- Zhejiang Highway Technicians College, Hangzhou, China
Traditional manual detection of rural road surface distress is time-consuming and labor-intensive. In this paper, we propose a Mask R-CNN algorithm specifically designed for detecting rural road surface defects. To enhance precision and recall rates, data augmentation techniques—such as image translation, flipping, and noise perturbation—were applied to a dataset of 4,000 high-quality images of rural road pavement defects. This combination of Mask R-CNN with data augmentation is a novel approach that addresses the unique challenges of rural road distress detection. Experimental results demonstrate that data augmentation significantly improves recognition precision. The Mask R-CNN algorithm outperforms the ScNet algorithm in terms of precision for detecting and segmenting rural road defects. Among the various models and backbones tested within Mask R-CNN, the ResNeXt-101-FPN backbone achieved the highest precision and recall rates. Additionally, three field tests further validate the feasibility and reliability of the developed algorithm for rural road distress detection. The system, combining the Mask R-CNN algorithm with data augmentation, effectively distinguishes between varying levels of severity and classifies defects based on characteristics such as size, shape, and location. This enables maintenance crews to prioritize repairs more efficiently, resulting in significant improvements in road safety and durability.
1 Introduction
Rural road surface damage is a critical factor that restricts traffic flow and safety in rural areas. Each year, China invests substantial manpower and resources into the inspection and detection of rural road surfaces. In the past, these inspections were primarily carried out through manual visual assessments, a method that is not only highly subjective but also costly (Azimi et al., 2020). As the length of rural roads in China continues to grow, traditional manual inspection methods have proven inadequate to meet the increasing demand for efficient and accurate assessments. With advancements in modern technology, automated road surface damage detection systems, based on digital image processing and computer hardware and software, have developed rapidly (Qian, 2024; Hu and Ren, 2023). Early detection methods mostly relied on traditional image processing techniques, such as threshold segmentation, edge detection, and wavelet transforms (Du Z. Y. et al., 2021). However, these methods were limited to detecting specific types of damage in controlled scenarios, making them unsuitable for addressing the challenges of detecting multiple types of damage in complex and varied environments.
Existing vehicle-mounted road surface detection equipment utilizes image processing technology to analyze road images captured by onboard cameras, enhancing detection efficiency and ensuring operator safety by extracting image features. However, due to the distance between the camera and the road surface, the images obtained may lack sufficient clarity, often causing small road surface damages on rural roads to be overlooked during subsequent manual reviews. Moreover, vehicle-mounted detection equipment is expensive, and the complex and variable conditions of rural roads pose additional challenges and limitations. While laser scanning technology offers high detection accuracy, its high cost significantly limits its widespread adoption, making it impractical for large-scale rural road surface detection in the near future (Arya et al., 2021).
With the rapid development of artificial intelligence technology, deep learning has made significant strides in efficiently detecting and identifying road surface damage. Currently, object detection methods primarily utilize Convolutional Neural Networks (CNNs) and Transformer technology. For instance, Xiao et al. designed a novel hybrid window attention ViTransformer framework for road crack detection. This framework extracts feature semantics locally through dense windows and globally through sparse windows, significantly improving semantic detail and detection accuracy (Xiao et al., 2023). Wang et al. proposed a dual-path network for road crack segmentation that combines the strengths of CNNs and Transformers (Wang et al., 2024). Xu et al. introduced a Locally Enhanced Transformer Network (LETNet) for detecting cracks in road surface images, incorporating a convolutional backbone and a locally enhanced module to address the shortcomings of Transformers in capturing both low-level and high-level local features (Xu Z. et al., 2022). Transformers, with their self-attention and multi-head attention mechanisms, can capture long-distance dependencies within images while maintaining a global perspective. Although Transformer computations are highly complex, they generally perform better with objects that exhibit significant variations in texture, shape, and scale.
CNNs are highly efficient in feature extraction and classification of data such as images, audio, and text, utilizing multiple layers of convolution, pooling, fully connected layers, and activation functions. They can be categorized into single-stage and two-stage algorithms. Single-stage detection algorithms include the YOLO (You Only Look Once) series and the Single Shot MultiBox Detector (SSD).
Ma et al. proposed an improved YOLO v3 algorithm based on the Median Flow (MF) algorithm for road crack detection, achieving a best accuracy of 98.47% and an F1 score of 0.958 (Ma et al., 2022). Wang et al. introduced an improved YOLO v5 model for road damage detection, combining the model with the Vision Transformer (ViT) to compute attention weights for image regions and generate new feature maps based on these weights, demonstrating high precision and speed in detecting longitudinal, transverse, and fatigue cracks (Wang et al., 2023). Du et al. trained a YOLOv3 algorithm using images of road damage captured by vehicle-mounted industrial cameras under various weather and lighting conditions, optimizing model parameters to improve detection accuracy and speed (Du Y. et al., 2021). Malhar et al. proposed a pavement pothole detection solution based on the YOLOv8 algorithm, using deep learning methods to identify pavement depressions in real-time, enabling autonomous vehicles to avoid potential hazards and reduce accident risks (Khan et al., 2024). Wan et al. developed a lightweight road damage detection algorithm called YOLO-LRDD, incorporating the Shuffle-ECANet module to reduce model parameters. The model utilizes BiFPN to enhance multi-scale feature fusion and extraction, while the FocalEIOU loss function addresses sample imbalance (Wan et al., 2022). Yan et al. proposed a novel deformable SSD model by adding deformable convolution layers to the SSD’s VGG16 backbone feature extraction network. The model’s road damage detection accuracy was validated using the PASCAL VOC2007 dataset (Yan and Zhang, 2021). Nomura et al. used onboard camera images to assess crack propagation in concrete bridges, showing that incorporating image recognition processing with CNN learning after YOLO detection could improve the accuracy of YOLO (Nomura et al., 2022).
While YOLO series and SSD algorithms offer fast detection speeds, making them suitable for real-time applications, their accuracy in complex scenarios (such as complicated backgrounds, lighting shadows, and occlusions) and precise bounding box localization is often lower than that of two-stage algorithms like Fast R-CNN and Faster R-CNN (Xu X. et al., 2022). In pavement distress detection, insufficient bounding box precision can lead to misidentification and mislocalization of distress areas, potentially affecting subsequent maintenance efforts. Two-stage algorithms, such as Fast R-CNN (Girshick, 2015) and Faster R-CNN (Ren et al., 2017), first generate potential target areas (candidate boxes) through selective search or region proposal networks (RPN). In the second stage, fine classification and bounding box regression are performed to complete object detection. Ibragimov et al. applied Faster R-CNN to detect longitudinal, transverse, and alligator cracks in pavements and proposed a framework to apply Faster R-CNN technology to full-size pavement images, enabling the detection of large-size images (Ibragimov et al., 2022). Song et al. (Song and Wang, 2021) used Faster R-CNN for the automatic recognition and localization of pavement cracks, potholes, oil seepage, and surface repairs, comparing it with CNN and K-means classification methods. The results showed that Faster R-CNN could more accurately locate pavement damage with bounding boxes (Song and Wang, 2021). Kang et al. proposed a method for automatic crack detection and parameter quantification based on Faster R-CNN, utilizing different bounding boxes and an improved tubular flow field (TuFF) algorithm to segment crack pixels and measure crack width and length (He et al., 2017). In 2018, He et al. introduced the Mask R-CNN algorithm (He et al., 2016), adding a mask segmentation network to Faster R-CNN for pixel-level segmentation of targets. This enhancement addresses pixel misalignment issues caused by the detection branch, allowing simultaneous target localization and pixel segmentation. Although deep learning technologies have made significant progress in high-grade road distress detection, their direct application to rural road scenarios still faces challenges such as feature confusion of road distress and the loss of small target features. Therefore, it is crucial to develop a pavement distress detection algorithm framework tailored to the characteristics of rural roads.
In this paper, we aim to propose a rural road pavement distress detection algorithm for rural roads in China. Compared to general object detection algorithms like YOLO and SSD, Mask R-CNN, with its pixel-level instance segmentation capability, can achieve sub-centimeter-level crack localization in complex rural road scenarios. Its multi-task joint optimization mechanism ensures a higher recall rate for small targets. Meanwhile, the dual-branch feature decoupling network effectively distinguishes the cross-material feature differences between asphalt cracks and cement panel fractures, overcoming the feature confusion defect caused by SSD’s single-stage detection. Therefore, the Mask R-CNN algorithm was selected for this study. To enhance its performance, data augmentation techniques such as image translation, flipping, and noise perturbation were integrated. The use of these techniques not only improves image quality but also significantly enhances the precision and recall rates of defect recognition, making this approach particularly effective for monitoring rural roads. This combination of Mask R-CNN with data augmentation is a novel approach that addresses the unique challenges of rural road distress detection. The paper is organized as follows: Section 2 outlines the architecture of Mask R-CNN, dataset collection, data annotation, and data augmentation techniques. Section 3 presents the experimental results and analysis. Finally, the main conclusions are drawn in Section 4.
2 Materials and methods
2.1 Mask R-CNN
2.1.1 Overall architecture
The Mask R-CNN architecture is a powerful and versatile extension of Faster R-CNN, designed for both object detection and instance segmentation tasks. Its network architecture is primarily divided into three main components: the Backbone layer, the Region Proposal Network (RPN), and the Head layer. The Head layer comprises ROIAlign, a class head, a bounding box head, and a mask head. The overall structure of the algorithm is illustrated in Figure 1.
Backbone is responsible for extracting feature information from the input image and generating feature maps. Mask R-CNN typically uses ResNet (Residual Network) as its backbone structure, with the main ResNet variants including ResNet18, ResNet34, ResNet50, ResNet101, and ResNet152, the primary difference between them being the number of layers updated through training. The backbone structure efficiently extracts image features, providing rich information for subsequent processing. The ResNet101 feature extraction network consists of two basic modules: the convolutional module (Conv Block) and the residual module (Identity Block), which enhance the feature extraction capability of the convolutional network. The backbone part compresses the input image at different scales and passes the resulting four feature maps of varying scales to the Neck part.
2.1.2 Neck
In the Neck part, the FPN (Feature Pyramid Network) is adopted for fusing the feature maps from the backbone ResNet and generating effective feature maps (Lin et al., 2017). FPN combines feature maps from different layers through a bottom-up pathway, a top-down pathway, and lateral connections to form a multi-scale feature pyramid, enabling the model to better detect and recognize objects at different scales. The ResNet101 and FPN architecture (illustrated with a 512 × 512 image) is shown in Figure 2.
2.1.3 Region proposal network (RPN)
The region extraction stage is carried out by the Region Proposal Network (RPN), which extracts target information through anchor boxes and generates proposal boxes (Elfwing et al., 2018), while also obtaining offsets via bounding box regression. In the RPN, the effective feature map is cropped to obtain proposal boxes, enabling an initial filtering of objects. The RoIAlign method is then used to aggregate regional features, cropping the effective feature layers to form localized feature layers. The use of ROIAlign, decreasing a limitation in the original Faster R-CNN’s ROI Pooling, is one of the critical innovations in Mask R-CNN. ROIAlign correctly aligns the extracted features with the RoIs by using bilinear interpolation to preserve spatial accuracy. This results in more precise object localization and segmentation, which is crucial for tasks that require fine-grained segmentation, like instance segmentation.
2.1.4 Head layer
The head output part is responsible for further processing the proposed regions, including bounding box classification prediction and mask prediction. The local feature layers are passed into a classification and regression model for object detection and into a semantic segmentation model for processing the proposed boxes, achieving semantic segmentation.
The output layer of the ROI Head primarily includes ROIAlign and three heads: the class head, the bounding box head, and the mask head. In the preceding RPN network, the anchors generated in the final step serve as the input for ROIAlign. These anchors are also used to calculate the IoU (Intersection over Union) with each ground truth bounding box, ultimately producing the outputs for localization and classification.
2.1.5 Loss functions
The overall loss function L of the Mask R-CNN can be expressed as:
where
2.2 Dataset collection
The dataset for this study was meticulously gathered from seventy-four rural roads (approximately 100 km) located in Zhejiang Province, China, using a road surface inspection vehicle. The vehicle was equipped with advanced imaging equipment, which allowed for high-resolution, accurate capture of road surface conditions across various regions. This collection process was crucial for the subsequent analysis of road surface types and the development of a robust classification system.
In total, 4,000 road surface images were collected during the study, split equally between two major categories:
(1) 2,000 asphalt pavement images: These images represent a diverse range of road surfaces made from asphalt, which is one of the most common materials used in road construction. The images span different road conditions, including well-maintained surfaces, worn-out surfaces, and surfaces with varying degrees of cracks and other damages.
(2) 2,000 cement concrete pavement images: These images cover a variety of cement-based road surfaces, which are commonly used in rural and urban areas. Like the asphalt images, these include different conditions, from newly constructed cement roads to older, more degraded surfaces with visible wear, cracks, and other types of damage.
The images were captured at different times of the day and under various weather conditions, ensuring that the dataset reflects a wide range of real-world scenarios that the system would encounter in practice.
Each image in the dataset captures specific details relevant to road surface inspection, such as:
(1) Surface texture: The fine details of the surface, such as cracks, potholes, and wear patterns.
(2) Color variations: Asphalt typically appears darker, while cement roads often have a lighter color, though weathering and dirt accumulation can influence this.
(3) Structural damage: The presence of potholes, cracks, or joint failures.
(4) Environmental conditions: Variations in lighting, weather (such as rain or fog), and time of day, which provide a more comprehensive set of training data for machine learning algorithms.
As shown in Figure 3, typical examples of both asphalt and cement roads are displayed. These images highlight the differences in road surface characteristics, with asphalt images showing varying levels of cracking, rutting, and wear, while cement images show joint lines, cracks, and possible surface flaking.
These images include various road conditions and environmental factors to ensure the diversity and representativeness of the dataset, providing a solid foundation for subsequent road surface detection and analysis. Each image has been appropriately annotated to facilitate the training and testing of model performance.
2.3 Data annotation
Examples of the annotated data are shown in Figure 4. The dataset was annotated using a self-developed data annotation tool, which generated corresponding JSON format annotation files. Road surface distresses are primarily classified into two types: area-based distresses and length-based distresses. Area-based distresses include broken slabs, alligator cracking, and potholes, while length-based distresses mainly refer to cracks.
2.4 Data augmentation
In real-world scenarios, road surface data is often influenced by various environmental factors such as lighting variations, dirt accumulation, and surface impurities. These factors can significantly affect the quality and clarity of the images, making it challenging for machine learning algorithms to accurately process and analyze the road surfaces. To address these challenges and enhance the performance of the algorithms, several image preprocessing techniques were applied to the collected road surface images.
The primary goal of the preprocessing steps is to improve the quality of the images, standardize the input data, and ensure that the algorithms can focus on the relevant features (e.g., cracks, potholes, surface texture) without being distracted by distortions or environmental factors. The following processing techniques were applied to the dataset:
(1) Perspective Transformation:
This step corrects for any distortions caused by the angle at which the image was captured. Perspective transformation helps to align the road surface features, making them appear as if they were taken from a top-down view. This is particularly useful when dealing with images taken at oblique angles, ensuring uniformity across the dataset.
(2) Noise Reduction:
Noise from various sources such as camera sensors, environmental interference, or image compression artifacts can degrade image quality. Gaussian blur or median filtering techniques were applied to reduce random noise while preserving important structural details in the images, like cracks or potholes.
(3) Size Adjustment:
Images were resized to a standard resolution to ensure consistency in input data. This step is essential for deep learning models, which often require inputs of a specific size to maintain computational efficiency and training stability.
(4) Brightness Adjustment:
Variations in lighting conditions can significantly affect the visibility of road surface features. Histogram equalization or contrast adjustment techniques were used to balance the brightness levels, making the features in the image more prominent and reducing the effect of lighting inconsistencies. This adjustment is crucial for ensuring that algorithms can identify defects in poorly lit or overexposed images.
(5) Pixel Smoothing:
Smoothing algorithms (such as bilateral filtering or box filtering) were applied to reduce high-frequency noise while maintaining important edges and contours. This process helps to ensure that subtle surface defects, like fine cracks, are not obscured by noise or pixelation.
(6) Other Transformations:
Data Augmentation: To further improve the robustness of the models, transformations such as rotation, flipping, scaling, and cropping were applied. These techniques help simulate various road conditions and angles, increasing the diversity of the dataset and making the model more adaptable to different input scenarios.
The specific data improvement methods used for different types of distresses and the quantities before and after improvement are presented in Table 1. Figure 5 illustrates a comparison of images before and after distortion correction. As shown in the figure, the image after distortion correction is clearer and more standardized, with structural features more distinct, which helps improve recognition accuracy. Figure 6 compares images before and after image processing, where the image processing methods mainly include brightening and denoising. As shown in the figure, the processed image has significantly reduced noise, with enhanced brightness and details, making it clearer and easier to recognize, thereby providing a better foundation for subsequent recognition processes.
3 Experimental results and analysis
3.1 Experimental setup
The hardware used for the experiments was a server running the Red Hat 11.4 operating system, a 64-bit system based on an x64 processor, with a total of 125 GB of RAM. The training was conducted using Python 3 as the programming language and PyTorch as the deep learning framework. During the experiments, Mask R-CNN with ResNet combined with FPN was used as the backbone network for model training, including three backbone variants: ResNeXt-101, ResNet-50-FPN, and ResNet-101-FPN. Additionally, the SCNet network (Liu et al., 2017) with ResNet-50 and ResNet-101 as backbone networks was also used for model training.
3.2 Model training evaluation metrics
In this experiment, the effect of the Mask R-CNN detection model is evaluated by the Intersection over Union (
In the study, the evaluation metrics included Average Precision (
Recall and Precision often influence each other; aiming for a high recall can lead to a decrease in Precision, resulting in false positives, while focusing on improving Precision may lower the recall, causing missed detections. The mAP@0.5 represents the mean Average Precision values across multiple classes when the Intersection over Union (
3.3 Result analysis
The experiments were conducted using the dataset allocation standards outlined in Table 1 for training, validation, and testing. The processing time per image for the algorithm was 0.155444 s. For training and validating cases, the loss function L (Equation 1) of the Mask R-CNN decreases along with increasing training steps, as shown in Figure 7. After 50 epochs, the total loss is close to 0.3, oscillating in a small range.
The algorithm’s recognition results are shown in Figure 8. From the figure, it can be observed that the algorithm successfully identifies a variety of defects commonly found on rural roads. These include net-shaped cracks, pits, ruptures, broken slabs, and other forms of road damage.
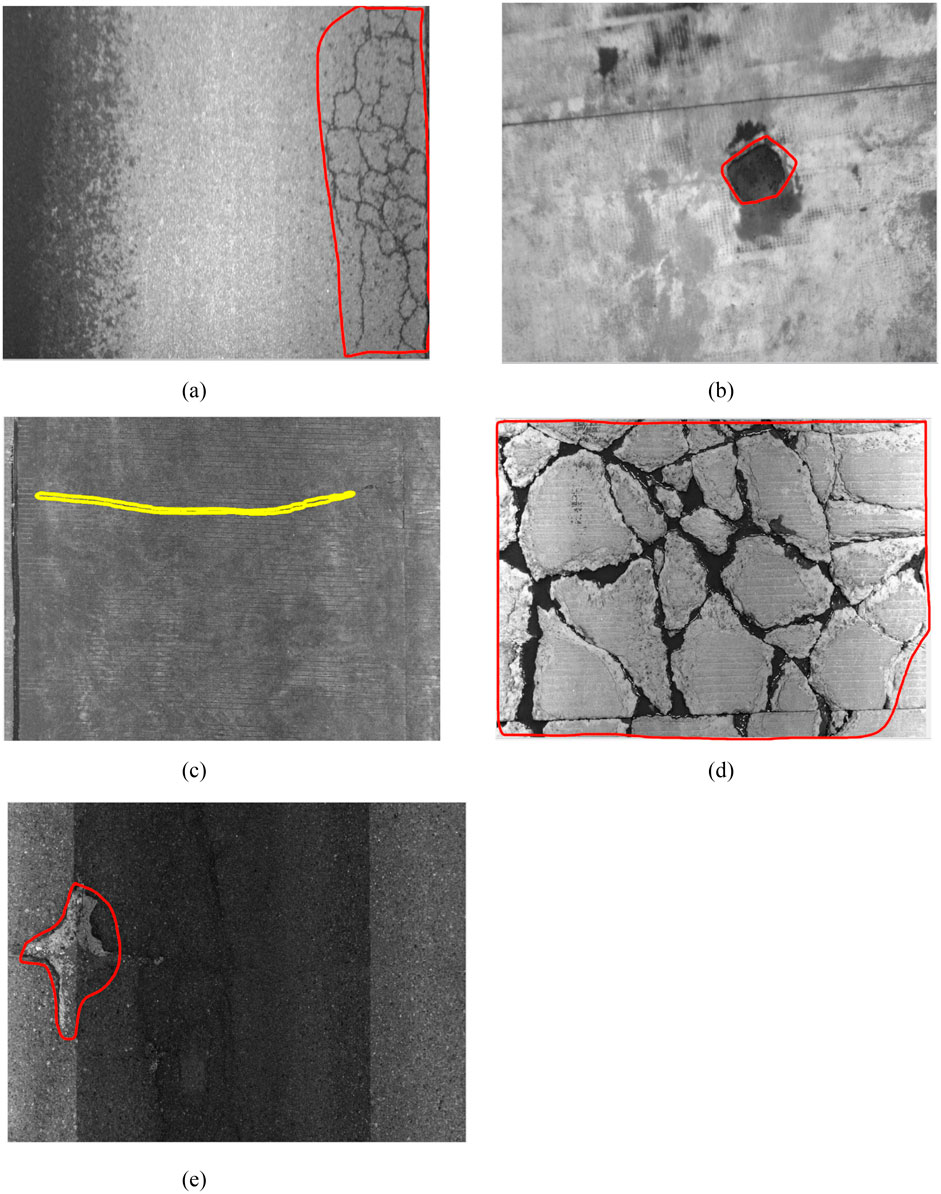
Figure 8. Algorithm recognition results. Note: The distress regions are delineated. (a) Net-Shaped Cracks; (b) Pits; (c) Cracks; (d) Broken Slabs; (e) Potholes.
The average precision rate (AP) from Equation 2 of the algorithm is presented in Table 2, and the average recall rate (AR) from Equation 3 is detailed in Table 3. The results show that the data augmentation indeed increases the precision of the recognition. Also, the experimental results suggest that the Mask R-CNN combined with the ResNeXt-101-FPN backbone network can have the best precision and recall rates among the different modes and backbone.
The AP and AR rates for different types of diseases are provided in Tables 4, 5. It is found that due to the different characteristics and nature of various road surface defects, the resulting AP and AR rates are also various. For example, potholes, pits, and broken slab have larger affected areas and more distinct features, which are less influenced by surrounding environmental factors, their AP and AR rates are higher compared to other defects. On the other hand, horizontal and vertical cracks and net-shaped cracks are small target defects with less obvious features and are more significantly affected by factors like lighting and water stains, leading to lower AP and AR rates.
3.4 Field tests
To assess the performance of the developed algorithm, field tests (see Figure 9) were conducted in Yuhang District, Hangzhou City, Zhejiang Province, China. Images were captured using a vehicle-mounted camera (see Figure 9, and an 8 MP RGB camera with 25 fps capture rate) while driving approximately 5 km on asphalt (60%) and cement concrete (40%) pavements, and these images were processed with the proposed algorithm.
Figure 10 shows comparisons of the model results with manual observations. It can be seen that the road distresses detected by the model are very similar to those identified through manual observations, which indicates the robustness and accuracy of the developed model. Table 6 presents the statistical results of three field tests. By comparing the identified distress areas with the actual distress areas, the average absolute area error rates ranged from 9.96% to 23.46%, demonstrating that the developed algorithm is both feasible and effective for detecting various types of distress on rural roads. Moreover, compared to traditional manual visual assessments, the time required to detect road distress was reduced by approximately 40%.
The ability to detect these defects with high accuracy demonstrates the robustness and versatility of the algorithm in handling different types of road surface deterioration. Moreover, the system is capable of distinguishing between different levels of severity and classifying defects according to their specific characteristics, such as size, shape, and location. This ensures that maintenance crews can prioritize repairs more effectively, potentially leading to significant improvements in road safety and longevity.
Additionally, the algorithm’s performance suggests that it could be integrated into an automated road monitoring system, providing continuous, real-time analysis of road conditions without the need for manual inspections. This could be particularly beneficial in rural areas, where road maintenance often faces resource and manpower limitations. The results also imply that the algorithm could be adapted to other types of infrastructure, such as bridges or tunnels, enhancing its applicability in various domains of civil engineering.
3.5 Limitation and future work
The current algorithm, compared to traditional manual recognition methods, significantly improves the efficiency of rural road defect detection. However, based on the current results, there is still room for improvement in both precision and recall rates. The primary source of error may be related to image quality. Since the images are captured by cameras installed on vehicles, factors such as vehicle speed, lighting, and weather conditions during image collection can affect image quality. In addition, the quality of rural roads is generally worse than that of regular roads, which further complicates image recognition. In the future, combining multiple deep learning models, such as integrating Mask R-CNN with YOLO, could help reduce false detection rates. Furthermore, repeated road inspections can improve the accuracy of recognition on road defect as well.
In the current algorithm, to improve the efficiency of manual visual inspection, we have adopted a strategy of low false positive and high false negative detection for common defects (such as cracks and repairs). This strategy helps maximize human efficiency to some extent. However, as data continues to accumulate and models are iteratively upgraded, future models will evolve towards a more balanced approach, aiming to reduce both false positives and false negatives simultaneously.
4 Conclusion
To address the limitations of traditional image processing and machine learning techniques in detecting rural road pavement defects, and to overcome the challenges posed by the low contrast between defects and their surrounding background, this paper proposes a Mask R-CNN-based algorithm for rural road pavement defect detection. The novelty of this approach lies in the integration of Mask R-CNN with data augmentation techniques, such as image translation, flipping, and noise perturbation, which significantly enhance the recognition accuracy of road surface defects. This method is specifically applied to rural roads in China. The developed algorithm enables precise detection and segmentation of road defects. After training on a dataset of 4,000 high-quality images of rural road pavement defects, the model’s loss value stabilized, confirming its effectiveness. Experimental results demonstrate that the Mask R-CNN algorithm outperforms the ScNet algorithm in terms of precision for defect detection and segmentation, showcasing its superior capability for rural road pavement distress detection. Additionally, three field tests validate the feasibility and reliability of the proposed algorithm in real-world conditions. The system, combining Mask R-CNN with data augmentation, effectively distinguishes between varying levels of severity and classifies defects based on characteristics such as size, shape, and location. This enables maintenance crews to prioritize repairs more efficiently, leading to significant improvements in road safety and durability.
Data availability statement
The original contributions presented in the study are included in the article/supplementary material, further inquiries can be directed to the corresponding author.
Author contributions
DL: Conceptualization, Funding acquisition, Formal Analysis, Project administration, Writing – original draft. HZ: Conceptualization, Funding acquisition, Methodology, Writing – review and editing. LC: Formal Analysis, Methodology, Software, Writing – original draft. YZ: Software, Validation, Writing – original draft. YL: Validation, Writing – original draft. RQ: Data curation, Writing – original draft. YJ: Data curation, Writing – original draft.
Funding
The author(s) declare that financial support was received for the research and/or publication of this article. This work was supported by Zhejiang Provincial Department of Transportation Science and Technology Program Project (No. 2024018).
Conflict of interest
The authors declare that the research was conducted in the absence of any commercial or financial relationships that could be construed as a potential conflict of interest.
Generative AI statement
The author(s) declare that no Generative AI was used in the creation of this manuscript.
Publisher’s note
All claims expressed in this article are solely those of the authors and do not necessarily represent those of their affiliated organizations, or those of the publisher, the editors and the reviewers. Any product that may be evaluated in this article, or claim that may be made by its manufacturer, is not guaranteed or endorsed by the publisher.
References
Arya, D., Maeda, H., Ghosh, S. K., and Bao, Y. (2021). Detection, visualization, quantification, and warning of pipe corrosion using distributed fiber optic sensors. Autom. Constr. 132, 103953. doi:10.1016/j.autcon.2021.103953
Azimi, M., Eslamlou, A. D., and Pekcan, G. (2020). Data-driven structural health monitoring and damage detection through deep learning: state-of-the-art review. Sensors 20 (10), 2778. doi:10.3390/s20102778
Du, Y., Pan, N., Xu, Z., Deng, F., Shen, Y., and Kang, H. (2021b). Pavement distress detection and classification based on YOLO network. Int. J. Pavement Eng. 22, 1659–1672. doi:10.1080/10298436.2020.1714047
Du, Z. Y., Yuan, J., Xiao, F. P., and Hettiarachchi, C. (2021a). Application of image technology on pavement distress detection: a review. Measurement 184, 109900. doi:10.1016/j.measurement.2021.109900
Elfwing, S., Uchibe, E., and Doya, K. (2018). Sigmoid-weighted linear units for neural network function approximation in reinforcement learning. Neural Netw. 107, 3–11. doi:10.1016/j.neunet.2017.12.012
Girshick, R. (2015). Fast R-CNN. Proc. IEEE Int. Conf. Comput. Vis., 1440–1448. doi:10.1109/ICCV.2015.169
He, K., Gkioxari, G., Dollár, P., Girshick, R., and Mask, R.-C. N. N. (2017). Proc. IEEE Int. Conf. Comput. Vis. (ICCV), 2980–2988. doi:10.1109/ICCV.2017.322
He, K., Zhang, X., Ren, S., and Jian, S. (2016). Deep residual learning for image recognition. Proc. IEEE Conf. Comput. Vis. Pattern Recognit. (CVPR), 770–778. doi:10.1109/CVPR.2016.90
Hu, L., and Ren, J. (2023). YOLO-LHD: an enhanced lightweight approach for helmet wearing detection in industrial environments. Front. Built Environ. 9, 1288445. doi:10.3389/fbuil.2023.1288445
Ibragimov, E., Lee, H. J., Lee, J. J., and Kim, N. (2022). Automated pavement distress detection using region-based convolutional neural networks. Int. J. Pavement Eng. 23 (6), 1981–1992. doi:10.1080/10298436.2020.1833204
Kang, D., Benipal, S. S., Gopal, D. L., and Cha, Y. J. (2020). Hybrid pixel-level concrete crack segmentation and quantification across complex backgrounds using deep learning. Autom. Constr. 118, 103291. doi:10.1016/j.autcon.2020.103291
Khan, M., Raza, M. A., Abbas, G., Othmen, S., Yousef, A., and Jumani, T. A. (2024). Pothole detection for autonomous vehicles using deep learning: a robust and efficient solution. Front. Built Environ. 9, 1323792. doi:10.3389/fbuil.2023.1323792
Lin, T. Y., Dollar, P., Girshick, R. ;, He, K., Hariharan, B., and Belongie, S. (2017). Feature pyramid networks for object detection. Proc. IEEE Conf. Comput. Vis. Pattern Recognit. (CVPR), 936–944. doi:10.1109/CVPR.2017.106
Liu, J. J., Hou, Q., Cheng, M.-M., Wang, C., and Feng, J. (2017). Improving convolutional networks with self-calibrated convolutions. Proc. IEEE/CVF Conf. Comput. Vis. Pattern Recognit. (CVPR)., 10093–10102. doi:10.1109/CVPR42600.2020.01011
Ma, D., Fang, H., Wang, N., Zhang, C., Dong, J., and Hu, H. (2022). Automatic detection and counting system for pavement cracks based on PCGAN and YOLO-MF. IEEE Trans. Intell. Transp. Syst. 23 (11), 22166–22178. doi:10.1109/tits.2022.3161960
Nomura, Y., Inoue, M., and Furuta, H. (2022). Evaluation of crack propagation in concrete bridges from vehicle-mounted camera images using deep learning and image processing. Front. Built Environ. 8, 972796. doi:10.3389/fbuil.2022.972796
Qian, Y. (2024). Intelligent railroad inspection and monitoring. Front. Built Environ. 10, 1389092. doi:10.3389/fbuil.2024.1389092
Ren, S., He, K., Girshick, R., and Jian, S. (2017). Faster R-CNN: towards real-time object detection with region proposal networks. IEEE Trans. Pattern Anal. Mach. Intell. 39 (6), 1137–1149. doi:10.1109/TPAMI.2016.2577031
Song, L., and Wang, X. (2021). Faster region convolutional neural network for automated pavement distress detection. Road. Mater. Pavement Des. 22 (1), 23–41. doi:10.1080/14680629.2019.1614969
Wan, F., Sun, C., He, H., Lei, G., Xu, L., and Xiao, T. (2022). YOLO-LRDD: a lightweight method for road damage detection based on improved YOLOv5s. EURASIP J. Adv. Signal Process. 2022, 98–18. doi:10.1186/s13634-022-00931-x
Wang, J., Sharma, P. K., Alfarraj, O., Tolba, A., Zhang, J., Wang, L., et al. (2024). Dual-path network combining CNN and transformer for pavement crack segmentation. Autom. Constr. 158, 105217. doi:10.1016/j.autcon.2023.105217
Wang, S. K., Chen, X. Q., and Dong, Q. (2023). Detection of asphalt pavement cracks based on vision transformer improved YOLO V5. J. Transp. Eng. Part B. Pavements 149 (2), 1–12. doi:10.1061/jpeodx.pveng-1180
Xiao, S. Z., Shang, K. K., Lin, K., Wu, Q., Gu, H., and Zhang, Z. (2023). Pavement crack detection with hybrid-window attentive vision transformers. Int. J. Appl. Earth Obs. Geoinf. 116, 103172. doi:10.1016/j.jag.2022.103172
Xu, X., Zhao, M., Shi, P., Ren, R., He, X., Wei, X., et al. (2022b). Crack detection and comparison study based on faster R-CNN and mask R-CNN. Sensors 22, 1215. doi:10.3390/s22031215
Xu, Z., Guan, H., Kang, J., Lei, X., Ma, L., Yu, Y., et al. (2022a). Pavement crack detection from CCD images with a locally enhanced transformer network. Int. J. Appl. Earth Obs. Geoinf. 110, 102825. doi:10.1016/j.jag.2022.102825
Keywords: rural road, mask R-CNN, distress detection, data augmentation, automation
Citation: Li D, Zhang H, Chen L, Zhou Y, Li Y, Qian R and Jiang Y (2025) Rural road surface distress detection algorithm based on mask R-CNN with data augmentation. Front. Built Environ. 11:1566979. doi: 10.3389/fbuil.2025.1566979
Received: 26 January 2025; Accepted: 05 May 2025;
Published: 15 May 2025.
Edited by:
Qiang Zhang, Guangxi University, ChinaReviewed by:
Koorosh Gharehbaghi, RMIT University, AustraliaSakdirat Kaewunruen, University of Birmingham, United Kingdom
Copyright © 2025 Li, Zhang, Chen, Zhou, Li, Qian and Jiang. This is an open-access article distributed under the terms of the Creative Commons Attribution License (CC BY). The use, distribution or reproduction in other forums is permitted, provided the original author(s) and the copyright owner(s) are credited and that the original publication in this journal is cited, in accordance with accepted academic practice. No use, distribution or reproduction is permitted which does not comply with these terms.
*Correspondence: Hang Zhang, emhhbmdoYW5nMDEwOEB5ZWFoLm5ldA==