- 1Graduate School of Education, Harvard University, Cambridge, MA, United States
- 2Department of Psychology, Harvard University, Cambridge, MA, United States
- 3Department of Brain and Cognitive Sciences, Massachusetts Institute of Technology, Cambridge, MA, United States
Pedagogy is a powerful way to learn about the world, and young children are adept at both learning from teaching and teaching others themselves. Theoretical accounts of pedagogical reasoning suggest that an important aspect of being an effective teacher is considering what learners need to know, as misconceptions about learners' beliefs, needs, or goals can result in less helpful teaching. One underexplored way in which teachers may fail to represent what learners know is by simply “going through the motions” of teaching, without actively engaging with the learner's beliefs, needs, and goals at all. In the current paper, we replicate ongoing work that suggests children are sensitive to when others are relying on automatic scripts in the context of teaching. We then look at the potential link to two related measures. First, we hypothesize that sensitivity to a teacher's perceived automaticity will be linked to classic measures of pedagogical sensitivity and learning—specifically, how children explore and learn about novel toys following pedagogical vs. non-pedagogical demonstrations. Second, we hypothesize that the development of Theory of Mind (ToM) (and age differences more broadly) relate to these pedagogical sensitivities. Our online adaptation of the novel toy exploration task did not invoke pedagogical reasoning as expected, and so we do not find robust links between these tasks. We do find that ToM predicts children's ability to detect automaticity in teaching when controlling for age. This work thus highlights the connections between sensitivity to teaching and reasoning about others' knowledge, with implications for the factors that support children's ability to teach others.
1 Introduction
Pedagogy is a powerful way to learn about the world. Instead of having to learn concepts from scratch through trial-and-error, or make discoveries solely through observation, learners can rely on knowledgeable, well-intentioned people to teach them new things about the world. Learning from others is so fundamental that some have suggested that the natural tendency to teach and learn from other people may itself be a cornerstone of human intelligence (Csibra and Gergely, 2009; Frith and Frith, 2010; Moll and Tomasello, 2007). Moreover, our ability to reason about teaching is early-emerging: Infants spontaneously engage in teaching behaviors within the first year of life (Knudsen and Liszkowski, 2013); they are sensitive to when information is communicated with the intention of teaching (Begus et al., 2016; Gergely et al., 2007; Geraghty et al., 2014); and when asked to teach others, children as young as three naturally engage in the same kinds of ostensive pedagogical cues that are seen in adults (Calero et al., 2015). Investigating the ability to teach and learn from others develops as it develops in early childhood can elucidate the cognitive mechanisms that support natural pedagogy.
Many proposals have been put forth to explain how humans are able to teach and learn from one another (Csibra and Gergely, 2009; Strauss and Ziv, 2012; Strauss et al., 2002; Tomasello, 2019). We take as our starting point a computational framework proposed by Shafto and Goodman (2008). Under this framework, pedagogy can be construed as a set of recursive, mutually dependent inferences: A teacher intentionally samples evidence with the goal of increasing a learner's belief in a target hypothesis; and the learner updates their belief in that hypothesis with the assumption that the evidence has been sampled pedagogically in this way (Bonawitz and Shafto, 2016; Shafto et al., 2012, 2014). This conceptualization of teaching leads to a key prediction: Learners should expect teachers to present evidence that would be maximally helpful to the learner in order to come to the correct solution to the problem.
Indeed, this assumption of “helpfulness” has been found to be true across a variety of different cues and contexts. Children expect teachers to provide true, fully informative demonstrations that prioritize information of higher utility (Bass et al., 2022; Bonawitz et al., 2011; Corriveau and Harris, 2009; Gweon et al., 2014; Jaswal and Neely, 2006; Pasquini et al., 2007). They are sensitive to subtle prosodic cues that indicate whether a question has been asked with the intention to teach or with the intention to solicit information (Bascandziev et al., 2025). Children are also able to go beyond the face value of evidence to reason about the unobservable aspects of teachers that might make them better or worse evidence selectors. In particular, models of pedagogy imply that good teachers should consider what learners need to know, because misconceptions about the beliefs, needs, or goals of learners can result in less helpful teaching. In line with this, research suggests that as teachers, young children can selectively teach the information that would be most helpful for different learners based on that learner's prior knowledge (Bass et al., 2019; Knudsen and Liszkowski, 2013; Ronfard and Corriveau, 2016; see Qiu et al., 2025 for a recent meta-analysis); and as learners, children understand that teachers with false beliefs about their learner's competence may not be able to teach them effectively (Bass et al., 2023). So, children's intuitive theories of pedagogy incorporate not only the evidence that is produced during teaching, but also an understanding that effective teaching requires teachers to accurately represent what learners need to know.
Past work has mainly investigated cases in which teachers have false or incomplete beliefs about a learner. However, there is a more common (and less studied) way in which teachers may fail to represent learners' knowledge: Teachers may simply be “going through the motions” of teaching, without actively engaging with the learner's beliefs, needs, and goals at all. The idea that behavior can be either rote and automatic, or reflective and thoughtful, is not itself new. This behavioral dichotomy has been explored theoretically, empirically, and neurally, in a variety of decision-making frameworks, and even across species (Botvinick, 2012; Dickinson, 1985; Dolan and Dayan, 2013; Etkin et al., 2015; Kahneman, 2011; Liljeholm et al., 2011). However, this past work has primarily focused on how individuals themselves behave. Our interest is in examining this behavioral dichotomy in the inverse direction: How might young learners interpret the actions of others as rote vs. reflective—specifically, in the context of learning and pedagogy?
In order to understand how the ability to infer automaticity in others relates to how children think about teaching, we draw on existing findings on the cognitive mechanisms that support children's pedagogical reasoning. In particular, we focus on Theory of Mind (ToM), the ability to reason about others' mental states, beliefs, and goals. Past work shows that many facets of ToM—including false-belief reasoning, knowledge-gap reasoning, and the ability to distinguish between intentions and outcomes—play a crucial role in supporting children's ability to reason about teaching (e.g., Carlson et al., 2013; Davis-Unger and Carlson, 2008; Strauss et al., 2002; Ye et al., 2021; Ziv et al., 2016). For instance, Bass et al. (2019) conducted three experiments investigating the development of children's ability to select evidence for others in the service of teaching. They found that ToM (as measured by a false-belief battery) predicted children's evidence selection abilities even when controlling for age. They also conducted a 6-week longitudinal experiment during which children's evidence selection abilities were trained. Children in this “pedagogical training” condition, which provided children feedback on the evidence they selected to teach others, showed improvements in their own ToM abilities from before to after the training, whereas those in a control condition did not show such ToM improvements. These findings highlight the inextricable links between the development of reasoning about the minds of others and evidential reasoning in pedagogy.
There is reason to believe that the ability to infer automaticity in the behavior of others is also tied to ToM and pedagogical reasoning. Automaticity inferences necessarily involve reasoning about others' mental states (or lack thereof), and so it stands to reason that ToM development may support this ability—but this has yet to be empirically demonstrated. Furthermore, recent work shows that adults are sensitive to others' automatic behavior not only in their everyday interactions with others (Ullman and Bass, 2024), but also specifically in pedagogical contexts (Bass et al., 2024). In these studies, “rote” teaching was operationalized by manipulating several different aspects of the teaching interaction, including the repetitiveness of feedback across students, the apparent attentiveness of the teacher to the student's readiness to receive feedback, and the presence or absence of speech disfluencies (e.g., “um,” “uh”). In all cases, adults consistently evaluated rote-seeming teachers as worse (Bass et al., 2024). So, by adulthood, people are sensitive to behavioral cues that could indicate automatic reasoning in others, and they believe that automatic behavior makes for worse teaching. But in order to understand how reasoning about others' automaticity factors into intuitive theories of pedagogy, we must investigate it in development, and tie it to established relevant cognitive mechanisms, such as ToM.
For the purposes of our experiments, we operationalized “rote” teaching using repetitiveness, where more repetitive feedback to different students should indicate more rote reasoning. We emphasize that repetitiveness is just one of many cues that could be used to infer others' automaticity (Bascandziev et al., 2025; Heller et al., 2015; Kidd et al., 2011), but it has been used to successfully induce judgments of rote-ness in past work with adults (Bass et al., 2024; Gershman et al., 2016), and thus serves as a reasonable starting place for initial investigations with children. Moreover, although the efficacy of individualized feedback has long been touted as an effective teaching strategy (e.g., Ambrose et al., 2010), our interest is in establishing whether children use such tailoring as a cue to the mental processes underlying the teacher's pedagogy.
We also note that in this paper, we investigated children's pedagogical reasoning as observers or recipients of teaching, and not as teachers themselves. However, the kinds of teaching strategies that children deploy align with what they think makes other people good teachers. For instance, Davis-Unger and Carlson (2008) found that with age, children tend to teach for longer periods of time, and use a more diverse range of teaching strategies. Children are also able to select representative samples to teach (and conversely misleading samples to deceive) others (Rhodes et al., 2015). Similarly, when learning from others, children prefer to learn from teachers who provide more information (Bass et al., 2022; Gweon et al., 2014), and they are sensitive to the idea that different learners may require different kinds of information in order to learn best (Bass et al., 2023; Bonawitz et al., 2011). Thus, although the current work may only be able to indirectly inform our understanding of children's teaching, we believe it has clear implications for models of pedagogical reasoning more broadly.
We sought to replicate ongoing work suggesting that children are sensitive to when others are relying on automatic scripts in the context of teaching (Bass et al., in prep.),1 and tie these sensitivities to pedagogical reasoning and Theory of Mind. This ongoing work found developmental effects of children's sensitivity to automatic behavior in both pedagogical and non-pedagogical contexts. However, that work leaves open central questions, including (1) how the ability to detect and evaluate automaticity in teaching is related to classic measures of pedagogical reasoning, and (2) what the cognitive mechanisms are that support these abilities in early childhood. In the current work, we recruited 3- to 7-year-old children, as this age range captures a prime transitional time in the development of both pedagogical reasoning (e.g., Bass et al., 2022) and ToM (e.g., Wellman and Liu, 2004). We made two general predictions. First, we hypothesized that sensitivity to a teacher's perceived automaticity would be linked to classic measures of pedagogical sensitivity and learning—specifically, how children explore and learn about novel toys following pedagogical vs. non-pedagogical demonstrations. A wealth of past work has found that children tend to constrain their exploration to demonstrated features of toys when those features are demonstrated in an intentional, pedagogical way (i.e., “This is how my toy works!”), as opposed to accidentally (i.e., “Whoops, did you see that?”; Bonawitz et al., 2011; Gweon et al., 2014; Shneidman et al., 2016; Yu et al., 2018; Jean et al., 2019). We expected that the degree to which children showed sensitivity to such pedagogical manipulations on a novel toy exploration task would be related to their ability to infer automaticity in teachers. Second, we hypothesized that the development of ToM (and age differences more broadly) would relate to these pedagogical sensitivities, while unrelated cognitive abilities (such as counterfactual reasoning) would not predict children's performance on these pedagogical tasks above and beyond effects of age.
2 Method
Children were recruited and run online via Children Helping Science, an online platform that allows families from geographically diverse areas to participate in unmoderated research studies at home on their own time (Scott and Schulz, 2017). More specifically, these studies were run as part of Project GARDEN, a research initiative aimed at building out an online platform for studying cognitive development in large samples of demographically diverse children (Sheskin et al., 2020). Children who participate in Project GARDEN complete many different tasks over several testing sessions (called “modules”), with the goal being to build a more complete understanding of how different facets of cognitive development relate to each other within children and over time.
We administered several different online tasks to explore the relationship between pedagogical reasoning and Theory of Mind, controlling for other cognitive abilities and age. In the primary task, we investigated children's ability to infer whether teachers are relying on rote, automatic reasoning processes. In a second task, we investigated how children explored a novel toy following intentionally pedagogical vs. accidental demonstrations from a teacher. On separate days, children also completed Theory of Mind and Counterfactual Reasoning batteries, respectively. Because the number of children who passed technical/comprehension checks to complete these modules through Project GARDEN fell below our pre-registered sample, we also collected data from an additional group of children. Our primary interests centered on the relationship between children's reasoning about automaticity in pedagogy and Theory of Mind, so we focused this additional testing on just the Rote Teaching task and a shortened version of the ToM battery, which we ran as a single testing session (see below for details). Children in this group completed the shortened ToM battery first, and the Rote Teaching task second.
Unless denoted as exploratory, all methods and analyses were pre-registered via AsPredicted and OSF. All reported p-values are 2-tailed. Pre-registrations, de-identified data, analysis scripts, and study materials can all be found in the following OSF repository.
2.1 Rote teaching task
Before beginning the task, parents provided informed consent, and children received training on how to click objects on the screen and say answers out loud. Children then began the primary experimental task. They were told that we (the researchers) were interested in learning about what kids think about teachers, and that they would be watching some videos of teachers and answering some questions about them.
The first part of the study was used for inclusion criteria only. Participants saw videos of an Accurate Teacher and an Inaccurate Teacher (order counterbalanced). In the Accurate Teacher video, an adult provided correct labels for familiar objects (e.g., labeling a rubber duck as a “duck”); in the Inaccurate Teacher video, an adult provided incorrect labels for familiar objects (e.g., labeling a bunny stuffed animal as a “cow”). After watching these videos, participants were asked to choose which teacher they would rather learn from.
The second part of the study included our primary manipulation. Participants saw videos of two different teachers, “Alex” and “Laura,” each of whom provided feedback to three different students on drawings they were ostensibly making. In one video, the teacher provided identical feedback to the three students (e.g., “Make sure to color inside the lines,” “Make sure to color inside the lines,” “Make sure to color inside the lines”); in the other video, the teacher instead provided unique feedback to each student (e.g., “Make sure to fill the whole page,” “Make sure to use some different colors,” “Make sure everything goes together”). We intentionally matched the length, positivity, and potential helpfulness of the verbal feedback across conditions as closely as possible. All children saw Alex first and Laura second. Because the teachers in these videos wore face masks, all children saw exactly the same visual stimuli, but with different audio dubbed on top, depending on the counterbalancing order to which they were randomly assigned (identical-unique, unique-identical). This allowed us to control for any potential confounds in the video stimuli themselves. Critically, children were not actually able to see the drawings themselves. This was to ensure that the actual relevance of the feedback for the projects was equally ambiguous across conditions. The video stimuli used in this study are available in our OSF repository.
After watching both of these videos, participants answered two forced-choice questions: (1) Which teacher was not really thinking carefully about the students and their drawings?2 (2) Which teacher would you rather learn from? On both questions, responses were coded as 1 if children chose the identical-feedback teacher and 0 if they chose the unique-feedback teacher.
2.2 Novel toy exploration task
At the end of the Rote Teaching task, families recruited through Project GARDEN were asked to continue on to complete the Novel Toy exploration task, which was hosted on an external Heroku web server and displayed to families in an iFrame within the Children Helping Science experiment page. In this second task, children saw two videos of teachers demonstrating a function on a virtual novel toy on a computer screen. Each teacher demonstrated one function on a different novel toy, each of which had a total of four hidden functions that could be activated. One teacher provided this demonstration intentionally and pedagogically (e.g., “This is my toy! I'm going to show you how this toy works… See that? I clicked here on the toy, then a duck appeared and made a noise…”). The other teacher provided the demonstration accidentally (e.g., “I've never seen this toy before! I wonder how it works… Oops, did you see that? I clicked here on the toy, then a pinwheel spun and made a noise…”). After watching each video, children were given the opportunity to play with the virtual toys themselves for up to 90 s. We collected data from children's clicks and mouse movements during these play periods. For the purposes of these analyses, and corresponding to in-person exploration measures previously found to vary by demonstration-type, the main dependent variables were (1) how many times children clicked on the demonstrated function, and (2) the proportion of children's clicks that were on the demonstrated function. We analyzed both the total number of clicks, and the proportion of clicks on the demonstrated function, because they may reflect subtly different processes related to the breadth and depth of children's exploration (Loewenstein, 1994; Henderson and Moore, 1979). Specifically, the total number of clicks on the demonstrated function may highlight whether different pedagogical contexts elicit variability in the depth of children's exploration (Nussenbaum and Hartley, 2019), whereas the proportion of total clicks on the demonstrated function may reflect how pedagogy relates to the breadth of exploration (i.e., how widely they search/explore), highlighting relations between pedagogical contexts and children's general curiosity (Blanco and Sloutsky, 2020). After children were done playing with each toy, they were asked to identify how to make each of the four functions activate.
2.3 Theory of Mind battery
The 7-item ToM battery was closely modeled on the work of Wellman and Liu (2004), and included the following tasks: (1) Diverse Desires (understanding that other people will act based on their own desires, even if they're different from your own); (2) Diverse Beliefs (understanding that other people will act based on their own beliefs, even if they're different from your own); (3) Knowledge Access (understanding that other people may not have the same knowledge as you); (4) Contents False Belief (understanding that people form beliefs based on their perception, and that those beliefs can be false); (5) Explicit False Belief (understanding that people can have false beliefs about the world, and expecting them to act on those false beliefs); (6) Belief-Emotion (understanding that people's beliefs lead them to experience emotions, even when those beliefs are false); and (7) Appearance-Reality Emotion (understanding that people might deliberately try to appear as though they're experiencing one emotion while actually experiencing a different emotion). See our online Supplementary material for a full description of each of these tasks.
Children who completed the ToM battery as part of Project GARDEN saw all seven tasks. For children who separately completed the ToM battery and the Rote Teaching task in a single testing session, we shortened the battery to five items by dropping the Diverse Desires and Diverse Beliefs items. We chose to drop these items for two main reasons. First, conceptually, we anticipated that these simpler tasks would be less predictive of the more sophisticated pedagogical reasoning abilities we were investigating here. Second, practically, within the GARDEN dataset we found that children's performance on the two simpler tasks were largely captured by their performance on the five more complex tasks: Of the children who gave correct responses on each of the five more complex tasks, an average of 86% also answered correctly on Diverse Desires and Diverse Beliefs (Aboody et al., in prep.).3
We created a composite ToM reasoning score by taking the sum of correct answers divided by the total number of questions attempted, excluding trials where participants failed an associated control question. Thus, scores ranged from 0 to 1.
2.4 Counterfactual reasoning battery
Participants first completed a short, five question training exercise to practice clicking and navigating the online platform. Children then completed 14 Counterfactual Reasoning tasks in three randomized blocks. The first block asked children six questions about different story worlds, varying where a character's object was located by the end of the storyline (Narrative Task, six total items; Rafetseder et al., 2010). This block assessed children's ability to arrive at a correct answer without considering past events in some questions (basic conditional reasoning), or factual details in others (counterfactual reasoning). The second randomized block tested children's ability to reason counterfactually in a physical domain (Physical Reasoning Task, four total items; Kominsky et al., 2021). The third randomized block tested children's ability to reason counterfactually when presented with a novel stimulus (Novel Structure Task, four total items; Nyhout and Ganea, 2019; Gopnik and Sobel, 2000). These measures strongly correlate (Wong et al., in prep.),4 so we generated a composite Counterfactual Reasoning battery score by dividing the sum of correct answers by the total questions attempted (maximum of 14 questions), resulting in scores ranging from 0 to 1. See our online Supplementary material for a full description of each of these tasks.
2.5 Participants and inclusion criteria
There were a total of 205 unique responses on the Rote Teaching/Novel Toy tasks completed through the GARDEN module, and 78 unique responses on the supplemental non-GARDEN Rote Teaching/ToM tasks.
We first dropped “ineligible” responses (N = 22 for GARDEN, N = 12 for non-GARDEN), which were responses that did not meet the inclusion criteria stated on the Children Helping Science study page. For instance, it could be that children participated in the task more than once; that they were not in the target age range for the study; that they did not finish the task; or that they had not completed the previous Project GARDEN modules.
Following past work (e.g., Gweon et al., 2014; Gweon and Asaba, 2018) and our pre-registration, in the Rote Teaching task we excluded children who did not choose the Accurate Teacher as the teacher they would want to learn from in the forced-choice attention-check (N = 49 for GARDEN, N = 18 for non-GARDEN). The key findings reported below also hold qualitatively when including children who failed this check; see our online Supplementary material for details.
In the Novel Toy task, three task-related exclusion criteria were pre-registered and applied. First, children who failed to correctly identify how to activate the demonstrated function were excluded (N = 41). Second, if the participant immediately clicked the “ALL DONE” button without interacting with either toy, they were excluded (N = 1). Third, we dropped individual observations that exceeded three Standard Deviations of the mean of the respective measure. Our two primary variables of interest were the difference scores between conditions (Pedagogical–Accidental) for the number of clicks on the demonstrated function, and the proportion of clicks on the demonstrated function. There were three datapoints that were more than three Standard Deviations away from the mean for the Number of Clicks difference score, and four datapoints for the Proportion of Clicks difference score. Therefore, we dropped a total of seven observations across these two measures for falling outside our three Standard Deviation cut-off.
Our final sample in the Rote Teaching task (combined across GARDEN and non-GARDEN datasets) consisted of N = 178 children (average age = 5.55 years, range = 3.00–7.98 years; N = 85 girls). Our final sample in the Novel Toy task (GARDEN only) consisted of N = 114 children (average age = 5.43 years, range = 3.18–7.40 years; N = 59 girls). There were 76 unique children that completed both the Rote Teaching and the Novel Toy tasks (average age = 5.71 years, range = 3.18–7.40 years; N = 38 girls).
2.6 Computing projected scores for Theory of Mind and counterfactual reasoning
For children from Project GARDEN, there was a time delay between when they completed the ToM and Counterfactual Reasoning modules and when they completed the Rote Teaching and Novel Toy module. This delay was not the same for all participants: Some children might have completed the modules only a few days apart, while others might have done so several months apart. Therefore, it was important to control for likely developmental change between the pretested measures and our teaching measures. To do so, we computed “projected” ToM and Counterfactual Reasoning scores to estimate what children's battery scores would be at the time of completing the Rote Teaching and Novel Toy tasks. We ran two linear regression models with age predicting ToM and Counterfactual Reasoning battery scores, for all children who completed either of these tasks. Age was positively and strongly linearly related to both ToM [r(409) = 0.505, p < 0.001] and Counterfactual Reasoning [r(283) = 0.710, p < 0.001]. The slope of the best-fit line was 0.093 for ToM scores and 0.161 for Counterfactual Reasoning scores. In other words, for every year-increase in age, children's ToM scores and Counterfactual Reasoning scores increased by 0.093 and 0.161, respectively. Of course, although the group was well-fit by linear models, different children certainly develop these abilities at different (and not necessarily linear) rates. However, given the stark differences across children in how much time passed between modules, we felt that using an imperfect group-based proxy for projecting scores would likely be more accurate and contribute less unwanted variance than using non-projected scores. To this end, we used these group-based slopes to impute ToM and Counterfactual Reasoning scores (capped at 1) for each child, based on how much time had passed between testing sessions and children's initial ToM and Counterfactual Reasoning scores: scoreprojected = slope(agemodule2 − agemodule1) + scoremodule1. Projected scores were used in all analyses described below (although we note that all findings reported below qualitatively replicate when using non-projected scores; see our online Supplementary material for details).
3 Results
3.1 Main effects: rote teaching task
We began by analyzing main effects of the Rote Teaching and Novel Toy tasks separately. In the Rote Teaching task (N = 178), we examined the proportion of children who chose each teacher (repetitive vs. unique) in each of the two test questions (“who wasn't really thinking?” and “who would you rather learn from?”). We found that 131 children (73.6%) chose the repetitive-feedback teacher as the one who wasn't really thinking. Similarly, we found that 125 children (70.2%) chose the unique-feedback teacher as the one they would prefer to learn from. Both of these proportions differed significantly from chance (ps < 0.001 by binomial test). These findings replicate ongoing work (Bass et al., in prep.)(see text footnote 1) and suggest that on the whole, children can indeed use repetitiveness as a cue to whether someone is “really thinking” and choose to learn from teachers who seem to be thinking carefully about their students (see Figure 1).
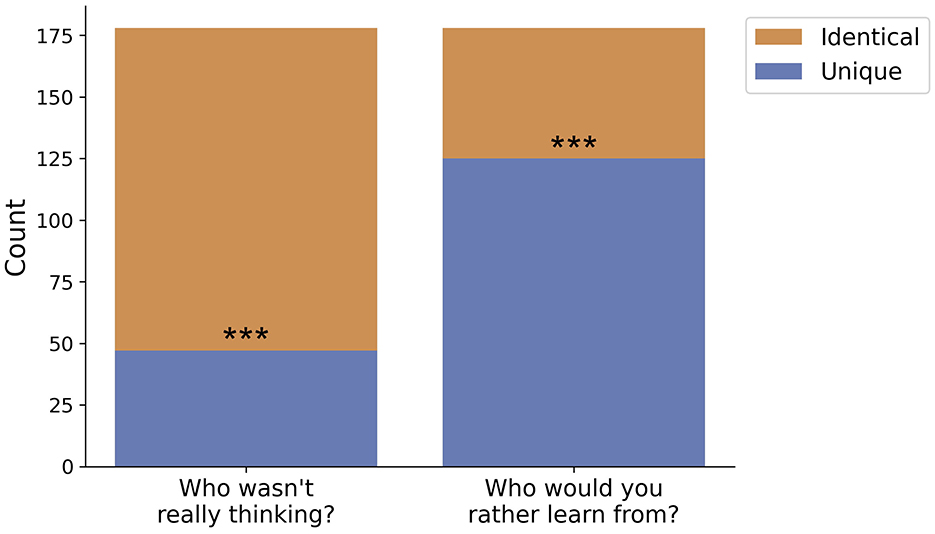
Figure 1. The proportion of children who chose the identical-feedback vs. unique-feedback teacher on each of the test questions in the Rote Teaching task. Children overwhelmingly chose the teacher who provided identical feedback as the one who wasn't really thinking, and the teacher who provided unique feedback as the one they would prefer to learn from. ***p < 0.001.
3.2 Main effects: novel toy task
In the Novel Toy task (N = 114), we compared difference scores between the Pedagogical and Accidental conditions on the number and proportion of demonstrated function clicks to 0, via one-sample t-tests. We would expect that children sensitive to pedagogical cues should focus on the demonstrated function more in the Pedagogical condition than in the Accidental condition, yielding positive difference scores. The number of clicks did not significantly differ across conditions [t(110) = 1.54, p = 0.126], while the proportion of clicks did [t(109) = 2.12, p = 0.036]; however, the direction of these effects was the opposite of what was predicted, with children clicking on the demonstrated function at greater rates in the accidental condition (M = 0.203, SD = 0.192) than in the pedagogical condition (M = 0.178, SD = 0.179). There are several possible, non-mutually exclusive reasons that our online pedagogical manipulation failed, which we address in the Discussion below. Whatever the case may be, the current results fail to replicate a wealth of past work finding that children tend to focus on features more when they were demonstrated pedagogically (Bonawitz et al., 2011; Gweon et al., 2014; Shneidman et al., 2016; Yu et al., 2018; Jean et al., 2019). While our novel toy task did not capture children's pedagogical reasoning in the way we expected based on the literature, it is still possible for children's behavior on this task to interact significantly with other measures, which we consider next.
3.3 Relationship between rote teaching and novel toy behaviors
Many of the subsequent analyses in our pre-registrations relied on a combined pedagogical reasoning composite score computed from the Rote Teaching and Novel Toy tasks. Therefore, despite the unpredicted lack of differences between conditions overall in the Novel Toy task, we still wanted to know whether behaviors in the Rote Teaching and Novel Toy tasks correlated with each other. To assess this, we ran two logistic regression models (N = 76) predicting whether the child chose the identical-feedback teacher as the one who wasn't thinking from each of the two Novel Toy difference scores (number of clicks on demonstration, proportion of clicks on demonstration). While the regression with the number of clicks was not significant [ = 1.62, p = 0.20], the model using proportion of clicks was [ = 5.06, p = 0.024, Pseudo-R2 = 0.056], with difference scores significantly predicting the likelihood of choosing the repetitive teacher (b = 5.26, SE = 2.47, z = 2.13, p = 0.033). Specifically, children who explored the demonstrated function more in the Pedagogical condition than in the Accidental condition (showing sensitivity to pedagogical cues) were also more likely to “correctly” choose the identical-feedback teacher as the one who wasn't thinking. So, despite the pedagogical manipulation in the novel toy task failing to reveal condition differences in the aggregate, those children who were sensitive to the teacher's pedagogical demonstration were also more attuned to cues to automaticity in teaching. However, we note that this result does not withstand controlling for age (Age: p < 0.001; Proportion of Clicks Difference Score: p = 0.152), suggesting that this result is weak, or potentially driven by external causal variables associated with maturation that support reasoning in both tasks.
For the pedagogical reasoning composite score, children were to receive a point if they chose the identical-feedback teacher as “not really thinking” and a point if they showed a positive difference between the Pedagogical and Accidental demonstrated function responses, yielding scores ranging from 0 to 2. However, an exploratory Fisher's exact test on whether children picked the rote teacher (1, 0) and whether children focused on the demonstrated function more in the pedagogical condition (1, 0) was not significant (p = 0.44). In addition to these mixed results, there is also the issue of statistical power: In our pre-registration, we anticipated needing over 200 participants in order to detect developmental effects in the pedagogical reasoning composite score. However, due to attrition and combined exclusion rates across the two tasks, we had only 76 participants with usable data across both tasks, compared with 178 usable data points in the Rote Teaching task. For these reasons, we opted to use only the Rote Teaching task as our primary outcome measure of pedagogical reasoning.
3.4 Cognitive mechanisms underlying pedagogical reasoning
We wanted to know whether children's age and ToM were related to their ability to reason about automaticity in teaching. We were primarily interested in ToM, as the detection of automatic behaviors implicitly requires reasoning about others' goals and mental states. The Counterfactual Reasoning battery primarily served as a control measure, accounting for a general measure akin to cognitive age of the participant. To this end, we ran a series of logistic regression models including age (in years, continuous), ToM (z-scored), and Counterfactual Reasoning (z-scored) as predictors of children's choices on the rote teaching task. Sample sizes vary across analyses and are reported at the beginning of each section. Results from these regression models are summarized in Tables 1, 2.
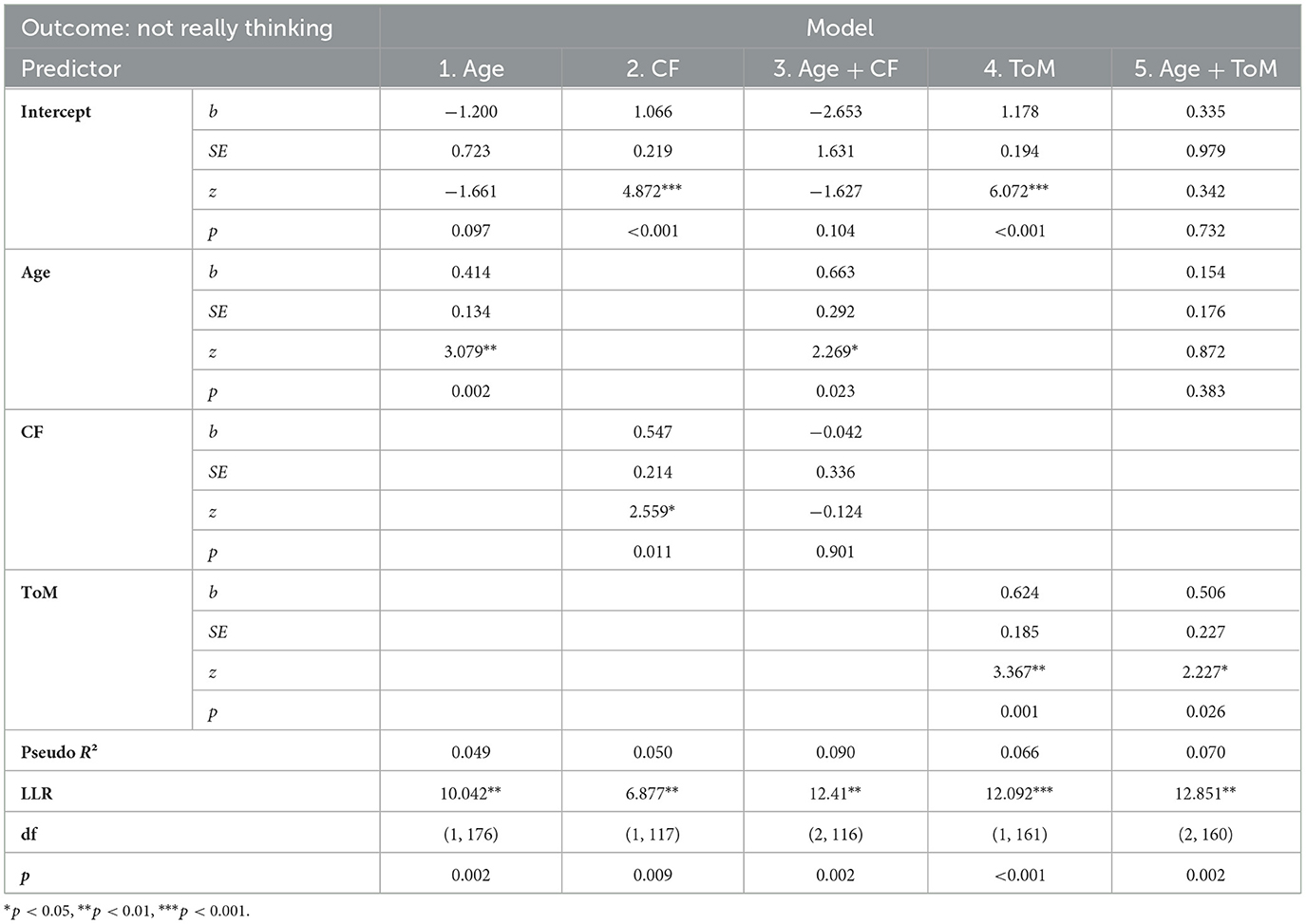
Table 1. Results from the five logistic regression models predicting children's choices on the “Who wasn't really thinking?” question in the Rote Teaching task from age, Counterfactual Reasoning, and ToM.
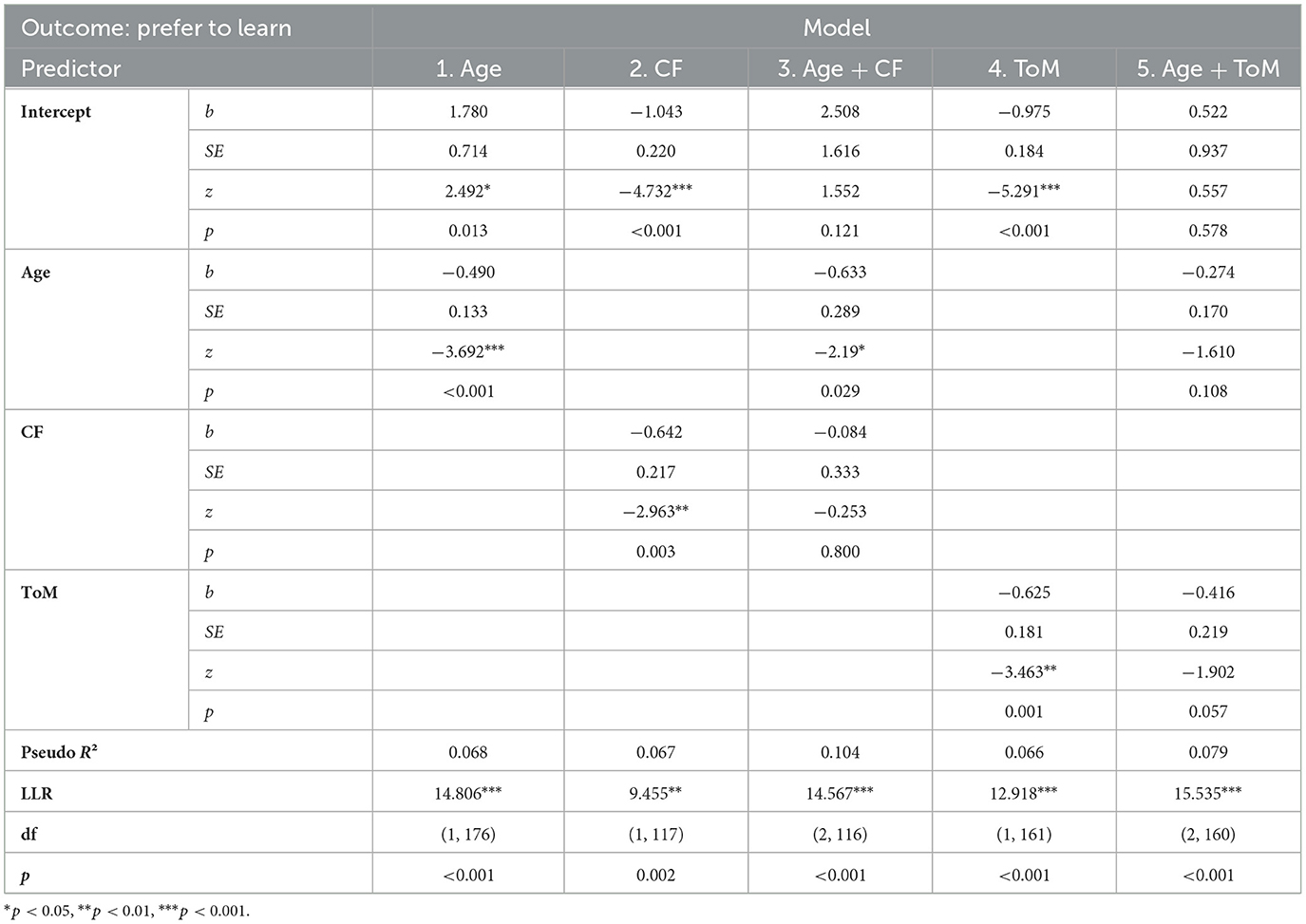
Table 2. Results from the five logistic regression models predicting children's choices on the “Who would you rather learn from?” question in the Rote Teaching task from age, Counterfactual Reasoning, and ToM.
3.4.1 Model 1: age
Following our pre-registered analysis plan, we first asked whether children's age was related to their reasoning about rote teaching. We ran two logistic regressions (N = 178) predicting children's choices on the Rote Teaching task from their age. These regressions were both significant, with older children being more likely to correctly choose the identical-feedback teacher as the one who wasn't really thinking (b = 0.414, SE = 0.134, z = 3.079, p = 0.002) and the unique-feedback teacher as the one they would prefer to learn from (b = −0.490, SE = 0.133, z = −3.692, p < 0.001; see Figure 2). Consistent with other work (Bass et al., in prep.)(see text footnote 1), these results demonstrate that children's ability to reason about automaticity in pedagogy improves with development.
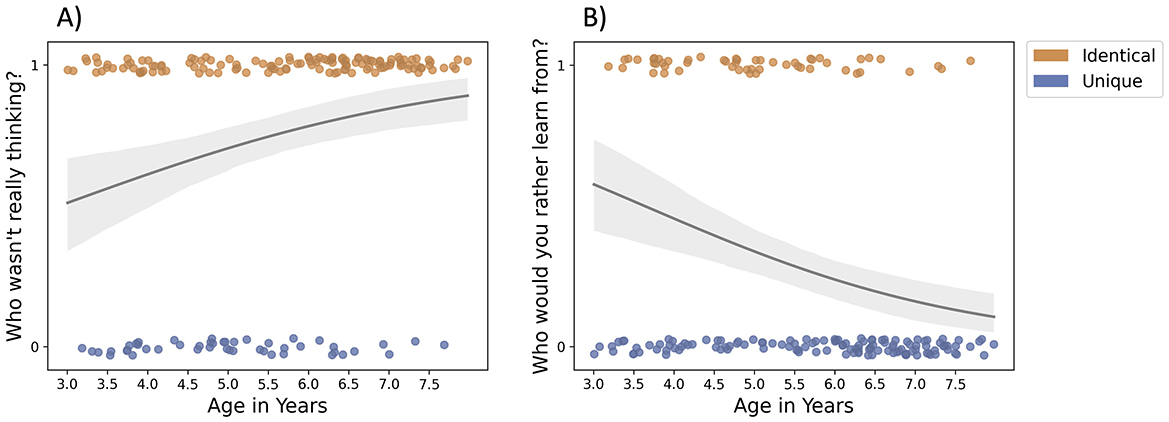
Figure 2. Results from the logistic regressions predicting children's choices on the Rote Teaching test questions [(A) “Who wasn't really thinking?”; (B) “Who would you rather learn from?”] from their age (Model 1). As they got older, children were more likely to choose the identical-feedback teacher as the one who wasn't really thinking, and the unique-feedback teacher as the one they would prefer to learn from.
To understand whether these are effects of maturation or the development of particular cognitive mechanisms, we next ran regression models including Counterfactual Reasoning and ToM. We start with Counterfactual Reasoning, which we expected not to explain performance on the Rote Teaching task above and beyond age.5 Then we investigated the role of ToM, which we predicted would be independently related to children's ability to reason about automaticity in teaching.
3.4.2 Models 2 and 3: counterfactual reasoning, age + counterfactual reasoning
We ran logistic regression models with Counterfactual Reasoning predicting children's choices on each test question in the Rote Teaching task (N = 119). These models were significant: Children's Counterfactual Reasoning scores were significantly related to their tendency to pick the identical-feedback teacher as the one who wasn't really thinking (b = 0.547, SE = 0.214, z = 2.559, p = 0.011), and the unique-feedback teacher as the one they would rather learn from (b = −0.642, SE = 0.217, z = −2.963, p = 0.003). However, when including age in the model, Counterfactual Reasoning scores no longer significantly predicted children's choices (ps ≥ 0.800), while age continued to be a significant predictor (not really thinking: b = 0.663, SE = 0.292, z = 2.269, p = 0.023; prefer to learn: b = −0.633, SE = 0.289, z = −2.190, p = 0.029). Therefore, as expected, Counterfactual Reasoning abilities did not explain children's ability to reason about automaticity in pedagogy above and beyond age.
3.4.3 Models 4 and 5: ToM, age + ToM
Finally, we asked whether ToM predicted children's ability to reason about automaticity in teaching (N = 163). We found that children's ToM scores were significantly related to their tendency to pick the identical-feedback teacher as the one who wasn't really thinking (b = 0.624, SE = 0.185, z = 3.367, p = 0.001), and to pick the unique-feedback teacher as the one they would rather learn from (b = −0.625, SE = 0.181, z = −3.463, p = 0.001; see Figure 3). When including age in the model, ToM continued to significantly predict children's ability to recognize the repetitive teacher as the one who was not really thinking (b = 0.506, SE = 0.227, z = 2.227, p = 0.026), while age did not (p = 0.383). Thus, independent of age, ToM predicts children's ability to detect automatic behavior. In the model predicting children's tendency to choose the unique-feedback teacher as the one they would rather learn from, neither age nor ToM remained significant predictors (ps ≥ 0.057), suggesting that mental state reasoning may specifically support children's ability to detect automaticity in pedagogical contexts, but not necessarily their subsequent decisions about from whom they would prefer to learn.
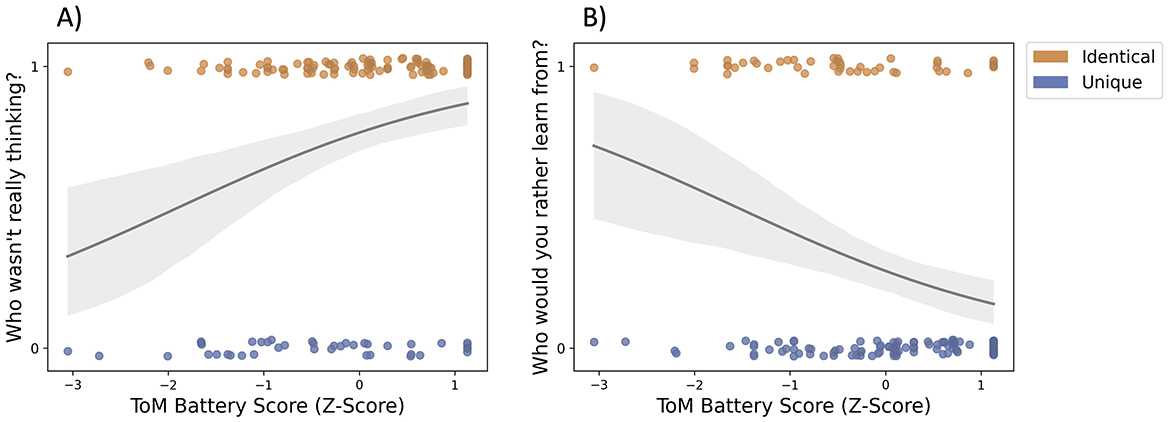
Figure 3. Results from the logistic regressions predicting children's choices on the Rote Teaching test questions [(A) “Who wasn't really thinking?”; (B) “Who would you rather learn from?”] from their ToM battery score (Model 4). Children who performed better on the ToM battery were more likely to choose the identical-feedback teacher as the one who wasn't really thinking, and the unique-feedback teacher as the one they would prefer to learn from.
4 Discussion
Teaching is a unique and powerful way to learn about the world. A better understanding of how young children learn from and teach others will lead to a clearer cognitive and theoretical picture of natural pedagogy. In the current paper, we investigated an unexplored factor in children's pedagogical reasoning: Their ability to detect when other people are operating on “automatic,” going through the motions of teaching. We sought to tie children's reasoning about rote behavior to established measures of pedagogical reasoning (i.e., a novel toy exploration task), and to mental-state reasoning abilities, which have been shown to support pedagogical reasoning in past work. We did not find a robust link between children's automaticity inferences and their exploration on the Novel Toy task. However, we did find that ToM predicted children's sensitivity to behavioral cues to automaticity, better than unrelated cognitive abilities (i.e., counterfactual reasoning), and even when controlling for age. This work highlights the deep connections between sensitivity to teaching and reasoning about others' knowledge.
Establishing a link between ToM and automaticity inferences is exciting for several reasons. For one, it extends past work on the connection between ToM and children's ability to teach (Bass et al., 2019; Davis-Unger and Carlson, 2008; Ziv et al., 2016) and learn from others (Wellman and Lagattuta, 2004). Our findings suggest that formal frameworks of ToM and models of pedagogy may need to be revised to additionally integrate the inference of rote behavior. The current findings also suggest how inferences about others' automatic behavior operate. Standard ToM models suggest that we attribute mental states to other people in order to explain their behavior. But how might we reason about people's behavior when we think they're acting automatically, with no active mental states to recover? The current findings suggest that, at a minimum, these abilities are intertwined in development.
Why was our novel toy exploration task unable to replicate the well-established finding that children focus more on demonstrated features in pedagogical contexts? While we intended our online novel toy task to be a classic measure of pedagogical sensitivity and learning, all previous work of which we are aware that has used this task have been run in person, with a live experimenter and physical toys. Novel toy teaching paradigms have not yet been successfully implemented in online, unmoderated studies, and there are many reasons that our task might have failed in this online format. For one, children might have interpreted the “accidental” condition as more intentional in this unmoderated context (e.g., “If they were really accidentally pressing the function, why were they recording themselves? Why did they demonstrate the function a second time?”). This would be commensurate with past work finding that 2-year-olds reproduce both pedagogical and non-pedagogical but intentional demonstrations at similar rates (Bazhydai et al., 2020; Vredenburgh et al., 2015). On the other hand, perhaps the pedagogical manipulation did work as intended, but uncertainty surrounding how digital functions work led children to believe there was more to discover about the demonstrated function in the accidental condition. There were also several methodological differences between our task and previous in-person novel toy exploration tasks (shorter playtime cutoffs, a within-subjects manipulation with two teachers and two toys, deterministic function activation, etc.), any number of which might have contributed to these unexpected results. In ongoing work, we are redesigning this task to address these possibilities, in order to understand whether pedagogical reasoning may still be assessed using an online and unmoderated novel toy paradigm.
In the current study, children agreed that repetition of information across students signals non-thinking in teachers, and that such rote behavior leads to less desirable teaching. However, we exclusively recruited U.S.-based children, and so whether these findings generalize across cultural contexts is an open question. Indeed, it has long been suggested that there is systematic cultural variation in how teaching unfolds both at home (LeVine et al., 2012) and in the classroom (Hofstede, 1986); and recent work has found cultural differences in children's sensitivity to others' knowledge when teaching and learning from others (e.g., Kim et al., 2018; Ye et al., 2025). Therefore, it is entirely possible that expectations about, and sensitivities to, rote behavior in teaching could vary dramatically across cultural contexts. This would be an exciting direction for future work.
Practically, our work has potential implications for classroom learning. Current approaches in education are moving toward automation in teaching. Since the COVID-19 pandemic, remote learning strategies such as virtual classrooms and pre-recorded lectures have become more common. Although there is a long history of adaptive learning and intelligent tutoring systems (e.g., see Martin et al., 2020; Mousavinasab et al., 2021 for reviews), more recently, Large Language Models and AI teaching assistants have emerged as possible tools for use in the classroom (Gligorea et al., 2023; Ezzaim et al., 2024). But we still know relatively little about how children reason about and learn from teachers who seem to be “not really there”—whether it is because they are a distracted teacher, a pre-recorded lecture, or an AI tutoring bot. The current work investigates children's reasoning about rote behavior when learning from other people, but in principle, either AI or human teachers can give the impression that they are behaving in an automatic way (or not). Our results show that children are sensitive to cues that indicate when others are behaving automatically; that they prefer to learn from teachers when they seem not to be behaving automatically; and that these abilities and preferences become more pronounced with development. This suggests important differences in how children may choose to engage with and learn from automated sources of teaching, and whether our findings generalize to learning scenarios involving other kinds of automatic teaching is an open question. It will be crucial for future work to explore how inferences about automaticity influence learning outcomes in both formal and informal educational contexts.
Beyond the specific findings of this work regarding pedagogy, we wish to highlight its contributions—and those of Project GARDEN as a whole—to the landscape of developmental research more broadly. The body of findings about children's ability to teach and learn from others and reason about others' minds is vast and diverse. But this work is often siloed, and so it can be difficult to build unified frameworks for understanding children's social and pedagogical reasoning. The current work is part of an ongoing effort in development to establish a “unified, discipline-wide, online Collaboration for Reproducible and Distributed Large-Scale Experiments (CRADLE)” (Sheskin et al., 2020, p. 675; see also Frank et al., 2017). By running large-scale studies and sharing data across research teams, while also leveraging the diverse pool of participants afforded by hosting unmoderated online studies, we can develop more sophisticated and generalizable theories of how different facets of cognition relate to one another across development.
A limitation of this work is that it does not directly investigate children's ability to teach others. Instead, we asked how children think about teachers and teaching more generally, with the assumption that understanding children's evaluations of teaching (and the factors that contribute to its development) may similarly inform our understanding of their execution of teaching. So, although our findings suggest the possibility that children may reason about automaticity when acting as teachers themselves, and that these abilities should be tied to mental-state reasoning, future work could directly investigate this question: As teachers, do children reason about their learners' automaticity? For adults, this feels intuitive: Many of us might be able to remember a time when we were trying to explain something to a child or student, and noticing a glazed look in their eyes. “Are you even paying attention to me right now?”, you might have inquired, only for them to respond with a robotic “uh-huh.” Indeed, under models of pedagogical reasoning, effective learning depends just as much on learners actively reasoning about the teacher and the evidence as it does on teachers actively reasoning about the learner's current beliefs and how different evidence will support revision. How children reason about and adapt their teaching in situations in which their student is merely going through the motions of learning would be an exciting direction for future work.
Our work provides important and novel insights into children's early developing knowledge and expectations about pedagogy as they relate to social reasoning more broadly. We extended past work on children's pedagogical reasoning, and showed that children become more sensitive to automatic-seeming behaviors in pedagogy with development. Furthermore, we found that the ability to detect automaticity is uniquely linked to the ability to reason about others' minds in more traditional Theory of Mind tasks. Our work suggests that when it comes to pedagogy, reasoning about what others are thinking supports reasoning about when others are thinking.
Data availability statement
The datasets presented in this study can be found in online repositories. The names of the repository/repositories and accession number(s) can be found below: https://osf.io/s7qgp/?view_only=405ce13a7fe94a00b45f3fa82ac125f3.
Ethics statement
These studies were approved by Committee on the Use of Human Subjects, Harvard University Area Institutional Review Board. The studies were conducted in accordance with the local legislation and institutional requirements. Informed consent for participation in this study was provided by the participants' legal guardians.
Author contributions
IB: Conceptualization, Data curation, Formal analysis, Investigation, Methodology, Project administration, Software, Validation, Visualization, Writing – original draft, Writing – review & editing, Funding acquisition. JC: Conceptualization, Data curation, Investigation, Methodology, Project administration, Software, Writing – original draft, Writing – review & editing, Validation. RA: Conceptualization, Data curation, Investigation, Methodology, Project administration, Writing – original draft, Writing – review & editing, Software, Validation. MW: Conceptualization, Data curation, Investigation, Methodology, Project administration, Writing – original draft, Writing – review & editing, Software, Validation. TU: Conceptualization, Methodology, Project administration, Resources, Supervision, Writing – original draft, Writing – review & editing. EB: Conceptualization, Funding acquisition, Methodology, Project administration, Resources, Supervision, Writing – original draft, Writing – review & editing.
Funding
The author(s) declare that financial support was received for the research and/or publication of this article. This work was funded by NSF DS (#2042489) to EB. Additionally, IB was supported on NSF STEM Ed IPRF (#2327447) RA on NSF SPRF SBE (#2204171), MW on an NSF GRFP award, and TU by the Jacobs Foundation.
Acknowledgments
We are deeply grateful to the children and families who participated in this research. Thanks to our incredible team of research assistants for help with study design, stimuli creation, coding, piloting, and sending compensation to participating families: Misha O'Keefe, Feifei Shen, Alexandre Lucas, Jazlyn Navarro Jimenez, Kate Fourie, Ransith Weerasinghe, Eliceo Caniza Velaquez, Cecilia (Wenxiu) Wang, Rolands Barkans, Amal Kashif Sabeeh, Yelim Lee, Assyl Zhanuzak, Duy Vu, and Kacper Malinowski. Finally, thank you to the technical support and project management teams at Children Helping Science and Project GARDEN, including Candice Mills, Ian Chandler-Campbell, Melissa Kline Struhl, and Mark Sheskin.
Conflict of interest
The authors declare that the research was conducted in the absence of any commercial or financial relationships that could be construed as a potential conflict of interest.
Generative AI statement
The author(s) declare that no Gen AI was used in the creation of this manuscript.
Publisher's note
All claims expressed in this article are solely those of the authors and do not necessarily represent those of their affiliated organizations, or those of the publisher, the editors and the reviewers. Any product that may be evaluated in this article, or claim that may be made by its manufacturer, is not guaranteed or endorsed by the publisher.
Supplementary material
Supplemental materials, as well as analysis scripts, de-identified data, pre-registrations, and study materials, can be found in our OSF repository.
Footnotes
1. ^Bass, I., Bonawitz, E., and Ullman, T. D. (in prep). Children's evaluations of automaticity in teaching.
2. ^While there are likely more neutral phrasings that could have been used for this question, our interest was in establishing whether children could reason about automatic behavior in teachers at all. So, for this “first pass” of establishing this capability in young children, we opted to use the more direct phrasing presented here.
3. ^Aboody, R., Gweon, H., Jara-Ettinger, J., and Bonawitz, E. (in prep). Theory of Mind development: a large-scale investigation.
4. ^Wong, M., Aboody, R., Kominsky, J. F., Rafetseder, E., and Bonawitz E. (in prep). Development of counterfactual reasoning in preschool aged children.
5. ^At the time of pre-registration, we were unaware that we would also have access to children's Counterfactual Reasoning scores as part of the GARDEN tasks. As a result, Models 2 and 3 were not pre-registered. However, the rationale and predictions for running these models were formulated before looking at the data.
References
Ambrose, S. A., Bridges, M. W., DiPietro, M., Lovett, M. C., and Norman, M. K. (2010). How Learning Works: Seven Research-Based Principles for Smart Teaching. Hoboken, NJ: John Wiley and Sons.
Bascandziev, I., Shafto, P., and Bonawitz, E. (2025). Prosodic cues support inferences about the question's pedagogical intent. Open Mind 9, 340-363. doi: 10.1162/opmi_a_00192
Bass, I., Bonawitz, E., Hawthorne-Madell, D., Vong, W. K., Goodman, N. D., and Gweon, H. (2022). The effects of information utility and teachers' knowledge on evaluations of under-informative pedagogy across development. Cognition 222:104999. doi: 10.1016/j.cognition.2021.104999
Bass, I., Espinoza, C., Bonawitz, E., and Ullman, T. D. (2024). Teaching without thinking: negative evaluations of rote pedagogy. Cogn. Sci. 48:e13470. doi: 10.1111/cogs.13470
Bass, I., Gopnik, A., Hanson, M., Ramarajan, D., Shafto, P., Wellman, H., et al. (2019). Children's developing Theory of Mind and pedagogical evidence selection. Dev. Psychol. 55, 286–302. doi: 10.1037/dev0000642
Bass, I., Mahaffey, E., and Bonawitz, E. (2023). Children use teachers' beliefs about their abilities to calibrate explore-exploit decisions. Top. Cogn. Sci. doi: 10.1111/tops.12714. [Epub ahead of print].
Bazhydai, M., Silverstein, P., Parise, E., and Westermann, G. (2020). Two-year-old children preferentially transmit simple actions but not pedagogically demonstrated actions. Dev. Sci. 23:e12941. doi: 10.1111/desc.12941
Begus, K., Gliga, T., and Southgate, V. (2016). Infants' preferences for native speakers are associated with an expectation of information. Proc. Natl. Acad. Sci. U.S.A. 113, 12397–12402. doi: 10.1073/pnas.1603261113
Blanco, N. J., and Sloutsky, V. M. (2020). Attentional mechanisms drive systematic exploration in young children. Cognition 202:104327. doi: 10.1016/j.cognition.2020.104327
Bonawitz, E., and Shafto, P. (2016). Computational models of development, social influences. Curr. Opin. Behav. Sci. 7, 95–100. doi: 10.1016/j.cobeha.2015.12.008
Bonawitz, E., Shafto, P., Gweon, H., Goodman, N. D., Spelke, E., and Schulz, L. (2011). The double-edged sword of pedagogy: instruction limits spontaneous exploration and discovery. Cognition 120, 322–330. doi: 10.1016/j.cognition.2010.10.001
Botvinick, M. M. (2012). Hierarchical reinforcement learning and decision making. Curr. Opin. Neurobiol. 22, 956–962. doi: 10.1016/j.conb.2012.05.008
Calero, C. I., Zylberberg, A., Ais, J., Semelman, M., and Sigman, M. (2015). Young children are natural pedagogues. Cogn. Dev. 35, 65–78. doi: 10.1016/j.cogdev.2015.03.001
Carlson, S. M., Koenig, M. A., and Harms, M. B. (2013). Theory of mind. WIREs Cogn. Sci. 4, 391–402. doi: 10.1002/wcs.1232
Corriveau, K., and Harris, P. L. (2009). Choosing your informant: weighing familiarity and recent accuracy. Dev. Sci. 12, 426–437. doi: 10.1111/j.1467-7687.2008.00792.x
Csibra, G., and Gergely, G. (2009). Natural pedagogy. Trends Cogn. Sci. 13, 148–153. doi: 10.1016/j.tics.2009.01.005
Davis-Unger, A. C., and Carlson, S. M. (2008). Development of teaching skills and relations to Theory of Mind in preschoolers. J. Cogn. Dev. 9, 26–45. doi: 10.1080/15248370701836584
Dickinson, A. (1985). Actions and habits: the development of behavioural autonomy. Philos. Trans. R. Soc. Lond. B Biol. Sci. 308, 67–78. doi: 10.1098/rstb.1985.0010
Dolan, R. J., and Dayan, P. (2013). Goals and habits in the brain. Neuron 80, 312–325. doi: 10.1016/j.neuron.2013.09.007
Etkin, A., Büchel, C., and Gross, J. (2015). The neural bases of emotion regulation. Nat. Rev. Neurosci. 16, 693–700. doi: 10.1038/nrn4044
Ezzaim, A., Dahbi, A., Aqqal, A., and Haidine, A. (2024). AI-based learning style detection in adaptive learning systems: a systematic literature review. J. Comput. Educ. doi: 10.1007/s40692-024-00328-9. [Epub ahead of print].
Frank, M. C., Bergelson, E., Bergmann, C., Cristia, A., Floccia, C., Gervain, J., et al. (2017). A collaborative approach to infant research: Promoting reproducibility, best practices, and theory-building. Infancy 22, 421–435. doi: 10.1111/infa.12182
Frith, U., and Frith, C. (2010). The social brain: allowing humans to boldly go where no other species has been. Philos. Trans. R. Soc. B Biol. Sci. 365, 165–176. doi: 10.1098/rstb.2009.0160
Geraghty, K., Waxman, S. R., and Gelman, S. A. (2014). Learning words from pictures: 15-and 17-month-old infants appreciate the referential and symbolic links among words, pictures, and objects. Cogn. Dev. 32, 1–11. doi: 10.1016/j.cogdev.2014.04.003
Gergely, G., Egyed, K., and Király, I. (2007). On pedagogy. Dev. Sci. 10, 139–146. doi: 10.1111/j.1467-7687.2007.00576.x
Gershman, S. J., Gerstenberg, T., Baker, C. L., and Cushman, F. A. (2016). Plans, habits, and Theory of Mind. PLoS ONE 11:e0162246. doi: 10.1371/journal.pone.0162246
Gligorea, I., Cioca, M., Oancea, R., Gorski, A. T., Gorski, H., and Tudorache, P. (2023). Adaptive learning using artificial intelligence in e-learning: a literature review. Educ. Sci. 13:1216. doi: 10.3390/educsci13121216
Gopnik, A., and Sobel, D. M. (2000). Detecting blickets: how young children use information about novel causal powers in categorization and induction. Child Dev. 71, 1205–1222. doi: 10.1111/1467-8624.00224
Gweon, H., and Asaba, M. (2018). Order matters: children's evaluation of under-informative teachers depends on context. Child Dev. 89, e278–e292. doi: 10.1111/cdev.12825
Gweon, H., Pelton, H., Konopka, J. A., and Schulz, L. E. (2014). Sins of omission: children selectively explore when teachers are under-informative. Cognition 132, 335–341. doi: 10.1016/j.cognition.2014.04.013
Heller, D., Arnold, J. E., Klein, N. M., and Tanenhaus, M. K. (2015). Inferring difficulty: flexibility in the real-time processing of disfluency. Lang. Speech 58(Pt. 2), 190–203. doi: 10.1177/0023830914528107
Henderson, B., and Moore, S. G. (1979). Measuring exploratory behavior in young children: a factor-analytic study. Dev. Psychol. 15, 113–119. doi: 10.1037/0012-1649.15.2.113
Hofstede, G. (1986). Cultural differences in teaching and learning. Int. J. Intercult. Relat. 10, 301–320. doi: 10.1016/0147-1767(86)90015-5
Jaswal, V. K., and Neely, L. A. (2006). Adults don't always know best: preschoolers use past reliability over age when learning new words. Psychol. Sci. 17, 757–758. doi: 10.1111/j.1467-9280.2006.01778.x
Jean, A., Daubert, E. N., Yu, Y., Shafto, P., and Bonawitz, E. (2019). “Pedagogical questions empower exploration,” in Proceedings of the 41st Annual Conference of the Cognitive Science Society, eds. A. K. Goel, C. M. Seifert, and C. Freksa (Montreal, QB: Cognitive Science Society), 485–491.
Kidd, C., White, K. S., and Aslin, R. N. (2011). Toddlers use speech disfluencies to predict speakers' referential intentions. Dev. Sci. 14, 925–934. doi: 10.1111/j.1467-7687.2011.01049.x
Kim, S., Paulus, M., Sodian, B., Itakura, S., Ueno, M., Senju, A., et al. (2018). Selective learning and teaching among Japanese and German children. Dev. Psychol. 54, 536–542. doi: 10.1037/dev0000441
Knudsen, B., and Liszkowski, U. (2013). One-year-olds warn others about negative action outcomes. J. Cogn. Dev. 14, 424–436. doi: 10.1080/15248372.2012.689387
Kominsky, J. F., Gerstenberg, T., Pelz, M., Sheskin, M., Singmann, H., Schulz, L., et al. (2021). The trajectory of counterfactual simulation in development. Dev. Psychol. 57, 253–268. doi: 10.1037/dev0001140
LeVine, R. A., LeVine, S., Schnell-Anzola, B., Rowe, M., and Dexter, E. (2012). Literacy and Mothering: How Women's Schooling Changes the Lives of the World's Children. Oxford: Oxford University Press. doi: 10.1093/acprof:oso/9780195309829.001.0001
Liljeholm, M., Tricomi, E., O'Doherty, J. P., and Balleine, B. W. (2011). Neural correlates of instrumental contingency learning: differential effects of action-reward conjunction and disjunction. J. Neurosci. 31, 2474–2480. doi: 10.1523/JNEUROSCI.3354-10.2011
Loewenstein, G. (1994). The psychology of curiosity: a review and reinterpretation. Psychol. Bull. 116, 75–98. doi: 10.1037/0033-2909.116.1.75
Martin, F., Chen, Y., Moore, R. L., and Westine, C. D. (2020). Systematic review of adaptive learning research designs, context, strategies, and technologies from 2009 to 2018. Educ. Technol. Res. Dev. 68, 1903–1929. doi: 10.1007/s11423-020-09793-2
Moll, H., and Tomasello, M. (2007). Cooperation and human cognition: the Vygotskian intelligence hypothesis. Philos. Trans. R. Soc. Lond. B Biol. Sci. 362, 639–648. doi: 10.1098/rstb.2006.2000
Mousavinasab, E., Zarifsanaiey, N. R., Niakan Kalhori, S., Rakhshan, M., Keikha, L., et al. (2021). Intelligent tutoring systems: a systematic review of characteristics, applications, and evaluation methods. Interact. Learn. Environ. 29, 142–163. doi: 10.1080/10494820.2018.1558257
Nussenbaum, K., and Hartley, C. A. (2019). Reinforcement learning across development: what insights can we draw from a decade of research? Dev. Cogn. Neurosci. 40:100733. doi: 10.1016/j.dcn.2019.100733
Nyhout, A., and Ganea, P. A. (2019). Mature counterfactual reasoning in 4-and 5-year-olds. Cognition 183, 57–66. doi: 10.1016/j.cognition.2018.10.027
Pasquini, E. S., Corriveau, K. H., Koenig, M., and Harris, P. L. (2007). Preschoolers monitor the relative accuracy of informants. Dev. Psychol. 43, 1216–1226. doi: 10.1037/0012-1649.43.5.1216
Qiu, F. W., Park, J., Vite, A., Patall, E., and Moll, H. (2025). Children's selective teaching and informing: a meta-analysis. Dev. Sci. 28:e13576. doi: 10.1111/desc.13576
Rafetseder, E., Cristi-Vargas, R., and Perner, J. (2010). Counterfactual reasoning: developing a sense of “nearest possible world”. Child Dev. 81, 376–389. doi: 10.1111/j.1467-8624.2009.01401.x
Rhodes, M., Bonawitz, E., Shafto, P., Chen, A., and Caglar, L. (2015). Controlling the message: preschoolers' use of information to teach and deceive others. Front. Psychol. 6:867. doi: 10.3389/fpsyg.2015.00867
Ronfard, S., and Corriveau, K. H. (2016). Teaching and preschoolers' ability to infer knowledge from mistakes. J. Exp. Child Psychol. 150, 87–98. doi: 10.1016/j.jecp.2016.05.006
Scott, K., and Schulz, L. (2017). Lookit (part 1): a new online platform for developmental research. Open Mind 1, 4–14. doi: 10.1162/OPMI_a_00002
Shafto, P., and Goodman, N. (2008). “Teaching games: statistical sampling assumptions for learning in pedagogical situations,” in Proceedings of the 30th Annual Conference of the Cognitive Science Society, eds. B. C. Love, K. McRae, and V. M. Sloutsky (Austin, TX: Cognitive Science Society), 1632–1637.
Shafto, P., Goodman, N. D., and Frank, M. C. (2012). Learning from others: the consequences of psychological reasoning for human learning. Perspect. Psychol. Sci. 7, 341–351. doi: 10.1177/1745691612448481
Shafto, P., Goodman, N. D., and Griffiths, T. L. (2014). A rational account of pedagogical reasoning: teaching by, and learning from, examples. Cogn. Psychol. 71, 55–89. doi: 10.1016/j.cogpsych.2013.12.004
Sheskin, M., Scott, K., Mills, C. M., Bergelson, E., Bonawitz, E., Spelke, E. S., et al. (2020). Online developmental science to foster innovation, access, and impact. Trends Cogn. Sci. 24, 675–678. doi: 10.1016/j.tics.2020.06.004
Shneidman, L., Gweon, H., Schulz, L. E., and Woodward, A. L. (2016). Learning from others and spontaneous exploration: a cross-cultural investigation. Child Dev. 87, 723–735. doi: 10.1111/cdev.12502
Strauss, S., and Ziv, M. (2012). Teaching is a natural cognitive ability for humans. Mind Brain Educ. 6, 186–196. doi: 10.1111/j.1751-228X.2012.01156.x
Strauss, S., Ziv, M., and Stein, A. (2002). Teaching as a natural cognition and its relations to preschoolers' developing Theory of Mind. Cogn. Dev. 17, 1473–1487. doi: 10.1016/S0885-2014(02)00128-4
Tomasello, M. (2019). Becoming Human: A Theory of Ontogeny. Cambridge, MA: Belknap Press of Harvard University Press. doi: 10.4159/9780674988651
Ullman, T. D., and Bass, I. (2024). The detection of automatic behavior in other people. Am. Psychol. 79, 1322–1336. doi: 10.1037/amp0001440
Vredenburgh, C., Kushnir, T., and Casasola, M. (2015). Pedagogical cues encourage toddlers' transmission of recently demonstrated functions to unfamiliar adults. Dev. Sci. 18, 645–654. doi: 10.1111/desc.12233
Wellman, H. M., and Lagattuta, K. H. (2004). Theory of Mind for learning and teaching: the nature and role of explanation. Cogn. Dev. 19, 479–497. doi: 10.1016/j.cogdev.2004.09.003
Wellman, H. M., and Liu, D. (2004). Scaling of theory-of-mind tasks. Child Dev. 75, 523–541. doi: 10.1111/j.1467-8624.2004.00691.x
Ye, N. N., Cui, Y. K., Ronfard, S., and Corriveau, K. H. (2025). The development of children's teaching varies by cultural input: evidence from China and the U.S. Front. Dev. Psychol. 3:1511224. doi: 10.3389/fdpys.2025.1511224
Ye, N. N., Heyman, G. D., and Ding, X. P. (2021). Linking young children's teaching to their reasoning of mental states: evidence from Singapore. J. Exp. Child Psychol. 209:105175. doi: 10.1016/j.jecp.2021.105175
Yu, Y., Landrum, A. R., Bonawitz, E., and Shafto, P. (2018). Questioning supports effective transmission of knowledge and increased exploratory learning in pre-kindergarten children. Dev. Sci. 21:e12696. doi: 10.1111/desc.12696
Keywords: automatic behavior, pedagogical reasoning, Theory of Mind, social cognition, cognitive development, counterfactual reasoning
Citation: Bass I, Colantonio J, Aboody R, Wong M, Ullman T and Bonawitz E (2025) Children's sensitivity to automatic behavior relates to pedagogical reasoning and Theory of Mind. Front. Dev. Psychol. 3:1574528. doi: 10.3389/fdpys.2025.1574528
Received: 11 February 2025; Accepted: 02 April 2025;
Published: 24 April 2025.
Edited by:
Marina Bazhydai, Lancaster University, United KingdomReviewed by:
Cecilia Ines Calero, Torcuato di Tella University, ArgentinaSunae Kim, Oxford Brookes University, United Kingdom
Samuel Ronfard, Boston University, United States
Copyright © 2025 Bass, Colantonio, Aboody, Wong, Ullman and Bonawitz. This is an open-access article distributed under the terms of the Creative Commons Attribution License (CC BY). The use, distribution or reproduction in other forums is permitted, provided the original author(s) and the copyright owner(s) are credited and that the original publication in this journal is cited, in accordance with accepted academic practice. No use, distribution or reproduction is permitted which does not comply with these terms.
*Correspondence: Ilona Bass, aWJhc3NAZmFzLmhhcnZhcmQuZWR1