- 1GenHotel—Laboratoire Européen de Recherche Pour La Polyarthrite Rhumatoïde, University Paris-Saclay, University Evry, Evry, France
- 2Sanofi R&D, AI and Deep Analytics—Omics Data Science, Chilly-Mazarin, France
- 3Lifeware Group, Inria Saclay, Palaiseau, France
Rheumatoid Arthritis (RA) is an autoimmune disease of unknown aetiology involving complex interactions between environmental and genetic factors. Its pathogenesis is suspected to arise from intricate interplays between signalling, gene regulation and metabolism, leading to synovial inflammation, bone erosion and cartilage destruction in the patients’ joints. In addition, the resident synoviocytes of macrophage and fibroblast types can interact with innate and adaptive immune cells and contribute to the disease’s debilitating symptoms. Therefore, a detailed, mechanistic mapping of the molecular pathways and cellular crosstalks is essential to understand the complex biological processes and different disease manifestations. In this regard, we present the RA-Atlas, an SBGN-standardized, interactive, manually curated representation of existing knowledge related to the onset and progression of RA. This state-of-the-art RA-Atlas includes an updated version of the global RA-map covering relevant metabolic pathways and cell-specific molecular interaction maps for CD4+ Th1 cells, fibroblasts, and M1 and M2 macrophages. The molecular interaction maps were built using information extracted from published literature and pathway databases and enriched using omic data. The RA-Atlas is freely accessible on the webserver MINERVA (https://ramap.uni.lu/minerva/), allowing easy navigation using semantic zoom, cell-specific or experimental data overlay, gene set enrichment analysis, pathway export or drug query.
1 Introduction
RA is an autoimmune, systemic disease affecting approximately 1% of the world population. It is the most common type of autoimmune arthritis and causes pain, swelling, and stiffness in the joints (McInnes and Schett, 2011; Smolen, Aletaha and McInnes, 2016). RA is considered a complex disease and is suspected to arise from several factors (Glocker et al., 2006), including genetic (Korczowska, 2014), environmental (Deane et al., 2017; Sigaux et al., 2019), epigenetic (Frank-Bertoncelj, Klein and Gay, 2017), but also infectious (Balandraud, Roudier and Roudier, 2004) or hormonal factors (Talsania and Scofield, 2017). RA’s pathogenesis is governed by complex interactions between components at different scales of the immune system, affecting signalling, metabolic and regulatory networks. Summarizing this scattered and fragmented knowledge in a formal and standardized representation can significantly facilitate understanding the underlying disease mechanisms.
In this direction, some efforts have already been made to assemble the available knowledge in molecular interaction maps. In 2010, a comprehensive RA-specific map (Wu et al., 2010) was published based on high throughput experiments (mRNA, miRNA), literature, publicly available datasets and the pathway database KEGG (Ogata et al., 1999) to establish the connections between different components of the map. A decade later, in 2020, a second effort to formalize RA knowledge was published using Wu et al., (2010) map as a basis, the RA-map (Singh et al., 2020). For this map, the researchers used the Systems Biology Graphical Notation (SBGN) standard and, more precisely, the Process Description (PD) language (Le Novère, 2015) to represent the molecular pathways implicated in the disease. The map was built as a global map using information from human-specific studies related to RA, focusing on small-scale experiments in various cell and tissue types (e.g., fibroblasts, macrophages, synovial tissue, and peripheral blood mononuclear cells (PBMC)). The RA-map was manually curated for each component and reaction, with defined cellular pathways and molecular signatures under expert validation. In addition to manual curation of the scientific literature, other sources such as the Ingenuity Pathway Analysis (IPA) (Krämer et al., 2014), DISNOR (Lo Surdo et al., 2018), or KEGG databases were used to obtain RA related information regarding molecular pathways and phenotypic signatures.
While the previously published RA-map offers a formalized representation of the major pathways implicated in the disease, it does not cover metabolic pathways. It has been shown experimentally that unbalanced metabolic pathways accumulate metabolic intermediates, driving immune response to aggravate chronic inflammation (Pucino et al., 2020). Targeting specific metabolic pathways has been seen to reduce inflammation in both in vitro and in vivo studies of arthritis (Fearon et al., 2019). Recent studies have also highlighted metabolic reprogramming as a possible mechanism involved in disease pathogenesis (Aghakhani, Zerrouk and Niarakis, 2020). Considering these advances, adding metabolic information to the RA-map becomes necessary. In this perspective, we identified four metabolic pathways to include in the RA-map for their involvement in the disease (Fearon et al., 2019), namely glycolysis, pentose phosphate pathway, citric acid cycle and oxidative phosphorylation.
Moreover, the RA-map, a global map constructed as a generic blueprint of a cell, contains information from multiple cellular types. In RA, immune cells such as T cells and B cells invade the synovial tissue and produce high amounts of cytokines such as tumour necrosis factor α (TNFα), interferon γ (IFNγ), interleukin (IL)-1β, IL-6, IL-17, and cause inflammation (Guo et al., 2018). Immune cells also interact with tissue-resident cells (e.g., fibroblasts and macrophages), leading to osteoclasts’ proliferation, bone erosion, and cartilage damage (Guo et al., 2018). In addition, dendritic cells produce vascular endothelial growth factors and promote extensive angiogenesis in RA (Bosisio et al., 2018). The representation of cell-specific molecular interactions helps understand the complex interplay between various cells and factors in the inflamed joint. In this direction, we selected three cell types of interest: fibroblast, macrophage (including the pro-inflammatory M1 and the anti-inflammatory M2), and CD4+ T helper cell 1 (Th1). These cell types are abundant in RA patients’ joints (Huber et al., 2006; Kinne et al., 2000; Li et al., 2017), and produce high amounts of cytokines (Schulze-Koops and Kalden, 2001; Bartok and Firestein, 2010), resist apoptosis and proliferate at high rates (Krämer et al., 2014; Yang, Chang and Wei, 2020). The cell type selection was also based on literature availability, including already existing cell-specific maps, specific signalling pathways, and gene expression datasets.
This paper introduces an attempt to create a multicellular atlas of the rheumatic joint to recapitulate existing knowledge related to the disease’s onset, progression, and pathogenesis. The RA-Atlas includes an updated version of the RA-map: the RA-map V2 (with metabolic pathways’ addition), a fibroblast-specific map, two maps for the M1 and M2 macrophages, and a CD4+ Th1 map (Figure 1). Our ambition is to expand the RA-Atlas in the future by including other relevant cell types like B cells, CD4+ regulatory T cells, dendritic cells, chondrocytes, osteoblasts, and osteoclasts.
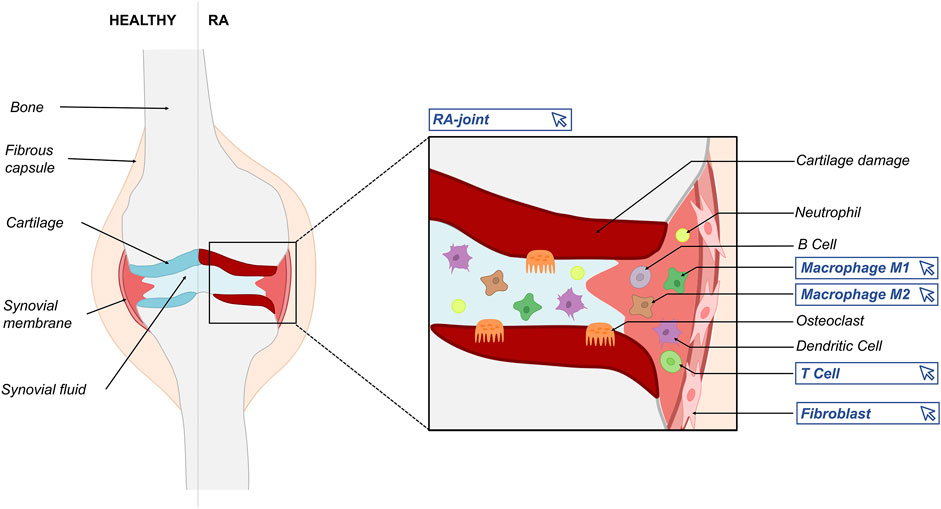
FIGURE 1. Schematic representation of the RA joint depicting the variety of actors implicated in RA’s pathogenesis. Blue frames indicate the different maps included in the RA-Atlas.
The maps can be used to provide high-quality curated information for disease-related pathways, a template for omic data analysis, and a starting point for dynamic computational models. The following sections outline our efforts to integrate low and high throughput experiments, combining prior knowledge, manual curation of scientific literature, omic data analysis, and systems biology standards to create state-of-the-art mechanistic representations of disease-related pathways.
2 Methodology and Data
2.1 A Common Strategy for Global and Cell-specific Maps
2.1.1 Map Construction
The original RA-map paper thoroughly describes the general methodology for constructing the different RA-Atlas maps (Singh et al., 2020). Briefly, we used the CellDesigner software (Funahashi et al., 2003) to construct the molecular interaction maps in the PD format, one of the three standard languages of SBGN (Le Novère, 2015). The various components of the maps (i.e., phenotype, protein, gene, RNA, simple molecule, ion, and complex) were distinguished in the form of specific glyphs and notations according to the said standards. Cellular compartments featured in each map differ according to their specificities. They are designed to reflect the molecular architecture of each map (e.g., extracellular space, plasma membrane, cytoplasm, nucleus, mitochondrion, endoplasmic reticulum, phenotypes, secreted compartment, and transmembrane domain). In addition, HUGO Gene Nomenclature Committee identifiers (HGNC) (Tweedie et al., 2021) were used for signalling and gene regulatory pathways components. The CellDesigner maps are available in Systems Biology Markup Language (SBML) format (Hucka et al., 2003). We provide a schematic representation of the major steps in Figure 2.
2.1.2 Curation Criteria
All the RA-Atlas maps underwent the first step of manual curation based on a broad study of literature and pathway databases to search for components and interactions involved in RA’s pathogenesis. We focused on small-scale experimental studies and added animal-based studies where the human-related information was not adequate to validate the role of components or interactions. However, the information based on animal studies remains limited and completely traceable (four molecules are involved in the Th1 map, four in macrophage maps and two in the fibroblast map). Including a component or a reaction in a map followed precise curation criteria: first, we included all components experimentally proven to be expressed in RA’s pathogenesis (and in a cell-specific manner for the cell-specific maps). Then, we included all reactions experimentally proven to occur in RA’s pathogenesis (and cell-specifically for the cell-specific maps). General pathway interactions were then added to complete specific pathways where disease-specific information was unavailable.
2.1.3 Annotations
Annotations were added to provide references for all species, reactions, and compartments present in the maps using MIRIAM (Minimal Information Requested In the Annotation of Models) (Novère et al., 2005), a standard for annotating and curating computational models and maps. MIRIAM annotations are added through the dedicated section of CellDesigner with the relation “bqbiol: is describedby”, which is used to link a component or a reaction to the literature or data that describes it (e.g., PubMed references (PMIDs), DOI, GEO, KEGG identifier). Annotated maps provide information about the various sources of information and help assess the maps’ specificity.
2.1.4 Visualization and Accessibility
All RA-Atlas maps presented in this work are available as online interactive maps on the standalone web server MINERVA (Molecular Interaction NEtwoRks VisuAlization) (Gawron et al., 2016). MINERVA platform allows for visual exploration, analysis and management of molecular networks encoded in systems biology formats, including CellDesigner, SBML and SBGN. MINERVA also provides automated content annotation and verification and overlaying experimental data. Our maps integrate data from various sources. We provide cell-specific overlays (cf. the overlay section) extracted from the literature and publicly available datasets to visualize cellular signatures on the different RA-Atlas maps.
2.2 RA-Map V2
2.2.1 Evaluation of the Prior RA-Map
The components and reactions present in the previous version of the RA-map were assessed, and bibliographical references were added wherever required to increase the overall confidence level of the map and limit false positives.
2.2.2 Metabolic Pathways Addition
Metabolic pathways of interest (i.e., glycolysis, pentose phosphate pathway, citric acid cycle and oxidative phosphorylation) were extracted from the PANTHER pathway database (Mi and Thomas, 2009), adapted to SBGN PD standards when required and added to the original RA-map. Metabolites and metabolic enzymes were named following BiGG IDs (King et al., 2016). In addition, considerable bibliographical work was conducted to find evidence linking the newly added metabolic pathways to the already present signalling and gene regulatory pathways.
2.2.3 Annotation Score
Thorough annotations throughout the manual curation process allowed us to calculate an annotation score for each compound present in the RA-map V2 based on the number of bibliographic references describing it.
2.3 Cell-specific Maps
To build cell-specific maps for M1 and M2 macrophages, fibroblasts, and Th1, we screened literature (small scale experiments, GWAS) for signalling pathways, genes or transcription factors associated with RA. If available, we also integrated pre-existing maps. We used cell/disease-specific markers or gene expression datasets to retrieve signalling pathways involved in RA pathogenesis. To define gene signatures and primary RA cellular outcomes, we used databases such as the MSig database (Liberzon et al., 2015), KEGG and biocuration. The retrieved gene signatures were used to connect secreted molecules to the cellular phenotypes included in the map. The cellular phenotypes include angiogenesis, apoptosis, bone erosion, cell chemotaxis, recruitment and infiltration, cell growth, survival and proliferation, hypoxia, inflammation, matrix degradation and osteoclastogenesis.
Two proprietary software were used to enrich the maps: IPA and MetaCore (Clarivate, 2022) version 22.1 build 70800, both displaying vast map databases. In MetaCore, we mapped the list of DEG to the internal map database using the “Pathway maps” option through the “One-click analysis” tab. The number of overlapping DEGs is displayed next to each identified map. The same analysis was done using IPA’s “canonical pathways” analysis. Highlighted DEG and their associated pathways were identified, added to the corresponding RA-Atlas map and further annotated with PMIDs or GEO accession numbers.
2.3.1 Datasets
Regarding the datasets, for the RA macrophage map, we used GSE97779, a publicly available microarray dataset from the GEO database (Edgar, 2002). The dataset contains nine RA synovial macrophages sample from nine patients and five peripheral blood monocyte-derived macrophages samples from five healthy donors. Gene expression was quantile normalized using the preprocessCore package (Bostald, 2021). GSE164498, a RNA-seq single-cell dataset from the GEO database, was used to provide M1 and M2 macrophage signatures. It contains 1766 M1 macrophage cells and 2063 M2 macrophage cells. M1 macrophages were polarized using LPS, and IFN-Gamma and M2 macrophage cells were polarized using IL-4 and IL-13. We used GSE109449, a RNA-seq single cell dataset available in the GEO database for the RA fibroblast map. It contains 384 freshly isolated fibroblasts in two RA and two osteoarthritis patients. For the RA Th1 map, we used SDY998, a single-cell RNA-seq dataset from the Immport database (Bhattacharya et al., 2018) containing 19 samples from RA patients and two synovial samples from osteoarthritis patients with three cell types: 1142 B cells, 1844 fibroblasts, 750 monocytes, and 1529 T cells. The gene expression was normalized using log2 (CPM). For the GSE analysis, we used four datasets: SDY998, GSE32901, GSE107011 and GSE135390. The first dataset is the same one used for DEA. The second is a microarray gene expression dataset containing PBMC samples from 5 healthy donors. It includes four CD4+ T cells subtypes: naive CD4+ T cells, Th1, Th17 rich, and Th17 poor memory CD4+ T cells. Gene expression was normalized using the log2 RMA method. The third dataset is bulk RNA-seq transcriptome profiling of 29 immune cell types, including Th1 and extracted from PBMC sorted from 4 healthy individuals. The counts were normalized using the Transcripts Per Million (TPM) method. The last dataset is RNA-seq gene expression profiling. It contains PBMC samples coming from 3 healthy donors. Several subtypes of CD4+ T cells are in the samples: Th1, TH2, TH22, Treg, naive CD4+ and Th17. Count data were normalized using TMM. In addition, we used GSE172188, a microarray gene expression dataset of synovial samples from ten patients with active RA treated with abatacept. For each patient, synovial biopsies were obtained from the same affected joint before (W0) and 16 weeks after (W16) starting treatment with abatacept.
2.3.2 Macrophage Maps
2.3.2.1 Macrophage Map Integration
The macrophage map was constructed as a PD graph on CellDesigner by integrating three maps as a base. The initial map was the RA-specific macrophage map from IPA including 44 molecules and 47 interactions associated with PMIDs. The second and third macrophage-specific maps (but not RA specific) were retrieved from the published literature (Oda et al., 2004; Wentker et al., 2017).
As the second and third macrophage maps were not RA-specific, we used the RA-specific marker gene lists from IPA as an overlay in MINERVA to identify disease-specific molecules and submodules. The Stream export plugin (Hoksza et al., 2019) in MINERVA allowed us to export these submodules of interest from the macrophages maps. We identified corresponding signalling pathways in the extracted submodules and completed them by adding molecules from the literature and pathway databases like KEGG.
2.3.2.2 RA Macrophage Map Splitting Into M1 and M2 Macrophage Maps
We split the RA macrophage map into two maps according to the M1 or M2 phenotype. We included Msig gene signatures for M1 and M2 macrophages and information available in the literature for each signalling pathway in the map. However, it was impossible to assign a phenotype for some pathways due to both phenotypes’ lack of information or expression. These pathways were kept in both M1 and M2 maps.
2.3.2.3 M1 and M2 Macrophages Map Enrichment
Differential Expression Analysis (DEA) was performed using the preprocessCore and Limma packages (Ritchie et al., 2015) in R on the GSE97779 dataset (cf. dataset section). The list of DEG was used for enrichment with IPA and MetaCore. To assign the newly identified pathways to the M1 or the M2 phenotype, DEA was performed on GSE164498 (cf. dataset section) using Bioturing software (Le et al., 2020) with the Venice method (Vuong et al., 2020) and M1 versus M2 macrophage cells to identify signature genes for both M1 and M2 phenotypes. The percentage of shared genes between each newly identified signalling pathway and M1 and M2 signatures was calculated. Based on these criteria, new pathways were assigned to the M1, M2 maps, or both.
2.3.3 Fibroblast Map
To build the RA fibroblast-specific map, we filtered non-fibroblast-specific components from the initial RA-map using the up-to-date RA fibroblast overlay available in MINERVA (cf. overlay section). Non-fibroblast-specific components were only kept if necessary to transduce the signal. We used the BioTuring software and the Venice method to perform DEA on GSE109449 (cf. dataset section). The filtered DEG list was used as an overlay in MINERVA to retrieve more RA fibroblast-specific components from the RA global map.
2.3.3.1 Fibroblast Map Enrichment
The same DEG list was used to enrich the fibroblast map with new signalling pathways using IPA and MetaCore software.
2.3.4 Th1 Map
Regarding the Th1 map, we did not integrate any pre-existing cell-specific map. Alternatively, we first searched the literature for the main signalling pathways expressed in the Th1 subtype and involved in RA’s pathogenesis (using queries like “Th1” and “rheumatoid arthritis”). In addition, we used the multicellular map of inflammation (Serhan et al., 2020) and its not-RA-specific Th1 submap to identify transcription factors and cell-specific receptors involved in RA pathogenesis. We integrated the SDY998 gene expression dataset (cf. dataset section) to enrich the map with new pathways. Clustering was performed on the T cells using the Seurat package (Hao et al., 2021) in R (Find_neighbors and Find_clusters functions). Based on the expression of the marker genes CXCR3, CCR7 and CCR6, the Th1 cluster was identified. We performed DEA using CXCR3+ CCR7- CCR6- Th1 cluster versus CCR7+ naive cells from RA samples using Seurat and Find_marker_genes function. The filtered DEG list was used to identify new pathways using IPA and MetaCore tools described above. To expand the Th1 map, we also performed Gene Set Enrichment Analysis (GSEA) using GSEA software (Subramanian et al., 2005), IPA gene sets and the SDY998 gene expression dataset. The list of enriched gene sets was filtered with a False Discovery Rate (FDR) less or equal to 5% to keep only the statistically significant ones. To ensure that the results are Th1 specific, we performed GSEA on three other datasets: GSE32901, GSE107011 and GSE135390 (cf. dataset section), and filtered the enriched gene sets with FDR less or equal to 5%. Only gene sets identified with the SDY998 dataset shared at least by one of the GSE32901, GSE107011 or GSE135390 datasets were kept. Only gene sets sharing less than 80% of their core enrichment genes were kept to avoid redundancy.
2.4 Overlays
We provide nine different sample-specific overlays, namely fibroblasts, synovial fluid, synovial tissue, PBMC, blood components, serum, chondrocytes, macrophages, and Th1 to calculate the cell-specificity of the RA-Atlas maps or visualize the cell-specific pathways in MINERVA.
Most overlays (i.e. synovial fluid, synovial tissue, PBMC, blood components, and chondrocytes) are updated versions of the overlays provided in the initial RA-map with the addition of new bibliographical references. The fibroblast overlay is also an updated version of the overlay provided in the initial paper with the addition of bibliographical references and the list of DEG obtained from DEA (see RA fibroblast map enrichment section). The overlay file now consists of 2,409 fibroblast-specific components. The macrophage overlay includes the RA macrophage-specific components identified in the literature, the DEG list obtained from DEA (cf. M1/M2 macrophages map enrichment with gene expression datasets section), and the 44 molecules present in the IPA RA-specific macrophage map (cf. Macrophage maps integration). It consists of 648 RA macrophage-specific molecules. The Th1 overlay consists of 523 molecules, including the list of DEG obtained previously, the core enrichment genes from the GSEA (cf. Th1 map section), and information found in the literature. Finally, the serum overlay was created to account for a new source of information used in the maps.
3 Results
3.1 RA-Map V2
The RA-map V2 illustrates signalling pathways, gene regulation, metabolic pathways, molecular mechanisms and phenotypes involved in RA’s pathogenesis. The map is compartmentalized in a way to represent the flow of information from the extracellular space (ligands) to the plasma membrane (ligands-receptors or metabolic transporters complexes) through the cytoplasm (signalling and metabolism), the nucleus (gene regulation) and the secreted compartments (phenotype activation).
The map is fully compliant with SBGN PD standards. It includes 720 species (329 proteins, 135 genes, 136 RNAs, 54 simple molecules, 1 ion and 65 molecular complexes), 9 phenotypes (angiogenesis, apoptosis, bone erosion, cell chemotaxis/recruitment/infiltration, cell growth/survival/proliferation, hypoxia, inflammation, matrix degradation and osteoclastogenesis) and 602 reactions. This new version of the map represents an update regarding the number of components. We have added 158 species, primarily involved in metabolic pathways, 156 reactions, primary interconnecting metabolic pathways with signalling and gene regulation pathways, and the hypoxic phenotype. We have included 225 additional references that make a total of 575 PMIDs for the whole map. The high number of references enhances confidence in the presented mechanisms. Indeed, 87% of the components included are supported with more than one experimental evidence and 65% more than 2 (Figure 3).
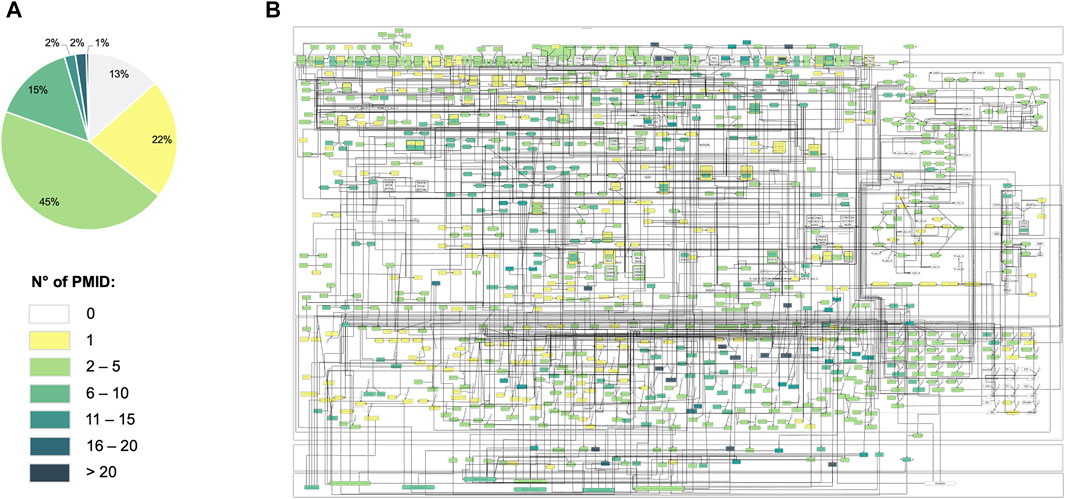
FIGURE 3. Annotation score of the RA-map V2. (A) Pie-chart of the annotation score’s distribution among the map’s components. (B) Overlay of the annotation scores on the RA-map V2. Each component is colored according to its annotation score.
In several cases, the map includes compounds with no bibliographic reference. For example, many simple molecules act as products or reactants of well-known biological reactions whose expressions are rarely highlighted in disease-specific experimental studies (e.g., ATP, ADP, NADH, NADPH, H2O, O2, CoA, and FADH). Also, molecules that act as pathway intermediates do not present bibliographical references. In this case, we have evidence that a pathway is expressed in a disease-specific manner, but not all intermediates have been studied experimentally. For instance, specific metabolic pathways are found to be expressed, however, experimental evidence is not available for every component.
Beyond the considerable effort of the initial RA-map to include the disease hallmarks (e.g., cytokines, chemokines, growth factors, toll-like receptors) and molecular pathways (e.g., JAK-STAT pathway, NF-KB pathway, and MAPK pathway), we aimed to expand the coverage in disease-specific metabolic dysregulated pathways. In particular, we focused on adding glycolysis, citric acid cycle, pentose phosphate pathway, and oxidative phosphorylation pathways as their interplay with inflammation and immunity mechanisms, among others, have been proven in RA (Weyand and Goronzy, 2017; Pucino et al., 2020). In addition, non-metabolic functions of metabolites and metabolic enzymes were illustrated, such as their transcription regulation function (e.g., Hexokinase 2 or Phosphoglycerate Kinase 1 (Kim and Dang, 2005)) or their involvement in disease-specific signalling pathways or phenotypes (e.g., Lactate (Yi et al., 2022), Glucose-6-Phosphate Isomerase (Zong et al., 2015) or Pyruvate (Chang and Wei, 2011)).
The RA-map V2 integrates information from several sources and cell types (Figure 4). The user can take advantage of its extensive annotation and assess the map’s coverage by opting for a specific representation of a particular cell type in MINERVA. The map is mainly composed of fibroblast specific information (73%) but other cell types, tissues and fluids involved in RA’s pathogenesis (Fang, Zhou and Nandakumar, 2020) are also represented, such as synovial tissue, synovial fluid, blood components, serum components, PBMC, chondrocytes and macrophages. Therefore, when interpreting those results, one must consider that a specific component can be common to several cell types or that this component is present only in one cell type.
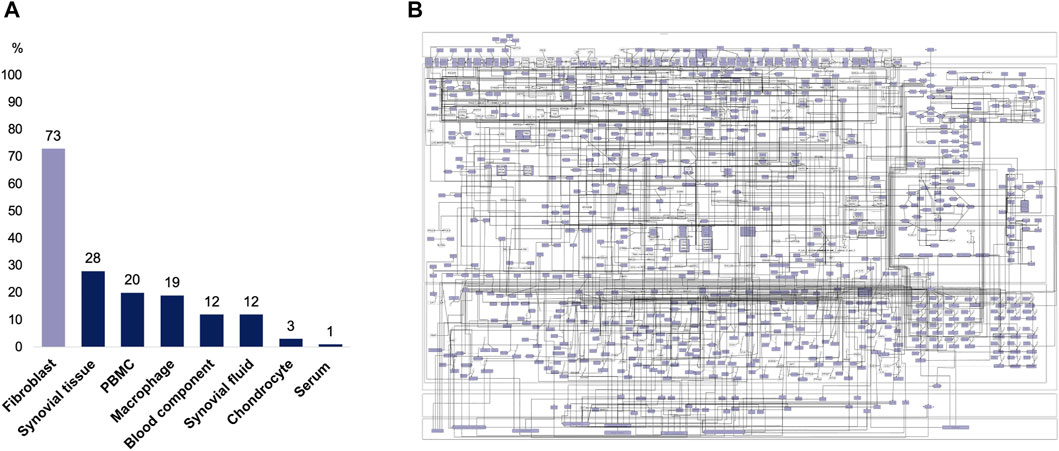
FIGURE 4. Cell-specificity of the RA-map V2 components. (A) Distribution of the RA-map V2 cell-specificity. (B) Visualization of the fibroblast-specific overlay on the RA-map V2.
3.2 RA Fibroblast Map
The RA fibroblast map results from an extensive effort to make the RA-map fibroblast-specific. It is based on manual curation of the RA-map, using literature and gene expression integration. Using the list of DEG, 23 components were identified as fibroblast-specific from the RA-map V2. In addition, new pathways were identified using IPA and MetaCore. The top 5 identified signalling pathways are shown in Table 1.
The enriched fibroblast-specific map comprises ten compartments from the extracellular space where the biological signal is induced to the secreted components’ and transmembrane proteins’ compartments. As the RA-map V2, it is SBGN PD compliant. It contains 853 species, including 411 proteins, 115 genes, 115 RNAs, 96 molecular complexes, 2 simple molecules and 9 phenotypes (8 of them coming from the initial RA-map, the 9th one being T cells activation). The components interact together via 509 reactions.
77% of the map’s components are RA fibroblast-specific (Figure 5). Some of the molecules that are not fibroblast-specific were added to the map to keep the signal transduction going from the extracellular part to the nucleus. Indeed, in some RA fibroblast-specific pathways, these molecules are needed to activate or inhibit intermediate proteins. Other molecules that are not RA fibroblast-specific are found in the extracellular space. These molecules can come from the synovial fluid and be secreted by other cell types to activate specific signalling pathways in the RA fibroblast. In this case, the secreted molecules do not have to be RA fibroblast-specific. Supporting literature was not always available for small molecules or adaptor proteins.
3.3 RA Macrophage Maps
We obtained the global macrophage map by converting the IPA cell and disease-specific map into an SBGN PD compliant map in CellDesigner. New pathways were added from the RA-specific submodules exported from the previously published maps (Oda et al., 2004; Wentker et al., 2017) like TLRs pathway, NFKB pathway, PI3K-AKT pathway, MAPK signalling pathway, and IL-18. Other pathways were identified from the literature, such as the IFN pathway, Notch pathway, IL10 and IL23 pathways.
KEGG, MSig database and literature screening allowed to add 8 phenotypes to the map. The phenotypes include inflammation, apoptosis, angiogenesis, extracellular matrix degradation, cell chemotaxis/migration, T cells activation, proliferation/survival and osteoclastogenesis.
3.3.1 RA Macrophage M1 Map
The RA macrophage M1-specific map includes all the signalling pathways from the RA macrophage map leading to the macrophage polarization into the pro-inflammatory M1 phenotype. It was enriched using gene expression dataset integration to identify new RA M1 macrophage-specific pathways using IPA and MetaCore. The top 5 identified signalling pathways are shown in Table 2.
The RA macrophage M1-specific map includes 640 species: 278 proteins, 76 genes, 76 RNAs, 114 molecular complexes, 8 simple molecules and the same phenotypes as the RA macrophage map. These components interact together via 448 reactions.
61% of the components of this map are RA macrophage-specific (Figure 5). In addition, all the receptors and secreted components are RA macrophage-specific. Although less literature is available compared to the RA Fibroblast map, gene expression datasets integration enabled us to keep a high RA macrophage specificity in the map.
3.3.2 RA Macrophage M2 Map
The M2 macrophage map includes all the signalling pathways from the RA macrophage map leading to the macrophage polarization into the anti-inflammatory M2 phenotype. After the enrichment step with IPA and MetaCore, additional pathways were added. The top 5 signalling pathways are shown in Table 2.
The enriched map includes 520 species: 243 proteins, 59 genes, 59 RNAs, 90 molecular complexes, three simple molecules and 7 of the 8 phenotypes from the RA macrophage map. Indeed, osteoclastogenesis is not associated with any of the secreted molecules in the RA M2 macrophage map. The Macrophage M2 map’s components interact together via 342 reactions.
55% of the components of this map are RA macrophage-specific (Figure 5). All the receptors and secreted components are RA macrophage-specific. We can see that fewer pathways are specific to the M2 phenotype in RA because RA disease is mainly associated with the M1 phenotype.
3.4 RA Th1 Map
We built the RA Th1 cell map through literature mining to identify the main cell-specific signalling pathways involved in RA’s pathogenesis. However, less information about the Th1 subtype was available in the literature than fibroblasts and macrophages. Hence, it was essential to expand the map using new methods. New signalling pathways were identified using IPA and MetaCore and the enriched gene sets from GSEA (Tables 3 and 4).
The map consists of 321 species, including 167 proteins, 29 genes, 29 RNAs, 64 molecular complexes, and 7 phenotypes. The phenotypes include inflammation, apoptosis, osteoclastogenesis, cell chemotaxis, angiogenesis, matrix degradation and proliferation. RA Th1 map’s components are connected through 179 reactions.
58% of the components of this map are RA Th1-specific, including all the transcription factors, most of the receptors, and secreted components (Figure 5).
3.5 Applications
Several options are available in MINERVA for the user to dive into the different RA-Atlas maps. Users can explore the maps using the search bar and get the locations of their compounds of interest and their associated references (Figure 6A) or visualize experimental data and cell-specific components using the appropriate overlays (Figure 6B). Users can exploit online queries for drug targets via DrugBank (Wishart et al., 2008) and CHEMBL (Gaulton et al., 2017).
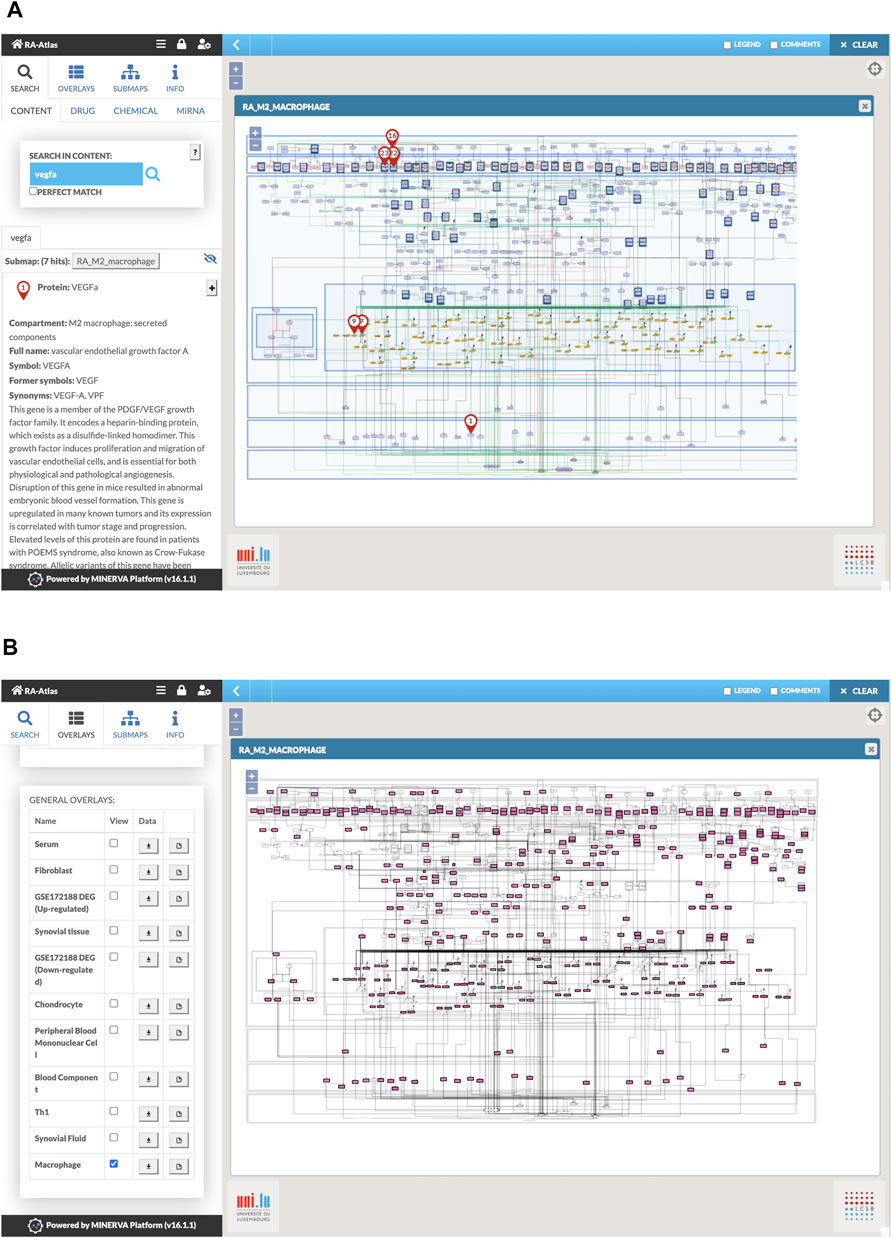
FIGURE 6. Visualization of RA macrophage M2 map in MINERVA. (A) Snapshot of the search for VEGFa. (B) Snapshot of the visualization of the macrophage overlay.
Users can also use plugins available in MINERVA, such as the Stream export, to export parts of the network upstream and downstream of a node. GSEA plugin can be used to calculate enrichment for uploaded user-provided data overlays. We performed GSEA by using a list of DEG from the GSE172188 dataset (cf. dataset section) between the paired post- and pre-treatment samples as an overlay to identify enriched signalling pathways from the fibroblast map in RA patients after treatment with abatacept compared to untreated RA patients. The list of DEG was downloaded from the supplementary materials of the associated publication (Triaille et al., 2021). Results of the GSE analysis for the upregulated genes are shown in Figure 7.
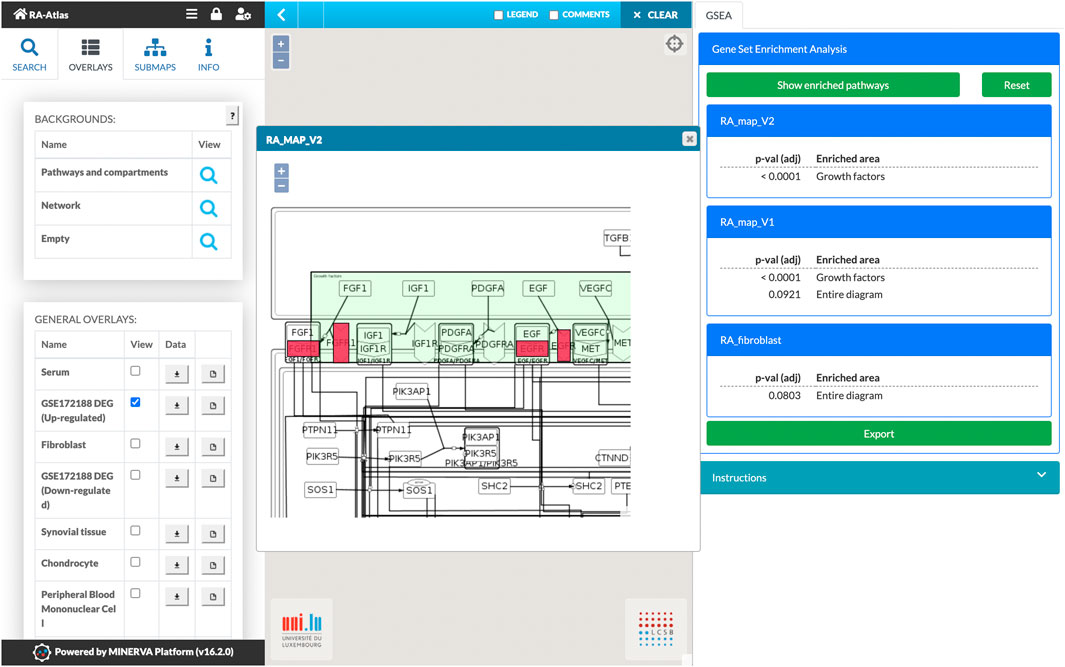
FIGURE 7. Gene set enrichment analysis on the RA-Atlas using the GSEA plugin and the dataset GSE172188. The dataset includes gene expression data of synovial tissue from 10 patients with active RA treated with abatacept. Enriched pathways are presented in the right panel along with their adjusted p-values. Results can also be visualized in the corresponding maps to illustrate the enriched components. Here, we show a zoom of the RA-map V2 with up-regulated genes highlighted in red color.
4 Discussion
Knowledge assembly in the form of networks is an active field in Systems Biology. Many public repositories such as KEGG (Ogata et al., 1999), Reactome (Gillespie et al., 2022), WikiPathways (Martens et al., 2021), Panther (Mi and Thomas, 2009), and proprietary platforms such as IPA (Krämer et al., 2014) and MetaCore (Clarivate, 2022) offer mechanistic insights into a variety of biological processes. However, most of the diagrams available can be generic, lack cell specificity, and without proper annotations and access to the literature used for their assembly. A key aspect of current research is to move beyond generic characterizations of biological processes toward more specific pathways considering cell, tissue, organ, and disease states.
International projects such as the Human Cell Atlas (Regev et al., 2017) and the HapMap (Tanaka, 2003) have significantly contributed to our understanding of the role of different cells in the human body and the specificities linked to the anatomical or functional localization of these cells. Moreover, advancements in single-cell techniques offer insights into unprecedented specificity for various cell types and subpopulations in normal and pathological conditions.
RA is a disease involving several cells and their crosstalks. Unfortunately, there is no cure, and the proposed therapies can only alleviate symptoms and increase survival. Hence, understanding the cellular interplay and intracellular cascades and their regulation could be advantageous in identifying novel therapeutic targets that could help relieve the heavy burden of the disease’s debilitating symptoms.
With this aim, we built the RA-Atlas, an interactive, manually curated and enriched with extensive omic data analysis representation of molecular mechanisms involved in RA’s pathogenesis. It includes an updated version of the global RA-map (Singh et al., 2020) with the addition of metabolic pathways, and cell-specific molecular interaction maps for resident fibroblasts, M1 and M2 resident macrophages and CD4+ Th1 cells. The diagrams depicting the biological mechanisms are formalized using the SBGN PD language (Le Novère, 2015). They are primarily based on high-quality manual curation of the scientific literature, enrichment, and cross-validation using expression data with either bulk or single-cell resolution. Furthermore, our group is an active player in the Disease Map consortium. This initiative aims at fostering collaborations for the creation of disease-specific maps such as The Parkinson’s map (Fujita et al., 2014), the AsthmaMap (Mazein and Knowles, 2018), the Atlas of Cancer Signalling Network (Kuperstein et al., 2015), The Atlas of Inflammation Resolution (Serhan et al., 2020), the Cystic Fibrosis Map: CyFi-MAP (Pereira et al., 2021), and more recently, the COVID19 Disease Map (Ostaszewski et al., 2021). In addition, the community works on developing best practices for annotation, curation, the use of systems biology standards, and the development of platforms and technology for handling complexity (Ostaszewski et al., 2019).
5 Adopting FAIR Principles
The RA-Atlas is a project that tries to implement the FAIR principles (Wilkinson et al., 2016) for findability, accessibility, interoperability and reproducibility. The Atlas being a part of the Disease Map community project (Mazein and Ostaszewski, 2018; Ostaszewski et al., 2019), it makes its dissemination among the community easier. The RA-Atlas is also freely accessible via a web browser and the platform MINERVA (Gawron et al., 2016). Its content is compliant with SBGN PD (Le Novère, 2015) for the representation, SBML (Hucka et al., 2003) for the construction, MIRIAM for the annotations (Novère et al., 2005), PMIDs and stable identifiers for the references. All entities are annotated using HGNC symbols (Tweedie et al., 2021) for signaling and gene regulation componenets and BiGG IDs (King et al., 2016) for metabolic compounds. Lastly, the extensive annotations and the access to content allow for transparent and facilitated reuse of the resource.
6 Perspectives
The long-term objective of this project is to construct a computational repository to decipher the interplay between cells of the innate and adaptive immunity in RA, which eventually leads to bone and cartilage breakdown. We wish to expand the RA-Atlas with molecular interaction maps of B cells, chondrocytes, osteoblasts, and osteoclasts and enrich existing cell-specific maps with relevant metabolic pathways. We will also create a top-level view focusing on mapping how the different cells interact to cope with complexity. Furthermore, intercellular interactions must be clearly defined, mapped, and described to provide a comprehensive view of the cellular interplay in RA. Lastly, our maps can serve as a basis for constructing executable disease networks (Singh et al., 2018; Aghamiri et al., 2020; Hall and Niarakis, 2021; Miagoux et al., 2021; Niarakis and Helikar, 2021), allowing for in-silico simulations, hypotheses formation and predictions.
Data Availability Statement
The RA-Atlas is freely accessible at https://ramap.uni.lu/minerva/. All PMIDs of scientific articles used for the map construction are available in the annotation section of the maps. All datasets used for the map construction are publicly available (cf. dataset section). All data and code used to generate results, including XML files of the maps, cell-specificity and confidence score calculations, overlays, and gene expression analysis are available on a GitLab repository at https://gitlab.com/genhotel/a-mechanistic-multicellular-atlas-of-the-rheumatic-joint (accessed on June 8, 2022).
Author Contributions
Conceptualization, AN; methodology, AN and FA; formal analysis, NZ, SA, FA, and AN; data curation, NZ, SA, and VS; writing—original draft preparation, NZ, SA, VS, and AN; writing—review and editing, NZ, SA, VS, FA, and AN; visualization, NZ and SA; supervision, FA, and AN; project administration, FA, and AN; All authors have read and agreed to the published version of the manuscript.
Funding
The work was supported by the public-private partnership grant (CIFRE contract, no 2020/0766), the doctorate program of the University of Paris Saclay, France, and Genopole.
Conflict of Interest
FA is employed by SANOFI-AVENTIS R&D, AN collaborates with SANOFI-AVENTIS R&D via a public-private partnership grant (CIFRE contract, n° 2020/0766), and NZ is the recipient of the CIFRE doctoral contract.
The remaining authors declare that the research was conducted in the absence of any commercial or financial relationships that could be construed as a potential conflict of interest.
Publisher’s Note
All claims expressed in this article are solely those of the authors and do not necessarily represent those of their affiliated organizations, or those of the publisher, the editors and the reviewers. Any product that may be evaluated in this article, or claim that may be made by its manufacturer, is not guaranteed or endorsed by the publisher.
Acknowledgments
The authors would like to thank the Luxembourg Elixir hub, Dr Marek Ostaszewski, Dr Piotr Gauron of the LCSB for their technical support, and Dr Zsolt Bocskei for helpful discussions.
References
Aghakhani, S., Zerrouk, N., and Niarakis, A. (2020). Metabolic Reprogramming of Fibroblasts as Therapeutic Target in Rheumatoid Arthritis and Cancer: Deciphering Key Mechanisms Using Computational Systems Biology Approaches. Cancers 13 (1), 35. doi:10.3390/cancers13010035
Aghamiri, S. S., Singh, V., Naldi, A., Helikar, T., Soliman, S., and Niarakis, A. (2020). Automated Inference of Boolean Models from Molecular Interaction Maps Using CaSQ. Bioinformatics 36 (16), 4473–4482. doi:10.1093/bioinformatics/btaa484
Balandraud, N., Roudier, J., and Roudier, C. (2004). Epstein-Barr Virus and Rheumatoid Arthritis. Autoimmun. Rev. 3 (5), 362–367. doi:10.1016/j.autrev.2004.02.002
Bartok, B., and Firestein, G. S. (2010). Fibroblast-Like Synoviocytes: Key Effector Cells in Rheumatoid Arthritis. Immunol. Rev. 233 (1), 233–255. doi:10.1111/j.0105-2896.2009.00859.x
Bhattacharya, S., Dunn, P., Thomas, C. G., Smith, B., Schaefer, H., Chen, J., et al. (2018). ImmPort, Toward Repurposing of Open Access Immunological Assay Data for Translational and Clinical Research. Sci. Data 5, 1–9. doi:10.1038/sdata.2018.15
Bosisio, D., Ronca, R., Salvi, V., Presta, M., and Sozzani, S. (2018). Dendritic Cells in Inflammatory Angiogenesis and Lymphangiogenesis. Curr. Opin. Immunol. 53, 180–186. doi:10.1016/j.coi.2018.05.011
Bostald, B. (2021). preprocessCore: A Collection of Pre-Processing Functions. Available at: https://github.com/bmbolstad/preprocessCore (Accessed April 12, 2022).
Chang, X., and Wei, C. (2011). Glycolysis and Rheumatoid Arthritis. Int. J. Rheumatic Dis. 14 (3), 217–222. doi:10.1111/j.1756-185X.2011.01598.x
Clarivate (2022). MetaBase & MetaCore, a Cortellis Solution. Available at: https://clarivate.com/products/biopharma/discovery-clinical-regulatory/early-research-intelligence-solutions/ (Accessed April 12, 2022).
Deane, K. D., Demoruelle, M. K., Kelmenson, L. B., Kuhn, K. A., Norris, J. M., and Holers, V. M. (2017). Genetic and Environmental Risk Factors for Rheumatoid Arthritis. Best Pract. Res. Clin. Rheumatology 31 (1), 3–18. doi:10.1016/j.berh.2017.08.003
Edgar, R. (2002). Gene Expression Omnibus: NCBI Gene Expression and Hybridization Array Data Repository. Nucleic Acids Res. 30 (1), 207–210. doi:10.1093/nar/30.1.207
Fang, Q., Zhou, C., and Nandakumar, K. S. (2020). Molecular and Cellular Pathways Contributing to Joint Damage in Rheumatoid Arthritis. Mediat. Inflamm. 2020, 1–20. doi:10.1155/2020/3830212
Fearon, U., Hanlon, M. M., Wade, S. M., and Fletcher, J. M. (2019). Altered Metabolic Pathways Regulate Synovial Inflammation in Rheumatoid Arthritis. Clin. Exp. Immunol. 197 (2), 170–180. doi:10.1111/cei.13228
Frank-Bertoncelj, M., Klein, K., and Gay, S. (2017). Interplay Between Genetic and Epigenetic Mechanisms in Rheumatoid Arthritis. Epigenomics 9 (4), 493–504. doi:10.2217/epi-2016-0142
Fujita, K. A., Ostaszewski, M., Matsuoka, Y., Ghosh, S., Glaab, E., Trefois, C., et al. (2014). Integrating Pathways of Parkinson's Disease in a Molecular Interaction Map. Mol. Neurobiol. 49 (1), 88–102. doi:10.1007/s12035-013-8489-4
Funahashi, A., Morohashi, M., Kitano, H., and Tanimura, N. (2003). CellDesigner: a Process Diagram Editor for Gene-Regulatory and Biochemical Networks. BIOSILICO 1 (5), 159–162. doi:10.1016/S1478-5382(03)02370-9
Gaulton, A., Hersey, A., Nowotka, M., Bento, A. P., Chambers, J., Mendez, D., et al. (2017). The ChEMBL Database in 2017. Nucleic Acids Res. 45 (D1), D945–D954. doi:10.1093/nar/gkw1074
Gawron, P., Ostaszewski, M., Satagopam, V., Gebel, S., Mazein, A., Kuzma, M., et al. (2016). MINERVA-a Platform for Visualization and Curation of Molecular Interaction Networks. npj Syst. Biol. Appl. 2 (1), 16020. doi:10.1038/npjsba.2016.20
Gillespie, M., Jassal, B., Stephan, R., Milacic, M., Rothfels, K., Senff-Ribeiro, A., et al. (2022). The Reactome Pathway Knowledgebase 2022. Nucleic Acids Res. 50 (D1), D687–D692. doi:10.1093/nar/gkab1028
Glocker, M. O., Guthke, R., Kekow, J., and Thiesen, H.-J. (2006). Rheumatoid Arthritis, a Complex Multifactorial Disease: On the Way toward Individualized Medicine. Med. Res. Rev. 26 (1), 63–87. doi:10.1002/med.20045
Guo, Q., Wang, Y., Xu, D., Nossent, J., Pavlos, N. J., and Xu, J. (2018). Rheumatoid Arthritis: Pathological Mechanisms and Modern Pharmacologic Therapies. Bone Res. 6 (1), 15. doi:10.1038/s41413-018-0016-9
Hall, B. A., and Niarakis, A. (2021). Data Integration in Logic-Based Models of Biological Mechanisms. Curr. Opin. Syst. Biol. 28, 100386. doi:10.1016/j.coisb.2021.100386
Hao, Y., Hao, S., Andersen-Nissen, E., Mauck, W. M., Zheng, S., Butler, A., et al. (2021). Integrated Analysis of Multimodal Single-Cell Data. Cell. 184 (13), 3573–3587. e29. doi:10.1016/j.cell.2021.04.048
Hoksza, D., Gawron, P., Ostaszewski, M., Smula, E., and Schneider, R. (2019). MINERVA API and Plugins: Opening Molecular Network Analysis and Visualization to the Community. Bioinformatics, 35, 4496–4498. doi:10.1093/bioinformatics/btz286
Huber, L. C., Distler, O., Tarner, I., Gay, R. E., Gay, S., and Pap, T. (2006). Synovial Fibroblasts: Key Players in Rheumatoid Arthritis. Rheumatology 45 (6), 669–675. doi:10.1093/rheumatology/kel065
Hucka, M., Finney, A., Sauro, H. M., Bolouri, H., Doyle, J. C., Kitano, H., et al. (2003). The Systems Biology Markup Language (SBML): A Medium for Representation and Exchange of Biochemical Network Models. Bioinformatics 19 (4), 524–531. doi:10.1093/bioinformatics/btg015
Kim, J.-w., and Dang, C. V. (2005). Multifaceted Roles of Glycolytic Enzymes. Trends Biochem. Sci. 30 (3), 142–150. doi:10.1016/j.tibs.2005.01.005
King, Z. A., Lu, J., Dräger, A., Miller, P., Federowicz, S., Lerman, J. A., et al. (2016). BiGG Models: A Platform for Integrating, Standardizing and Sharing Genome-Scale Models. Nucleic Acids Res. 44 (D1), D515–D522. doi:10.1093/nar/gkv1049
Kinne, R. W., Bräuer, R., Stuhlmüller, B., Palombo-Kinne, E., and Burmester, G.-R. (2000). Macrophages in Rheumatoid Arthritis. Arthritis Res. 2 (3), 189–202. doi:10.1186/ar86
Korczowska, I. (2014). Rheumatoid Arthritis Susceptibility Genes: An Overview. Wjo 5 (4), 544. doi:10.5312/wjo.v5.i4.544
Krämer, A., Green, J., Pollard, J., and Tugendreich, S. (2014). Causal Analysis Approaches in Ingenuity Pathway Analysis. Bioinforma. Oxf. Engl. 30 (4), 523–530. doi:10.1093/bioinformatics/btt703
Kuperstein, I., Bonnet, E., Nguyen, H.-A., Cohen, D., Viara, E., Grieco, L., et al. (2015). Atlas of Cancer Signalling Network: A Systems Biology Resource for Integrative Analysis of Cancer Data with Google Maps. Oncogenesis 4 (7), e160. doi:10.1038/oncsis.2015.19
Le Novère, N. (2015). Quantitative and Logic Modelling of Molecular and Gene Networks. Nat. Rev. Genet. 16 (3), 146–158. doi:10.1038/nrg3885
Le, T., Phan, T., Pham, M., Tran, D., Lam, L., Nguyen, T., et al. (2020). BBrowser: Making Single-Cell Data Easily Accessible. bioRxiv, Available at: https://www.biorxiv.org/content/10.1101/2020.12.11.414136v1%0Ahttps://www.biorxiv.org/content/10.1101/2020.12.11.414136v1.abstract.
Li, S., Yin, H., Zhang, K., Wang, T., Yang, Y., Liu, X., et al. (2017). Effector T Helper Cell Populations Are Elevated in the Bone Marrow of Rheumatoid Arthritis Patients and Correlate with Disease Severity. Sci. Rep. 7 (1), 4776. doi:10.1038/s41598-017-05014-8
Liberzon, A., Birger, C., Thorvaldsdóttir, H., Ghandi, M., Mesirov, J. P., and Tamayo, P. (2015). The Molecular Signatures Database Hallmark Gene Set Collection. Cell. Syst. 1 (6), 417–425. doi:10.1016/j.cels.2015.12.004
Lo Surdo, P., Calderone, A., Iannuccelli, M., Licata, L., Peluso, D., Castagnoli, L., et al. (2018). DISNOR: A Disease Network Open Resource. Nucleic Acids Res. 46 (D1), D527–D534. doi:10.1093/nar/gkx876
Martens, M., Ammar, A., Riutta, A., Waagmeester, A., Slenter, D. N., Hanspers, K., et al. (2021). WikiPathways: Connecting Communities. Nucleic Acids Res. 49 (D1), D613–D621. doi:10.1093/nar/gkaa1024
Mazein, A., Knowles, R. G., Adcock, I., Chung, K. F., Wheelock, C. E., Maitland‐van der Zee, A. H., et al. (2018). AsthmaMap: An Expert‐Driven Computational Representation of Disease Mechanisms. Clin. Exp. Allergy 48 (8), 916–918. doi:10.1111/cea.13211
Mazein, A., Ostaszewski, M., Kuperstein, I., Watterson, S., Le Novère, N., Lefaudeux, D., et al. (2018). Systems Medicine Disease Maps: Community-Driven Comprehensive Representation of Disease Mechanisms. npj Syst. Biol. Appl. 4 (1), 21. doi:10.1038/s41540-018-0059-y
McInnes, I. B., and Schett, G. (2011). The Pathogenesis of Rheumatoid Arthritis. N. Engl. J. Med. 365 (23), 2205–2219. doi:10.1056/NEJMra1004965
Mi, H., and Thomas, P. (2009). “PANTHER Pathway: An Ontology-Based Pathway Database Coupled with Data Analysis Tools,” in Methods in Molecular Biology. Editor Clifton, N. J. (Totowa, NJ: Humana Press), 123–140. doi:10.1007/978-1-60761-175-2_7
Miagoux, Q., Singh, V., de Mézquita, D., Chaudru, V., Elati, M., Petit-Teixeira, E., et al. (2021). Inference of an Integrative, Executable Network for Rheumatoid Arthritis Combining Data-Driven Machine Learning Approaches and a State-Of-The-Art Mechanistic Disease Map. Jpm 11 (8), 785. doi:10.3390/jpm11080785
Niarakis, A., and Helikar, T. (2021). A Practical Guide to Mechanistic Systems Modeling in Biology Using a Logic-Based Approach. Briefings Bioinforma. 22 (4), 1–19. doi:10.1093/bib/bbaa236
Novère, N. L., Finney, A., Hucka, M., Bhalla, U. S., Campagne, F., Collado-Vides, J., et al. (2005). Minimum Information Requested in the Annotation of Biochemical Models (MIRIAM). Nat. Biotechnol. 23 (12), 1509–1515. doi:10.1038/nbt1156
Oda, K., Kimura, T., Matsuoka, Y., Funahashi, A., Muramatsu, M., Kitano, H., et al. (2004). Molecular Interaction Map of a Macrophage. AfCS Res. Rep. 2 (14), 1–12.
Ogata, H., Goto, S., Sato, K., Fujibuchi, W., Bono, H., and Kanehisa, M. (1999). KEGG: Kyoto Encyclopedia of Genes and Genomes. Nucleic Acids Res. 27 (1), 29–34. doi:10.1093/nar/27.1.29
Ostaszewski, M., Gebel, S., Kuperstein, I., Mazein, A., Zinovyev, A., Dogrusoz, U., et al. (2019). Community-Driven Roadmap for Integrated Disease Maps. Briefings Bioinforma. 20 (2), 659–670. doi:10.1093/bib/bby024
Ostaszewski, M., Niarakis, N., Mazein, A., Kuperstein, I., Phair, R., Orta-Resendiz, A., et al. (2021). COVID19 Disease Map, a Computational Knowledge Repository of Virus–Host Interaction Mechanisms. Mol. Syst. Biol. 17 (10), 1–22. doi:10.15252/msb.202110387
Pereira, C., Mazein, A., Farinha, C. M., Gray, M. A., Kunzelmann, K., Ostaszewski, M., et al. (2021). CyFi-MAP: An Interactive Pathway-Based Resource for Cystic Fibrosis. Sci. Rep. 11 (1), 22223. doi:10.1038/s41598-021-01618-3
Pucino, V., Certo, M., Varricchi, G., Marone, G., Ursini, F., Rossi, F. W., et al. (2020). Metabolic Checkpoints in Rheumatoid Arthritis. Front. Physiol. 11 (April), 1–12. doi:10.3389/fphys.2020.00347
Regev, A., Teichmann, S. A., Lander, E. S., Amit, I., Benoist, C., Birney, E., et al. (2017). The Human Cell Atlas. eLife 6, 1–30. doi:10.7554/eLife.27041
Ritchie, M. E., Phipson, B., Wu, D., Hu, Y., Law, C. W., Shi, W., et al. (2015). Limma Powers Differential Expression Analyses for RNA-Sequencing and Microarray Studies. Nucleic Acids Res. 43 (7), e47. doi:10.1093/nar/gkv007
Schulze-Koops, H., and Kalden, J. R. (2001). The Balance of Th1/Th2 Cytokines in Rheumatoid Arthritis. Best Pract. Res. Clin. Rheumatology 15 (5), 677–691. doi:10.1053/berh.2001.0187
Serhan, C. N., Gupta, S. K., Perretti, M., Godson, C., Brennan, E., Li, Y., et al. (2020). The Atlas of Inflammation Resolution (AIR). Mol. Aspects Med. 74 (September), 100894. doi:10.1016/j.mam.2020.100894
Sigaux, J., Biton, J., André, E., Semerano, L., and Boissier, M.-C. (2019). Air Pollution as a Determinant of Rheumatoid Arthritis. Jt. Bone Spine 86 (1), 37–42. doi:10.1016/j.jbspin.2018.03.001
Singh, V., Kalliolias, G. D., Ostaszewski, M., Veyssiere, M., Pilalis, E., Gawron, P., et al. (2020). RA-map: Building a State-Of-The-Art Interactive Knowledge Base for Rheumatoid Arthritis. Database 2020, 1–18. doi:10.1093/database/baaa017
Singh, V., Ostaszewski, M., Kalliolias, G. D., Chiocchia, G., Olaso, R., Petit-Teixeira, E., et al. (2018). Computational Systems Biology Approach for the Study of Rheumatoid Arthritis: From a Molecular Map to a Dynamical Model. Genomics Comput. Biol. 4 (1), 100050. doi:10.18547/gcb.2018.vol4.iss1.e100050
Smolen, J. S., Aletaha, D., and McInnes, I. B. (2016). Rheumatoid Arthritis. Lancet 388 (10055), 2023–2038. doi:10.1016/S0140-6736(16)30173-8
Subramanian, A., Tamayo, P., Mootha, V. K., Mukherjee, S., Ebert, B. L., Gillette, M. A., et al. (2005). Gene Set Enrichment Analysis: a Knowledge-Based Approach for Interpreting Genome-wide Expression Profiles. Proc. Natl. Acad. Sci. U.S.A. 102 (43), 15545–15550. doi:10.1073/pnas.0506580102
Talsania, M., and Scofield, R. H. (2017). Menopause and Rheumatic Disease. Rheumatic Dis. Clin. N. Am. 43 (2), 287–302. doi:10.1016/j.rdc.2016.12.011
Tanaka, T. (2003). The International HapMap Project. Nature 426 (6968), 789–796. doi:10.1038/nature02168
Triaille, C., Durez, P., Sokolova, T., Tilman, G., Méric de Bellefon, L., Galant, C., et al. (2021). Common Transcriptomic Effects of Abatacept and Other DMARDs on Rheumatoid Arthritis Synovial Tissue. Front. Immunol. 12 (August), 1–9. doi:10.3389/fimmu.2021.724895
Tweedie, S., Braschi, B., Gray, K., Jones, T. E. M., Seal, R. L., Yates, B., et al. (2021). Genenames.org: The HGNC and VGNC Resources in 2021. Nucleic Acids Res. 49 (D1), D939–D946. doi:10.1093/nar/gkaa980
Vuong, H., Truong, T., Phan, T., and Pham, S. (2020). Venice: A New Algorithm for Finding Marker Genes in Single-Cell Transcriptomic Data. bioRxiv. doi:10.1101/2020.11.16.384479
Wentker, P., Eberhardt, M., Dreyer, F. S., Bertrams, W., Cantone, M., Griss, K., et al. (2017). An Interactive Macrophage Signal Transduction Map Facilitates Comparative Analyses of High-Throughput Data. J. I. 198 (5), 2191–2201. doi:10.4049/jimmunol.1502513
Weyand, C. M., and Goronzy, J. J. (2017). Immunometabolism in Early and Late Stages of Rheumatoid Arthritis. Nat. Rev. Rheumatol. 13 (5), 291–301. doi:10.1038/nrrheum.2017.49
Wilkinson, M. D., Dumontier, M., Aalbersberg, I. J., Appleton, G., Axton, M., Baak, A., et al. (2016). The FAIR Guiding Principles for Scientific Data Management and Stewardship. Sci. Data 3 (1), 160018. doi:10.1038/sdata.2016.18
Wishart, D. S., Knox, C., Guo, A. C., Cheng, D., Shrivastava, S., Tzur, D., et al. (2008). DrugBank: A Knowledgebase for Drugs, Drug Actions and Drug Targets. Nucleic Acids Res. 36 (Suppl. l_1), D901–D906. doi:10.1093/nar/gkm958
Wu, G., Zhu, L., Dent, J. E., and Nardini, C. (2010). A Comprehensive Molecular Interaction Map for Rheumatoid Arthritis. PLoS ONE 5 (4), e10137. doi:10.1371/journal.pone.0010137
Yang, X., Chang, Y., and Wei, W. (2020). Emerging Role of Targeting Macrophages in Rheumatoid Arthritis: Focus on Polarization, Metabolism and Apoptosis. Cell. Prolif. 53 (7), 1–12. doi:10.1111/cpr.12854
Yi, O., Lin, Y., Hu, M., Hu, S., Su, Z., Liao, J., et al. (2022). Lactate Metabolism in Rheumatoid Arthritis: Pathogenic Mechanisms and Therapeutic Intervention with Natural Compounds. Phytomedicine 100 (February), 154048. doi:10.1016/j.phymed.2022.154048
Keywords: rheumatoid arthritis, autoimmune disease, molecular interaction maps, systems biology graphical notation, bio-curation
Citation: Zerrouk N, Aghakhani S, Singh V, Augé F and Niarakis A (2022) A Mechanistic Cellular Atlas of the Rheumatic Joint. Front. Syst. Biol. 2:925791. doi: 10.3389/fsysb.2022.925791
Received: 21 April 2022; Accepted: 10 June 2022;
Published: 11 July 2022.
Edited by:
Angelyn Lao, De La Salle University, PhilippinesReviewed by:
Narmada Sambaturu, Los Alamos National Laboratory (DOE), United StatesJing Wu, Southern Medical University, China
Copyright © 2022 Zerrouk, Aghakhani, Singh, Augé and Niarakis. This is an open-access article distributed under the terms of the Creative Commons Attribution License (CC BY). The use, distribution or reproduction in other forums is permitted, provided the original author(s) and the copyright owner(s) are credited and that the original publication in this journal is cited, in accordance with accepted academic practice. No use, distribution or reproduction is permitted which does not comply with these terms.
*Correspondence: Anna Niarakis, YW5uYS5uaWFyYWtpQHVuaXYtZXZyeS5mcg==
†These authors have contributed equally to this work