculture to gear toward sustainability-oriented initiatives (Giovannucci et al., 2012; Herrero and Thornton, 2013; FAO, 2017; Pretty et al., 2018). The objective is to reduce, avoid or redress environmental impacts and lessen the intense competition for input resources such as land, water, energy, and nutrients caused by conventional practices (Garnett et al., 2013; Jägermeyr et al., 2016; Kamilaris et al., 2017; Pretty et al., 2018; Calicioglu et al., 2019). Consequently, a variety of approaches (e.g., “ecological intensification,” “agroecological intensification,” and “sustainable intensification”) and technologies (e.g., “Precision Agriculture”) promoting transitions to sustainable practices have emerged. We argue that all these concepts can be summarized in the following common denominator: “a blend of technological, social, and institutional innovations at different scales around a common agricultural asset, resource, or subsystem represents a pivot to underpin sustainability transitions in agriculture.”
Sustainability transitions is a social and technical (sociotechnical) concept defined as a set of “long-term, multi-dimensional, and fundamental transformation processes through which [well]-established [organizations] shift to more sustainable modes of production and consumption” (Markard et al., 2012). This definition implies that the application of the concept in agriculture presents some serious challenges. A first challenge is the need for a genuine participation and interaction of both established and innovative stakeholders or organizations. Second, within and between (established) organizations, the interactions/relations between individuals and (agricultural) technologies and tools existing at a certain level often differ considerably from those employed at other levels. In other words, established stakeholders and organizations are often sociotechnically stratified (Abbott, 1999; Ingram, 2015). Third, the identification and contribution of all other concerned stakeholders are required to ensure that both social and non-social expectations are met (Cunge and Erlich, 1999; Rousseau et al., 2005; Abbott and Vojinovic, 2010a,b, 2014). Last but not least, it is a multi-disciplinary exercise involving a comprehensive knowledge of the different subsystems and subdomains in agriculture (Killham, 2011; Zomorodian et al., 2018; Al-Jawad et al., 2019). This challenge, in turn, leads to the first challenge mentioned above. Thus, the implementation of the sustainability transitions concept in agriculture can be thought as a continuous, and even iterative and circular, process of engaging all concerned stakeholders around the same common agricultural asset, resource, or subsystem discussed above.
Water is a unique agricultural input that connects, is affected by (quantitatively and qualitatively) and affects all agricultural subsystems (Zhu et al., 2019). Its use and management are often influenced by a mix of highly complex economic, engineering, social, hydrologic, environmental, and even political factors (Zomorodian et al., 2018; Bjornlund and Bjornlund, 2019). These influences are expected to become more challenging to cope with in light of the anticipated increase in agricultural water demand due to climate change impacts and global population growth. Furthermore, the conflicting behavior, preferences, and goals of water stakeholders further complicate the management of available water resources (Walker et al., 2015; Zomorodian et al., 2018). However, despite the challenges imposed by the water stakeholders ecosystem, roughly half of the total agricultural water use is lost somewhere between the point of abstraction and the crop, and thus, not effectively used (Knox et al., 2012). In this same vein, water scarcity, rather than a lack of arable land, will be the major constraint to increase agricultural production over the next few decades (Watkins, 2006; Hanjra and Qureshi, 2010). Therefore, water represents the abovementioned common agricultural asset/resource to underpin agricultural sustainability transitions. It is unarguably the most critical resource to achieve sustainable agriculture (Chartzoulakis and Bertaki, 2015).
Sustainable agriculture is often seen as an umbrella term for knowledge-intensive systems that require, beyond the application of domain knowledge, a new kind of knowledge (El Bilali and Allahyari, 2018). This latter is the knowledge of how to apply domain knowledge in new or unprecedented ways, i.e., a metaknowledge. In a context of using water resources as pivot to achieve agricultural sustainability, the common denominator we identified above, this metaknowledge is known as Hydroinformatics (Abbott, 1999). This discipline has emerged to deliver unprecedented results in situations where stakeholders engagement/mobilization around water resources planning and management are most needed, and environmental stakes are high. A landmark example of the significance of this discipline in mobilizing stakeholders is the social breakthrough achieved by applying hydroinformatics tools from design to finish of the bridge and tunnel connection between Denmark and Sweden (the Oresund Project; Thorkilsen and Dynesen, 2001). In this project, hydroinformatics, through the use of technologies of persuasion to communicate complex decision problems, was significantly successful in adequately informing politicians, responsible authorities, and ordinary citizens about the project, empowering, and persuading them as well to act as trusted stakeholders. Another related example has been introduced by Abdullaev and Rakhmatullaev (2014) to demonstrate the practical application of hydroinformatics in supporting the implementation of the concept of “Integrated Water Resources Management” (IWRM; GWP, 2000; Furlong et al., 2015) across diverse institutional settings in Central Asia.
Several efficiency-based techniques and approaches aligned with the knowledge management function and the sociotechnical dimension of hydroinformatics have been proposed and applied to address the problem of agricultural water resources sustainability. These include: the IWRM concept (GWP, 2000; Furlong et al., 2015); the conjunctive use of surface water and groundwater resources (Singh, 2014); novel irrigation management systems (Sandler et al., 2004; Evans and Sadler, 2008; Gumiere et al., 2014; Pelletier et al., 2015; Vanderleest et al., 2016; Caron et al., 2017; Bjornlund and Bjornlund, 2019); and minimization of food waste and losses (Kummu et al., 2012; Pretty et al., 2018; Bjornlund and Bjornlund, 2019; among others). An efficiency-promoting paradigm with a focus on water management includes Precision Irrigation (PI; Perea et al., 2018) for crops and aquaculture, and Precision Livestock Farming (PLF) for dairy, egg, and meat production (Fournel et al., 2017). PI and PLF have emerged to make intensive use of information and communication technologies for spatio-temporal data collection and processing in support of improved farm productivity, and cost-effectiveness, sustainability of operations and environmental quality. They entail that farms (livestock, crops, aquaculture, etc.) are continuously monitored, and the collected data mined using integrated modeling approaches (e.g., water application models, biophysical simulation models, and empirical models) to make decisions and ensure favorable production conditions (Fournel et al., 2017; Perea et al., 2018). Using water resources as a pivot, these knowledge-intensive techniques represent an application for the data-mining-for-knowledge-discovery line of development of hydroinformatics (Abbott, 1999). Thus, with its (meta)knowledge-producing and management function, hydroinformatics represents a viable path forward to achieve smart, i.e., sustainable agriculture (Allahyari, 2009).
Although water resources productivity or efficiency represents a cornerstone to achieve agricultural sustainability, it is not a panacea (Wichelns, 2014; Pretty et al., 2018). In this regard, substitution-based approaches consisting mainly of changes in consumption behaviors have been proposed (van Huis and Oonincx, 2017; Pretty et al., 2018; Bjornlund and Bjornlund, 2019). These approaches require the application of the technologies of persuasion, a subset of hydroinformatics' technologies, to change individuals' inner world and achieve behavioral changes (Abbott, 1999; Thorkilsen and Dynesen, 2001). This suggests that the inherent quantitative way of working of hydroinformatics must be complemented by its qualitative way of working (Vojinovic and Abbott, 2017). Thereby, hydroinformatics represents a prospective enabler of stakeholders engagement to achieve sustainable agriculture. It is exactly this persuasive knowledge-management purpose that Abbott (1999) had anticipated, and hence, must be fulfilled by hydroinformatics in order to realize its full sociotechnical potential, particularly in sustainable agriculture (DiSalvo et al., 2010; El Bilali and Allahyari, 2018).
This non-exhaustive overview of the interplay between agriculture and hydroinformatics highlights the anticipated role and relevance of the discipline proposed herein. This paper, thus, introduces agricultural hydroinformatics as a new interdisciplinary field of research. In addition, it proposes a general conceptual framework taking into account the distinctive features associated with the sociotechnical dimension of hydroinformatics when applied in agriculture. It exemplifies the quantitative and qualitative interdependencies necessary to support the development of sociotechnical environments in which hydroinformatics-enabling social “transmutations” necessary to achieve agricultural sustainability can be catalyzed. It underlies the social and technical “threads” to be woven together in order to extend from a hydroinformatics of the quantities into a hydroinformatics of the qualities (i.e., subjective experiences, perceptions; Vojinovic and Abbott, 2017), a necessary condition to unleash the potential of the application of hydroinformatics in agriculture.
The paper is organized as follows: section 2 is dedicated to the definition and roles of the discipline and provides rationales for the proposed framework; section 3 discusses the applicability of the framework using examples from agricultural water development projects in horticultural farming. The objective of this section is not to demonstrate the applicability of the framework, but only to propose a blueprint on how to develop it; section 4 discusses opportunities and challenges associated with the implementation of agricultural hydroinformatics; and section 5, before summarizing and concluding, discusses the development of new research areas to achieve the full potential of this emerging framework.
2. Agricultural Hydroinformatics: Conceptual Framework, Definitions, and Roles
Owing to the inevitable and complex interactions between water and the agricultural subsystems, hydroinformatics combines, coordinates and interplays with several scientific fields of study and practices when applied in agriculture. This synergy gives rise to a multi-purpose conceptual framework that captures the wholeness and inter-dependencies necessary to conduct holistic agricultural water systems studies and modeling.
2.1. The Conceptual Framework
A first factor that highlights the synergy between hydroinformatics and existing disciplines in agriculture is water over-extraction for growing purposes (i.e., at the operational level), because of its two-faced (beneficial and detrimental) impacts. Some implications include, for example: (i) land subsidence (Deng et al., 2018) which is a subject of geology; (ii) potential loss of livelihoods and ecosystems due to flooding risks, water scarcity and, thus, food security which are subjects of hydrology, hydraulics, ecology, economics, and sociology; (iii) the implementation of water governance institutions along with water rights and water conflicts resolution which are subjects of legal sciences. The resulting impacts and changes of these implications often in turn generate new social and technical developments that characterize the circular nature of hydroinformatics in supporting decisions that improve society (Vojinovic and Abbott, 2017; Makropoulos and Savić, 2019).
A second factor is water stress at the farm level as it is regarded as the greatest abiotic inhibitor of agricultural yield (Ciais et al., 2005; Gumiere et al., 2020). Such a factor has potentially destructive impacts on plant phenotypes such as growth, yield, efficiency and resistance (Houle et al., 2010) and livestock phenotypes such as feed intake, body mass, and milk production (Chedid et al., 2014; Sharma et al., 2015). Consequently, agricultural hydroinformatics may have a role to play in phenomics research (Houle et al., 2010; Furbank and Tester, 2011) and potentially in genomics research as well (Chaves et al., 2003; Tuberosa and Salvi, 2006). This role may be further extended into new gene-based crop and livestock models of interactions between genotypes, growth environments, and farmers' management decisions and practices to improve farm performance (Antle et al., 2017). Furthermore, water stress also influences the physiology of both plants (e.g., photosynthetic activity) and livestock (Osakabe et al., 2014; Fournel et al., 2017). Last but not least, pest-management regimes (pest management science) and waste management practices in agriculture are known to have consequences on water quality with implications on water stress (Sims and Wolf, 1994; Larson et al., 1997; Burkholder et al., 2006; Zhang et al., 2008).
The overviewed synergy between fields of studies and practices embodies the sociotechnical nature of the application of hydroinformatics in agriculture and, accordingly, it sets the basis for the generic conceptual framework depicted in Figure 1. Together, the components of the framework exemplify the wholeness and inter-dependencies necessary to conduct agricultural systems studies and modeling. Such an endeavor requires the weaving of the naturalistic perspective (i.e., clearly defined quantities) and the hydro-social perspective (subjective/phenomenological in nature) of water resources management (Biswas and Dune, 1971; Walker et al., 2015; Vojinovic et al., 2016; Vojinovic and Abbott, 2017; Zomorodian et al., 2018; Ženko and Menga, 2019). As such, the framework accounts for those aspects of water resources management that may not at all be expressed in numerical and/or monetary terms (Vojinovic and Van Teeffelen, 2007; Ten Veldhuis, 2011; Walker et al., 2015; Vojinovic et al., 2016). Thus, it exemplifies an anchor or starting point for extending from the rather narrow techno-centric approach of hydroinformatics toward a broader hydroinformatics of the qualities (Vojinovic and Abbott, 2017). In doing so, the potential of hydroinformatics in its scope and reach will be unleashed.
The framework also offers the opportunity to delineate the scope of future sociotechnological development for agricultural hydroinformatics. In this regard, it can be viewed as: (a) the data flows inventory necessary when conducting sustainable water resources management in agriculture, and (b) a discipline-delineated approach for the engineering of information and simulation models development and integration in agriculture. These two facets confirm the multi-purpose nature of the framework. The framework is amenable to capture, for example, the underlying data infrastructure needed to support the Plants in silico vision (Zhu et al., 2016), as well as the multi-scale crop modeling framework for climate change adaptation assessment as proposed by Peng et al. (2020). In these and similar contexts, agricultural hydroinformatics is expected to evolve with additional links to other fields of study and the development of new classes of tools and technologies. Accordingly, it may develop to (a) move the frontiers of the subfield currently known as AgInformatics or Agroinformatics (Gupta, 2009; De Montis et al., 2017; Gustafson et al., 2017) and (b) carry in its own right the sociotechnical implications of agricultural systems, an important aspect that is overlooked in the literature on agroinformatics (Sørensen et al., 2010; Lokuge et al., 2016; De Montis et al., 2017; Gustafson et al., 2017).
2.2. Definitions of Agricultural Hydroinformatics
In light of the proposed framework, agricultural hydroinformatics can be intrinsically defined as “the production and use of knowledge from data flows relevant to the understanding and management of water in agriculture, together with interactions with, transformation by, and impacts on surrounding environments (e.g., plants, soil, animals, people) and vice versa”. However, the agriculture sector represents a typical example of a technology ecosystem within which several technologies interact and influence one another's evolution. In such an ecosystem, agricultural hydroinformatics as a technology itself will interact with other technologies (e.g., farm machinery) either directly (e.g., usage and integration of data from agro-equipment) or indirectly (e.g., modeling of agro-equipment impacts on water). We have identified many components of the ecosystem, often referred to as reverse salient in the literature on sociology of technology (Hughes, 1987), that fails to deliver an acceptable level of technological capabilities to facilitate the integration and evolution of hydroinformatics therein. Therefore, we further define agricultural hydroinformatics as “the technology that should identify, support and promote corrective measures, and ultimately apply those (if necessary) capable of enhancing the performance delivery of the ecosystem of technologies as a whole.”
2.3. Anticipated Roles of Agricultural Hydroinformatics
In light of the second definition presented above, hydroinformatics, when integrated in the agricultural technology ecosystem, will impose both physical and digital constraints on the design, functionalities, characteristics of the ecosystem itself and respond to similar constraints that will contribute to the whole ecosystem evolution. This suggests that agricultural hydroinformatics carries the responsibility to define what we call, using an object-oriented software engineering metaphor, a set of role types as part of a role model that describes the functionalities of and collaborations between its inner and outer components in the technology ecosystem. This metaphor triggers the idea that agricultural hydroinformatics will hold an evolutionary influence on the other technologies it interacts with. Therefore, using social media parlance, agricultural hydroinformatics is a technology influencer and role model. Overall, it can be regarded as a blueprint for implementation and adoption of information and communication technologies in agriculture.
Last but not least, agricultural hydroinformatics may play a unique role in the definition of control and response variables based on local water systems conditions in support of the scientific roadmap toward the redefinition of the water planetary boundary concept as proposed by Gleeson et al. (2020). For instance, five of the six proposed new water planetary sub-boundaries (e.g., soil moisture, precipitation, evapotranspiration, groundwater) have direct connections with water management in agriculture.
3. Applicability of the Proposed Framework
The proposed framework embodies a certain degree of generality that makes it amenable to transcend both spatial (field, farm, watershed, regional, national, and global) and temporal (from the planning stage to food distribution stage) scales of food modeling and production systems. Therefore, it is suitable to be used in a wide range of agricultural development situations beyond connections between agro-economic and water engineering development and its socioeconomic impacts. We have identified at least three preponderant use cases for which the framework can be applied to support and guide sustainable agricultural development. They are: (i) data and information modeling, (ii) agricultural systems simulation and modeling, and (iii) engineering of simulation models source codes. We need to reiterate that the objective of this section is not to demonstrate the applicability of the framework, but only to propose a blueprint on how to develop it. However, the applicability will be exemplified through the detailed discussion of real-world case studies.
To better guide the discussion, especially on the data and information modeling, we must introduce a use case in the form of a complex situation where the majority, if not all, the components of the framework can be at play simultaneously. Such a use case must draw on prior practical situations that involve the tasks of water management for various (large-scale) purposes and at different spatial scales. Similar to the Oresund Project discussed before, this project to be selected as an example must also involve political and environmental stakeholders. Accordingly, it must address concerns, expectations, and requirements of a range of both formal and informal groups. In addition, it would do more justice to the utility of the framework to use at least a project that may have involved spatio-temporal sustainability-related simulations to persuade stakeholders during the planning stage through the project approval process and construction completion phase.
The Brazilian Rio Sao Francisco transbasin diversion project (de Andrade et al., 2011), owing to its unique socioeconomic, hydrologic and geologic context, is a particular project that fits well the stated criteria. It evolved under strict environmental laws; it required socially and environmentally conscious and participative stakeholders; it was designed to supply water to the most water-scarce area of the country with only 3.3% of water resources, but 29% of the national population; and it went through a tribulated approval process mostly due to a lack of solid technical plans, and public consensus about its potential benefits (de Andrade et al., 2011). In addition, agricultural water demand accounted for the largest percentage of the total demand (Maneta et al., 2009; Roman, 2017). Last but not least, the project has attracted the attention of a large community of both national and international researchers, making it a highly studied and adequately documented case.
3.1. Data and Information Modeling
In data and information modeling scenarios, the first step is to examine which component (s) of the proposed framework (Figure 1) is relevant. To determine this, the type of questions to be asked is for example: what kind of both quantitative and qualitative data or variables should be considered? The answer to this question would lead to an inventory of variables pertaining to each relevant component of the framework. This is the starting point to determine data flows consideration for a particular agricultural water management scenario using the proposed framework. If we consider the legal sciences component, the answer to this question is that the data model may include, for example, entities associated with description of laws or legal frameworks established to govern or constrain the operation and management of water development projects, as well as description of water governance institutions.
We present a preliminary example in Figure 2 with sufficient details depicting how some of the framework components can be used to develop information models for agriculture-related water development projects and farm management. We acknowledge that the example is non-exhaustive in the sense that it does not present a complete data model that includes all the components of the framework. This is also not the main objective of the paper. Therefore, instead of introducing a whole new information model, we tap into the Observations Data Model (ODM2; Horsburgh et al., 2016), a water-focused data model that has caught the attention of the agricultural research community (Janssen et al., 2017). It has been developed as a general information model to integrate both in situ sensors data, and ex-situ analyses results data, two situations that are common in the agriculture domain. It has also been successfully applied to four different disciplines (hydrology, rock geochemistry, soil geochemistry, and biogeochemistry) to meet field and laboratory data management needs (Hsu et al., 2017; Horsburgh et al., 2019).
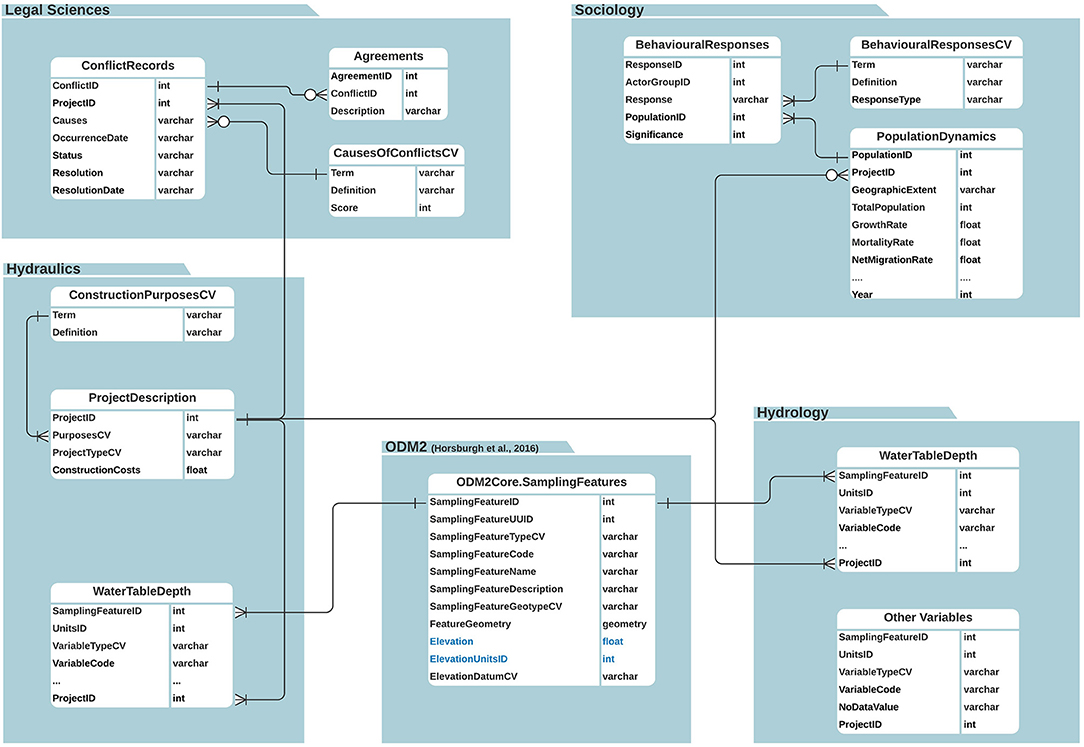
Figure 2. Example of data model schema conceptualized based off the agricultural hydroinformatics framework and the ODM2 developed by Horsburgh et al. (2016). It depicts four components of the proposed framework with related table definitions that may be used in developing a data model. The identifier field for each tuple (row) in the tables ends with the string “ID” with or without the symbol.
The example data model shows how some of the project attributes such as construction costs, distance of transfer and annual volume of water transferred (Shumilova et al., 2018) can be represented in the envisioned data model. The consideration of this simple metadata set is important for several reasons. First and foremost, there is no dedicated and maintained database on water transfer projects, particularly with irrigation as a purpose (Shumilova et al., 2018). Second, one can capitalize on the data flows from the proposed framework to include food traceability information as part of the data model by linking food chains to water supply sources. Thus, the information model can be extended to support more accurate and detailed embedded or virtual water (D'Odorico et al., 2010; Konar et al., 2011; MacDonald et al., 2015) and water footprint (Hoekstra, 2017) profiles. This is a growingly accepted factor to be accounted for in sustainable resource-use decision-making processes (Zipper et al., 2020). In addition, the hydraulics component may include time series of flow rate and diverted flow rate. Although not presented in the example, the data model may contain provision to record the characteristics of both the donor and receiving basins under the hydraulics component of the framework. A many-to-many donor-receiver relationship must be maintained as well to enable the ability of a donor basin to supply more than one receiver basin and vice versa. The purposes of the project (e.g., agriculture, mining, steelworks, domestic supply), a determinant factor in assessing economic impacts of water development projects (Xiao et al., 2019), may as well be included in the data model (e.g., as a controlled-vocabularies-based entity; see ConstructionPurposesCV and ProjectDescription entities in Figure 2).
Transboundary water development projects, such as the Brazilian Rio Sao Francisco transbasin and the Oresund Project, are often significant sources of water disputes and legal challenges (Thorkilsen and Dynesen, 2001; de Andrade et al., 2011; De Stefano et al., 2017). This suggests the need for the relationship shown between the hydraulics and legal sciences components in Figure 2. The latter component is presumed to contain mostly qualitative variables which are often associated with a set of weights or scores to quantify their importance (Vojinovic et al., 2016). Therefore, for the purpose of the example, we introduce an entity named CausesOfConflictCV, an entity to host controlled vocabularies describing causes of water conflict. A similar score-based variable approach was used to develop the Basins at Risk (BAR) scale that aims to systematically assess the process of international water conflict resolution (Wolf et al., 2003). Another example is the Water Events Scale (WES; Bernauer et al., 2012) depicting the intensity and impact of domestic cooperative and conflicting water-related events. The BAR and WES scales may also be included in the form of controlled vocabularies as entities of the legal sciences component.
Similarly, the hydrology component may contain hydro-meteorological variables recorded as time series. To not delve into the details of fully documenting hydrological data, we show how entities under the hydrology component, i.e., observational data derived from both sensors and specimens, can be described and juxtaposed to entities already defined in the ODM2. In Figure 2, we show an example of entity (WaterTableDepth) that has its additional characteristics defined in the ODM2. This extension of our example data model to ODM2 is achieved through the ODM2Core.SamplingFeatures entity, used to store the physical characteristics of the river basins which include spatially referenced data. This juxtaposition requires slight modifications in the ODM2 Core schema, such as the elimination of tables such as ODM2Core.Variables and the update of others such as the ODM2Core.SamplingFeatures table (see rows highlighted in blue in Figure 2) which serves as the point of connection.
Although groundwater is not directly withdrawn in the donor basin, for such a large-scale project, subsidence rates may be monitored in the donor basin. Accordingly, the geology component may be used to record measured subsidence rates and geologic characteristics of the donor and receiver basin. The description of such a variable would follow the same structure defined for the variables under the hydrology component as in Figure 2, i.e., the use of variable names as controlled vocabularies (e.g., water table depth, land subsidence) for the entity name.
The sociology component can be modeled as a set of entities representing relevant qualitative and quantitative variables. There is a systematic gap in the literature on the qualitative entities that should be considered. However, we draw from the literature on the field of socio-hydrology that aims to investigate the co-evolution and dynamics of social-water systems (Sivapalan et al., 2012). Research work on this subject (Elshafei et al., 2014; Kandasamy et al., 2014; van Emmerik et al., 2014; Kotir et al., 2016) suggest that the dynamics of the population is a quantitative variable that should be included. Therefore, we include an entity with attributes relevant to represent this phenomenon in Figure 2. The different types of social/behavioral responses (e.g., contractive, expansive, accommodating) and benefits of stakeholders/actors as well as impacts (Biswas and Dune, 1971; Abdullaev and Mollinga, 2010; Wheeler et al., 2013; Liu et al., 2015; Walker et al., 2015; Vojinovic et al., 2016; Srinivasan et al., 2017; Vojinovic and Abbott, 2017; Zomorodian et al., 2018; Ženko and Menga, 2019) at both the donor and receiver basins should be considered. Figure 2 shows an entity named BehavioralResponses with parameters describing how behavioral responses from each group of actors can be represented in the information model. The responses can be further segmented into classes (Neutral, Low, Medium, High) according to their level of significance or relative importance, which can themselves be standardized terms. This entity or the sociology component can also be extended to account for changes in behavioral responses and interactions through time or adjusted to consider the need of the pluralistic water research concept (PWR; Evers et al., 2017).
The discussed model for inter-basin water transfer projects may be extended to integrate a farm-level and field-level data model. Again, the field ProjectID can be used to link this data model to the example shown in Figure 2. Such a model may adapt, mimic, and extend, for example, the ODM2 discussed above. Depending on the type of farm, the data model may contain provision to record farm machinery operation information and accordingly may integrate entities for a Farm Machinery Management Information System (FMMIS; Fountas et al., 2015b). Ultimately, it may be extended to integrate components of a Farm Management Information System (FMIS; Fountas et al., 2015a). In addition, this data model may also contain description to record waste management practices, pest management practices, soil properties and water status (soil science component) through the ODM extension, phenomic data including crop water status (phenomics component) and ultimately genomic data (genomics component). For livestock farming, Fournel et al. (2017) provide a list of environmental, physiological and behavioral variables for farm-level data and they fall under the phenomics component and meteorology which we assume is part of the hydrology component of the proposed framework.
The current trend in agriculture is toward more complex, technologically-enabled farm production systems with increasing control and supervision (Lindblom et al., 2017; Wolfert et al., 2017; Peng et al., 2020). With the ideas presented in this section, we bring forth the vision to treat agricultural production as a sociotechnical phenomenon and promote a sociotechnical-system approach to data and information models development as epitomized by the proposed framework. The discussed data model, due to its holistic nature, may be called a Sustainability-oriented Agricultural Data Model (SADaM). As highlighted above, agricultural hydroinformatics, in its role as a technology influencer, bears the responsibility to build on and adapt existing progress and proven best practices in information modeling such as the ODM2 to deliver a fully-fledged data model. This task constitutes a first and foremost step toward building a strong foundation for new and more profound changes in the agricultural sector. As a consequence, we anticipate and discuss below the development of more advanced data acquisition systems and tools/middleware enabling direct data and metadata streaming to database systems implementing the SADaM information model.
Our attempt to represent qualitative variables as part of the information model example in Figure 2 highlights an outstanding gap in the literature on socio-hydrology. This is the need for the development of a common nomenclature and controlled vocabularies to represent qualitative processes such as attitudes of stakeholders toward water availability or scarcity. Well-known efforts such as the Open Geospatial Consortium (OGC), World Meteorological Organization (WMO), and the Consortium of Universities for the Advancement of Hydrologic Sciences, Inc. (CUAHSI) are of very little or no relevance. Nevertheless, Wheeler et al. (2013) lay the groundwork for such future research effort on this subject. The development of such a data model also involves the need to capture not only the semantics of each component, but also the lineage relationships between the processes and the resulting outputs as part of the metadata development processes. This may ultimately lead to a specialized ontology integrating the elements and relationships in the proposed framework. This effort may build on prior ontology-development effort as this practice has been promoted and is widely implemented by the hydroinformatics community (Abbott, 1999; Islam and Piasecki, 2006; Beran and Piasecki, 2009; Garrido et al., 2012; Liu et al., 2013; Brodaric and Hahmann, 2014; Yu et al., 2015; Brodaric et al., 2016).
3.2. Agricultural Systems Simulations Models Development
We have discussed how the proposed framework can be applied to develop linked data models through a discipline-delineated entities approach whereby variables are arranged under their corresponding component of the framework. The same approach can be adopted to architect agricultural systems simulation models. The first step is to identify the target processes to be simulated. These can be crop yields, water use, or demand and evapotranspiration rate. The second step is then to identify the relevant variables (raw or processed data) and new or existing models that output the relevant variables (data products) for the identified target processes. For example, the simulation of crop water demands (hydraulics component) requires a model (hydrology component) to estimate the potential evapotranspiration rate (data product). A more complex example can be the simulation of crop growth (phenomics component) response to water and nutrients. This requires a water budget model (hydrology component), nutrients budget model (soil science component), nutrients transport model (soil science component), infiltration and runoff models (hydrology component).
The identified models fall under a certain component (e.g., hydrology) but are able to pull data (raw, processed, aggregated, model results) from any component with the relevant data. Thus, simulation models of various complexity level can be built on top of and tap into a database that implements the data model discussed above. In such a data system and simulation model configuration, the new model results can be saved back to the data system with its provenance information (e.g., Rousseau et al., 2000). We present in Figure 3 the four-layer skeleton of such a system in the form of a multi-layer Artificial Neural Networks architecture for knowledge workflows engineering. It shows the relationships between: (a) first layer: raw data (e.g., temperature from sensors), (b) second layer: processed data from the previous layer (e.g., minimum and maximum daily temperatures), (c) third layer: simple models (e.g., evapotranspiration) that use data from either of the first two layers or from both of them, and (d) fourth layer: complex physical models (e.g., Hydrus; Simunek et al., 1999; AquaCrop; Vanuytrecht et al., 2014; AquaCrop open-source version; Foster et al., 2017) that, for example, suggest the amount of water to apply for irrigation or quantify crop yield response to water. This last layer may also include data-driven models (e.g., machine learning models) similar to the example reported by Gumiere et al. (2020) and can pull data from any combination of the three layers on top.
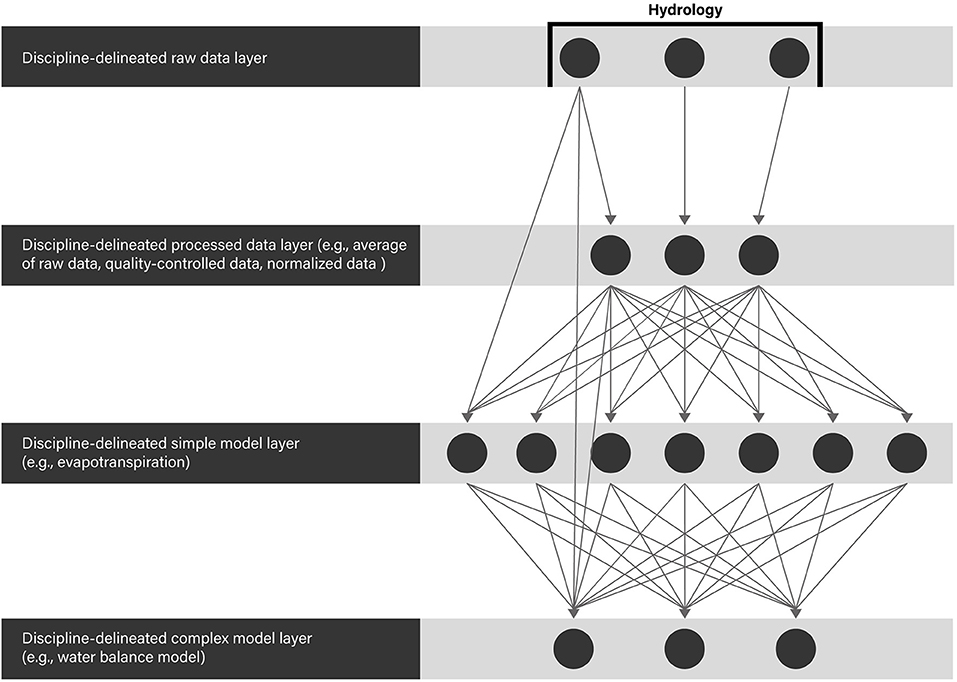
Figure 3. Architecture of the envisioned knowledge workflow management of agricultural hydroinformatics.
The versatility of the framework confers to agricultural hydroinformatics the ability to promote sustainability through water management-centric practices. These practices are in turn supported by a sociotechnical-system approach built on information and simulation models. We, therefore, envision an agricultural simulation system or environment that boosts reproducibility of research through systematic and formal records of the relationships among raw data, the processes that produce results and the results themselves as highlighted by Pasquier et al. (2017). This feature of the framework makes it particularly well-suited to support the delivery of integrated modeling structures for complex agricultural systems using, for example, the system dynamics method (e.g., see Berthiaume et al., 2005). This method has gained grounds in a broad spectrum of applications, primarily when water resources and agricultural production systems sustainability objectives are pursued (Walters et al., 2016; Zomorodian et al., 2018). It subdivides a system into an interconnected series of flows (inputs and outputs) and stocks (reservoirs) affecting one another through a feedback mechanism, thereby providing decision-makers with possible consequences of system perturbations (Zomorodian et al., 2018). A preliminary example of the application of the system dynamics approach to simulate agricultural production systems (crop and livestock) sustainability by Walters et al. (2016) demonstrates that five (5) clusters of flows and stocks (Economics, Crop Production, Livestock Production, Environmental Quality, and Social Quality) are reflected in our proposed framework.
3.3. Simulation Models Source Codes Engineering
The framework brings forth the ability to guide the engineering of simulation models source codes. In line with the new architecture of the Decision Support System for Agrotechnology Transfer (DSSAT) cropping system model presented in Jones et al. (2003), the framework imposes a modular structure in which components separate along scientific discipline lines; it is structured to allow easy replacement or addition of components or sub-components, and enable greater cooperation in interdisciplinary research and in the application of knowledge to solve problems at all levels. In addition, it promotes the granularity of simulation models development by supporting the generation of source codes incrementally through the combination of atomically-defined model algorithm blocks. A block can be developed to comply with the Single Responsibility Principle or Separation of Concerns of software engineering (Martin, 2002) and thus, is responsible for a single task (e.g., computation of hourly averages). An anticipated benefit is that a block of source codes responsible to compute a specific data product within or for a model can be developed under one component of the framework and reused under any other component of the framework. Thus, from a software engineering point of view, a block of codes may be designed to serve as a single channel to output a specific time series from the database or data product from a model to any component or any model within a component of the framework.
4. Opportunities and Challenges for Agricultural Hydroinformatics
Although the agricultural domain is well-stratified with its techniques and approaches for knowledge generation and consumption, we anticipate that hydroinformatics will find many not-currently-apparent opportunities to underpin social, institutional, and technological innovations around water resources management toward agricultural sustainability. We anticipate that more opportunities will occur when, for example, the agricultural hydroinformaticist explores the business and phenomenological dimensions of hydroinformatics. In line with this “entrepreneurial” endeavor, Kamilaris et al. (2017) call for the creation of radically new services to promote, for example, the practices and benefits of (big) data collection and analysis in agriculture. Next, we highlight opportunities and challenges to accelerate social and technological transformations through the application of hydroinformatics in agriculture.
4.1. High-Performance Data Acquisition Systems Development
The complexity of a data management system that implements the information model discussed here will evidently require more automation and capabilities from data acquisition systems. In this regard, a best practice we have learned through experience is that the development in data acquisition systems must take place after the data management planning phase. This constitutes the main reason why we introduce the discussion on data and information modeling first. The primary rationale for such order is that when data acquisition devices/systems are designed and developed with no consideration of the (digital) constraints imposed by a data management system that prioritizes data standardization, they tend to obey what we may call the “data logging rules or philosophy.” This implies that the devices or data acquisition systems (i.e., current dataloggers and sensors) simply collect and operate on data values. In other words, they have no formal support for metadata annotations, a critically important feature to reduce the manual data-to-metadata-mapping effort on data managers (when data standardization is adopted) and increase the useability and value of the data. Furthermore, the configuration process (e.g., programming) of those devices often allows the user or operator to influence the data production process. This influence occurs through tasks that are as simple as allowing the operator to set names for variables being measured. This is an outlet for all three types of heterogeneities (schematic, semantic, syntactic) in the collected data. As pointed out by Kruize et al. (2013), it usually results in substantial manual and cognitive efforts to preprocess and harmonize the collected data. In addition, it becomes more complicated to annotate the data with corresponding metadata. These shortcomings are also present in data acquisition systems used in the agricultural sector.
A review of the literature reveals that current (farms) field data acquisition systems are not designed to be, and thus, are not considered as an integral part of FMISs (see Nikkilä et al., 2010; Kaloxylos et al., 2012; Kruize et al., 2013; Zia et al., 2013; Fountas et al., 2015a; López-Riquelme et al., 2017; Paraforos et al., 2017; for examples of FMIS building blocks). Such a technical deficiency or schism may explain why data acquisition and management, for on-farm decision support, research and policy decision-making purposes, is recognized as the most pressing limitations of agricultural systems models (Capalbo et al., 2017; Jones et al., 2017). In the context of precision agriculture, which is a growingly accepted practice to achieve sustainability, these limitations are mainly attributed to the heterogeneities of the collected and available datasets (Nikkilä et al., 2010; Wolfert et al., 2017). Two widely cited reasons are that the data flows involved originate from various instruments and technologies (Fournel et al., 2017; El Bilali and Allahyari, 2018), and from several (sub)disciplines and sources (Capalbo et al., 2017; Janssen et al., 2017). Therefore, the development of more advanced technologies for data acquisition that resolve schematic, semantic and syntactic heterogeneities is another area wherein the impacts of hydroinformatics is most needed. The hydroinformatics community has made significant development in this area with the advent of the Internet of Things (IoT) paradigm, low-cost, and open-source software and hardware (e.g., Wong and Kerkez, 2016; Horsburgh et al., 2019). Although in some hydroinformatics case studies, the devices automatically ingest the collected data to a standardized data management system, they still adopt the data logging philosophy, reveling an opportunity for agricultural hydroinformatics to introduce some new technologies using new approaches. Below, we introduced characteristics of potential data acquisition systems that are more suitable to close existing gaps toward a more integrated farm sensing and FMIS.
A potential innovative solution that could get at least three birds with one stone is a new generation (second generation with respect to data loggers) of sensing systems for both point-based and spatial monitoring designed to be deployed in the field with a (minimum) knowledge base of the object being monitored, in addition to user-defined deployment-context metadata. Celicourt et al. (2020) have created a preliminary prototype of such system mainly to cope with the data management issues previously mentioned. An immediate and first benefit is that the devices (for point-based and geospatial observations) could work autonomously and in synergy to make decisions in the field. Second, they can themselves be programmed or configured to automatically create new metadata and/or derive metadata from original deployment-context metadata and document the decisions made. Subsequently, the documented decision along with farm field statuses may ultimately be submitted via some Application Programming Interfaces (APIs) to the backend of a cyberinfrastructure. Third, the devices may be also designed to output human and machine-readable data in standardized formats such as WaterML (Almoradie et al., 2013).
A prototype of this second generation of data acquisition system may simply consist in a Soil Matric Tension (SMT) sensor along with other relevant sensors attached to a microcomputer (e.g., Raspberry Pi, BeagleBone Black) or microcontroller (e.g., Arduino), but with onboard deployment-context information as highlighted in the aforementioned ODM version 2.0 example, in addition to farm characteristics. The knowledge base can contain information that is as minimal as the root zone tension range of −4 to −7.5 kPa and water table depth range of 500–600 mm (Vanderleest et al., 2016) for cranberry productivity optimization, for example. In situations where an irrigation event occurs in a specific field, the data acquisition system can be programmed to automatically generate the data and metadata necessary (e.g., water volume, duration, soil matric potential, weather conditions, field or bed identifier, etc.) to document such event according to a relevant agri-food standard. The file may be downloaded on-site, or the content can be encoded and transmitted to a web server where it is accessible to a FMIS. This latter may, in turn, formats and shares the received information using the same standard format.
This generation of sensing devices (front-end) can be integrated in a feedback loop with crops and livestock models (backend of a cyberinfrastructure) to assess the inevitable gap between experimental results and field implementation and take on-the-fly corrective measures. Thorkilsen and Dynesen (2001) implemented a feedback monitoring programme with a similar mechanism in the context of the aforementioned Oresund project which was quite effective in ensuring the fulfillment of environmental objectives and design criteria during and after the project construction phase.
A third and more futuristic generation of farm monitoring systems include those based on artificial intelligence techniques (e.g., computer vision, natural language processing) along with other relevant techniques to automatically determine relevant information about the farm fields (e.g., geospatial extent, type of crop, growth stage, crop statuses) being monitored. Contrary to the second generation previously mentioned, this one would be capable of generating nearly all of the necessary metadata (auto-generated metadata) needed to document the collected information including geographic positions of the sensors or all components of overall sensing system. Such a system would require a new kind of “built-in” metadata that is relevant to help, in a first stage, the interpretation of the auto-generated metadata, and in a second stage, the collected data.
As an example, such a third-generation field-data acquisition system may consist of a SMT sensor that automatically reports its deployment depth (e.g., 250 mm) as a piece of metadata. This SMT sensor may rely on either a patched depth sensor (assuming in prototyping stage) or an integrated one (e.g., using Radio-Frequency IDentification (RFID) technology) that behaves as multi-parameter sensor. In this situation, the depth sensor has its own, preferably built-in, metadata (e.g., accuracy, variable, unit, etc.) that describes the depth measurement for the SMT sensor. The SMT sensor also has a similar set of, preferably built-in, metadata. In this example, the interpretation of the SMT sensor data involves the knowledge of: (a) the sensor metadata itself, (b) the auto-generated metadata by the depth sensor and (c) the metadata of the depth sensor. This last set of metadata is a new kind of data that bears the potential to help in understanding the first two sets (a, b) of metadata and is, therefore, a “meta-metadata” or “super-metadata” or “root metadata.” The SMT sensor may be further retrofitted with a GPS device and an accelerometer to automatically report its deployment position (latitude, longitude, and/or altitude). This would further simplify the metadata provision process and allows automatic management (commissioning and decommissioning) of the sensor. In this case, the characteristics of the GPS device and accelerometer would serve as additional super-metadata for the SMT sensor. Although the hydroinformatics community has not dealt with the management of this new kind of metadata before, the experiences developed therein in terms of metadata management and ontology development may guide the path forward, as this concept is applied to data and information modeling.
The ideas presented above set agricultural hydroinformatics in a position to help address the major lag time in systems to exploit new advances in data, information and communication technology (Berger and Hovav, 2013; Fountas et al., 2015a; Antle et al., 2017; Janssen et al., 2017). Consequently, recent efforts to improve and accelerate the rate of agricultural innovation and meeting the growing global need for food and fiber have been limited by the need for improved data, models and knowledge products (Fountas et al., 2015a; Capalbo et al., 2017; Jones et al., 2017). The ideas presented here as possible pathways for new sensing systems for farm monitoring are intended to alleviate the usability problems and the requirements for farmers to manually input data that they are not familiar with. These two obstacles are identified as important limiting factors to the adoption of FMIS (Alvarez and Nuthall, 2006; Nikkilä et al., 2010; Kruize et al., 2013).
4.2. Novel Approaches to Simulation Models Development and Integration
A common limitation of most crop and environmental models is that the model is distributed solely as a compiled software package (Foster et al., 2017). Furthermore, Jones et al. (2017) acknowledge that major advances are needed to achieve the next generation of simulation models that move beyond the inclusion of economic and sustainability issues to meet, for example, the agenda of Sustainable Development Goals (SDG). In line with this vision, our proposed framework promotes the level of flexibility in terms of modeling approach, models implementation and modularity, types of models, and models-integration extent needed to tackle the complexity of agricultural systems modeling. To this end, the development of open web-based computational infrastructures or tools ecosystems that support the creation of models source codes through the combination of atomically-defined models components or subsystems components may be handy. To facilitate model integration, such a tool ecosystem may be designed to automatically encapsulate the generated source codes for each model within the structure of a chosen modeling framework (e.g., OpenMI; Gregersen et al., 2007). Although the implementation process can be quite a complex task to achieve a resulting set of coupled models that is as simple as the prescriptive sociohydrology framework presented by Elshafei et al. (2014), it would be instrumental in delivering truly reusable, modular and highly granular models. Such an infrastructure bears the potential to be transformed into a new kind of decision-support system which is that of the creation of integrated agricultural models and even DSS, thus a meta-DSS. Last but not least, it may be further extended to enable the generation of the resulting models in different programming languages (e.g., Fortran, C, C++, Python) using the Abstract Syntax Trees (AST) concept (Noonan, 1985). This is another opportunity for agricultural hydroinformatics to play its role of a technology influencer.
4.3. Innovative Technologies Adoption
We have hitherto identified a number of avenues to overcome challenges pertaining mostly to improvement in agricultural data quality and management along with model implementation and a new kind of DSS to facilitate models integration. However, these challenges represent quite a narrow view of the challenges ecosystem to achieve agricultural sustainability and meet future demands for food and fiber. Here, we aim to move the horizon a little further to include those related to innovations adoption with a focus on precision agriculture, which is our paradigm of interest. Adoption challenges are preponderant as they represent serious barriers toward practical applications, improvement, adaptation, and maturation of innovations to empower farmers of all classes as genuine stakeholders. The objective is on-farm inputs optimization to achieve lower food production costs and food prices, higher inputs productivity, environmental sustainability, food security, and safety.
Despite the experienced and potential benefits of precision agricultural technologies (PATs), including profitability and productivity (Capalbo et al., 2017; Griffin et al., 2017; El Bilali and Allahyari, 2018), their adoption is still limited in farming practice. This can be seen as a particular case of the more general problems of adoption and diffusion of new technologies or products that also occur outside the agricultural domain studied by Moore (1991) as the chasm theory. In the agriculture domain, a major impediment to adoption is the lack of technology delivery mechanism (Zhang et al., 2002) that often hinders the perceived benefits and usability to justify upfront investments in new technologies. This stumbling factor is often attributed to the implementation problem of FMISs incorporating support for farm managers to implement decisions (McCown, 2002; Rossi et al., 2014; Lindblom et al., 2017). They fail to capture the “tacit” knowledge and practical needs of farmers and are, therefore, not adapted to the trade-offs and high complexity that characterize farmers' decision making processes (Lindblom et al., 2017). This results in what McCown et al. (2009) called the relevance gap, a discrepancy between scientific knowledge implemented in agricultural decision support systems (AgriDSS) and practical needs and experience-based knowledge of farmers.
The relevance gap indicates that the sociotechnological nature of AgriDSS has not been (adequately) captured over the last 30 years of research and development (Lindblom et al., 2017). It is also supporting the evidence that the transformation in the role of farmers, agricultural technologists, and scientists from knowers to consumers of knowledge, whereby the latter becomes a kind of product to be consumed through socioinstitutional arrangements, has yet to occur. Such a transformation has been instrumental to the success of hydroinformatics as it is applied to water systems (Thorkilsen and Dynesen, 2001; Abdullaev and Rakhmatullaev, 2014) and it is now an essential aspect of the discipline (Vojinovic and Abbott, 2017). It is also a precondition for agriculture to enter the postmodern era and for agricultural hydroinformatics to become a postmodern technology that transforms farmers' behaviors toward water management practices in particular and agricultural production practices in general.
The relevance gap is driving a growing interest in the adoption of sociotechnical approaches to agricultural technologies in general and (big) data management and DSS development in particular by integrating stakeholders in the development process (Rossi et al., 2014; Fountas et al., 2015a; Janssen et al., 2017; Jones et al., 2017; Lundström and Lindblom, 2018; Rose et al., 2018a,b; Gutiérrez et al., 2019; Jakku et al., 2019; Rupnik et al., 2019). Such approaches are implemented through a number of so-called sustainability theoretical and conceptual frameworks that include the Multi-Level Perspective (MLP), the social practice theory discussed by Jakku et al. (2019) and the Agricultural Innovation Systems (AIS) approach introduced by Kilelu et al. (2013). However, the efforts appear to be at an infancy stage. Nevertheless, this convergence in development approaches toward what we can call the hydroinformatics ways of working further justify its relevance to agriculture. It presents an opportunity for agricultural hydroinformatics to promote and accelerate the adoption of existing and future technologies in agriculture to deliver better decisions. That is the kind of situation therein Abbott and Jonoski (2001) anticipated hydroinformatics could play a vital role in producing and consuming conjunctive (social and technical) knowledge that relates water-dependent soil-cultural practices and plant cultivation practices in new ways to promote justifiable productivity. To this end, they have proposed a three-part conceptual framework representing a generic hydroinformatics knowledge self-management system for knowledge-intensive applications such as precision agriculture and aquaculture, health care services. Kaloxylos et al.'s (2012) view of future Farm Management Systems shares some similarities with the proposed system. However, Abbott and Jonoski (2001) acknowledge that the realization of a corresponding prototype represents the greatest sociotechnical challenge of hydroinformatics due to socioinstitutional arrangements it may require.
The current trend toward the conceptualization of water systems as cyber-physical infrastructures (Makropoulos and Savić, 2019) is another convergence point between ongoing developments on water systems management and (precision) agriculture. This concept offers the opportunity to develop an integrated data infrastructure and drive real-time operational decisions along with predictive capabilities to improve farm-level management and water systems operations (Wolfert et al., 2014, 2017; Capalbo et al., 2017). However, the volume and variety of data involved in precision agriculture, acceptedly named “big data,” are far more complex than those in water systems. Cyber-physical configurations enabled by the IoT paradigm and Cloud Computing technologies hold an opportunity to transform the entire food supply chain into smart webs of connected, context-sensitive, uniquely-identifiable, remotely-controlled things that are context-sensitive and can be identified and controlled remotely (Wolfert et al., 2014). Furthermore, these configurations can be viewed as an extension of either of the two generations of data acquisition systems proposed above. They set the stage for a more advanced farm management technology ecosystem enabling end-to-end support for the FAIR (Findable, Accessible, Interoperable, Reusable) data principles (Wilkinson et al., 2016) and also the implementation of the Digital Twin concept (Ding et al., 2019).
The practical implementation or, at the very least, a prototype of these configurations is indeed challenging (Wolfert et al., 2014, 2017), but a lean and agile prototyping approach can shed enough light to move the development forward while opening up pathways to many opportunities. From a technical point of view, implementations with a focus on “big data” management may play a preponderant role in engineering the aforementioned whole-system data model (SADaM) as the “big data” paradigm hauls a growing emphasis on metadata for data provenance (Janssen et al., 2017; Wolfert et al., 2017; Coble et al., 2018). From a socioinstitutional point of view, the adoption of the “big data” approach will certainly highlight factors and conditions that may influence the uptake of these new approaches to farm management. The complexity of repatriating and processing large private and public datasets is perceived as a possible cause of the deceiving adoption of precision agriculture technologies (Lawes and Robertson, 2011). In this regard, Wolfert et al. (2017) noticed significant shifts in roles and power relations among different players composing the agricultural “big data” landscape. But, this landscape, as described, does not hitherto contain any knowledge provider in the “hydroinformatics” sense as defined by Abbott and Jonoski (2001); Abbott and Vojinovic (2010a). Nevertheless, owing to the entwined nature of knowledge and power, we anticipate the growing dynamics in those power relations will mutate into the Michel Foucault's problematics of “power/knowledge” structures. Fortunately, the three-part conceptual framework by Abbott and Jonoski (2001), discussed earlier on, has been proposed to specifically invert these structures through an inversion in knowledge relations. Realizing a smoothly-working prototype of this proposed example will certainly bring around many business opportunities which is a basic tenet of hydroinformatics (Abbott, 1999; Abbott and Jonoski, 2001).
Beyond the relevance gap, a specific, but determinant, component of stakeholders engagement that remains a serious stumbling factor to the theoretical and conceptual frameworks mentioned above when applied in agri-food sustainability transitions is the penetration of innovative knowledge into the mainstream agricultural regime (Ingram, 2015). For instance, the most prominent transition framework applied on agricultural sociotechnical transitions, namely Multi-Level Perspective (Rip and Kemp, 1998; Geels, 2010), suffers from a variety of inadequacies highlighted in Hassink et al. (2018) and Ingram (2015). Consequently, it is increasingly being complemented with other frameworks focusing on human-related and social factors (El Bilali, 2018) which is a symptom of the inaptitude of the broad range of existing frameworks to effectively tackle agricultural sustainability transitions. From a hydroinformatics stand-point, we are tempted to consider this strong resistance of mainstream agricultural regime to niche knowledge as a situation embodying the same problematics of “power/knowledge” mentioned above. Accordingly, for having overcome resistance in organizations strongly stratified (again, in the sociotechnical sense) as highlighted with two examples in the introduction, hydroinformatics is in a unique position to “crack” mainstream agricultural regime strata, by analogy to the hydraulic fracturing technique for natural gas extraction. In this regard, Abbott (1999) suggested that the problem be tackled through (and we quote) “the provision of knowledge to very many persons over electronic networks [sociotechnical environments], and [more importantly, the provision] of capabilities for people to express their fears, concerns and aspirations over these same networks, and thence to interact and organize themselves.” Thus, this is a further opportunity for agricultural hydroinformatics to play an underpinning role in facilitating the creation of sociotechnical environments in which the transformations or transitions necessary to achieve sustainable agricultural systems can be catalyzed.
4.4. Streamlined Agricultural Knowledge Management Workflows
The data heterogeneity problem in data sources discussed above has led to a number of scattered standards development efforts, including data management standards and data vocabularies (White et al., 2013), field devices communication (e.g., CANbus, ModBus; Suprem et al., 2013; Ojha et al., 2015), and thesaurus- and ontology-based developments (Janssen et al., 2017; Kamilaris et al., 2017). However, a superficial examination of some of them reveals that they suffer from several shortcomings, including incompleteness and inadequate description of variable names (e.g., use of abbreviations) and physical units which increases the mental workload necessary to work with them.
Data heterogeneity resolution at the cyber-infrastructure level is a particular area wherein the hydroinformatics community has made significant practical advances (Horsburgh et al., 2008, 2016; Beran and Piasecki, 2009; Abdullaev and Rakhmatullaev, 2014). A major example is the CUAHSI's Hydrologic Information System (HIS), developed as a cyberinfrastructure to support the management and dissemination of fixed-point field observations (Horsburgh et al., 2008). The system has seen widespread adoption in enabling the interoperability of observations through the development and standardized use of the ODM discussed above. Thus, data heterogeneity resolution at both the instrumentation and cyber-infrastructure levels represents the last piece of the opportunities puzzle for hydroinformatics to fully play its role of a technology influencer. In this context, agricultural hydroinformatics is a prime candidate to develop fully streamlined agricultural knowledge management workflows that adhere to the architecture presented in Figure 3. Such an architecture represents an instance of the anticipated knowledge chain concept in the form of a set of linked steps by which data are processed into information, knowledge and finally wisdom as used in decision-making processes discussed by Janssen et al. (2017).
We highlight that special attention needs to be paid to the treatment of the units in knowledge management workflows, which may further complicate the integration of datasets. Celicourt and Piasecki (2016) have already developed a unit-of-measurements management and conversion tool named “HydroUnits” based on the units Controlled Vocabularies developed by CUAHSI. Thus, we are aware of the deficiencies and opportunities to improve this existing set of units. The improvement would include, for example, compliance with the Unified Code for Units of Measure (UCUM) to achieve greater interoperability (Schadow and McDonald, 2013). In addition, the CUAHSI units certainly need to be revised and augmented to become what we may call “AgroUnits.” This latter may ultimately serve to improve the deficiencies of existing ontologies used in agriculture.
The development of agricultural knowledge management workflows would be an important step toward the next generation of FMIS as water resources management support is identified as a key bottleneck that hinders farm efficiency (Kruize et al., 2013). In addition, it creates the opportunity to incorporate support for whole-farm-system data provenance capture and preservation, in addition to traceability along the whole food system chain. This holds the benefits of helping companies, governments, and farmers in the food chain to make informed decisions (Janssen et al., 2017).
5. Summary and Conclusion
Agricultural production sustainability has become a critical, but challenging approach to meet food demands of the growing global population. This requires major transformations in agricultural practices through technological, social, and institutional innovations. Water resources planning, management and usage at the field, farm, river basin, regional, and national scales represent a pivotal strategy to underpin these transformations. In this context, we have introduced a blueprint for a new interdisciplinary field of research in agriculture named agricultural hydroinformatics. It is intended to employ hydroinformatics approaches to promote a holistic approach to agricultural water and knowledge management by considering the economic, engineering, social, hydrologic, environmental, and even political factors that influence agricultural water resources planning, management, and usage. Furthermore, it must foster adoption of technologies as a path forward to achieve sustainable agriculture in light of the anticipated increase in agricultural water demand due to climate change impacts and global population growth.
We have introduced a new general conceptual framework that stems from the particularities of the sociotechnical dimension of using hydroinformatics in agriculture. The framework is designed to support, not only integrated agricultural resources and assets management, but also agricultural sustainability in general. It can also be used as a simple tool to gauge the degree of integration of (agricultural) water resources management programs or initiatives as it depicts the components (e.g., soil, water, land, crop, people) that may need to be considered. It also enables the development of an entirely integrated but discipline-delineated knowledge management workflows for agricultural production consisting of raw data, metadata-annotated data, storage and dissemination, models, and decision support system.
We acknowledge that this blueprint for the proposed framework is freshly introduced and therefore, it has not yet been vetted by the hydroinformatics and agricultural research communities. Although it is developed to be as generic as possible, we believe it may evolve in many ways and under many circumstances. For example, the framework may evolve by integrating new fields of research, disciplines and practices as they emerge. The introduction of new fields and practices may lead to splitting a component or aggregation of two or more components of the framework. Sociohydrology is a typical example of an emerging field of research that may lead to some transformations of the framework. These anticipated mutations, therefore, will give rise to more specialized as well as more mature and either more specialized or more generic versions of the framework. Multidisciplinary forms of intra- and inter-universities collaborative efforts as well as development of new curricula to train agricultural hydroinformaticists will play an important role in paving the way for this new field of research and framework to gain momentum. This is challenging, as the topics to be covered may range from information and decision theories to social sciences to legal sciences to ethics and philosophy of science; to name but a few. These initiatives will serve as a ground work for agricultural hydroinformatics to make a more significant impact in driving agricultural sustainability transitions.
Some major development must also occur in various technology-related research areas through collaboration between universities, research centers and technology companies applying the business dimension of hydroinformatics. These include, for example, the development of self-managed in-situ sensors, farm machinery and unmanned automated vehicles (UAVs) systems that are easy to deploy and require as little human intervention as possible. An important aspect of this self-management capability includes, for example, the embedding of artificial intelligence algorithms to enable sensing and control systems to perform and record automated signal and data quality control procedures. Another aspect is the automated management of both raw and processed data through the development of standardized data models with provision for sufficient ancillary information to facilitate data interpretation, retrieval and integration. A third and critical aspect is the involvement of stakeholders including farmers throughout the research and development processes to tackle the knowledge and technology relevance issue and facilitate the adoption of resulting technologies. The phenomenological dimension of hydroinformatics will play a significant role here. This latter aspect may be instrumental in gauging the level of system intelligence needed at the farm level and the cloud level.
Lastly, the transformation in the role of farmers, agricultural technologists, and scientists from knowers to consumers of knowledge represents a milestone for agriculture to enter the postmodern era and for agricultural hydroinformatics to become a postmodern technology. Therefore, research and development efforts must be conjugated toward a knowledge self-management system prototype as described in the paper to reverse the growing power/knowledge relationships related to innovative technologies adoption, and concepts such as big data and knowledge-intensive agriculture. This would be a turning point toward the co-creation of knowledge to the benefits of all categories of farmers.
It is when these ideas are turned into practical applications through the establishment of appropriate and adequate sociotechnical arrangements, as well as the provision of personalized and customized services with tangible benefits, that agricultural hydroinformatics will fully play its role as a blueprint for the adoption of information technologies in agriculture to the benefit of all farmers.
Data Availability Statement
The original contributions presented in the study are included in the article/supplementary material, further inquiries can be directed to the corresponding author/s.
Author Contributions
PC wrote the first draft of the manuscript. AR, SG, and MC review and edit the manuscript. All authors contributed to the article and approved the submitted version.
Funding
This research was supported by a grant (RDCPJ 477937-14—Gestion intégrée des ressources en eau dans la production de canneberges) from the Natural Sciences and Engineering Research Council (NSERC) of Canada. We also acknowledge the contribution of CentrEau—Quebec Water Research Centre in the form a postdoctoral scholarship through the New Research Projects Grants program.
Conflict of Interest
The authors declare that the research was conducted in the absence of any commercial or financial relationships that could be construed as a potential conflict of interest.
Acknowledgments
We thank Dr. Michael Piasecki (The City College of New York, USA) for his comments on the paper. We also acknowledge the contribution of Karene Gauthier (Université Laval, Canada) who designed the figures used herein.
Abbreviations
AIS, agricultural innovation systems; AgMIP, agricultural model intercomparison and improvement project; AgriDSS, agricultural decision support systems; API, application programming interfaces; AST, abstract syntax trees; BAR, basins at risk; CUAHSI, consortium of universities for the advancement of hydrologic sciences, Inc.; DSS, decision support systems; DSSAT, decision support system for agrotechnology transfer; FAIR, findable, accessible, interoperable, reusable; FAO, food and agriculture organization; FMIS, farm management information systems; FMMIS, farm machinery management information systems; GPS, global positioning system; GWP, global water partnership; HIS, hydrologic information system; IoT, internet of things; IWRM, integrated water resources management; MLP, multi-level perspective; ODM, observations data model; OGC, open geospatial consortium; PAT, precision agricultural technologies; PI, precision irrigation; PLF, precision livestock farming; PWR, pluralistic water research; RFID, radio-frequency identification; SADaM, sustainability-oriented agricultural data model; SDG, sustainable development goals; SMT, soil matric tension; UAV, unmanned automated vehicles; UCUM, unified code for units of measure; WaterML, water markup language; WES, water events scale; WMO, world meteorological organization.
References
Abbott, M. B. (1999). Introducing hydroinformatics. J. Hydroinform. 1, 3–19. doi: 10.2166/hydro.1999.0002
Abbott, M. B., and Jonoski, A. (2001). The democratisation of decision-making processes in the water sector II. J. Hydroinform. 3, 35–48. doi: 10.2166/hydro.2001.0005
Abbott, M. B., and Vojinovic, Z. (2010a). Realising social justice in the water sector: 1. J. Hydroinform. 12, 97–117. doi: 10.2166/hydro.2010.065
Abbott, M. B., and Vojinovic, Z. (2010b). Realising social justice in the water sector: 2. J. Hydroinform. 12, 225–239. doi: 10.2166/hydro.2009.065
Abbott, M. B., and Vojinovic, Z. (2014). Towards a hydroinformatics praxis in the service of social justice. J. Hydroinform. 16, 516–530. doi: 10.2166/hydro.2013.198
Abdullaev, I., and Mollinga, P. P. (2010). The socio-technical aspects of water management: emerging trends at grass roots level in Uzbekistan. Water 2, 85–100. doi: 10.3390/w2010085
Abdullaev, I., and Rakhmatullaev, S. (2014). Data management for integrated water resources management in Central Asia. J. Hydroinform. 16, 1425–1440. doi: 10.2166/hydro.2014.097
Al-Jawad, J. Y., Alsaffar, H. M., Bertram, D., and Kalin, R. M. (2019). A comprehensive optimum integrated water resources management approach for multidisciplinary water resources management problems. J. Environ. Manage. 239, 211–224. doi: 10.1016/j.jenvman.2019.03.045
Allahyari, M. S. (2009). Agricultural sustainability: Implications for extension systems. Afr. J. Agric. Res. 4, 781–786.
Almoradie, A., Jonoski, A., Popescu, I., and Solomatime, D. (2013). Web Based Access to Water Related Data Using OGC WaterML 2.0. International Journal of Advanced Computer Science and Applications (IJACSA), EnviroGRIDS Special Issue on–Building a Regional Observation System in the Black Sea Catchment, 83-89.
Alvarez, J., and Nuthall, P. (2006). Adoption of computer based information systems: the case of dairy farmers in Canterbury, NZ, and Florida, Uruguay. Comput. Electron. Agric. 50, 48–60. doi: 10.1016/j.compag.2005.08.013
Antle, J. M., Jones, J. W., and Rosenzweig, C. E. (2017). Next generation agricultural system data, models and knowledge products: Introduction. Agric. Syst. 155, 186–190. doi: 10.1016/j.agsy.2016.09.003
Beran, B., and Piasecki, M. (2009). Engineering new paths to water data. Comput. Geosci. 35, 753–760. doi: 10.1016/j.cageo.2008.02.017
Berger, R., and Hovav, A. (2013). Using a dairy management information system to facilitate precision agriculture: the case of the AfiMilk® system. Inform. Syst. Manage. 30, 21–34. doi: 10.1080/10580530.2013.739885
Bernauer, T., Böhmelt, T., Buhaug, H., Gleditsch, N. P., Tribaldos, T., Weibust, E. B., et al. (2012). Water-related intrastate conflict and cooperation (WARICC): a new event dataset. Int. Interact. 38, 529–545. doi: 10.1080/03050629.2012.697428
Berthiaume, P., Bigras-Poulin, M., and Rousseau, A. N. (2005). Dynamic simulation model of nitrogen fluxes in swine housing and outdoor storage facilities. Biosyst. Eng. 92, 453–467. doi: 10.1016/j.biosystemseng.2005.08.008
Biswas, A. K., and Dune, R. W. (1971). Sociological aspects of water development. JAWRA 7, 1137–1143. doi: 10.1111/j.1752-1688.1971.tb05051.x
Bjornlund, V., and Bjornlund, H. (2019). Understanding agricultural water management in a historical context using a socioeconomic and biophysical framework. Agric. Water Manage. 213, 454–467. doi: 10.1016/j.agwat.2018.10.037
Brodaric, B., Booth, N., Boisvert, E., and Lucido, J. (2016). Groundwater data network interoperability. J. Hydroinform. 18, 210–225. doi: 10.2166/hydro.2015.242
Brodaric, B., and Hahmann, T. (2014). “Toward a foundational hydro ontology for water data interoperability,” in Proceedings of the 11th International Conference on Hydroinformatics, 1–5.
Burkholder, J., Libra, B., Weyer, P., Heathcote, S., Kolpin, D., Thorne, P. S., et al. (2006). Impacts of waste from concentrated animal feeding operations on water quality. Environ. Health Perspect. 115, 308–312. doi: 10.1289/ehp.8839
Calicioglu, O., Flammini, A., Bracco, S., Bellù, L., and Sims, R. (2019). The future challenges of food and agriculture: an integrated analysis of trends and solutions. Sustainability 11:222. doi: 10.3390/su11010222
Capalbo, S. M., Antle, J. M., and Seavert, C. (2017). Next generation data systems and knowledge products to support agricultural producers and science-based policy decision making. Agric. Syst. 155, 191–199. doi: 10.1016/j.agsy.2016.10.009
Caron, J., Pelletier, V., Kennedy, C. D., Gallichand, J., Gumiere, S., Bonin, S., et al. (2017). Guidelines of irrigation and drainage management strategies to enhance cranberry production and optimize water use in North America. Can. J. Soil Sci. 97, 82–91. doi: 10.1139/CJSS-2016-0086
Celicourt, P., and Piasecki, M. (2016). HydroUnits: supporting dimensional analysis in hydrologic computing systems using sensor-based standards. J. Hydroinform. 18, 168–184. doi: 10.2166/hydro.2015.075
Celicourt, P., Sam, R., and Piasecki, M. (2020). Rapid prototyping of an automated sensor-to-server environmental data acquisition system using a FAIR-based approach (Manuscript submitted for publication).
Chartzoulakis, K., and Bertaki, M. (2015). Sustainable water management in agriculture under climate change. Agric. Agric. Sci. Proc. 4, 88–98. doi: 10.1016/j.aaspro.2015.03.011
Chaves, L. F., Ramoni-Perazzi, P., Lizano, E., and Añez, N. (2003). Morphometrical changes in eggs of Rhodnius prolixus (Heteroptera: Reduviidae) during development. Entomotropica 18, 83–88.
Chedid, M., Jaber, L. S., Giger-Reverdin, S., Duvaux-Ponter, C., and Hamadeh, S. K. (2014). Water stress in sheep raised under arid conditions. Can. J. Anim. Sci. 94, 243–257. doi: 10.4141/cjas2013-188
Ciais, P., Reichstein, M., Viovy, N., Granier, A., Ogée, J., Allard, V., et al. (2005). Europe-wide reduction in primary productivity caused by the heat and drought in 2003. Nature 437, 529–533. doi: 10.1038/nature03972
Coble, K. H., Mishra, A. K., Ferrell, S., and Griffin, T. (2018). Big data in agriculture: a challenge for the future. Appl. Econ. Perspect. Policy 40, 79–96. doi: 10.1093/aepp/ppx056
Cunge, J. A., and Erlich, M. (1999). Hydroinformatics in 1999: what is to be done? J. Hydroinform. 1, 21–31. doi: 10.2166/hydro.1999.0003
de Andrade, J. G. P., Barbosa, P. S. F., Souza, L. C. A., and Makino, D. L. (2011). Interbasin water transfers: the Brazilian experience and international case comparisons. Water Resour. Manage. 25, 1915–1934. doi: 10.1007/s11269-011-9781-6
De Montis, A., Modica, G., and Arcidiacono, C. (2017) “AgInformatics,” in Encyclopedia of Big Data, eds L. Schintler and C. McNeely (Cham: Springer), 1–4.
De Stefano, L., Petersen-Perlman, J. D., Sproles, E. A., Eynard, J., and Wolf, A. T. (2017). Assessment of transboundary river basins for potential hydro-political tensions. Global Environ. Change 45, 35–46. doi: 10.1016/j.gloenvcha.2017.04.008
Deng, X., Li, F., Zhao, Y., and Li, S. (2018). Regulation of deep groundwater based on MODFLOW in the water intake area of the South-to-North Water Transfer Project in Tianjin, China. J. Hydroinform. 20, 989–1007. doi: 10.2166/hydro.2018.126
Ding, K., Chan, F. T., Zhang, X., Zhou, G., and Zhang, F. (2019). Defining a digital twin-based cyber-physical production system for autonomous manufacturing in smart shop floors. Int. J. Product. Res. 57, 6315–6334. doi: 10.1080/00207543.2019.1566661
DiSalvo, C., Sengers, P., and Brynjarsdóttir, H. (2010). “Mapping the landscape of sustainable HCI,” in Proceedings of the SIGCHI Conference on Human Factors in Computing Systems (Atlanta, GA), 1975–1984.
D'Odorico, P., Laio, F., and Ridolfi, L. (2010). Does globalization of water reduce societal resilience to drought? Geophys. Res. Lett. 37:L13403. doi: 10.1029/2010GL043167
El Bilali, H. (2018). Transition heuristic frameworks in research on agro-food sustainability transitions. Environ. Dev. Sustain. 22, 1–36. doi: 10.1007/s10668-018-0290-0
El Bilali, H., and Allahyari, M. S. (2018). Transition towards sustainability in agriculture and food systems: role of information and communication technologies. Inform. Process. Agric. 5, 456–464. doi: 10.1016/j.inpa.2018.06.006
Elshafei, Y., Sivapalan, M., Tonts, M., and Hipsey, M. R. (2014). A prototype framework for models of sociohydrology: identification of key feedback loops and parameterisation approach. Hydrol. Earth Syst. Sci. 18, 2141–2166. doi: 10.5194/hess-18-2141-2014
Evans, R. G., and Sadler, E. J. (2008). Methods and technologies to improve efficiency of water use. Water Resources Res. 44:W00E04. doi: 10.1029/2007WR006200
Evers, M., Höllermann, B., Almoradie, A. D. S., Garcia Santos, G., and Taft, L. (2017). The pluralistic water research concept: a new human-water system research approach. Water 9:933. doi: 10.3390/w912093
FAO (2017). The State of Food and Agriculture 2017. Leveraging Food Systems for Inclusive Rural Transformation. Rome: FAO. Available online at: http://www.fao.org/3/a-I7658e.pdf (accessed August 5, 2019).
Foster, T., Brozović, N., Butler, A. P., Neale, C. M. U., Raes, D., Steduto, P., et al. (2017). AquaCrop-OS: An open source version of FAO's crop water productivity model. Agric. Water Manage. 181, 18–22. doi: 10.1016/j.agwat.2016.11.015
Fountas, S., Carli, G., Sørensen, C. G., Tsiropoulos, Z., Cavalaris, C., Vatsanidou, A., et al. (2015a). Farm management information systems: current situation and future perspectives. Comput. Electron. Agric. 115, 40–50. doi: 10.1016/j.compag.2015.05.011
Fountas, S., Sorensen, C. G., Tsiropoulos, Z., Cavalaris, C., Liakos, V., and ans Gemtos, T. (2015b). Farm machinery management information system. Comput. Electron. Agric. 110, 131–138. doi: 10.1016/j.compag.2014.11.011
Fournel, S., Rousseau, A. N., and Laberge, B. (2017). Rethinking environment control strategy of confined animal housing systems through precision livestock farming. Biosyst. Eng. 155, 96–123. doi: 10.1016/j.biosystemseng.2016.12.005
Furbank, R. T., and Tester, M. (2011). Phenomics-technologies to relieve the phenotyping bottleneck. Trends Plant Sci. 16, 635–644. doi: 10.1016/j.tplants.2011.09.005
Furlong, C., Guthrie, L., De Silva, S., and Considine, R. (2015). Analysing the terminology of integration in the water management field. Water Policy 17, 46–60. doi: 10.2166/wp.2014.185
Garnett, T., Appleby, M. C., Balmford, A., Bateman, I. J., Benton, T. G., Bloomer, P., et al. (2013). Sustainable intensification in agriculture: premises and policies. Science 341, 33–34. doi: 10.1126/science.1234485
Garrido, J., Requena, I., and Mambretti, S. (2012). Semantic model for flood management. J. Hydroinform. 14, 918–936. doi: 10.2166/hydro.2012.064
Geels, F. W. (2010). Ontologies, sociotechnical transitions (to sustainability), and the multi-level perspective. Res. Policy 39, 495–510. doi: 10.1016/j.respol.2010.01.022
Giovannucci, D., Scherr, S. J., Nierenberg, D., Hebebrand, C., Shapiro, J., Milder, J., et al. (2012). “Food and Agriculture: the future of sustainability,” in The Sustainable Development in the 21st Century (SD21) Report for Rio. New York, NY: United Nations, Department of Economic and Social Affairs, Division for Sustainable Development, 20.
Gleeson, T., Wang-Erlandsson, L., Zipper, S. C., Porkka, M., Jaramillo, F., Gerten, D., et al. (2020). The water planetary boundary: interrogation and revision. One Earth 2, 223–234. doi: 10.1016/j.oneear.2020.02.009
Gregersen, J. B., Gijsbers, P. J. A., and Westen, S. J. P. (2007). OpenMI: Open modelling interface. J. Hydroinform. 9, 175–191. doi: 10.2166/hydro.2007.023
Griffin, T. W., Miller, N. J., Bergtold, J., Shanoyan, A., Sharda, A., and Ciampitti, I. A. (2017). Farm's sequence of adoption of information-intensive precision agricultural technology. Appl. Eng. Agric. 33:521. doi: 10.13031/aea.12228
Gumiere, S. J., Camporese, M., Botto, A., Lafond, J. A., Paniconi, C., Gallichand, J., et al. (2020). Machine learning vs. physics-based modeling for real-time irrigation management. Front. Water 2:8. doi: 10.3389/frwa.2020.00008
Gumiere, S. J., Lafond, J. A., Hallema, D. W., Périard, Y., Caron, J., and Gallichand, J. (2014). Mapping soil hydraulic conductivity and matric potential for water management of cranberry: characterisation and spatial interpolation methods. Biosyst. Eng. 128, 29–40. doi: 10.1016/j.biosystemseng.2014.09.002
Gupta, S. (2009). “Bioinformatics-research applications,” in Bioinformatics: Applications in Life and Environmental Sciences ed M. H. Fulekar (Dordrecht: Springer), 207–214.
Gustafson, A., Erdmann, J., Milligan, M., Onsongo, G., Pardey, P., Prather, T., et al. (2017). “A platform for computationally advanced collaborative agroinformatics data discovery and analysis,” in Proceedings of the Practice and Experience in Advanced Research Computing 2017 on Sustainability, Success and Impact (New Orleans, LA: ACM).
Gutiérrez, F., Htun, N. N., Schlenz, F., Kasimati, A., and Verbert, K. (2019). A review of visualisations in agricultural decision support systems: An HCI perspective. Comput. Electr. Agric. 163:104844. doi: 10.1016/j.compag.2019.05.053
GWP (2000). Integrated Water Resources Management, 22. Stockholm: GWP Secretariat. TAC Background Papers No. 4.
Hanjra, M. A., and Qureshi, M. E. (2010). Global water crisis and future food security in an era of climate change. Food Policy 35, 365–377. doi: 10.1016/j.foodpol.2010.05.006
Hassink, J., Grin, J., and Hulsink, W. (2018). Enriching the multi-level perspective by better understanding agency and challenges associated with interactions across system boundaries. The case of care farming in the Netherlands: multifunctional agriculture meets health care. J. Rural Stud. 57, 186–196. doi: 10.1016/j.jrurstud.2017.12.018
Herrero, M., and Thornton, P. K. (2013). Livestock and global change: emerging issues for sustainable food systems. Proc. Natl. Acad. Sci. U.S.A. 110, 20878–20881. doi: 10.1073/pnas.1321844111
Hoekstra, A. Y. (2017). Water footprint assessment: evolvement of a new research field. Water Resour. Manage. 31, 3061–3081. doi: 10.1007/s11269-017-1618-5
Horsburgh, J. S., Aufdenkampe, A. K., Mayorga, E., Lehnert, K. A., Hsu, L., Song, L., et al. (2016). Observations data model 2: a community information model for spatially discrete Earth observations. Environ. Model. Softw. 79, 55–74. doi: 10.1016/j.envsoft.2016.01.010
Horsburgh, J. S., Caraballo, J., Ramírez, M., Aufdenkampe, A. K., Arscott, D. B., and Damiano, S. G. (2019). Low-cost, open-source, and low-power: but what to do with the data? Front. Earth Sci. 7:67. doi: 10.3389/feart.2019.00067
Horsburgh, J. S., Tarboton, D. G., Maidment, D. R., and Zaslavsky, I. (2008). A relational model for environmental and water resources data. Water Resour. Res. 44:W05406. doi: 10.1029/2007WR006392
Houle, D., Govindaraju, D. R., and Omholt, S. (2010). Phenomics: the next challenge. Nat. Rev. Genet. 11:855. doi: 10.1038/nrg2897
Hsu, L., Mayorga, E., Horsburgh, J., Carter, M., Lehnert, K., and Brantley, S. (2017). Enhancing interoperability and capabilities of earth science data using the Observations Data Model 2 (ODM2). Data Sci. J. 16, 1–16. doi: 10.5334/dsj-2017-004
Hughes, T. P. (1987). “The evolution of large technological systems,” in The Social Construction of Technological Systems: New Directions in the Sociology and History of Technology, eds W. Bijker, T. Hughes, and T. Pinch (Cambridge, MA: MIT Press), 51.
Ingram, J. (2015). Framing niche-regime linkage as adaptation: an analysis of learning and innovation networks for sustainable agriculture across Europe. J. Rural Stud. 40, 59–75. doi: 10.1016/j.jrurstud.2015.06.003
Islam, A. K. M., and Piasecki, M. (2006). A generic metadata description for hydrodynamic model data. J. Hydroinform. 8, 141–148. doi: 10.2166/hydro.2006.017b
Jägermeyr, J., Gerten, D., Schaphoff, S., Heinke, J., Lucht, W., and Rockström, J. (2016). Integrated crop water management might sustainably halve the global food gap. Environ. Res. Lett. 11:025002. doi: 10.1088/1748-9326/11/2/025002
Jakku, E., Taylor, B., Fleming, A., Mason, C., Fielke, S., Sounness, C., and Thorburn, P. (2019). “If they don't tell us what they do with it, why would we trust them?” Trust, transparency and benefit-sharing in Smart Farming. NJAS-Wageningen J Life Sci. 90:100285. doi: 10.1016/j.njas.2018.11.002
Janssen, S. J., Porter, C. H., Moore, A. D., Athanasiadis, I. N., Foster, I., Jones, J. W., et al. (2017). Towards a new generation of agricultural system data, models and knowledge products: information and communication technology. Agric. Syst. 155, 200–212. doi: 10.1016/j.agsy.2016.09.017
Jones, J. W., Antle, J. M., Basso, B., Boote, K. J., Conant, R. T., Foster, I., et al. (2017). Toward a new generation of agricultural system data, models, and knowledge products: state of agricultural systems science. Agric. Syst. 155, 269–288. doi: 10.1016/j.agsy.2016.09.021
Jones, J. W., Hoogenboom, G., Porter, C. H., Boote, K. J., Batchelor, W. D., Hunt, L. A., et al. (2003). The DSSAT cropping system model. Eur. J. Agron. 18, 235–265. doi: 10.1016/S1161-0301(02)00107-7
Kaloxylos, A., Eigenmann, R., Teye, F., Politopoulou, Z., Wolfert, S., Shrank, C., et al. (2012). Farm management systems and the Future Internet era. Comput. Electron. Agric. 89, 130–144. doi: 10.1016/j.compag.2012.09.002
Kamilaris, A., Kartakoullis, A., and Prenafeta-Bold, F. X. (2017). A review on the practice of big data analysis in agriculture. Comput. Electron. Agric. 143, 23–37. doi: 10.1016/j.compag.2017.09.037
Kandasamy, J., Sounthararajah, D., Sivabalan, P., Chanan, A., Vigneswaran, S., and Sivapalan, M. (2014). Socio-hydrologic drivers of the pendulum swing between agricultural development and environmental health: a case study from Murrumbidgee River basin, Australia. Hydrol. Earth Syst. Sci. 18, 1027–1041. doi: 10.5194/hess-18-1027-2014
Kilelu, C. W., Klerkx, L., and Leeuwis, C. (2013). Unravelling the role of innovation platforms in supporting co-evolution of innovation: Contributions and tensions in a smallholder dairy development programme. Agric. Syst. 118, 65–77. doi: 10.1016/j.agsy.2013.03.003
Killham, K. (2011). Integrated soil management-moving towards globally sustainable agriculture. J. Agric. Sci. 149, 29–36. doi: 10.1017/S0021859610000845
Knox, J. W., Kay, M. G., and Weatherhead, E. K. (2012). Water regulation, crop production, and agricultural water management-Understanding farmer perspectives on irrigation efficiency. Agric. Water Manage. 108, 3–8. doi: 10.1016/j.agwat.2011.06.007
Konar, M., Dalin, C., Suweis, S., Hanasaki, N., Rinaldo, A., and Rodriguez-Iturbe, I. (2011). Water for food: the global virtual water trade network. Water Resour. Res. 47:W05520. doi: 10.1029/2010WR010307
Kotir, J. H., Smith, C., Brown, G., Marshall, N., and Johnstone, R. (2016). A system dynamics simulation model for sustainable water resources management and agricultural development in the Volta River Basin, Ghana. Sci. Tot. Environ. 573, 444–457. doi: 10.1016/j.scitotenv.2016.08.081
Kruize, J. W., Robbemond, R. M., Scholten, H., Wolfert, J., and Beulens, A. J. M. (2013). Improving arable farm enterprise integration-review of existing technologies and practices from a farmer's perspective. Comput. Electron. Agric. 96, 75–89. doi: 10.1016/j.compag.2013.04.017
Kummu, M., De Moel, H., Porkka, M., Siebert, S., Varis, O., and Ward, P. J. (2012). Lost food, wasted resources: global food supply chain losses and their impacts on freshwater, cropland, and fertiliser use. Sci. Tot. Environ. 438, 477–489. doi: 10.1016/j.scitotenv.2012.08.092
Larson, S. J., Capel, P. D., and Majewski, M. S. (1997). Pesticides in Surface Waters: Distribution, Trends, and Governing Factors. Chelsea, MI: Ann Arbor Press. 373.
Lawes, R. A., and Robertson, M. J. (2011). Whole farm implications on the application of variable rate technology to every cropped field. Field Crops Res. 124, 142–148. doi: 10.1016/j.fcr.2011.01.002
Lindblom, J., Lundström, C., Ljung, M., and Jonsson, A. (2017). Promoting sustainable intensification in precision agriculture: review of decision support systems development and strategies. Precis. Agric. 18, 309–331. doi: 10.1007/s11119-016-9491-4
Liu, D., Tian, F., Lin, M., and Sivapalan, M. (2015). A conceptual socio-hydrological model of the co-evolution of humans and water: case study of the Tarim River basin, western China. Hydrol. Earth Syst. Sci. 19, 1035–1054. doi: 10.5194/hess-19-1035-2015
Liu, Q., Bai, Q., Kloppers, C., Fitch, P., Bai, Q., Taylor, K., et al. (2013). An ontology-based knowledge management framework for a distributed water information system. J. Hydroinform. 15, 1169–1188. doi: 10.2166/hydro.2012.152
Lokuge, S., Sedera, D., Atapattu, M., and Samaranayaka, D. (2016). “Exploring the role of IS in agriculture: creating an agenda towards agri-informatics,” in Pacific Asia Conference On Information Systems (PACIS) (Chiayi City: Association For Information System).
López-Riquelme, J. A., Pavón-Pulido, N., Navarro-Hellín, H., Soto-Valles, F., and Torres-Sánchez, R. (2017). A software architecture based on FIWARE cloud for Precision Agriculture. Agric. Water Manage. 183, 123–135. doi: 10.1016/j.agwat.2016.10.020
Lundström, C., and Lindblom, J. (2018). Considering farmers' situated knowledge of using agricultural decision support systems (AgriDSS) to Foster farming practices: the case of CropSAT. Agric. Syst. 159, 9–20. doi: 10.1016/j.agsy.2017.10.004
MacDonald, G. K., Brauman, K. A., Sun, S., Carlson, K. M., Cassidy, E. S., Gerber, J. S., et al. (2015). Rethinking agricultural trade relationships in an era of globalization. BioScience 65, 275–289. doi: 10.1093/biosci/biu225
Makropoulos, C., and Savić, D. A. (2019). Urban hydroinformatics: past, present and future. Water 11:1959. doi: 10.3390/w11101959
Maneta, M. P., Torres, M., Wallender, W. W., Vosti, S., Kirby, M., Bassoi, L. H., et al. (2009). Water demand and flows in the São Francisco River Basin (Brazil) with increased irrigation. Agric. Water Manage. 96, 1191–1200. doi: 10.1016/j.agwat.2009.03.008
Markard, J., Raven, R., and Truffer, B. (2012). Sustainability transitions: an emerging field of research and its prospects. Res. Policy 41, 955–967. doi: 10.1016/j.respol.2012.02.013
Martin, R. C. (2002). Agile Software Development: Principles, Patterns, and Practices. Upper Saddle River, NJ: Prentice Hall.
McCown, R. L. (2002). Changing systems for supporting farmers' decisions: Problems, paradigms, and prospects. Agric Syst, 74, 179–220. doi: 10.1016/S0308-521X(02)00026-4
McCown, R. L., Carberry, P. S., Hochman, Z., Dalgliesh, N. P., and Foale, M. A. (2009). Re-inventing model-based decision support with Australian dryland farmers. 1. Changing intervention concepts during 17 years of action research. Crop Past. Sci. 60, 1017–1030. doi: 10.1071/CP08455
Moore, G. A. (1991). Crossing the Chasm: Marketing and Selling High-Tech Goods to Mainstream Customers. New York, NY: Harper Business.
Nikkilä, R., Seilonen, I., and Koskinen, K. (2010). Software architecture for farm management information systems in precision agriculture. Comput. Electron. Agric. 70, 328–336. doi: 10.1016/j.compag.2009.08.013
Noonan, R. E. (1985). An algorithm for generating abstract syntax trees. Comput. Lang. 10, 225–236. doi: 10.1016/0096-0551(85)90018-9
Ojha, T., Misra, S., and Raghuwanshi, N. S. (2015). Wireless sensor networks for agriculture: the state-of-the-art in practice and future challenges. Comput. Electron. Agric. 118, 66–84. doi: 10.1016/j.compag.2015.08.011
Osakabe, Y., Osakabe, K., Shinozaki, K., and Tran, L. S. P. (2014). Response of plants to water stress. Front. Plant Sci. 5:86. doi: 10.3389/fpls.2014.00086
Paraforos, D. S., Vassiliadis, V., Kortenbruck, D., Stamkopoulos, K., Ziogas, V., Sapounas, A. A., et al. (2017). Multi-level automation of farm management information systems. Comput. Electron. Agric. 142, 504–514. doi: 10.1016/j.compag.2017.11.022
Pasquier, T., Lau, M. K., Trisovic, A., Boose, E. R., Couturier, B., Crosas, M., et al. (2017). If these data could talk. Sci. Data 4:170114. doi: 10.1038/sdata.2017.114
Pelletier, V., Gallichand, J., Gumiere, S., Pepin, S., and Caron, J. (2015). Water table control for increasing yield and saving water in cranberry production. Sustainability 7, 10602–10619. doi: 10.3390/su70810602
Peng, B., Guan, K., Tang, J., Ainsworth, E. A., Asseng, S., Bernacchi, C. J., et al. (2020). Towards a multiscale crop modelling framework for climate change adaptation assessment. Nat. Plants 6, 338–348. doi: 10.1038/s41477-020-0625-3
Perea, R. G., Daccache, A., Díaz, J. R., Poyato, E. C., and Knox, J. W. (2018). Modelling impacts of precision irrigation on crop yield and in-field water management. Precis. Agric. 19, 497–512. doi: 10.1007/s11119-017-9535-4
Pretty, J., Benton, T. G., Bharucha, Z. P., Dicks, L. V., Flora, C. B., Godfray, H. C. J., et al. (2018). Global assessment of agricultural system redesign for sustainable intensification. Nat. Sustain. 1:441. doi: 10.4324/9781138638044
Roman, P. (2017). The São Francisco interbasin water transfer in Brazil: tribulations of a megaproject through constraints and controversy. Water Altern. 10, 395–419. Available online at: http://www.water-alternatives.org/
Rose, D. C., Keating, C., and Morris, C. (2018b). Understanding How to Influence Farmers' Decision-Making Behaviour: A Social Science Literature Review, Report for the Agriculture and Horticulture Development Board, Supported by UEA Consulting Ltd. Available online at: https://ahdb.org.uk/knowledge-library/understand-how-to-influence-farmers-decision-making-behaviour (accessed April 25, 2020).
Rose, D. C., Parker, C., Fodey, J., Park, C., Sutherland, W. J., and Dicks, L. V. (2018a). Involving stakeholders in agricultural decision support systems: improving user-centred design. Int. J. Agric. Manag. 6, 80–89. doi: 10.5836/ijam/2017-06-80
Rossi, V., Salinari, F., Poni, S., Caffi, T., and Bettati, T. (2014). Addressing the implementation problem in agricultural decision support systems: the example of vite. net®. Comput. Electron. Agric. 100, 88–99. doi: 10.1016/j.compag.2013.10.011
Rousseau, A. N., Mailhot, A., Quilbé, R., and Villeneuve, J. P. (2005). Information technologies in a wider perspective: integrating management functions across the urban-rural interface. Environ. Model. Softw. 20, 443–455. doi: 10.1016/j.envsoft.2004.02.008
Rousseau, A. N., Mailhot, A., Turcotte, R., Duchemin, M., Blanchette, C., Roux, M., et al. (2000). GIBSI: an integrated modelling system prototype for river basin management. Hydrobiologia 422/423, 465–475. doi: 10.1023/A:1017030618572
Rupnik, R., Kukar, M., Vračar, P., Košir, D., Pevec, D., and Bosnić, Z. (2019). AgroDSS: A decision support system for agriculture and farming. Comput. Electron. Agric. 161, 260–271. doi: 10.1016/j.compag.2018.04.001
Sandler, H. A., DeMoranville, C. J., and Autio, W. R. (2004). Economic comparison of initial vine density, nitrogen rate, and weed management strategy in commercial cranberry. HortTechnology 14, 267–274. doi: 10.21273/HORTTECH.14.2.0267
Schadow, G., and McDonald, C. J. (2013). The Unified Code for Units of Measure, version 2.1. Regenstrief Institute and the UCUM Organization. Available online at: https://ucum.org/ucum.html (accessed on June 18, 2020).
Sharma, B., Molden, D., and Cook, S. (2015). “Water use efficiency in agriculture: measurement, current situation and trends,” in Managing Water and Fertilizer for Sustainable Agricultural Intensification, eds P. Drechsel, P. Heffer, H. Magen, R. Mikkelsen, R., and D. Wichelns [Paris; Colombo; Horgen: International Fertilizer Industry Association (IFA); International Water Management Institute (IWMI); International Plant Nutrition Institute (IPNI); International Potash Institute (IPI)], 39–64.
Shumilova, O., Tockner, K., Thieme, M., Koska, A., and Zarfl, C. (2018). Global water transfer megaprojects: a potential solution for the water-food-energy nexus? Front. Environ. Sci. 6:150. doi: 10.3389/fenvs.2018.00150
Sims, J. T., and Wolf, D. C. (1994). “Poultry waste management: agricultural and environmental issues,” in Advances in Agronomy, Vol. 52 ed D. L. Sparks (New York, NY: Academic Press), 1–83.
Simunek, J., Sejna, M., and Van Genuchten, M. T. (1999). The HYDRUS-2D Software Package. International Ground Water modelling Center.
Singh, A. (2014). Simulation-optimization modelling for conjunctive water use management. Agric. Water Manage. 141, 23–29. doi: 10.1016/j.agwat.2014.04.003
Sivapalan, M., Savenije, H. H., and Blöschl, G. (2012). Socio-hydrology: a new science of people and water. Hydrol. Process. 26, 1270–1276. doi: 10.1002/hyp.8426
Sørensen, C. G., Fountas, S., Nash, E., Pesonen, L., Bochtis, D., Pedersen, S. M., et al. (2010). Conceptual model of a future farm management information system. Comput. Electron. Agric. 72, 37–47. doi: 10.1016/j.compag.2010.02.003
Srinivasan, V., Sanderson, M., Garcia, M., Konar, M., Blöschl, G., and Sivapalan, M. (2017). Prediction in a socio-hydrological world. Hydrol. Sci. J. 62, 338–345.
Suprem, A., Mahalik, N., and Kim, K. (2013). A review on application of technology systems, standards and interfaces for agriculture and food sector. Comput. Stand. Interfaces 35, 355–364. doi: 10.1016/j.csi.2012.09.002
Ten Veldhuis, J. A. E. (2011). How the choice of flood damage metrics influences urban flood risk assessment. J. Flood Risk Manage. 4, 281–287. doi: 10.1111/j.1753-318X.2011.01112.x
Thorkilsen, M., and Dynesen, C. (2001). An owner's view of hydroinformatics: its role in realising the bridge and tunnel connection between Denmark and Sweden. J. Hydroinform. 3, 105–135. doi: 10.2166/hydro.2001.0011
Tuberosa, R., and Salvi, S. (2006). Genomics-based approaches to improve drought tolerance of crops. Trends Plant Sci. 11, 405–412. doi: 10.1016/j.tplants.2006.06.003
van Emmerik, T. H. M., Li, Z., Sivapalan, M., Pande, S., Kandasamy, J., Savenije, H. H. G., et al. (2014). Socio-hydrologic modeling to understand and mediate the competition for water between agriculture development and environmental health: Murrumbidgee River basin, Australia. Hydrol. Earth Syst. Sci. 18, 4239–4259. doi: 10.5194/hess-18-4239-2014
van Huis, A., and Oonincx, D. G. (2017). The environmental sustainability of insects as food and feed. A review. Agron. Sustain. Dev. 37:43. doi: 10.1007/s13593-017-0452-8
Vanderleest, C. P., Caron, J., and Bland, W. L. (2016). Water table level management as an irrigation strategy for cranberry (Vaccinium macrocarpon Aiton). Can. J. Soil Sci. 97, 11–19. doi: 10.1139/cjss-2016-0001
Vanuytrecht, E., Raes, D., Steduto, P., Hsiao, T. C., Fereres, E., Heng, L. K., et al. (2014). AquaCrop: FAO's crop water productivity and yield response model. Environ. Model. Softw. 62, 351–360. doi: 10.1016/j.envsoft.2014.08.005
Vojinovic, Z., and Abbott, M. B. (2017). Twenty-five years of hydroinformatics. Water 9:59. doi: 10.3390/w9010059
Vojinovic, Z., Hammond, M., Golub, D., Hirunsalee, S., Weesakul, S., Meesuk, V., et al. (2016). Holistic approach to flood risk assessment in areas with cultural heritage: a practical application in Ayutthaya, Thailand. Nat. Hazards 81, 589–616. doi: 10.1007/s11069-015-2098-7
Vojinovic, Z., and Van Teeffelen, J. (2007). An integrated stormwater management approach for small islands in tropical climates. Urban Water J. 4, 211–231. doi: 10.1080/15730620701464190
Walker, W. E., Loucks, D. P., and Carr, G. (2015). Social responses to water management decisions. Environ. Process. 2, 485–509. doi: 10.1007/s40710-015-0083-5
Walters, J. P., Archer, D. W., Sassenrath, G. F., Hendrickson, J. R., Hanson, J. D., Halloran, J. M., et al. (2016). Exploring agricultural production systems and their fundamental components with system dynamics modelling. Ecol. Model. 333, 51–65. doi: 10.1016/j.ecolmodel.2016.04.015
Watkins, K. (2006). Human Development Report 2006-Beyond Scarcity: Power, Poverty and the GlobalWater Crisis. UNDP Human Development Reports, United Nations Development Programme, New York, NY, United States
Wheeler, S., Zuo, A., and Bjornlund, H. (2013). Farmers' climate change beliefs and adaptation strategies for a water scarce future in Australia. Global Environ. Change 23, 537–547. doi: 10.1016/j.gloenvcha.2012.11.008
White, J. W., Hunt, L. A., Boote, K. J., Jones, J. W., Koo, J., Kim, S., et al. (2013). Integrated description of agricultural field experiments and production: the ICASA Version 2.0 data standards. Comput. Electron. Agric. 96, 1–12. doi: 10.1016/j.compag.2013.04.003
Wichelns, D. (2014). Do estimates of water productivity enhance understanding of farm-level water management? Water 6, 778–795. doi: 10.3390/w6040778
Wilkinson, M. D., Dumontier, M., Aalbersberg, I. J., Appleton, G., Axton, M., Baak, A., et al. (2016). The FAIR guiding principles for scientific data management and stewardship. Sci. Data 3. doi: 10.1038/sdata.2016.18
Wolf, A. T., Yoffe, S. B., and Giordano, M. (2003). International waters: identifying basins at risk. Water Policy 5, 29–60. doi: 10.2166/wp.2003.0002
Wolfert, S., Ge, L., Verdouw, C., and Bogaardt, M. J. (2017). Big data in smart farming-a review. Agric. Syst. 153, 69–80. doi: 10.1016/j.agsy.2017.01.023
Wolfert, S., Goense, D., and Sørensen, C. A. G. (2014). “A future internet collaboration platform for safe and healthy food from farm to fork,” in 2014 Annual SRII Global Conference (San Jose, CA), 266–273.
Wong, B. P., and Kerkez, B. (2016). Real-time environmental sensor data: an application to water quality using web services. Environ. Model. Softw. 84, 505–517. doi: 10.1016/j.envsoft.2016.07.020
Xiao, Z., Liang, Z., Li, B., Hu, Y., and Wang, J. (2019). Integrated impact assessment method for the water transfer project on regional development. J. Hydroinform. 21, 638–651. doi: 10.2166/hydro.2019.025
Yu, J., Leighton, B., Car, N., Seaton, S., and Hodge, J. (2015). The eReefs data brokering layer for hydrological and environmental data. J. Hydroinform. 18, 152–167. doi: 10.2166/hydro.2015.165
Ženko, M., and Menga, F. (2019). Linking water scarcity to mental health: hydro-social interruptions in the Lake Urmia Basin, Iran. Water 11:1092. doi: 10.3390/w11051092
Zhang, G., Chakraborty, P., Li, J., Sampathkumar, P., Balasubramanian, T., Kathiresan, K., et al. (2008). Passive atmospheric sampling of organochlorine pesticides, polychlorinated biphenyls, and polybrominated diphenyl ethers in urban, rural, and wetland sites along the coastal length of India. Environ. Sci. Technol. 42, 8218–8223. doi: 10.1021/es8016667
Zhang, N., Wang, M., and Wang, N. (2002). Precision agriculture-a worldwide overview. Comput. Electron. Agric. 36, 113–132. doi: 10.1016/S0168-1699(02)00096-0
Zhu, T., Ringler, C., and Rosegrant, M. W. (2019). Viewing agricultural water management through a systems analysis lens. Water Resour. Res. 55, 1778–1791. doi: 10.1029/2017WR021007
Zhu, X. G., Lynch, J. P., LeBauer, D. S., Millar, A. J., Stitt, M., and Long, S. P. (2016). Plants in silico: why, why now and what? An integrative platform for plant systems biology research. Plant Cell Environ. 39, 1049–1057. doi: 10.1111/pce.12673
Zia, H., Harris, N. R., Merrett, G. V., Rivers, M., and Coles, N. (2013). The impact of agricultural activities on water quality: a case for collaborative catchment-scale management using integrated wireless sensor networks. Comput. Electr. Agric. 96, 126–138. doi: 10.1016/j.compag.2013.05.001
Zipper, S. C., Jaramillo, F., Erlandsson, L. W., Cornell, S., Gleeson, T., Porkka, M., et al. (2020). Integrating the water planetary boundary with water management from local to global scales. Earth's Future. doi: 10.1029/2019EF001377
Keywords: hydroinformatics and agriculture, agricultural sustainability transitions, agricultural technology ecosystem, technology influencer, technology co-evolution, agricultural water management
Citation: Celicourt P, Rousseau AN, Gumiere SJ and Camporese M (2020) Agricultural Hydroinformatics: A Blueprint for an Emerging Framework to Foster Water Management-Centric Sustainability Transitions in Farming Systems. Front. Water 2:586516. doi: 10.3389/frwa.2020.586516
Received: 23 July 2020; Accepted: 25 November 2020;
Published: 23 December 2020.
Edited by:
Heye Reemt Bogena, Helmholtz-Verband Deutscher Forschungszentren (HZ), GermanyReviewed by:
Stefan Rilling, Fraunhofer Society (FHG), GermanyAnusuriya Devaraju, University of Bremen, Germany
Copyright © 2020 Celicourt, Rousseau, Gumiere and Camporese. This is an open-access article distributed under the terms of the Creative Commons Attribution License (CC BY). The use, distribution or reproduction in other forums is permitted, provided the original author(s) and the copyright owner(s) are credited and that the original publication in this journal is cited, in accordance with accepted academic practice. No use, distribution or reproduction is permitted which does not comply with these terms.
*Correspondence: Paul Celicourt, cGF1bC5jZWxpY291cnQuMUB1bGF2YWwuY2E=