- 1Department of Civil, Environmental and Architectural Engineering, University of Padova, Padua, Italy
- 2Department of Environmental Science and Policy Management, University of California, Berkeley, Berkeley, CA, United States
Data assimilation applications in integrated surface-subsurface hydrological models (ISSHMs) are generally limited to scales ranging from the hillslope to local or meso-scale catchments. This is because ISSHMs resolve hydrological processes in detail and in a physics-based fashion and therefore typically require intensive computational efforts and rely on ground-based observations with a small spatial support. At the other end of the spectrum, there is a vast body of literature on remote sensing data assimilation for land surface models (LSMs) at the continental or even global scale. In LSMs, some hydrological processes are usually represented with a coarse resolution and in empirical ways, especially groundwater lateral flows, which may be very important and yet often neglected. Starting from the review of some recent progress in data assimilation for physics-based hydrological models at multiple scales, we stress the need to find a common ground between ISSHMs and LSMs and suggest possible ways forward to advance the use of data assimilation in integrated hydrological models.
Introduction
Physics-based hydrological modeling
Physics-based hydrological models are important in earth and environmental sciences thanks to their inherent capability of being applicable to the widest possible range of scales and environmental conditions (Fatichi et al., 2016). These models are increasingly being used to predict future water resources quantity and quality in response to climate and land use change, to monitor and assess hydroclimatic hazards (such as floods and droughts), and in general to understand the intertwined dynamics between the hydrological, atmospheric, and carbon cycles. They are playing an ever-increasing role, also thanks to their capabilities of considering multiple compartments of the terrestrial water cycle (Clark et al., 2015). Depending on the scale of interest, we refer here to two different categories of physics-based hydrological models: local to meso-scale integrated surface-subsurface hydrological models (ISSHMs) and continental to global-scale land surface and hydrological models (LSMs/GHMs).
Integrated surface-subsurface hydrological models (Furman, 2008; Maxwell et al., 2014; Paniconi and Putti, 2015; Kollet et al., 2018) typically resolve the Richardson-Richards equation (or some approximation of it) in the subsurface, coupled with some form of the shallow water equation for surface flow. Although their spatial resolution varies widely (from a few meter to several hundred meters), due to their high computational requirements and need for detailed parameterization, ISSHMs are usually applied to relatively small spatial domains (i.e., from hillslope to regional or meso-scale catchments), with a handful of notable recent exceptions at the continental scale (Keune et al., 2016, 2018; Maxwell and Condon, 2016; Kollet et al., 2018; Condon and Maxwell, 2019; Condon et al., 2020a,b).
Global hydrological and land surface models (LSM/GHM), on the other hand, were first developed in the context of earth system modeling to resolve energy and mass balance processes at the interface between the land surface and the atmosphere. Their spatial resolution typically ranges from 10 to 50 km, which are often inadequate to fully capture the fine-scale variability of surface and subsurface hydrological processes. As an example, they typically lack the representation of processes in highly heterogeneous areas such as mountains and coasts, which are also amongst the most vulnerable regions to hydro-climatic hazards (Balica et al., 2012; Elalem and Pal, 2015).
Regardless of the scale of interest, both ISSHMs and LSM/GHMs rely on accurate representation of the hydrological physical processes as well as input meteorological forcing data (e.g., precipitation, air temperature, wind speed, etc.), and parameters (e.g., land cover, soil type, soil properties, etc.), all of which can be difficult to obtain and can lead to large uncertainties in modeled hydrological states and fluxes (Collier et al., 2018), especially when generalizing a model to larger spatial domain. These uncertainties (and errors) inherently affect all estimates of hydrological states (e.g., water table or soil moisture) obtained through modeling. However, they can be quantified and limited through data assimilation approaches.
Data assimilation
Data Assimilation (DA) refers to a suite of statistical techniques that incorporate observation data into mathematical models, with the goal of optimizing estimates of the system state (and possibly parameters). The results of a DA framework should be a statistically optimal estimate, superior to that from either the model or observations alone (Evensen et al., 2022). DA was pioneered by meteorologists and has been used to improve operational weather forecasts for decades. The first application of data assimilation in ISSHMs dates to the early 2000s (Paniconi et al., 2003). Since then, much progress has been made, also thanks to the widespread diffusion of ensemble-based assimilation methods such as the ensemble Kalman filter and its variants (Evensen, 2003). Data assimilation for LSM/GHMs has a similar history, with significant advancements over the past decade for estimating hydrological land surface variables (Reichle et al., 2002; de Rosnay et al., 2014).
In general, LSM/GHM DA previous developments involve univariate data assimilation, i.e., assimilation of one observation to adjust model states (e.g., soil moisture) or parameters such as precipitation scaling factors (e.g., Liu and Margulis, 2019; Girotto et al., 2021), albedo (Navari et al., 2018), and vegetation properties (Smith et al., 2020). More recently, the scientific community started to target multi-observational approaches. The simultaneous assimilation of multiple observation data strongly improves model predictions compared with single observation and/or state estimation alone (e.g., Girotto et al., 2019; Kumar et al., 2019; Khaki et al., 2020). Data assimilation applications in ISSHMs typically involve the assimilation of multiple measurements in both the surface (soil moisture, streamflow) and subsurface compartments (pressure head, water table depth) with or without the update of model parameters (e.g., Camporese et al., 2009a,b; Pasetto et al., 2012; Rasmussen et al., 2015, 2016; Zhang et al., 2015, 2016; Ridler et al., 2018; Gebler et al., 2019; He et al., 2019).
While promising in overcoming models and observations uncertainties, current literature in data assimilation for physically based hydrological studies agree that: (i) it is very challenging to improve model estimates in one compartment assimilating measurements from another (Camporese et al., 2009b; Zhang et al., 2016; Botto et al., 2018); (ii) the joint update of system states and model parameters usually leads to better results (Botto et al., 2018; Gebler et al., 2019); (iii) data assimilation applications in integrated surface-subsurface hydrological models are generally limited to scales that go from the hillslope (Botto et al., 2018; Gebler et al., 2019) to local-scale (He et al., 2019) or meso-scale catchments (Rasmussen et al., 2016; Ridler et al., 2018), mainly due to computational constraints; (iv) the robustness of the assimilation application depends upon the accurate characterization of the combined modeling and observation uncertainties, a task that can be particularly challenging especially for large spatial domains (Kumar et al., 2017).
Statement of the problem: The scale gap
From the analysis of recent literature in hydrological data assimilation, a distinct scale gap emerges. Ground observations can have a high time resolution but are typically characterized by a very small spatial support, making them ideal for assimilation in local catchment-scale or hillslope models, where the size of the computational cell/element can be of the same order of magnitude of the measurement volume. However, they are expensive with respect to the (limited) spatial coverage they offer. At the other end of the spectrum, satellite-based observations are under continuous development to reduce their uncertainties and provide additional information, thanks to their large spatial coverage; for this reason, they are commonly assimilated in LSMs/GHMs. However, their use with ISSHMs have been hindered by their typically coarse resolution, which is not consistent with scale requirements of ISSHMs (Samaniego et al., 2010; Or et al., 2015; Or, 2020). In addition, hyper-resolution modeling at continental to global scales with ISSHMs still requires prohibitive computational efforts for them to be used in ensemble-based data assimilation frameworks. The main goal of this mini-review is to discuss possible new directions for hydrological modeling, observations, and DA methodologies, which could lead to advances in data assimilation for larger spatial domains or to unlock the potential of remote sensing data for assimilation in physics-based hydrological models.
Bridging the gaps between spatial scales
In the following, we discuss recent developments, outstanding challenges, and possible research directions in physics-based hydrological modeling (Section Recent advances and outstanding challenges in physically based hydrological modeling), relevant observations (Section Recent advances and outstanding challenges in observation data), and assimilation systems (Section Data assimilation for non-gaussian systems).
Recent advances and outstanding challenges in physically based hydrological modeling
In many cases, the breadth of differences in the data generated from in-situ and remote sensing observations raises significant questions on how to best use these data in modeling development and testing. Land surface and groundwater have intertwined processes, yet their scientific modeling fields are still disconnected.
Over the past decade, the LSM/GHM modeling community has been embracing hyper-resolution (~1-km or finer) integrated surface-subsurface hydrological modeling (Wood et al., 2011; Bierkens et al., 2015; Condon et al., 2021), therefore providing the opportunity for the two modeling categories (ISSHMs and LSM/GHMs) to start meeting in the middle while resolving regional to continental scale surface-subsurface hydrological processes. Moving LSM/GHMs to higher resolutions and/or ISSHMs to larger scales is posing huge challenges, mainly represented by uncertainties due to the lack of fine scale model processes and parameters knowledge (Beven and Cloke, 2012). Some of these challenges are listed as follows.
First, while meso-scale natural processes, such as precipitation-induced runoff or evaporation, are included in most LSM/GHMs, more local anthropogenic processes, such as irrigation, are rarely modeled, especially in LSMs (Bierkens et al., 2015). Human driven processes can directly control the groundwater (GW) table, for instance, by lowering the GW table through pumping and by increasing recharge via flood irrigation. To date, representing human-induced impacts in continental to global scale hydrological models remains a major challenge, mostly due to the lack of accurate global irrigation information (Wada et al., 2017).
Second, despite advancements in land surface model complexity, most LSM/GHMs route moisture in the vertical dimension only, and allow no lateral communication between vertical soil columns, i.e., they do not represent GW dynamics. Such an assumption implies that controls of GW on land-surface interactions, and consequently hydro-climatic hazards, may be overlooked (Keune et al., 2016; Maxwell and Condon, 2016). Subsurface flow can also be crucial for a series of applications including (and not limited to) drought monitoring, flood predictions, and water resources management. Only recently, the land surface community started to recognize that lateral groundwater flow significantly interacts with surface processes such as vegetation dynamics (Gochis et al., 2018; Zeng et al., 2018; Forrester and Maxwell, 2020; Rummler et al., 2022), hydro-climatic hazards (Felsberg et al., 2021), and atmospheric forecasting (Getirana et al., 2020) and consequently to put efforts in representing this process (e.g., Batelis et al., 2020).
Third, although the number of high-resolution datasets such as topography, land use, geology, and soil properties are increasing, these data are also the products of remote sensing, land surface models, statistical downscaling techniques, or combinations thereof and thus affected by inaccuracies and uncertainties.
Finally, executing physically based models at high spatial resolution, while resolving surface and subsurface water dynamics in a coupled way and over large regions is computationally demanding, both in terms of CPU time and storage requirements (Kollet et al., 2010; Maxwell, 2013).
These challenges suggest the need to develop new modeling approaches at continental and global scales that can properly simulate hydrological processes (especially lateral subsurface flows) at resolutions compatible with remote sensing data (i.e., resolutions used in the LSM/GHM community). To this end, machine learning and deep learning techniques are recently being explored to emulate complex subsurface physical processes (Radmanesh et al., 2020; Tran et al., 2021) and also to link modeled estimates with indirect measurements of the state variables (e.g., to link moisture states to radiances observations, Section Earth observations).
Recent advances and outstanding challenges in observation data
Earth observations
Earth observation data (aka satellite or remote sensing) is essential to monitor hydrologic variables such as soil moisture and groundwater table because it provides a bird's-eye view of the Earth's dynamics. In the last several decades, the number of spaceborne sensors has rapidly increased. These observing systems can detect subsurface hydrologic states such as the soil moisture at various spatial and temporal resolutions (e.g., from ~1 to 40 km resolutions, Entekhabi et al., 2010; Fang et al., 2021). Despite this apparent wealth of new data, there are lingering methodological challenges to translate these types of observations into water table depth. In fact, the basic remote sensing principle involves the direct interaction between an incident radiation (e.g., natural solar radiation or active radiation sent from a sensor) and the targets of interest. Groundwater is a hidden resource and therefore cannot be directly observed and measured from space. Ground-based water table depth monitoring and reporting are scarce and variable across the globe, and frequently limited to developed regions. For this reason, little is still known about the global patterns of the water table depth and its interactions with the land, urban and natural ecosystems, hindering our capabilities to fully exploit the potential of water table information in data assimilation for global-scale integrated hydrological modeling.
Possible ways forward are offered by the fact that groundwater can be indirectly observed through other quantities, for example evaporation fluxes (Miralles et al., 2011) and total terrestrial water storage (TWS) (Famiglietti and Rodell, 2013).
Water table dynamics are linked to the atmosphere through evapotranspiration and recharge. From a water budget perspective, the accurate account of water losses via evapotranspiration and runoff should lead to improved estimates of recharge fluxes, and thus water table depth. Recent studies have shown that assimilation of remotely sensed evapotranspiration estimates can inform the groundwater table dynamics (Gelsinari et al., 2020).
Since 2002, TWS estimates have been derived from the Gravity Recovery and Climate Experiment (GRACE) and its follow-on mission (GRACE-FO). These missions provide valuable information on groundwater conditions beyond what can be seen at the surface (Li et al., 2012; Scanlon et al., 2018) because it measures the water storage changes in the entire terrestrial water storage (i.e., including snow, vegetation biomass, surface soil moisture, root-zone soil moisture, and GW). However, limitations still exist for using GRACE data in operational groundwater dynamics monitoring. The major limitations are related to (i) the data delivery latency; (ii) the relatively coarse spatial and temporal resolutions of GRACE observations; and (iii) the fact that TWS is an aggregated observation of multiple water storage components. The assimilation of GRACE data in an LSM allows us to downscale the TWS observations spatially and temporally into its various water storage components such as surface and root zone soil moisture and groundwater table (Girotto et al., 2016; Kumar et al., 2016).
Another interesting opportunity is given by processes linking changes in GW level to deformation of the Earth's crust and thus vertical land motion (Erban et al., 2014; Darvishi et al., 2021). As opposed to tectonic and sediment compaction, the vertical land motion associated with surface water or groundwater table changes can be visible at sub-annual temporal scales. Some GW systems are more susceptible to compaction compared to others. In some cases, depletion and recharge of aquifers can cause vertical land motion with rates up to ~10 cm/year (Carlson et al., 2020). Elastic deformation typically results in small-magnitude recoverable displacements (on the mm to cm scale) of the land surface (Shirzaei et al., 2020). When the effective stress exceeds a stress threshold, the deformation is inelastic and results in permanent (irreversible) subsidence. Recent studies have quantified the elastic contributions to vertical land motion and related it to GW (Chaussard and Farr, 2019; Smith and Knight, 2019; Hsu et al., 2020; Lu et al., 2020; Ojha et al., 2020; Tangdamrongsub and Šprlák, 2021). Deriving accurate global soil compaction parameters and modules to be coupled to hydrological models can be complex, if not impossible. For data assimilation, this limitation can be addressed with the development of an artificial intelligence (i.e., neural network) forward model to link vertical deformation to changes in the variable of interest (i.e., groundwater table) (Smith and Majumdar, 2020; Naghibi et al., 2022).
In addition to improved estimates of the driving forces, as detailed above, an accurate, detailed and spatio-temporal assessment of groundwater fluxes requires also a reliable estimate of soil and aquifer parameters, to avoid errors in parameters leading to over- or underestimation of fluxes. Starting from prior parameter distributions that can be extracted from globally available datasets (e.g., Batjes, 1997; Hengl et al., 2017), data assimilation allows for their dynamic update, which can lead to improved model predictions (e.g., Hung et al., 2022).
Intermediate-Scale sensing technologies
One possible way forward to expand the capabilities of DA for integrated hydrological modeling is offered by novel sensing technologies at intermediate scales that go beyond the local support scale typical of ground observations. While traditional soil moisture, water table, and pressure head observations have a support volume of a few cubic centimeters or decimeters, fiber optics sensing (FOS), airborne electro-magnetic methods (AEMs), cosmic ray neutron sensing (CRNS), and unmanned aerial vehicles (UAVs) represent relatively cheap (compared to the possible extent of the surveys) options to collect hydrologically relevant data at scales ranging from tens of meters to hundreds of kilometers.
Fiber optic sensing can be used to measure, among other variables, strain, displacement, pressure, and temperature with high spatial resolution for distances of several kilometers (Leone, 2022). Fiber optic distributed temperature sensing (FO-DTS), in particular, represents a promising technique to measure soil water at high spatial resolutions (<1 m), thanks to the relationship between soil thermal properties and soil moisture content (Sayde et al., 2010, 2014; Steele-Dunne et al., 2010; Striegl and Loheide, 2012; Dong et al., 2017; Vidana Gamage et al., 2018). However, to the best of our knowledge, FOS data have never been used for DA studies in integrated hydrological modeling and still represent an underexploited opportunity.
Much progress has been made in the past decade to assimilate hydrogeophysical observations in hydrological models. In particular, Electrical Resistivity (ER) data, thanks to their sensitivity to soil moisture and salinity, have demonstrated their usefulness in parameterizing subsurface systems (e.g., Camporese et al., 2015; Crestani et al., 2015; Manoli et al., 2015; Tso et al., 2020). However, all these studies have focused on local-scale systems, while hydrogeophysics now offers the opportunity to survey large extensions of land with AEM methods. They potentially allows for the estimation of key groundwater parameters, such as hydraulic conductivity, with remotely sensed geophysical data collected over thousands of square kilometers, while still being able to capture heterogeneities at scales of interest for physics-based hydrological models (~101 m) (e.g., Christensen et al., 2017; Knight et al., 2018; Vittecoq et al., 2019). So far, AEM surveys have been typically conducted to get a static picture of the subsurface structure and parameters. However, if repeated over time (despite their costs), these surveys might provide valuable datasets to be used in data assimilation studies for integrated hydrological modeling, such as variations of groundwater levels over large areas, which could complement information provided by GRACE.
Cosmic ray neutron probes are used in several fields of hydrology as they offer the possibility of estimating average snow water equivalent (Schattan et al., 2017) and soil moisture over areas of 130–240 m of radius and to depths of ~15–80 cm, depending on the soil moisture itself (Köhli et al., 2015). Using an Ensemble Adjustment Kalman Filter, Patil et al. (2021) assimilated neutron counts for a 655 km2 catchment into the Noah-MP land surface model, showing that incorporating information from CRNS can improve parameter and soil moisture estimates and paving the way for future applications also in ISSHMs.
The increasing use of UAVs in all science disciplines, whereby multiple types of sensors can be easily deployed over relatively large areas, also provides us with plenty of opportunities to assimilate high-resolution data at intermediate scales. Assimilation of UAV-collected data is becoming common in weather modeling (Sun et al., 2020; Jensen et al., 2021) and crop modeling (Yu et al., 2019; Peng et al., 2021). However, incorporation of UAV data in physics-based hydrological modeling is still largely unexplored. Tang et al. (2018) used river bathymetry data collected through UAV surveys, in conjunction with measurements of hydraulic head assimilated via EnKF, to improve model predictions and parameters with HydroGeoSphere (Brunner and Simmons, 2012), an ISSHM, for a river catchment in Switzerland. Given the capabilities of UAV-mounted sensors to measure a wide range of hydrological (e.g., soil moisture) and vegetation (e.g., leaf area index) variables, we see much room for DA studies in physics-based hydrological modeling in the next few years.
Data assimilation for non-gaussian systems
A common approach in data assimilation is the ensemble Kalman filter (EnKF; Evensen, 2003) that dynamically and sequentially updates model error covariance information by producing an ensemble of model predictions, which are individual model realizations perturbed by the assumed model error (Reichle et al., 2002). EnKF systems have been targeted by both ISSHMs (e.g., Camporese et al., 2009b; Pasetto et al., 2012) and LSM/GHMs (de Rosnay et al., 2014; Kumar et al., 2016; Girotto et al., 2019). Some common challenges include the need of spatially localizing EnKF covariances to remove spurious correlations, and most importantly its assumption of multi-gaussianity, which must be met for both system states and observations. If this assumption is not satisfied, the EnKF only gives sub-optimal results or, in the worst cases, fails to give an adequate representation of the variables of interest. Other, arguably more sophisticated methods, include particle filter (PF) techniques (e.g., Crisan, 2001) or combinations of EnKF and PF (e.g., Stordal et al., 2011). Like the EnKF, the PF is a sequential Monte Carlo approach, but it does not depend on the assumption of Gaussian distributions, in principle being able to accommodate every probability distribution functions, including multimodal ones. However, PF techniques typically require larger ensembles to characterize the full probability distribution of the state variables and consequently their uncertainties via resampling sets of state variables. Due to this disadvantage, PF applications in physics-based hydrological models have been limited to a few cases (Pasetto et al., 2012; Zhang et al., 2017).
Interesting new developments for non-Gaussian DA applications in physics-based hydrological modeling could derive from applications of the transport theory (Villani, 2009). Ning et al., 2014 showed how transportation metrics in the framework of optimal mass transport can be used with variational data assimilation to obtain optimal estimates of the system state in the presence of systematic model errors or bias, for which no prior knowledge is available. More recently, Ramgraber et al. (2021) introduced their work on non-linear smoothing, whereby transport methods are applied as a pathway for non-linear/non-Gaussian generalization of two different ensemble Kalman-type smoothers.
Compared to traditional EnKF implementations, DA algorithms based on PF or transport methods require additional computational effort. However, due to the increased availability of ever more powerful computers, we expect this is going to be less of an issue in the near future.
Conclusions
Physics-based integrated hydrological modeling have been declined in different ways by two scientific communities. On one hand, integrated surface-subsurface hydrological models (ISSHMs), solving flow and transport processes in detail, are typically applied to local to meso-scale catchments. On the other hand, land surface and global hydrological models (LSMs/GHMs) focus on continental to global scale applications, paying more attention to hydrological processes at the land-atmosphere interface and representing subsurface processes with simplified approaches. Accordingly, data assimilation has long been used by the two communities in different ways, exploiting data types consistent with their respective scales, i.e., ground-based measurements for ISSHMs and remote sensing observations for LSMs/GHMs.
In this mini review, we advocate for the two communities to find common ground for advancing data assimilation at intermediate scales (Figure 1). This can be done by pursuing new hydrological modeling approaches that can result in less computational requirements for ISSHMs and more accurate process representation for LSMs/GHMs. Also, we gave an overview of opportunities for exploiting state-of-the-art technologies providing data types at intermediate scales that can be of interest for assimilation in both categories of models. Finally, as non-gaussianity is a common issue in hydrological models and makes the most used DA algorithms sub-optimal, we suggest exploring alternative and innovative DA methods that can lead to a more plausible representation of parameter and process spatial variability.
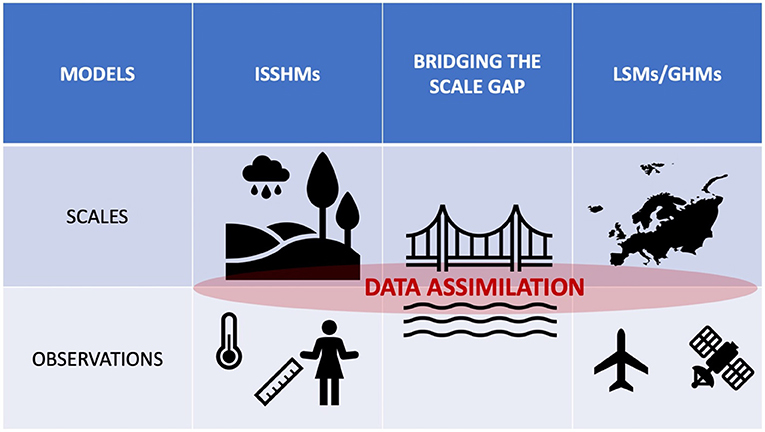
Figure 1. Data assimilation combines the strengths of modeled and observed hydrological estimates. Integrated surface-subsurface hydrological models (ISSHMs) typically operate within spatial scales ranging from hillslope to local-scale or meso-scale catchments and its data assimilation techniques primarily incorporate local ground-based observations. Land Surface (LSMs) and Global Hydrological models (GHMs) operate within continental and global spatial scales and their data assimilation applications utilize remotely sensed observations. This mini review discusses possible new directions for data assimilation technologies which could potentially bridge the scale gaps between the ISSHM and LSM/GHM scientific communities.
Ultimately, these developments can contribute to bridge the gap between the two DA and modeling communities, with obvious benefits for both.
Author contributions
All authors listed have made a substantial, direct, and intellectual contribution to the work and approved it for publication.
Conflict of interest
The authors declare that the research was conducted in the absence of any commercial or financial relationships that could be construed as a potential conflict of interest.
Publisher's note
All claims expressed in this article are solely those of the authors and do not necessarily represent those of their affiliated organizations, or those of the publisher, the editors and the reviewers. Any product that may be evaluated in this article, or claim that may be made by its manufacturer, is not guaranteed or endorsed by the publisher.
References
Balica, S. F., Wright, N. G., and Van der Meulen, F. (2012). A flood vulnerability index for coastal cities and its use in assessing climate change impacts. Nat. Haz. 64, 73–105. doi: 10.1007/s11069-012-0234-1
Batelis, S.-C., Rahman, M., Kollet, S., Woods, R., and Rosolem, R. (2020). Towards the representation of groundwater in the Joint UK land environment simulator. Hydrol. Process. 34, 2843–2863. doi: 10.1002/hyp.13767
Batjes, N. H. (1997). A world dataset of derived soil properties by FAO–UNESCO soil unit for global modelling. Soil Use Manage. 13, 9–16. doi: 10.1111/j.1475-2743.1997.tb00550.x
Beven, K. J., and Cloke, H. L. (2012). Comment on: Hyperresolution global land surface modeling: meeting a grand challenge for monitoring Earth's terrestrial water by Eric F Wood et al. Water Resour. Res. 48, 1801. doi: 10.1029/2011WR010982
Bierkens, M. F., Bell, V. A., Burek, P., Chaney, N., Condon, L. E., David, C. H., et al. (2015). Hyper-resolution global hydrological modelling: what is next? “Everywhere and locally relevant.” Hydrol. Process. 29, 310–320. doi: 10.1002/hyp.10391
Botto, A., Belluco, E., and Camporese, M. (2018). Multi-source data assimilation for physically based hydrological modeling of an experimental hillslope. Hydrol. Earth Syst. Sci. 22, 4251–4266. doi: 10.5194/hess-22-4251-2018
Brunner, P., and Simmons, C. T. (2012). HydroGeoSphere: a fully integrated, physically based hydrological model. Ground Water 50, 170–176. doi: 10.1111/j.1745-6584.2011.00882.x
Camporese, M., Cassiani, G., Deiana, R., Salandin, P., and Binley, A. (2015). Coupled and uncoupled hydrogeophysical inversions using ensemble K alman filter assimilation of ERT-monitored tracer test data. Water Resour. Res. 51, 3277–3291. doi: 10.1002/2014WR016017
Camporese, M., Paniconi, C., Putti, M., and Salandin, P. (2009a). Comparison of data assimilation techniques for a coupled model of surface and subsurface flow. Vadose Zone J. 8, 837–845. doi: 10.2136/vzj2009.0018
Camporese, M., Paniconi, C., Putti, M., and Salandin, P. (2009b). Ensemble Kalman filter data assimilation for a process-based catchment scale model of surface and subsurface flow. Water Resour. Res. 45, e2008WR007031. doi: 10.1029/2008WR007031
Carlson, G., Shirzaei, M., Werth, S., Zhai, G., and Ojha, C. (2020). Seasonal and long-term groundwater unloading in the central valley modifies crustal stress. J. Geophys. Res. Solid Earth 125, e2019JB018490. doi: 10.1029/2019JB018490
Chaussard, E., and Farr, T. G. (2019). A new method for isolating elastic from inelastic deformation in aquifer systems: application to the San Joaquin Valley, CA. Geophys. Res. Lett. 46, 10800–10809. doi: 10.1029/2019GL084418
Christensen, N. K., Ferre, T. P. A., Fiandaca, G., and Christensen, S. (2017). Voxel inversion of airborne electromagnetic data for improved groundwater model construction and prediction accuracy. Hydrol. Earth Syst. Sci. 21, 1321–1337. doi: 10.5194/hess-21-1321-2017
Clark, M. P., Fan, Y., Lawrence, D. M., Adam, J. C., Bolster, D., Gochis, D. J., et al. (2015). Improving the representation of hydrologic processes in earth system models. Water Resour. Res. 51, 5929–5956. doi: 10.1002/2015WR017096
Collier, N., Hoffman, F. M., Lawrence, D. M., Keppel-Aleks, G., Koven, C. D., Riley, W. J., et al. (2018). The international land model benchmarking (ILAMB) system: design, theory, and implementation. J. Adv. Model. Earth Syst. 10, 2731–2754. doi: 10.1029/2018MS001354
Condon, L. E., Atchley, A. L., and Maxwell, R. M. (2020a). Evapotranspiration depletes groundwater under warming over the contiguous United States. Nat. Commun. 11, 873. doi: 10.1038/s41467-020-14688-0
Condon, L. E., Kollet, S., Bierkens, M. F., Fogg, G. E., Maxwell, R. M., Hill, M. C., et al. (2021). Global groundwater modeling and monitoring: opportunities and challenges. Water Resourc. Res. 57, e2020WR029500. doi: 10.1029/2020WR029500
Condon, L. E., Markovich, K. H., Kelleher, C. A., McDonnell, J. J., Ferguson, G., and McIntosh, J. C. (2020b). Where is the bottom of a watershed? Water Resourc. Res. 56, e2019WR026010. doi: 10.1029/2019WR026010
Condon, L. E., and Maxwell, R. M. (2019). Simulating the sensitivity of evapotranspiration and streamflow to large-scale groundwater depletion. Sci. Adv. 5, eaav4574. doi: 10.1126/sciadv.aav4574
Crestani, E., Camporese, M., and Salandin, P. (2015). Assessment of hydraulic conductivity distributions through assimilation of travel time data from ERT-monitored tracer tests. Adv. Water Resour. 84, 23–36. doi: 10.1016/j.advwatres.2015.07.022
Crisan, D. (2001). “Particle Filters - A Theoretical Perspective,” in Sequential Monte Carlo Methods in Practice, eds A. Doucet, N. de Freitas and Gordon, N. (New York, NY: Statistics for Engineering and Information Science). Available online at: https://doi.org/10.1007/978-1-4757-3437-9_2
Darvishi, M., Destouni, G., Aminjafari, S., and Jaramillo, F. (2021). Multi-Sensor InSAR assessment of ground deformations around lake mead and its relation to water level changes. Remote Sens. 13, 406. doi: 10.3390/rs13030406
de Rosnay, P., Balsamo, G., Albergel, C., Muñoz-Sabater, J., and Isaksen, L. (2014). Initialisation of land surface variables for numerical weather prediction. Surv. Geophys. 35, 607–621. doi: 10.1007/s10712-012-9207-x
Dong, J., Agliata, R., Steele-Dunne, S., Hoes, O., Bogaard, T., Greco, R., et al. (2017). The impacts of heating strategy on soil moisture estimation using actively heated fiber optics. Sensors 17, 2102. doi: 10.3390/s17092102
Elalem, S., and Pal, I. (2015). Mapping the vulnerability hotspots over Hindu-Kush Himalaya region to flooding disasters. Weather Clim. Extrem. 8, 46–58. doi: 10.1016/j.wace.2014.12.001
Entekhabi, D., Njoku, E. G., O'Neill, P. E., Kellogg, K. H., Crow, W. T., Edelstein, W. N., et al. (2010). The soil moisture active passive (SMAP) mission. Proc. IEEE 98, 704–716. doi: 10.1109/JPROC.2010.2043918
Erban, L. E., Gorelick, S. M., and Zebker, H. A. (2014). Groundwater extraction, land subsidence, and sea-level rise in the Mekong Delta, Vietnam. Environ. Res. Lett. 9, 084010. doi: 10.1088/1748-9326/9/8/084010
Evensen, G. (2003). The ensemble Kalman filter: theoretical formulation and practical implementation. Ocean Dyn. 53, 343–367. doi: 10.1007/s10236-003-0036-9
Evensen, G., Vossepoel, F. C., and van Leeuwen, P. J. (2022). Data Assimilation Fundamentals: A Unified Formulation of the State and Parameter Estimation Problem. Springer Nature. doi: 10.1007/978-3-030-96709-3 Available online at: https://link.springer.com/book/10.1007/978-3-030-96709-3
Famiglietti, J. S., and Rodell, M. (2013). Water in the balance. Science 340, 1300–1301. doi: 10.1126/science.1236460
Fang, B., Lakshmi, V., Cosh, M. H., and Hain, C. (2021). Very high spatial resolution downscaled SMAP radiometer soil moisture in the CONUS using VIIRS/MODIS data. IEEE J. Select. Top. Appl. Earth Observ. Remote Sens. 14, 4946–4965. doi: 10.1109/JSTARS.2021.3076026
Fatichi, S., Vivoni, E. R., Ogden, F. L., Ivanov, V. Y., Mirus, B., Gochis, D., et al. (2016). An overview of current applications, challenges, and future trends in distributed process-based models in hydrology. J. Hydrol. 537, 45–60. doi: 10.1016/j.jhydrol.2016.03.026
Felsberg, A., De Lannoy, G. J., Girotto, M., Poesen, J., Reichle, R. H., and Stanley, T. (2021). Global soil water estimates as landslide predictor: the effectiveness of SMOS, SMAP and GRACE observations, land surface simulations and data assimilation. J. Hydrometeorol. 22, 1065–1084. doi: 10.1175/JHM-D-20-0228.1
Forrester, M. M., and Maxwell, R. M. (2020). Impact of lateral groundwater flow and subsurface lower boundary conditions on atmospheric boundary layer development over complex terrain. J. Hydrometeorol. 21, 1133–1160. doi: 10.1175/JHM-D-19-0029.1
Furman, A. (2008). Modeling coupled surface–subsurface flow processes: a review. Vadose Zone J. 7, 741–756. doi: 10.2136/vzj2007.0065
Gebler, S., Kurtz, W., Pauwels, V. R. N., Kollet, S. J., Vereecken, H., and Hendricks Franssen, H.-J. (2019). Assimilation of high-resolution soil moisture data into an integrated terrestrial model for a small-scale head-water catchment. Water Resour. Res. 55, 10358–10385. doi: 10.1029/2018WR024658
Gelsinari, S., Doble, R., Daly, E., and Pauwels, V. R. (2020). Feasibility of improving groundwater modeling by assimilating evapotranspiration rates. Water Resourc. Res. 56, e2019WR025983. doi: 10.1029/2019WR025983
Getirana, A., Rodell, M., Kumar, S., Beaudoing, H. K., Arsenault, K., Zaitchik, B., et al. (2020). GRACE improves seasonal groundwater forecast initialization over the United States. J. Hydrometeorol. 21, 59–71. doi: 10.1175/JHM-D-19-0096.1
Girotto, M., De Lannoy, G. J., Reichle, R. H., and Rodell, M. (2016). Assimilation of gridded terrestrial water storage observations from GRACE into a land surface model. Water Resour. Res. 52, 4164–4183. doi: 10.1002/2015WR018417
Girotto, M., Reichle, R., Rodell, M., and Maggioni, V. (2021). Data assimilation of terrestrial water storage observations to estimate precipitation fluxes: a synthetic experiment. Remote Sens. 13, 1223. doi: 10.3390/rs13061223
Girotto, M., Reichle, R. H., Rodell, M., Liu, Q., Mahanama, S., and De Lannoy, G. J. (2019). Multi-sensor assimilation of SMOS brightness temperature and GRACE terrestrial water storage observations for soil moisture and shallow groundwater estimation. Remote Sens. Environ. 227, 12–27. doi: 10.1016/j.rse.2019.04.001
Gochis, D. J., Barlage, M., Dugger, A., FitzGerald, K., Karsten, L., McAllister, M., et al. (2018). The WRF-Hydro modeling System Technical Description,(Version 5.0). NCAR Technical Note 107.
He, X., Lucatero, D., Ridler, M.-E., Madsen, H., Kidmose, J., Hole, Ø., et al. (2019). Real-time simulation of surface water and groundwater with data assimilation. Adv. Water Resour. 127, 13–25. doi: 10.1016/j.advwatres.2019.03.004
Hengl, T., Mendes de Jesus, J., Heuvelink, G. B., Ruiperez Gonzalez, M., Kilibarda, M., Blagoti,ć, A., et al. (2017). SoilGrids250m: global gridded soil information based on machine learning. PLoS ONE 12, e0169748. doi: 10.1371/journal.pone.0169748
Hsu, Y.-J., Fu, Y., Bürgmann, R., Hsu, S.-Y., Lin, C.-C., Tang, C.-H., et al. (2020). Assessing seasonal and interannual water storage variations in Taiwan using geodetic and hydrological data. Earth Planet. Sci. Lett. 550, 116532. doi: 10.1016/j.epsl.2020.116532
Hung, P. C., Schalge, B., Baroni, G., Vereecken, H., and Hendricks Franssen, H. (2022). Assimilation of groundwater level and soil moisture data in an integrated land surface-subsurface model for southwestern Germany. Water Resourc. Res. 58, e2021WR031549. doi: 10.1029/2021WR031549
Jensen, A. A., Pinto, J. O., Bailey, S. C., Sobash, R. A., de Boer, G., Houston, A. L., et al. (2021). Assimilation of a coordinated fleet of uncrewed aircraft system observations in complex terrain: EnKF system design and preliminary assessment. Mthly. Weather Rev. 149, 1459–1480. doi: 10.1175/MWR-D-20-0359.1
Keune, J., Gasper, F., Goergen, K., Hense, A., Shrestha, P., Sulis, M., et al. (2016). Studying the influence of groundwater representations on land surface-atmosphere feedbacks during the European heat wave in 2003. J. Geophys. Res. Atmosph. 121, 13301–13325. doi: 10.1002/2016JD025426
Keune, J., Sulis, M., Kollet, S., Siebert, S., and Wada, Y. (2018). Human water use impacts on the strength of the continental sink for atmospheric water. Geophys. Res. Lett. 45, 4068–4076. doi: 10.1029/2018GL077621
Khaki, M., Hendricks Franssen, H.-J., and Han, S. C. (2020). Multi-mission satellite remote sensing data for improving land hydrological models via data assimilation. Sci. Rep. 10, 18791. doi: 10.1038/s41598-020-75710-5
Knight, R., Smith, R., Asch, T., Abraham, J., Cannia, J., Viezzoli, A., et al. (2018). Mapping aquifer systems with airborne electromagnetics in the Central Valley of California. Groundwater 56, 893–908. doi: 10.1111/gwat.12656
Köhli, M., Schrön, M., Zreda, M., Schmidt, U., Dietrich, P., and Zacharias, S. (2015). Footprint characteristics revised for field-scale soil moisture monitoring with cosmic-ray neutrons. Water Resour. Res. 51, 5772–5790. doi: 10.1002/2015WR017169
Kollet, S., Gasper, F., Brdar, S., Goergen, K., Hendricks-Franssen, H.-J., Keune, J., et al. (2018). Introduction of an experimental terrestrial forecasting/monitoring system at regional to continental scales based on the terrestrial systems modeling platform (v1. 1.0). Water 10, 1697. doi: 10.3390/w10111697
Kollet, S. J., Maxwell, R. M., Woodward, C. S., Smith, S., Vanderborght, J., Vereecken, H., et al. (2010). Proof of concept of regional scale hydrologic simulations at hydrologic resolution utilizing massively parallel computer resources. Water Resour. Res. 46, e2009WR008730. doi: 10.1029/2009WR008730
Kumar, S. V., Dong, J., Peters-Lidard, C. D., Mocko, D., and Gómez, B. (2017). Role of forcing uncertainty and background model error characterization in snow data assimilation. Hydrol. Earth Syst. Sci. 21, 2637–2647. doi: 10.5194/hess-21-2637-2017
Kumar, S. V., Jasinski, M., Mocko, D. M., Rodell, M., Borak, J., Li, B., et al. (2019). NCA-LDAS land analysis: development and performance of a multisensor, multivariate land data assimilation system for the national climate assessment. J. Hydrometeorol. 20, 1571–1593. doi: 10.1175/JHM-D-17-0125.1
Kumar, S. V., Zaitchik, B. F., Peters-Lidard, C. D., Rodell, M., Reichle, R., Li, B., et al. (2016). Assimilation of gridded GRACE terrestrial water storage estimates in the North American land data assimilation system. J. Hydrometeorol. 17, 1951–1972. doi: 10.1175/JHM-D-15-0157.1
Leone, M. (2022). Advances in fiber optic sensors for soil moisture monitoring: a review. Results Optics 7, 100213. doi: 10.1016/j.rio.2022.100213
Li, B., Rodell, M., Zaitchik, B. F., Reichle, R. H., Koster, R. D., and van Dam, T. M. (2012). Assimilation of GRACE terrestrial water storage into a land surface model: evaluation and potential value for drought monitoring in western and central Europe. J. Hydrol. 446, 103–115. doi: 10.1016/j.jhydrol.2012.04.035
Liu, Y., and Margulis, S. A. (2019). Deriving Bias and uncertainty in MERRA-2 snowfall precipitation over high mountain Asia. Front. Earth Sci. 7, 280. doi: 10.3389/feart.2019.00280
Lu, C.-Y., Hu, J.-C., Chan, Y.-C., Su, Y.-F., and Chang, C.-H. (2020). The relationship between surface displacement and groundwater level change and its hydrogeological implications in an alluvial fan: case study of the Choshui River, Taiwan. Remote Sens. 12, 3315. doi: 10.3390/rs12203315
Manoli, G., Rossi, M., Pasetto, D., Deiana, R., Ferraris, S., Cassiani, G., et al. (2015). An iterative particle filter approach for coupled hydro-geophysical inversion of a controlled infiltration experiment. J. Comput. Phys. 283, 37–51. doi: 10.1016/j.jcp.2014.11.035
Maxwell, R. M. (2013). A terrain-following grid transform and preconditioner for parallel, large-scale, integrated hydrologic modeling. Adv. Water Resour. 53, 109–117. doi: 10.1016/j.advwatres.2012.10.001
Maxwell, R. M., and Condon, L. E. (2016). Connections between groundwater flow and transpiration partitioning. Science 353, 377–380. doi: 10.1126/science.aaf7891
Maxwell, R. M., Putti, M., Meyerhoff, S., Delfs, J.-O., Ferguson, I. M., Ivanov, V., et al. (2014). Surface-subsurface model intercomparison: a first set of benchmark results to diagnose integrated hydrology and feedbacks. Water Resour. Res. 50, 1531–1549. doi: 10.1002/2013WR013725
Miralles, D. G., Holmes, T. R. H., De Jeu, R. A. M., Gash, J. H., Meesters, A., and Dolman, A. J. (2011). Global land-surface evaporation estimated from satellite-based observations. Hydrol. Earth Syst. Sci. 15, 453–469. doi: 10.5194/hess-15-453-2011
Naghibi, S. A., Khodaei, B., and Hashemi, H. (2022). An integrated InSAR-machine learning approach for ground deformation rate modeling in arid areas. J. Hydrol. 608, 127627. doi: 10.1016/j.jhydrol.2022.127627
Navari, M., Margulis, S. A., Tedesco, M., Fettweis, X., and Alexander, P. M. (2018). Improving greenland surface mass balance estimates through the assimilation of MODIS albedo: a case study along the K-transect. Geophys. Res. Lett. 45, 6549–6556. doi: 10.1029/2018GL078448
Ning, L., Carli, F. P., Ebtehaj, A. M., Foufoula-Georgiou, E., and Georgiou, T. T. (2014). Coping with model error in variational data assimilation using optimal mass transport. Water Resour. Res. 50, 5817–5830. doi: 10.1002/2013WR014966
Ojha, C., Werth, S., and Shirzaei, M. (2020). Recovery of aquifer-systems in Southwest US following 2012–2015 drought: evidence from InSAR, GRACE and groundwater level data. J. Hydrol. 587, 124943. doi: 10.1016/j.jhydrol.2020.124943
Or, D. (2020). The tyranny of small scales—on representing soil processes in global land surface models. Water Resour. Res. 56, e2019WR024846. doi: 10.1029/2019WR024846
Or, D., Lehmann, P., and Assouline, S. (2015). Natural length scales define the range of applicability of the R ichards equation for capillary flows. Water Resour. Res. 51, 7130–7144. doi: 10.1002/2015WR017034
Paniconi, C., Marrocu, M., Putti, M., and Verbunt, M. (2003). Newtonian nudging for a richards equation-based distributed hydrological model. Adv. Water Resour. 26, 161–178. doi: 10.1016/S0309-1708(02)00099-4
Paniconi, C., and Putti, M. (2015). Physically based modeling in catchment hydrology at 50: survey and outlook. Water Resour. Res. 51, 7090–7129. doi: 10.1002/2015WR017780
Pasetto, D., Camporese, M., and Putti, M. (2012). Ensemble Kalman filter versus particle filter for a physically-based coupled surface–subsurface model. Adv. Water Resour. 47, 1–13. doi: 10.1016/j.advwatres.2012.06.009
Patil, A., Fersch, B., Hendricks Franssen, H.-J., and Kunstmann, H. (2021). Assimilation of cosmogenic neutron counts for improved soil moisture prediction in a distributed land surface model. Front. Water 115, 729592. doi: 10.3389/frwa.2021.729592
Peng, X., Han, W., Ao, J., and Wang, Y. (2021). Assimilation of LAI derived from UAV multispectral data into the SAFY model to estimate maize yield. Remote Sens. 13, 1094. doi: 10.3390/rs13061094
Radmanesh, F., Golabi, M. R., Khodabakhshi, F., Farzi, S., and Zeinali, M. (2020). Modeling aquifer hydrograph: performance review of conceptual MODFLOW and simulator models. Arab. J. Geosci. 13, 1–9. doi: 10.1007/s12517-020-5230-2
Ramgraber, M., Weatherl, R., Blumensaat, F., and Schirmer, M. (2021). Non-Gaussian parameter inference for hydrogeological models using stein variational gradient descent. Water Resour. Res. 57, e2020WR029339. doi: 10.1029/2020WR029339
Rasmussen, J., Madsen, H., Jensen, K. H., and Refsgaard, J. C. (2015). Data assimilation in integrated hydrological modeling using ensemble Kalman filtering: evaluating the effect of ensemble size and localization on filter performance. Hydrol. Earth Syst. Sci. 19, 2999–3013. doi: 10.5194/hess-19-2999-2015
Rasmussen, J., Madsen, H., Jensen, K. H., and Refsgaard, J. C. (2016). Data assimilation in integrated hydrological modelling in the presence of observation bias. Hydrol. Earth Syst. Sci. 20, 2103–2118. doi: 10.5194/hess-20-2103-2016
Reichle, R. H., McLaughlin, D. B., and Entekhabi, D. (2002). Hydrologic data assimilation with the ensemble Kalman filter. Mthly. Weather Rev. 130, 103–114. doi: 10.1175/1520-0493(2002)130<0103:HDAWTE>2.0.CO;2
Ridler, M.-E., Zhang, D., Madsen, H., Kidmose, J., Refsgaard, J. C., and Jensen, K. H. (2018). Bias-aware data assimilation in integrated hydrological modelling. Hydrol. Res. 49, 989–1004. doi: 10.2166/nh.2017.117
Rummler, T., Wagner, A., Arnault, J., and Kunstmann, H. (2022). Lateral terrestrial water fluxes in the LSM of WRF-Hydro: benefits of a 2D groundwater representation. Hydrol. Process. 36, e14510. doi: 10.1002/hyp.14510
Samaniego, L., Kumar, R., and Attinger, S. (2010). Multiscale parameter regionalization of a grid-based hydrologic model at the mesoscale. Water Resour. Res. 46, e2008WR007327. doi: 10.1029/2008WR007327
Sayde, C., Buelga, J. B., Rodriguez-Sinobas, L., El Khoury, L., English, M., van de Giesen, N., et al. (2014). Mapping variability of soil water content and flux across 1–1000 m scales using the actively heated fiber optic method. Water Resour. Res. 50, 7302–7317. doi: 10.1002/2013WR014983
Sayde, C., Gregory, C., Gil-Rodriguez, M., Tufillaro, N., Tyler, S., van de Giesen, N., et al. (2010). Feasibility of soil moisture monitoring with heated fiber optics. Water Resour. Res. 46, e2009WR007846. doi: 10.1029/2009WR007846
Scanlon, B. R., Zhang, Z., Save, H., Sun, A. Y., Schmied, H. M., Van Beek, L. P., et al. (2018). Global models underestimate large decadal declining and rising water storage trends relative to GRACE satellite data. Proc. Nat. Acad. Sci. U.S.A. 115, E1080–E1089. doi: 10.1073/pnas.1704665115
Schattan, P., Baroni, G., Oswald, S. E., Schöber, J., Fey, C., Kormann, C., et al. (2017). Continuous monitoring of snowpack dynamics in alpine terrain by aboveground neutron sensing. Water Resour. Res. 53, 3615–3634. doi: 10.1002/2016WR020234
Shirzaei, M., Freymueller, J., Törnqvist, T. E., Galloway, D. L., Dura, T., and Minderhoud, P. S. (2020). Measuring, modelling and projecting coastal land subsidence. Nat. Rev. Earth Environ. 2, 40–58. doi: 10.1038/s43017-020-00115-x
Smith, R., and Knight, R. (2019). Modeling land subsidence using InSAR and airborne electromagnetic data. Water Resour. Res. 55, 2801–2819. doi: 10.1029/2018WR024185
Smith, R. G., and Majumdar, S. (2020). Groundwater storage loss associated with land subsidence in Western United States mapped using machine learning. Water Resourc. Res. 56, e2019WR026621. doi: 10.1029/2019WR026621
Smith, W. K., Fox, A. M., MacBean, N., Moore, D. J., and Parazoo, N. C. (2020). Constraining estimates of terrestrial carbon uptake: new opportunities using long-term satellite observations and data assimilation. New Phytol. 225, 105–112. doi: 10.1111/nph.16055
Steele-Dunne, S. C., Rutten, M. M., Krzeminska, D. M., Hausner, M., Tyler, S. W., Selker, J., et al. (2010). Feasibility of soil moisture estimation using passive distributed temperature sensing. Water Resour. Res. 46, e2009WR008272. doi: 10.1029/2009WR008272
Stordal, A. S., Karlsen, H. A., Nævdal, G., Skaug, H. J., and Vallès, B. (2011). Bridging the ensemble Kalman filter and particle filters: the adaptive Gaussian mixture filter. Comput. Geosci. 15, 293–305. doi: 10.1007/s10596-010-9207-1
Striegl, A. M., and Loheide, S. P. II. (2012). Heated distributed temperature sensing for field scale soil moisture monitoring. Groundwater 50, 340–347. doi: 10.1111/j.1745-6584.2012.00928.x
Sun, Q., Vihma, T., Jonassen, M. O., and Zhang, Z. (2020). Impact of assimilation of radiosonde and UAV observations from the Southern Ocean in the polar WRF model. Adv. Atmosph. Sci. 37, 441–454. doi: 10.1007/s00376-020-9213-8
Tang, Q., Schilling, O. S., Kurtz, W., Brunner, P., Vereecken, H., and Hendricks Franssen, H.-J. (2018). Simulating flood-induced riverbed transience using unmanned aerial vehicles, physically based hydrological modeling, and the ensemble kalman filter. Water Resour. Res. 54, 9342–9363. doi: 10.1029/2018WR023067
Tangdamrongsub, N., and Šprlák, M. (2021). The assessment of hydrologic-and flood-induced land deformation in data-sparse regions using GRACE/GRACE-FO data assimilation. Remote Sens. 13, 235. doi: 10.3390/rs13020235
Tran, H., Leonarduzzi, E., De la Fuente, L., Hull, R. B., Bansal, V., Chennault, C., et al. (2021). Development of a deep learning emulator for a distributed groundwater–surface water model: ParFlow-ML. Water 13, 3393. doi: 10.3390/w13233393
Tso, C.-H. M., Johnson, T. C., Song, X., Chen, X., Kuras, O., Wilkinson, P., et al. (2020). Integrated hydrogeophysical modelling and data assimilation for geoelectrical leak detection. J. Contam. Hydrol. 234, 103679. doi: 10.1016/j.jconhyd.2020.103679
Vidana Gamage, D. N., Biswas, A., Strachan, I. B., and Adamchuk, V. I. (2018). Soil water measurement using actively heated fiber optics at field scale. Sensors 18, 1116. doi: 10.3390/s18041116
Villani, C. (2009). Optimal Transport: Old and New, Vol. 338. Springer. Available online at: https://link.springer.com/book/10.1007/978-3-540-71050-9
Vittecoq, B., Reninger, P.-A., Lacquement, F., Martelet, G., and Violette, S. (2019). Hydrogeological conceptual model of andesitic watersheds revealed by high-resolution heliborne geophysics. Hydrol. Earth Syst. Sci. 23, 2321–2338. doi: 10.5194/hess-23-2321-2019
Wada, Y., Bierkens, M. F., Roo, A., de Dirmeyer, P. A., Famiglietti, J. S., Hanasaki, N., et al. (2017). Human–water interface in hydrological modelling: current status and future directions. Hydrol. Earth Syst. Sci. 21, 4169–4193. doi: 10.5194/hess-21-4169-2017
Wood, E. F., Roundy, J. K., Troy, T. J., Van Beek, L. P. H., Bierkens, M. F., Blyth, E., et al. (2011). Hyperresolution global land surface modeling: meeting a grand challenge for monitoring Earth's terrestrial water. Water Resour. Res. 47, e2010WR010090. doi: 10.1029/2010WR010090
Yu, X., Moraetis, D., Nikolaidis, N. P., Li, B., Duffy, C., and Liu, B. (2019). A coupled surface-subsurface hydrologic model to assess groundwater flood risk spatially and temporally. Environ. Model. Softw. 114, 129–139. doi: 10.1016/j.envsoft.2019.01.008
Zeng, Y., Xie, Z., Liu, S., Xie, J., Jia, B., Qin, P., et al. (2018). Global land surface modeling including lateral groundwater flow. J. Adv. Model. Earth Syst. 10, 1882–1900. doi: 10.1029/2018MS001304
Zhang, D., Madsen, H., Ridler, M. E., Kidmose, J., Jensen, K. H., and Refsgaard, J. C. (2016). Multivariate hydrological data assimilation of soil moisture and groundwater head. Hydrol. Earth Syst. Sci. 20, 4341–4357. doi: 10.5194/hess-20-4341-2016
Zhang, D., Madsen, H., Ridler, M. E., Refsgaard, J. C., and Jensen, K. H. (2015). Impact of uncertainty description on assimilating hydraulic head in the MIKE SHE distributed hydrological model. Adv. Water Resour. 86, 400–413. doi: 10.1016/j.advwatres.2015.07.018
Keywords: integrated surface-subsurface hydrological models, land surface models, remote sensing, data assimilation, groundwater, soil moisture
Citation: Camporese M and Girotto M (2022) Recent advances and opportunities in data assimilation for physics-based hydrological modeling. Front. Water 4:948832. doi: 10.3389/frwa.2022.948832
Received: 20 May 2022; Accepted: 21 July 2022;
Published: 11 August 2022.
Edited by:
Harrie-Jan Hendricks Franssen, Helmholtz Association of German Research Centres (HZ), GermanyReviewed by:
Alain Pascal Francés, Laboratório Nacional de Energia e Geologia, PortugalBenjamin Fersch, Karlsruhe Institute of Technology (KIT), Germany
Damiano Pasetto, Ca' Foscari University of Venice, Italy
Copyright © 2022 Camporese and Girotto. This is an open-access article distributed under the terms of the Creative Commons Attribution License (CC BY). The use, distribution or reproduction in other forums is permitted, provided the original author(s) and the copyright owner(s) are credited and that the original publication in this journal is cited, in accordance with accepted academic practice. No use, distribution or reproduction is permitted which does not comply with these terms.
*Correspondence: Matteo Camporese, bWF0dGVvLmNhbXBvcmVzZUB1bmlwZC5pdA==
†These authors have contributed equally to this work