- 1National Research Council, IRIB-CNR, Institute for Biomedical Research and Innovation, Messina, Italy
- 2Department of Mathematical and Computer Sciences, Physical Sciences and Earth Sciences, University of Messina, Messina, Italy
This paper examines different computational models for Calcium wave propagation in astrocytes. Through a comparative analysis of models by Goldbeter, De Young-Keizer, Atri, Li-Rinzel, and De Pittà and of experimental data, the study highlights the model contributions for the understanding of Calcium dynamics. Tracing the evolution from simple to complex models, this work emphasizes the importance of integrating experimental data in order to further refine these models. The results allow to improve our understanding of the physiological functions of astrocytes, suggesting the importance of more accurate astrocyte models.
Introduction
The field of neuroscience, and more specifically computational neuroscience, has in recent decades focused almost exclusively on the study and modeling of neuronal components and dynamics at both the cellular and network levels, almost completely neglecting the role of astrocytes except for their metabolic and homeostatic activity. Recent studies have shown that astrocyte Ca2+ variation is associated with the modulation of neuronal signaling through the uptake and release of neurotransmitters (Haydon and Carmignoto, 2006; Volterra and Meldolesi, 2005; Khakh and Mccarthy, 2015; Pasti et al., 1997; Fiacco and Mccarthy, 2006; Kofuji and Araque, 2021; Semyanov et al., 2020; Verkhratsky and Nedergaard, 2018; Khakh and Sofroniew, 2015). A growing body of research demonstrates that astrocytes are more than merely passive read-out units (Temburni and Jacob, 2001); rather, they play a significant role in controlling the activity of neuronal synapses (Fellin et al., 2006; Perea et al., 2009; Clarke and Barres, 2013). Astrocytes have a sort of chemical excitability based on variations in intracellular Calcium concentration, despite not being electrically excitable cells that is, they cannot produce action potentials. Astrocytes control the number of neurotransmitters in the synaptic cleft by regulating intracellular and intercellular Calcium dynamics; thereby controlling the synaptic signal current between two neurons. It is now known that astrocyte Ca2+ signaling is essential for proper functioning of neuronal activity and dysfunction of astrocyte dynamics is implicated in the onset of neurodegeneration (Kang et al., 2005; Nadkarni and Jung, 2003; Tian et al., 2005; Verkhratsky et al., 2010; Eddleston and Mucke, 1993; Rappold and Tieu, 2010; Eid et al., 2008; Madinier et al., 2013; Mitroshina et al., 2022; Jiang et al., 2024).
The discovery that astrocytes are responsible for neuronal activity has led to the creation of various mathematical and computational models for simulating astrocyte dynamics. Of these, those relating to the modulation of intracellular Ca2+ waves occupy particular importance due to their importance in cell communication. Research on glia entered a new era with the fundamental discovery in the 1980s that astrocytes express a wide range of receptors for neurotransmitters. Subsequent research has shown that the release of neurotransmitters during synaptic activity can activate these receptors and cause an increase in Ca2+ in astrocytes. In turn, this mechanism can cause the release of gliotransmitters such as glutamate, ATP and D-serine, which are capable of activating neuronal receptors, thus modifying the electrical excitability of neurons and synaptic transmission, triggering intercellular communication between astrocytes and neurons (Araque et al., 1999; Fellin et al., 2004; Schipke and Kettenmann, 2004; Jourdain et al., 2007). Thanks to these findings, the theory of “tripartite synapses” was developed, which considers astrocytes as the third component of the signal integration unit (Volterra et al., 2002). Recently, much research has been conducted on the mechanism of chemical transmitter release from astrocytes. Of all the gliotransmitters, glutamate has undoubtedly attracted the most attention due to the fundamental discovery by Anne Cornell-Bell and colleagues that glutamate evokes increased Calcium concentrations in astrocytes (Cornell-Bell et al., 1990).
Various studies have been done to confirm that astrocytes possess specific receptors for glutamate on the outer surface of the plasma membrane (mGluRs) (Anderson and Swanson, 2000; Backus et al., 1989; Condorelli et al., 1997). The function of glial mGluRs is still almost unknown, on the contrary, there is much evidence on the role of ionotropic glutamate receptors in glial cells (Dantoni et al., 2008; Verkhratsky and Steinhäuser, 2000; Kondoh et al., 2001; Seifert and Steinhäuser, 2001). Astrocytes release glutamate, which diffuses into the extra synaptic space and binds to metabotropic glutamate receptors (mGluRs) or NMDA receptors (NMDARs) of neighboring presynaptic terminals in turn, they may respond to the glutamate released at the synaptic level with an increase in intracellular Ca2+ that may trigger the release of further glutamate by astrocytes (Malarkey and Parpura, 2008; Skowrońska et al., 2019; Santello and Volterra, 2009; Montana et al., 2006).
Modeling and theoretical study of Ca2+ dynamics involving the IP3 receptor channel are the main topics of the review. It also provides a synopsis of the experimental results.
The models presented in this review are united by the fact that the dynamics of IP3 and the compartmental changes of Ca2+ are integrated in a set of ordinary differential equations. System parameters have a sensitive effect on the propagation of released Ca2+. Therefore, instead of reviewing the results of each study, we will present the ideas and techniques employed.
Section of models
The Goldbeter model
Pioneering models for intracellular Ca2+ signaling include the Goldbeter et al. model (Santello and Volterra, 2009), which predicts the occurrence of periodic spikes of the ion in the absence of IP3 oscillations, indicating that repetitive Ca2+ spikes do not necessarily require a concomitant periodic change in IP3 and can be induced by external stimulation. The model assumes the existence of two distinct internal stores, one sensitive to IP3 and the other sensitive to Ca2+. The IP3 produced by agonist stimulation leads to a release of Ca2+ from the IP3-sensitive store via the IP3Rs. The released Ca2+ will stimulate a further release from the Ca2+ sensitive store (see Figure 1), which self-amplifies above a threshold value for cytosolic Ca2+ concentration (C), representing a model for Induced Calcium Release (CIRC). Depletion of the Ca2+-sensitive pool (CER) limits the release. This model makes the critical assumption that the Ca2+ in the IP3-sensitive store remains constant as the extracellular medium rapidly replenishes it. The model lacks a mechanism for IP3-dependent Ca2+ inhibition. The two variables in the model are the concentration of free Ca2+ in the cytosol and in the IP3-insensitive repository (e.g., the endoplasmic reticulum or sarcoplasmic reticulum); these variables are denoted Z and Y, respectively. Assuming that buffering is linear with respect to Ca2+ concentration, the time evolution of the systems is governed by the two kinetic equations:
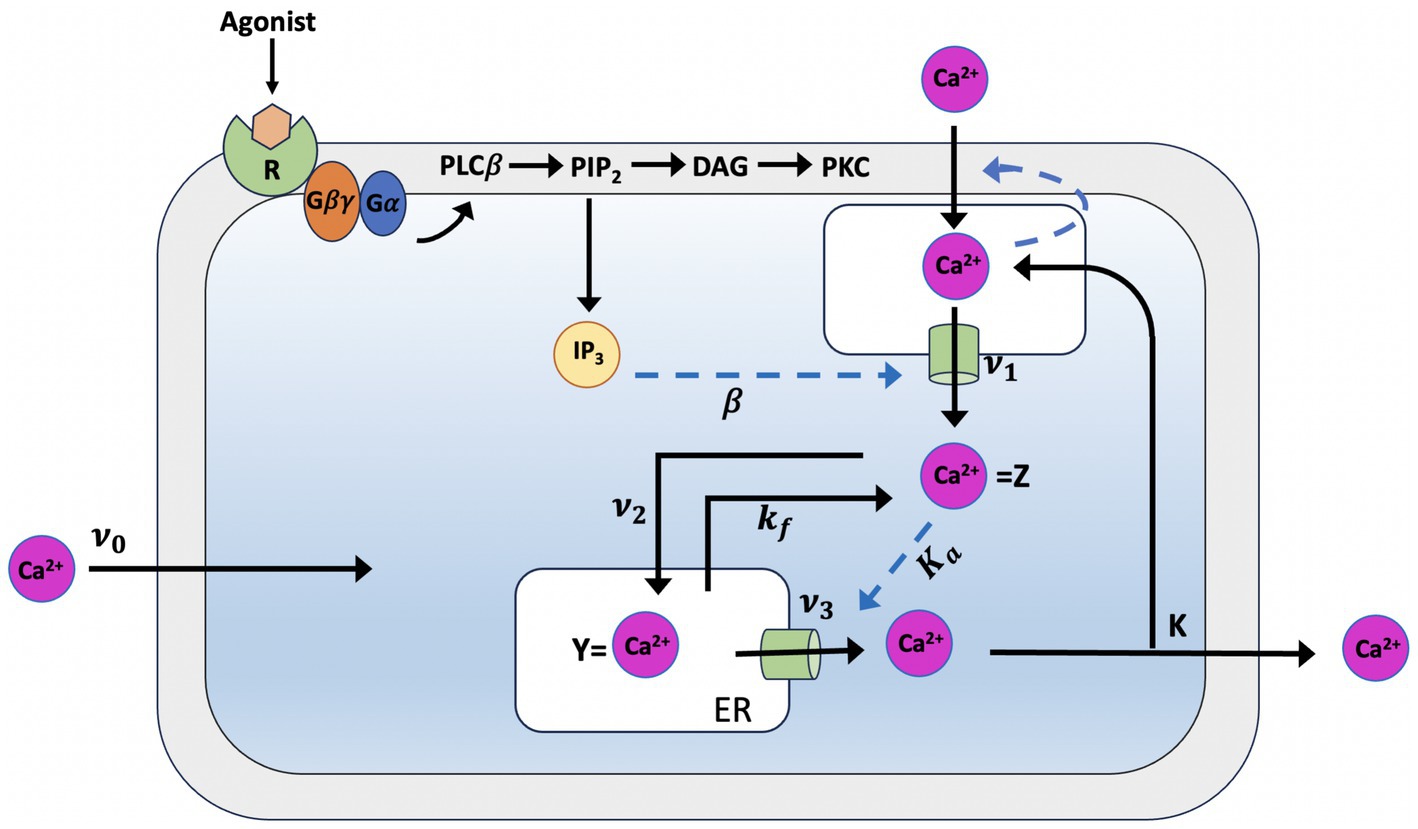
Figure 1. Illustration of the production mechanism of Ca2+ oscillations according to Goldbeter model, which is based on Ca2+ release induced by intracellular stores. Ca2+ release is modulated by IP3 from an IP3-sensitive store located in the cytosol which also indirectly controls the influx of external Ca2+ into this store. In the model, determines the constant Ca2+ flux in the cytosol, which is controlled by each level of InsP3. Z, the cytosolic Ca2+ concentration, passes from a phase of low concentration, during which priming Ca2+ is transferred into the InsP3-insensitive pool, to a phase in which the Ca2+ stored in that pool (Y) is released into the cytosol this phase is characterized by short peaks of Ca2+. The parameter refers to the influx of extracellular Ca2+ into the cytosol, to the influx of cytosolic Ca2+ from the cell to the extracellular space and to the passive loss of Y in Z (see text for details).
In Equation 1, the ν0 parameter, which is assumed take constant, relates to the Ca2+ input from the extracellular medium into the cell; kZ, which is assumed to be linear, pertains the outflow of Ca2+ into outflow from the cell, which occurs even in the absence of external stimulation. ν1β denotes the InsPs-modulated release of Ca2+; ν2 indicates the rate of ATP-driven pumping of Ca2+ from the cytosol into the InsP3-insensitive store, while ν3 represents the rate of transport from this pool into the cytosol; finally, the term kfY refers to a nonactivated transport of C into CER.
When the cell receives an external signal, this triggers an increase in InsP3, which leads to a rise in the saturation function β and, subsequently, to an increase in cytosolic Ca2+.
Were VM2 and VM3 denote, respectively, the maximum rates of Ca2+ pumping into and release from the intracellular store; these processes are described by Hill functions whose cooperativity coefficients are taken as n and m; p denotes the degree of cooperativity of the activation process; K2, KR, and KA are threshold constants for pumping, release, and activation.
The Goldbeter model assumes that two different types of pools are required for Ca2+ oscillations, some of which are sensitive to InsP3 and others with RyR and thus sensitive to Ca2+. Due to the InsP3R’s inherent sensitivity to both Ca2+ and InsP3, this proved unneeded. Subsequently, Dupont and Goldbeter formulated a version of the model that assumes the existence of a single pool in which Ca2+ and IP3 are co-agonists for the induction of Ca2+ release (Dupont and Goldbeter, 1993; Table 1).
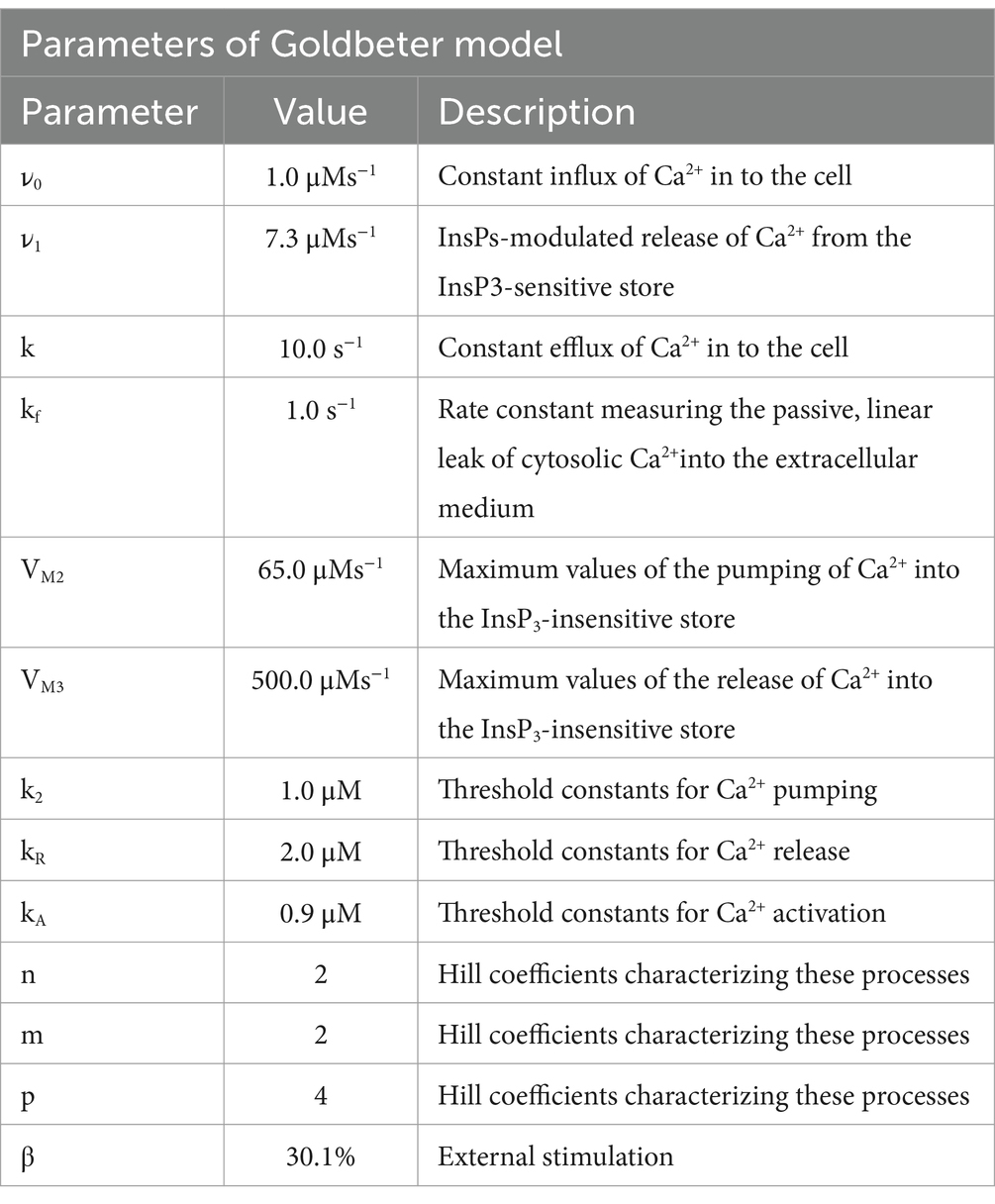
Table 1. Parameters of the Goldbeter model (Goldbeter et al., 1990).
The De Young-Keizer model
In 1992, the De Young-Keizer model (Young et al., 1992) studied the properties of the IP3 receptor/ Ca2+ channel; in particular, it examined the biphasic response of the IP3 receptor/channel to cytosolic Ca2+ and how this could be sufficient to induce Ca2+ oscillations. The rate constants in the equations were fitted to the kinetic and equilibrium data and the model successfully reproduced a series of in vivo and in vitro experiments (Berridge and Irvine, 1989; Mouillac et al., 1990; Smrcka et al., 1991; Taylor and Exton, 1987). The model incorporates a positive Ca2+ feedback mechanism on IP3 production by phospholipase-C (PLC). It was noted that this enriches the properties of oscillations and leads to Ca2+ oscillations accompanied by IP3 oscillations (see Figure 2). They created a simplified model of the IP3 receptor/channel by assuming that Ca2+ conduction is mediated by three equivalent, independent subunits, all of which must be in a conducting state before the receptor allows Ca2+ to flow. There are three binding sites on each subunit, one for IP3, one for Ca2+ activation and one for Ca2+ inactivation. Consequently, each subunit can exist in eight states, with transitions controlled by first and second order rate constants for association and dissociation, respectively. Each state is labeled with Sijk the first index refers to the IP3 binding site, the second to the Ca2+ activation site and the third to the Ca2+ inactivation site; i,j,k take the value 0 or 1 depending on whether the binding site is unoccupied or occluded (see Figures 3, 4).
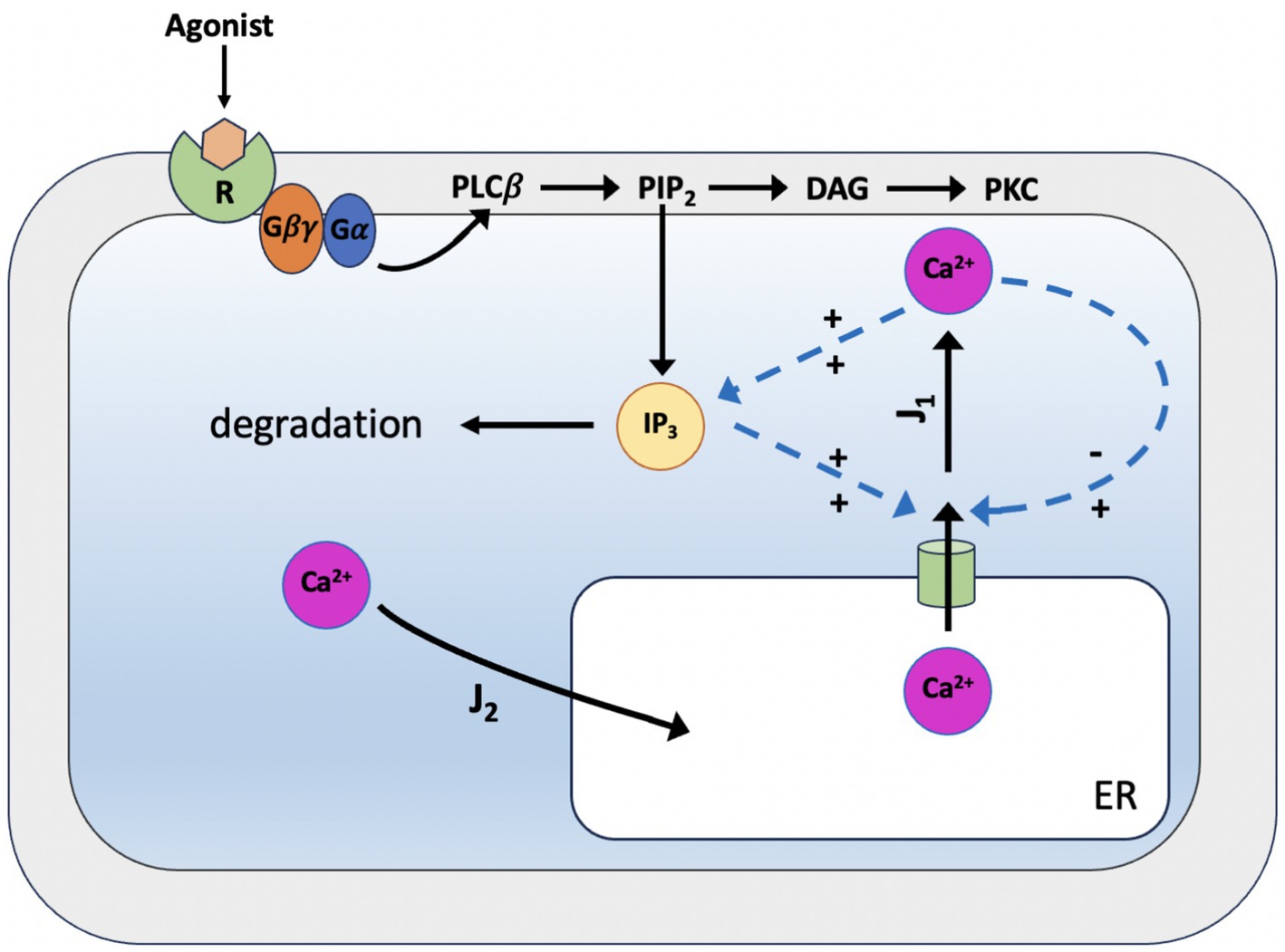
Figure 2. Scheme of the simplified De Young-Keizer kinetic model describing the properties of Ca2+ activation and inhibition by the inositol 1,4,5-trisphosphate (IP3) receptor in the endoplasmic reticulum. J1 is the outward flux of Ca2+, and J2 is the inward flux. J1 has two components, the Ca2+ flux through the IP3 receptor/channel and a constant leak flux. J2 represents the flux facilitated by the ATP-dependent Ca2+ pumps which actively transport Ca2+ from the cytosol back into the endoplasmic reticulum. The model incorporates the activity of Ca2+-ATPase, which is responsible for pumping Ca2+ back into the endoplasmic reticulum, and results in oscillations of cytoplasmic Ca2+ concentrations when the IP3 concentration is held constant. This occurs with only a single pool of Ca2+ available for release from the endoplasmic reticulum.
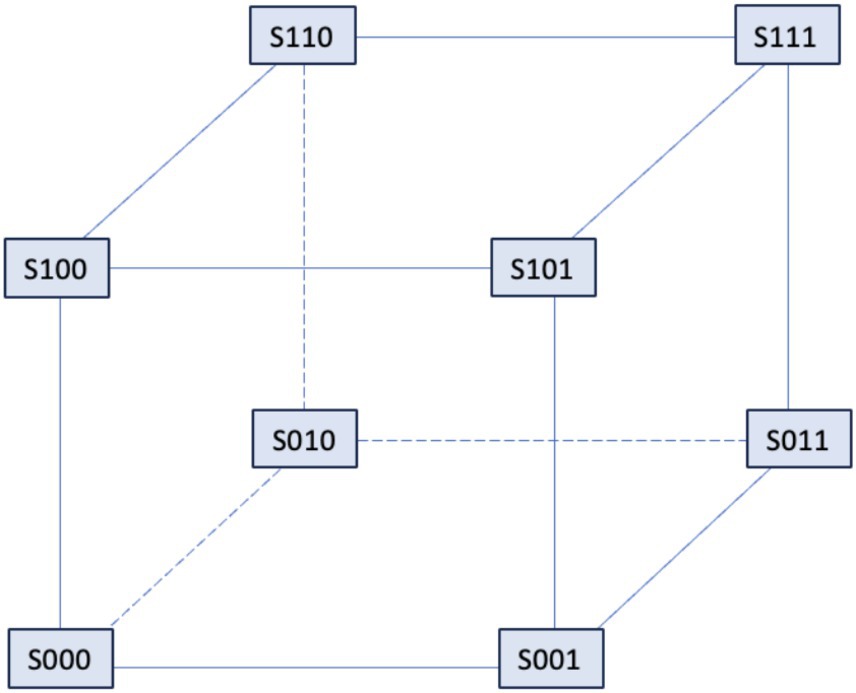
Figure 3. The IP3 receptor’s single unit’s binding mechanism. The probability that the component will be in one of the states [i,j,k], where i, j, and k can take the values 0 and 1, is shown by Sijk. The IP3 binding site’s condition is indicated by the first index, the activating Ca2+ binding site by the second, and the inhibitory Ca2+ binding site by the third. The corresponding binding site is unbound if an index is zero, and bound if an index is one.
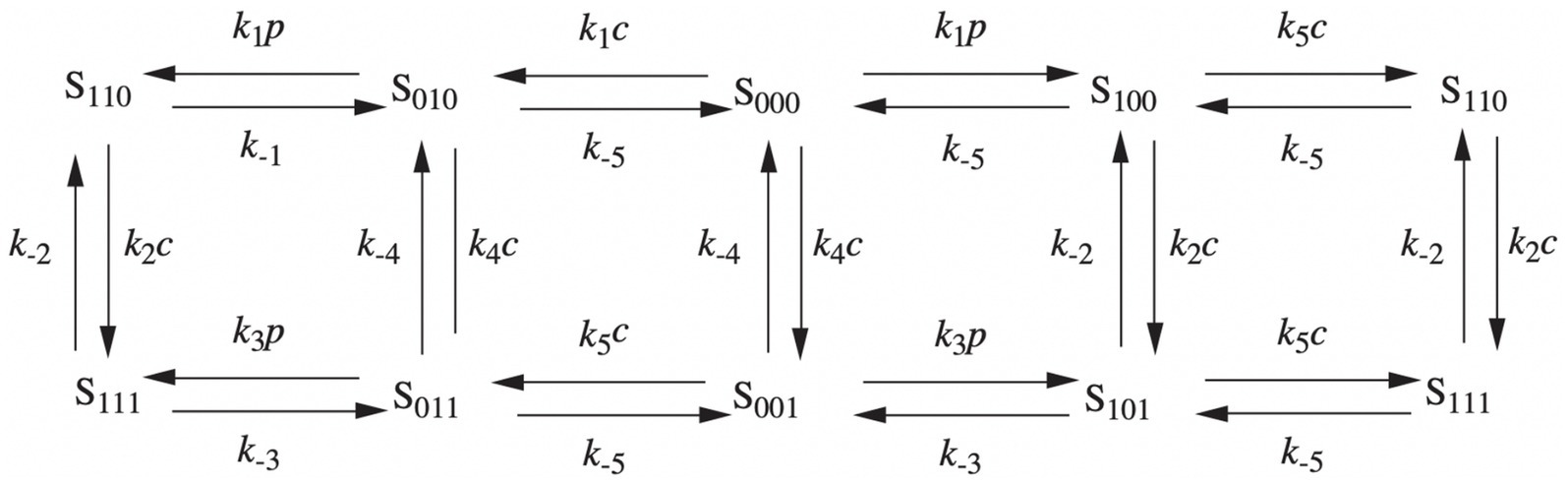
Figure 4. Binding diagram of De Young-Keizer IP3 pattern in two dimensions. c stands for Ca2+ and p for IP3.
The 24 not-all-independent speed constants of the model were reduced to 10 constants, by introducing the following two assumptions:
i. the rate constants are independent of whether or not Ca2+ is bound to the activation site
ii. Ca2+ activation kinetics do not depend on IP3 or Ca2+ inactivation.
Since experimental data indicate that the receptor subunits act cooperatively, for the channel to be open and in conduction, all three subunits must be in the S110 state (one bound to IP3 and one to activating Ca2+). The gives rise to seven differential equations for the receptor states. Although there are eight states, only seven are independent. As far as mass-action kinetics are concerned, the Ordinary Differential Equations (ODEs) for the receptor states have the present form:
where p denotes [IP3] and c denotes [Ca2+].
The DeYoung and Keizer model consists of seven ODEs for receptor states with the following Equations 5–7 that describing the [Ca2+] handling of the IP3-sensitive Ca2+ pool and the IP3 production:
where c is the cytosolic free Ca2+ concentration, J1 is the outward flux of Ca2+ and J2 is the inward flux (see Figure 2).
J1 has two components, the Ca2+ flux through the IP3 receptor/channel and a constant leak flux. c1 is the ratio between the volume of the ER and the volume of the cytosol. cER and c are the Ca2+ in the ER and cytosolic Calcium, respectively; 1 is the max Ca2+ channel flux, 2 is the Ca2+ leak flux constant; 3 is the Max Ca2+ uptake and K3 is the Activation constant for ATP-Ca2+ pump (Table 2).
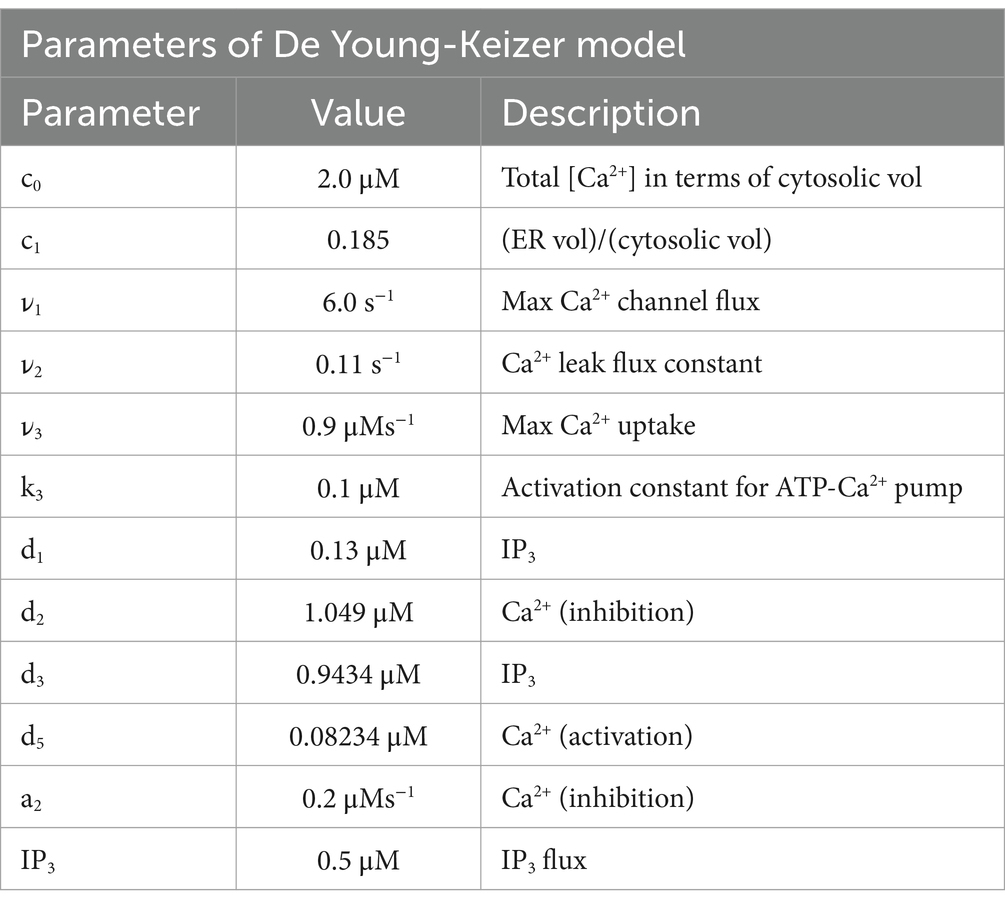
Table 2. Parameters of the De Young-Keizer model (Young et al., 1992).
The Atri model
In 1993, Atri et al. constructed a minimalist model for Ca2+ wave oscillations (Smrcka et al., 1991). The model, which served as the basis for a number of other models, proved simple enough to allow an understanding of the oscillatory phenomena underlying the spatio-temporal properties of Ca2+. A single intracellular Ca2+ pool that releases Ca2+ through the IP3R is included in the model. It is believed that Ca2+ modulates the IP3R in a biphasic manner, with intermediate Ca2+ acting to increase Ca2+ release while low and high Ca2+ act to block it (see Figure 5). The model takes its cue from Finch et al. (1991), and distinguishes between the time scales of channel activation and inactivation, where inactivation proceeds at a slower rate than activation. This temporal separation is critical for the spatial propagation of the Ca2+ signal, as inactivation must occur more gradually than activation to ensure the effective transmission of waves.
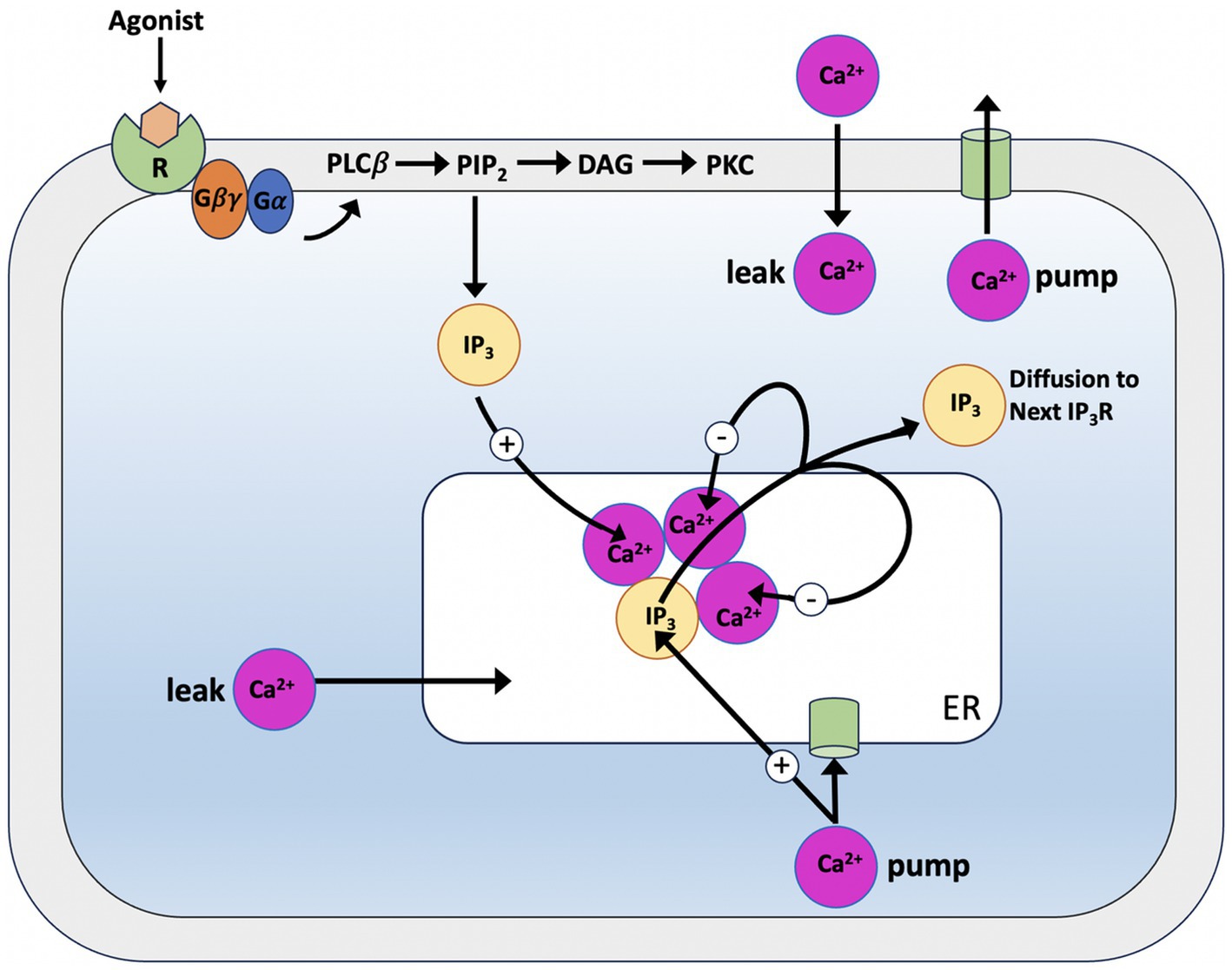
Figure 5. Schematic illustration of the Atri model. When IP3 reaches the binding sites of the IP3 receptor (IP3R), it allows Calcium to leave the endoplasmic reticulum by opening a Calcium-permeable channel. After leaving the channel, Calcium diffuses to the next storage site, inactivating the channel (−) and increasing (+) the sensitivity of the IP3R to IP3. Ca2+ pumps are used to return Calcium to the storage site.
The model equation is:
According to Atri et al., there are three binding domains on the IP3 receptor, the first of which binds IP3 and the other two bind Ca2+; when IP3 is linked to domain 1 Ca2+ is attached to domain 2, but Ca2+ is not bound to domain 3, the receptor merely passes the Ca2+ current. Consequently, Ca2+ binds to domain 2 of the receptor to activate it and to domain 3 to deactivate it. Based on functionality, each binding domain consists of a certain number of binding sites. Assuming domain independence, the steady-state Ca2+ flux through the IP3 receptor, J1, is given by:
Where in Equation 9 is the probability that IP3 is bound to domain 1, is the probability that Ca2+ is bound to domain 2 and 1; is the probability that Ca2+ is bound to domain 3; is a constant and represents the maximum total Ca2+ influx through the IP3 receptors.
Thus, if we let c denote [Ca2+] can P denote [IP3] then the following Equations 9–19 result:
Note that the expression of assumes that Ca2+ binds to the inactivating domain in a cooperative manner and while and are instantaneous functions of [Ca2+] and [IP3], acts on a slower time scale, therefore:
The dimensionless variable represents the proportion of IP3 that have not been closed by Ca2+ and it is described by:
is the steady-state value of as a function of the intracellular Calcium concentration c,
is the time constant for the dynamics of (Table 3).
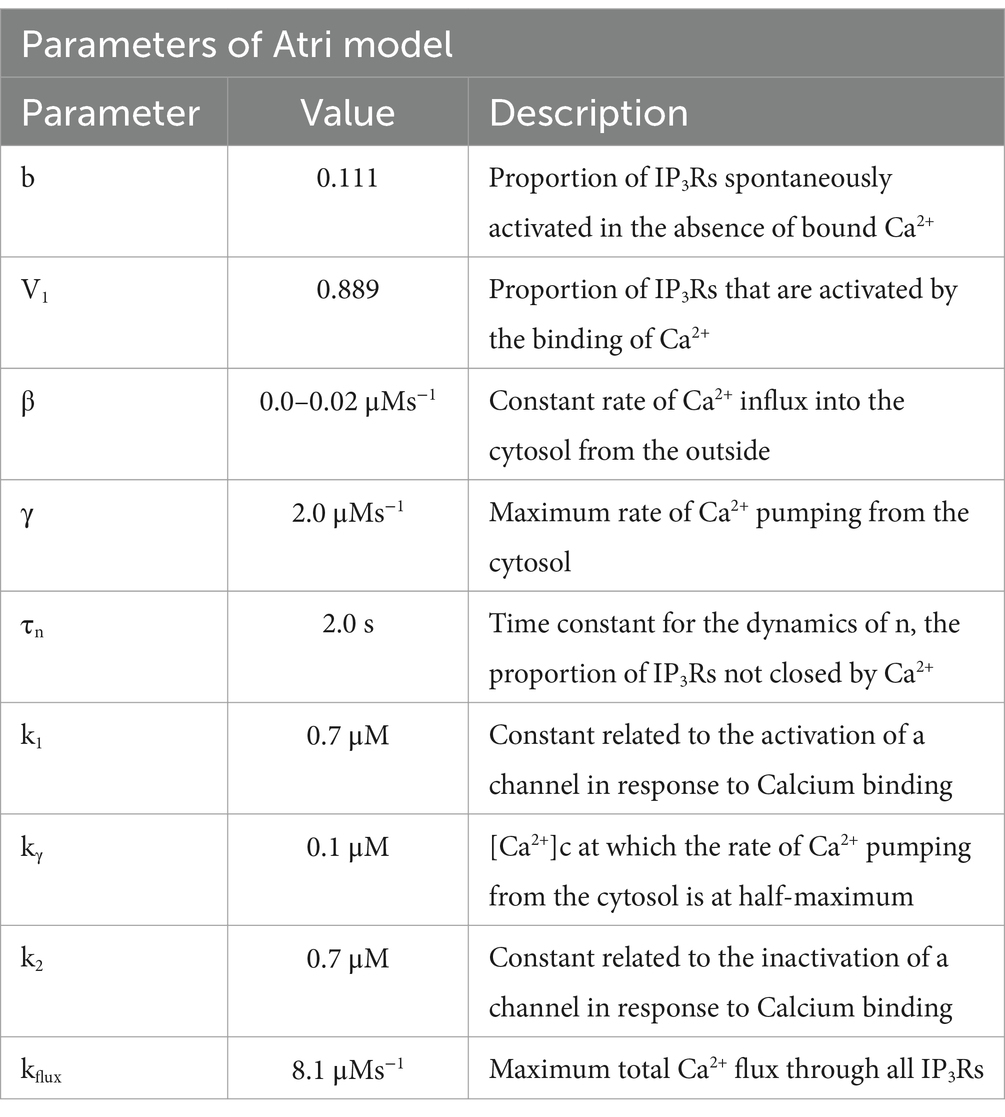
Table 3. Parameters of the Atri model (Atri et al., 1993).
The Li and Rinzel model
In 1994, Yue-Xian Li and John Rinzel deduced a model that reduces the De Young-Keizer model to a two-variable system to describe Calcium dynamics. This was mainly done by identifying the binding rates involving IP3 and activating Ca2+ molecules as faster rates than the binding rate of deactivating Ca2+. This made it possible to essentially split the model into two halves, with and without deactivating Ca2+ binding. The two dynamic variables of the LR model are the concentration of free cytosolic Ca2+ (C) and the fraction of open subunits of the inositol triphosphate receptor (h) (see Figure 6; Li and Rinzel, 1994); this result was obtained by using the method of multiple scales to solve the equations of the De Young-Keizer model on a succession of faster time scales to reduce it to a 2D system:
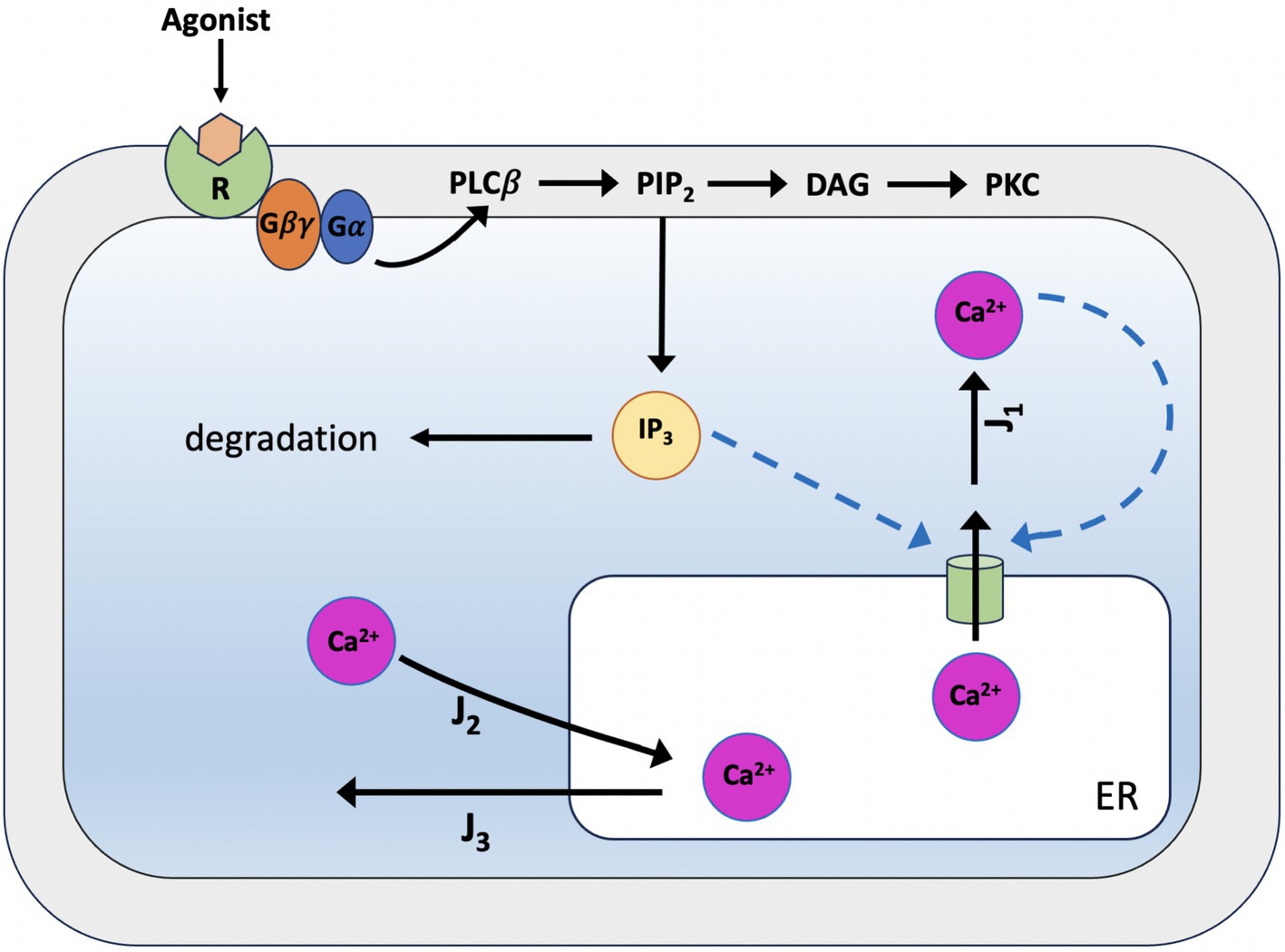
Figure 6. Schematic representation of Calcium dynamics according to the Li-Rinzel model. The model focuses on an individual cell situated within an extracellular environment devoid of Ca2+, thus negating the influx and efflux of Ca2+ through the cell membrane. Consequently, the intracellular Ca2+ dynamics are prompted by IP3, which is initially required to open the IP3 receptors on the ER membrane and prime the channels for Calcium-mediated feedback activation in the cytoplasm. Subsequently, the Calcium dynamics are governed by the interplay between Calcium-induced Calcium release (CICR), a non-linear amplification process regulated by the Calcium-dependent opening of channels to the ER’s Calcium stores, and the activity of the active SERCA pumps, which facilitate a reverse flow. Basal Ca2+ levels, on the other hand, are determined by the balance between a nonspecific passive loss of Ca2+ from the ER stores into the cytoplasm and the active uptake by SERCA pumps.
and
with J1, J2, and J3 given by the equations:
Were J1 is a release of Ca2+, mutually controlled by Ca2+ and by IP3 concentration; J2 is a passive loss of Ca2+ from the endoplasmic reticulum (ER) to the cytosol; and J3 an active absorption of Ca2+ in ER due to the action of the pumps. Again, in Equation 22, is the fraction of channel not yet inactivated by Ca2+.
Along with the gating variables:
Therefore, the level of IP3 is directly controlled by the signals affecting the cell from its external environment. In turn, the level of IP3 determines the dynamic behavior of the LR model. The Calcium signal can therefore be considered as coded information relating to the level of IP3 (Table 4).
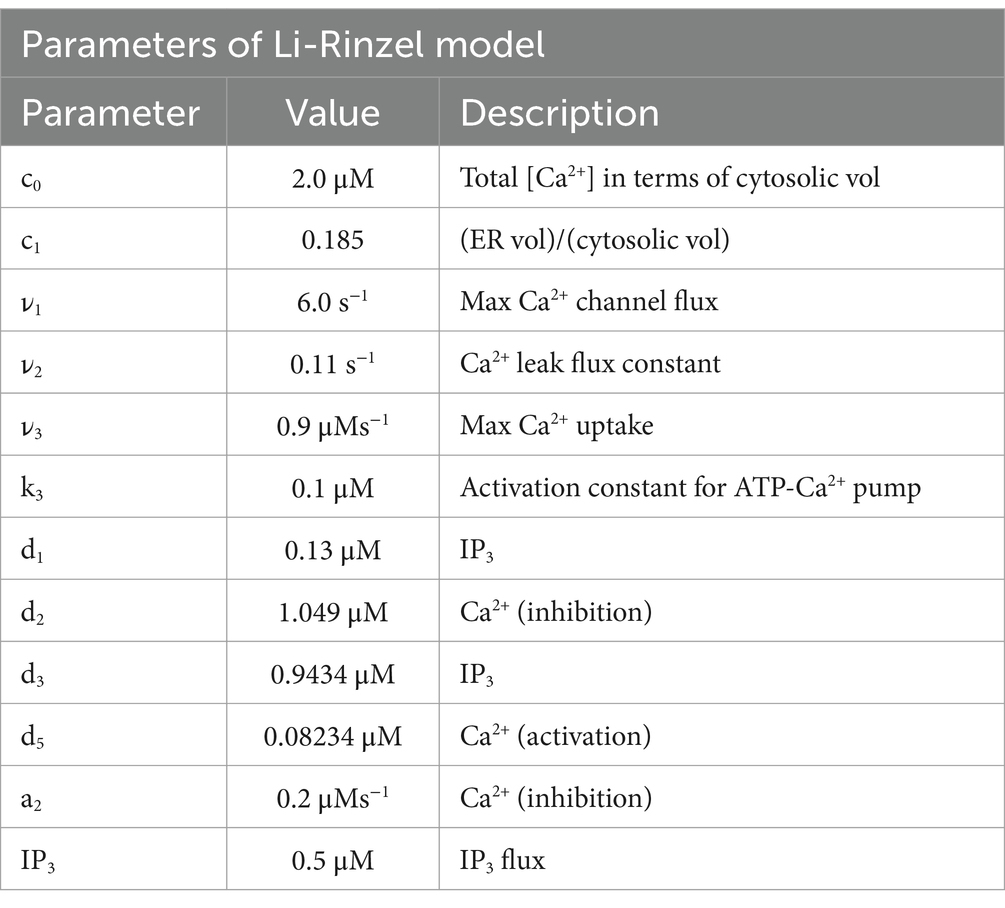
Table 4. Parameters of the Li-Rinzel model (Li and Rinzel, 1994).
Most models for Ca2+ dynamics are derived from the two-variable models mentioned so far. Since the realization of the pioneering models mentioned above, the intracellular dynamics of Ca2+ and IP3 have been characterized much more comprehensively, and above all, specific and more sophisticated models for intracellular and extracellular Ca2+ dynamics in astrocytes have been realized. When astrocytes respond to stimulation, they register a variety of spatiotemporal dynamics of Ca2+ elevation, each of which may have its own coding. Understanding the biophysical mechanisms underlying the rich Ca2+ dynamics in astrocytes is important because distinct coding patterns may correspond to different downstream signaling, including gliotransmission and consequently control of synaptic function.
More recently, models have also been created for subcellular Ca2+ increases linked to metabotropic glutamate receptors (mluRs). Here, the models offer the possibility of establishing a link between the properties of mGluRs and their implication in intracellular Ca2+ dynamics.
Glutamate is the most abundant excitatory neurotransmitter in the brain and plays a crucial role in various physiological processes, including learning, memory, and synaptic plasticity.
As demonstrated by electron microscopy the outer surface of the plasma membrane of astrocytes has specific receptors for glutamate. Smith et al. (2014) showed that cultured astrocytes responded to extracellular glutamate with rapid and oscillatory elevations of intracellular free Ca2+ concentration (Innocenti et al., 2000; Dani et al., 1992; Charles et al., 1991). In 1994, Mennerick and Zorumski experimentally demonstrated that astrocytes are able to uptake and transport 90% of glutamate from the extracellular space (Mennerick and Zorumski, 1994); Parpura and Haydon subsequently demonstrated that astrocytes modulate neuronal excitability through the release of glutamate linked to physiologically relevant increases in Ca2+ (Shao and Mccarthy, 1994; Parpura and Haydon, 2000).
Metabotropic glutamate receptors (mGluRs) are membrane proteins capable of responding to glutamate, the central nervous system’s main excitatory neurotransmitter as a result, they are crucial in the transmission of signals between cells in the nervous system. Research employing in situ hybridization and immunocytochemistry reveals that mGluR3 is the most often expressed mGluR subtype in glia (Smith, 1992; Petralia et al., 1996; Wroblewska et al., 1998). Astrocytes express Group I mGluR subtypes, which includes mGluR1 and 5, reviews can be found in Barres, 1991 (Barres, 1991; Steinhäuser and Gallo, 1996; Parpura et al., 1994; Testa et al., 1995; Miller et al., 1995; Winder and Conn, 1996; Hermans and Challiss, 2001; Verkhratsky et al., 1998; Biber et al., 1999; Cai et al., 2000; Aronica et al., 2003; Perea and Araque, 2007; Araque and Navarrete, 2010; Sun et al., 2013).
It is interesting to note that cell lines that express the mGluR5 receptor are the primary source of concurrent InsP3 and Ca2+ oscillations. These glutamate-induced Ca2+ oscillations have unusual characteristics, so it is plausible that different oscillatory mechanisms prevail depending on the receptor type (Kummer et al., 2000; Lemon et al., 2003; De Pittà et al., 2009).
When glutamate binds to its membrane receptor, a sequence of events is set off: the ethorotrimeric G-protein, which is named for its three distinct polypeptide subunits, α, β, and γ, interacts with the receptor to create a receptor-G-protein complex on the inner membrane surface. When the α subunit interacts with the receptor, it undergoes a conformational shift that releases the GDP attached to it and replaces it with GTP. This, in turn, activates the phospholipase C-β (PI-PLCβ) that is specific to phosphatidyl-inositol. PI-PLCβ is located on the inner surface of the membrane, linked to the interaction between its PH domain and a PIP2 molecule immersed in the bilayer. The PI-PLCβ enzyme catalyzes a reaction that cleaves PIP2 into two molecules, inositol 1,4,5-triphosphate (IP3) and diglycerol (DAG). The resultant IP3 molecules diffuse into the cytoplasm and attach to a particular IP3 receptor found on the smooth endoplasmic reticulum surface (Rosa et al., 2022). DAG stimulates PKC activity, which in turn phosphorylates the mGlu5 receptor at Ser-839. This phosphorylation leads to the uncoupling of the receptor from the G protein signaling cascade.
Modeling studies have not always been conducted in tandem with experimental research on mGlur receptor-mediated Ca2+ signaling; although mGlur receptors are highly expressed in the central nervous system (CNS) and have been linked to several pathophysiological processes as well as neuro-psychiatric disorders (Nicoletti et al., 2011; Spooren et al., 2001).
The De Pittà model
Young et al. (1992), Li and Rinzel (1994), and Höfer et al. (2002) models as a starting point, in De Pittà et al. (2009) constructed a generic model for glutamate-induced Ca2+ (Glu) dynamics in astrocytes, including additional biochemical processes relevant for a more realistic description of astrocyte activity. Such extensions include the production and degradation of IP3 within the astrocyte cell, mediated by two membrane-associated enzymes, PLCβ and PLCδ (see Figure 7). Later, De Pittà and Berry (2019) further refined their model by focusing on the rate of IP3 production following activation of glutamate receptors mGluRs, building a new model. The De Pittà model for IP3/Ca2+ signaling is constituted by three ODES, respectively, for intracellular Ca2+ (C), the IP3R gating (h), and the mass balance equation for intracellular IP3 lumping terms. Regarding the differential equations for the variables C and h above, the De Pittà model considers the original Li-Rinzel model formulation described for the CICR and provides a more detailed description of IP3 production and degradation, proposing a three-variable model for glutamate-induced intracellular Calcium dynamics caused by synaptic activity in astrocytes.
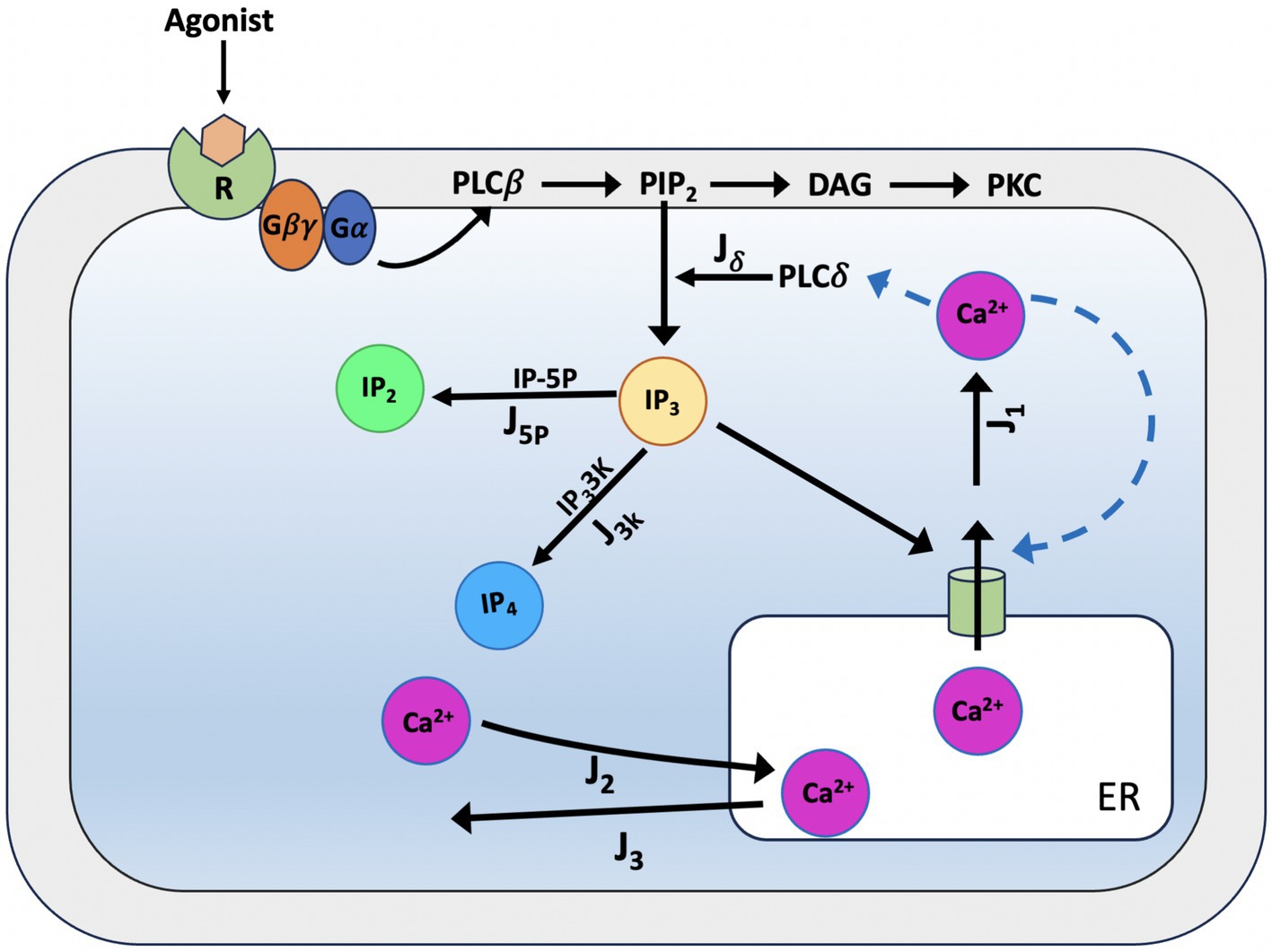
Figure 7. Schematic representation of Ca2+ dynamics and IP3 production according to the De Pittà model. When glutamate binds to metabotropic glutamate receptors (mGluR1/5), the receptor activates a Gq protein, which subsequently stimulates phospholipase C-beta (PLC-β). PLC-β hydrolyses phosphatidylinositol 4,5-bisphosphate (PIP2) into two second messengers: inositol 1,4,5-trisphosphate (IP3) and diacylglycerol (DAG). IP3 diffuses into the cytoplasm and binds to endoplasmic reticulum (ER) receptors (Jδ), triggering the release of Calcium ions (Ca2+) and initiating downstream cellular responses. IP3 can then be degraded by IP3-3-kinase (IP3-3 K) (J3K) to inositol 1,3,4,5-tetrakisphosphate (IP4) or by inositol polyphosphate 5-phosphatase (IP-5P) (J5p) to inositol 1,4-bisphosphate (IP2), modulating the signaling cascade. For the CICR, the model considers the original formulation of the Li-Rinzel model (Li and Rinzel, 1994).
In astrocytes, IP3 together with diacylglycerol (DAG) is produced by hydrolysis of phosphatidylinositol 4,5-bisphosphate (PIP2) by two phosphoinositide-specific phospholipase C (PLC) isoenzymes, PLCβ and PLCδ (Rebecchi and Pentyala, 2000). PLCβ is primarily controlled by cell surface receptors; hence, its activity is linked to the level of external stimulation (i.e., the extracellular glutamate) and as such, it pertains to the glutamate-dependent IP3 metabolism. PLCδ is the enzyme responsible of endogenous IP3 production in astrocytes, it is essentially activated by increased intracellular Ca2+ levels (Rhee and Bae, 1997). The model proposed for PLCδ -mediated IP3 production (Jδ) (Equation 29) derived from structural and mutational studies (Höfer et al., 2002; Pawelczyk and Matecki, 1998).
where Oδ is the maximal rate of IP3 production by PLCδ and Kδ is the inhibition constant of PLCδ activity. According to experiments, PLCδ activity is inhibited by high IP3 concentrations (> 1 μM) because they compete with PIP2 for the enzyme’s binding (Allen and Barres, 2009).
In astrocytes there are two several pathways for IP3 degradation: the dephosphorylation of IP3 by inositol polyphosphate 5-phosphatase (IP-5P), and the phosphorylation of phosphorylation of IP3 by the IP33-kinase (IP3-3 K). For the description of the two IP3 degradation dynamics we use the relations given by Equations 30, 31:
where O5p is the maximal rate of IP-5P mediated IP3 degradation in the linear approximation.
For IP3-3 K degradation we can write:
where O3k is the maximal rate of IP3 degradation by IP3-3 K.
In summary, the De Pittà model of Ca2+ dynamics with endogenous IP3 metabolism (Equation 32) is based on the two LR equations but the IP3 concentration (I) is now provided by a third coupled differential Equations 20a, 20, 21, 21b, 33.
The model highlights the complex biochemical reactions coupled with Ca2+ dynamics via the different second messengers (Table 5).
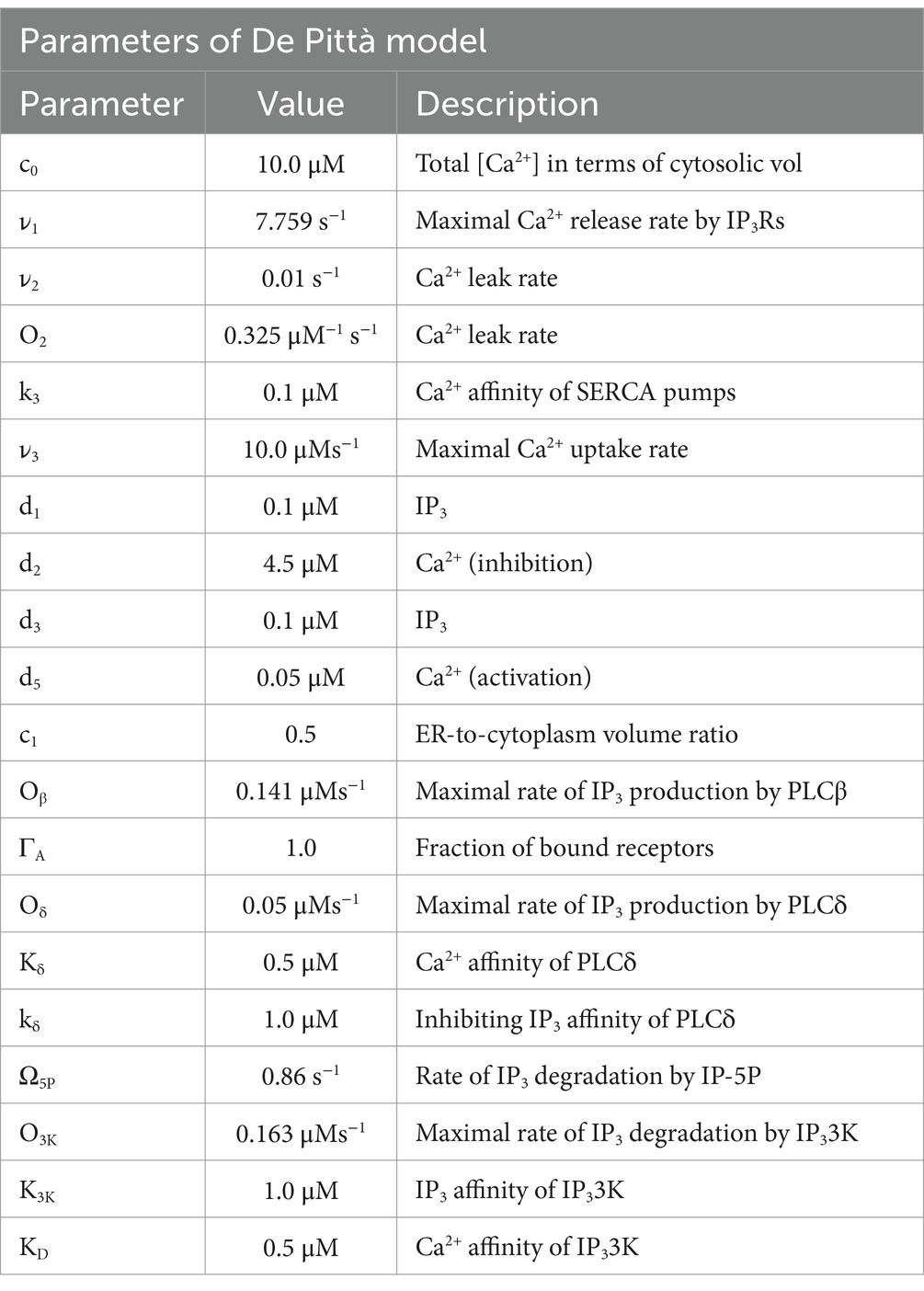
Table 5. Parameters of the De Pittà model (De Pittà and Berry, 2019).
Data sources
For the acquisition of experimental data, the methodology described in the article “Dynamics of Astrocytes Ca2+ Signaling: A Low-Cost Fluorescence Customized System for 2D Cultures” was adopted (Musotto et al., 2024), this study provides temporal and spatial data of Calcium signaling in astrocytes using an innovative and inexpensive fluorescence imaging system designed for two-dimensional (2D) cell cultures. The analysis was performed on immortalized human astrocytes, the raw data for all cells in the well analyzed are shown in Figure 8.
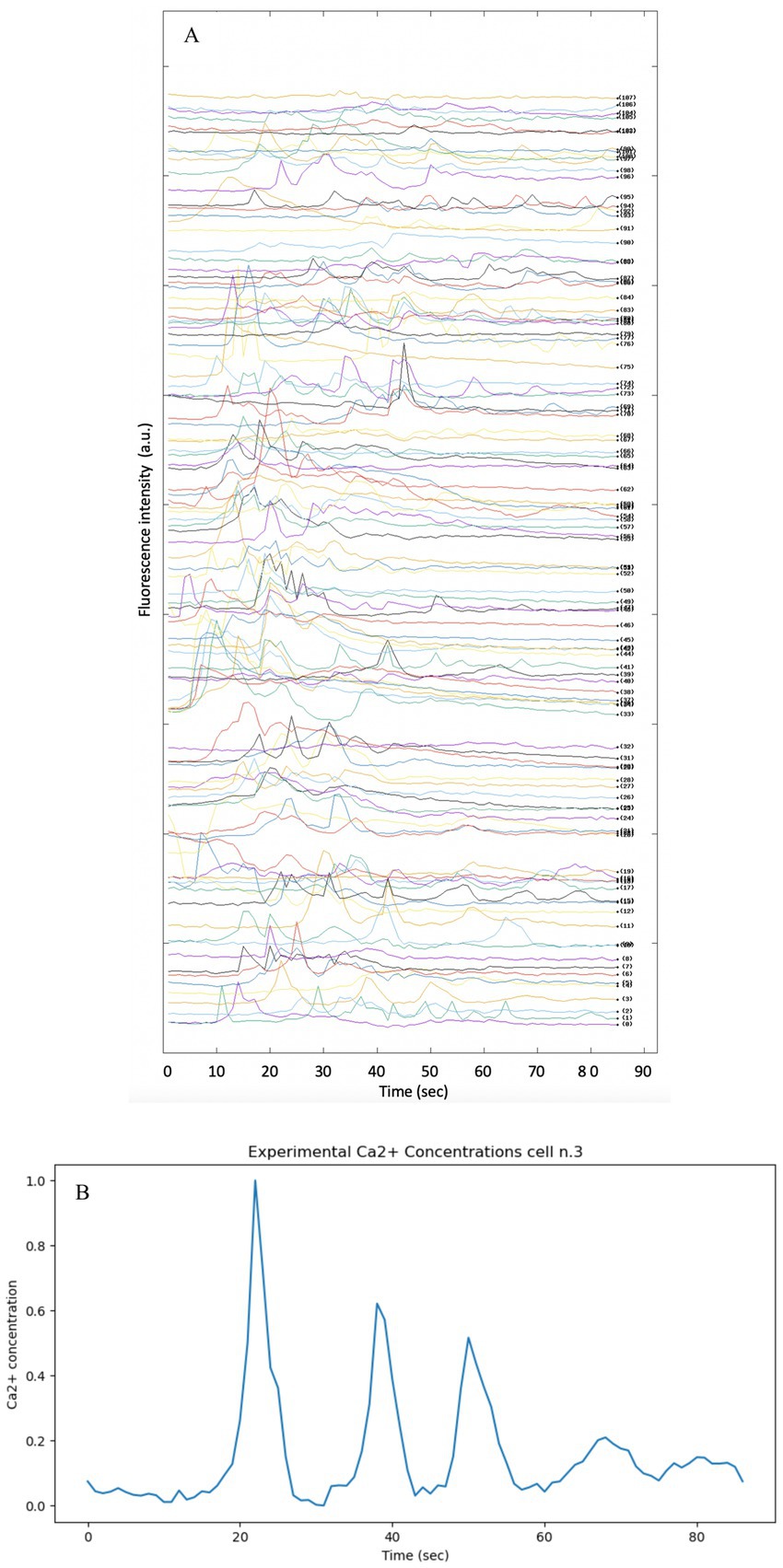
Figure 8. Fluorescence intensity profiles showing the spontaneous activity of intracellular Ca2+ in immortalized human astrocytes. The methodology and instrument described in Musotto et al. (2024) was used to produce the experimental data. (A) Split image of each Ca2+ cell present in the well under analysis. The Ca2+ signal of each cell present in the well was split in order to provide all data simultaneously. (B) Intensity vs. time profile of Ca2+ fluorescence intensity in cell n.3.
The background was subtracted from the raw data and normalized by calculating the change in fluorescence (ΔF) from baseline fluorescence (F₀) (Wamhoff et al., 2002). This normalization process is essential to ensure that the data reflect true physiological changes rather than artifacts introduced by variable dye loading.
In order to visualize the variables on different scales and to facilitate comparison between theoretical and experimental data, all data were scaled by the min-max normalization method in the range [0,1]. In order to compare the theoretical Ca2+ signal obtained from the models reported in the article, cell no. Three was chosen arbitrarily (see Figure 8B)
Comparing model predictions with experimental data makes it possible to assess the accuracy and reliability of models, identify discrepancies and refine models accordingly.
Results
The Goldbeter model
The Goldbeter model is known to describe intracellular Calcium oscillations, which in many biological situations exhibit regular and periodic behavior, but is highly sensitive to the parameters that govern it; in this form, it appears to be insufficient to explain the experimental data on Ca2+ dynamics in astrocytes. The theoretical model, as reported in the original article, describes the Calcium dynamics over a shorter time interval (10 s), while the experimental data cover a longer period (87 s). By extending the integration time of the model to 87 s, so as to be comparable with the experimental time, it can be observed that the Z oscillations persist throughout the interval with a fairly stable amplitude and frequency. The oscillations do not disappear and the system does not converge to a static equilibrium, but seems to maintain a repetitive oscillation pattern. The pattern is set to produce sustained oscillations that continue for longer times. The parameters of the pattern determine how fast Calcium enters, is released and is removed from the various compartments of the cell. To adapt the model to the much slower experimental Ca2+ dynamics, the model parameters must be modified. The experimental data provided show less regular behavior and more unpredictable amplitude variations. The large differences observed suggest that the actual biological system is more complex and requires optimization of model parameters or more refined modeling (Figure 9).
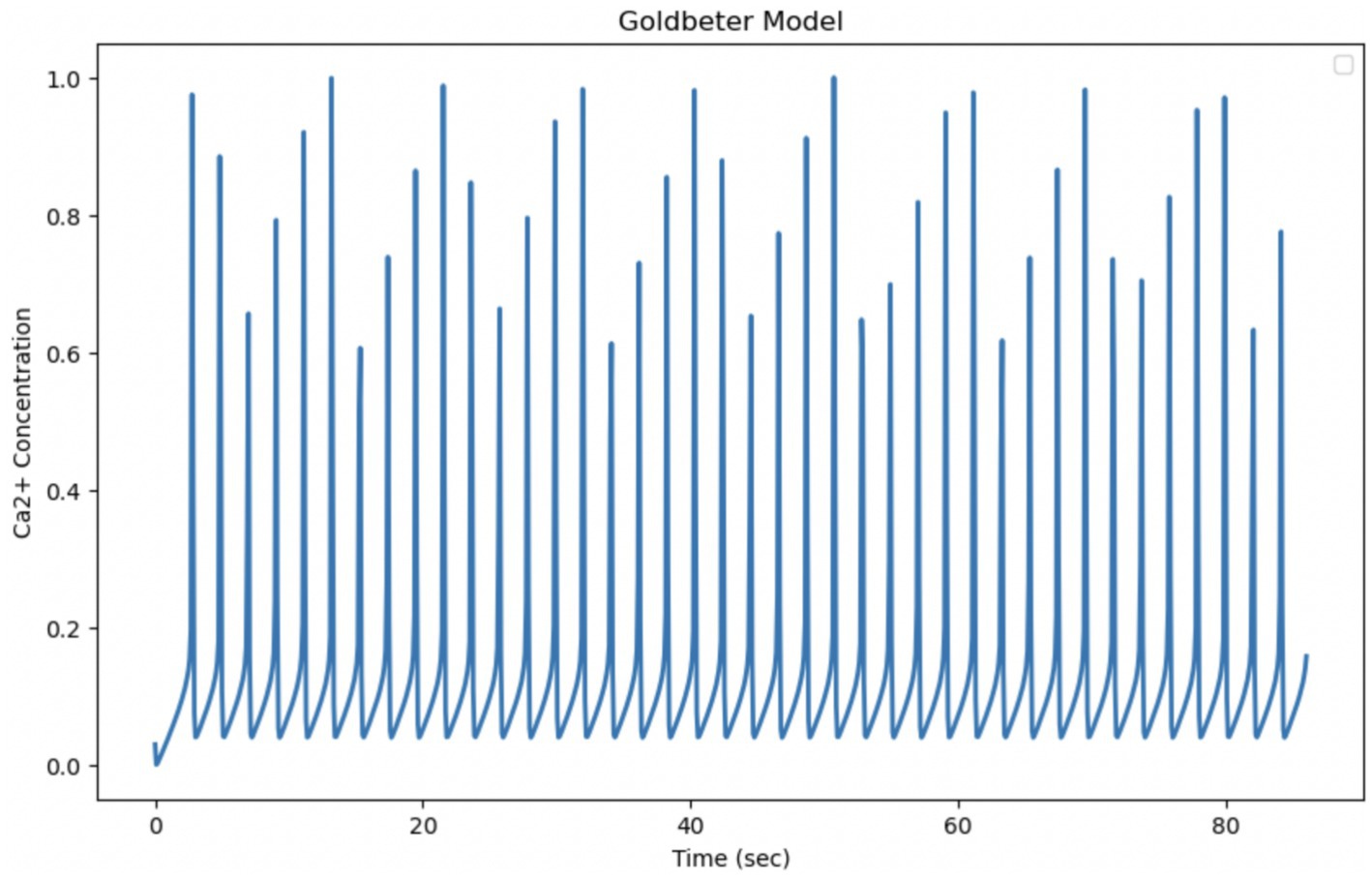
Figure 9. Simulation of the Golbeter model (Goldbeter et al., 1990). Curves obtained by integrating Equations 1 and 2 with the parameters shown in Table 1. Fluctuations of cytosolic Ca2+ concentration in 87 s, a time comparable to the experimental observation time. Goldbeter et al. obtained the reported fluctuations with an external stimulation β of 30.1%.
The Atri model
The Atri model is based on a simplified system of differential equations that mainly considers the release and pumping of intracellular Calcium. By extending the simulation of the model to make it temporally comparable with experimental data, whose observation time is equal to 87 s, it can be seen that the oscillations are regular, with stable amplitude and average frequency. The experimental data, on the other hand, show changes in the behavior of Calcium over a period of 87 s, with an initial activation phase, a maximum peak, and a subsequent decline. This indicates that the biological system may have richer temporal dynamics that the model cannot fully reproduce. These discrepancies suggest that the model, in its current form, fails to fully capture the complexity of the experimental behavior of intracellular Calcium in astrocytes. A key factor in the Atri model is the gating variable n, which regulates the opening of Calcium release channels. This variable introduces a feedback mechanism that can influence the frequency of oscillations, making the model more flexible with respect to the timing of oscillation (Figure 10).
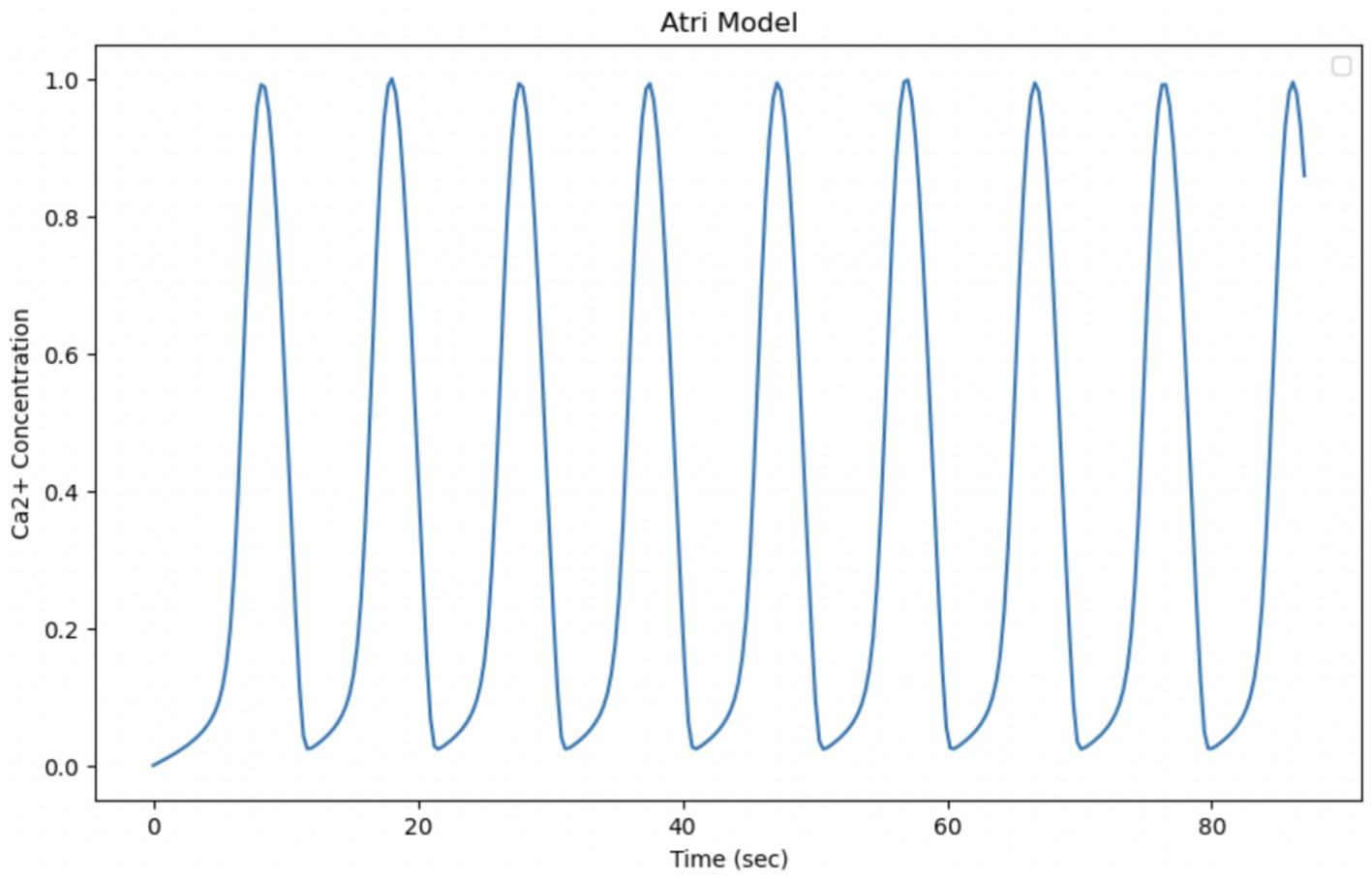
Figure 10. Simulation of the Atri model. Curves obtained by integrating Equations 8, 19 with the parameters shown in Table 3. Oscillation of cytosolic Ca2+ concentration in 87 s, time comparable with experimental observation time.
The Li-Rinzel model
The Li-Rinzel model originates from a reduction of the more complex model of De Young and Keizer, with the aim of simplifying the description of intracellular Calcium oscillations while maintaining the ability to reproduce experimentally observed phenomena. The model is particularly useful for describing the regulation of Calcium release via IP₃ receptors in the endoplasmic reticulum. It explicitly introduces the Calcium concentration in the endoplasmic reticulum as a dynamic variable, which makes it more detailed in its description of the Calcium release and reabsorption cycle and capable of reproducing more regular and structured oscillations than simpler models. The ability of the model to generate slow Ca2+ input-dependent oscillations, as in Figure 5 of the article “Equations for InsP, Receptor-mediated [Ca2+], Oscillations Derived from a Detailed Kinetic Model: A Hodgkin-Huxley Like Formalism,” makes it more suitable for comparison with our experimental data on Ca2+ signaling in astrocytes. However, the regularity of oscillations predicted by the model may be less realistic than experimentally observed oscillations, which tend to be more irregular and less predictable (Figure 11).
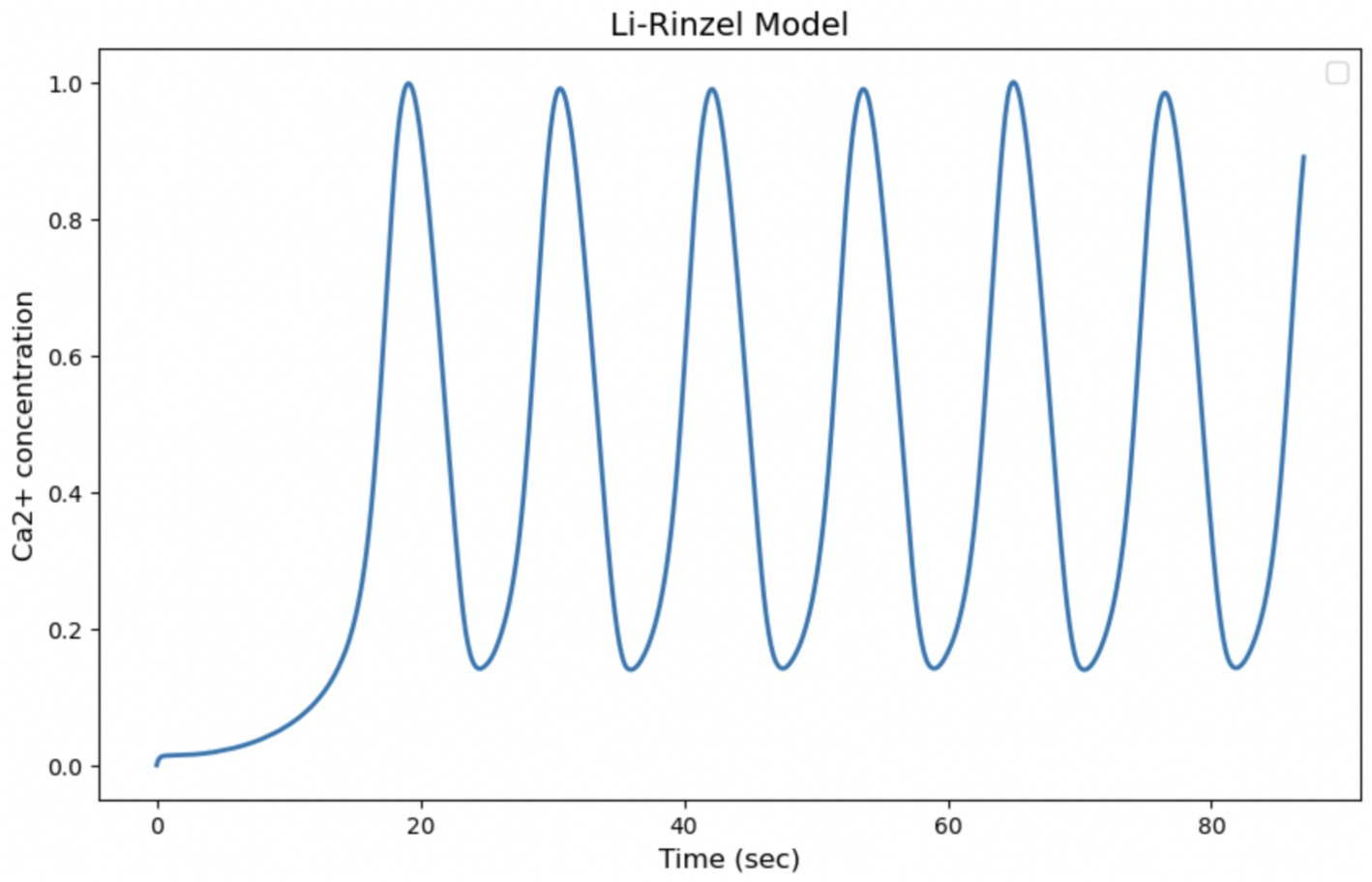
Figure 11. Simulation of the Li-Rinzel model. Curves obtained by integrating Equations 20, 21 with the parameters shown in Table 4. Oscillation of cytosolic Ca2+ concentration in 87 s, time comparable with experimental observation time.
The De Pittà model
The De Pittà model is a powerful tool to describe intracellular Calcium oscillations regulated by G-protein-coupled receptors. In the model, G-protein-coupled receptors, when activated, induce the release of IP₃, which in turn stimulates the release of Calcium from the endoplasmic reticulum. The released Calcium can further activate Calcium release channels through the process of induced Calcium release (CICR), creating positive feedback. Like many other Calcium oscillation models, De Pittà includes positive feedback (via CICR) and negative feedback (via Calcium reabsorption in the endoplasmic reticulum or degradation of the IP₃ signal). These mechanisms are crucial for the generation of regular oscillations. Although it provides a realistic description of IP3 and CICR mediated Calcium release, it has some limitations compared to experimental data, particularly with regard to its ability to capture the irregularity and variability of Calcium oscillations. The experimental data show much more dynamic and complex behavior, with significant variations in amplitude and frequency that the model does not fully reproduce in its current form. In order to have a better fit to the experimental data, the parameters could be calibrated. Optimization of Calcium release and absorption rates, as well as IP₃ dynamics, could improve the fit of the model (Figure 12; Table 6).
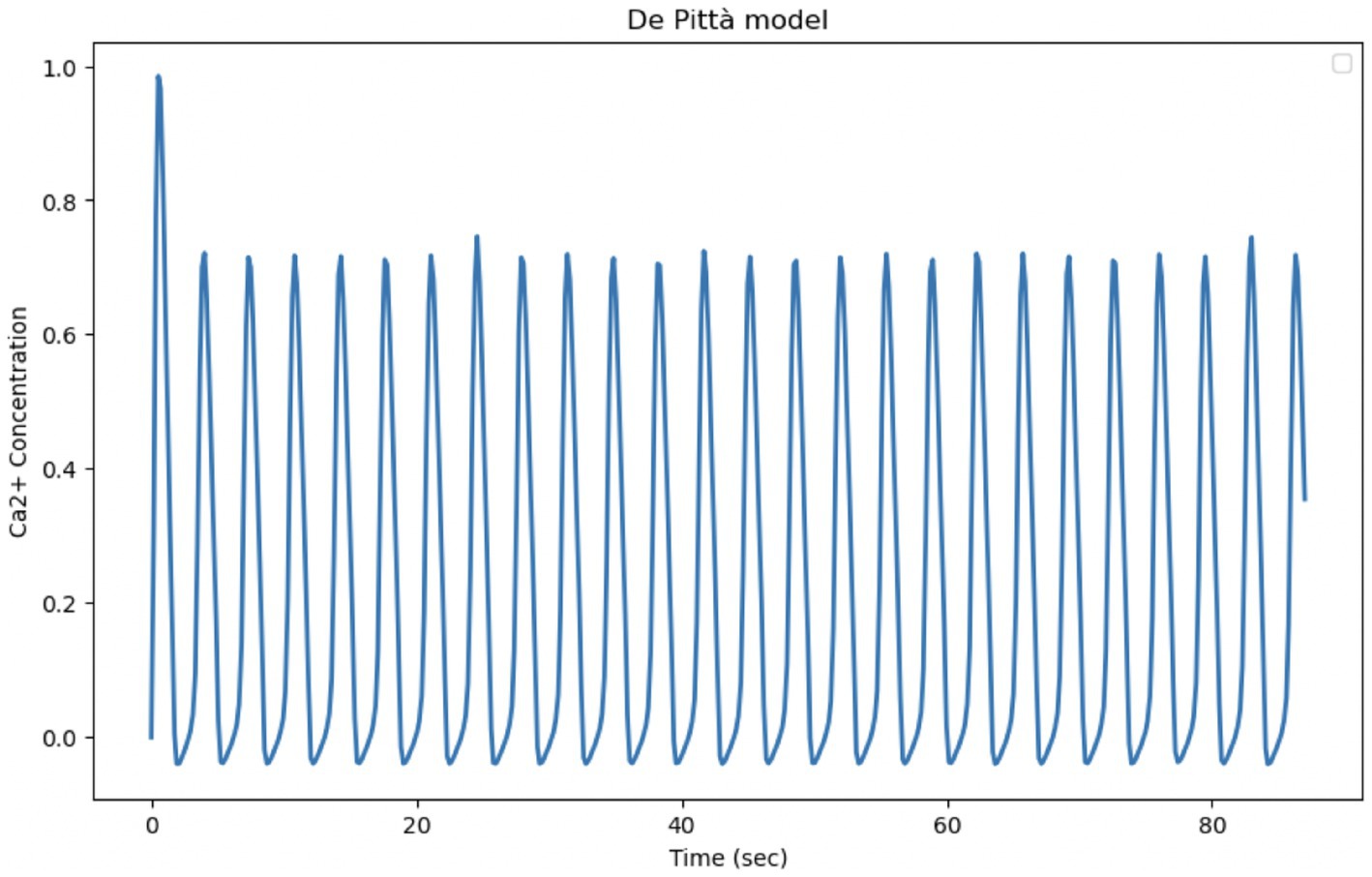
Figure 12. Reproduction of the De Pittà model. Curves obtained by integrating Equations 20, 21 of the Li-Rinzel model relating to Calcium-induced Calcium dynamics (CIRC) with Equation 31 relating to IP3 production and degradation. The model parameters are shown in Table 4. Oscillation of cytosolic Ca2+ concentration of the model in 87 s, time comparable with experimental observation time.
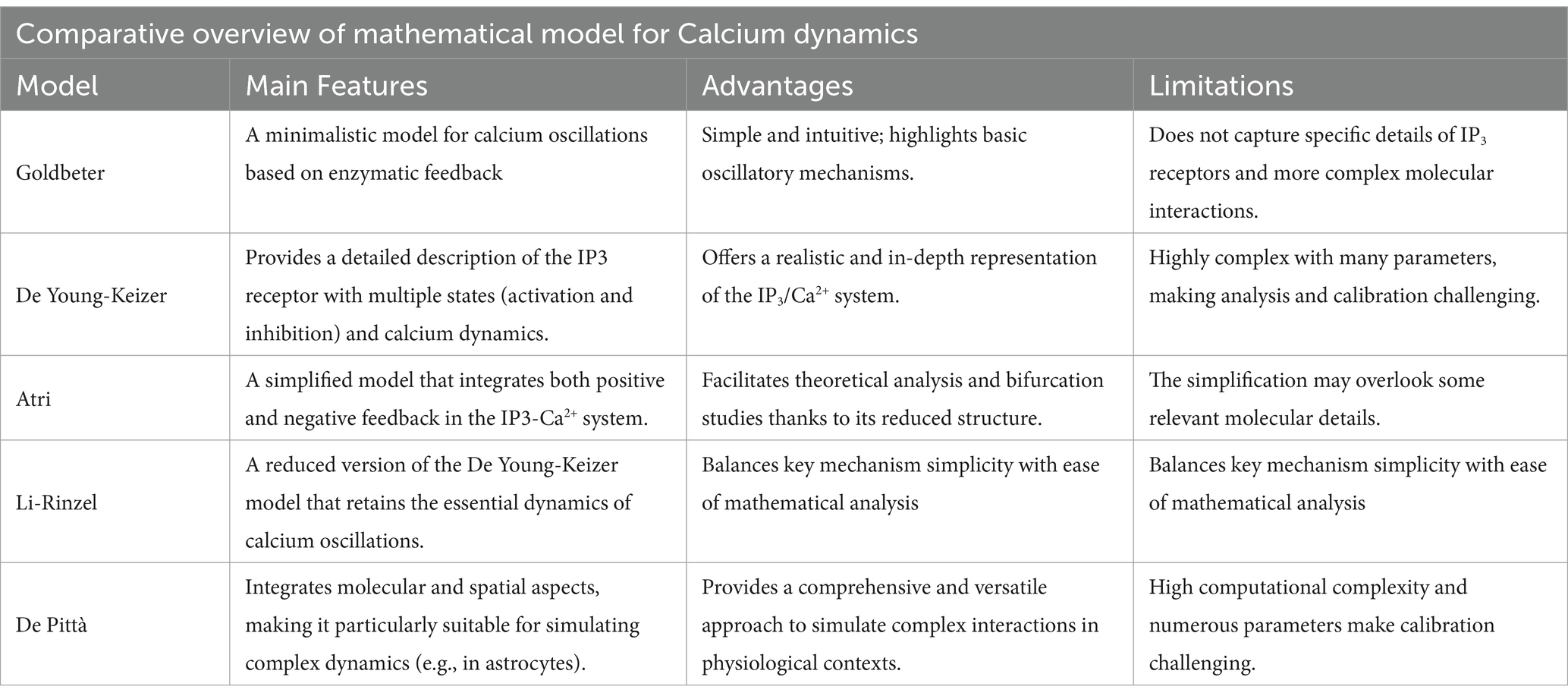
Table 6. This table summarizes the main features, advantages, and limitations of the Goldbeter, De Young-Keizer, Atri, Li-Rinzel, and De Pittà models, providing with a quick reference to understand the merits and constraints of each modeling approach.
Discussion
Over the past 20 years, many computational models for intracellular Ca2+ dynamics have been developed. They differ according to the level of description, from the microscopic level, for which stochastic models must be used, to the macroscopic level, which requires deterministic models. In this review, five models of intracellular Ca2+ dynamics were evaluated (Goldbeter et al., 1990; Young et al., 1992; Atri et al., 1993; Li and Rinzel, 1994; De Pittà and Berry, 2019), implementing the equations based on what was presented in the original publications. Our aim was to reproduce the simulation results of the original articles and compare them with the experimental data in our possession (Musotto et al., 2024) in order to determine which model was most suitable.
The aim of the mathematical models analyzed in this contribution is to interpret the emergence of complex intracellular Calcium dynamics as the result of interdependent Ca2+ fluxes between the cytosol and intracellular stores, driven by the interaction with IP3. The models are described by systems of non-linear ordinary differential equations (ODEs), which are capable of supporting self-sustained Calcium oscillations. These phenomenological models have been developed to reproduce Calcium flow behavior comparable with available experimental data and have played a crucial role in the advancement of neuroscience, serving as a bridge between experimental observations and the development of more in-depth theories. All models discussed here are described by deterministic equations, meaning that the effects of stochastic fluctuations due to microscopic inhomogeneities and noise due to spatial localization or random fluctuations are neglected. Indeed, one of the limitations of deterministic models is that they produce oscillations that are too regular compared to those observed experimentally. The addition of stochastic components could improve the models’ ability to fit the experimental data. It has been shown that IP3 channels are distributed in clusters on the ER membrane, generating Ca2+ signals on multiple scales, ranging from local puffs to global intra- and extracellular waves. It should be pointed out that our observation of intracellular Ca2+ dynamics in astrocytes is given by whole-cell oscillations. These signals are believed to include release from the multiple compartmentalized processes within the cell (Bindocci et al., 2017; Smith and Parker, 2009) that give rise to the observed global Ca2+ oscillations.
From the study of the models reported in this paper, a common problem emerges: the period of intracellular Ca2+ fluctuations are faster than that observed experimentally. The period of Calcium fluctuations in astrocytes is generally slower, often occurring within seconds or minutes. Research indicates that Calcium signals in astrocytes can be attributed to delayed release from internal stores, leading to slower kinetics than in neuron (Ma et al., 2021). Furthermore, astrocyte Calcium transients can be influenced by various signaling pathways, including those mediated by inositol trisphosphate (IP3) and ryanodine receptors, which contribute to the complexity and variability of astrocyte Calcium dynamics (Stobart et al., 2018; Corkrum et al., 2019). The frequency and duration of these Calcium events can vary significantly depending on the physiological state of the astrocytes and the surrounding neuronal activity (Schnell et al., 2011; Mcdougal et al., 2013). In summary, while Calcium fluctuations in some cells and neurons are rapid and occur on the millisecond scale, astrocyte Calcium signaling operates on a slower time scale, typically between seconds and minutes, with the possibility of intercellular propagation of Calcium waves. Of the five models studied, only four were implemented, as the Li-Rinzel model is a simplification of the De Young-Keizer model and it was decided not to simulate the latter because it was too computationally expensive. The models analyzed in this review were simulated using the parameters reported in the original studies. Each model has unique mechanisms and parameters that influence the dynamics of Ca2+ signaling. A general comparison of the four implemented models shows that they have different abilities to modulate Ca2+ frequencies, varying in complexity and adaptability. Goldbeater model generates constant frequencies that depend on the parameters of Ca2+ release and accumulation without direct influence from IP3. This model is suitable for constant and rhythmic cellular responses. In Atri model, the frequency of oscillations varies depending on the levels of IP3 and the rate of binding of IP3 to its receptors. The oscillations are influenced by spatial diffusion, which allows variable frequencies and the formation of Ca2+ waves ideal for complex communications between different cellular regions. In Li and Rinzel model, the frequency of Ca2+ oscillations are dependent on the concentration of IP3. As IP3 increases, the frequency of Ca2+ oscillations also increase. This model is used to analyze cellular responses that must vary gradually with external stimuli. The most complex of the models analyzed, the G-ChI model, describes the Ca2+ frequency as a result of the dynamic interaction between Ca2+, IP3, and GPCR receptors. Oscillations in this model respond to different synaptic stimuli, with frequencies modulated by enzymes such as PLC and PKC. It therefore allows a highly adjustable frequency, ideal for neurobiological functions in astrocytes.
In the present contribution, a comparison of five models representing different modeling approaches, with the aim of identifying which of these bests fit our experimental data. The selected model will be used as a basis for developing a model with a structure derived from the application of physical principles as in Gawthrop and Crampin (2017). Considering the heterogeneity of astrocytes reported in the literature (Khakh and Sofroniew, 2015), future extensions of the model should include parameters representing the phenotypic and functional variability of the cells to obtain simulations that better reflect the complexity of astrocytic responses observed experimentally. Moreover, since Ca2+ puffs (irregular) and oscillations (much more regular) can be observed in the same cell for different stimulus levels, the study of Ca2+ dynamics offers the fascinating possibility of studying the transition from a stochastic to a deterministic regime.
It should also be pointed out that although in vitro models allow us to gain insight into the cellular mechanisms underlying Ca2+ regulation, they tend to simplify the cellular environment by isolating astrocytes from other cell types and their natural interactions. Indeed, as pointed out by Stogsdill et al. (2023), astrocytes are an integral part of complex neural networks, and their Ca2+ activity is influenced by signals from neurons and other glial cells. The use of computational models built by integrating both in vitro and in vivo experimental data allow a better understanding of Ca2+ signaling and its role in neuronal functions (Manninen et al., 2018).
Conclusion
The article reports the findings of a comparative study of some of the most significant models for Calcium dynamics in astrocytes. The evolution from minimal models to more complex models, that consider additional biochemical processes for a more realistic description of astrocyte activity, is discussed. We compared mathematical models and experimental data of Ca2+ in astrocytes. The experimental data reveal complex oscillatory dynamics with different frequencies and amplitudes, reflecting the intricate regulatory mechanisms of Ca2+ signaling in astrocytes. Our analysis shows that the Goldbeter model, although effective in generating stable oscillations, does not allow to capture the variability in measured frequency and amplitude. The Atri model introduces a spatial wave dynamic, which could mimic some variations in the dynamics of experimental oscillations, but fails to reproduce the full range of dynamic behavior. The Li-Rinzel model, through IP3-dependent modulation, provides a closer approximation of the experimental data, allowing for frequency adjustments; however, it remains limited in capturing amplitude variability. The De Pittà model, on the other hand, aligns more closely with experimental observations, as it incorporates detailed GPCR and enzymatic feedback mechanisms that allow for both frequency and amplitude modulation. This latter model successfully replicates the observed changes in Ca2+ dynamics, making it suitable for studying the role of astrocytes in neural signaling and synaptic regulation.
It is important to note that astrocytes exhibit remarkable heterogeneity in their morphology, molecular expression, and functional responses, which varies across different brain regions and microcircuits (Khakh and Sofroniew, 2015). Incorporating this cellular diversity into computational models could enhance their ability to accurately reflect the range of astrocytic calcium dynamics observed experimentally.
Mathematical modeling of Ca2+ signaling in astrocytes has emerged as a critical tool for understanding the complex dynamics of the glial cells in the central nervous system. These models help elucidate the mechanisms by which astrocytes respond to neuronal activity and maintain homeostasis through Calcium signaling.
In summary, mathematical models of Ca2+ signaling in astrocytes are essential for deciphering the complex interactions between astrocytes and neurons. These models not only improve our understanding of normal physiological processes, but also provide a framework for studying the altered Calcium dynamics associated with various neurological disorders. Future research using these models will likely continue to reveal the intricate role of astrocytes in brain function and their potential as therapeutic targets in neurodegenerative diseases.
Author contributions
RM: Conceptualization, Data curation, Formal analysis, Funding acquisition, Investigation, Methodology, Project administration, Resources, Software, Supervision, Validation, Visualization, Writing – original draft, Writing – review & editing. UW: Data curation, Supervision, Writing – review & editing. GP: Resources, Supervision, Validation, Visualization, Writing – review & editing.
Funding
The author(s) declare that financial support was received for the research and/or publication of this article. This research was funded by the project “NeuroSuite: Un nuovo sistema intelligente e predittivo per il supporto alla decisione clinica nelle neuro-fragilità” (Cod. F/350230/01-05/X60), supported by the Italian Ministry of Enterprises and Made in Italy (Ministero delle Imprese e del Made in Italy).
Conflict of interest
The authors declare that the research was conducted in the absence of any commercial or financial relationships that could be construed as a potential conflict of interest.
Generative AI statement
The author(s) declare that no Gen AI was used in the creation of this manuscript.
Publisher’s note
All claims expressed in this article are solely those of the authors and do not necessarily represent those of their affiliated organizations, or those of the publisher, the editors and the reviewers. Any product that may be evaluated in this article, or claim that may be made by its manufacturer, is not guaranteed or endorsed by the publisher.
References
Allen, N. J., and Barres, B. A. (2009). Glia more than just brain glue. Nature 457, 675–677. doi: 10.1038/457675a
Anderson, C. M., and Swanson, R. A. (2000). Astrocyte glutamate transport: review of properties, regulation, and physiological functions. Glia 32, 1–14. doi: 10.1002/1098-1136(200010)32:1<1::AID-GLIA10>3.0.CO;2-W
Araque, A., and Navarrete, M. (2010). Glial cells in neuronal network function. Philos. Trans. R. Soc. B Biol. Sci. 365, 2375–2381. doi: 10.1098/rstb.2009.0313
Araque, A., Sanzgiri, R. P., Parpura, V., and Haydon, P. G. (1999). Astrocyte-induced modulation of synaptic transmission. Can. J. Physiol. Pharmacol. 77, 699–706. doi: 10.1139/y99-076
Aronica, E., Gorter, J. A., Ijlst-Keizers, H., Rozemuller, A. J., Yankaya, B., Leenstra, S., et al. (2003). Expression and functional role of mGluR3 and mGluR5 in human astrocytes and glioma cells: opposite regulation of glutamate transporter proteins. Eur. J. Neurosci. 17, 2106–2118. doi: 10.1046/j.1460-9568.2003.02657.x
Atri, A., Amundson, J., Clapham, D., and Sneyd, J. (1993). A single-pool model for intracellular calcium oscillations and waves in the Xenopus laevis oocyte. Biophys. J. 65, 1727–1739. doi: 10.1016/S0006-3495(93)81191-3
Backus, K. H., Kettenmann, H., and Schachner, M. (1989). Pharmacological characterization of the glutamate receptor in cultured astrocytes. J. Neurosci. Res. 22, 274–282. doi: 10.1002/jnr.490220307
Barres, B. A. (1991). Glial ion channels. Curr. Opin. Neurobiol. 1, 354–359. doi: 10.1016/0959-4388(91)90052-9
Berridge, M. J., and Irvine, R. F. (1989). Inositol phosphates and cell signalling. Nature 341, 197–205. doi: 10.1038/341197a0
Biber, K., Laurie, D. J., Berthele, A., Sommer, B., Tölle, T. R., Gebicke-Härter, P. J., et al. (1999). Expression and signaling of group I metabotropic glutamate receptors in astrocytes and microglia. J. Neurochem. 72, 1671–1680. doi: 10.1046/j.1471-4159.1999.721671.x
Bindocci, E., Savtchouk, I., Liaudet, N., Becker, D., Carriero, G., and Volterr, A. (2017). Three-dimensional Ca2+ imaging advances understanding of astrocyte biology. Science 356:eaai8185. doi: 10.1126/science.aai8185
Cai, Z., Schools, G. P., and Kimelberg, H. K. (2000). Metabotropic glutamate receptors in acutely isolated hippocampal astrocytes: developmental changes of mGluR5 mRNA and functional expression. Glia 29, 70–80. doi: 10.1002/(SICI)1098-1136(20000101)29:1<70::AID-GLIA7>3.0.CO;2-V
Charles, A. C., Merrill, J. E., Dirksen, E. R., and Sanderson, M. J. (1991). Intercellular signaling in glial cells: calcium waves and oscillations in response to mechanical stimulation and glutamate. Neuron 6, 983–992. doi: 10.1016/0896-6273(91)90238-U
Clarke, L. E., and Barres, B. A. (2013). Emerging roles of astrocytes in neural circuit development. Nat. Rev. Neurosci. 14, 311–321. doi: 10.1038/nrn3484
Condorelli, D. F., Dell'Albani, P., Corsaro, M., Giuffrida, R., Caruso, A., Salinaro, A. T., et al. (1997). Metabotropic glutamate receptor expression in cultured rat astrocytes and human gliomas. Neurochem. Res. 22, 1127–1133. doi: 10.1023/A:1027317319166
Corkrum, M., Rothwell, P. E., Thomas, M. J., Kofuji, P., and Araque, A. (2019). Opioid-mediated astrocyte neuron signaling in the nucleus accumbens. Cells 8:586. doi: 10.3390/cells8060586
Cornell-Bell, A. H., Finkbeiner, S. M., Cooper, M. S., and Smith, S. J. (1990). Glutamate induces calcium waves in cultured astrocytes: long-range glial signaling. Science 247, 470–473. doi: 10.1126/science.1967852
Dani, J. W., Chernjavsky, A., and Smith, S. J. (1992). Neuronal activity triggers calcium waves in hippocampal astrocyte networks. Neuron 8, 429–440. doi: 10.1016/0896-6273(92)90271-E
Dantoni, S., Berretta, A., Bonaccorso, C. M., Bruno, V., Aronica, E., Nicoletti, F., et al. (2008). Metabotropic glutamate receptors in glial cells. Neurochem. Res. 33, 2436–2443. doi: 10.1007/s11064-008-9694-9
De Pittà, M., and Berry, H. (2019). A neuron–glial perspective for computational neuroscience. Comput. Gliosci., 3–35. doi: 10.1007/978-3-030-00817-8
De Pittà, M., Goldberg, M., Volman, V., Berry, H., and Ben-Jacob, E. (2009). Glutamate regulation of calcium and IP3 oscillating and pulsating dynamics in astrocytes. J. Biol. Phys. 35, 383–411. doi: 10.1007/s10867-009-9155-y
Dupont, G., and Goldbeter, A. (1993). One-pool model for Ca2+ oscillations involving Ca2+ and inositol 1, 4, 5-trisphosphate as co-agonists for Ca2+ release. Cell Calcium 14, 311–322. doi: 10.1016/0143-4160(93)90052-8
Eddleston, M., and Mucke, L. (1993). Molecular profile of reactive astrocytes implications for their role in neurologic disease. Neuroscience 54, 15–36. doi: 10.1016/0306-4522(93)90380-X
Eid, T., Williamson, A., Lee, T.-S. W., Petroff, O. A., and de Lanerolle, N. C. (2008). Glutamate and astrocytes key players in human mesial temporal lobe epilepsy? Epilepsia 49, 42–52. doi: 10.1111/j.1528-1167.2008.01492.x
Fellin, T., Pascual, O., Gobbo, S., Pozzan, T., Haydon, P. G., and Carmignoto, G. (2004). Neuronal synchrony mediated by astrocytic glutamate through activation of extra synaptic NMDA receptors. Neuron 43, 729–743. doi: 10.1016/j.neuron.2004.08.011
Fellin, T., Pascual, O., and Haydon, P. G. (2006). Astrocytes coordinate synaptic networks: balanced excitation and inhibition. Physiology 21, 208–215. doi: 10.1152/physiol.00161.2005
Fiacco, T. A., and Mccarthy, K. D. (2006). Astrocyte calcium elevations: properties, propagation, and effects on brain signaling. Glia 54, 676–690. doi: 10.1002/glia.20396
Finch, E. A., Turner, T. J., and Goldin, S. M. (1991). Calcium as a coagonist of inositol 1, 4, 5-trisphosphate-induced calcium release. Science 252, 443–446. doi: 10.1126/science.2017683
Gawthrop, P. J., and Crampin, E. J. (2017). Bond graph modelling of chemoelectrical energy transduction. IET Syst. Biol. 11, 127–138. doi: 10.1049/iet-syb.2017.0006
Goldbeter, A., Dupont, G., and Berridge, M. J. (1990). Minimal model for signal-induced Ca2+ oscillations and for their frequency encoding through protein phosphorylation. Proc. Natl. Acad. Sci. 87, 1461–1465. doi: 10.1073/pnas.87.4.1461
Haydon, P. G., and Carmignoto, G. (2006). Astrocyte control of synaptic transmission and neurovascular coupling. Physiol. Rev. 86, 1009–1031. doi: 10.1152/physrev.00049.2005
Hermans, E., and Challiss, R. A. J. (2001). Structural, signalling and regulatory properties of the group I metabotropic glutamate receptors: prototypic family C G-protein-coupled receptors. Biochem. J. 359, 465–484. doi: 10.1042/bj3590465
Höfer, T., Venance, L., and Giaume, C. (2002). Control and plasticity of intercellular calcium waves in astrocytes: a modeling approach. J. Neurosci. 22, 4850–4859. doi: 10.1523/JNEUROSCI.22-12-04850.2002
Innocenti, B., Parpura, V., and Haydon, P. G. (2000). Imaging extracellular waves of glutamate during calcium signaling in cultured astrocytes. J. Neurosci. 20, 1800–1808. doi: 10.1523/JNEUROSCI.20-05-01800.2000
Jiang, H., Aćimović, J., Manninen, T., Ahokainen, I., Stapmanns, J., Lehtimäki, M., et al. (2024). Modeling neuron-astrocyte interactions in neural networks using distributed simulation.
Jourdain, P., Bergersen, L. H., Bhaukaurally, K., Bezzi, P., Santello, M., Domercq, M., et al. (2007). Glutamate exocytosis from astrocytes controls synaptic strength. Nat. Neurosci. 10, 331–339. doi: 10.1038/nn1849
Kang, N., Xu, J., Xu, Q., Nedergaard, M., and Kang, J. (2005). Astrocytic glutamate release-induced transient depolarization and epileptiform discharges in hippocampal CA1 pyramidal neurons. J. Neurophysiol. 94, 4121–4130. doi: 10.1152/jn.00448.2005
Khakh, B. S., and Mccarthy, K. D. (2015). Astrocyte calcium signaling: from observations to functions and the challenges therein. Cold Spring Harb. Perspect. Biol. 7:a020404. doi: 10.11017/cshperspect.a020404
Khakh, B. S., and Sofroniew, M. V. (2015). Diversity of astrocyte functions and phenotypes in neural circuits. Nat. Neurosci. 18, 942–952. doi: 10.1038/nn.4043
Kofuji, P., and Araque, A. (2021). G-protein-coupled receptors in astrocyte–neuron communication. Neuroscience 456, 71–84. doi: 10.1016/j.neuroscience.2020.03.025
Kondoh, T., Nishizaki, T., Aihara, H., and Tamaki, N. (2001). NMDA-responsible, APV-insensitive receptor in cultured human astrocytes. Life Sci. 68, 1761–1767. doi: 10.1016/S0024-3205(01)00971-7
Kummer, U., Olsen, L. F., Dixon, C. J., Green, A. K., Bornberg-Bauer, E., and Baier, G. (2000). Switching from simple to complex oscillations in calcium signaling. Biophys. J. 79, 1188–1195. doi: 10.1016/S0006-3495(00)76373-9
Lemon, G., Gibson, W. G., and Bennett, M. R. (2003). Metabotropic receptor activation, desensitization and sequestration I: modelling calcium and inositol 1, 4, 5-trisphosphate dynamics following receptor activation. J. Theor. Biol. 223, 93–111. doi: 10.1016/S0022-5193(03)00079-1
Li, Y.-X., and Rinzel, J. (1994). Equations for InsP3 receptor-mediated [Ca2+] oscillations derived from a detailed kinetic model: a Hodgkin-Huxley like formalism. J. Theor. Biol. 166, 461–473. doi: 10.1006/jtbi.1994.1041
Ma, Z., Wei, L., Du, X., Hou, S., Chen, F., Jiao, Q., et al. (2021). Two-photon calcium imaging of neuronal and astrocytic responses: the influence of electrical stimulus parameters and calcium signaling mechanisms. J. Neural Eng. 18:046096. doi: 10.1088/1741-2552/ac1f2b
Madinier, A., Bertrand, N., Rodier, M., Quirié, A., Mossiat, C., Prigent-Tessier, A., et al. (2013). Ipsilateral versus contralateral spontaneous post-stroke neuroplastic changes: involvement of BDNF? Neuroscience 231, 169–181. doi: 10.1016/j.neuroscience.2012.11.054
Malarkey, E. B., and Parpura, V. (2008). Mechanisms of glutamate release from astrocytes. Neurochem. Int. 52, 142–154. doi: 10.1016/j.neuint.2007.06.005
Manninen, T., Havela, R., and Linne, M. (2018). Computational models for calcium-mediated astrocyte functions. Front. Comput. Neurosci. 12:14. doi: 10.3389/fncom.2018.00014
Mcdougal, D. H., Hermann, G. E., and Rogers, R. C. (2013). Astrocytes in the nucleus of the solitary tract are activated by low glucose or glucoprivation: evidence for glial involvement in glucose homeostasis. Front. Neurosci. 7:249. doi: 10.3389/fnins.2013.00249
Mennerick, S., and Zorumski, C. F. (1994). Glial contributions to excitatory neurotransmission in cultured hippocampal cells. Nature 368, 59–62. doi: 10.1038/368059a0
Miller, L. D., Petrozzino, J. J., and Connor, J. A. (1995). G protein-coupled receptors mediate a fast excitatory postsynaptic current in CA3 pyramidal neurons in hippocampal slices. J. Neurosci. 15, 8320–8330. doi: 10.1523/JNEUROSCI.15-12-08320.1995
Mitroshina, Е. V., Pakhomov, A. M., Krivonosov, M., Yarkov, R. S., Gavrish, M. S., Shkirin, A. V., et al. (2022). Novel algorithm of network calcium dynamics analysis for studying the role of astrocytes in neuronal activity in Alzheimer’s disease models. Int. J. Mol. Sci. 23:15928. doi: 10.3390/ijms232415928
Montana, V., Malarkey, E. B., Verderio, C., Matteoli, M., and Parpura, V. (2006). Vesicular transmitter release from astrocytes. Glia 54, 700–715. doi: 10.1002/glia.20367
Mouillac, B., Balestre, M.-N., and Guillon, G. (1990). Positive feedback regulation of phospholiphase C by vasopressin-induced calcium mobilization in WRK1 cells. Cell Signal 2, 497–507.
Musotto, R., Wanderlingh, U., D'Ascola, A., Spatuzza, M., Catania, M. V., De Pittà, M., et al. (2024). Dynamics of astrocytes Ca2+ signaling: a low-cost fluorescence customized system for 2D cultures. Front. Cell Dev. Biol. 12:1320672. doi: 10.3389/fcell.2024.1320672
Nadkarni, S., and Jung, P. (2003). Spontaneous oscillations of dressed neurons: a new mechanism for epilepsy? Phys. Rev. Lett. 91:268101. doi: 10.1103/PhysRevLett.91.268101
Nicoletti, F., Bockaert, J., Collingridge, G. L., Conn, P. J., Ferraguti, F., Schoepp, D. D., et al. (2011). Metabotropic glutamate receptors: from the workbench to the bedside. Neuropharmacology 60, 1017–1041. doi: 10.1016/j.neurophar.2010.10.022
Parpura, V., Basarsky, T. A., Liu, F., Jeftinija, K., Jeftinija, S., and Haydon, P. G. (1994). Glutamate-mediated astrocyte-neuron signalling. Nature 369, 744–747. doi: 10.1038/369744a0
Parpura, V., and Haydon, P. G. (2000). Physiological astrocytic calcium levels stimulate glutamate release to modulate adjacent neurons. Proc. Natl. Acad. Sci. 97, 8629–8634. doi: 10.1073/pnas.97.15.8629
Pasti, L., Volterra, A., Pozzan, T., and Carmignoto, G. (1997). Intracellular calcium oscillations in astrocytes: a highly plastic, bidirectional form of communication between neurons and astrocytes in situ. J. Neurosci. 17, 7817–7830. doi: 10.1523/JNEUROSCI.17-20-07817.1997
Pawelczyk, T., and Matecki, A. (1998). Localization of phospholipase C δ3 in the cell and regulation of its activity by phospholipids and calcium. Eur. J. Biochem. 257, 169–177. doi: 10.1046/j.1432-1327.1998.2570169.x
Perea, G., and Araque, A. (2007). Astrocytes potentiate transmitter release at single hippocampal synapses. Science 317, 1083–1086. doi: 10.1126/science.1144640
Perea, G., Navarrete, M., and Araque, A. (2009). Tripartite synapses: astrocytes process and control synaptic information. Trends Neurosci. 32, 421–431. doi: 10.1016/j.tins.2009.05.001
Petralia, R. S., Wang, Y. X., Zhao, H. M., and Wenthold, R. J. (1996). Ionotropic and metabotropic glutamate receptors show unique postsynaptic, presynaptic, and glial localizations in the dorsal cochlear nucleus. J. Comp. Neurol. 372, 356–383. doi: 10.1002/(SICI)1096-9861(19960826)372:3<356::AID-CNE3>3.0.CO;2-1
Rappold, P. M., and Tieu, K. (2010). Astrocytes and therapeutics for Parkinson's disease. Neurotherapeutics 7, 413–423. doi: 10.1016/j.nurt.2010.07.001
Rebecchi, M. J., and Pentyala, S. N. (2000). Structure, function, and control of phosphoinositide-specific phospholipase C. Physiol. Rev. 80, 1291–1335. doi: 10.1152/physrev.2000.80.4.1291
Rhee, S. G., and Bae, Y. S. (1997). Regulation of phosphoinositide-specific phospholipase C isozymes. J. Biol. Chem. 272, 15045–15048. doi: 10.1074/jbc.272.24.15045
Rosa, M., Giovanni, P., and Ulderico, W. (2022). Neuron and astrocyte computational models for describing the brain complexity. Atti della Accademia Peloritana dei Pericolanti-Classe di Scienze Fisiche, Matematiche e Naturali. 100.2:1. doi: 10.1478/AAPP.1002LC1
Santello, M., and Volterra, A. (2009). Synaptic modulation by astrocytes via Ca2+-dependent glutamate release. Neuroscience 158, 253–259. doi: 10.1016/j.neuroscience.2008.03.039
Schipke, C. G., and Kettenmann, H. (2004). Astrocyte responses to neuronal activity. Glia 47, 226–232. doi: 10.1002/glia.20029
Schnell, C., Fresemann, J., and Hülsmann, S. (2011). Determinants of functional coupling between astrocytes and respiratory neurons in the pre-Bötzinger complex. PLoS One 6:e26309. doi: 10.1371/journal.pone.0026309
Seifert, G., and Steinhäuser, C. (2001). Ionotropic glutamate receptors in astrocytes. Prog. Brain Res. 132, 287–299. doi: 10.1016/S0079-6123(01)32083-6
Semyanov, A., Henneberger, C., and Agarwal, A. (2020). Making sense of astrocytic calcium signals from acquisition to interpretation. Nat. Rev. Neurosci. 21, 551–564. doi: 10.1038/s41583-020-0361-8
Shao, Y., and Mccarthy, K. D. (1994). Plasticity of astrocytes. Glia 11, 147–155. doi: 10.1002/glia.440110209
Skowrońska, K., Obara-Michlewska, M., Zielińska, M., and Albrecht, J. (2019). NMDA receptors in astrocytes: in search for roles in neurotransmission and astrocytic homeostasis. Int. J. Mol. Sci. 20:309. doi: 10.3390/ijms20020309
Smith, K. L., John, C. S., Elizabeth, S. I, Ongür, D., Cohen, B. M., Barry, S. M., et al. (2014). Exploring the role of central astrocytic glutamate uptake in ethanol reward in mice. Alcohol. Clin. Exp. Res. 38, 1307–1314. doi: 10.1111/acer.12361
Smith, I. F., and Parker, I. (2009). Imaging the quantal substructure of single IP3R channel activity during Ca2+ puffs in intact mammalian cells. Proc. Natl. Acad. Sci. 106, 6404–6409. doi: 10.1073/pnas.0810799106
Smrcka, A. V., Hepler, J. R., Brown, K. O., and Sternweis, P. C. (1991). Regulation of polyphosphoinositide-specific phospholipase C activity by purified Gq. Science 251, 804–807. doi: 10.1126/science.1846707
Spooren, W. P., Gasparini, F., Salt, T. E., and Kuhn, R. (2001). Novel allosteric antagonists shed light on mglu5 receptors and CNS disorders. Trends Pharmacol. Sci. 22, 331–337. doi: 10.1016/S0165-6147(00)01694-1
Steinhäuser, C., and Gallo, V. (1996). News on glutamate receptors in glial cells. Trends Neurosci. 19, 339–345. doi: 10.1016/0166-2236(96)10043-6
Stobart, J. L., Ferrari, K. D., Barrett, M. J. P., Glück, C., Stobart, M. J., Zuend, M., et al. (2018). Cortical circuit activity evokes rapid astrocyte calcium signals on a similar timescale to neurons. Neuron 98, 726–735.e4. doi: 10.1016/j.neuron.2018.03.050
Stogsdill, J. A., Harwell, C. C., and Goldman, S. A. (2023). Astrocytes as master modulators of neural networks: synaptic functions and disease-associated dysfunction of astrocytes. Ann. N. Y. Acad. Sci. 1525, 41–60. doi: 10.1111/nyas.15004
Sun, W., McConnell, E., Pare, J.-F., Xu, Q., Chen, M., Peng, W., et al. (2013). Glutamate-dependent neuroglial calcium signaling differs between young and adult brain. Science 339, 197–200. doi: 10.1126/science.1226740
Taylor, S. J., and Exton, J. H. (1987). Guanine-nucleotide and hormone regulation of polyphosphoinositide phospholipase C activity of rat liver plasma membranes. Bivalent-cation and phospholipid requirements. Biochem. J. 248, 791–799. doi: 10.1042/bj2480791
Temburni, M. K., and Jacob, M. H. (2001). New functions for glia in the brain. Proc. Natl. Acad. Sci. 98, 3631–3632. doi: 10.1073/pnas.081073198
Testa, C. M., Standaert, D. G., Landwehrmeyer, G. B., Penney, J. B. Jr., and Young, A. B. (1995). Differential expression of mGluR5 metabotropic glutamate receptor mRNA by rat striatal neurons. J. Comp. Neurol. 354, 241–252. doi: 10.1002/cne.903540207
Tian, G.-F., Azmi, H., Takano, T., Xu, Q., Peng, W., Lin, J., et al. (2005). An astrocytic basis of epilepsy. Nat. Med. 11, 973–981. doi: 10.1038/nm1277
Verkhratsky, A., and Nedergaard, M. (2018). Physiology of astroglia. Physiol. Rev. 98, 239–389. doi: 10.1152/physrev.00042.2016
Verkhratsky, A., Olabarria, M., Noristani, H. N., Yeh, C. Y., and Rodriguez, J. J. (2010). Astrocytes in Alzheimer's disease. Neurotherapeutics 7, 399–412. doi: 10.1016/j.nurt.2010.05.017
Verkhratsky, A., Orkand, R. K., and Kettenmann, H. (1998). Glial calcium: homeostasis and signaling function. Physiol. Rev. 78, 99–141. doi: 10.1152/physrev.1998.78.1.99
Verkhratsky, A., and Steinhäuser, C. (2000). Ion channels in glial cells. Brain Res. Rev. 32, 380–412. doi: 10.1016/S0165-0173(99)00093-4
Volterra, A., Magistretti, P. J., and Haydon, P. G. (2002). The tripartite synapse: glia in synaptic transmission : Oxford University Press.
Volterra, A., and Meldolesi, J. (2005). Astrocytes, from brain glue to communication elements: the revolution continues. Nat. Rev. Neurosci. 6, 626–640. doi: 10.1038/nrn1722
Wamhoff, B. R., Dixon, J. L., and Sturek, M. (2002). Atorvastatin treatment prevents alterations in coronary smooth muscle nuclear Ca2+ signaling in diabetic dyslipidemia. J. Vasc. Res. 39, 208–220. doi: 10.1159/000063686
Winder, D. G., and Conn, P. J. (1996). Roles of metabotropic glutamate receptors in glial function and glial-neuronal communication. J. Neurosci. Res. 46, 131–137. doi: 10.1002/(SICI)1097-4547(19961015)46:2<131::AID-JNR1>3.0.CO;2-I
Wroblewska, B., Santi, M. R., and Neale, J. H. (1998). N-acetylaspartylglutamate activates cyclic AMP-coupled metabotropic glutamate receptors in cerebellar astrocytes. Glia 24, 172–179. doi: 10.1002/(SICI)1098-1136(199810)24:2<172::AID-GLIA2>3.0.CO;2-6
Keywords: model, calcium wave, astrocytes, simulation, experimental data
Citation: Musotto R, Wanderlingh U and Pioggia G (2025) Ca2+ waves in astrocytes: computational modeling and experimental data. Front. Cell. Neurosci. 19:1536096. doi: 10.3389/fncel.2025.1536096
Edited by:
Qingchao Qiu, Michael E. DeBakey VA Medical Center, United StatesReviewed by:
Marcello Melone, Marche Polytechnic University, ItalySeung-Eon Roh, Johns Hopkins University, United States
Copyright © 2025 Musotto, Wanderlingh and Pioggia. This is an open-access article distributed under the terms of the Creative Commons Attribution License (CC BY). The use, distribution or reproduction in other forums is permitted, provided the original author(s) and the copyright owner(s) are credited and that the original publication in this journal is cited, in accordance with accepted academic practice. No use, distribution or reproduction is permitted which does not comply with these terms.
*Correspondence: Rosa Musotto, cm9zYS5tdXNvdHRvQGlyaWIuY25yLml0