- 1Department of Anesthesiology, Emory University School of Medicine, Atlanta, GA, United States
- 2Research Division, Atlanta VA Medical Center, Atlanta, GA, United States
Electroencephalographic recordings (EEG) present an opportunity to monitor changes in human brain electrical activity during changing states of consciousness like sleep or general anesthesia. Frontal EEG recordings during surgical interventions with anesthetic-induced unconsciousness help to estimate the patients' level of (un)consciousness.
EEG-Based Monitoring of the Level of Consciousness: Commercial Devices
The classical way to extract information from the recorded EEG relevant for assessment of the level of anesthesia is the application of algorithms that evaluate changes in the oscillatory behavior of the EEG that is mainly derived from frontal EEG montages placed on the patients' forehead. These calculations are most often performed in the frequency domain, i.e., after transformation of the signal, e.g., by the Fourier Transform. The most prominent commercial system, the bispectral index (BIS, Medtronic, Dublin, Ireland) evaluates changes in the log ratio of the 30 to 47 Hz and 11 to 20 Hz EEG band power (BetaRatio) as well as a ratio of the sum of bispectrum peaks in the 0.5 to 47 Hz and the 40 to 47 Hz range (SynchFastSlow) (Rampil, 1998). The bispectrum presents a higher order spectrum that evaluates the phase correlation of different frequency components and is able to identify nonlinear signal properties (Rampil, 1998). The BetaRatio subparameter outperforms SynchFastSlow and BIS in separating consciousness from unconsciousness (Schneider et al., 2004). SynchFastSlow dominates BIS calculation during surgical levels of anesthesia (Rampil, 1998). State and Response entropy (GE Healthcare, Chicago, IL) evaluate changes in the shape of the power spectrum (Viertio-Oja et al., 2004). Other devices like the CSI (Danmeter, Odense, Denmark), IoC (Morpheus Medical, Barcelona, Spain), or qCON (Quantium Medical, Mataro, Spain) use ratios of EEG band power (Jensen et al., 2006, 2014; Revuelta et al., 2008). The IoC also processes information from the EEG after transformation to a time series of symbols (Revuelta et al., 2008). The Narcotrend (Narcotrend, Hannover, Germany) utilizes information from the spectral domain as well from autoregressive modeling in the time domain (Kreuer and Wilhelm, 2006). The PSI from the SEDLine monitor (Masimo, Irvine, CA) processes spectral power from different frequency bands as well as interhemispheric power gradients and synchrony (Prichep et al., 2004). The Brain Anesthesia Response (BAR) monitor (Cortical Dynamics Ltd., North Perth, Australia) takes a different approach. It generates its index by modeling EEG dynamics (Liley et al., 2010b). These devices have in common, that combining the subparameters is performed by a proprietary algorithm, and hence the contribution of each parameter to the index is not known. In general, these indices track the suppression of high frequency EEG activity and the activation of low frequent oscillations, as triggered by many common anesthetics (Brown et al., 2010). By using spectral power, or parameters derived from it, as key parameters, the monitoring systems may dismiss signal information content by neglecting the phase component of the signals' frequency and only exploiting information from the amplitude spectrum (Callegaro, 2012). Further, the devices susceptibility to muscle activity (Messner et al., 2003; Schuller et al., 2015), especially by including high EEG frequencies as well as the time delay, necessary for index calculation (Pilge et al., 2006; Zanner et al., 2009; Kreuzer et al., 2012) may present a limiting factor in performance to reliably track the anesthetic state.
EEG-Based Consciousness Monitoring in Research: Time-Domain Analytical Approaches
More recent approaches to extract information from the EEG at different levels of anesthesia use nonlinear parameters that reflect signal information content, complexity, and/or predictability. These approaches seem capable to extract non-linear information from the signal as investigated with surrogate techniques, while linear measures like spectral entropy or the Hurst exponent did not detect these non-linearities (Jordan et al., 2009; Anier et al., 2010). In EEG and anesthesia research the most prominent players are approximate entropy (ApEn) (Pincus, 1991; Bruhn et al., 2000) and permutation entropy (PeEn) (Bandt and Pompe, 2002; Jordan et al., 2008; Olofsen et al., 2008) for single channel analysis and cross approximate entropy (Pincus Steven et al., 1996; Hudetz, 2002; Kreuzer et al., 2010), (symbolic) transfer entropy (Schreiber, 2000; Imas et al., 2005; Staniek and Lehnertz, 2008; Jordan et al., 2013), or order recurrence plots (Groth, 2006) for bivariate analysis. These measures are applied to the EEG time domain, usually after band-pass filtering of the EEG to a wide frequency range with a low pass filter set to around 25 to 30 Hz to limit EEG signal contamination by electromyographic activity (EMG). Frontal EMG activity can occur in the entire frequency range but seems to peak between 25 and 30 Hz (Goncharova et al., 2003). The mentioned entropy measures usually define consecutive amplitude values or their ranks as pieces of information, called motifs. The EEG is then represented as series of motifs. The user can define the length m of a motif (the number of amplitude values it is generated from), a time lag parameter τ (to consider only each τth amplitude value to define a motif of length m), and a shift k (to shift k amplitude values from the first amplitude value of the previous motif to start generation of the next motif of length m with lag τ). Figure 1 presents the impact of k and τ on motif generation.
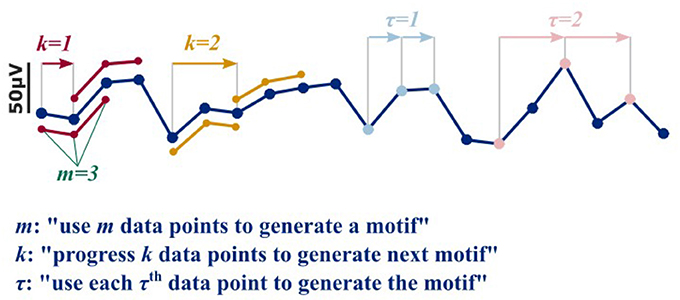
Figure 1. In order to generate a motif as used for the nonlinear, entropy based approaches, motive length m, time delay τ, and shift k have to be defined. The parameter k defines the shift. For a k = 1, the first motif of length m = 3 starts at data point i, and the second at i+k = 2 and so on (red). For a k of 2, the first motif would start at data point i, and the second at i+k = 3, and so on (yellow). The parameter τ defines how many data points are left out to generate the motif. E.g., for a τ = 1 and m = 3, the data points i, i+1, and i+2 are used to generate the motif (light blue). For a τ = 1, the data points i, i+2, and i+4 are used (pink).
The transfer entropies that quantify directed information flow include another parameter δ (Staniek and Lehnertz, 2008) to define transfer lags or transmission time of the motif of information between the two channels. The time lag parameters δ may evaluate changes in signal information roughly associated with a certain frequency range. When compared to spectral approaches and commercial monitors, the univariate measures ApEn and PeEn showed higher performance in distinguishing EEG recorded during consciousness from EEG recorded during unconsciousness and to reflect different levels of general anesthesia (Bruhn et al., 2000; Jordan et al., 2008; Liang et al., 2015). A newly proposed, multimodal index, integrates PeEn to separate consciousness from unconsciousness and ApEn to scale different levels of anesthesia (Schneider et al., 2014). The use of the bivariate transfer entropies revealed a loss of cortical feedback connectivity as a key mechanism of anesthetic-induced unconsciousness that is universal for most anesthetics (Ku et al., 2011; Jordan et al., 2013; Lee et al., 2013; Ranft et al., 2016). Interestingly the parameter settings were targeted toward the EEG beta frequency range. This frequency range may play an important role in synchronizing different cortical regions (von Stein and Sarnthein, 2000; Bassett et al., 2009; Hipp et al., 2011). So although these nonlinear parameters are applied to a wide frequency range, their intrinsic setting defines the information to be extracted from the signal.
Different Entropies Evaluate Different Properties
These findings are a strong claim to include nonlinear analysis techniques in commercial “depth of anesthesia” monitoring as well as to extend the EEG electrode layout to at least one electrode placed in parietal or occipital regions to be able to monitor the loss of cortical feedback activity. Further, there is something to be mentioned regarding “entropy analysis” in anesthesiology. Often, for instance at conferences there is just a discussion about “entropy” analysis without defining what method really has been used. These measures, even if they share the term “entropy” analyze different signal features. A very prominent example is the spectral entropy, a measure evaluating the change in shape of the power spectrum (Viertio-Oja et al., 2004). It evaluates the changes in the frequency domain, so it cannot be compared to analytical techniques in the time domain. Another example is the difference between ApEn and PeEn. The ordinal PeEn evaluates the probability distribution of amplitude rank patterns in the signal, while ApEn evaluates the probability of similar absolute amplitude patterns detected in the signal remain similar if they are extended by one more amplitude value. In order to define similarity of two absolute amplitude values, the algorithm contains a tolerance that acts like a low pass filter on the signal, while the formation of rank pattern in the PeEn is more like a high pass that removes slow underlying trends in amplitude from the signal. Figure 2 presents a graphical example of the described differences. As mentioned earlier, ApEn and PeEn have different strengths. ApEn seems strong in scaling different levels of unconsciousness, while PeEn presents a strong parameter to separate consciousness from unconsciousness (Schneider et al., 2014).
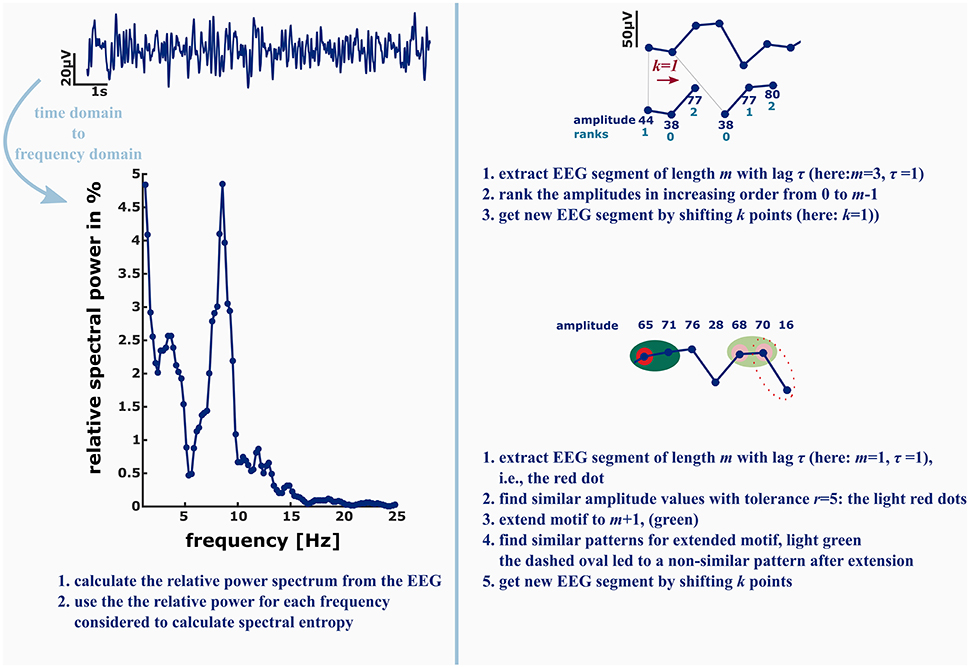
Figure 2. Left: in order to calculate the spectral entropy as for example used in the GE Entropy Module, the EEG power spectrum is calculated from the recording. The spectral entropy value reflects the shape of the power spectrum. The more uniformly distributed the power is among the frequencies, the higher is the spectral entropy value. Right: permutation entropy (PeEn, top) and approximate entropy (ApEn, bottom) in contrast are directly derived from the EEG time series. For the ordinal PeEn motifs of length m are represented as a series of ranks, with the lowest amplitude value being equal to rank 0 and the highest amplitude value being equal to rank m−1.
Hence, the EEG time series is converted to a series of rank patterns. The more uniform the probability distribution of the m! possible rank patterns, the higher is PeEn. ApEn evaluates the predictability of a time series by evaluating the occurrence of similar patterns of length m. Similar means that the maximum difference of the EEG amplitude values is smaller than a tolerance r. The concept of ApEn is to evaluate the probability, that if a similar pattern of length m was detected, the patterns extended to m+1 will be similar as well. The higher this probability, the lower ApEn will be.
Proposed Relationships between EEG Frequency and Communication
Although the nonlinear approaches seem to reflect the level of consciousness in a superior way, there is a strong point in favor of continuing to use spectral analyses, together with the aforementioned approaches to optimize monitoring. It is the assumption that (frontal) EEG oscillations of certain frequencies seem to correlate with interactions of the monitored cortical area with other cortical or subcortical areas. In general, the EEG mainly reflects cortical activity (Fisch and Spehlmann, 1999), but this cortical activity also carries information from subcortical regions. Frontal EEG theta power for instance seems associated with working memory (Klimesch et al., 1994; Summerfield and Mangels, 2005). The prominent alpha peak that develops in the EEG power spectrum during general anesthesia is potentially caused by synchronous activity in the thalamocortical loop (John and Prichep, 2005; Ching et al., 2010). But this thalamocortical relationship and the contribution of each region to alpha EEG is controversially discussed, as nicely reviewed by Liley and coworkers. The thalamus may not present the principal source because thalamocortical projections are sparse, the amplitude of thalamocortical excitatory postsynaptic potentials is small, the corticocortical activity is more coherent than thalamocortical activity, the isolated cortex is able to generate rhythmic oscillations, and drugs may modulate alpha oscillations in cortex and thalamus in a different way (Liley et al., 2010a). As mentioned earlier, activity in EEG beta-band may play a role in synchronizing cortical regions (von Stein et al., 1999; John and Prichep, 2005; Bassett et al., 2009; Hipp et al., 2011). Hence changes in these frequency bands' spectral power may help to understand anesthetic-induced changes in brain activity among different regions and possibly target different components of general anesthesia. These relationships between EEG frequency and the brain's communication structure may help future research to improve EEG based patient monitoring in anesthesia with a new focus on adding an “anesthesia quality” component to monitoring, i.e., to associate EEG recorded during anesthesia maintenance and emergence with adverse outcomes like pain or delirium following anesthesia.
Correlation of Intraoperative EEG Markers and Adverse Outcomes
The current monitoring systems as well as the presented results using nonlinear approaches to EEG-based monitoring focus on a reliable separation of different hypnotic levels that range from “fully awake” to “(burst) suppression.” Hence, these monitoring systems/approaches may be able to prevent too deep levels of general anesthesia. Prevention of too deep anesthesia may help to reduce delirious outcomes (Chan et al., 2013). But there is no algorithm component that specifically deals with the detection of intraoperative EEG markers that may be associated with postoperative adverse outcomes. There seems increasing evidence that investigation of EEG alpha-band activity may present a good start to research intraoperative EEG and its association with post-anesthetic adverse outcomes, at least for the commonly used propofol and inhaled ethers. For anesthesia emergence, results suggest that returning from anesthesia-induced unconsciousness may be more complex than anesthesia induction. The patients' EEG can follow different emergence trajectories that put patients at higher or lower risk when it comes to adverse outcomes in the postoperative care unit. Patients that abruptly transition from spectral EEG patterns of unconsciousness to spectral “wake” EEG seem more vulnerable to express pain and delirium in the postoperative care unit than patients that show episodes of non-slow wave anesthesia during emergence (Chander et al., 2014; Hight et al., 2014; Garcia et al., 2016; Kreuzer et al., 2017). During anesthesia maintenance patients most often develop a so called alpha peak in frontal EEG for the most common anesthetics propofol and sevoflurane that seems to reflect reverberations in the thalamocortical loop at least in part caused by hyperpolarization of the thalamus (Akeju et al., 2014b). Evaluation of spectral alpha peak properties may help to estimate “anesthesia quality.” Strong surgical stimulation can cause a reduction of the peak (Kochs et al., 1994) and may even lead to disappearance of the peak. Because of the possible association of alpha oscillations with the thalamus, this reduction may be caused by desynchronization of thalamocortical activity that may represent arousal (McCormick and Bal, 1997).
Patients that are not expressing strong alpha power during anesthesia or react to surgical stimulation in a stronger fashion may be at higher risk of delirium in postoperative care unit (PACU-D, unpublished data). Although PACU-D is a transient phenomenon current results highlight the association with postoperative long-term complications (Card et al., 2015; Garcia et al., 2016). Hence, avoiding or detecting PACU-D as early as possible may help to decrease the risk of developing long-term adverse outcomes. Information from the EEG alpha range may help to identify this subset of patients at risk. The correlation of lower alpha power and PACU-D may reflect a patient population with a “frailer” brain that is not able to maintain a state of stable thalamocortical synchronization. So it would definitely make sense to additionally monitor the patients' EEG reaction following surgical stimuli, adding a nociception component to EEG-based monitoring.
There is (Almost) No EEG Based (Combined Hypnosis and) Analgesia/Nociception Monitoring
Hagihira et al. showed that bicoherence peaks around 10 Hz and around 20 Hz, that are typical for gas anesthesia, decrease with noxious stimulation if no opioid is given (Hagihira et al., 2004). But these observations have not been used for current monitors of nociception. These devices use processed EEG like the BIS as subparameters (Ellerkmann et al., 2013; Castro et al., 2016), a wide range of spectral band power (Jensen et al., 2014), or non EEG information from heart rate variability (Ledowski et al., 2013), modeled drug and opioid concentrations (Luginbühl et al., 2010), or the polysynaptic spinal withdrawal reflex (Von Dincklage et al., 2012). One exception is the BAR. It uses two measures, cortical state and cortical input that are designed to reflect the hypnotic and analgesic component of anesthesia (Liley et al., 2010b). The Cortical Dynamics website claims that BAR detect the effects of a range of analgesic agents and hence lead the way toward a combined EEG-based analgesia and anesthesia monitoring.
Besides the susceptibility of the intraoperative EEG alpha peak to stimulation, age also influences EEG power. There is a negative absolute alpha power and total frontal EEG power to age relationship (Klimesch, 1997; Purdon et al., 2015) and age presents a risk factor for the development of delirious outcomes (Deiner and Silverstein, 2009) after general anesthesia. Cortical thinning seems to occur with age (Fjell et al., 2009). Consequently the number of (pyramidal) neurons and the number of synapses decreases as well (Teplan, 2002). The decrease in volume and neurons may present a reason for the observed reduction on total EEG power. As a consequence, the brain's communication may become more fragile and less robust to influences like surgical stimuli. All these associations indicate the usefulness to pay attention to what is happening to EEG alpha oscillations during general anesthesia maintenance and emergence. Previous research and commercial applications for monitoring anesthesia have not specifically focused on this EEG frequency range, as mentioned earlier. The addition of information extracted from the EEG alpha range may help to include a factor predictive for adverse outcomes to “depth of anesthesia” monitoring. All the findings regarding adverse outcomes base on frequency domain analyses. Additional information from nonlinear analytical approaches in the time domain may help to optimize and improve intraoperative monitoring to identify patients at risk for adverse outcomes in the future. While this article mainly deals with the EEG alpha range, probably numerous other markers in other EEG frequencies from frontal and non-frontal electrode locations exist that may help to optimize monitoring. Further the described findings are probably not valid for certain drugs like (S-)ketamine or dexmeditomitine that affect EEG activity in completely different ways than sevoflurane or propofol (Maksimow et al., 2006; Akeju et al., 2014a).
I think that around 20 years after the introduction of EEG based anesthesia monitoring to the operating room and ongoing optimization of analytical algorithms, the inclusion to consider the well-being of the patient in the postoperative period seems the logical next step. Recent and future findings from the correlation of intraoperative EEG (alpha) activity may help to introduce a new generation of anesthesia monitoring. It may present the transition from EEG-based “depth of anesthesia” to “quality of anesthesia” monitoring.
Author Contributions
The author confirms being the sole contributor of this work and approved it for publication.
Conflict of Interest Statement
The author declares that the research was conducted in the absence of any commercial or financial relationships that could be construed as a potential conflict of interest.
Acknowledgments
I want to thank Paul García and Keith McGregor from the Center for Visual and Neurocognitive Rehabilitation at the Atlanta VA for financial support.
References
Akeju, O., Pavone, K. J., Westover, M. B., Vazquez, R., Prerau, M. J., Harrell, P. G., et al. (2014a). A comparison of propofol-and dexmedetomidine-induced electroencephalogram dynamics using spectral and coherence analysis. J. Am. Soc. Anesthesiol. 121, 978–989. doi: 10.1097/aln.0000000000000419
Akeju, O., Westover, M. B., Pavone, K. J., Sampson, A. L., Hartnack, K. E., Brown, E. N., et al. (2014b). Effects of sevoflurane and propofol on frontal electroencephalogram power and coherence. Anesthesiology 121, 990–998. doi: 10.1097/ALN.0000000000000436
Anier, A., Lipping, T., Jäntti, V., Puumala, P., and Huotari, A.-M. (2010). editors. Entropy of the EEG in transition to burst suppression in deep anesthesia: surrogate analysis. Conf. Proc. IEEE Eng. Med. Biol. Soc. 2010, 2790–2793. doi: 10.1109/IEMBS.2010.5626366
Bandt, C., and Pompe, B. (2002). Permutation entropy: a natural complexity measure for time series. Phys. Rev. Lett. 88:174102. doi: 10.1103/PhysRevLett.88.174102
Bassett, D. S., Bullmore, E. T., Meyer-Lindenberg, A., Apud, J. A., Weinberger, D. R., and Coppola, R. (2009). Cognitive fitness of cost-efficient brain functional networks. Proc. Natl. Acad. Sci. U.S.A. 106, 11747–11752. doi: 10.1073/pnas.0903641106
Brown, E. N., Lydic, R., and Schiff, N. D. (2010). General anesthesia, sleep, and coma. New Eng. J. Med. 363, 2638–2650. doi: 10.1056/NEJMra0808281
Bruhn, J., Ropcke, H., and Hoeft, A. (2000). Approximate entropy as an electroencephalographic measure of anesthetic drug effect during desflurane anesthesia. Anesthesiology 92, 715–726. doi: 10.1097/00000542-200003000-00016
Callegaro, L. (2012). Electrical Impedance: Principles, Measurement, and Applications. Boca Raton, FL: CRC Press.
Card, E., Pandharipande, P., Tomes, C., Lee, C., Wood, J., Nelson, D., et al. (2015). Emergence from general anaesthesia and evolution of delirium signs in the post-anaesthesia care unit. Br. J. Anaesth. 115:7. doi: 10.1093/bja/aeu442
Castro, A., de Almeida, F. G., Amorim, P., and Nunes, C. S. (2016). A novel multivariate steady-state index during general anesthesia (STAN). J. Clin. Monit. Comput. doi: 10.1007/s10877-016-9905-x. [Epub ahead of print].
Chan, M. T., Cheng, B. C., Lee, T. M., Gin, T., and Group, C. T. (2013). BIS-guided anesthesia decreases postoperative delirium and cognitive decline. J. Neurosurg. Anesthesiol. 25, 33–42. doi: 10.1097/ANA.0b013e3182712fba
Chander, D., Garcia, P. S., MacColl, J. N., Illing, S., and Sleigh, J. W. (2014). Electroencephalographic variation during end maintenance and emergence from surgical anesthesia. PLoS ONE 9:e106291. doi: 10.1371/journal.pone.0106291
Ching, S., Cimenser, A., Purdon, P. L., Brown, E. N., and Kopell, N. J. (2010). Thalamocortical model for a propofol-induced α-rhythm associated with loss of consciousness. Proc. Natl. Acad. Sci. U.S.A. 107, 22665–22670. doi: 10.1073/pnas.1017069108
Deiner, S., and Silverstein, J. (2009). Postoperative delirium and cognitive dysfunction. Br. J. Anaesth. 103(Suppl. 1), i41–i46. doi: 10.1093/bja/aep291
Ellerkmann, R. K., Grass, A., Hoeft, A., and Soehle, M. (2013). The response of the composite variability index to a standardized noxious stimulus during propofol-remifentanil anesthesia. Anesth. Analg. 116, 580–588. doi: 10.1213/ANE.0b013e31827ced18
Fisch, B. J., and Spehlmann, R. (1999). Fisch and Spehlmann's EEG Primer: Basic Principles of Digital and Analog. London: EEG: Elsevier Health Sciences.
Fjell, A. M., Westlye, L. T., Amlien, I., Espeseth, T., Reinvang, I., Raz, N., et al. (2009). High consistency of regional cortical thinning in aging across multiple samples. Cereb. Cortex 19, 2001–2012. doi: 10.1093/cercor/bhn232
Garcia, P. S., Hesse, S. D., Hight, D., Kreuzer, M., Gaskell, A., Lee, S., et al. (2016). “Delirium in the post-anesthesia care unit: risk factors and clinical significance,” in The Anesthesiology Annual Meeting (Chicago, IL).
Goncharova, I. I., McFarland, D. J., Vaughan, T. M., and Wolpaw, J. R. (2003). EMG contamination of EEG: spectral and topographical characteristics. Clin. Neurophysiol. 114, 1580–1593. doi: 10.1016/S1388-2457(03)00093-2
Groth, A. (2006). Visualization of coupling in time series by order recurrence plots. Phys. Rev. 72(4 Pt 2):046220. doi: 10.1103/PhysRevE.72.046220
Hagihira, S., Takashina, M., Mori, T., Ueyama, H., and Mashimo, T. (2004). Electroencephalographic bicoherence is sensitive to noxious stimuli during isoflurane or sevoflurane anesthesia. J. Am. Soc. Anesthesiol. 100, 818–825. doi: 10.1097/00000542-200404000-00011
Hight, D. F., Dadok, V. M., Szeri, A. J., García, P. S., Voss, L., and Sleigh, J. W. (2014). Emergence from general anesthesia and the sleep-manifold. Front. Syst. Neurosci. 8:146. doi: 10.3389/fnsys.2014.00146
Hipp, J. F., Engel, A. K., and Siegel, M. (2011). Oscillatory synchronization in large-scale cortical networks predicts perception. Neuron 69, 387–396. doi: 10.1016/j.neuron.2010.12.027
Hudetz, A. G. (2002). Effect of volatile anesthetics on interhemispheric EEG cross-approximate entropy in the rat. Brain Res. 954, 123–131. doi: 10.1016/S0006-8993(02)03358-9
Imas, O. A., Ropella, K. M., Ward, B. D., Wood, J. D., and Hudetz, A. G. (2005). Volatile anesthetics disrupt frontal-posterior recurrent information transfer at gamma frequencies in rat. Neurosci. Lett. 387, 145–150. doi: 10.1016/j.neulet.2005.06.018
Jensen, E. W., Litvan, H., Revuelta, M., Rodriguez, B. E., Caminal, P., Martinez, P., et al. (2006). Cerebral state index during propofol anesthesia: a comparison with the bispectral index and the A-Line ARX index. Anesthesiology 105, 28–36. doi: 10.1097/00000542-200607000-00009
Jensen, E. W., Valencia, J. F., Lopez, A., Anglada, T., Agusti, M., Ramos, Y., et al. (2014). Monitoring hypnotic effect and nociception with two EEG-derived indices, qCON and qNOX, during general anaesthesia. Acta Anaesthesiol. Scand. 58, 933–941. doi: 10.1111/aas.12359
John, E. R., and Prichep, L. S. (2005). The anesthetic cascade: a theory of how anesthesia suppresses consciousness. Anesthesiology 102, 447–471. doi: 10.1097/00000542-200502000-00030
Jordan, D., Ilg, R., Riedl, V., Schorer, A., Grimberg, S., Neufang, S., et al. (2013). Simultaneous electroencephalographic and functional magnetic resonance imaging indicate impaired cortical top–down processing in association with anesthetic-induced unconsciousness. Anesthesiology 119, 1031–1042. doi: 10.1097/ALN.0b013e3182a7ca92
Jordan, D., Stockmanns, G., Kochs, E. F., Pilge, S., and Schneider, G. (2008). Electroencephalographic order pattern analysis for the separation of consciousness and unconsciousness: an analysis of approximate entropy, permutation entropy, recurrence rate, and phase coupling of order recurrence plots. Anesthesiology 109, 1014–1022. doi: 10.1097/ALN.0b013e31818d6c55
Jordan, D., Stockmanns, G., Kochs, E. F., and Schneider, G. (2009). “Is detection of different anesthetic levels related to nonlinearity of the electroencephalogram?,” in 4th European Conference of the International Federation for Medical and Biological Engineering. IFMBE Proceedings, Vol. 22, eds J. Sloten, P. Verdonck, M. Nyssen, and J. Haueisen (Berlin; Heidelberg: Springer), 335–339.
Klimesch, W. (1997). EEG-alpha rhythms and memory processes. Int. J. Psychophysiol. 26, 319–340. doi: 10.1016/S0167-8760(97)00773-3
Klimesch, W., Schimke, H., and Schwaiger, J. (1994). Episodic and semantic memory: an analysis in the EEG theta and alpha band. Electroencephalogr. Clin. Neurophysiol. 91, 428–441. doi: 10.1016/0013-4694(94)90164-3
Kochs, E., Bischoff, P., Pichlmeier, U., and Schulte am Esch, J. (1994). Surgical stimulation induces changes in brain electrical activity during isoflurane/nitrous oxide anesthesia. A topographic electroencephalographic analysis. Anesthesiology 80, 1026–1034. doi: 10.1097/00000542-199405000-00012
Kreuer, S., and Wilhelm, W. (2006). The narcotrend monitor. Best Pract. Res. Clin. Anaesthesiol. 20, 111–119. doi: 10.1016/j.bpa.2005.08.010
Kreuzer, M., Hentschke, H., Antkowiak, B., Schwarz, C., Kochs, E. F., and Schneider, G. (2010). Cross-approximate entropy of cortical local field potentials quantifies effects of anesthesia-a pilot study in rats. BMC Neurosci. 11:122. doi: 10.1186/1471-2202-11-122
Kreuzer, M., Whalin, M. K., Hesse, S., Riso, M., and García, P. S. (2017). Anesthetic management of a patient with multiple previous episodes of postanesthesia care unit delirium. Anesth. Analg. Case Rep. doi: 10.1213/xaa.0000000000000497. [Epub ahead of print].
Kreuzer, M., Zanner, R., Pilge, S., Paprotny, S., Kochs, E. F., and Schneider, G. (2012). Time delay of monitors of the hypnotic component of anesthesia: analysis of state entropy and index of consciousness. Anesth. Analg. 115, 315–319. doi: 10.1213/ANE.0b013e31825801ea
Ku, S.-W., Lee, U., Noh, G.-J., Jun, I.-G., and Mashour, G. A. (2011). Preferential inhibition of frontal-to-parietal feedback connectivity is a neurophysiologic correlate of general anesthesia in surgical patients. PLoS ONE 6:e25155. doi: 10.1371/journal.pone.0025155
Ledowski, T., Tiong, W., Lee, C., Wong, B., Fiori, T., and Parker, N. (2013). Analgesia nociception index: evaluation as a new parameter for acute postoperative pain. Br. J. Anaesth. 111, 627–629. doi: 10.1093/bja/aet111
Lee, U., Ku, S., Noh, G., Baek, S., Choi, B., and Mashour, G. A. (2013). Disruption of frontal–parietal communication by ketamine, propofol, and sevoflurane. Anesthesiology 118, 1264–1275. doi: 10.1097/ALN.0b013e31829103f5
Liang, Z., Wang, Y., Sun, X., Li, D., Voss, L. J., Sleigh, J. W., et al. (2015). EEG entropy measures in anesthesia. Front. Comput. Neurosci. 9:16. doi: 10.3389/fncom.2015.00016
Liley, D. T., Bojak, I., Dafilis, M. P., van Veen, L., Frascoli, F., and Foster, B. L. (2010a). Bifurcations and state changes in the human alpha rhythm: theory and experiment. Model. Phase Trans. Brain 4, 117–145. doi: 10.1007/978-1-4419-0796-7_6
Liley, D. T., Sinclair, N. C., Lipping, T., Heyse, B., Vereecke, H. E., and Struys, M. M. (2010b). Propofol and remifentanil differentially modulate frontal electroencephalographic activity. J. Am. Soc. Anesthesiol. 113, 292–304. doi: 10.1097/aln.0b013e3181e3d8a6
Luginbühl, M., Schumacher, P. M., Vuilleumier, P., Vereecke, H., Heyse, B., Bouillon, T. W., et al. (2010). Noxious stimulation response index a novel anesthetic state index based on hypnotic–opioid interaction. J. Am. Soc. Anesthesiol. 112, 872–880. doi: 10.1097/ALN.0b013e3181d40368
Maksimow, A., Särkelä, M., Långsjö, J., Salmi, E., Kaisti, K., Yli-Hankala, A., et al. (2006). Increase in high frequency EEG activity explains the poor performance of EEG spectral entropy monitor during S-ketamine anesthesia. Clin. Neurophysiol. 117, 1660–1668. doi: 10.1016/j.clinph.2006.05.011
McCormick, D. A., and Bal, T. (1997). Sleep and arousal: thalamocortical mechanisms. Annu. Rev. Neurosci. 20, 185–215. doi: 10.1146/annurev.neuro.20.1.185
Messner, M., Beese, U., Romstöck, J., Dinkel, M., and Tschaikowsky, K. (2003). The bispectral index declines during neuromuscular block in fully awake persons. Anesth. Analg. 97, 488–491. doi: 10.1213/01.ANE.0000072741.78244.C0
Olofsen, E., Sleigh, J. W., and Dahan, A. (2008). Permutation entropy of the electroencephalogram: a measure of anaesthetic drug effect. Br. J. Anaesth. 101, 810–821. doi: 10.1093/bja/aen290
Pilge, S., Zanner, R., Schneider, G., Blum, J., Kreuzer, M., and Kochs, E. (2006). Time delay of index calculation: analysis of cerebral state, bispectral, and narcotrend indices. Anesthesiology 104, 488–494. doi: 10.1097/00000542-200603000-00016
Pincus, S. M. (1991). Approximate entropy as a measure of system complexity. Proc. Natl. Acad. Sci. U.S.A. 88, 2297–2301. doi: 10.1073/pnas.88.6.2297
Pincus Steven, M., Mulligan, T., Iranmanesh, A., Gheorghiu, S., Godschalk, M., and Veldhuis, J. D. (1996). Older males secrete luteinizing hormone and testosterone more irregularly, and jointly more asynchronously, than younger males. Proc. Natl. Acad. Sci. U.S.A. 93, 14100–14105. doi: 10.1073/pnas.93.24.14100
Prichep, L., Gugino, L., John, E., Chabot, R., Howard, B., Merkin, H., et al. (2004). The patient state index as an indicator of the level of hypnosis under general anaesthesia. Br. J. Anaesth. 92, 393–399. doi: 10.1093/bja/aeh082
Purdon, P., Pavone, K., Akeju, O., Smith, A., Sampson, A., Lee, J., et al. (2015). The ageing brain: age-dependent changes in the electroencephalogram during propofol and sevoflurane general anaesthesia. Br. J. Anaesth. 115(Suppl. 1), i46–i57. doi: 10.1093/bja/aev213
Rampil, I. J. (1998). A Primer for EEG signal processing in anesthesia. Anesthesiology 89, 980–1002. doi: 10.1097/00000542-199810000-00023
Ranft, A., Golkowski, D., Kiel, T., Riedl, V., Kohl, P., Rohrer, G., et al. (2016). Neural correlates of sevoflurane-induced unconsciousness identified by simultaneous functional magnetic resonance imaging and electroencephalography. J. Am. Soc. Anesthesiol. 125, 861–872. doi: 10.1097/aln.0000000000001322
Revuelta, M., Paniagua, P., Campos, J. M., Fernandez, J. A., Martinez, A., Jospin, M., et al. (2008). Validation of the index of consciousness during sevoflurane and remifentanil anaesthesia: a comparison with the bispectral index and the cerebral state index. Br. J. Anaesth. 101, 653–658. doi: 10.1093/bja/aen245
Schneider, G., Jordan, D., Schwarz, G., Bischoff, P., Kalkman, C. J., Kuppe, H., et al. (2014). Monitoring depth of anesthesia utilizing a combination of electroencephalographic and standard measures. Anesthesiology 120, 819–828. doi: 10.1097/ALN.0000000000000151
Schneider, G., Schöniger, S., and Kochs, E. (2004). Does bispectral analysis add anything but complexity? BIS sub-components may be superior to BIS for detection of awareness. Br. J. Anaesth. 93, 596–597. doi: 10.1093/bja/aeh612
Schreiber, T. (2000). Measuring information transfer. Phys. Rev. Lett. 85:461. doi: 10.1103/PhysRevLett.85.461
Schuller, P., Newell, S., Strickland, P., and Barry, J. (2015). Response of bispectral index to neuromuscular block in awake volunteers. Br. J. Anaesth. 115(Suppl. 1), i95–i103. doi: 10.1093/bja/aev072
Staniek, M., and Lehnertz, K. (2008). Symbolic transfer entropy. Phys. Rev. Lett. 100:158101. doi: 10.1103/PhysRevLett.100.158101
Summerfield, C., and Mangels, J. A. (2005). Coherent theta-band EEG activity predicts item-context binding during encoding. Neuroimage 24, 692–703. doi: 10.1016/j.neuroimage.2004.09.012
Teplan, M. (2002). Fundamentals of EEG measurement. Meas. Sci. Rev. 2, 1–11. Available online at: http://www.measurement.sk/2002/S2/Teplan.pdf
Viertio-Oja, H., Maja, V., Sarkela, M., Talja, P., Tenkanen, N., Tolvanen-Laakso, H., et al. (2004). Description of the entropy algorithm as applied in the Datex-Ohmeda S/5 entropy module. Acta Anaesthesiol. Scand. 48, 154–161. doi: 10.1111/j.0001-5172.2004.00322.x
Von Dincklage, F., Correll, C., Schneider, M., Rehberg, B., and Baars, J. (2012). Utility of nociceptive flexion reflex threshold, bispectral index, composite variability index and noxious stimulation response index as measures for nociception during general anaesthesia. Anaesthesia 67, 899–905. doi: 10.1111/j.1365-2044.2012.07187.x
von Stein, A., Rappelsberger, P., Sarnthein, J., and Petsche, H. (1999). Synchronization between temporal and parietal cortex during multimodal object processing in man. Cereb. Cortex 9, 137–150. doi: 10.1093/cercor/9.2.137
von Stein, A., and Sarnthein, J. (2000). Different frequencies for different scales of cortical integration: from local gamma to long range alpha/theta synchronization. Int. J. Psychophysiol. 38, 301–313. doi: 10.1016/S0167-8760(00)00172-0
Zanner, R., Pilge, S., Kochs, E. F., Kreuzer, M., and Schneider, G. (2009). Time delay of electroencephalogram index calculation: analysis of cerebral state, bispectral, and Narcotrend indices using perioperatively recorded electroencephalographic signals. Br. J. Anaesth. 103, 394–399. doi: 10.1093/bja/aep198
Keywords: EEG, anesthesia, monitoring, delirium, adverse outcomes
Citation: Kreuzer M (2017) EEG Based Monitoring of General Anesthesia: Taking the Next Steps. Front. Comput. Neurosci. 11:56. doi: 10.3389/fncom.2017.00056
Received: 01 February 2017; Accepted: 07 June 2017;
Published: 22 June 2017.
Edited by:
Logan James Voss, Waikato District Health Board, New ZealandReviewed by:
Anthony G. Hudetz, University of Michigan, United StatesDavid Liley, Swinburne University of Technology, Australia
Copyright © 2017 Kreuzer. This is an open-access article distributed under the terms of the Creative Commons Attribution License (CC BY). The use, distribution or reproduction in other forums is permitted, provided the original author(s) or licensor are credited and that the original publication in this journal is cited, in accordance with accepted academic practice. No use, distribution or reproduction is permitted which does not comply with these terms.
*Correspondence: Matthias Kreuzer, bWF0dGhpYXMua3JldXplckBlbW9yeS5lZHU=