- 1Department of Fisheries and Aquatic Sciences, University of Eldoret, Eldoret, Kenya
- 2Department of Fisheries and Aquatic Sciences, Mzuzu University, Mzuzu, Malawi
- 3Département of Chemistry and Physics, Institut Supérieur Pédagogique de Bukavu (ISP/Bukavu), Bukavu, Republic of the Congo
Factors influencing the spatio-temporal dynamics of plankton communities in small tropical lakes are not well-understood. This study assessed plankton communities in response to spatial (six sampling sites) and seasonal (wet vs. dry seasons) changes in environmental variables in Lake Kanyaboli, a small satellite lake on the northern shores of Lake Victoria, Kenya. Water quality variables, including pH, conductivity (EC), dissolved oxygen (DO), temperature, Secchi depth (SD), nitrates (), nitrites (), ammonium (), soluble reactive phosphorus (SRP), total nitrogen (TN), total phosphorus (TP), and chlorophyll-a (Chl-a), were monitored monthly at six sites spread throughout the lake for 1 year. Phytoplankton and zooplankton samples were collected and analyzed for taxon composition and abundance. Two-way ANOVA showed no significant interaction between site and season for all variables. Likewise, there were no significant spatial differences for all variables except Chl- a. A t-test showed significant seasonal differences in SD, DO, , , , and TN. Thirty phytoplankton genera were identified belonging to Bacillariophyceae, Chlorophyceae, Cryptophyceae, Cyanophyceae, Euglenoidae, Trebouxiophyceae, and Zygnematophyceae, with Chlorophyceae being the most dominant (42.30%). Zooplankton comprised of 15 genera, belonging to Copepoda (55.4%), Rotifera (27.9%), and Cladocera (16.7%). Two-way ANOVA for plankton abundance showed no significant interaction between site and season, but there were significant differences in community composition between the wet and dry seasons. Canonical correspondence analysis identified water clarity (Secchi depth) and concentrations of dissolved fractions of nitrogen and phosphorus as the major water quality variables driving variation in the composition of plankton communities in the lake. This study showed that seasonality was a major driver of changes in plankton community composition between dry and wet seasons through changes in the concentrations of nutrients (, , , TN, and TP). Lake Kanyaboli's phytoplankton community indicated a non-equilibrial state, perhaps due to short residence times of water, especially during the wet season, and dense macrophytes fringing the lake that increase nutrient uptake and limit the dominance of select phytoplankton species. This study shows the importance of long-term studies covering dry and wet seasons to understand the dynamics of plankton communities and their drivers in small tropical waterbodies to inform management and conservation.
Introduction
Phytoplankton and zooplankton communities link basal resources and higher trophic levels in aquatic food webs (Sindt and Wolf, 2021). Patterns of phytoplankton and zooplankton diversity and abundance in lakes can reflect the composition of materials and elements (nutrients, organic matter, sediments) contributed by influent rivers (Frost and Culver, 2001). Several factors, including temperature, light, the amount of organic matter, electrical conductivity, grazing intensity, concentrations of nutrients, and water mixing influence the production and composition of phytoplankton in aquatic ecosystems (Tian et al., 2013; Jiang et al., 2014; Wang et al., 2015; Hardikar et al., 2017). The concentration of nutrients, especially nitrogen (N) and phosphorus (P), is the principal limiting factor for phytoplankton production in lakes and reservoirs (Wang et al., 2015). Subsequently, phytoplankton composition influences nutrient recycling, grazing rates by zooplankton, and food web dynamics (Cetinić et al., 2006). Similar to phytoplankton, zooplanktons also respond to changes in environmental conditions by changing their behavior, abundance, and community structure in response to changes in environmental conditions and productivity of phytoplankton (Ndebele-Murisa et al., 2010; Jose et al., 2015; Abdul et al., 2016).
Assessment of the spatio-temporal variation in phytoplankton and zooplankton communities in response to changes in environmental variables, both natural and human-induced, is vital for management decisions. The diversity, composition, and abundance of phytoplankton and zooplankton communities have been used as bioindicators of water quality and overall functioning, including the trophic status, of aquatic ecosystems (Pinto-Coelho et al., 2005; George et al., 2012; Thakur et al., 2013; Hemraj et al., 2017; Stamou et al., 2021). Many tropical lakes are now considered eutrophic due to the frequent occurrence of blooms of blue-green algae (cyanobacteria) that threaten water quality and fish production due to nutrient and organic matter loading from catchment areas (Paerl et al., 2011; Onyango et al., 2020; Díaz-Torres et al., 2021). Simiyu et al. (2018) noted that Lake Victoria regularly experiences cyanobacterial blooms in the Nyanza Gulf associated with fish kills and the temporary shutdown of the drinking water supply. The lake has also experienced a shift in its phytoplankton community from diatom and chlorophyte dominance to near-continuous dominance of filamentous and colonial cyanobacteria due to P and N (from N fixation) enrichment and depletion of silica in the water column (Hecky et al., 2010). The impact of cyanobacterial blooms (mainly Microcystis) on fishes includes embryonic hatching perturbations, reduced survival and growth rates, changes in behavior, increased neurotoxicity and osmoregulation, increased liver activity and heart rates, as well as histopathological effects (Rodger et al., 1994; Malbrouck and Kestemont, 2006; Mitsoura et al., 2013; Yu et al., 2021).
Water quality deterioration is common in reservoirs and lakes receiving high loads of suspended solids, organic matter, and nutrients from human activities (Mustapha, 2009; Ling et al., 2017; Lee and Oh, 2018). Agricultural and biomass extractive practices have been linked to changes in water quality and biological communities in tropical lakes and reservoirs (Liu et al., 2011; Moncayo-Estrada et al., 2012; Walumona et al., 2021; Kondowe et al., 2022). Human activities in the catchment areas, characteristics of the drainage basin, and the recipient ecosystem's size are the primary factors controlling the trophic status of lakes and reservoirs (Walker et al., 2007). Changes in land use and land cover have been linked to changes in water quality of inland waters as a result of sediment and nutrient input, which strongly influence the structure of biological communities and overall ecosystem functioning (Catherine et al., 2013; Kibena et al., 2014; Bucak et al., 2018; Leech et al., 2018; Namugize et al., 2018). Small and shallow waterbodies in tropical and subtropical regions are also associated with seasonal changes in wind speed and rainfall, which influence plankton assemblages through water mixing and nutrient loading (Geraldes and Boavida, 2004; Zhu et al., 2014). Seasonal changes also influence Secchi depth (James and Chimney, 2008), rates of flushing and residence times of water (Geraldes and Boavida, 2004; Borges et al., 2008; Srifa et al., 2016; Ismail et al., 2019). Small water bodies are more vulnerable to eutrophication due to the large catchment area to surface area ratio and shallowness (Catherine et al., 2013) despite high rates of water cycling enabled by short residence times (Cardille et al., 2004). This phenomenon may reduce the intensity of algal blooms during the wet season because of increased flushing rates and decreased light levels. However, the resultant increases in nutrient loads enhance the formation of algal blooms after discharge levels decline (Srifa et al., 2016). The existence of dynamic conditions such as discharge, flushing rate, temperature, and limiting nutrients that influence the lake ecosystem is vital to avoid the perpetual occurrence of one or two competing dominant phytoplankton assemblage, leading to an equilibrium state (Komárková and Tavera, 2003). The competitive exclusion principle suggests that the superior competitor species would dominate a constant environment and lead to a low community richness and diversity (Figueredo and Giani, 2009).
Seasonal variations of environmental factors generally result in considerable changes in the structure of aquatic communities. However, limited studies have explored how plankton communities in small water bodies maintain stability, or lack thereof, in response to changes in environmental conditions. Studies have shown that changes in the composition and abundance of phytoplankton and zooplankton communities are linked to fluctuations in environmental factors due to seasonality and human activities (Sommer et al., 1986; Talling, 1987; Pijanowska, 1990; Chang et al., 2003). This is in agreement with the plankton paradox (Hutchinson, 1961), which postulates that most systems exhibit non-equilibrial conditions in terms of community composition due to fluctuations in physical, chemical and biological factors. However, as per the competitive exclusion principle, stable environmental conditions are associated with the dominance of few competitive species leading to low diversity (Figueredo and Giani, 2009). Seasonal changes in environmental conditions are not well-pronounced in tropical regions due to the largely stable temperature, but the potential of seasonality to influence the composition of plankton communities is not extensively studied (Talling, 1987). In deep tropical lakes, the thickness of the diurnal mixed layer is less stable due to higher radiative heating and lower stability (Lewis, 1987; Talling and Lemoalle, 1998). Similar to deep tropical lakes, shallow lakes in tropical regions also experience short-term thermal stratification that is easily destabilized by wind action or convection at night (Figueredo and Giani, 2009; Yang et al., 2018). On the other hand, recurrent seasonal dynamics in temperature, stratification, light, and nutrient availability influence spatio-temporal phytoplankton composition in temperate regions (Arhonditsis et al., 2003; Grover and Chrzanowski, 2006). Therefore, phytoplankton and zooplankton adaptation to changing environmental factors is key to their colonization and succession.
A limited number of studies have investigated the diversity and composition of phytoplankton and zooplankton communities in small water bodies (shallow lakes and reservoirs) in the Afrotropics (Mutune et al., 2005; Wilfred et al., 2005; Babu et al., 2015). However, long-term studies on the relationship between plankton assemblages and environmental factors related to anthropogenic activities are limited, in addition to studies on the equilibrium state of plankton communities in small waterbodies. This scenario is evident across the Afrotropics, leading to the unavailability of long-term data on plankton ecology (Aka et al., 2000; Figueredo and Giani, 2009; Fonseca and de Mattos Bicudo, 2011). Often, emphasis is placed on studies on economic benefits over ecological, conservation and aesthetic benefits deriving from small water bodies. For instance, long-term monitoring studies on ecology and water quality have been done in the large economically important lakes such as Naivasha (Ballot et al., 2009) and Baringo (Walumona et al., 2021), including the African great lakes such as Victoria (Sitoki et al., 2010), Kivu (Hyangya et al., 2021), Malawi (Macuiane et al., 2016), and Tanganyika (Kelly et al., 2017). Recently more attention has been given to cyanobacteria-dominated ecosystems because of compromised and dwindling domestic water supply, ecological and economic consequences linked to eutrophication and fish contamination by algal toxins (Bicudo et al., 2007; Jeppensen et al., 2007; Simiyu et al., 2018; Kimambo et al., 2019). Therefore, long-term studies and constant monitoring of small water bodies are crucial to understanding the influence of human activities on water quality and the structure of aquatic communities to guide management strategies to curb organic pollution and nutrient loading that are major causes of algal blooms that compromise the fisheries and water supply of small water bodies.
This study investigated changes in the composition and diversity of plankton communities in Lake Kanyaboli, a small and shallow lake in western Kenya. The major aim of the study was to understand the major factors influencing the spatial and temporal dynamics of plankton (phytoplankton and zooplankton) communities and their potential use as bioindicators of water quality in tropical lakes. In addition, the phytoplankton equilibrium state of the lake was assessed as described by Sommer et al. (1993), i.e., the phytoplankton community is considered to be at equilibrium when; (i) 1, 2, or 3 species contribute to more than 80% of the biomass, (ii) their existence or coexistence persists for more than 2 weeks, and (iii) during that period the total biomass does not increase significantly. We hypothesized that water quality and plankton communities in Lake Kanyaboli vary more temporally than spatially due to its small size. We also hypothesized that plankton communities in Lake Kanyaboli are not at equilibrium state because the short water residence time in the lake increase flushing of nutrients making them unavailable to phytoplankton, hence regulating the growth of few competitive species (Scheffer et al., 1993). The data generated in this study are essential for understanding drivers of plankton community structure, productivity and the ecological status of small water bodies in tropical and other regions. These data are needed to aid in the development of biomonitoring programs for the management and conservation of small water bodies.
Materials and Methods
Description of the Study Area
The present study was done in Lake Kanyaboli, a small shallow lake with an area of 10.5 km2 and an average depth of 3 m in the northern shores of Lake Victoria. The lake is part of the extensive Yala Swamp, a papyrus-dominated wetland fringing the northern shores of Lake Victoria. Besides Lake Kanyaboli, Yala Swamp also contains Lake Sare (with an area of 5 km2 and an average depth of 5 m) and Lake Namboyo (with an area of 0.01 km2 and an average depth of 17 m) (Angienda et al., 2011). Lake Kanyaboli is the largest among the three, while Lake Namboyo is the smallest but the deepest. Lake Kanyaboli lies between latitudes 0°05S'N and 0°02'N and longitudes 34°09'E and 34°ll'E, (Abila et al., 2008; Opiyo and Dadzie, 2009). The lake is important because it provides refuge to haplochromine species that have been lost from Lake Victoria. Cichlids, including three species of tilapiines (Oreochromis esculentus, Oreochromis variabilis, and Oreochromis leucostictus), Coptodon zillii and several haplochromine species dominate the fish fauna of the lake (Aloo, 2003). Other fish species occurring in the lake include Clarias gariepinus, Protopterus aethiopicus, and Xenoclarias sp. (Aloo, 2003). Lake Kanyaboli also provides livelihoods for riparian communities through fisheries, agriculture and the handicraft industry (Abila, 2005).
The study area is characterized by two wet seasons annually, long rains from March to June and short rains from October to December. Likewise, there is a long dry season from July to September and a shorter one from January to February. Anyona (1997) noted that Lake Kanyaboli is within the lake-shore belt, receiving <1,300 mm annually. The region's climate is influenced by the movements of the inter-tropical zone and its proximity to Lake Victoria.
Lake Kanyaboli and Yala Wetland need the government's protection since Kenya is a signatory to the Ramsar convention; hence it is obligated to ensure the wise use of the wetland regardless of its Ramsar status. Yala Swamp harbors critically endangered sitatunga antelope (Tragecephalus spekeii) in the swamps' dense papyrus that fringes the lake. The swamp is among the sixty (60) crucial Kenyan bird areas, hosting at least 172 bird species, some of which are globally threatened or biome-restricted (CGS (County Government of Siaya), 2015; Muoria et al., 2015). However, the wetland continues to experience anthropogenic stress due to extractive services and agricultural activities, which also affect Lake Kanyaboli.
Lake Kanyaboli has been experiencing water quality changes due to activities in the catchment area and the riparian areas around the Yala Swamp (Kondowe et al., 2022). Major human activities include subsistence and commercial agriculture in the reclaimed part of the swamp besides harvesting papyrus and wood. Part of Yala Swamp, estimated at 2,300 ha, was reclaimed for agriculture in 1965–1970 (Owiyo et al., 2014). The reclamation efforts, which started around 1954, resulted from the high productivity of Yala Swamp. Moreover, reclamation efforts in the past resulted in a lack of water inflow into Lake Kanyaboli due to blockage of the artificial feeder canal. This substantially affected the physico-chemical and biological water quality properties of the lake (Okemwa, 1981; Aloo, 2003; Kinaro, 2008). The most recent activities include commercial farming and further wetland reclamation by Dominion Farms Ltd. (Muoria et al., 2015), which also helped renovate the feeder canals, the retention dike and the outlet canal before its closure after 14 years. Currently, the 9 km feeder canal connecting the Yala River and Lake Kanyaboli is the primary water source for the lake. The feeder canal has an estimated discharge rate of 5 m3/s at maximum capacity (Burgess, 2008), while the lake's volume is estimated at 3.15 × 107 m3 (0.0315 km3). Therefore, the lake's water retention time is estimated at 66.8 days at maximum discharge capacity, although this may vary in real-time because the inflow discharge is regulated. The lake also experiences constant wind blowing over it, which averages 2.14 ms-1 between February 2021 and April 2021 based on Deeper Smart Sonar Pro+ (ITGAM0303, Deeper, UAB, Antakalnio, Lithuania) readings.
Sampling Sites
A total of six stations were evenly distributed around the lake and used for sampling. These included stations in the littoral zone (Gangu, Hotel, Kadenge), open water (Open water), near the inflow (Inlet lake side), and outflow (Outlet lake side) (Figure 1). The littoral and inflow zones had a depth range of 1.13–2.42 m during the dry season and 1.24–2.51 m during the wet season. The open water had a depth of 3.63 m during the wet season, while in the dry season, the depth was 3.32 m. The Outflow zone had a depth of 3.74 m during the wet season and 3.51 m during the dry season.
Sample Collection and Analysis
Samples were collected monthly for 1 year (February 2020 to February 2021), covering the wet (March, April, June, October, November, and December) and dry (January, February, July, August, and September) seasons. At each sampling site and during each sampling occasion, water temperature, pH, Secchi depth (SD), dissolved oxygen concentration (DO), and electrical conductivity (EC) were measured in situ using a YSI multi-probe water quality meter (556 MPS, Yellow Springs Instruments, Ohio, USA). Water samples collected from all the sampling stations were filtered immediately in the field using pre-combusted 0.70 μm GF/F Whatman filters into triplicates of acid-washed HDPE bottles and placed in a cooler box for transport to the laboratory. In addition, unfiltered water samples were collected in acid-washed HDPE bottles in triplicates for analysis of total phosphorus (TP) and total nitrogen (TN). Preservation and transportation of TP and TN samples were done the same way as the water samples for filterable nutrients. Filter papers were placed in a cooler box in the dark at 4°C and transported to the laboratory, where they were kept frozen until chlorophyll-a analysis. All water variables were collected for 1 year except chlorophyll-a, which was recorded for 6 months (June–November 2020).
Phytoplankton and zooplankton samples were collected on the surface (0 m depth) at each sampling site. One liter of water was collected using a conical plankton net (50 μm mesh size) and fixed in situ using 10% Lugol's solution before transport to the laboratory for analysis.
In the laboratory, filterable nutrients (, , , and SRP) and non-filterable nutrients (TP and TN) were analyzed following standard colorimetric methods (APHA, 2017). SRP, , , and were determined using the molybdenum blue method, dichloro-isocyanurate—salicylate method, cadmium reduction method, and azo—dye complex formation, respectively. Conversely, TP was analyzed using the ascorbic acid reduction method, while the persulfate digestion method was used for TN. Chlorophyll-a concentration was analyzed following pigment extraction from the thawed filters using 95% acetone (APHA, 2017).
Before spectrophotometric analysis, chlorophyll-a was extracted using 95% acetone and centrifugation for 15 min at 4,800 rpm in a centrifuge as described by Swanepoel et al. (2008). The absorbance of chlorophyll-a was measured using a spectrophotometer at 750 and 665 nm. The absorbance at 750 nm was subtracted from the other wavelength to obtain a turbidity-corrected value. Acetone (90%) was used as a blank. To calculate the concentration of chlorophyll-a, the formula below by Yang et al. (2007) was used:
Where: Subscripts indicate absorbance before acid addition (Ab) and after acidification (Aa); V1 is volume of solvent (ml); V2 is volume of sample (ml).
Processing of phytoplankton and zooplankton for identification and enumeration was done using sedimentation method according to Swanepoel et al. (2008), Intergovernmental Oceanographic Commission of UNESCO (2010) and Soares et al. (2011). Prior to phytoplankton and zooplankton taxonomic identification (species composition) and counting (abundance), a 10 mL sample was placed in measuring cylinder and allowed to settle by gravity for 24 h then excess water was siphoned off (Swanepoel et al., 2008). After that, the settled sample was homogenized, from which replicate samples of 1 ml were pipetted to slide (covered with coverslip) and examined under an Olympus inverted microscope (CK40, Japan) at ×40 magnification to identify species and enumerate them. The sample on the slide was left for 20 min to settle before identification and enumeration. After enumeration, the plankton abundances (phytoplankton and zooplankton) were extrapolated to 1 L and expressed as individuals per liter (Ind/L). Identification to genus level was done using Prescott (1982) and Bellinger and Sigee (2015) keys for phytoplankton, while Witty (2004) and Dang et al. (2015) keys were used for zooplankton.
Data Analysis
We used a two-way analysis of variance (ANOVA) to test for differences in water physico-chemical variables among the six sampling sites and seasons (dry and wet) with sites and seasons as main factors and site × season interaction term. Two-way ANOVA was also used to compare the total abundance and taxon richness of phytoplankton and zooplankton taxa between seasons (dry and wet) and the six sampling sites with season and sites as the main factors and a season × sites interaction. Where there were no significant seasonal differences, data were pooled, and one-way ANOVA was tested for differences among sites, followed by Tukey multiple post-hoc comparisons of the means. Where there were no significant site differences, data were pooled for all sites, and seasons were compared using two-sample t-tests. Before analysis, count data on plankton abundance were log (x+1) transformed while the rest of the response variables were log-transformed to meet normality assumptions.
Principal Component Analysis (PCA) was used to reduce the dimensionality of the physico-chemistry data. We included two PCs to describe the spatial and temporal (seasonal) changes in water quality. PCAs were statistically assessed using permutational analysis of variance (PERMANOVA), based on Bray-Curtis similarity matrices (McArdle and Anderson, 2001).
Two-way nested analysis of similarities (ANOSIM) was used to compare average rank similarities of phytoplankton and zooplankton communities between the wet and dry seasons, with sampling sites nested within seasons. ANOSIM calculates a test statistic, the R-statistic, which varies between 0 and 1; higher values indicate greater differences between factors. Non-metric multidimensional scaling (NMDS) was then used to visualize the community composition of phytoplankton and zooplankton in different sites and seasons (Clarke and Gorley, 2006) and confirm the differences in ANOSIM. Dissimilarity matrices based on the Bray–Curtis coefficients (Bray and Curtis, 1957) were derived using un-transformed abundances data. The goodness of fit of the ordination was assessed by the magnitude of the associated stress value; a value of <0.2 corresponds to a good ordination (Kashian et al., 2007).
To determine which key phytoplankton and zooplankton taxa were responsible for the differences observed between seasons, hence indicator taxa for changes in water quality, similarity percentages analysis (SIMPER) was used. The percentage contribution of each taxon to the overall dissimilarity per season was quantified. SIMPER is a strictly pairwise analysis between two-factor levels (Clarke and Warwick, 2001), and in this case, comparisons were made between dry and wet seasons.
Canonical correspondence analysis (CCA) was used to investigate the relationship between the plankton communities and environmental variables. The output was displayed as triplots, in which the plotted points for taxa could be related to physico-chemical variables that were represented as rays.
Species occurrence (presence-absence) and distribution data were summarized for each site and the two seasons (wet and dry) using the number of species (S), the total number of individuals, and relative abundance of each species. Several diversity indices were calculated for the whole lake and for each month by summing species-specific abundances across all sites. Shannon's diversity index (H′) was derived as a measure of diversity (Magurran, 2004). The Simpson index (D) (Simpson, 1949) was used as a measure of species richness, and an associated index (E) was used as a measure of evenness. The species diversity indices analyses were performed in PAST version 3.02.
Statistical analyses were performed with R version 3.3.3. (R Development Core, 2017), using the vegan package (Oksanen et al., 2013). Figures were created in MS Office Excel (2016) and R version 3.3.3 (Kilonzo et al., 2014).
Results
Physico-Chemical Characteristics
There were no spatial variations in water quality variables (Figure 2A), but there was a strong seasonal variation in most variables (Figure 2A, Table 1). The PCA biplot of physico-chemistry data indicated that sites were not separated, but seasons were (Figures 1A,B). Principal component 1 (PC 1) and Principal component 2 (PC 2) of the PCA explained 33.0 and 17.1% of the total variation in water quality variables, respectively. The PCA findings suggest a stronger seasonal effect than the spatial effect.
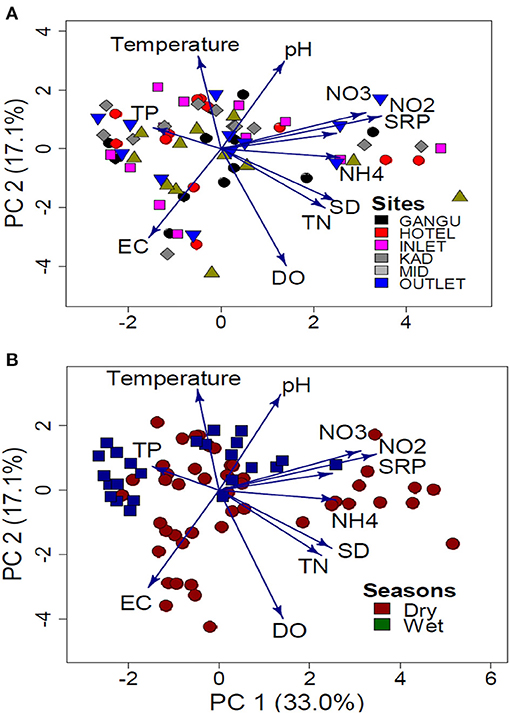
Figure 2. PCA comparison of physico-chemical variables in Lake Kanyaboli based on: (A) site (B) season from February 2020 to February 2021. KAD, Kadenge; MID, Open water.
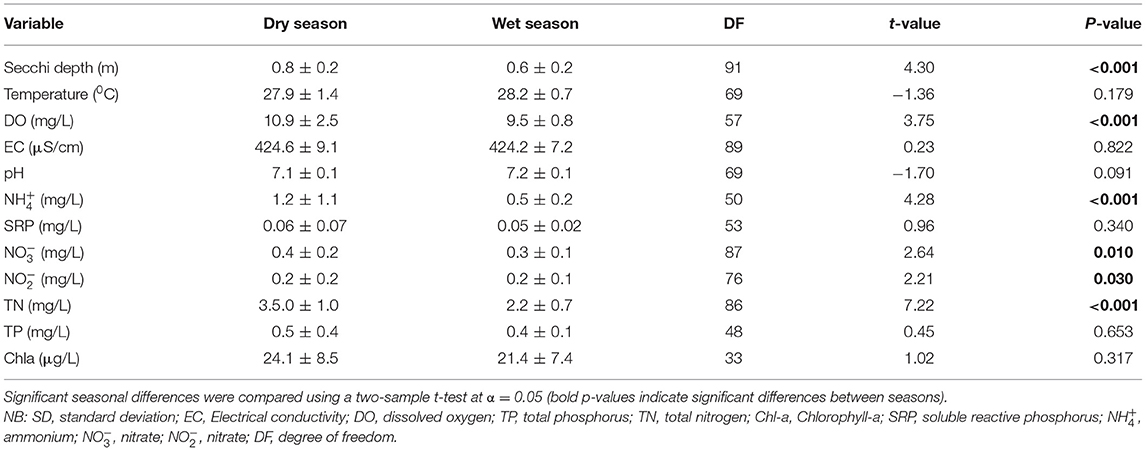
Table 1. Mean values (±SD) of water quality variables and nutrient concentrations during the dry and wet seasons in Lake Kanyaboli, Kenya during the study period from February 2020 to February 2021.
There were significant seasonal differences in water transparency as measured by the Secchi disc [t(91) = 4.30, p ≤ 0.001), and the concentrations of dissolved oxygen [t(57) = 3.75, p ≤ 0.001), p ≤ 0.001), ammonium [t(50) = 4.28, p ≤ 0.001), nitrate [t(87) = 2.64, p = 0.010], nitrite [t(76) = 2.21, p = 0.030], and total nitrogen [t(86) = 7.22, p ≤ 0.001] (Table 1). On the other hand, two-sample t-tests showed no significant seasonal differences in chlorophyll-a concentration (Table 1). Two-way ANOVA yielded no significant interaction between spatial and season variation in physico-chemical variables. The concentration of chlorophyll-a showed no interaction between site and season [F(5, 24) = 0.96, p = 0.462], but one-way ANOVA indicated spatial differences [F(5, 30) = 61.54, p = <0.001]. Multiple comparisons of means using Turkey's post-hoc test indicated that the concentration of chlorophyll-a in the littoral zone at the Hotel (34.97 ± 3.36 μg/L) and Kadenge (28.49 ± 3.06 μg/L) sampling stations were statistically different from each other and the rest of the sites while littoral zone site Gangu (23.52 ± 1.11 μg/L) and inflow zone site Inlet Lake Side (21.45 ± 4.19 μg/L) were the same but statistically different from the rest of the sites. Furthermore, the Open water site (9.03 ± 0.81), and outflow zone site Outlet Lake Side (11.29 ± 2.03 μg/L) were the same but statistically different from other sites.
Phytoplankton and Zooplankton Composition and Abundance
The number of phytoplankton taxa recorded during the 12 months of the study period indicated that March-2020 had the highest number of taxa (24) while November-2020 had the lowest (6) (Table 2). Similarly, the Shannon diversity index (H′) and the Simpson's diversity index (D1) had the highest phytoplankton diversity in March-2020, while November-2020 had the lowest ranging from 1.56 to 2.89 and 0.77 to 0.93, respectively (Table 2). Simpson's evenness index (E) showed that October-2020 and February-2021 had the lowest and highest evenness, respectively. Likewise, Simpson's dominance (D2) was higher in November-2020 (0.23) while March-2020 had the lowest (0.07).
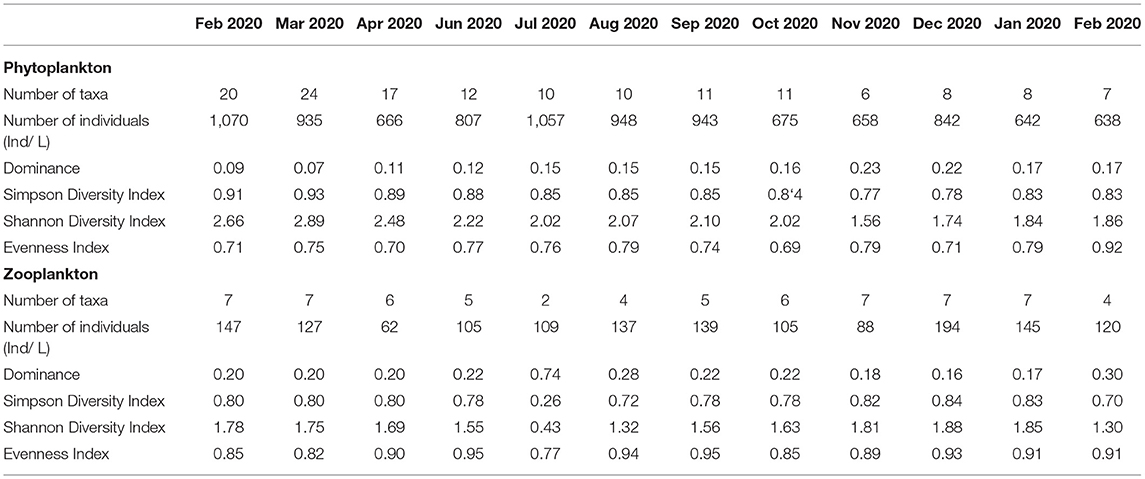
Table 2. Temporal changes in diversity indices and number of taxa of phytoplankton and zooplankton in Lake Kanyaboli during the study period from February 2020 to February 2021.
The number of zooplankton taxa was highest (7) in February-2020, March-2020, November-2020, December-2020, and January-2020, while July-2020 (2) had the lowest number of taxa (Table 2). The Shannon (H') and Simpson's (D1) diversity indices had the highest values in December-2020, while July-2020 had the lowest value ranging from 0.43 to 1.88 and 0.26 to 0.84, respectively (Table 2). The Simpson's evenness index (E) was lowest for July-2020 (0.77) while August-2020 had the highest (0.94). However, July-2020 (0.74) had high Simpson's dominance (D2) while Decemebr-2020 had the lowest (0.16) (Table 2).
Two-way ANOVA indicated no significant interaction between site and season in the abundance of phytoplankton [F(5, 2148) = 1.25, p = 0.281] and zooplankton [F(5, 1068) = 0.71, p = 0.618]. However, analysis of main effects showed significant differences between seasons for phytoplankton (F(5, 2148) = 36.61, p ≤ 0.001] and zooplankton (F(5, 1068) = 101.49, p ≤ 0.001] while there were no spatial differences for phytoplankton and zooplankton.
The Chrolophyceae (green algae) was the most dominant phytoplankton group in terms of abundance at all the 6 sampling stations. Bacillariophyceae was the least abundant group in the outflow zone (Outlet lake Side). At the same time, Cryptophyceae was the least abundant at the littoral zone (Gangu, Hotel, and Kadenge), inflow zone (Inlet lake Side), and open water stations (Figure 3A). Zooplanktons had 2 groups dominating the 6 stations: rotifers that dominated the inflow zone (Inlet lake Side) and the outflow zone (Outlet lake Side). In contrast, copepods dominated the littoral zone (Gangu, Hotel, and Kadenge) and open water (Figure 3B).
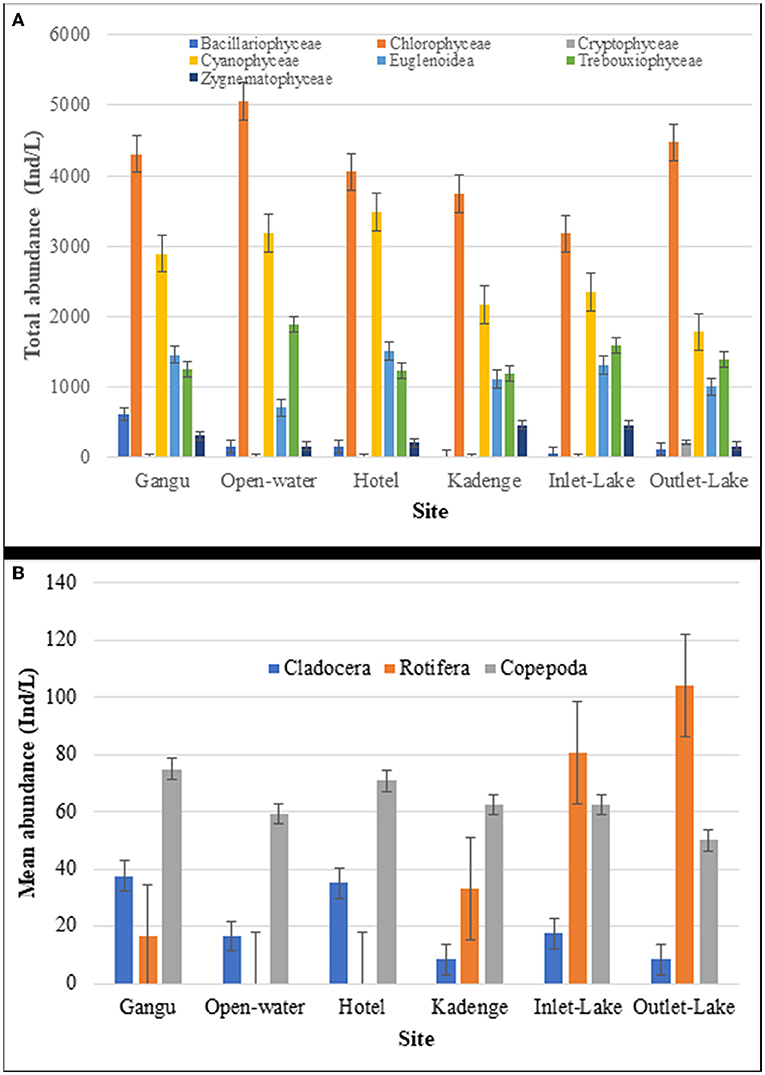
Figure 3. Comparison of mean plankton abundance at different sampling stations in Lake Kanyaboli from February 2020 to February 2021 [(A): phytoplankton; (B): zooplankton].
During January-2020 and February-2021, phytoplankton abundance was the lowest while February-2020 recorded the highest (Figure 4A). On the other hand, zooplankton abundance was lowest for April-2020 while December-2020 recorded the highest (Figure 4B). Overall, both phytoplankton and zooplankton had higher abundance during the dry season (January, February, July, August and September) compared to the wet season (March, April, June, October, November, and December).
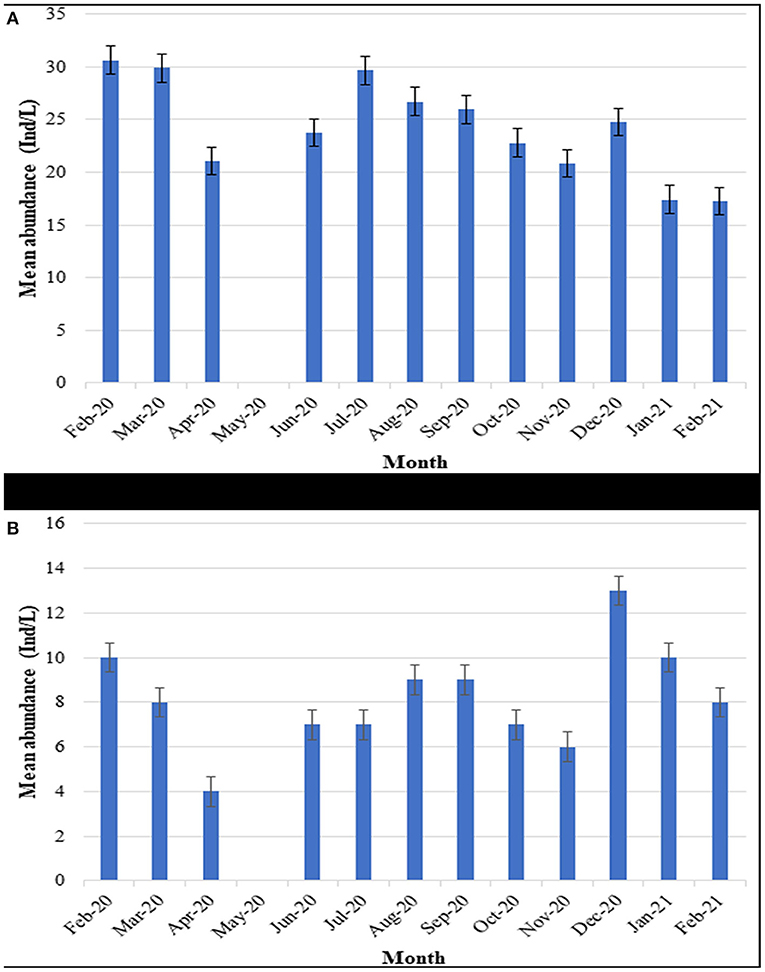
Figure 4. Temporal variations of phytoplankton and zooplankton abundance in Lake Kanyaboli from February 2020 to February 2021 [(A): monthly variations of phytoplankton; (B): monthly variations of zooplankton].
A mean total abundance of 9,913 ± 470 Ind/L of phytoplankton was recorded in Lake Kanyaboli during the study period (Table 3). The phytoplankton community was represented by 30 genera comprised of Bacillariophyceae (5 genera), Chlorophyceae (9 genera), Cryptophyceae (1 genus), Cyanophyceae (10 genera), Euglenoidae (2 genera), Trebouxiophyceae (2 genera), and Zygnematophyceae (1 genus) (Table 2). These represented broad groups of green algae (Chlorophyceae, Trebouxiophyceae, and Zygnematophyceae), blue-green algae (Cyanophyceae), euglenophytes (Euglenoidae), and diatoms (Bacillariophyceae). The structure and composition of phytoplankton were dominated by green algae (42.3%), followed by blue-green algae (26.93%), euglenophytes (11.85%), and diatoms (1.76%). Crucigenia sp. were more abundant (1,925 Ind/L), while Pediastrum sp. contributed the least (8 Ind/L) toward total abundance (Table 3). The dry and wet seasons recorded the same number of taxa but in varying compositions (Table 3). Three genera were absent in each season, i.e., Diatoma, Scenedesmus, and Pediastrum during the dry season and Hyalodicus, Diploneis, and Dictyosphaerium during the wet season.
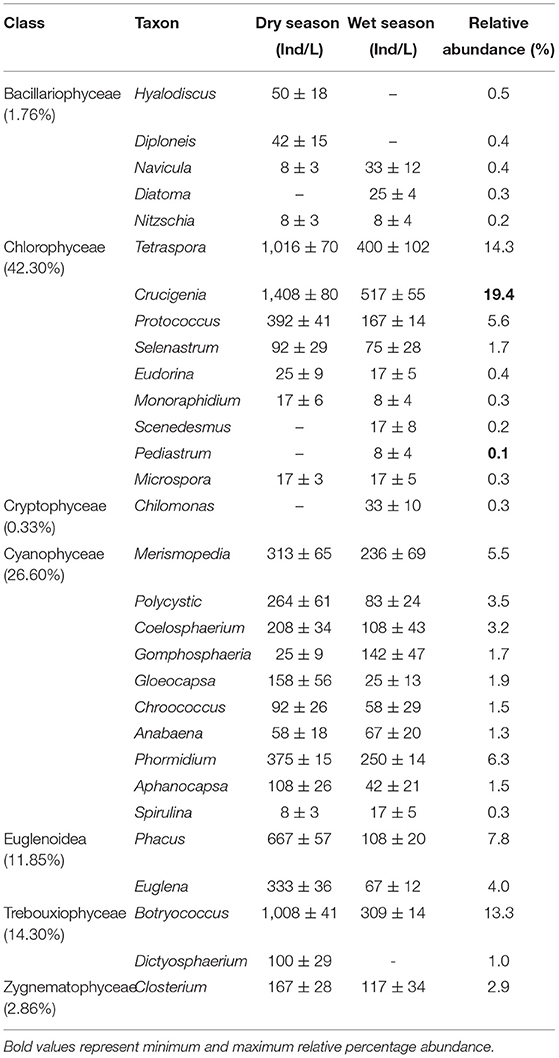
Table 3. Mean (±SD) abundance of different phytoplankton taxa recorded in Lake Kanyaboli during the study period from February 2020 to February 2021.
The abundance of zooplankton (1,476 ± 84 Ind/L) was averaged during the sampling period. Nauplius sp. (266 Ind/L) contributed most to total abundance while Ceriodaphnia sp., Asplanchina sp. and Leptodora sp. had the same abundance (17 Ind/L) individually contributed the least to the total abundance (Table 4). The zooplankton community was represented by 15 genera grouped into Rotifera, Copepoda, and Cladocera (Table 4). There were more copepods (55.41%) while Cladocerans were the least (16.73%). Overall, the dry season (14) had more taxa than the wet season (10) (Table 4). Among the 15 genera recorded during the study, Eubranchipus, Ceriodaphnia, Diaptomus, Microcodon, and Asplanchina were absent during the wet season, while Leptodora taxon was absent during dry season.
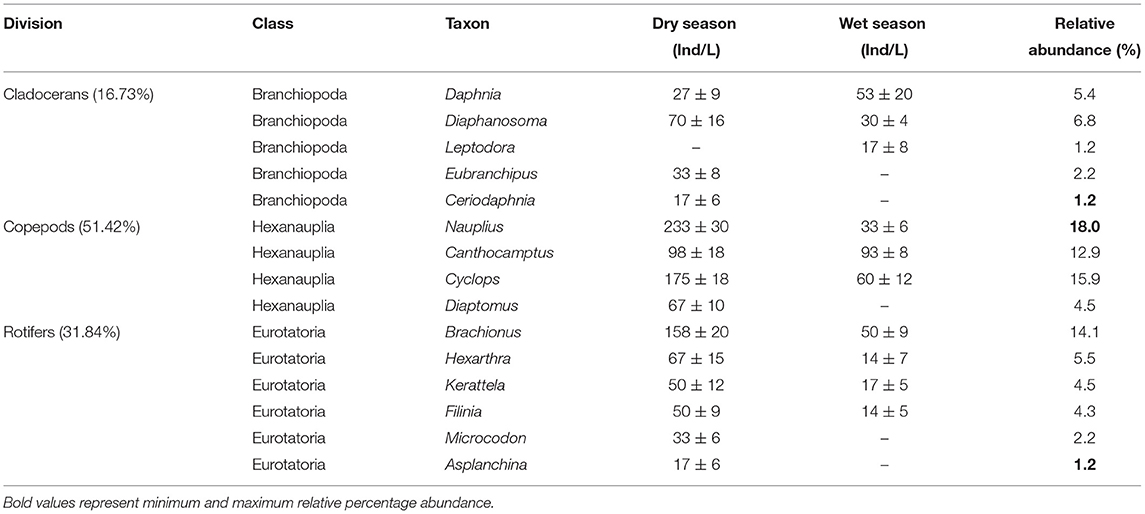
Table 4. Mean abundance of zooplankton taxa recorded in Lake Kanyaboli from February 2020 to February 2021.
ANOSIM indicated lack of significant differences in phytoplankton community composition for un-transformed abundance data among sites (R-statistic = 0.01, p = 0.44), and between seasons (R-statistic = 0.01, p = 0.40). For zooplankton, ANOSIM indicated significant differences for un-transformed abundance data between seasons (R-statistic = 0.12, p < 0.0001), but not among sites (R-statistic = 0.08, p < 0.89). These findings suggest a stronger seasonal effect compared to spatial effects for zooplanktons. Non-metric multidimensional scaling (NMDS) did not show any clear temporal and spatial separation of phytoplankton and zooplankton (Figures 5A–D).
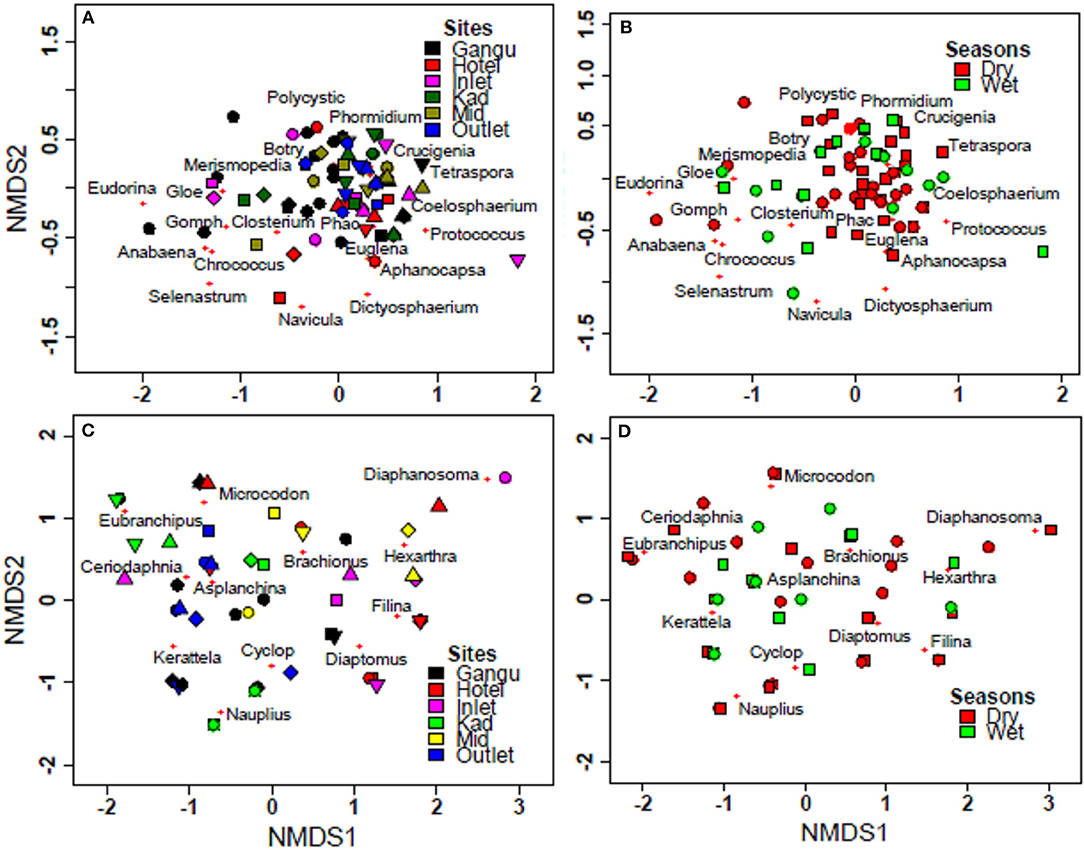
Figure 5. NMDS comparison of phytoplankton and zooplankton in Lake Kanyaboli from February 2020 to February 2021. (A) phytoplankton site comparisons, (B) phytoplankton seasonal comparisons, (C) zooplankton site comparisons (D) zooplankton seasonal comparisons. KAD, Kadenge; MID, Open water.
Results of SIMPER analysis showed that Crucigenia sp. contributed the highest proportion (12.14 %) to the dissimilarity between seasons while Microspora sp. contributed the least (0.16%) (Table 5). Nauplius sp. accounted for the most proportion (16.84%) to zooplankton dissimilarity, while Ceriodaphnia sp. accounted for the least (1.31 %) (Table 6).
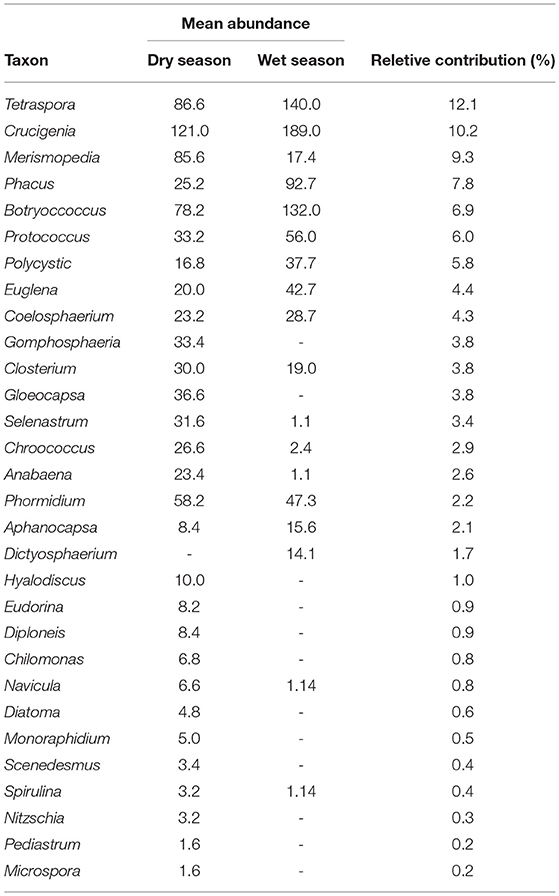
Table 5. Phytoplankton ranked abundance SIMPER contributors to percentage (%) dissimilarity in phytoplankton composition between the wet and dry seasons.
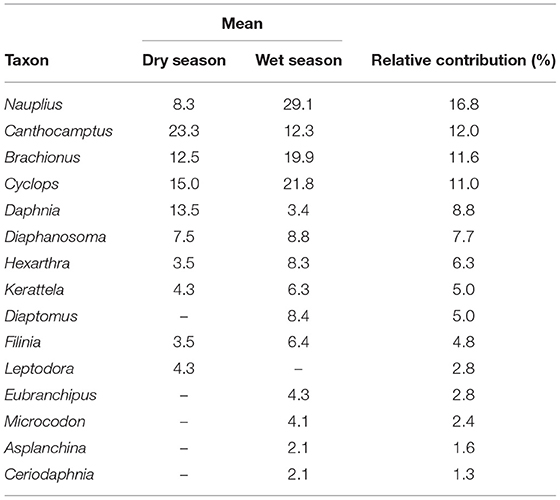
Table 6. Zooplankton ranked abundance SIMPER contributors to % dissimilarity in phytoplankton composition between the wet and dry seasons.
Relationship Between Water Quality and Plankton Communities
There were clear associations between water quality variables and phytoplankton and zooplankton communities. For phytoplankton, the first CCA axis (CCA 1) accounted for the greatest variance (% explained 30.4–37.0%) while the second axis (CCA 2) accounted for 16.2–22.8% (Figures 6A,B). Phytoplankton taxa such as Gomphospheria sp., Selenastrum sp., Chrococcus sp., Navicula sp., and Anabaena sp. were positively correlated with increasing EC (Figure 6B). Likewise, Coelosphaerium sp. and Spirulina sp. were positively correlated with pH, , and Secchi depth (Figure 6B). Similarly, Protococcus sp., Botryococcus sp., Phormidium sp., Crucigena sp., Tetraspora sp., Eudorina sp., and Diatoma sp. were positively correlated with DO, and SRP (Figure 6B). Furthermore, Chilomonas sp., Phacus sp., Polycystic sp., Euglena sp., Merismopedia sp., and Closterium sp. were negatively correlated with , temperature, TN, and TP (Figure 6B).
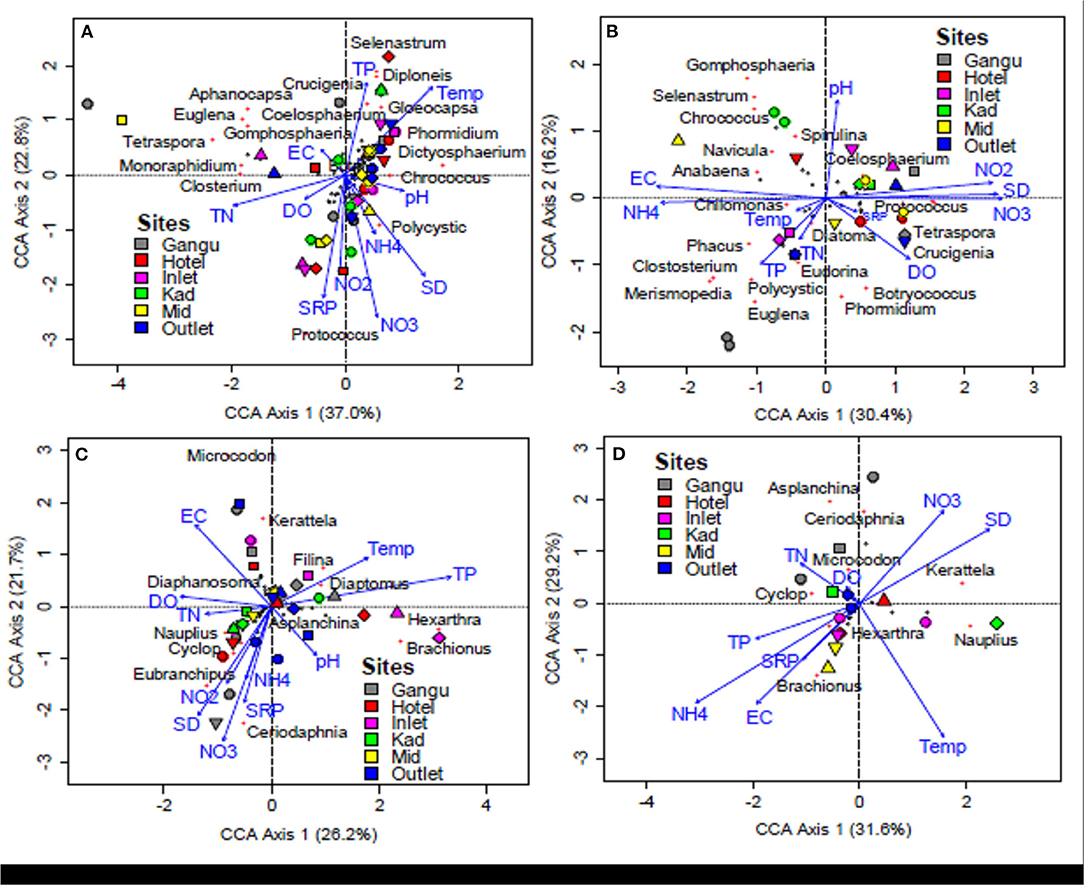
Figure 6. Canonical correspondence analysis (CCA) between plankton and physico-chemical variable among sites in Lake Kanyaboli from February 2020 to February 2021. (A,B) phytoplankton site comparisons, (C,D) zooplankton site comparisons. KAD, Kadenge; MID, Open water.
For zooplankton, the first CCA axis (CCA 1) also accounted for the greatest variance (% explained 26.2–31.6%) while the second axis (CCA 2) accounted for 21.7–29.2% (Figures 6C,D). Zooplankton genera such as Asplanchina sp., Microcodon sp., Ceriodaphnia sp., and Cyclop sp. were positively correlated with DO and TN (Figure 6D). At the same time, Kerattela sp. was also positively correlated with and Secchi depth (Figure 6D). On the other hand, Branchionus sp. showed a negative correlation with TP, , SRP, and EC, while Hexarthra sp. and Nauplius sp. were positively correlated with temperature (Figure 6D).
Discussion
The spatial and temporal analysis of the plankton (phytoplankton and zooplankton) community structure and composition in Lake Kanyaboli between February 2020 and February 2021 revealed strong seasonality with minimal or homogeneous spatial variation. A total of seven phytoplankton classes (Bacillariophyceae, Chlorophyceae, Cryptophyceae, Cyanophyceae, Euglenoidae, Trebouxiophyceae, and Zygnematophyceae) were identified, while three zooplankton classes, including Branchiopoda (Cladocerans), Hexanauplia (Copepods) and Eurotatoria (Rotifers) were identified in the lake during the study period. Chlorophyceae dominated the phytoplankton community, while Hexanauplia (Copepods) dominated the zooplankton community. Phytoplankton recorded the same number (30) of genera during the wet and dry seasons but in varying composition, while zooplanktons were more diverse in the dry season (14 genera) than during the wet season (10 genera). Both phytoplankton and zooplankton recorded higher abundance during the dry than during the wet season. The plankton abundance (phytoplankton and zooplankton) showed homogeneity among sites. However, phytoplankton composition showed dominance of Chlorophyceae in all the sites (Figure 3A). For zooplankton, rotifers dominated inflow and outflow zones while copepods dominated in the littoral zone and open water (Figure 3B). The results confirm our hypotheses that plankton (phytoplankton and zooplankton) communities would vary more between the seasons than spatially in Lake Kanyaboli. No single taxon or a combination of two or three taxa accounted for more than 80% of the phytoplankton abundance. This also confirms our hypothesis on lack of equilibrium in the phytoplankton community in the lake.
Dynamics in Environmental Conditions
There was a stronger seasonal variation in physico-chemical water quality variable than there were spatial over the lake, owing to the small size of the lake that is largely well-mixed, and with short water residence times. Secchi depth showed seasonal variation with reduced levels (increased turbidity) during the wet season due to high discharge rates from effluent rivers (canal) (Srifa et al., 2016). The concentrations of dissolved oxygen, ammonium, nitrates, nitrites, and total nitrogen were also higher during the dry season than the wet season (Figure 2). High nutrient concentration during the dry season is not a common feature of inland waters in which nutrients are often linked to runoff from agricultural catchments (Kilonzo et al., 2014; Srifa et al., 2016; R Development Core, 2017). In Lake Kanyaboli, the concentration of nutrients during the dry season is most likely linked to evaporation that concentrates solutes, while dilution would be the cause for low concentrations during the wet season (Okech et al., 2018; Olele and Ekelemu, 2021). Morabito et al. (2018) reported a negative correlation between the amount of rainfall and the concentrations of phosphorus and silica and linked it to the dilution of the in-lake concentrations after high rainfall. The dilution effect can also be enhanced by the short water residence times in lakes, such as Lake Kanyaboli, that drain disproportionately large drainage catchments compared to their surface area (Cardille et al., 2004). According to Walker et al. (2007), the characteristics of the drainage basin and the mean depth of the lake or reservoir are the primary factors controlling nutrient enrichment.
Dynamics in Phytoplankton Communities
Phytoplankton production in lakes is regulated by the availability of nutrients (nitrogen and phosphorus) and water clarity (Wang et al., 2015). For example, nitrogen and phosphorus enrichment results in the phytoplankton community shifting from diatom and chlorophyte dominance to cyanobacteria dominance (Hecky et al., 2010). Phytoplankton composition subsequently influences nutrient recycling and food web dynamics through bottom-up control (Cetinić et al., 2006). Similarly, phytoplankton composition influences zooplankton communities, which are also regulated by water quality variables such as nutrients, temperature and water clarity (Waya et al., 2014).
Lake Kanyaboli's plankton community showed a significant relationship with water quality variables (Figure 6). Phytoplankton taxa such as Coelosphaerium sp., Spirulina sp., Protococcus sp., Tetraspora sp., Botryococcus sp., Phormidium sp., and Crucigena sp. were positively correlated with high nutrient concentrations and high water clarity (Secchi depth). In contrast, Chilomonas sp., Phacus sp., Polycystic sp., Euglena sp., Merismopedia sp., Closterium sp., Eudorina sp., and Diatoma sp. were negatively correlated with nutrients. These patterns potentially indicate the regulatory effect of nutrient concentration and water clarity on phytoplankton communities in this lake. The distribution and abundance of Cyanophyceae taxa such as Coelosphaerium sp., Anabaena sp., Spirulina sp., and Phormidium sp. are associated with eutrophic water bodies rich in nutrients (Loza et al., 2014; Sharma et al., 2016). On the other hand, Merismopedia sp. is associated with good water quality hence the negative correlation with nutrients (Willén, 2000; Abdel-Hamid et al., 2014). In a similar study, Wang et al. (2015) found that phytoplankton community structure was significantly influenced by nutrients, conductivity and turbidity in the Shengjin Lake in China. Furthermore, positive relationships of phytoplankton concentration with and have also previously been observed elsewhere (Armi et al., 2010).
Chlorophyll-a, which is an indirect indicator of phytoplankton productivity, showed significant temporal and spatial differences, but seasonal variations were stronger. The concentration of chlorophyll-a was lower in open water compared to the littoral zones. Similarly, open water also exhibited low phytoplankton and zooplankton abundance compared to the littoral zone though not statistically different. Frost and Culver (2001) also reported a low abundance of phytoplankton in open water compared to inshore areas in Lake Erie influenced by nutrient availability. This reinforces the productivity of littoral zones compared to open waters due to constant mixing (Wondie et al., 2007).
Overall, phytoplankton abundance in Lake Kanyaboli showed a dominance of green algae (Table 3), contrary to a previous study in the same lake by Wilfred et al. (2005) that reported dominance of blue-green algae in a sample by a relative abundance of over 50%. The dominance of green algae was also in contrast to many African lakes and reservoirs associated with the dominance of blue-green algae, such as Lake Victoria and Lake Malawi (El-Otify et al., 2003). The dominance of green algae in Lake Kanyaboli is an indication that the quality of the water is better than in most lakes that are heavily influenced by nutrient and organic matter loading from agricultural and urbanized catchments (Hecky et al., 2010; Niyoyitungiye et al., 2019). However, previous studies have shown that the lake has once been eutrophic and hypereutrophic when the feeder canal was blocked, preventing water replenishment from River Yala, except by rainfall and backflow from Lake Victoria (Abila, 2005; Kondowe et al., 2022). Similarly, Ballot et al. (2009) reported a shift in the phytoplankton dominance from Cyanophyceae to Chlorophyceae in Lake Naivasha due to improving water quality. In contrast, a shift from Chlorophyceae to Cyanophyceae was observed in Lake Olodien due to deteriorating water quality (Ballot et al., 2009).
The composition of phytoplankton in lake Kanyaboli can also be linked to wind turbulence. Lake Kanyaboli experienced an average wind speed of 2.14 m.s−1 during the study period, which is slightly above the wind speed (2 m.s-1) that hinders the formation of cyanobacterial blooms (Blottiere, 2015). This probably favored the establishment of green algae over cyanobacteria (Blottiere, 2015; Visser et al., 2015; Zhou et al., 2015). Wind-induced mixing resuspends nutrients that give fast-growing chlorophytes a competitive advantage over the relatively slow-growing cyanobacteria, hence the former's proliferation (Jensen et al., 1994).
Lake Kanyaboli's phytoplankton community composition (Table 3) indicated that the lake is in a non-equilibrium state because no single species or a combination of two or three species contributed more than 80% of the total abundance that existed for more than a month (Sommer et al., 1993). Tropical lakes are associated with constant mixing because of a less stable diurnal mixed layer due to higher radiative heating facilitating dynamism in the lake processes (Lewis, 1987; Talling and Lemoalle, 1998). Moreover, Lake Kanyaboli is characterized by dense floating papyrus macrophytes that contribute to phytoplankton dynamics through uptake and storing of nutrients that become unavailable to the primary producers. The impact of macrophytes on phytoplankton dynamics has been reported in other smaller and shallow water bodies (Scheffer et al., 1993; Takamura et al., 2003; Fonseca and Bicudo, 2010). Therefore, the lake is probably in constant transition between turbid and clear states due to water exchange through the lake as well as macrophyte-driven nutrient uptake, preventing the perpetual dominance of selected phytoplankton species. Scheffer et al. Scheffer et al. (1993) noted that macrophytes suppress algal growth due to reduced nutrient availability and release of allelopathic substances by some plants that are toxic to algae. A combination of all these factors may have facilitated a shift in the plankton community's structure, resulting in a non-equilibrium state and improved water quality. These findings are in concordance with Hutchinson (1961), who noted that most lake systems exhibit non-equilibrium conditions due to the seasonal and/or annual fluctuations in physical, chemical, and biological environmental factors.
Dynamics in Zooplankton Communities
Similar to phytoplankton, there were also significant responses in some zooplankton taxa to seasonal changes in water quality in Lake Kanyaboli. Zooplankton genera such as Asplanchina sp., Microcodon sp., Ceriodaphnia sp., Cyclop sp. Kerattela sp., Hexarthra sp., and Nauplius sp. were positively correlated with high concentrations of dissolved oxygen and nutrients and increased water clarity (Figure 6D). On the other hand, Branchionus sp. showed a negative correlation with high levels of nutrients (TP, , SRP), electrical conductivity and water temperature. The correlation of Kerattela sp. with nutrients and Secchi depth indicates that the genera can be used as indicators of eutrophic conditions. Previous studies have reported that Rotifera species in genera Brachionus, Polyarthra, Keratella, and Filinia are bioindicators of organic pollution in inland waters (Ejsmont-Karabin, 2012; Rosińska et al., 2019; Zakaria and El-Naggar, 2019; Krupa et al., 2020). Işkin et al. (2020) also observed a strong nutrient effect on zooplankton and their sensitivity to increasing water temperature through direct effects on ontogeny and physiology and indirectly by altering their food sources.
The zooplankton community in Lake Kanyaboli is dominated by copepods, followed by rotifers and cladocerans. Other studies in Lake Kivu (Isumbisho et al., 2006), Lake Victoria (Ngupula et al., 2010) and Lake Shinji–Ohashi and River–Lake Nakaumi (Uye et al., 2000) have also reported a dominance of copepods. Their dominance is related to relatively small size and being successful feeders (Waya et al., 2014). The low density of cladocerans could be attributed to either predation by planktivorous fish or eutrophication since they are more sensitive to changes in the water quality (Zakaria and El-Naggar, 2019). The variability in the zooplankton community further shows size-selection predation by planktivorous fishes in the lake, such as the haplochromines, which prefer large-sized zooplankton (Hansson et al., 2007). Thus, cladocerans, rather than copepods, are preyed on more (Helenius et al., 2015). Moreover, the small-sized nauplii dominated copepods, and their small size makes them less preferable to large fish instead they are mostly consumed by young fish (Hansson et al., 2007). These phenomena explain disparities in the abundances among the zooplankton groups in our study.
Seasonal Variation in Phytoplankton and Zooplankton
The temporal species diversity indices for phytoplankton and zooplankton also showed seasonal variations with dry months recording predominantly high diversity (Table 2). This probably further indicates the season's influence as a plankton community driver due to variations in physico-chemical variables. High plankton densities in the dry season have also been observed elsewhere for phytoplankton (Deng et al., 2007) and zooplankton (Shukla and Singh, 2013; Razak and Sharip, 2019). Tian et al. (2013) noted that phytoplankton succession is primarily determined by the interactions and seasonal cycles of chemical (nutrients) and physical (weather) factors. The proliferation of phytoplankton is common in the dry season, positively affecting the zooplankton community and biomass (Razak and Sharip, 2019). However, our study's average phytoplankton diversity index results were lower than Wang et al. (2015) from Shengjin Lake, China. The lower diversity index could be predicting competition for nutrients between phytoplankton and macrophytes resulting in restricted primary production. In addition, the low biomass of phytoplankton could also mean overall deteriorating ecosystem health linked to organic pollution since the quality and quantity of phytoplankton and zooplankton assemblages act as water quality indicators (Pinto-Coelho et al., 2005; George et al., 2012). This is consistent with the occurrence of Closterium sp., Navicula sp., and Nitzschia sp. in the lake that is associated with the introduction of organic pollution (Yusuf, 2020). Furthermore, the present study also reported Euglenoidae (Euglena sp. and phacus sp.) phytoplankton, which could signal anthropogenic linked nutrient enrichment (George et al., 2012).
Generally, the strong seasonal variation in abundance and composition of plankton communities in Lake Kanyaboli are consistent with studies in other small lakes and reservoirs (de Souza Barroso et al., 2018; Kraus et al., 2019; Nguyen et al., 2019). This has been linked to seasonally induced factors such as flooding, making the water turbid hence, reducing productivity (Morabito et al., 2018). In addition, small lakes and reservoirs are associated with quick water transit due to the shorter residence time (Borges et al., 2008; Ismail et al., 2019). This phenomenon potentially affects sedimentation, leading to phosphorus loss before settling at the bottom sediments, which could be used during resuspension. Combining these factors probably reduces the intensity of algal blooms during the wet season but enhances the formation of algal blooms during the dry season after discharge levels decline (Srifa et al., 2016). The lack of spatial variation in plankton communities in this study was unexpected, given the strong variation in human activities in the vicinity of the sampling sites.
Conclusion
This study presents the diversity and composition of phytoplankton and zooplankton in a small tropical lake and their relationship with physico-chemical variables. The large catchment area to lake ratio and anthropogenic activities in the vicinity of the lake influence the physico-chemical characteristics of the lake and its communities. The role of seasonality as a driver of the physico-chemistry and ecology of the lake was exhibited in changes in water clarity (Secchi depth) and the concentration of dissolved oxygen and nutrients (ammonium, nitrate, nitrite, and total nitrogen) with higher levels during the dry season. The plankton communities in the lake were also seasonally influenced through changes in environmental conditions. Phytoplankton taxa such as Merismopedia were identified as potential bioindicators of good water quality and were associated with low nutrient concentrations, while Navicula sp., and Anabaena sp. were indicators of nutrient enrichment. For zooplankton, Kerattela sp. was associated with high nutrient concentration and was a good indicator of eutrophication in the lake. The lake's complex heterogeneous physical and chemical variables, in conjunction with other food web components, such as zooplankton and fishes, were vital in maintaining a non-equilibrium state in which proliferation of algal blooms doesn't occur. The long-term data we collected in this study highlight the importance of continuous monitoring of water quality and biological communities in small tropical lakes as a basis for understanding their structural and functional dynamics. This study contributes to understanding the drivers of the structure and composition of biological communities in small tropical water bodies that are increasingly influenced by human activities.
Data Availability Statement
The original contributions presented in the study are included in the article/supplementary material, further inquiries can be directed to the corresponding author.
Author Contributions
BK: conceptualization, investigation, methodology, original draft preparation, data curation, and formal analysis. FM: funding acquisition, supervision, visualization, validation, reviewing, and editing. PR and WS: funding acquisition, supervision, and reviewing. AS: investigation, formal analysis, and editing. RW: visualization, reviewing, and editing. All authors contributed to the article and approved the submitted version.
Funding
The present work was supported by Intra-Africa Academic Mobility Project: - Collaborative Training in Fisheries and Aquaculture in East, Central, and Southern Africa (COTRA) funded by European Union (EU).
Conflict of Interest
The authors declare that the research was conducted in the absence of any commercial or financial relationships that could be construed as a potential conflict of interest.
Publisher's Note
All claims expressed in this article are solely those of the authors and do not necessarily represent those of their affiliated organizations, or those of the publisher, the editors and the reviewers. Any product that may be evaluated in this article, or claim that may be made by its manufacturer, is not guaranteed or endorsed by the publisher.
Acknowledgments
Special thanks are due to fishers at Kadenge, Gangu, and Kombo beach for their assistance during fieldwork. BK is also grateful to Henry Lubanga of the University of Eldoret for the service rendered during sampling and laboratory analyses.
References
Abdel-Hamid, M., Abdel-Aal, E., and Azzab, Y. (2014). Spatial quality improvement of a toxic industrial effluent, based on physico-chemistry, algal community changes and algal bioassay. Af. J. Aquatic Sci. 39, 1–16. doi: 10.2989/16085914.2013.870524
Abdul, W. O., Adekoya, E. O., Ademolu, K. O., Omoniyi, I. T., Odulate, D. O., Akindokun, T. E., et al. (2016). The effects of environmental parameters on zooplankton assemblages in tropical coastal estuary, South-west, Nigeria. Egypt. J. Aquatic Res. 42, 281–287. doi: 10.1016/j.ejar.2016.05.005
Abila, R. (2005). Biodiversity and sustainable management of a tropical wetland lake ecosystem: a case study of Lake Kanyaboli, Kenya. FWU Water Resour. Publications 3, 1–11.
Abila, R., Salzburger, W., Ndonga, M. F., Owiti, D. O., Barluenga, M., and Meyer, A. (2008). The role of the Yala swamp lakes in the conservation of Lake Victoria region haplochromine cichlids: evidence from genetic and trophic ecology studies. Lakes Reservoirs 13, 95–104 doi: 10.1111/j.1440-1770.2008.00366.x
Aka, M., Pagano, M., Saint-Jean, L., Arfi, R., Bouvy, M., Cecchi, P., et al. (2000). Zooplankton variability in 49 shallow tropical reservoirs of Ivory Coast (West Africa). Int. Rev. Hydrobiol. 85, 491–504. doi: 10.1002/1522-2632(200008)85:4<491::AID-IROH491>3.0.CO;2-G
Aloo, P. A. (2003). Biological diversity of the Yala Swamp lakes, with special emphasis on fish species composition, in relation to changes in the Lake Victoria Basin (Kenya): threats and conservation measures. Biodivers. Conserv. 12, 905–920. doi: 10.1023/A:1022869624524
Angienda, P. O., Lee, H. J., Elmer, K. R., Abila, R., Waindi, E. N., and Meyer, A. (2011). Genetic structure and gene flow in an endangered native tilapia fish (Oreochromis esculentus) compared to invasive Nile tilapia (Oreochromis niloticus) in Yala swamp, East Africa. Conserv. Genet. 12, 243–255. doi: 10.1007/s10592-010-0136-2
Anyona, G. O. (1997). An Investigation Into Some Water Quality Problems of Lake Kanyaboli in Kenya. MSc Thesis, University of Nairobi, Nairobi, Kenya.
APHA. (2017). Standard Methods for the Examination of Water and Wastewater. Washington, DC: American Public Health Association. American Water Works Association. Water Environment Federation, 1545.
Arhonditsis, G., Brett, M. T., and Frodge, J. (2003). Environmental control and limnological impacts of a large recurrent spring bloom in Lake Washington, USA. Environ. Manage. 31, 0603–0618. doi: 10.1007/s00267-002-2891-4
Armi, Z., Turki, S., Trabelsi, E., and Maiz, N. B. (2010). First recorded proliferation of Coolia monotis (Meunier, 1919) in the North Lake of Tunis (Tunisia) correlation with environmental factors. Environ. Monit. Assess. 164, 423–433. doi: 10.1007/s10661-009-0903-z
Babu, J. M., Getabu, A. M., Nyaundi, K., Jembe, D., Boera, P., Sitoki, L., et al. (2015). Phytoplankton distribution and abundance in small water bodies of the Lake Victoria Basin of Kenya. Int. J. Fisheries Aquatic Stud. 2, 166–169.
Ballot, A., Kotut, K., Novelo, E., and Krienitz, L. (2009). Changes of phytoplankton communities in Lakes Naivasha and Oloidien, examples of degradation and salinization of lakes in the Kenyan Rift Valley. Hydrobiologia 632, 359–363. doi: 10.1007/s10750-009-9847-0
Bellinger, E. G., and Sigee, D. C. (2015). Freshwater Algae: Identification Enumeration and Use as Bioindicators. West Sussex: John Wiley and Sons. doi: 10.1002/9781118917152
Bicudo, D. C., Fonseca, B. M., Bini, L. M., Crossetti, L. O., Bicudo, C. E. M., and Araújo-Jesus, T. (2007). Undesirable side effects of water hyacinth control in a shallow tropical reservoir. Freshw. Biol. 52, 1120–1133. doi: 10.1111/j.1365-2427.2007.01738.x
Blottiere, L. (2015). The Effects of Wind-Induced Mixing on the Structure and Functioning of Shallow Freshwater Lakes in a Context of Global Change. PhD Dissertation, Paris Sud University, Paris.
Borges, P. A. F., Train, S., and Rodrigues, L. C. (2008). Spatial and temporal variation of phytoplankton in two subtropical Brazilian reservoirs. Hydrobiologia 607, 63–74. doi: 10.1007/s10750-008-9367-3
Bray, J. R., and Curtis, J. T. (1957). An ordination of the upland forest communities of southern Wisconsin. Ecol. Monogr. 27, 326–349. doi: 10.2307/1942268
Bucak, T., Trolle, D., Tavşanoglu, Ü. N., Çakiroglu, A. I., Özen, A., Jeppesen, E., et al. (2018). Modeling the effects of climatic and land use changes on phytoplankton and water quality of the largest Turkish freshwater lake: Lake Beyşehir. Sci. Total Environ. 621, 802–816. doi: 10.1016/j.scitotenv.2017.11.258
Burgess, C. (2008). Overview of Dominion Farms. Retrieved from: https://landmatrix.org/media/uploads/dominionfarmskenyablogspotcoza200801overview-of-dominion-farmshtml.pdf (accessed November 28, 2021).
Cardille, J., Coe, M. T., and Vano, J. A. (2004). Impacts of climate variation and catchment area on water balance and lake hydrologic type in groundwater-dominated systems: a generic lake model. Earth Interact. 8, 1–24. doi: 10.1175/1087-3562(2004)8<1:IOCVAC>2.0.CO;2
Catherine, A., Mouillot, D., Maloufi, S., Troussellier, M., and Bernard, C. (2013). Projecting the impact of regional land-use change and water management policieson lake water quality: an application to periurban lakes and reservoirs. PLoS ONE 8, e72227. doi: 10.1371/journal.pone.0072227
Cetinić, I., Viličić, D., Burić, Z., and Olujć, G. (2006). “Phytoplankton seasonality in a highly stratified karstic estuary (Krka, Adriatic Sea),” in Marine Biodiversity. Developments in Hydrobiology, Vol 183, eds H. Queiroga, M. R. Cunha, A. Cunha, M. H. Moreira, V. Quintino, A. M. Rodrigues, J. Serôdio, and R. M. Warwick (Dordrecht: Springer). doi: 10.1007/1-4020-4697-9_3
CGS (County Government of Siaya). (2015). Report of the joint committee on agriculture, tourism, water and delegated legislation on Yala Swamp. Clerk's Chambers, County Assembly of Siaya, Assembly Buildings, Siaya, Kenya.
Chang, F. H., Zeldis, J., Gall, M., and Hall, J. (2003). Seasonal and spatial variation of phytoplankton assemblages, biomass and cell size from spring to summer across the north-eastern New Zealand continental shelf. J. Plankton Res. 25, 737–758. doi: 10.1093/plankt/25.7.737
Clarke, K. R., and Warwick, R. M. (2001). Change in Marine Communities. An Approach to Statistical Analysis and Interpretation. 2nd edn, 1–168.
Dang, P. D., Van Khoi, N., Nga, L. T. N., Thanh, D. N., and Hai, H. T. (2015). Identification Handbook of Freshwater Zooplankton of the Mekong River and its Tributaries. Vientiane: Mekong River Commission.
de Souza Barroso, H., Santos, J. A., Marins, R. V., and Lacerda, L. D. (2018). Assessing temporal and spatial variability of phytoplankton composition in a large reservoir in the Brazilian northeastern region under intense drought conditions. J. Limnol. 77, 130–146. doi: 10.4081/jlimnol.2017.1698
Deng, D. G., Xie, P., Zhou, Q., Yang, H., and Guo, L. G. (2007). Studies on temporal and spatial variations of phytoplankton in Lake Chaohu. J. Integr. Plant Biol. 49, 409–418. doi: 10.1111/j.1744-7909.2007.00390.x
Díaz-Torres, O., de Anda, J., Lugo-Melchor, O. Y., Pacheco, A., Orozco-Nunnelly, D. A., Shear, H., et al. (2021). Rapid changes in the phytoplankton community of a subtropical, shallow, hypereutrophic lake during the rainy season. Front. Microbiol. 12, 617151. doi: 10.3389/fmicb.2021.617151
Ejsmont-Karabin, J. (2012). The usefulness of zooplankton as lake ecosystem indicators: rotifer trophic state index. Polish J. Ecol. 60, 339–350.
El-Otify, A. M., Shafik, H. M., and Szoke, E. (2003). Analyses of physico-chemical characteristics and phytoplankton communities of Lake Nasser during the last two decades. Acta Bot. Hung. 45, 75–100. doi: 10.1556/ABot.45.2003.1-2.8
Figueredo, C. C., and Giani, A. (2009). Phytoplankton community in the tropical lake of Lagoa Santa (Brazil): conditions favoring a persistent bloom of Cylindrospermopsis raciborskii. Limnologica 39, 264–272. doi: 10.1016/j.limno.2009.06.009
Fonseca, B. M., and Bicudo, C. E. D. M. (2010). How important can the presence/absence of macrophytes be in determining phytoplankton strategies in two tropical shallow reservoirs with different trophic status?. J. Plankton Res. 32, 31–46. doi: 10.1093/plankt/fbp107
Fonseca, B. M., and de Mattos Bicudo, C. E. (2011). Phytoplankton seasonal and vertical variations in a tropical shallow reservoir with abundant macrophytes (Ninféias Pond, Brazil). Hydrobiologia. 665, 229–245. doi: 10.1007/s10750-011-0626-3
Frost, P. C., and Culver, D. A. (2001). Spatial and temporal variability of phytoplankton and zooplankton in western Lake Erie. J. Freshw. Ecol. 16, 435–443. doi: 10.1080/02705060.2001.9663833
George, B., Nirmal Kumar, J. I., and Kumar, R. N. (2012). Study on the influence of hydro-chemical parameters on phytoplankton distribution along Tapi estuarine area of Gulf of Khambhat, India. Egypt. J. Aquatic Res. 38, 157–170. doi: 10.1016/j.ejar.2012.12.010
Geraldes, A. M., and Boavida, M. J. L. (2004). Limnological variations of a reservoir during two successive years: One wet, another dry. Lakes Reservoirs 9, 143–152. doi: 10.1111/j.1440-1770.2004.00240.x
Grover, J. P., and Chrzanowski, T. H. (2006). Seasonal dynamics of phytoplankton in two warm temperate reservoirs: association of taxonomic composition with temperature. J. Plankton Res. 28, 1–17. doi: 10.1093/plankt/fbi095
Hansson, L. A., Nicolle, A., Brodersen, J., Romare, P., Anders Nilsson, P., Brönmark, C., and Skov, C. (2007). Consequences of fish predation, migration, and juvenile ontogeny on zooplankton spring dynamics. Limnol. Oceanogr. 52, 696–706. doi: 10.4319/lo.2007.52.2.0696
Hardikar, R., Haridevi, C. K., Chowdhury, M., Shinde, N., Ram, A., Rokade, M. A., et al. (2017). Seasonal distribution of phytoplankton and its association with physico-chemical parameters in coastal waters of Malvan, west coast of India. Environ. Monit. Assess. 189, 151. doi: 10.1007/s10661-017-5835-4
Hecky, R. E., Muginne, R., Ramlal, P. S., Talbot, M. R., and Kling, G. W. (2010). Multiple stressors cause rapid ecosystem change in Lake Victoria. Freshw. Biol. 55(Suppl. 1), 19–42. doi: 10.1111/j.1365-2427.2009.02374.x
Helenius, L. K., Aymà Padrós, A., Leskinen, E., Lehtonen, H., and Nurminen, L. (2015). Strategies of zooplanktivory shape the dynamics and diversity of littoral plankton communities: a mesocosm approach. Ecol. Evolut. 5, 2021–2035. doi: 10.1002/ece3.1488
Hemraj, D. A., Hossain, M. A., Ye, Q., Qin, J. G., and Leterme, S. C. (2017). Plankton bioindicators of environmental conditions in coastal lagoons. Estuar. Coast. Shelf Sci. 184, 102–114. doi: 10.1016/j.ecss.2016.10.045
Hyangya, B. L., Riziki, J. W., Masilya, P. M., Zabene, F. Z., Alunga, G. L., Kaningini, B. M., et al. (2021). Physico-chemical characterization of littoral water of Lake Kivu (Southern basin, Central Africa) and use of water quality index to assess their anthropogenic disturbances. World Water Pol. 7, 166–193. doi: 10.1002/wwp2.12059
Intergovernmental Oceanographic Commission of UNESCO (2010). Microscopic and Molecular Methods for Quantitative Phytoplankton Analysis, eds B. Karlson, C. Cusack, and E. Bresnan (Paris: UNESCO). (IOC Manuals and Guides, no. 55.) (IOC/2010/MG/55), 110.
Işkin, U., Filiz, N., Cao, Y., Neif, É. M., Ögl,ü, B., Lauridsen, T. L., et al. (2020). Impact of nutrients, temperatures, and a heat wave on zooplankton community structure: an experimental approach. Water 12, 3416. doi: 10.3390/w12123416
Ismail, A. H., Lim, C. C., and Wan Omar, W. M. (2019). Evaluation of spatial and temporal variations in zooplankton community structure with reference to water quality in Teluk Bahang Reservoir, Malaysia. Trop. Ecol. 60, 186–198. doi: 10.1007/s42965-019-00023-2
Isumbisho, M., Sarmento, H., Kaningini, B., Micha, J. C., and Descy, J. P. (2006). Zooplankton of Lake Kivu, East Africa, half a century after the Tanganyika sardine introduction. J. Plankton Res. 28, 971–989. doi: 10.1093/plankt/fbl032
James, T. and Chimney, M. (2008). Hurricane effects on a shallow lake ecosystem, Lake Okeechobee, Florida (USA). Fundamental Appl. Limnol. 172, 273–287. doi: 10.1127/1863-9135/2008/0172-0273
Jensen, J. P., Jeppesen, J., Olrik, K., et al. (1994). Impact of nutrients and physical factors on the shift Cyanobacteria to Chlorophytea in shallow Danish lakes. Can. J. Fish. Aquat. Sci. 51, 1692–1699 doi: 10.1139/f94-170
Jeppensen, E. M. Søndergaard, M., Meerhoff, T. L., Lauridsen, and Jensen, J. T. (2007). Shallow lakes restoration by nutrient loading reduction: some recent findings and challenges ahead. Hydrobiologia 584, 239–252. doi: 10.1007/s10750-007-0596-7
Jiang, Y.-J., He, W., Liu, W.-X., Qin, N., Ouyang, H.-L., Wang, Q.-M., et al. (2014). The seasonal and spatial variations of phytoplankton community and their correlation with environmental factors in a large eutrophic Chinese lake (Lake Chaohu). Ecol. Indic. 40, 58–67. doi: 10.1016/j.ecolind.2014.01.006
Jose, E. C., Furio, E. F., Borja, V. M., Gatdula, N. C., and Santos, M. D. (2015). Zooplankton composition and abundance and its relationship with physicochemical parameters in Manila Bay. Oceanography 3, 136. doi: 10.4172/2167-0331.1000136
Kashian, D. M., Romme, W. H., and Regan, C. M. (2007). Reconciling divergent interpretations of quaking aspen decline on the northern Colorado Front Range. Ecol. Appl. 17, 1296–1311. doi: 10.1890/06-1431.1
Kelly, B., Mtiti, E., McIntyre, P. B., and Vadeboncoeur, Y. (2017). Stable isotopes reveal nitrogen loading to Lake Tanganyika from remote shoreline villages. Environ. Manag. 59, 264–273. doi: 10.1007/s00267-016-0787-y
Kibena, J., Nhapi, I., and Gumindoga, W. (2014). Assessing the relationship between water quality parameters and changes in land use patterns in the Upper Manyame River, Zimbabwe. Phys. Chem. Earth A/B/C 67, 153–163. doi: 10.1016/j.pce.2013.09.017
Kilonzo, F., Masese, F. O., Van Griensven, A., Bauwens, W., Obando, J., and Lens, P. N. (2014). Spatial-temporal variability in water quality and macro-invertebrate assemblages in the Upper Mara River basin, Kenya. Phys. Chem. Earth. Parts A/B/C, 67, 93–104. doi: 10.1016/j.pce.2013.10.006
Kimambo, O. N., Gumbo, J. R., and Chikoore, H. (2019). The occurrence of cyanobacteria blooms in freshwater ecosystems and their link with hydro-meteorological and environmental variations in Tanzania. Heliyon. 5, e01312. doi: 10.1016/j.heliyon.2019.e01312
Kinaro, Z. O. (2008). Wetland Conversion to Large-Scale Agricultural Production; Implications on the Livelihoods of Rural Communities, Yala Swamp, Lake Victoria Basin, Kenya. Department of Water and Environmental Studies, Linkopings University, Sweden.
Komárková, J., and Tavera, R. (2003). “Steady state of phytoplankton assemblage in the tropical Lake Catemaco (Mexico),” in Phytoplankton and Equilibrium Concept: The Ecology of Steady-State Assemblages. Developments in Hydrobiology, Vol 172, eds L. Naselli-Flores, J. Padisák, M. T. Dokulil (Dordrecht: Springer). doi: 10.1007/978-94-017-2666-5_17
Kondowe, B. N., Masese, F. O., Raburu, P. O., Singini, W., and Walumona, R. J. (2022). Water quality and ecology of Lake Kanyaboli, Kenya: current status and historical changes. Lakes Reservoirs 27, e12401. doi: 10.1111/lre.12401
Kraus, C. N., Bonnet, M. P., de Souza Nogueira, I., Morais Pereira Souza Lobo, M. T., da Motta Marques, D., Garnier, J., et al. (2019). Unraveling flooding dynamics and nutrients' controls upon phytoplankton functional dynamics in Amazonian floodplain lakes. Water 11, 154. doi: 10.3390/w11010154
Krupa, E., Romanova, S., Berkinbaev, G., Yakovleva, N., and Sadvakasov, E. (2020). Zooplankton as indicator of the ecological state of protected aquatic ecosystems (Lake Borovoe, Burabay National Nature Park, Northern Kazakhstan). Water 12, 2580. doi: 10.3390/w12092580
Lee, J. K., and Oh, J. M. (2018). A study on the characteristics of organic matter and nutrients released from sediments into agricultural reservoirs. Water 10, 980. doi: 10.3390/w10080980
Leech, D. M., Pollard, A. I., Labou, S. G., and Hampton, S. E. (2018). Fewer blue lakes and more murky lakes across the continental US: Implications for planktonic food webs. Limnol. Oceanogr. 63, 2661–2680. doi: 10.1002/lno.10967
Lewis, W. M. Jr. (1987). Tropical limnology. Annu. Rev. Ecol. Syst. 18, 159–184. doi: 10.1146/annurev.es.18.110187.001111
Ling, T.-Y., Gerunsin, N., Soo, C.-L., Nyanti, L., Sim, S.-F., and Grinang, J. (2017). Seasonal changes and spatial variation in water quality of a large young tropical reservoir and its downstream river. J. Chem. 2017, 1–16. doi: 10.1155/2017/8153246
Liu, W., Zhang, Q., and Liu, G. (2011). Effects of watershed land use and lake morphometry on the trophic state of Chinese lakes: implications for eutrophication control. CLEAN–Soil Air. Water 39, 35–42. doi: 10.1002/clen.201000052
Loza, V., Perona, E., and Mateo, P. (2014). Specific responses to nitrogen and phosphorus enrichment in cyanobacteria: factors influencing changes in species dominance along eutrophic gradients. Water Res. 48:622–631. doi: 10.1016/j.watres.2013.10.014
Macuiane, M., Hecky, R., and Guildford, S. (2016). Temporal and spatial changes in water quality in Lake Malawi/Niassa, Africa: implications for cage aquaculture management. Oceanogr. Fish. 1, 555552.
Malbrouck, C., and Kestemont, P. (2006). Effects of microcystins on fish. Environ. Toxicol. Chem. 25, 72–86. doi: 10.1897/05-029R.1
McArdle, B. H., and Anderson, M. J. (2001). Fitting multivariate models to community data: a comment on distance-based redundancy analysis. Ecology 82, 290–297. doi: 10.1890/0012-9658(2001)0820290:FMMTCD2.0.CO;2
Mitsoura, A., Kagalou, I., Papaioannou, N., Berillis, P., Mente, E., and Papadimitriou, T. (2013). The presence of microcystins in fish Cyprinus carpio tissues: a histopathological study. Int. Aquatic Res. 5, 8. doi: 10.1186/2008-6970-5-8
Moncayo-Estrada, R., Lyons, J., Escalera-Gallardo, C., and Lind, O. T. (2012). Long-term change in the biotic integrity of a shallow tropical lake: a decadal analysis of the Lake Chapala fish community. Lake Reserv. Manag. 28, 92–104. doi: 10.1080/07438141.2012.661029
Morabito, G., Rogora, M., Austoni, M., and Ciampittiello, M. (2018). Could the extreme meteorological events in Lake Maggiore watershed determine a climate-driven eutrophication process? Hydrobiologia 824, 163–175. doi: 10.1007/s10750-018-3549-4
Muoria, P., Field, R., Matiku, P., Munguti, S., Mateche, E., Shati, S., et al. (2015). Yala Swamp Ecosystem Service Assessment. Nairobi, Kenya.
Mustapha, M. K. (2009). Influence of watershed activities on the water quality and fish assemblages of a tropical African reservoir. Rev. Biol. Trop. 57, 707–719. doi: 10.15517/rbt.v57i3.5486
Mutune, M. D., Omondi, R., and Owili, M. (2005). “Systematics and distribution of zooplankton in Lake Victoria basin, Kenya,” in 11th World Lakes Conference Nairobi, Kenya.
Namugize, J. N., Jewitt, G., and Graham, M. (2018). Effects of land use and land cover changes on water quality in the uMngeni river catchment, South Africa. Phys. Chem. Earth A/B/C 105, 247–264. doi: 10.1016/j.pce.2018.03.013
Ndebele-Murisa, M. R., Musil, C. F., and Raitt, L. (2010). A review of phytoplankton dynamics in tropical African lakes. South Afri. J. Sci. 106, 13–18. Available online at: https://hdl.handle.net/10520/EJC97007
Ngupula, G. W., Waya, R. K., and Ezekiel, C. N. (2010). Spatial and temporal patterns in abundance and distribution of zooplankton in the Tanzanian waters of Lake Victoria. Aquat. Ecosyst. Health Manag. 13, 451–457. doi: 10.1080/14634988.2010.525077
Nguyen, H. Q., Curry, R. A., Monk, W. A., Culp, J., and Linnansaari, T. (2019). Seasonal differences in plankton community structure are more pronounced than spatial patterns in the head pond and downstream portions of a large impounded river. Inland Waters 9, 348–361. doi: 10.1080/20442041.2018.1563397
Niyoyitungiye, L., Giri, A., and Mishra, B. P. (2019). Effect of Physico-Chemical attributes on the abundance and spatial distribution of fish species in Lake Tanganyika, Burundian coast. Int. J. Adv. Res. 7, 410–424. Availalbe online at: https://hal.archives-ouvertes.fr/hal-02462893/document
Okech, E. O., Kitaka, N., Oduor, S. O., and Verschuren, D. (2018). Trophic state and nutrient limitation in Lake Baringo, Kenya. Afr. J. Aquat. Sci. 43, 169–173. doi: 10.2989/16085914.2018.1462139
Okemwa, E. N. (1981). “A preliminary survey of the fishes and limnology of Lake Kanyaboli and Lake Sare in Western Kenya,” in Proceedings of the Workshop of the Kenya Marine and Fisheries Research on 'Aquatic Resources of Kenya, 192–211.
Oksanen, J., Blanchet, F. G., Kindt, R., Legendre, P., Minchin, P. R., O'hara, R. B., et al. (2013). Package ‘vegan’. Community ecology package, version, 2, 1–295.
Olele, N. F., and Ekelemu, J. K. (2021). Physicochemical and periphyton/phytoplankton study of Onah Lake, Asaba, Nigeria. Afri. J. Gen. Agri. 4, 183–194. Availalbe online at: https://ojs.klobexjournals.com/index.php/ajga/article/view/765/823
Onyango, D. M., Orina, P. S., Ramkat, R. C., Kowenje, C., Githukia, C. M., Lusweti, D., et al. (2020). Review of current state of knowledge of microcystin and its impacts on fish in Lake Victoria. Lake Reservoir: Res Manag. 25, 350–361. doi: 10.1111/lre.12328
Opiyo, S., and Dadzie, S. (2009). Diet and food utilization in Oreochromis esculentus (Graham) in Lake Kanyaboli, Kenya. Fisheries Manage. Ecol. 1. 79–90. doi: 10.1111/j.1365-2400.1970.tb00008.x
Owiyo, P., Kiprono, E., and Sutter, P. (2014). The effect of dominion irrigation project on environmental conservation in Yala Swamp, Siaya District, Kenya. Int. J. Sci. Res. 3, 82–87.
Paerl, H. W., Xu, H., McCarthy, M. J., Zhu, G., Qin, B., Li, Y., et al. (2011). Controlling harmful cyanobacterial blooms in a hyper-eutrophic lake (Lake Taihu, China): the need for a dual nutrient (N & P) management strategy. Water Res. 45, 19731983. doi: 10.1016/j.watres.2010.09.018
Pijanowska, J. (1990). Cyclomorphosis in Daphnia: an adaptation to avoid invertebrate Hydrobiologia. 200, 41–50. doi: 10.1007/BF00048621
Pinto-Coelho, R., Pinel-Alloul, B., Méthot, G., and & Havens, K. (2005). Crustacean zooplankton in lakes and reservoirs of temperate and tropical regions: variation with trophic status. Canad. J.Fisheries Aquatic Sci. 62, 348–361. doi: 10.1139/f04-178
Prescott, G. W. (1982). Algae of the Western Great Lakes Area With an Illustrated Key to the Genera of Desmids and Freshwater Diatoms. Koeningstein: Otto Koeltz Science Publishers.
Razak, S. B. A., and Sharip, Z. (2019). Spatio-temporal variation of zooplankton community structure in tropical urban waterbodies along trophic and urban gradients. Ecol. Processes 8, 1–12. doi: 10.1186/s13717-019-0196-2
Rodger, H. D., Tunbull, T., Eddwardds, C., and Codd, G. (1994). Cyanobacterial bloom associated pathology in brown trout Salmo trutta L. in Loch Leven, Scotland. J. Fish Dis. 17, 177–181. doi: 10.1111/j.1365-2761.1994.tb00211.x
Rosińska, J., Romanowicz-Brzozowska, W., Kozak, A., and Gołdyn, R. (2019). Zooplankton changes during bottom-up and top-down control due to sustainable restoration in a shallow urban lake. Environ. Sci. Pollut. Res. 26, 19575–19587. doi: 10.1007/s11356-019-05107-z
Scheffer, M., Hosper, S. H., Meijer, M. L., Moss, B., and Jeppesen, E. (1993). Alternative equilibria in shallow lakes. Trends Ecol. Evolut. 8, 275–279. doi: 10.1016/0169-5347(93)90254-M
Sharma, R. C., Singh, N., and Chauhan, A. (2016). The influence of physico-chemical parameters on phytoplankton distribution in a head water stream of Garhwal Himalayas: a case study. Egypt. J. Aquat. Res. 42, 11–21. doi: 10.1016/j.ejar.2015.11.004
Shukla, P. P., and Singh, A. (2013). A seasonal variations of plankton population of Maheshara Lake in Gorakhpur, India. World J. Zool. 8, 9–16.
Simiyu, B. M., Oduor, S. O., Rohrlack, T., Sitoki, L., and Kurmayer, R. (2018). Microcystin content in phytoplankton and in small fish from eutrophic Nyanza Gulf, Lake Victoria, Kenya. Toxins 10, 275. doi: 10.3390/toxins10070275
Sindt, A. R., and Wolf, M. C. (2021). Spatial and temporal trends of Minnesota River phytoplankton and zooplankton. River Res. Appl. 2021, 1–20. doi: 10.1002/rra.3796
Sitoki, L., Gichuki, J., Ezekiel, C., Wanda, F., Mkumbo, O. C., and Marshall, B. E. (2010). The environment of Lake Victoria (East Africa): current status and historical changes. Int. Rev. Hydrobiol. 95, 209–223. doi: 10.1002/iroh.201011226
Soares, M. C. S., Lobão, L. M., Vidal, L. O., Noyma, N. P., Barros, N. O., Cardoso, S. J., et al. (2011). “Light microscopy in aquatic ecology: Methods for plankton communities studies,” in Methods in Molecular Biology (Methods and Protocols), Vol 689, eds H. Chiarini-Garcia and R. Melo (Totowa, NJ: Humana Press). doi: 10.1007/978-1-60761-950-5_13
Sommer, U., Gliwicz, Z. M., Lampert, W., and Duncan, A. (1986). The PEG-model of seasonal succession of planktonic events in fresh waters. Archiv für Hydrobiol. 106, 433–471.
Sommer, U., Padisák, J., Reynolds, C. S., and Juhász-Nagy, P. (1993). Hutchinson's heritage: the diversity-disturbance relationship in phytoplankton. Hydrobiologia 249, 1–7. doi: 10.1007/BF00008837
Srifa, A., Phlips, E. J., Cichra, M. F., and Hendrickson, J. C. (2016). Phytoplankton dynamics in a subtropical lake dominated by cyanobacteria: cyanobacteria “Like it Hot” and sometimes dry. Aquatic Ecol. 50, 163–174. doi: 10.1007/s10452-016-9565-4
Stamou, G., Katsiapi, M., Moustaka-Gouni, M., and Michaloudi, E. (2021). The neglected zooplankton communities as indicators of ecological water quality of Mediterranean lakes. Limnetica. 40, 359–373. doi: 10.23818/limn.40.24
Swanepoel, A., du Preez, H., Schoeman, C., Janse van Vuuren, S., and Sundram, A. (2008). Condensed Laboratory Methods for Monitoring Phytoplankton, Including Cyanobacteria, in South African freshwaters. Water Research Commission Report No. TT 323(08). Gezina, South Africa.
Takamura, N., Kadono, Y., Fukushima, M., Nakagawa, M., and Kim, B. H. (2003). Effects of aquatic macrophytes on water quality and phytoplankton communities in shallow lakes. Ecol. Res. 18, 381–395. doi: 10.1046/j.1440-1703.2003.00563.x
Talling, J. E. (1987). The phytoplankton of Lake Victoria (East Africa). Arch. Hydrobiol. 25, 229–256.
Talling, J. F., and Lemoalle, J. (1998). Ecological Dynamics of Tropical Inland Waters. Cambridge: Cambridge University Press.
Thakur, R. K., Jindal, R., Singh, U. B., and Ahluwalia, A. S. (2013). Plankton diversity and water quality assessment of three freshwater lakes of Mandi (Himachal Pradesh, India) with special reference to planktonic indicators. Environ. Monitor. Access. 185, 8355–8373. doi: 10.1007/s10661-013-3178-3
Tian, C., Lu, X., Pei, H., Hu, W., and Xie, J. (2013). Seasonal dynamics of phytoplankton and its relationship with the environmental factors in Dongping Lake, China. Environ. Monitor. Assess. 185, 2627–2645. doi: 10.1007/s10661-012-2736-4
Uye, S., Shimazu, T., Yamamuro, M., Ishitobi, Y., and Kamiya, H. (2000). Geographical and seasonal variations in mesozooplankton abundance and biomass in relation to environmental parameters in Lake Shinji–Ohashi River–Lake Nakaumi brackish-water system, Japan. J. Marine Syst. 26, 193–207. doi: 10.1016/S0924-7963(00)00054-3
Visser, P. M., Ibelings, B. W., Bormans, M., and Huisman, J. (2015). Artificial mixing to control cyanobacterial blooms: a review. Aquatic Ecol. 50, 423–441. doi: 10.1007/s10452-015-9537-0
Walker, J. L., Younos, T., and Zipper, C. E. (2007). Nutrients in Lakes and Reservoirs: A Literature Review for use in Nutrient Criteria Development. VirginiaWater Resource Research Center SR34. Virginia, USA.
Walumona, J. R., Odoli, C. O., Raburu, P., Amisi, F. M., Murakaru, M. J., Kondowe, B. N., et al. (2021). Spatio-temporal variations in selected water quality parameters and trophic status of Lake Baringo, Kenya. Lakes Reserv. 26, e12367. doi: 10.1111/lre.12367
Wang, L., Wang, C., Deng, D., Zhao, X., and Zhou, Z. (2015). Temporal and spatial variations in phytoplankton: correlations with environmental factors in Shengjin Lake, China. Environ. Sci. Pollut. Res. 22, 14144–14156. doi: 10.1007/s11356-015-4640-2
Waya, R. K., Limbu, S. M., Ngupula, G. W., Mwita, C. J., and Mgaya, Y. D. (2014). Spatial patterns of zooplankton distribution and abundance in relation to phytoplankton, fish catch and some water quality parameters at Shirati Bay, Lake Victoria-Tanzania. Tanzania J. Sci. 40, 20–32.
Wilfred, O., Wakwabi, E., Sitoki, L., Guya, F., Jembe, T., and Ogutu, Z. (2005). “Effects of physical mixing on the environment of satellite lakes and dams of Lake Victoria, Kenya,” in 11th World Lakes Conference (Nairobi).
Willén, E. (2000). Phytoplankton in water quality assessment–an indicator concept. Hydrol. Limnol. Aspects Lake Monitoring 57, 80.
Witty, L. M. (2004). Practical Guide to Identifying Freshwater Crustacean Zooplankton. Sudbury, ON: Cooperative Freshwater Ecology Unit.
Wondie, A., Mengistu, S., Vijverberg, J., and Dejen, E. (2007). Seasonal variation in primary production of a large high altitude tropical lake (Lake Tana, Ethiopia): effects of nutrient availability and water transparency. Aquat. Ecol. 41, 195–207. doi: 10.1007/s10452-007-9080-8
Yang, C., Song, X., and Sun, B. (2007). Comparison of simple methods for extraction and measurement of phytoplanktonic chlorophyll-a. Mar. Sci. 31, 6–9.
Yang, W., Zheng, Z., Zheng, C., Lu, K., Ding, D., and Zhu, J. (2018). Temporal variations in a phytoplankton community in a subtropical reservoir: an interplay of extrinsic and intrinsic community effects. Sci. Total Environ. 612, 720–727. doi: 10.1016/j.scitotenv.2017.08.044
Yu, Y., Zhang, Q., Liu, G., Deng, Y., Kang, J., Zhang, F., et al. (2021). Proteomic analysis of zebrafish brain damage induced by Microcystis aeruginosa bloom. Sci. Total Environ. 795, 148865. doi: 10.1016/j.scitotenv.2021.148865
Yusuf, Z. H. (2020). Phytoplankton as bioindicators of water quality in Nasarawa reservoir, Katsina State Nigeria. Acta Limnologica Brasiliensia. 32, e4. doi: 10.1590/S2179-975X3319
Zakaria, H., A., and El-Naggar, H. (2019). Long-term variations of zooplankton community in Lake Edku, Egypt. Egypt. J. Aquat. Biol. Fisheries 23, 215–226. doi: 10.21608/ejabf.2019.53997
Zhou, J., Qin, B., Casenave, C., Han, X., Yang, G., Wu, T., et al. (2015). Effects of wind wave turbulence on the phytoplankton community composition in large, shallow Lake Taihu. Environ. Sci. Pollut. Res. 22, 12737–12746. doi: 10.1007/s11356-015-4535-2
Keywords: water quality, plankton, Yala Swamp, anthropogenic activities, nutrients, Lake Kanyaboli
Citation: Kondowe BN, Masese FO, Raburu PO, Singini W, Sitati A and Walumona RJ (2022) Seasonality in Environmental Conditions Drive Variation in Plankton Communities in a Shallow Tropical Lake. Front. Water 4:883767. doi: 10.3389/frwa.2022.883767
Received: 25 February 2022; Accepted: 28 March 2022;
Published: 04 May 2022.
Edited by:
Tatenda Dalu, University of Mpumalanga, South AfricaReviewed by:
Mmabaledi Buxton, Botswana International University of Science and Technology, BotswanaLinton Munyai, University of Venda, South Africa
Copyright © 2022 Kondowe, Masese, Raburu, Singini, Sitati and Walumona. This is an open-access article distributed under the terms of the Creative Commons Attribution License (CC BY). The use, distribution or reproduction in other forums is permitted, provided the original author(s) and the copyright owner(s) are credited and that the original publication in this journal is cited, in accordance with accepted academic practice. No use, distribution or reproduction is permitted which does not comply with these terms.
*Correspondence: Frank O. Masese, fmasese@uoeld.ac.ke; f.masese@gmail.com