- 1Department of Neurology, Institute of Neurology, Ruijin Hospital, Shanghai Jiao Tong University School of Medicine, Shanghai, China
- 2Department of Neurology, Ruijin Hospital, Luwan Branch, Shanghai Jiao Tong University School of Medicine, Shanghai, China
Objectives: This study aimed to explore the relationship between 18 single nucleotide polymorphisms (SNPs) and Alzheimer’s disease (AD) within the southern Chinese population.
Methods: A total of 420 participants, consisting of 215 AD patients and 205 sex- and age-matched controls, were recruited. The SNaPshot technique and polymer chain reaction (PCR) were used to detect the 18 SNPs. Combined with the apolipoprotein E (APOE) ε4 allele and age at onset, we performed an association analysis between these SNPs and AD susceptibility. Furthermore, we analyzed SNP-associated gene expression using the expression quantitative trait loci analysis.
Results: Our study found that rs17125924 of FERMT2 was associated with the risk of developing AD in the dominant (P = 0.022, odds ratio [OR] = 1.57, 95% confidence interval [CI]: 1.07–2.32) and overdominant (P = 0.005, OR = 1.76, 95% CI: 1.18–2.61) models. Moreover, compared with APOE ε4 non-carriers, the frequency of the G-allele at rs17125924 was significantly higher among AD patients in APOE ε4 allele carriers (P = 0.029). The rs9271058 of HLA-DRB1 (dominant, overdominant, and additive models), rs9473117 of CD2AP (dominant and additive models), and rs73223431 of PTK2B (dominant, overdominant, and additive models) were associated with early onset AD (EOAD). Using the genotype-tissue expression (GTEx) and Braineac database, we found a significant association between rs9271058 genotypes and HLA-DRB1 expression levels, while the CC genotype at rs9473117 and the TT genotype of rs73223431 increased CD2AP and PTK2B gene expression, respectively.
Conclusion: Our study identifies the G-allele at rs17125924 as a risk factor for developing AD, especially in APOE ε4 carriers. In addition, we found that rs9271058 of HLA-DRB1, rs9473117 of CD2AP, and rs73223431 of PTK2B were associated with EOAD. Further studies with larger sample sizes are needed to confirm our results.
Introduction
Alzheimer’s disease (AD), characterized by progressive memory loss and behavioral changes, accounts for two-thirds of dementia cases, posing a significant burden on the affected families and society (Fransquet et al., 2018). While familial AD reported cases are few, almost 95% of the cases are sporadic (Masters et al., 2015). The etiology of sporadic AD remains unclear, but it is likely caused by a combination of genetic and environmental risk factors (Blennow et al., 2006; Dorszewska et al., 2016). Recently, an increasing number of studies have focused on the heritability of AD, as the classical amyloid hypothesis insufficiently explains the pathogenesis of AD (Hardy, 1997; Gatz et al., 2006). The identification of AD-susceptibility loci may, therefore, provide the basis for a helpful and complementary method for the timely and reliable diagnosis of this disease (Belcavello et al., 2015). Over the past few decades, genome-wide association studies (GWAS), which overcome sample size limitations, were taken advantage of to study genetic changes that may contribute to AD. Meta-analyses of GWAS in populations of European ancestry have identified multiple susceptibility genes associated with AD, such as CLU, CR1, MS4A4, CD2AP, CD33, and EPHA1 (Lambert et al., 2009; Naj et al., 2011). In East Asian studies, no significant differences were observed in the genotype and estimated allele frequency distribution of single nucleotide polymorphisms (SNPs) within ABCA7, CD2AP, and EPHA1 (Tan et al., 2013), suggesting that the genetic basis for AD susceptibility may be ethnicity-specific. Our previous study found that rs3865444 of CD33 and rs610932 of MS4A6A may contribute to AD risk in the Chinese Han population, which was in line with the findings of preceding GWAS studies (Deng et al., 2012).
Early onset AD (EOAD), with onset in individuals younger than 65 years, differs from late-onset AD (LOAD) in that-although genetic factors play an important role in both types-they are influenced by different susceptibility genes (Jiang et al., 2013; Tellechea et al., 2018). Approximately 10% of EOAD cases are familial in nature, based on autosomal dominant variants in the genes encoding amyloid precursor protein (APP), presenilin 1 (PSEN1), and presenilin 2 (PSEN2) (Kunkle et al., 2017). The remaining early onset sporadic cases are thought to be predominantly polygenic variants, the accumulation of which can result in EOAD at an early stage of life (Barber et al., 2017). The apolipoprotein E (APOE) ε4 allele, which plays a vital role in liquid absorption and redistribution, is regarded as the strongest genetic risk factor for LOAD (Liu et al., 2013; Qiu et al., 2019).
Recently, a GWAS meta-analysis of non-Hispanic whites confirmed 20 previously described LOAD risk loci and proposed 5 such novel loci including IQCK, ACE, ADAM10, ADAMTS1, and WWOX (Kunkle et al., 2019). In addition, 9 new susceptibility loci for AD have also been identified in a GWAS meta-analysis in individuals of European descent (Jansen et al., 2019). Repeating the GWAS results in different ethnic populations can aid in identifying the SNPs associated with AD (Chanock et al., 2007). To the best of our knowledge, due to varying allele frequencies between different races, the association of these candidate loci reported in the above GWAS with AD in the Chinese population is not known. Therefore, in this study, we selected 18 SNPs (FERMT2 rs17125924, HLA-DRB1 rs9271058 and rs6931277, CD2AP rs9473117, APH1B rs35408871, NDUFAF6 rs4735340, ADAMTS20 rs7295246, EPHA1 rs10808026 and rs11763230, ADAM10 rs593742 and rs442495, INPP5D rs10933431, PTK2B rs73223431, CR1 rs2093760, MS4A6A rs7935829, CLNK rs6448451, CD2AP-TNFRSF21 rs9381563, CLU rs4236673) from aforementioned studies, aiming to explore the relationship between these genes and AD risk in the southern Chinese population. Moreover, we attempted to assess the potential effect of these loci on gene expression using multiple expression quantitative trait loci (eQTL) datasets.
Materials and Methods
Study Population
From September 2016 to March 2019, a total of 215 patients with AD (135 women and 80 men, mean age at onset ± SD: 71.95 ± 8.46 years) were recruited from the outpatient clinic at the Department of Neurology, Ruijin Hospital, affiliated to Shanghai Jiao Tong University School of Medicine, China. All enrolled subjects were evaluated by at least two experienced neurologists and underwent a standard series of examinations, including medical history, physical examinations, as well as neuropsychological and neuroimaging tests. The results met the criteria for probable AD defined by National Institute of Neurological and Communicative Disorders and Stroke–Alzheimer’s Disease and Related Disorders Association (NINCDS-ADRDA criteria) (Dubois et al., 2007). Participants with a history of other neurological diseases that may cause dementia, such as stroke, Parkinson’s disease, brain tumor, multiple sclerosis, and major depression, were excluded (McKhann et al., 2011; Janelidze et al., 2018). The age at the onset of the disease was determined by the medical history provided by the caregiver. The control group consisted of 205 healthy volunteers matched for sex and age (121 women and 84 men, mean age ± SD: 70.74 ± 7.82 years). Healthy subjects were carefully assessed by a physician to confirm the absence of cognitive decline symptoms, thus ensuring they did not fulfill the criteria of mild cognitive impairment (MCI) or dementia (McKhann et al., 2011; Jack et al., 2018). This study was approved by the Ethics Committee of the Ruijin Hospital affiliated to the Shanghai Jiao Tong University School of Medicine (2018-No.6).
Genotype Analysis
The genomic DNA was extracted using the phenol-chloroform-isopropyl alcohol method from 2 mL of blood collected in EDTA anti-coagulation tubes. The SNaPshot technique (Applied Biosystems, Foster City, CA, United States) was used to genotype SNPs. Polymer chain reaction (PCR) and extension primers were designed using the Primer5 software (version 5.00, PREMIER Biosoft International). The length of PCR fragments ranged from 80 to 240 bp. The PCR products were purified by phosphorylase (FastAP, Applied Biosystems) and exonuclease I (EXO I, Applied Biosystems) and subsequently extended using the ABI SNaPshot Multiplex kit (Applied Biosystems). The extension product was purified by FastAP and loaded on ABI3730xl (Applied Biosystems). The results were analyzed using GeneMapper 4.0 (Applied Biosystems). Primer sequences are listed in Supplementary Table 1. The SNPs in APOE (rs429358 and rs7412) were genotyped by the polymerase chain reaction–restriction fragment length polymorphism (PCR–RFLP) method as previously described (Corder et al., 1993).
Gene Expression Analysis
Expression quantitative trait loci were examined using two different databases. The Braineac eQTL dataset is a public database developed by the United Kingdom Brain Expression Consortium (UKBEC), which includes 10 brain regions from 134 postmortem individuals of European descent without neurodegenerative disorders (Ramasamy et al., 2014). The GTEx project has collected genotypes and gene expression data from 54 non-diseased tissue sites across nearly 1,000 individuals1.
Statistical Analyses
All statistical analyses were performed using the SPSS 25.0 software package (SPSS Inc., Chicago, IL, United States). Differences in age, level of education and Mini−Mental State Examination (MMSE) scores between the two groups were examined by t-test. Chi-square test was used to compare the differences in sex proportions and APOE status, as well as in allele and genotype frequencies. The Hardy-Weinberg equilibrium (HWE) of the entire cohort was also tested using the chi-square test. The risk of each SNP was estimated using logistic regression analysis after adjusting for age and sex, and four genetic models including dominant, recessive, overdominant, and additive models were applied. The following definitions were used assuming A represents the major allele and a represents the minor allele: dominant was defined as 1 (aa + Aa) versus 0 (AA); recessive as 1 (aa) versus 0 (AA + Aa); additive as 0 (AA) versus 1 (Aa) versus 2 (aa); and overdominant as 1 (Aa) versus 0 (AA + aa). P-values < 0.05 were considered statistically significant. Multiple tests were performed using the Bonferroni correction method. The genetic power of each SNP was calculated using Power and Sample Size software (version 3.1.6).
Results
The Study Population’s Demographic Characteristics
In this study, we analyzed 215 patients with AD and 205 age- and sex-matched healthy controls. Table 1 shows the main demographic and clinical information of these subjects. Compared to controls, AD patients were found to be less educated, which is consistent with the findings of previous studies (Xu et al., 2016; Larsson et al., 2017).
Association Analysis of SNPs With AD in Different Genetic Models
For all SNPs, genotype distributions were in HWE. The minimum allele and genotype frequencies of these SNPs are listed in Supplementary Table 2. No significant differences were found in the allele frequencies of those SNPs between AD patients and controls. In contrast, regarding SNP genotype frequencies, we found that, at rs1715924, the genotypes GG and GA conferred a higher risk for AD than the genotype AA (Table 2). The relationship between target SNP and AD risk was studied by four genetic models including dominant, recessive, overdominant, and additive model. Following adjustment for age and sex, only one SNP, rs17125924 of FERMT2, was associated with the risk of AD in the dominant (P = 0.022, odds ratio [OR] = 1.57, 95% confidence interval [CI]: 1.07–2.32) and overdominant (P = 0.005, OR = 1.76, 95% CI: 1.18–2.61) models (Table 3 and Supplementary Table 3). However, after Bonferroni correction, these associations did not persist. As expected, the APOE status increased the risk of disease (Table 1). We stratified these data by APOE ε4 status in order to find out whether this allele affects the relationship between SNPs and AD susceptibility. In APOE ε4 carriers, the allele and genotype frequencies of FERMT2 rs17125924 were significantly different between AD patients and controls (allele: P = 0.029, OR = 1.895, 95% CI: 1.06–3.38; genotype: P = 0.036), with allele G found to be higher in the case group than in the control (Table 2). After adjustment for age and sex, the dominant, overdominant and additive models of rs17125924 were associated with AD (dominant model: P = 0.009, OR = 2.739, 95% CI: 1.28–5.86; overdominant model: P = 0.015, OR = 2.66, 95% CI: 1.21–5.85; additive model: P = 0.03, OR = 2.022, 95% CI: 1.07–3.82) (Table 2). These associations could not be confirmed after Bonferroni correction. In APOE ε4 non-carriers, there were no significant differences in the allele frequency between AD patients and controls, but we observed a lower distribution of GG homozygosity at rs17125924 in the former group, suggesting a protective effect in the recessive model (Table 2).
Association Analysis of Subgroups Stratified by the Age of Onset
Subsequently, the AD patients were divided into two subgroups depending on whether the age of onset was below (EOAD) or above (LOAD) 65 years. Forty-nine (22.79%) of the AD patients had EOAD, 34 (69.39%) of which were women, while in LOAD patients, 101 (60.84%) were women. There were no discrepancies in sex between EOAD and LOAD patients (P = 0.277, chi-square test). We found that rs9271058 of HLA-DRB1 was associated with the risk of developing EOAD in allele and genotype frequencies (allele: P = 0.011, OR = 2.038, 95% CI: 1.17–3.56; genotype: P = 0.038). Another significant discrepancy was observed in the genotype frequencies of FERMT2 rs17125924 polymorphism (P = 0.024) (Supplementary Table 4). After adjustments for age and sex, rs9271058 of HLA-DRB1 and rs73223431 of PTK2B were associated with the risk of EOAD in the dominant, overdominant, and additive models (rs9271058: dominant model: P = 0.03, OR = 2.563, 95% CI: 1.10–5.99; overdominant model: P = 0.049, OR = 2.363, 95% CI: 1.01–5.56; additive model: P = 0.033, OR = 2.23, 95% CI: 1.07–4.68; rs73223431: dominant model: P = 0.007, OR = 3.108, 95% CI: 1.36–7.09; overdominant model: P = 0.027, OR = 2.523, 95% CI: 1.11–5.72; additive model: P = 0.008, OR = 2.438, 95% CI: 1.26–4.71). Additionally, rs9473117 of CD2AP was associated with the risk of developing EOAD in both the dominant and additive models (dominant model: P = 0.035, OR = 0.327, 95% CI: 0.12–0.93; additive model: P = 0.034, OR = 0.39, 95% CI: 0.16–0.93). The results also showed an association between rs35408871 of APH1B and EOAD in the recessive model (P = 0.047, OR = 0.36, 95% CI: 0.13–0.99). However, all statistically significant differences were eliminated after Bonferroni correction (Table 3 and Supplementary Table 5). With regard to LOAD, we only found that rs11763230 of EPHA1 may be associated with AD susceptibility using the recessive model (P = 0.046, OR = 0.111, 95% CI: 0.01–0.96) (Supplementary Tables 6, 7).
Association Analysis Between SNPs and Relevant Gene Expression in Normal Human Brain
In order to fully understand the influence of relevant loci on the onset of AD, we selected four candidate loci based on the above results and explored the association between genotype and gene expression in normal human brain by applying eQTL analysis. Using GTEx, the results show that the AA genotype of rs9271058 was associated with higher levels of HLA-DRB1 in brain regions including the cerebellum, caudate (basal ganglia), cortex, putamen (basal ganglia), nucleus accumbens (basal ganglia), frontal cortex (BA9), cerebellar hemisphere, anterior cingulate cortex (BA24), hypothalamus, amygdala, hippocampus, and spinal cord (cervical c-1) (Table 4). Using the Braineac database, the C-allele of rs9473117 increased CD2AP expression in thalamus (P = 4.6×10–4, with probe set 2,909,469) and cerebellar cortex (P = 7.9×10–4 with probe set 2,909,468). In addition, the TT genotype of rs73223431 enhanced PTK2B expression in temporal cortex (P = 9.9×10–5, with probe set 3,091,308) (Figure 1). No significant association was found between genotypes at rs17125924 and cerebral FERMT2 expression levels in GTEx and the Braineac database.
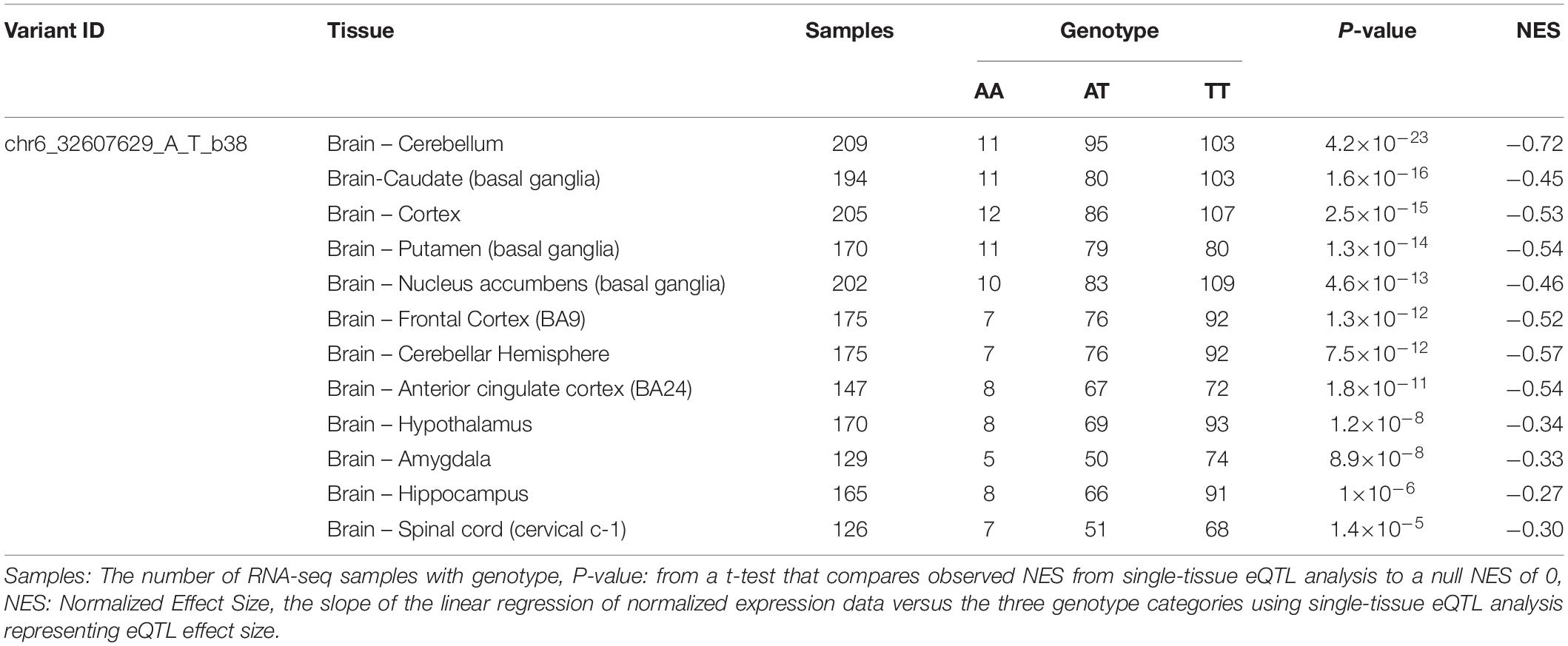
Table 4. Effect of rs9271058 on HLA-DRB1 gene expression in different regions of normal human brain in GTEx.
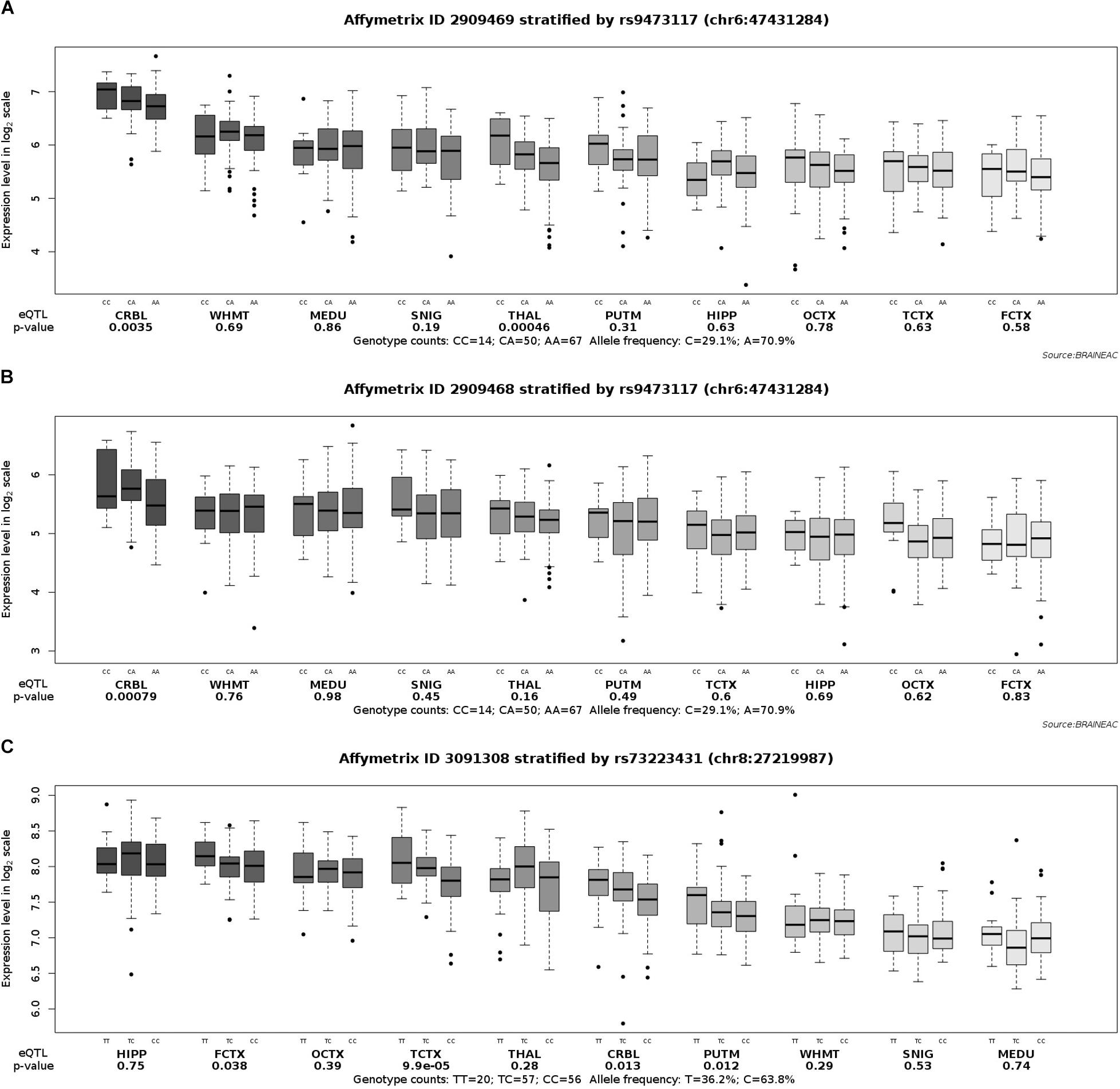
Figure 1. Effect of rs9473117 and rs73223431 on CD2AP and PTK2B expression in normal human brain regions in Braineac. (A) C allele of rs9473117 increased CD2AP expression in thalamus with probe set 2,909,469 (P = 4.6×10–4), (B) C allele of rs9473117 increased CD2AP expression in cerebellar cortex with probe set 2,909,468 (P = 7.9×10–4), (C) Carriers of T allele and especially TT genotype have more pronounced increased of PTK2B expression in temporal cortex with probe set 3,091,308 (P = 9.9×10–5).
Discussion
In this study, we validated an association between the rs17125924 of FERMT2 and the risk of AD in the southern Chinese population. When the allele and genotype distribution were stratified by APOE ε4 status, the discrepancy was even more significant in APOE ε4 carriers. Individuals with a homozygous GG-allele at rs17125924 were found to have a higher susceptibility to AD. In addition, when the patients were divided into two groups according to the age at the onset of AD (EOAD and LOAD), we observed that rs9271058 of HLA-DRB1 (dominant, overdominant, and additive models), rs9473117 of CD2AP (dominant and additive models), and rs73223431 of PTK2B (dominant, overdominant, and additive models) were possibly associated with EOAD. There was a tendency of rs35408871 within APH1B to be linked to the risk of EOAD in the recessive model. However, rs11763230 of EPHA1 was the sole loci amongst our chosen SNPs that may contribute to a risk of LOAD in the recessive model. The association of the remaining 12 SNPs with AD did not replicate in the southern Chinese population.
FERMT2, also known as kindlin-2, is important in integrin activation and cell-cell adhesion (Lai-Cheong et al., 2010). As previously reported, FERMT2 silencing leads to an increase in Aβ production, thus demonstrating its regulatory impact on APP metabolism (Chapuis et al., 2017). A new study found that FERMT2 expression in human neurons has effects on both Aβ and Tau (Sullivan et al., 2019). Knockdown of FERMT2 in these cells by either viral-mediated delivery of shRNA or genome editing resulted in a reduction in the extracellular Aβ40 and Aβ42 levels and a reduction in total and phospho-tau (Sullivan et al., 2019). A meta-analysis of GWAS in individuals of European ancestry identified rs17125944 of FERMT2 as a risk factor for LOAD, even though this was inconsistent in different ethnic groups (Lambert et al., 2013). Zhang et al. (2016) could not corroborate this association in the northern Han Chinese population. No studies have yet been conducted to assess the relationship between SNP rs17125924 within the FERMT2 gene and AD susceptibility in the Asian population. We provide evidence that the G-allele at rs17125924 located within the intronic regions of FERMT2 is a risk factor for AD and is particularly prominent in APOE ε4 carriers.
Our findings suggest that rs9271058, which is located at 17793 base pairs upstream of the transcription start point of the HLA-DRB1 gene, is associated with the risk of developing EOAD. HLA-DRB1 is a member of major histocompatibility complex Class II (MHCII), which is associated with autoimmune and infectious diseases and is a key player in the regulation of numerous immune responses (Price et al., 1999; Trowsdale and Knight, 2013). Studies have shown that immune activation and inflammation exacerbate the process of neurodegeneration in the brains of AD patients (Serpente et al., 2014; Shadfar et al., 2015). According to a GWAS-based meta-analysis, rs9271192 of HLA-DRB1 was identified as a novel susceptibility locus associated with AD in Caucasians (Lambert et al., 2013). Furthermore, Tan et al. found that the C-allele at rs9271192 may contribute to LOAD risk in the northern Chinese population (Lu et al., 2017). The present study provides supporting evidence for the association between rs9271058 and EOAD, which should be further validated in future studies.
To date, it remains unknown whether rs9473117, which is located at 14198 base pairs downstream of the transcription start point of CD2AP, is related to the risk of AD in the Chinese population. CD2-associated protein (CD2AP) is an adaptor protein that is expressed in brain capillaries (Li et al., 2000) and binds to cortactin, a protein involved in the regulation of receptor-mediated endocytosis (Lynch et al., 2003). Case-control studies of the southern Chinese population found the C-allele at rs9296559 of CD2AP to be associated with the risk of sporadic AD and suggested decreased expression of CD2AP in peripheral blood lymphocytes of AD patients as a potential biomarker (Tao et al., 2017). In a meta-analysis of East Asian, American, Canadian, and European populations, the polymorphism of rs9349407 within CD2AP was shown to contribute to AD susceptibility (Chen et al., 2015). Our results demonstrated that rs9473117 near CD2AP is likely to be associated with EOAD, indicating that this locus may affect the onset of the disease. However, as the EOAD population included in our study was small, these observations require confirmation with larger sample sizes.
The PTK2B gene, which is highly expressed in the central nervous system, encodes a cytoplasmic protein tyrosine kinase (Lev et al., 1995). The interaction between PTK2B and hyperphosphorylated and oligomeric Tau in the brain is involved in pathophysiological processes of AD (Dourlen et al., 2017). In a mouse model of AD, the levels of PTK2B Tyr-402 phosphorylation were shown to be reduced in the hippocampus, and the behavioral and molecular phenotype could be rescued by PTK2B overexpression (Giralt et al., 2018). Although the rs28834970 of PTK2B has been identified to be a genetic contributor to the susceptibility of LOAD in several studies (Lambert et al., 2013; Jiao et al., 2015), no studies, to date, have investigated whether this genetic polymorphism is associated with EOAD. Thus, our study is the first to suggest a link between a PTK2B SNP and the risk of developing EOAD, which is demonstrated by the T-allele at rs73223431.
Both rs35408871 of APH1B and rs11763230 of EPHA1 are intronic. The anterior pharynx-defective 1B (APH1B) is one of the four core subunits of the γ-secretase complex (Yonemura et al., 2016). EPHA1, located on chromosome 7q34.1, is a member of the ephrin family of tyrosine kinase receptors that play a role in cell morphology and motility, as well as in synaptic plasticity (Martinez et al., 2005). In addition, rs11767557 and rs11771145 of EPHA1 have been associated with reduced LOAD risk (Lambert et al., 2013; Karch and Goate, 2015). In our study, we found that homozygosity for the G-allele at rs35408871 may have a protective effect on EOAD susceptibility, whereas homozygosity for the T-allele at rs11763230 may protect from LOAD susceptibility.
A GWAS meta-analysis has identified the polymorphisms rs593742 and rs442495 within ADAM10 as novel risk loci associated with AD (Jansen et al., 2019; Kunkle et al., 2019). Our examination failed to replicate the association between these genetic loci and the risk for AD in the Chinese population, which may be explained by the heterogeneity of ethnic origin and/or small sample size. Also, we aimed to investigate the association of candidate loci with the age at the onset of AD and confirmed that the candidate genes including HLA-DRB1, CD2AP, and PTK2B may contribute to the development of early onset dementia. Prior studies have demonstrated that many eQTL influence the expression of local transcripts and distant genes (Battle et al., 2014). Application of eQTL database allows us to better understand human gene expression and its relationship to genetic variation, which provides a vital opportunity to explore potential functional impact for AD. Using the GTEx database, we further corroborated that the polymorphism of rs9271058 was associated with HLA-DRB1 expression levels in several brain regions, which is consistent with the relevance between HLA-DRB1 and the pathogenesis of AD in previous GWAS results (Lambert et al., 2013; Wang et al., 2017). The differences of DNA methylation and transcriptional regulation between brain regions can significantly influence the gene expression (Kang et al., 2011). In addition, using the Braineac database, we found that the genotypes of rs9473117 and rs73223431 affected CD2AP and PTK2B gene expression, respectively. Collectively, these data prove that polymorphism at susceptible loci may affect gene expression and accelerate the onset of AD. Positive eQTL associations can illustrate the validity of this analysis to understand the roles of risk variants in disease.
This study has some limitations, the most important one being the small sample size because of its single-centered nature. In the future, we plan to perform a meta-analysis on the Asian population with an increased sample size to confirm our results. Moreover, we did not include all the novel genome-wide loci identified in the recent GWAS in our study (Jansen et al., 2019; Kunkle et al., 2019), such as IQCK, ACE, and WWOX, because the minor allele frequencies of these SNPs were rare. In follow-up studies, more candidate loci for AD susceptibility should be assessed. Meanwhile, regular follow up with the patients included in this study will be conducted to observe the cognitive changes and to explore the relationship between genetic polymorphisms and clinical progression.
Conclusion
In conclusion, the SNP rs1715924 of FERMT2 was associated with AD in the southern Chinese population, with a particularly significant risk in APOE ε4 carriers. This suggests that this polymorphism may interact with APOE to increase the AD risk in a Han Chinese population. The SNPs rs9271058 of HLA-DRB1, rs9473117 of CD2AP, and rs73223431 of PTK2B were found to be associated with EOAD. Considering the association between these loci and AD risk as demonstrated in here, investigating the roles of risk genes in AD pathogenesis is critical in future studies.
Data Availability Statement
The datasets analyzed in this study can be found in the NCBI dbSNP Short Genetic Variation Database: https://www.ncbi.nlm.nih.gov/SNP/snp_viewBatch.cgi?sbid=106 3084.
Ethics Statement
The studies involving human participants were reviewed and approved by the Ethics Committee of the Ruijin Hospital affiliated to the Shanghai Jiao Tong University School of Medicine. The patients/participants provided their written informed consent to participate in this study.
Author Contributions
YY collected AD and control data, performed the statistical analysis, and drafted the manuscript. AZ, YQ, and RY helped to collect the AD data. YL, WX, and YW helped to collect the control data. YD designed the study and revised the manuscript.
Funding
This study was supported by grants from the National Natural Science Foundation of China (81571029) and the Natural Science Foundation of Shanghai (19ZR1432500) to YD.
Conflict of Interest
The authors declare that the research was conducted in the absence of any commercial or financial relationships that could be construed as a potential conflict of interest.
Acknowledgments
We thank all the patients and healthy controls who participated in this study.
Supplementary Material
The Supplementary Material for this article can be found online at: https://www.frontiersin.org/articles/10.3389/fnagi.2020.00016/full#supplementary-material
Footnotes
References
Barber, I. S., Braae, A., Clement, N., Patel, T., Guetta-Baranes, T., Brookes, K., et al. (2017). Mutation analysis of sporadic early-onset Alzheimer’s disease using the NeuroX array. Neurobiol. Aging 49, 215.e1–215.e8. doi: 10.1016/j.neurobiolaging.2016.09.008
Battle, A., Mostafavi, S., Zhu, X., Potash, J. B., Weissman, M. M., McCormick, C., et al. (2014). Characterizing the genetic basis of transcriptome diversity through RNA-sequencing of 922 individuals. Genome Res. 24, 14–24. doi: 10.1101/gr.155192.113
Belcavello, L., Camporez, D., Almeida, L. D., Morelato, R. L., Batitucci, M. C., and de Paula, F. (2015). Association of MTHFR and PICALM polymorphisms with Alzheimer’s disease. Mol. Biol. Rep. 42, 611–616. doi: 10.1007/s11033-014-3806-1
Blennow, K., de Leon, M. J., and Zetterberg, H. (2006). Alzheimer’s disease. Lancet 368, 387–403. doi: 10.1016/s0140-6736(06)69113-7
Chanock, S. J., Manolio, T., Boehnke, M., Boerwinkle, E., Hunter, D. J., Thomas, G., et al. (2007). Replicating genotype-phenotype associations. Nature 447, 655–660. doi: 10.1038/447655a
Chapuis, J., Flaig, A., Grenier-Boley, B., Eysert, F., Pottiez, V., Deloison, G., et al. (2017). Genome-wide, high-content siRNA screening identifies the Alzheimer’s genetic risk factor FERMT2 as a major modulator of APP metabolism. Acta Neuropathol. 133, 955–966. doi: 10.1007/s00401-016-1652-z
Chen, H., Wu, G., Jiang, Y., Feng, R., Liao, M., Zhang, L., et al. (2015). Analyzing 54,936 samples supports the association between CD2AP rs9349407 polymorphism and Alzheimer’s disease susceptibility. Mol. Neurobiol. 52, 1–7. doi: 10.1007/s12035-014-8834-2
Corder, E. H., Saunders, A. M., Strittmatter, W. J., Schmechel, D. E., Gaskell, P. C., Small, G. W., et al. (1993). Gene dose of apolipoprotein E type 4 allele and the risk of Alzheimer’s disease in late onset families. Science 261, 921–923. doi: 10.1126/science.8346443
Deng, Y. L., Liu, L. H., Wang, Y., Tang, H. D., Ren, R. J., Xu, W., et al. (2012). The prevalence of CD33 and MS4A6A variant in Chinese Han population with Alzheimer’s disease. Hum. Genet. 131, 1245–1249. doi: 10.1007/s00439-012-1154-6
Dorszewska, J., Prendecki, M., Oczkowska, A., Dezor, M., and Kozubski, W. (2016). Molecular basis of familial and sporadic Alzheimer’s disease. Curr. Alzheimer Res. 13, 952–963. doi: 10.2174/1567205013666160314150501
Dourlen, P., Fernandez-Gomez, F. J., Dupont, C., Grenier-Boley, B., Bellenguez, C., Obriot, H., et al. (2017). Functional screening of Alzheimer risk loci identifies PTK2B as an in vivo modulator and early marker of Tau pathology. Mol. Psychiatry 22, 874–883. doi: 10.1038/mp.2016.59
Dubois, B., Feldman, H. H., Jacova, C., Dekosky, S. T., Barberger-Gateau, P., Cummings, J., et al. (2007). Research criteria for the diagnosis of Alzheimer’s disease: revising the NINCDS-ADRDA criteria. Lancet Neurol. 6, 734–746. doi: 10.1016/s1474-4422(07)70178-3
Fransquet, P. D., Lacaze, P., Saffery, R., McNeil, J., Woods, R., and Ryan, J. (2018). Blood DNA methylation as a potential biomarker of dementia: a systematic review. Alzheimers Dement. 14, 81–103. doi: 10.1016/j.jalz.2017.10.002
Gatz, M., Reynolds, C. A., Fratiglioni, L., Johansson, B., Mortimer, J. A., Berg, S., et al. (2006). Role of genes and environments for explaining Alzheimer disease. Arch. Gen. Psychiatry 63, 168–174. doi: 10.1001/archpsyc.63.2.168
Giralt, A., de Pins, B., Cifuentes-Diaz, C., Lopez-Molina, L., Farah, A. T., Tible, M., et al. (2018). PTK2B/Pyk2 overexpression improves a mouse model of Alzheimer’s disease. Exp. Neurol. 307, 62–73. doi: 10.1016/j.expneurol.2018.05.020
Hardy, J. (1997). Amyloid, the presenilins and Alzheimer’s disease. Trends Neurosci. 20, 154–159. doi: 10.1016/s0166-2236(96)01030-2
Jack, C. R. Jr., Bennett, D. A., Blennow, K., Carrillo, M. C., Dunn, B., Haeberlein, S. B., et al. (2018). NIA-AA research framework: toward a biological definition of Alzheimer’s disease. Alzheimers Dement. 14, 535–562. doi: 10.1016/j.jalz.2018.02.018
Janelidze, S., Mattsson, N., Stomrud, E., Lindberg, O., Palmqvist, S., Zetterberg, H., et al. (2018). CSF biomarkers of neuroinflammation and cerebrovascular dysfunction in early Alzheimer disease. Neurology 91, e867–e877. doi: 10.1212/WNL.0000000000006082
Jansen, I. E., Savage, J. E., Watanabe, K., Bryois, J., Williams, D. M., Steinberg, S., et al. (2019). Genome-wide meta-analysis identifies new loci and functional pathways influencing Alzheimer’s disease risk. Nat. Genet. 51, 404–413. doi: 10.1038/s41588-018-0311-9
Jiang, T., Yu, J. T., Tian, Y., and Tan, L. (2013). Epidemiology and etiology of Alzheimer’s disease: from genetic to non-genetic factors. Curr. Alzheimer Res. 10, 852–867. doi: 10.2174/15672050113109990155
Jiao, B., Liu, X., Zhou, L., Wang, M. H., Zhou, Y., Xiao, T., et al. (2015). Polygenic analysis of late-onset Alzheimer’s disease from mainland China. PLoS One 10:e0144898. doi: 10.1371/journal.pone.0144898
Kang, H. J., Kawasawa, Y. I., Cheng, F., Zhu, Y., Xu, X., Li, M., et al. (2011). Spatio-temporal transcriptome of the human brain. Nature 478, 483–489. doi: 10.1038/nature10523
Karch, C. M., and Goate, A. M. (2015). Alzheimer’s disease risk genes and mechanisms of disease pathogenesis. Biol. Psychiatry 77, 43–51. doi: 10.1016/j.biopsych.2014.05.006
Kunkle, B. W., Grenier-Boley, B., Sims, R., Bis, J. C., Damotte, V., Naj, A. C., et al. (2019). Genetic meta-analysis of diagnosed Alzheimer’s disease identifies new risk loci and implicates Abeta, tau, immunity and lipid processing. Nat. Genet. 51, 414–430. doi: 10.1038/s41588-019-0358-2
Kunkle, B. W., Vardarajan, B. N., Naj, A. C., Whitehead, P. L., Rolati, S., Slifer, S., et al. (2017). Early-onset Alzheimer disease and candidate risk genes involved in endolysosomal transport. JAMA Neurol. 74, 1113–1122. doi: 10.1001/jamaneurol.2017.1518
Lai-Cheong, J. E., Parsons, M., and McGrath, J. A. (2010). The role of kindlins in cell biology and relevance to human disease. Int. J. Biochem. Cell Biol. 42, 595–603. doi: 10.1016/j.biocel.2009.10.015
Lambert, J. C., Heath, S., Even, G., Campion, D., Sleegers, K., Hiltunen, M., et al. (2009). Genome-wide association study identifies variants at CLU and CR1 associated with Alzheimer’s disease. Nat. Genet. 41, 1094–1099. doi: 10.1038/ng.439
Lambert, J. C., Ibrahim-Verbaas, C. A., Harold, D., Naj, A. C., Sims, R., Bellenguez, C., et al. (2013). Meta-analysis of 74,046 individuals identifies 11 new susceptibility loci for Alzheimer’s disease. Nat. Genet. 45, 1452–1458. doi: 10.1038/ng.2802
Larsson, S. C., Traylor, M., Malik, R., Dichgans, M., Burgess, S., and Markus, H. S. (2017). Modifiable pathways in Alzheimer’s disease: mendelian randomisation analysis. BMJ Clin. Res. 359:j5375. doi: 10.1136/bmj.j5375
Lev, S., Moreno, H., Martinez, R., Canoll, P., Peles, E., Musacchio, J. M., et al. (1995). Protein tyrosine kinase PYK2 involved in Ca(2+)-induced regulation of ion channel and MAP kinase functions. Nature 376, 737–745. doi: 10.1038/376737a0
Li, C., Ruotsalainen, V., Tryggvason, K., Shaw, A. S., and Miner, J. H. (2000). CD2AP is expressed with nephrin in developing podocytes and is found widely in mature kidney and elsewhere. Am. J. Physiol. Renal Physiol. 279, F785–F792. doi: 10.1152/ajprenal.2000.279.4.F785
Liu, C. C., Liu, C. C., Kanekiyo, T., Xu, H., and Bu, G. (2013). Apolipoprotein E and Alzheimer disease: risk, mechanisms and therapy. Nat. Rev. Neurol. 9, 106–118. doi: 10.1038/nrneurol.2012.263
Lu, R. C., Yang, W., Tan, L., Sun, F. R., Tan, M. S., Zhang, W., et al. (2017). Association of HLA-DRB1 polymorphism with Alzheimer’s disease: a replication and meta-analysis. Oncotarget 8, 93219–93226. doi: 10.18632/oncotarget.21479
Lynch, D. K., Winata, S. C., Lyons, R. J., Hughes, W. E., Lehrbach, G. M., Wasinger, V., et al. (2003). A cortactin-CD2-associated protein (CD2AP) complex provides a novel link between epidermal growth factor receptor endocytosis and the actin cytoskeleton. J. Biol. Chem. 278, 21805–21813. doi: 10.1074/jbc.M211407200
Martinez, A., Otal, R., Sieber, B. A., Ibanez, C., and Soriano, E. (2005). Disruption of ephrin-A/EphA binding alters synaptogenesis and neural connectivity in the hippocampus. Neuroscience 135, 451–461. doi: 10.1016/j.neuroscience.2005.06.052
Masters, C. L., Bateman, R., Blennow, K., Rowe, C. C., Sperling, R. A., and Cummings, J. L. (2015). Alzheimer’s disease. Nat. Rev. Dis. Primers 1:15056. doi: 10.1038/nrdp.2015.56
McKhann, G. M., Knopman, D. S., Chertkow, H., Hyman, B. T., Jack, C. R. Jr., Kawas, C. H., et al. (2011). The diagnosis of dementia due to Alzheimer’s disease: recommendations from the National Institute on Aging-Alzheimer’s Association workgroups on diagnostic guidelines for Alzheimer’s disease. Alzheimers Dement. 7, 263–269. doi: 10.1016/j.jalz.2011.03.005
Naj, A. C., Jun, G., Beecham, G. W., Wang, L. S., Vardarajan, B. N., Buros, J., et al. (2011). Common variants at MS4A4/MS4A6E, CD2AP, CD33 and EPHA1 are associated with late-onset Alzheimer’s disease. Nat. Genet. 43, 436–441. doi: 10.1038/ng.801
Price, P., Witt, C., Allcock, R., Sayer, D., Garlepp, M., Kok, C. C., et al. (1999). The genetic basis for the association of the 8.1 ancestral haplotype (A1, B8, DR3) with multiple immunopathological diseases. Immunol. Rev. 167, 257–274. doi: 10.1111/j.1600-065x.1999.tb01398.x
Qiu, Q., Lin, X., Sun, L., Zhu, M. J., Wang, T., Wang, J. H., et al. (2019). Cognitive decline is related to high blood glucose levels in older Chinese adults with the ApoE epsilon3/epsilon3 genotype. Transl. Neurodegener. 8:12. doi: 10.1186/s40035-019-0151-2
Ramasamy, A., Trabzuni, D., Guelfi, S., Varghese, V., Smith, C., Walker, R., et al. (2014). Genetic variability in the regulation of gene expression in ten regions of the human brain. Nat. Neurosci. 17, 1418–1428. doi: 10.1038/nn.3801
Serpente, M., Bonsi, R., Scarpini, E., and Galimberti, D. (2014). Innate immune system and inflammation in Alzheimer’s disease: from pathogenesis to treatment. Neuroimmunomodulation 21, 79–87. doi: 10.1159/000356529
Shadfar, S., Hwang, C. J., Lim, M. S., Choi, D. Y., and Hong, J. T. (2015). Involvement of inflammation in Alzheimer’s disease pathogenesis and therapeutic potential of anti-inflammatory agents. Arch. Pharm. Res. 38, 2106–2119. doi: 10.1007/s12272-015-0648-x
Sullivan, S. E., Liao, M., Smith, R. V., White, C., Lagomarsino, V. N., Xu, J., et al. (2019). Candidate-based screening via gene modulation in human neurons and astrocytes implicates FERMT2 in Abeta and TAU proteostasis. Hum. Mol. Genet. 28, 718–735. doi: 10.1093/hmg/ddy376
Tan, L., Yu, J. T., Zhang, W., Wu, Z. C., Zhang, Q., Liu, Q. Y., et al. (2013). Association of GWAS-linked loci with late-onset Alzheimer’s disease in a northern Han Chinese population. Alzheimers Dement. 9, 546–553. doi: 10.1016/j.jalz.2012.08.007
Tao, Q. Q., Liu, Z. J., Sun, Y. M., Li, H. L., Yang, P., Liu, D. S., et al. (2017). Decreased gene expression of CD2AP in Chinese patients with sporadic Alzheimer’s disease. Neurobiol. Aging 56, 212.e5–212.e10. doi: 10.1016/j.neurobiolaging.2017.03.013
Tellechea, P., Pujol, N., Esteve-Belloch, P., Echeveste, B., Garcia-Eulate, M. R., Arbizu, J., et al. (2018). Early- and late-onset Alzheimer disease: are they the same entity? Neurologia 33, 244–253. doi: 10.1016/j.nrl.2015.08.002
Trowsdale, J., and Knight, J. C. (2013). Major histocompatibility complex genomics and human disease. Annu. Rev. Genomics Hum. Genet. 14, 301–323. doi: 10.1146/annurev-genom-091212-153455
Wang, Z. X., Wang, H. F., Tan, L., Liu, J., Wan, Y., Sun, F. R., et al. (2017). Effects of HLA-DRB1/DQB1 genetic variants on neuroimaging in healthy, mild cognitive impairment, and Alzheimer’s disease cohorts. Mol. Neurobiol. 54, 3181–3188. doi: 10.1007/s12035-016-9890-6
Xu, W., Tan, L., Wang, H. F., Tan, M. S., Tan, L., Li, J. Q., et al. (2016). Education and risk of dementia: dose-response meta-analysis of prospective cohort studies. Mol. Neurobiol. 53, 3113–3123. doi: 10.1007/s12035-015-9211-5
Yonemura, Y., Futai, E., Yagishita, S., Kaether, C., and Ishiura, S. (2016). Specific combinations of presenilins and Aph1s affect the substrate specificity and activity of gamma-secretase. Biochem. Biophys. Res. Commun. 478, 1751–1757. doi: 10.1016/j.bbrc.2016.09.018
Keywords: Alzheimer’s disease, single nucleotide polymorphisms, FERMT2, HLA-DRB1, CD2AP, PTK2B
Citation: Yan Y, Zhao A, Qui Y, Li Y, Yan R, Wang Y, Xu W and Deng Y (2020) Genetic Association of FERMT2, HLA-DRB1, CD2AP, and PTK2B Polymorphisms With Alzheimer’s Disease Risk in the Southern Chinese Population. Front. Aging Neurosci. 12:16. doi: 10.3389/fnagi.2020.00016
Received: 13 October 2019; Accepted: 17 January 2020;
Published: 04 February 2020.
Edited by:
Changiz Geula, Northwestern University, United StatesReviewed by:
Murali Vijayan, Texas Tech University Health Sciences Center, United StatesRoberta Marongiu, Cornell University, United States
Copyright © 2020 Yan, Zhao, Qui, Li, Yan, Wang, Xu and Deng. This is an open-access article distributed under the terms of the Creative Commons Attribution License (CC BY). The use, distribution or reproduction in other forums is permitted, provided the original author(s) and the copyright owner(s) are credited and that the original publication in this journal is cited, in accordance with accepted academic practice. No use, distribution or reproduction is permitted which does not comply with these terms.
*Correspondence: Yulei Deng, ZHlsMTA3ODZAcmpoLmNvbS5jbg==