- 1Department of Radiology, The Second Affiliated Hospital of Zhejiang University School of Medicine, Hangzhou, China
- 2Department of Neurology, The Second Affiliated Hospital of Zhejiang University School of Medicine, Hangzhou, China
Background: Early-onset Alzheimer’s disease (EOAD) presents a different clinical profile than late-onset Alzheimer’s disease (LOAD). Neuroimaging studies have demonstrated that patients with EOAD present more atrophy and functional disconnection than LOAD patients. However, it remains unknown whether the interhemispheric functional disconnection or its underlying structural impairment contributes to the different clinical profiles of EOAD and LOAD.
Methods: According to the arbitrary cut-off age of 65, we included 22 EOAD patients, 27 LOAD patients and 38 healthy controls (further divided into 21 relatively young and 17 old controls). Participants underwent resting-state functional MRI, diffusion tensor imaging (DTI) and comprehensive neuropsychological assessments. We used voxel-mirrored homotopic connectivity (VMHC) to examine interhemispheric functional connectivity. Then, we calculated the diffusion index based on tract-based spatial statistics (TBSS). Two-sample t-tests were used to assess the interhemispheric connectivity differences between each patient group and its corresponding control group.
Results: We found that the EOAD patients had lower VMHC in the hippocampus, parahippocampal gyrus (PHG), superior temporal gyrus (STG) and inferior parietal cortex (IPC) than did controls. Consistently, the EOAD patients exhibited white matter (WM) tract impairment in the posterior regions. On the other hand, the LOAD patients displayed increased VMHC and impaired WM tracts in the frontal region. Correlation analyses showed that VMHC in the IPC was related to executive function in the EOAD patients (r = −0.67, P < 0.05).
Conclusion: In contrast to the LOAD patients, patients with EOAD exhibited more widely disrupted interhemispheric functional and structural connectivity, which overlapped well across brain regions. In addition, for the EOAD patients, decreased interhemispheric connectivity related to executive deficits. Our study suggested that different interhemispheric connectivity damage patterns may contribute to the distinct clinical profiles in EOAD and LOAD.
Introduction
As the most common form of dementia, Alzheimer’s disease (AD) is characterized by memory and other cognitive ability deficits. According to the arbitrary age cut-off of 65 years, we can further classify AD into early-onset AD (EOAD) and late-onset AD (LOAD; McKhann et al., 1984; Filley et al., 1986). Although they share the same neuropathological hallmarks, EOAD features different clinical characteristics from LOAD. Specifically, EOAD patients have worse attention, executive function, language and visuospatial function, but less memory impairment (Karas et al., 2007; Stopford et al., 2008; Smits et al., 2012; Joubert et al., 2016). Moreover, patients with EOAD have a more aggressive disease course and more psychiatric problems than LOAD patients (Koss et al., 1996; Toyota et al., 2007; Koedam et al., 2010). Until now, the underlying mechanisms have remained unclear.
AD has been considered a disconnection syndrome, to some extent, that features a potential decrease in interhemispheric connectivity (Lakmache et al., 1998; Delbeuck et al., 2003; Bartzokis et al., 2004; Liu et al., 2014). Notably, a previous functional MRI study demonstrated that AD patients could perform normally in intrahemispheric-based tasks while performing poorly on tasks requiring interhemispheric communication (Lakmache et al., 1998). This phenomenon is supported by morphologic studies documenting corpus callosum (CC) atrophy in AD patients (Wang et al., 2006; Chaim et al., 2007). Furthermore, microstructural studies demonstrated that EOAD patients have a widespread pattern of white matter (WM) loss, especially in the CC splenium and dorsal temporoparietal regions (Canu et al., 2012). In contrast, WM loss in LOAD patients is mainly confined to memory-related areas (Canu et al., 2012). Building on these studies, we therefore hypothesized that different clinical symptoms between EOAD and LOAD patients might be attributed to distinct deficits in interhemispheric functional or structural connectivity.
Voxel-mirrored homotopic connectivity (VMHC) assesses interhemispheric resting-state functional connectivity (RSFC) by quantifying the RSFC in one hemisphere and its mirrored counterpart in the other. The approach has been well validated in previous studies with AD patients (Wang et al., 2015; Luo et al., 2016), individuals with a high risk of developing AD (Luo et al., 2016), and healthy aging individuals (Zuo et al., 2010). Specifically, these studies proved that interhemispheric connectivity calculated by VMHC can adequately reflect the functional consequences of pathologies. Furthermore, we examined WM connectivity by diffusion tensor imaging (DTI), based on the hypothesis that there is a strong link between structural and functional connectivity. Diffusion indices, including fractional anisotropy (FA), mean diffusivity (MD), axial diffusivity (AxD) and radial diffusivity (RD), were used to assess interhemispheric structural connectivity (Liu et al., 2011; Li et al., 2013). Currently, based on multi-modal MRI imaging methods, we aimed to test the hypothesis that patients with EOAD and LOAD have different damage patterns of interhemispheric connectivity. Moreover, we sought to clarify the relationships between neuroimaging indices and cognitive performance.
Materials and Methods
Subjects
We recruited patients from the Second Affiliated Hospital of Zhejiang University School of Medicine. The Medical Ethics Committee of the Second Affiliated Hospital, Zhejiang University School of Medicine, approved this study, and all participants provided informed consent at the beginning of the study. The diagnosis of early AD was made by an experienced neurologist (JZ) according to the NINCDS-ADRDA Alzheimer’s Criteria for “probable AD” (McKhann et al., 1984). The cut-off of 65 years of age was used to distinguish EOAD from LOAD, primarily established on clinical grounds (Amaducci et al., 1986; Filley et al., 1986; Kim et al., 2005). The neurologist confirmed the age of onset according to the information given by the patient and caregiver. The inclusion criteria for AD included: (a) having an MMSE scores between 20 and 26 (inclusive); (b) having a clinical dementia rating (CDR) of 0.5 or 1.0; and (c) satisfying the NINCDS/ADRDA criteria for probable AD (McKhann et al., 1984). Normal controls (NC) consisted of family members of patients and volunteers recruited through advertisements posted in the hospital. The inclusion criteria for NC the following: (a) having an MMSE between 24 and 30 (inclusive); (b) having a CDR score of 0; (c) having a normal Wechsler Memory Scale Logical Memory (WMS-LM) delay score (in detail: ≥9 for subjects with 16 or more years of education; ≥5 for subjects with 8–15 years of education; and ≥3 for 0–7 years of education); (d) non-clinical depression (geriatric depression scale, GDS score <5); and (e) non-demented. We excluded subjects with the following manifestations: (1) significant medical, neurological (other than probable AD), or psychiatric illness; (2) a history of obvious head trauma; (3) use of non-AD-related medication known to influence cerebral function; (4) signs of clinical depression (GDS score ≥5); and (5) alcohol or drug abuse; and (6) left-handedness.
In the end, we included 24 EOAD and 27 LOAD right-handed patients. Given the significant difference in age between the EOAD and LOAD patients, 21 young controls and 17 old controls were included to match the EOAD and LOAD patients, respectively. Table 1 shows the details of the demographic characteristics.
Neuropsychological Tests
All patients underwent extensive neuropsychological testing, including assessment of general cognition (Mini-Mental State Examination, MMSE; Montreal Cognitive Assessment scale, MoCA) and other cognitive domains, involving memory function (Auditory Verbal Learning Test, AVLT; WMS-LM, immediate and delayed memory), language (Boston Naming Test, BNT), attention (Trail Making Test part A, TMT-A) and executive functioning (Trail Making Test part B, TMT-B). NC were only assessed by the MMSE, MoCA and WMS-LM, immediate and delayed memory.
MRI Data Acquisition
We acquired both the structural and resting-state functional image data on a 3.0-Tesla GE Discover 750 MRI Scanner. Foam padding and earplugs were used to limit head motion and reduce scanner noise. T1-weighted images were acquired using a Sag FSPGR 3D ACC sequence with the following parameters: repetition time (RT) = 7.336 ms; echo time (TE) = 3.036 ms; inversion time (TI) = 450 ms; 196 sagittal slices; within plane FOV = 256 × 256 mm2; voxel size = 1.02 × 1.02 × 1.2 mm3; flip angle = 11°; and bandwidth = 244.141 Hz/pix. Resting-state functional MRI (rsfMRI) images were obtained with the following parameters: 205 time points; TR = 2,000 ms; TE = 30 ms; flip angle = 77°; number of slices = 38; slice thickness = 4 mm; spatial resolution = 3.75 × 3.75 × 4 mm3; and matrix = 64 × 64. DTI was obtained using the Ax DTI 30 sequence with the following parameters: 30 non-linear directions, b-value = 1,000 s/mm2, TR/TE = 6,000 ms/80.8 ms, 35 axial slices, slice thickness = 2 mm, FOV = 256 mm × 256 mm2, acquisition matrix = 128 × 128, and number of averages = 1. According to the scan protocol, all subjects were instructed to close their eyes and keep at rest calmly during the course of the scan.
Imaging Pre-processing and VMHC Estimation
We pre-processed all data using the Data Processing Assistant and Resting-State FMRI (DPARSF, version 2.2; Chao-Gan and Yu-Feng, 20101) with Statistical Parametric Mapping 8 (SPM82) on the MATLAB platform. First, we discarded the first 10 image volumes of rsfMRI scans due to the signal equilibrium and subject’s adaptation to the scanning noise. Then, we corrected the remaining 195 images for timing differences (37th slice as the reference slice) and head motion (24 parameters; Friston et al., 1996). Here, we discarded the image data with more than 2.5 mm maximum displacement in any of the x, y, or z directions or 2.5° of any angular motion (two EOAD patients excluded Supplementary Material, Part 6). Subsequently, based on rigid-body transformation, we coregistered the T1-weighted image to the mean rsfMRI image and spatially normalized these images to the Montreal Neurological Institute (MNI) space. A standardized image was subsequently re-sampled into 3 mm × 3 mm × 3 mm cubic voxels. To decrease spatial noise, we smoothed the rsfMRI images with a Gaussian kernel of 8 mm × 8 mm × 8 mm full width at half maximum (FWHM). We also performed a detrend and filter (0.01 Hz < f < 0.08 Hz) to discard the bias from the high-frequency physiological noise and the low-frequency drift. Finally, data were scrubbed to further reduce motion-related artifacts by using a frame-wise displacement (FD) threshold of 0.5, with which bad points were interpolated using the nearest neighbor (Yan et al., 2013). To remove residual effects of motion and other non-neuronal factors, we controlled for covariates including 24 head motion parameters and signals of WM and cerebrospinal fluid (CSF). Additionally, we considered the FD value of each subject as a covariate in a subsequent analysis procedure.
Regarding the VMHC analysis, we transformed pre-processed images by applying the rsfMRI image to a symmetric brain template, obtained by flipping the left or right hemispheres along the X-axis midline and averaging with the original image. Then, the T1 and rsfMRI images from each subject that had been normalized to the MNI space were co-registered nonlinearly to this group-specific symmetric template. Finally, we estimated the homotopic RSFC based on the Pearson correlation between every pair of symmetrical interhemispheric voxels’ time series. The resultant correlations constituted the VMHC map, followed by Fisher’s Z transformation. Notably, we used a unilateral brain mask to present results because the VMHC results were symmetric.
Seed-Based Analysis
To reflect the all-around functional connectivity, seed-based analyses were complementarily performed. Five seed region of interests (ROIs) were selected to anchor the Default Mode Network (DMN), the left executive control network, the right executive control network, the language network, and the higher visual network (Lehmann et al., 2015). The average time series were extracted from 8 mm spheres around the peak intensity voxels. Then, a general analysis was performed to produce a subject-level intrinsic connectivity map with age, education and gender as covariates. The statistical analysis and results are listed in Supplementary Material, Part 1.
Tract-Based Spatial Statistics (TBSS)
We analyzed DTI data following the standard procedure with PANDA version 1.3.03 (Cui et al., 2013). Tract-based spatial statistics (TBSS) were used to calculate the diffusivity indices. First, we corrected eddy current distortions by registering the DW images to a b0 image with an affine transformation. Then, voxel-wise maps of FA, MD, AxD and RD were calculated with the DTIFIT program. Subsequently, a mean FA image was created by directly registering individual FA volumes to the FMRIB58 template (Smith et al., 2006) by non-linear registrations and thinned to represent the mean FA skeleton (FA > 0.25 overlaid with the mean FA image). Finally, the diffusion index data from individual subjects were projected onto this skeleton to create individual images.
Gray Matter Volume Analysis
The T1-weighted images were pre-processed using the Computational Anatomy Toolbox (CAT124). First, the T1 image was spatially registered to the tissue probability maps (TPM) and then segmented into gray matter (GM), WM and CSF. ICBM152 space was used to perform the affine registration to the stereotactic MNI space. Then, high-dimensional DARTEL normalization and nonlinear modulation using the Jacobian determinants derived from the normalization process were performed. Subsequently, we smoothed the GM image with a Gaussian kernel of 8 mm × 8 mm × 8 mm to reduce potential inaccuracies during the normalization step. Moreover, we extracted the GM volume (GMV) in every ROI based on the Hammers atlas provided by CAT12 and used these data in subsequent analyses.
Statistical Analyses
We analyzed the demographic data using the Chi-squared test for categorical data and t-test for continuous data (SPSS version 19.0).
Then, we examined the VMHC differences between EOAD and LOAD in a voxel-wise manner based on REST software5). In detail, we performed a two-sample t-test between the patient and control groups by setting the statistical threshold at P < 0.001 and cluster size >10 voxels (uncorrected). Moreover, to evaluate the impact of WM lesion, we additionally performed the analysis by adding WM load as a covariate. For detail, Supplementary Material, Part 5.
Regarding the DTI results, voxel-wise group comparisons were carried out using non-parametric, two-sample t-tests (randomize, Smith et al., 2006) in the patient vs. its corresponding control group (corrected by threshold-free cluster enhancement p < 0.05). We used the mean FA skeleton as a mask with a threshold of 0.25 and set the number of permutations at 5,000 (Smith and Nichols, 2009).
Regarding GMV results, voxel-wise group comparisons were performed using the two-sample t-test between the EOAD patients, LOAD patients and age-matched controls with age, gender, and education as the covariates. Moreover, a significant threshold (P < 0.005, cluster size >500) was applied after correction for multiple comparisons using a false discovery rate (FDR) procedure. Moreover, ROI-based group comparisons were performed in patients vs. NC, within the young and old groups (mainly focusing on HP and medial temporal lobe (MTL)).
We defined brain regions showing significant between-group differences as ROIs and extracted the values within them. Then, we conducted Pearson correlation analyses between the interhemispheric RSFC, diffusion indices, GMV and behavioral data. Moreover, regression analysis with neuropsychological tests as dependent variables and age of onset, gender, education and neuroimaging data as independent variables were performed to define whether neuroimaging variables predicted cognitive functions and whether the diagnostic group impacts these correlations.
Results
Demographics
The EOAD and LOAD patients matched well with their respective controls for age, gender, education level, GDS and FD value (P > 0.05, Table 1). Regarding the neuropsychological profiles, both EOAD and LOAD patients performed worse than their respective controls in MMSE, MoCA, WMS-LM and AVLT scores (P < 0.05, Table 2).
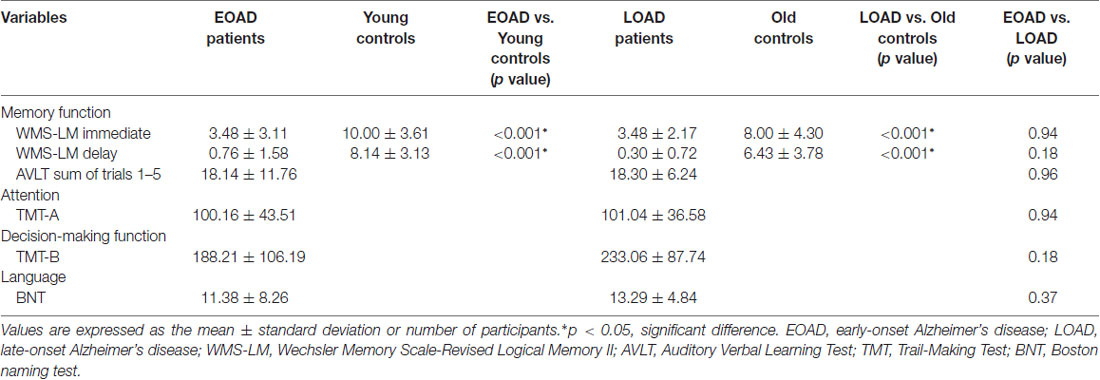
Table 2. Neuropsychological test performance of early-onset and late-onset Alzheimer’s disease (AD) patients.
Interhemispheric Functional Connectivity Differences
The EOAD patients showed lower interhemispheric RSFC in the hippocampus (HP), parahippocampal gyrus (PHG), superior temporal gyrus (STG) and inferior parietal cortex (IPC) than the young controls (Figure 1). In contrast, the LOAD patients showed greater interhemispheric RSFC in the medial frontal gyrus (MFG) than the old controls (Table 3 and Figure 1).
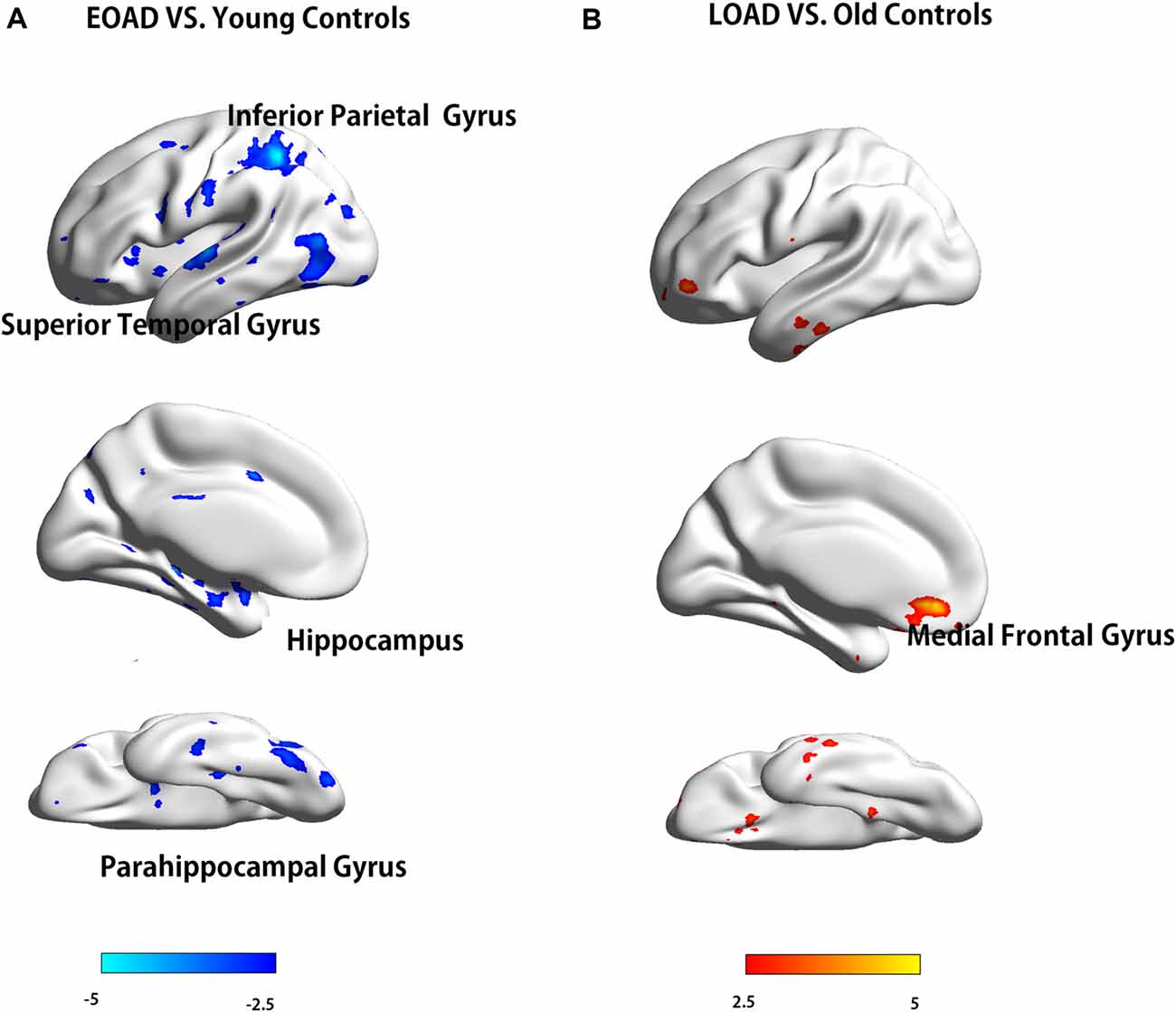
Figure 1. Difference in VMHC between patients and controls (EOAD patients vs. young controls; LOAD patients vs. old controls). Comparison of interhemispheric RSFC between patients and controls. (A) The EOAD patients showed significantly decreased interhemispheric RSFC in the HP, PHG, STG and IPC. (B) The LOAD patients had increased VMHC in the MFG (P < 0.001, cluster size >10 voxels, uncorrected). Note: only the left side of the image was displayed due to the symmetric template used in the analysis. VMHC, voxel-mirrored homotopic connectivity; EOAD, early-onset Alzheimer’s disease; LOAD, late-onset Alzheimer’s disease; RSFC, resting-state functional connectivity; HP, hippocampus; PHG, parahippocampal gyrus; STG, superior temporal gyrus; IPC, inferior parietal cortex; MFG, medial frontal gyrus.
Interhemispheric Structural Connectivity Differences
Compared to the young controls, the EOAD patients showed reduced FA in widespread WM regions (posterior thalamic radiation, genu of CC and anterior corona radiata) and increased MD (in posterior parts of the brain, including the genu of CC, CC splenium and parietooccipital regions). Additionally, the EOAD patients showed increased AxD (in the body of CC, bilateral superior corona radiata, CC splenium and parietooccipital regions) and increased RD (in the posterior corona radiata, anterior corona radiata, right fornix, right cerebral peduncle, right external capsule and inferior temporal gyrus, Figure 2).
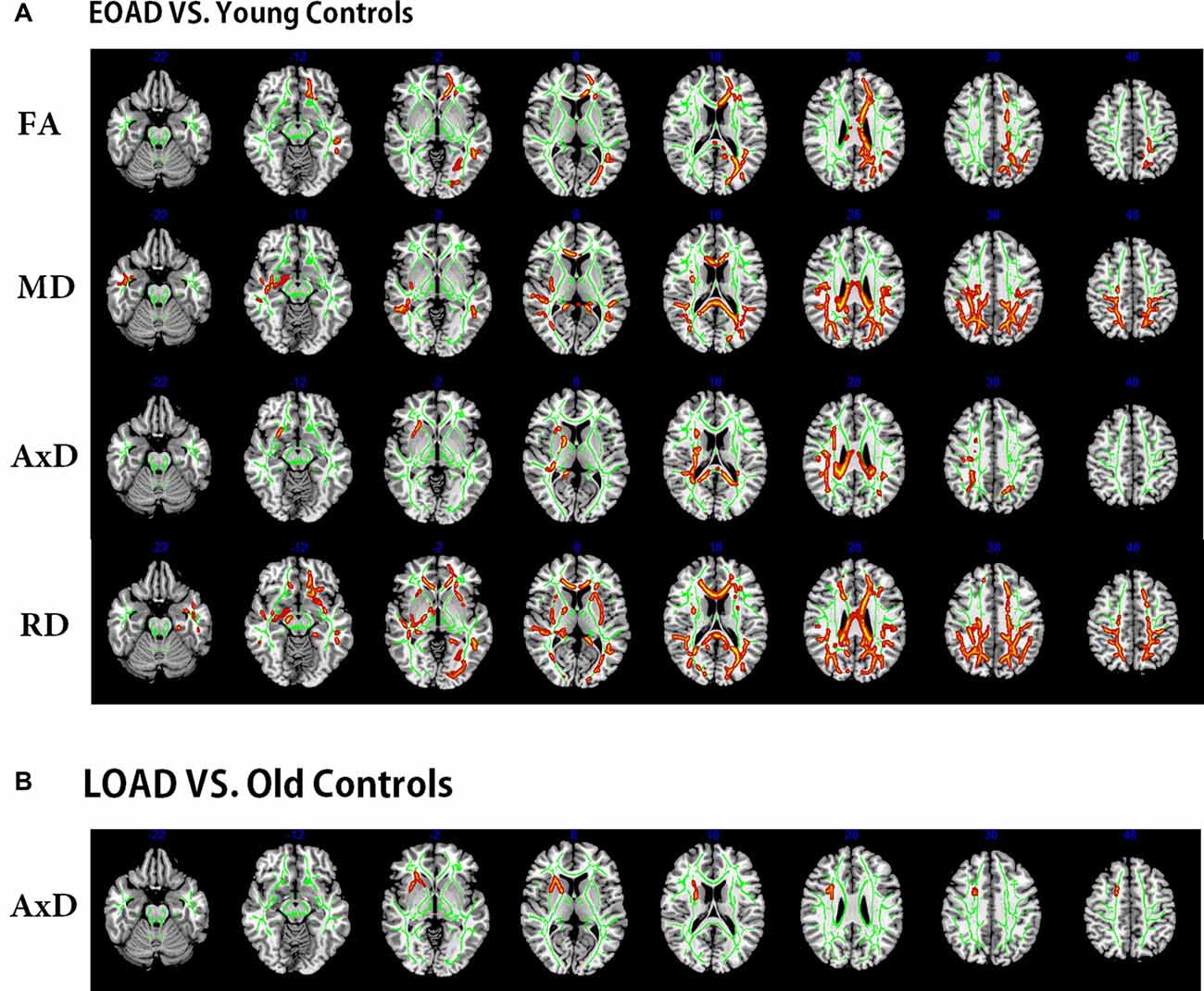
Figure 2. Tract-based spatial statistics (TBSS) results of the diffusion indices between the patients and controls (EOAD patients vs. young; LOAD patients vs. old controls). Green represents the mean white matter (WM) skeleton of all subjects. (A) Red-yellow voxels represent the WM regions with reduced FA (first row), increased MD (second row), increased AxD (third row), and increased RD (fourth row) in the EOAD patients compared with the young controls (p < 0.05, corrected). (B) Red-yellow voxels represent the WM regions with increased AxD in the LOAD patients compared with the old controls (p < 0.05, corrected). EOAD, early-onset Alzheimer’s disease; LOAD, late-onset Alzheimer’s disease; FA, fractional anisotropy; MD, mean diffusivity; AxD, axial diffusivity; RD, radial diffusivity.
The LOAD patients only showed increased AxD in the right anterior corona radiata (Figure 2) when compared to AxD in the old controls. No significant difference was observed between the LOAD patients and old controls in FA, MD and RD indices (overlap map of VMHC and DTI in Supplementary Material, Part 2).
Gray Matter Volume Difference
The EOAD patients showed decreased GMV in the PHG and IPC, while the LOAD patients showed decreased GMV in the PHG, compared to their respective controls. Moreover, the ROI-based comparison showed that the LOAD patients had a smaller HP and MTL volume than the EOAD patients (Supplementary Material, Part 3).
Correlation of Interhemispheric Connectivity With Behavioral Data
In the EOAD group, the VMHC of the IPC was negatively associated with the TMT-B scores (r = −0.67, P < 0.05, Figure 3A) and MD of the CC splenium positively associated with the TMT-B scores (r = 0.76, P < 0.001, Figure 3B). In the LOAD group, GMV in the HP positively correlated with the AVLT scores (p = 0.005, r = 0.56; Supplementary Material, Part 3). Similar results were also found in the regression analysis (Supplementary Material, Part 4).
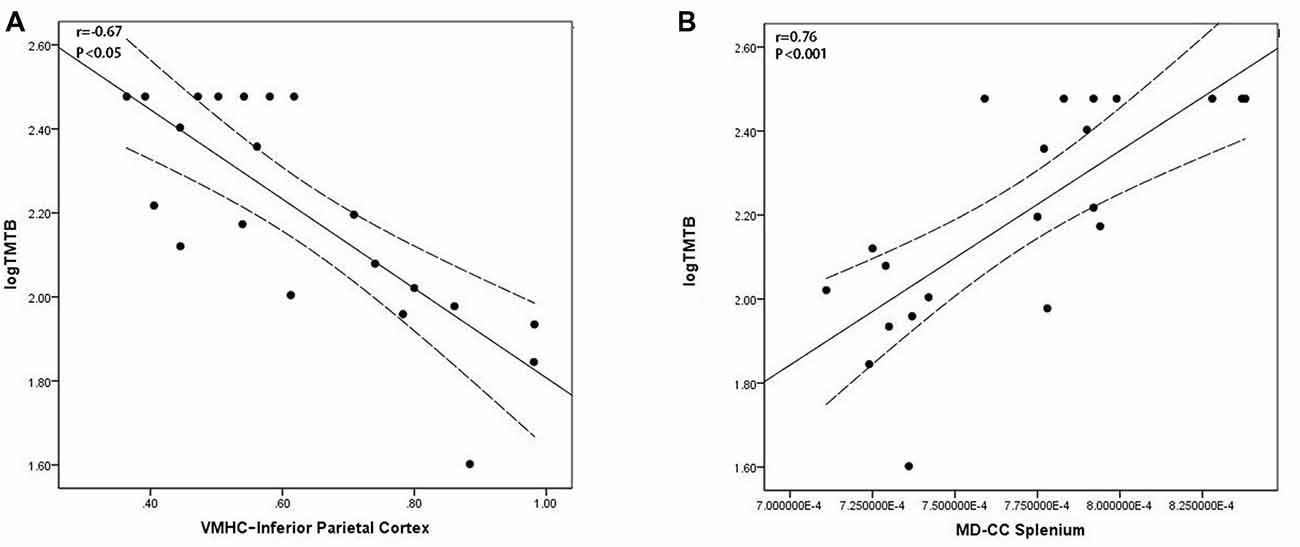
Figure 3. Scatter plot diagram of the correlation between executive performance and VMHC/diffusion indices in the EOAD patients. Scatter plot diagram displaying the 95% confidence band of the best-fit line. (A) The decreased VMHC of the IPC was negatively correlated with executive function (r = −0.67, P < 0.05). (B) The increased MD of the CC splenium was positively correlated with executive function (r = 0.76, P < 0.001). VMHC, voxel-mirrored homotopic connectivity; EOAD, early-onset Alzheimer’s disease; IPC, inferior parietal cortex; MD, mean diffusivity; CC, corpus callosum.
Discussion
Building on multi-modal MRI methods, we found distinct impairment patterns of interhemispheric functional and structural connectivity in the EOAD and LOAD patients. Our results showed that: (1) the EOAD patients had widespread decreased VMHC relative to the LOAD patients; (2) meanwhile, the EOAD patients showed extensive WM deficits, especially in the posterior regions. In contrast, only the medial frontal regions showed changes in diffusion indices in the LOAD patients compared with old controls; and (3) decreased communication between the bilateral IPC and corresponding deficits in interhemispheric structural pathways contributed to the executive function impairment within the EOAD patients.
In this study, we observed decreased interhemispheric RSFC in the HP and PHG in the EOAD patients. These structures are in charge of information encoding and convergence (Eichenbaum, 2000) and are tightly associated with declarative memory (Burwell et al., 1995; Suzuki, 1996). Previous studies have reported that these regions are susceptible to AD-related pathophysiological changes (Braak and Braak, 1991; Van Hoesen et al., 2000), which eventually cause neuronal damage and cell death (Yan et al., 1996; Hardy and Selkoe, 2002). Morphological studies may support these findings by indicating cortical atrophy in the medial temporal and parahippocampal regions in EOAD patients (Ishii et al., 2005; Möller et al., 2013; Caso et al., 2015). Functionally, our results extended previous rsfMRI studies that documented impaired functional connectivity in the HP and PHG in EOAD (Adriaanse et al., 2014; Gour et al., 2014) and further found interhemispheric functional connectivity impairments. However, we would note that Lehmann et al. (2015) once found the opposite results that EOAD displayed preserved DMN connectivity. Our seed-based functional analysis also found similar results, which showed relatively preserved DMN connectivity but more non-DMN connectivity impairments in EOAD (for details see Supplementary Material, Part 3). We inferred that the different methods might explain this discrepancy because VMHC reflects interhemispheric connectivity, while seed-based connectivity analysis focuses more on the intra-network function. On the other hand, our results showed that the STG had decreased interhemispheric functional connectivity in the EOAD patients. Although the STG is not typically a primary target site in AD, the STG possesses polysensory information interactions with the PHG (Suzuki and Amaral, 1994) and is involved in both semantic and syntactic processing. Prior studies also reported that EOAD patients had hypoperfusion and hypometabolism in the lateral temporal cortex (Ichimiya et al., 1994; Kemp et al., 2003; Frisoni et al., 2005; Shiino et al., 2008; Migliaccio et al., 2015). Furthermore, evidence from [11C] PiB-PET also suggested that decreased VMHC in the STG may derive from amyloid deposition (Cho et al., 2013). Moreover, decreased ceruloplasmin levels, which reflects decreased cellular metabolic processes and protective ability against oxidative damage, in the STG in AD patients may be another reason (Connor et al., 1993). In summary, our results suggested interhemispheric functional disruption in the EOAD patients involving the cortical-hippocampal system.
The EOAD patients also had decreased functional connectivity between the bilateral IPC. The IPC is regarded as a component of the executive frontoparietal network (Seeley et al., 2007) and showed functional and structural impairments in EOAD patients. Functionally, Gour et al. (2014) reported decreased functional connectivity in EOAD patients in the IPC. This was also proven by our seed-based network analysis and suggested its vulnerability to functional impairments in both interhemispheric and intra-network connectivity Supplementary Material, Part 1). Structurally, prior studies demonstrated that patients with EOAD had more severe atrophy and hypometabolism than LOAD patients in parietal regions (Sakamoto et al., 2002; Ishii et al., 2005; Kim et al., 2005; Möller et al., 2013; Verclytte et al., 2016). Specifically, Ossenkoppele et al. (2012) found both an increased amyloid burden and metabolic dysfunction in parietal regions in EOAD; further analysis found metabolic impairment in the parietal cortex was related to executive function and attention (Ossenkoppele et al., 2012). This evidence is consistent with our regression analysis results showing that IPC VMHC and DTI in the parietooccipital region independently contribute to executive function in EOAD. Thus, we speculated that information integration processes between the bilateral IPC might contribute to executive function.
Considering the strong link between functional and structural connectivity, we proposed that WM integrity abnormalities may underlie the pathological mechanisms of VMHC deficits in EOAD patients. In particular, we found widespread WM alterations in the EOAD patients, especially in the CC, corona radiata, thalamic radiation and posterior brain regions. Similarly, previous studies reported WM alterations in EOAD patients, mainly involving the CC and dorsal temporoparietal regions (Canu et al., 2012; Caso et al., 2015; Daianu et al., 2016). Moreover, Caso et al. (2015) reported WM damage in the medial temporal and parahippocampal regions in EOAD patients. Anatomically, these WM tracts work as the basic structure for information exchange between hemispheres (Wakana et al., 2004; Silk et al., 2009). More specifically, the CC splenium connects the temporal, parietal, and occipital regions (de Lacoste et al., 1985), while the fornix works as the major afferent and output tract of the HP (Aggleton and Brown, 1999). Thus, we hypothesized that WM impairments in the posterior regions contributed to VMHC reduction in the temporal and parietal regions in the EOAD patients. Notably, we also found an association between the CC splenium microstructural breakdown and executive function in the EOAD patients. The high consistency across different imaging modalities mutually provided conclusive support for our findings in the EOAD patients.
Regarding the LOAD patients, we found increased interhemispheric RSFC with impaired WM in the orbital part of the MFG. Previous functional studies also found increased connectivity in the frontal regions and assumed it to be a compensatory effect in LOAD patients (Agosta et al., 2012; Adriaanse et al., 2014; Gour et al., 2014). Additionally, Gour et al. (2014) reported increased functional connectivity in frontal regions and its correlation with executive function in LOAD patients. This is partially in line with our network analysis, which found increased connectivity in the prefrontal region in the LOAD patients. Our VMHC results validated these findings by demonstrating increased interhemispheric functional connections in frontal regions and its corresponding WM impairments. Moreover, we found greater GMV loss in the LOAD patients, especially in the medial temporal region, which was significantly correlated with memory. Regression analysis showed that GMV in the medial temporal region independently contributed to memory in the LOAD patients. Thus, we proposed a possible mechanism underlying LOAD: GMV loss, rather than interhemispheric connectivity, was more related to cognitive decline in the LOAD patients. Although the LOAD patients had severe GM loss, the relatively well-preserved WM structure provided the structural base for interhemispheric functional connectivity compensation.
Unexpectedly, we found no interhemispheric functional connectivity decreases in the LOAD patients. Our observation is somewhat different from previous studies demonstrating functional impairments in memory-related regions in LOAD patients (Hodges, 2006; de Waal et al., 2012; Adriaanse et al., 2014; Gour et al., 2014). Some reasons may account for this inconsistency. First, GM atrophy usually precedes WM degeneration in AD (Reid and Evans, 2013). The current study mainly focused on AD patients at an early clinical stage, which may be associated with less WM impairments. At this stage, the interhemispheric cooperation may still mostly preserve function in the LOAD patients. In addition, different cultural customs between Eastern and Western elderly should be noted. In contrast to most previous studies, our study was conducted in Chinese elderly. For example, eating food with chopsticks may significantly improve the interhemispheric communication in LOAD patients, who have relatively milder forms of AD pathologies than EOAD patients have. The work of Demirakca investigated the impact of life training on brain plasticity and found strengthened interhemispheric functional connectivity (Demirakca et al., 2016).
There were some limitations to this study. First, the small sample size decreased the statistical power. Thus, the findings should be replicated in a larger sample in the future. Second, the natural human brain is not entirely symmetrical. However, we co-registered rsfMRI data to a symmetrical structural T1 template and measured the functional correlations between mirrored regions. Although our interhemispheric structural connectivity results mostly validated the functional results, this potential bias cannot be neglected in future studies. Third, our study mainly focused on subjects in the early stages of AD with a relatively high MMSE score, which may not be representative of the whole spectrum of AD severity. In future studies, we should include more AD patients at different stages. Finally, the absence of PET amyloid data is another limitation considering its importance in the AD diagnosis.
Conclusion
In summary, we observed different impairment patterns of interhemispheric connectivity in the EOAD and LOAD patients based on multi-modal imaging methods. The EOAD patients showed widespread interhemispheric impairments of the MTL, STG, and IPC. However, we found a compensatory effect of frontal interhemispheric communication in the LOAD patients. Correspondingly, the WM alterations showed a large overlap with the functional changes, suggesting its role as the structural base of bi-hemispheric functional changes.
Author Contributions
K-CL designed the study and wrote the first draft of the manuscript. XL analyzed the MRI data and wrote the protocol. Q-ZZ and J-JX collected the clinical and MRI data. JZ diagnosed and collected the patients. X-JX, P-YH, Z-JS and M-MZ assisted with the study design and interpretation of findings. All authors have contributed to and approved the final manuscript.
Funding
This study was funded by National Key Research and Development Program of China (Grant No. 2016YFC1306600), Fundamental Research Funds for the Central Universities (Grant No. 2017XZZX001-01), Zhejiang Provincial Natural Science Foundation of China (Grant No. LZ14H180001), Projects of Medical and Health Technology Development Program in Zhejiang Province (Grant No. 2015KYB174), Young Talents Research Fund, Chinese Medicine Science and Technology Project of Zhejiang Province (Grant No. 2018ZQ035).
Conflict of Interest Statement
The authors declare that the research was conducted in the absence of any commercial or financial relationships that could be construed as a potential conflict of interest.
Footnotes
- ^ http://rfmri.org/DPARSF
- ^ www.fil.ion.ucl.ac.uk/spm/
- ^ www.nitrc.org/projects/panda/
- ^ http://dbm.neuro.uni-jena.de/cat12/
- ^ www.restfmri.net
Supplementary Material
The Supplementary Material for this article can be found online at: https://www.frontiersin.org/articles/10.3389/fnagi.2018.00261/full#supplementary-material
References
Adriaanse, S. M., Binnewijzend, M. A., Ossenkoppele, R., Tijms, B. M., van der Flier, W. M., Koene, T., et al. (2014). Widespread disruption of functional brain organization in early-onset Alzheimer’s disease. PLoS One 9:e102995. doi: 10.1371/journal.pone.0102995
Aggleton, J. P., and Brown, M. W. (1999). Episodic memory, amnesia, and the hippocampal-anterior thalamic axis. Behav. Brain Sci. 22, 425–489. doi: 10.1017/s0140525x99002034
Agosta, F., Pievani, M., Geroldi, C., Copetti, M., Frisoni, G. B., and Filippi, M. (2012). Resting state fMRI in Alzheimer’s disease: beyond the default mode network. Neurobiol. Aging 33, 1564–1578. doi: 10.1016/j.neurobiolaging.2011.06.007
Amaducci, L. A., Rocca, W. A., and Schoenberg, B. S. (1986). Origin of the distinction between Alzheimer’s disease and senile dementia: how history can clarify nosology. Neurology 36, 1497–1499. doi: 10.1212/wnl.36.11.1497
Bartzokis, G., Sultzer, D., Lu, P. H., Nuechterlein, K. H., Mintz, J., and Cummings, J. L. (2004). Heterogeneous age-related breakdown of white matter structural integrity: implications for cortical “disconnection” in aging and Alzheimer’s disease. Neurobiol. Aging 25, 843–851. doi: 10.1016/j.neurobiolaging.2003.09.005
Braak, H., and Braak, E. (1991). Neuropathological staging of Alzheimer-related changes. Acta Neuropathol. 82, 239–259. doi: 10.1007/bf00308809
Burwell, R. D., Witter, M. P., and Amaral, D. G. (1995). Perirhinal and postrhinal cortices in the rat: a review of the neuroanatomical literature and comparison with findings from the monkey brain. Hippocampus 5, 390–408. doi: 10.1002/hipo.450050503
Canu, E., Frisoni, G. B., Agosta, F., Pievani, M., Bonetti, M., and Filippi, M. (2012). Early and late onset Alzheimer’s disease patients have distinct patterns of white matter damage. Neurobiol. Aging 33, 1023–1033. doi: 10.1016/j.neurobiolaging.2010.09.021
Caso, F., Agosta, F., Mattavelli, D., Migliaccio, R., Canu, E., Magnani, G., et al. (2015). White matter degeneration in atypical Alzheimer disease. Radiology 277, 162–172. doi: 10.1148/radiol.2015142766
Chaim, T. M., Duran, F. L., Uchida, R. R., Périco, C. A., de Castro, C. C., Busatto, G. F., et al. (2007). Volumetric reduction of the corpus callosum in Alzheimer’s disease in vivo as assessed with voxel-based morphometry. Psychiatry Res. 154, 59–68. doi: 10.1016/j.pscychresns.2006.04.003
Chao-Gan, Y., and Yu-Feng, Z. (2010). DPARSF: A MATLAB toolbox for “Pipeline” data analysis of resting-state fMRI. Front Syst. Neurosci. 4:13. doi: 10.3389/fnsys.2010.00013
Cho, H., Seo, S. W., Kim, J. H., Suh, M. K., Lee, J. H., Choe, Y. S., et al. (2013). Amyloid deposition in early onset versus late onset Alzheimer’s disease. J. Alzheimers Dis. 35, 813–821. doi: 10.3233/JAD-121927
Connor, J. R., Tucker, P., Johnson, M., and Snyder, B. (1993). Ceruloplasmin levels in the human superior temporal gyrus in aging and Alzheimer’s disease. Neurosci. Lett. 159, 88–90. doi: 10.1016/0304-3940(93)90805-u
Cui, Z., Zhong, S., Xu, P., He, Y., and Gong, G. (2013). PANDA: a pipeline toolbox for analyzing brain diffusion images. Front. Hum. Neurosci. 7:42. doi: 10.3389/fnhum.2013.00042
Daianu, M., Mendez, M. F., Baboyan, V. G., Jin, Y., Melrose, R. J., Jimenez, E. E., et al. (2016). An advanced white matter tract analysis in frontotemporal dementia and early-onset Alzheimer’s disease. Brain Imaging Behav. 10, 1038–1053. doi: 10.1007/s11682-015-9458-5
de Lacoste, M. C., Kirkpatrick, J. B., and Ross, E. D. (1985). Topography of the human corpus callosum. J. Neuropathol. Exp. Neurol. 44, 578–591. doi: 10.1097/00005072-198511000-00004
de Waal, H., Stam, C. J., de Haan, W., van Straaten, E. C., Scheltens, P., and van der Flier, W. M. (2012). Young Alzheimer patients show distinct regional changes of oscillatory brain dynamics. Neurobiol. Aging 33, 1008.e25–1008.e31. doi: 10.1016/j.neurobiolaging.2011.10.013
Delbeuck, X., Van der Linden, M., and Collette, F. (2003). Alzheimer’s disease as a disconnection syndrome? Neuropsychol. Rev. 13, 79–92. doi: 10.1023/A:1023832305702
Demirakca, T., Cardinale, V., Dehn, S., Ruf, M., and Ende, G. (2016). The exercising brain: changes in functional connectivity induced by an integrated multimodal cognitive and whole-body coordination training. Neural Plast. 2016:8240894. doi: 10.1155/2016/8240894
Eichenbaum, H. (2000). A cortical-hippocampal system for declarative memory. Nat. Rev. Neurosci. 1, 41–50. doi: 10.1038/35036213
Filley, C. M., Kelly, J., and Heaton, R. K. (1986). Neuropsychologic features of early- and late-onset Alzheimer’s disease. Arch. Neurol. 43, 574–576. doi: 10.1001/archneur.1986.00520060038014
Frisoni, G. B., Testa, C., Sabattoli, F., Beltramello, A., Soininen, H., and Laakso, M. P. (2005). Structural correlates of early and late onset Alzheimer’s disease: voxel based morphometric study. J. Neurol. Neurosurg. Psychiatry 76, 112–114. doi: 10.1136/jnnp.2003.029876
Friston, K. J., Williams, S., Howard, R., Frackowiak, R. S., and Turner, R. (1996). Movement-related effects in fMRI time-series. Magn. Reson. Med. 35, 346–355. doi: 10.1002/mrm.1910350312
Gour, N., Felician, O., Didic, M., Koric, L., Gueriot, C., Chanoine, V., et al. (2014). Functional connectivity changes differ in early and late-onset Alzheimer’s disease. Hum. Brain Mapp. 35, 2978–2994. doi: 10.1002/hbm.22379
Hardy, J. A., and Selkoe, D. J. (2002). The amyloid hypothesis of Alzheimer’s disease: progress and problems on the road to therapeutics. Science 197, 353–356. doi: 10.1126/science.1072994
Hodges, J. R. (2006). Alzheimer’s centennial legacy: origins, landmarks and the current status of knowledge concerning cognitive aspects. Brain 129, 2811–2822. doi: 10.1093/brain/awl275
Ichimiya, A., Herholz, K., Mielke, R., Kessler, J., Slansky, I., and Heiss, W. D. (1994). Difference of regional cerebral metabolic pattern between presenile and senile dementia of the Alzheimer type: a factor analytic study. J. Neurol. Sci. 123, 11–17. doi: 10.1016/0022-510x(94)90197-x
Ishii, K., Kawachi, T., Sasaki, H., Kono, A. K., Fukuda, T., Kojima, Y., et al. (2005). Voxel-based morphometric comparison between early- and late-onset mild Alzheimer’s disease and assessment of diagnostic performance of z score images. AJNR Am. J. Neuroradiol. 26, 333–340.
Joubert, S., Gour, N., Guedj, E., Didic, M., Guériot, C., Koric, L., et al. (2016). Early-onset and late-onset Alzheimer’s disease are associated with distinct patterns of memory impairment. Cortex 74, 217–232. doi: 10.1016/j.cortex.2015.10.014
Karas, G., Scheltens, P., Rombouts, S., van Schijndel, R., Klein, M., Jones, B., et al. (2007). Precuneus atrophy in early-onset Alzheimer’s disease: a morphometric structural MRI study. Neuroradiology 49, 967–976. doi: 10.1007/s00234-007-0269-2
Kemp, P. M., Holmes, C., Hoffmann, S. M., Bolt, L., Holmes, R., Rowden, J., et al. (2003). Alzheimer’s disease: differences in technetium-99m HMPAO SPECT scan findings between early onset and late onset dementia. J. Neurol. Neurosurg. Psychiatry 74, 715–719. doi: 10.1136/jnnp.74.6.715
Kim, E. J., Cho, S. S., Jeong, Y., Park, K. C., Kang, S. J., Kang, E., et al. (2005). Glucose metabolism in early onset versus late onset Alzheimer’s disease: an SPM analysis of 120 patients. Brain 128, 1790–1801. doi: 10.1093/brain/awh539
Koedam, E. L., Lauffer, V., van der Vlies, A. E., van der Flier, W. M., Scheltens, P., and Pijnenburg, Y. A. (2010). Early-versus late-onset Alzheimer’s disease: more than age alone. J. Alzheimers Dis. 19, 1401–1408. doi: 10.3233/JAD-2010-1337
Koss, E., Edland, S., Fillenbaum, G., Mohs, R., Clark, C., Galasko, D., et al. (1996). Clinical and neuropsychological differences between patients with earlier and later onset of Alzheimer’s disease: a CERAD analysis, Part XII. Neurology 46, 136–141. doi: 10.1212/WNL.46.1.136
Lakmache, Y., Lassonde, M., Gauthier, S., Frigon, J. Y., and Lepore, F. (1998). Interhemispheric disconnection syndrome in Alzheimer’s disease. Proc. Natl. Acad. Sci. U S A 95, 9042–9046. doi: 10.1073/pnas.95.15.9042
Lehmann, M., Madison, C., Ghosh, P. M., Miller, Z. A., Greicius, M. D., Kramer, J. H., et al. (2015). Loss of functional connectivity is greater outside the default mode network in nonfamilial early-onset Alzheimer’s disease variants. Neurobiol. Aging 36, 2678–2686. doi: 10.1016/j.neurobiolaging.2015.06.029
Li, H., Liang, Y., Chen, K., Li, X., Shu, N., Zhang, Z., et al. (2013). Different patterns of white matter disruption among amnestic mild cognitive impairment subtypes: relationship with neuropsychological performance. J. Alzheimers Dis. 36, 365–376. doi: 10.3233/JAD-122023
Liu, Y., Spulber, G., Lehtimäki, K. K., Könönen, M., Hallikainen, I., Gröhn, H., et al. (2011). Diffusion tensor imaging and tract-based spatial statistics in Alzheimer’s disease and mild cognitive impairment. Neurobiol. Aging 32, 1558–1571. doi: 10.1016/j.neurobiolaging.2009.10.006
Liu, Y., Yu, C., Zhang, X., Liu, J., Duan, Y., Alexander-Bloch, A. F., et al. (2014). Impaired long distance functional connectivity and weighted network architecture in Alzheimer’s disease. Cereb. Cortex 24, 1422–1435. doi: 10.1093/cercor/bhs410
Luo, X., Qiu, T., Xu, X., Huang, P., Gu, Q., Shen, Z., et al. (2016). Decreased inter-hemispheric functional connectivity in cognitively intact elderly APOE ε4 carriers: a preliminary study. J. Alzheimers Dis. 50, 1137–1148. doi: 10.3233/JAD-150989
Möller, C., Vrenken, H., Jiskoot, L., Versteeg, A., Barkhof, F., Scheltens, P., et al. (2013). Different patterns of gray matter atrophy in early- and late-onset Alzheimer’s disease. Neurobiol. Aging 34, 2014–2022. doi: 10.1016/j.neurobiolaging.2013.02.013
McKhann, G., Drachman, D., Folstein, M., Katzman, R., Price, D., and Stadlan, E. M. (1984). Clinical diagnosis of Alzheimer’s disease: report of the NINCDS-ADRDA work group under the auspices of department of health and human services task force on Alzheimer’s disease. Neurology 34, 939–944. doi: 10.1212/WNL.34.7.939
Migliaccio, R. F. A., Possin, K. L., Canu, E., Filippi, M., Rabinovici, G. D., Rosen, H. J., et al. (2015). Mapping the progression of atrophy in early and late onset Alzheimer’s disease. J. Alzheimers Dis. 46, 351–364. doi: 10.3233/JAD-142292
Ossenkoppele, R., Zwan, M. D., Tolboom, N., van Assema, D. M., Adriaanse, S. F., Kloet, R. W., et al. (2012). Amyloid burden and metabolic function in early-onset Alzheimer’s disease: parietal lobe involvement. Brain 135, 2115–2125. doi: 10.1093/brain/aws113
Reid, A. T., and Evans, A. C. (2013). Structural networks in Alzheimer’s disease. Eur. Neuropsychopharmacol. 23, 63–77. doi: 10.1016/j.euroneuro.2012.11.010
Sakamoto, S., Ishii, K., Sasaki, M., Hosaka, K., Mori, T., Matsui, M., et al. (2002). Differences in cerebral metabolic impairment between early and late onset types of Alzheimer’s disease. J. Neurol. Sci. 200, 27–32. doi: 10.1016/s0022-510x(02)00114-4
Seeley, W. W., Menon, V., Schatzberg, A. F., Keller, J., Glover, G. H., Kenna, H., et al. (2007). Dissociable intrinsic connectivity networks for salience processing and executive control. J. Neurosci. 27, 2349–2356. doi: 10.1523/JNEUROSCI.5587-06.2007
Shiino, A., Watanabe, T., Kitagawa, T., Kotani, E., Takahashi, J., Morikawa, S., et al. (2008). Different atrophic patterns in early- and late-onset Alzheimer’s disease and evaluation of clinical utility of a method of regional z-score analysis using voxel-based morphometry. Dement. Geriatr. Cogn. Disord. 26, 175–186. doi: 10.1159/000151241
Silk, T. J., Vance, A., Rinehart, N., Bradshaw, J. L., and Cunnington, R. (2009). White-matter abnormalities in attention deficit hyperactivity disorder: a diffusion tensor imaging study. Hum. Brain Mapp. 30, 2757–2765. doi: 10.1002/hbm.20703
Smith, S. M., Jenkinson, M., Johansen-Berg, H., Rueckert, D., Nichols, T. E., Mackay, C. E., et al. (2006). Tract-based spatial statistics: voxelwise analysis of multi-subject diffusion data. Neuroimage 31, 1487–1505. doi: 10.1016/j.neuroimage.2006.02.024
Smith, S. M., and Nichols, T. E. (2009). Threshold-free cluster enhancement: addressing problems of smoothing, threshold dependence and localisation in cluster inference. Neuroimage 44, 83–98. doi: 10.1016/j.neuroimage.2008.03.061
Smits, L. L., Pijnenburg, Y. A., Koedam, E. L., van der Vlies, A. E., Reuling, I. E., Koene, T., et al. (2012). Early onset Alzheimer’s disease is associated with a distinct neuropsychological profile. J. Alzheimers Dis. 30, 101–108. doi: 10.3233/JAD-2012-111934
Stopford, C. L., Snowden, J. S., Thompson, J. C., and Neary, D. (2008). Variability in cognitive presentation of Alzheimer’s disease. Cortex 44, 185–195. doi: 10.1016/j.cortex.2005.11.002
Suzuki, W. A. (1996). Neuroanatomy of the monkey entorhinal, perirhinal and parahippocampal cortices: organization of cortical inputs and interconnections with amygdala and striatum. Semin. Neurosci. 8, 3–12. doi: 10.1006/smns.1996.0002
Suzuki, W. A., and Amaral, D. C. (1994). The perirhinal and parahippocampal corrices of the macaque monkey: cortical afferenta. J. Comp. Neurol. 350, 497–533. doi: 10.1002/cne.903500402
Toyota, Y., Ikeda, M., Shinagawa, S., Matsumoto, T., Matsumoto, N., Hokoishi, K., et al. (2007). Comparison of behavioral and psychological symptoms in early-onset and late-onset Alzheimer’s disease. Int. J. Geriatr. Psychiatry 22, 896–901. doi: 10.1002/gps.1760
Van Hoesen, G. W., Augustinack, J. C., Dierking, J., Redman, S. J., and Thangavel, R. (2000). The parahippocampal gyrus in Alzheimer’s disease. Clinical and preclinical neuroanatomical correlates. Ann. N Y Acad. Sci. 911, 254–274. doi: 10.1111/j.1749-6632.2000.tb06731.x
Verclytte, S., Lopes, R., Lenfant, P., Rollin, A., Semah, F., Leclerc, X., et al. (2016). Cerebral hypoperfusion and hypometabolism detected by arterial spin labeling MRI and FDG-PET in early-onset Alzheimer’s disease. J. Neuroimaging 26, 207–212. doi: 10.1111/jon.12264
Wakana, S., Jiang, H., Nagae-Poetscher, L. M., van Zijl, P. C., and Mori, S. (2004). Fiber tract-based atlas of human white matter anatomy. Radiology 230, 77–87. doi: 10.1148/radiol.2301021640
Wang, P. J., Saykin, A. J., Flashman, L. A., Wishart, H. A., Rabin, L. A., Santulli, R. B., et al. (2006). Regionally specific atrophy of the corpus callosum in AD, MCI and cognitive complaints. Neurobiol. Aging 27, 1613–1617. doi: 10.1016/j.neurobiolaging.2005.09.035
Wang, Z., Wang, J., Zhang, H., McHugh, R., Sun, X., Li, K., et al. (2015). Interhemispheric functional and structural disconnection in Alzheimer’s disease: a combined resting-state fMRI and DTI study. PLoS One 10:e0126310. doi: 10.1371/journal.pone.0126310
Yan, S. D., Chen, X., Fu, J., Chen, M., Zhu, H., Roher, A., et al. (1996). RAGE and amyloid-β peptide neurotoxicity in Alzheimer’s disease. Nature 382, 685–691. doi: 10.1038/382685a0
Yan, C. G., Cheung, B., Kelly, C., Colcombe, S., Craddock, R. C., Di Martino, A., et al. (2013). A comprehensive assessment of regional variation in the impact of head micromovements on functional connectomics. Neuroimage 76, 183–201. doi: 10.1016/j.neuroimage.2013.03.004
Keywords: early-onset Alzheimer’s disease, resting-state functional MRI, voxel-mirrored homotopic connectivity, diffusion tensor imaging, corpus callosum, gray matter volume
Citation: Li K-C, Luo X, Zeng Q-Z, Xu X-J, Huang P-Y, Shen Z-J, Xu J-J, Zhou J and Zhang M-M (2018) Distinct Patterns of Interhemispheric Connectivity in Patients With Early- and Late-Onset Alzheimer’s Disease. Front. Aging Neurosci. 10:261. doi: 10.3389/fnagi.2018.00261
Received: 20 March 2018; Accepted: 14 August 2018;
Published: 06 September 2018.
Edited by:
Guido Gainotti, Università Cattolica del Sacro Cuore, ItalyReviewed by:
Panteleimon Giannakopoulos, Université de Genève, SwitzerlandYing Han, Capital Medical University, China
Copyright © 2018 Li, Luo, Zeng, Xu, Huang, Shen, Xu, Zhou and Zhang. This is an open-access article distributed under the terms of the Creative Commons Attribution License (CC BY). The use, distribution or reproduction in other forums is permitted, provided the original author(s) and the copyright owner(s) are credited and that the original publication in this journal is cited, in accordance with accepted academic practice. No use, distribution or reproduction is permitted which does not comply with these terms.
*Correspondence: Min-Ming Zhang, emhhbmdtaW5taW5nQHpqdS5lZHUuY24=
† These authors have contributed equally to this work