- 1Health Informatics Center, Faculty of Medicine, Universidade Federal de Minas Gerais, Belo Horizonte, Brazil
- 2Department of Obstetrics and Gynecology, Faculty of Medical Sciences, Universidade Estadual de Campinas, Campinas, Brazil
- 3Department of Pediatrics, Faculty of Medicine, Universidade Federal de Minas Gerais, Belo Horizonte, Brazil
- 4Department of Gynecology and Obstetrics, Faculty of Medicine, Universidade Federal de Minas Gerais, Belo Horizonte, Brazil
- 5Arts Faculty, Universidade Federal de Minas Gerais, Belo Horizonte, Brazil
Objective: Medication adherence involves patients correctly taking medications as prescribed. This review evaluates whether artificial intelligence (AI) based tools contribute to adherence-related insights or avoid medication intake errors.
Methods: We assessed studies employing AI tools to directly benefit patient medication use, promoting adherence or avoiding self-administration error outcomes. The search strategy was conducted on six databases in August 2024. ROB2 and ROBINS1 assessed the risk of bias.
Results: The review gathered seven eligible studies, including patients from three clinical trials and one prospective cohort. The overall risk of bias was moderate to high. Three reports drew on conceptual frameworks with simulated testing. The evidence identified was scarce considering measurable outcomes. However, based on randomized clinical trials, AI-based tools improved medication adherence ranging from 6.7% to 32.7% compared to any intervention controls and current practices, respectively. Digital intervention using video and voice interaction providing real-time monitoring pointed to AI's potential to alert to self-medication errors. Based on conceptual framework reports, we highlight the potential of cognitive behavioral approaches tailored to engage patients in their treatment.
Conclusion: Even though the present evidence is weak, smart systems using AI tools are promising in helping patients use prescribed medications. The review offers insights for future research.
Systematic review registration: https://www.crd.york.ac.uk/PROSPERO/view/CRD42024571504, identifier: CRD42024571504.
1 Introduction
The proper use of prescribed medications is crucial for disease management, affecting health outcomes and enhancing the efficiency of healthcare systems. The World Health Organization (WHO) defines adherence as the alignment of an individual's actions with healthcare professional recommendations (1). Nonadherence negatively impacts patient health and healthcare systems resulting in suboptimal outcomes, disease progression, unnecessary costs, and inappropriate treatment modifications. These consequences are particularly pronounced in resource-constrained settings, where nonadherence increases inefficiencies and contributes to higher morbidity and mortality (2).
Non-adherence to medication doses has been found in 50% of patients, with dramatic consequences on their management of chronic conditions (3). Poor medication adherence has also been reported in half of patients due to failure to take their medications as prescribed (4). This involves both underdosing and overdosing. Insufficient dosage can reduce therapeutic benefits, while overdosing—such as compensating for missed doses—may lead to adverse effects (5). Inconsistent adherence can result in a myriad of consequences, including increased adverse events, unnecessary hospitalizations, antimicrobial resistance, higher costs, disease progression, and treatment failure or death (4, 6). Medication errors remain a major concern for health systems because they threaten patient safety, particularly affecting people with low health literacy, the elderly, and those with mental health disorders, all of whom are especially vulnerable (7, 8).
There is increasing recognition that aspects beyond patients' control can affect medication adherence (9). Healthcare providers' communication and behavior significantly influence medication use, with common breakdowns including inadequate explanations of medication instructions, inadequate approaches to patients' reluctance to use medications and patients' beliefs regarding health and treatment (5). Given these issues, effective communication between prescribers and patients is critical. Adherence interventions should consider not only patients but also the broader healthcare context. Recent research highlights the importance of interventions that incorporate structural and counseling components and include appropriately qualified and motivated health professionals to promote medication adherence and persistence (5).
Electronic prescribing systems have largely gained preference in many countries, contributing to improve the accuracy and efficiency of medication management and eliminate problems with readability and access to prescriptions (10). While legibility, growing number of drugs available in the market, and drug interaction alerts are already targeted by digital technology, other challenges still demand smarter systems such as the need for personalized treatment and directions adapted to patients' literacy. To meet these challenges, electronic systems are integrated with other organizational tools, such as electronic health records and pharmacy information systems (11). The benefits include reduced healthcare costs, minimized prescribing errors, improved medication outcomes, increased patient safety, and enhanced clinical decision-making.
Recent advances in Artificial Intelligence (AI) have created new opportunities for addressing long-standing challenges in healthcare. AI refers to the use of computerized systems to model intelligent behavior with minimal human intervention (12). Retrospective studies using machine learning (ML) to predict medication adherence have gained ground (13, 14). However, AI algorithms in applications ranging from early detection and diagnosis to the management and treatment of medical conditions as well as the improvement of patient engagement still need to be tested (15). The integration of AI into prescribing systems offers significant opportunities as well as notable challenges, particularly in optimizing medication management, providing real-time prediction on patient adherence patterns based on clinical data, personalizing treatment regimens, and minimizing the risk of adverse drug interactions (16, 17).
The objective of this article was to review evidence on AI-based tools that contribute to adherence improvement, adherence measurement or avoidance of medication intake errors, helping patients take their medications safely and correctly, thereby improving medication adherence compared to standard practices.
2 Methods
We conducted a focused review following the Preferred Reporting Items for Systematic Review and Meta-Analyses (PRISMA) statement (18). The present review was registered on PROSPERO under the registration number CRD42024571504 (Available at: https://www.crd.york.ac.uk/prospero/display_record.php?ID=CRD42024571504). A research question was formulated and refined using the PI(E)COS framework: Can artificial intelligence approaches enhance prescription directions to help patients safely and correctly take medications, thereby improving medication adherence compared to standard practices?
2.1 Search strategy
The search strategy was formulated by a librarian and information science professional on July 20, 2024, in collaboration with the research group. The development of the strategy was unrestricted, provided the publication had an abstract in English. The databases searched included MEDLINE/Pubmed, Cochrane Library, Embase, SCOPUS, Web of Science, and Lilacs using descriptors and filters specific to each database. The following key terms and their variations were searched: (Patient OR Clients) AND (“Computer Reasoning” OR “Artificial Intelligence” OR “Machine Intelligence” OR “Computational Intelligence” OR “Transfer Learning” OR “Learning, Transfer” OR “Natural Language Processing” OR “Machine Learning”) AND (Prescription OR Prescriptions OR “Electronic Prescribing”) AND (“Medication Adherence” OR “Drug Adherence” OR “Medication Non-Adherence” OR “Drug Compliance”). The full search strategy is outlined in the Supplementary Information.
2.2 Eligibility criteria
The present review intends to summarize evidence regarding new generations of systems with AI-based adherence prediction models as long as they contribute to understanding or improving medication adherence outcomes or to mitigate outpatients' self-medication intake errors and improve medication adherence. Studies investigating patients prescribed medication by an authorized professional for self-administration; approaches based on AI tools and medication adherence or self-administration errors were included. In instances of duplicate publications or secondary analyses of included studies, the publication with the longest follow-up period or the most comprehensive information was selected. There were no restrictions on the types of study design eligible for inclusion.
The exclusion criteria were as follows: studies on AI tools targeting prevention of prescribers' errors such as alerts on drug interactions or polypharmacy, or support to prescribers in determining dosage, administration route, and frequency of use; studies focused solely on digital reminders to remind patients of their medication schedules without AI-based decision-making; and inaccessible full publication or without an abstract in English.
2.3 Outcomes
To address the research question, we define the outcomes as follows:
• The primary outcome is AI tool's advantages in improving medication adherence alone or compared to standard practices. The metrics to assess medication adherence were quantitative or qualitative, as reported in the primary studies.
• The secondary outcome is the comparison of medication self-administration errors between the intervention using AI tools and standard practices. The metrics to assess the outcome were the occurrence of self-medication errors using quantitative or qualitative results, as reported in the primary studies.
The medical term self-medication error refers to medication taken at the wrong time or dose, confused with other medications, or wrongly stored.
2.4 Selection of studies and extraction of variables
All articles retrieved from the electronic databases were organized using StaRt® (State of the Art through Systematic Review) software. After removing duplicated studies, the titles and abstracts were independently screened by two researchers (E.M.L. and C.S.D) according to the inclusion and exclusion criteria. References of review studies related to the topic were manually screened. The articles selected for full-text reading were subsequently independently evaluated by two researchers. A third researcher (Z.S.N.R) was considered in case of any disagreement between the researchers. When additional information was needed or questions arose about the data from selected articles, the authors were contacted. If no response was received by the submission deadline for the systematic review, the researchers reserved the right to exclude the paper and data.
Data from the studies included for quantitative and qualitative analyses were independently extracted by pairs of researchers (E.M.L. and C.S.D, Z.S.N.R and F.R.O) into the StaRt® software and organized into a database. The data were subsequently checked by a third researcher (Z.S.N.R). The extracted data referred to: the author and year of publication, country of origin of the study, main objectives, study design, population characteristics, type of systems used, metrics related to medication adherence, and indicators of self-medication errors, AI tools advantages. A complete list of the extracted variables is available in the Supplementary Information. All researchers underwent standardized training in screening and data extraction processes to ensure consistency in the review process. Given the heterogeneity in study designs and results, a meta-analysis was deemed infeasible. Therefore, the findings were summarized in a qualitative synthesis.
2.5 Evaluation of study quality
Two independent investigators (G.M.V.P. and F.R.O.) conducted the quality analysis of both randomized and non-randomized studies. Randomized studies were assessed using the Cochrane Handbook for Systematic Reviews of Interventions (19), classifying the risk of bias as “low”, “high”, or “some concerns” in the domains of bias arising from the randomization process; bias due to deviations from intended interventions; bias due to missing outcome data; bias in the measurement of the outcome; bias in the selection of the reported result. We used the Revised Cochrane Risk of Bias Tool (ROB2) (20). Non-randomized studies were evaluated using the Risk Of Bias In Non-randomized Studies of Interventions (ROBINS-I) (21), a tool that organizes and presents evidence related to bias across seven domains: confounding, selection of participants, classification of interventions, deviations from intended interventions, missing data, measurement of outcomes, and reporting of results.
Additionally, we applied the minimum report recommended by ESPACOMP Medication Adherence Reporting Guidelines framework (22), which provides structured recommendations for reporting adherence-related research, as Supplementary Table S4.
3 Results
3.1 Study selection
The search strategy retrieved 159 reports and six were identified by citation search. Upon cross-checking seven databases, 74 duplicates were removed (Figure 1). Only the Cochrane Library search yielded no results. Screening by title and abstract, all four reviewers selected 28 reports for complete reading. We identified six reports using a citation search; however, only three reports met eligibility criteria after full reading. During this step, we contacted the authors of two reports to clarify and ask questions regarding eligibility criteria and data extraction. We received a reply from only one of the reports' authors, and their clarification led to the exclusion of the report for not meeting the eligibility criteria. The second report was also excluded due to the lack of response from the author. At the end of the process, seven reports met the inclusion criteria. Studies without a focus on patient support to safely and correctly take medication were the main causes for exclusion. Detailed excluded studies, with reasons, are in Supplementary Tables S1, S2.
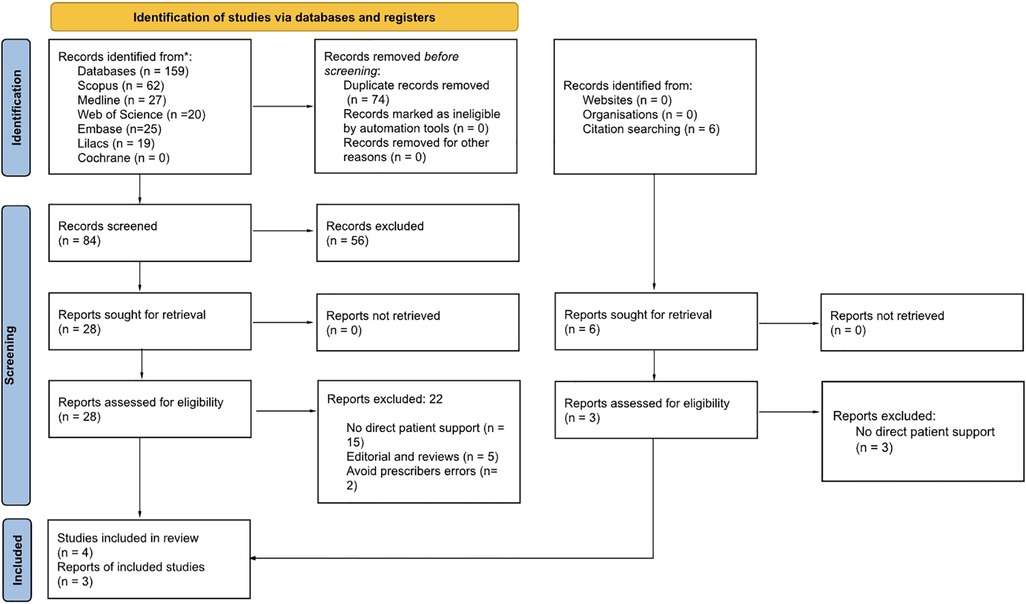
Figure 1. Flowchart of the review process. Adapted with permission from PRISMA 2020 flow diagram template for systematic reviews by Page et al., licensed under CC BY 4.0.
3.2 Study characteristics
Most studies included in this review were conducted in the United States, representing 5 out of 7 studies (71.5%), as shown in Table 1. Four studies involved real-world scenarios, including three clinical trials (23–25) and one prospective observational study (26). Collectively, these studies analyzed data from a total of 37,633 patients. Notably, the AiCure software platform was tested on 33,344 patients in a study by Gracey B, et al. (25), with an additional 75 patients in the study by Bain EE, et al. (23), both supporting private-sector healthcare applications. The majority of the selected studies focused on adult patients receiving medication for chronic disease management.
The clinical trials provided important comparisons between AI-based interventions and conventional treatment approaches (23, 24), while one of them, additionally, included a comparison with a group that did not receive any intervention (24).
In contrast, studies from Brazil (27), India (28) and one from the United States (29) were limited to simulation-based approaches, without testing in real-world settings.
3.3 Results of syntheses
Studies reporting results of AI tools assisting patients in correctly taking their medication made use of various technologies, as summarized in Table 2. Characteristics of AI-based tools are detailed in Supplementary Table S3. These included mobile applications (23, 26), smart call centers with live call interventions (25), and conversational AI platforms like Amazon Alexa® (24).
Studies exploring solutions in simulated scenarios made use of an intelligent system with wireless integration with home devices systems (27), a user-friendly IoT-based system with cloud computing, ML wireless integration systems with smart pill bottles, sensors, and cameras (28), and predictive ML framework of models to trigger real-time actions alerted by intervention, such as SMS, voice calls, and interaction by mobile applications (29).
A common requirement across the selected studies was targeting the need for timely interventions. To achieve this, data input often began with real-time patient monitoring, utilizing technologies like an AI Platform accessible on mobile devices for patient identification and medication ingestion confirmation using ML algorithms (23, 26, 27). In other cases, voice interaction played a crucial role, particularly in voice-based conversational AI application (24), and interventions using AI in AllazoEngine-powered call center (25). These systems often integrated alerts that provided feedback to patients, caregivers, doctors, or call centers, particularly in cases of suspicious behavior, such as incorrect medication use.
Framework-based reports explored further possibilities of intelligent systems for patient monitoring using IoT-integrated sensors and smart devices to track medication adherence (27). Systems incorporating smart pill bottles, weight sensors, and camera modules for tracking pill consumption, pill count, and pill recognition were found to be potentially valuable for generating data. These data are meant to be prospectively used by ML algorithms, such as the YOLOv4 ML pre-trained model, for pill detection and recognition (28).
Clinical trial outcomes demonstrated a promising impact of systems using AI tools on patient medication adherence, with different studies employing various metrics to measure success. In the randomized clinical trial by Gracey B. et al. (25), the AI group was found to be 6.1% more likely to adhere to their treatment compared to the control group (p = 0.04). In a prospective cohort study, Koesmahargyo et al. (26) reported that the accuracy of the XGBoost classifier algorithm in predicting patient adherence improved over time, utilizing both real-time and historical adherence data based on AI Cure mobile application. Using the same proprietary mobile application, Bain EE et al. reported cumulative pharmacokinetic adherence over 24 weeks of 89.7% (SD 24.92) in the group using AI Platform on mobile device interventions, compared to 71.9% (SD 39.81) in the control group (23). Although this study did not provide formal statistical comparisons, both systems demonstrated the ability to deliver real-time patient monitoring, enabling timely detection of medication misuse. Another clinical trial, using a voice-based conversational AI application to support type 2 diabetes patients, conducted by Nayak A. et al. (24), showed that insulin adherence rates were 32.7% higher in the AI-voice application compared to the standard care group (95% Confidence Interval from 8.0% to 57.4%, p = 0.01). Furthermore, we extracted information from selected studies that reveal the potential scalability of AI tool solutions identified in this review, particularly in reducing the need for clinical visits and enabling healthcare providers to focus on high-risk patients prone to non-adherence. Table 2 presents the advantages of AI tools from our perspective analysis.
Studies without real-world care scenario testing proposed intelligent systems using ML algorithms and wireless networks with the potential to assist patients in avoiding self-medication errors (27, 28). One study focused on processing data to classify adherence and optimize treatment outcomes (29).
3.4 Risk of bias in studies
Figure 2 illustrates the evaluation of bias risk utilizing the Revised Cochrane Risk of Bias Tool (ROB2) (20), and the Risk of Bias in Non-randomized Studies of Interventions (ROBINS-1) (21), as delineated in the Cochrane Handbook for Systematic Reviews of Interventions (19).
The randomization process presented some concerns in two studies and was classified as high-risk in one. Deviation from the intended intervention was assessed as high risk in one study, low risk in another, and raised some concerns in a third study. The risk associated with missing outcome data was deemed low in two studies and presented some concerns in one study. The measurement of outcomes was classified as high risk in one study (24) and moderate in two others (23, 25). Lastly, the selection of reported results was evaluated as moderate across all three studies. Considering the ROBINS-I assessment, we classified one study (26) as an overall moderate risk of bias.
Adherence measurement quality according to the Emerge checklist (22) was presented in Supplementary Table S4.
4 Discussion
4.1 Main findings
Evidence regarding AI tool interventions directly benefiting patient medication adherence or reducing self-medication errors is still limited. The lack of measurable outcomes based on well-controlled studies and moderate to low quality of evidence emerged as a relevant issue in this focused review. We may account for this scarcity of studies due to the relatively recent introduction of AI tools in real healthcare settings (30) and the inherent complexity of implementing innovations that influence patient behavior (31). However, our protocol's comprehensive search strategy, which included six databases without restrictions on language or publication year, provided that the title and abstract were in English, ensured a broad and inclusive scope. This way, we alert the need for further studies demonstrating an effective use of AI tools for this proposal.
To discuss our results, we considered real-world scenario studies and studies based on simulations separately. Regarding real-world scenario studies, all four studies conducted in real healthcare environments were within the private sector in the USA, using prospective designs. Digital companies have historically led the AI tools introduction in healthcare (15, 32). Despite a large investment from technology companies, experts, and researchers, the true impact of AI algorithms on improving people's health remains difficult to measure. One of the challenges in evaluating smart systems performance is designing studies that ethically determine the usefulness of intervention with real-time judgments, preferably using a blinded and randomized approach compared with current practices (15).
The randomized clinical trials, which we classified as moderate risk of bias, compared groups of standard care, and control without interventions with users of smart systems using AI tools. These trials demonstrated the potential of ML models to timely identify patients at high risk of non-adherence and deliver opportune alert interventions through interactions via call centers (25) or custom voice AI generator (24). It is important to recognize that AI tools were not the sole driver of positive outcomes in these trials; rather, it was a component of broader patient surveillance strategies. For instance, in Gracey B, et al. report, although the difference between the AI tools intervention was 6.1% more likely to be adherent compared to the control group without any intervention (p = 0.04), the AI tools intervention had no significant effect when compared to traditional live-call monitoring (p = 0.08) (25). This implies that while AI can enhance adherence, its impact may be modest and often works in conjunction with other aspects of patient care such as surveillance using call centers itself.
The outcomes from Bain EE, et al. (23) had limited value since it included only a few patients and no comparative statistical test between the AI Platform on mobile devices intervention and the control group. Furthermore, the distribution of patients between intervention and control groups was not randomized, leading to a classification as high risk of bias. Despite this, the study reported a value of 35.8% detection of suspicious incorrect drug administration behavior flagged by the AI platform, in the intervention group. Even so, progress is expected in intelligent systems with AI in this area of healthcare, as they can improve accuracy over time, as presented by Koesmahargyo et al. (26) real-time ML algorithms predicting adherence among a mix of primary conditions. As a limitation, we noticed such progress had no confidence intervals or statistical comparisons for the model's accuracy by Area Under Curve (AUC), and the risk of bias risk was considered moderate.
Regarding bias risk in studies based on conceptual frameworks, the quality of evidence was not evaluated due to the absence of real scenario outcomes and intervention vs. control comparisons. It should be noted that following our review protocol (PROSPERO 2024 CRD42024571504), which included overall study designs, we decided to include studies on conceptual frameworks, as long as they met our inclusion criteria. Even though these studies are based on simulation, their outcomes were useful in pointing to the relevance of personalizing the interaction with each patient, considering patient beliefs and attitudes (29), using remote, real-time measurement of medication intake with IoT-based systems and ML algorithms, and allowing for proactive clinical intervention to optimize health outcomes (27, 28). The AI synergy with the Internet of Things (IoT) promises to enhance the next generation of smart systems in healthcare providing real-time surveillance (33).
Studies involving retrospective analysis of databases to predict medication adherence were excluded (Supplementary Tables S1, S2). Despite their potential for further providing smart interventions, our interest was to look for AI systems that can interact in a personalized way with patients, helping them with drug treatment and measuring adherence or avoiding self-administration errors. In this review, we opted for a broad search strategy combined with well-defined eligibility criteria to ensure the inclusion of a diverse range of AI-based interventions, expecting that AI-based approaches would leverage features that characterize patients, their conditions, and their relationship with prescribed medications providing solutions to the targeted strategies.
4.2 Limitations of evidence
We encountered different methods for measuring medication adherence, which was a challenge to synthesize the data extracted in this review and to interpret the results. While only seven studies met the inclusion criteria, this reflects the current state of evidence. The heterogeneity in the included studies evolving different applications based on AI tools reinforces the need for more standardized and well-controlled research. Although there is a report of a psychometric scale to measure treatment adherence, this has not been used since 2019 and was not used in any of the selected studies (34). The expected synthesis using the meta-analysis included in our review protocol was not possible. Medication nonadherence is a multifactorial issue influenced by patients' ability to remember their medications, the clarity of instructions, and the complexity of treatment regimens (5). These factors often contribute to unintentional nonadherence, also referred to as passive nonadherence, which stems from forgetfulness or carelessness. This relevant nonadherence perspective was not a concern in most of the selected studies.
Additionally, patients' beliefs about medications, and their perceived view of medication necessity and affordability, also play a role (35). Moreover, intentional nonadherence is increasingly acknowledged as a significant factor, with its determinants being complex and including cost, adverse effects, patient preferences, disagreement on treatment necessity, and communication breakdowns between patients and providers (36). In this review, the study by Julius et al. extended the analysis of adherence beyond the simple act of taking or not taking medication, exploring the integration of non-clinical data, such as patients' beliefs, attitudes, knowledge, and perceptions, into a conceptual model (29). Their study highlighted the potential of smart systems to personalize interventions, delivering functions inspired by health belief theory to address individual patient needs.
Another important issue raised in our review was the diversity of clinical conditions covered by the studies, limiting generalization based on outcomes. It is essential to recognize that different disease groups present unique challenges to achieving patient adherence. For example, systems designed to support patients with schizophrenia (23) focused on addressing cognitive impairment associated with the condition. In contrast, the voice-based conversational AI application developed by Nayak et al. was tailored to help patients with type 2 diabetes titrate basal insulin at home, aiming to achieve rapid glycemic control (24). Furthermore, it is important to consider that the number of patients involved in the studies varied from 32 to 33,344 exposed to AI-based tools.
Based on the EMERGE framework (22), we found substantial variability in how adherence was defined, and measured. We reported across studies, which has important implications for the interpretation of our review findings (Supplementary Table S4). Studies in the phase of implementation directly measured adherence behavior through pharmacy claims data (25), AI-based video monitoring (23, 26), and self-reported adherence logs (23). In contrast, others relied on predictive models to provide early intervention for high-risk patients of non-adherence (29) or IoT-based adherence tracking still in prototyping (27, 28), which were not tested in real-world patient populations. These inconsistencies were limiting factors to allow comparability between studies and introduced heterogeneity into our findings. At the same time, this analysis was important to recommend that future studies ensure more transparency in the way they report medication adherence.
5 Conclusion
Based on this review, the evidence supporting AI tools to assist patients in adhering to prescribed medications is still weak. Part of the outcomes are influenced not solely by systems using AI-based tools but also by their integration into smart elements within already effective monitoring practices. Nonetheless, this review highlights AI's growing influence in healthcare and offers insights for future research. It underscores the current applications of AI in medication adherence while identifying key areas for further exploration and development.
Data availability statement
The datasets presented in this study can be found in online repositories. The names of the repository/repositories and accession number(s) can be found in the article/Supplementary Material.
Author contributions
ZR: Conceptualization, Formal analysis, Funding acquisition, Investigation, Methodology, Project administration, Supervision, Writing – original draft. GP: Conceptualization, Data curation, Methodology, Validation, Writing – review & editing. CD: Data curation, Formal analysis, Methodology, Validation, Writing – review & editing. EL: Data curation, Formal analysis, Validation, Writing – original draft. IO: Data curation, Formal analysis, Methodology, Validation, Writing – review & editing. AP: Conceptualization, Data curation, Supervision, Validation, Writing – original draft, Writing – review & editing.
Funding
The author(s) declare that financial support was received for the research and/or publication of this article. This work was supported by the Brazilian Ministry of Health/DECIT/CNPq (00758/2024-5) in partnership with the Gates Foundation [Grant Number INV-009289]. Under the grant conditions of the Foundation.
Acknowledgments
Our thanks to Marina Nogueira Ferraz, librarian and information science professional for assisting with the search strategy.
Conflict of interest
The authors declare that they have no known competing financial or commercial interests or personal relationships that could have appeared to influence the work reported in this paper.
Generative AI statement
The author(s) declare that no Generative AI was used in the creation of this manuscript.
Publisher's note
All claims expressed in this article are solely those of the authors and do not necessarily represent those of their affiliated organizations, or those of the publisher, the editors and the reviewers. Any product that may be evaluated in this article, or claim that may be made by its manufacturer, is not guaranteed or endorsed by the publisher.
Supplementary material
The Supplementary Material for this article can be found online at: https://www.frontiersin.org/articles/10.3389/fdgth.2025.1523070/full#supplementary-material
References
1. Sabaté E. Adherence to Long-Term Therapies: Evidence for Action. Geneva: World health organization (2003).
2. Al Qasem A, Smith F, Clifford S. Adherence to medication among chronic patients in middle eastern countries: review of studies. East Mediterr Health J. (2011) 17(4):356–63.22259896
3. Brown MT, Bussell JK. Medication adherence: WHO cares? Mayo Clin Proc. (2011) 86(4):304–14. doi: 10.4065/mcp.2010.0575
4. Haynes RB, Ackloo E, Sahota N, McDonald HP, Yao X. Interventions for enhancing medication adherence. Cochrane Database Syst Rev. (2008) (2):CD000011. doi: 10.1002/14651858.CD000011.pub3. Update in: Cochrane Database Syst Rev. (2014) (11):CD000011. doi: 10.1002/14651858.CD000011.pub418425859
5. Ryan RE, Santesso N, Lowe D, Hill S, Grimshaw JM, Prictor M, et al. Interventions to improve safe and effective medicines use by consumers: an overview of systematic reviews. Cochrane Database Syst Rev. (2014) 2014(4):CD007768. doi: 10.1002/14651858.CD007768.pub3
6. Viswanathan M, Golin CE, Jones CD, Ashok M, Blalock SJ, Wines RCM, et al. Interventions to improve adherence to self-administered medications for chronic diseases in the United States: a systematic review. Ann Intern Med. (2012) 157(11):785. doi: 10.7326/0003-4819-157-11-201212040-00538
7. Mira JJ, Lorenzo S, Guilabert M, Navarro I, Pérez-Jover V. A systematic review of patient medication error on self-administering medication at home. Expert Opin Drug Saf. (2015) 14(6):815–38. doi: 10.1517/14740338.2015.1026326
8. Kim M, Suh D, Barone JA, Jung SY, Wu W, Suh DC. Health literacy level and comprehension of prescription and nonprescription drug information. Int J Environ Res Public Health. (2022) 19(11):6665. doi: 10.3390/ijerph19116665
9. O’Connor PJ. Improving medication adherence: challenges for physicians, payers, and policy makers. Arch Intern Med. (2006) 166(17):1802. doi: 10.1001/archinte.166.17.1802
10. Wrzosek N, Zimmermann A, Balwicki Ł. A survey of Patients’ opinions and preferences on the use of E-prescriptions in Poland. Int J Environ Res Public Health. (2021) 18(18):9769. doi: 10.3390/ijerph18189769
11. Bouraghi H, Imani B, Saeedi A, Mohammadpour A, Saeedi S, Khodaveisi T, et al. Challenges and advantages of electronic prescribing system: a survey study and thematic analysis. BMC Health Serv Res. (2024) 24(1):689. doi: 10.1186/s12913-024-11144-3
12. Hamet P, Tremblay J. Artificial intelligence in medicine. Metab Clin Exp. (2017) 69:S36–40. doi: 10.1016/j.metabol.2017.01.011
13. Warren D, Marashi A, Siddiqui A, Eijaz AA, Pradhan P, Lim D, et al. Using machine learning to study the effect of medication adherence in opioid use disorder. PLoS One. (2022) 17(12):e0278988. doi: 10.1371/journal.pone.0278988
14. Gu Y, Zalkikar A, Liu M, Kelly L, Hall A, Daly K, et al. Predicting medication adherence using ensemble learning and deep learning models with large scale healthcare data. Sci Rep. (2021) 11(1):18961. doi: 10.1038/s41598-021-98387-w
15. Doyen S, Dadario NB. 12 plagues of AI in healthcare: a practical guide to current issues with using machine learning in a medical context. Front Digit Health. (2022) 4:765406. doi: 10.3389/fdgth.2022.765406
16. Babel A, Taneja R, Mondello Malvestiti F, Monaco A, Donde S. Artificial intelligence solutions to increase medication adherence in patients with non-communicable diseases. Front Digit Health. (2021) 3:669869. doi: 10.3389/fdgth.2021.669869
17. Reis ZSN, Pagano AS, De Oliveira IJR, Dias CDS, Lage EM, Mineiro EF, et al. Evaluating LLM-supported instructions for medication use: first steps towards a comprehensive model. Mayo Clin Proc Digit Health. (2024) 2(4):632–44. doi: 10.1016/j.mcpdig.2024.09.006
18. Page MJ, McKenzie JE, Bossuyt PM, Boutron I, Hoffmann TC, Mulrow CD, et al. The PRISMA 2020 statement: an updated guideline for reporting systematic reviews. BMJ. (2021) 372:n71. doi: 10.1136/bmj.n71
19. Higgins JPT, Green S. Cochrane Handbook for Systematic Reviews of Interventions: Cochrane Book Series. 1st ed. Wiley (2008). doi: 10.1002/9780470712184
20. Sterne JAC, Savović J, Page MJ, Elbers RG, Blencowe NS, Boutron I, et al. Rob 2: a revised tool for assessing risk of bias in randomised trials. BMJ. (2019) 366:l4898. doi: 10.1136/bmj.l4898
21. Sterne JA, Hernán MA, Reeves BC, Savović J, Berkman ND, Viswanathan M, et al. ROBINS-I: a tool for assessing risk of bias in non-randomised studies of interventions. BMJ. (2016) 355:i4919. doi: 10.1136/bmj.i4919
22. De Geest S, Zullig LL, Dunbar-Jacob J, Helmy R, Hughes DA, Wilson IB, et al. ESPACOMP medication adherence reporting guideline (EMERGE). Ann Intern Med. (2018) 169:30–5. doi: 10.7326/M18-0543
23. Bain EE, Shafner L, Walling DP, Othman AA, Chuang-Stein C, Hinkle J, et al. Use of a novel artificial intelligence platform on mobile devices to assess dosing compliance in a phase 2 clinical trial in subjects with schizophrenia. JMIR MHealth UHealth. (2017) 5(2):e18. doi: 10.2196/mhealth.7030
24. Nayak A, Vakili S, Nayak K, Nikolov M, Chiu M, Sosseinheimer P, et al. Use of voice-based conversational artificial intelligence for basal insulin prescription management among patients with type 2 diabetes: a randomized clinical trial. JAMA Netw Open. (2023) 6(12):e2340232. doi: 10.1001/jamanetworkopen.2023.40232
25. Gracey B, Jones CA, Cho D, Conner S, Greene E. Improving medication adherence by better targeting interventions using artificial intelligence-a randomized control study. Value Health. (2018) 21:S76. doi: 10.1016/j.jval.2018.04.532
26. Koesmahargyo V, Abbas A, Zhang L, Guan L, Feng S, Yadav V, et al. Accuracy of machine learning-based prediction of medication adherence in clinical research. Psychiatry Res. (2020) 294:113558. doi: 10.1016/j.psychres.2020.113558
27. João Da Silva V, Da Silva Souza V, Guimarães Da Cruz R, Mesquita Vidal Martínez De Lucena J, Jazdi N, Ferreira De Lucena Junior V. Commercial devices-based system designed to improve the treatment adherence of hypertensive patients. Sensors. (2019) 19(20):4539. doi: 10.3390/s19204539
28. Aparna R, Aravinda Kashyap KS, Shreyas KR, Sowmya RB, Rao JE, Suman M. Medisync: an IoT and ML-powered medication adherence solution. 2023 7th International Conference on Computation System and Information Technology for Sustainable Solutions (CSITSS). Bangalore, India: IEEE (2023). p. 1–6. Available at: https://ieeexplore.ieee.org/document/10334070/
29. Julius MS, Alo UR, Onu FU, Akobundu CI. Machine learning framework to predict patient non-adherence to medication using non-clinical data: a prognosis approach. Proceedings of the 9th International Conference on Computer and Communications Management. Singapore Singapore: ACM (2021). p. 98–103. Available at: https://dl.acm.org/doi/10.1145/3479162.3479177
30. Yu KH, Beam AL, Kohane IS. Artificial intelligence in healthcare. Nat Biomed Eng. (2018) 2(10):719–31. doi: 10.1038/s41551-018-0305-z
31. World Health Organization. Regulatory Considerations on Artificial Intelligence for Health. Geneva: World Health Organization (2023).
32. Rachlin H. Making IBM’s computer, Watson, human. Behav Anal. (2012) 35(1):1–16. doi: 10.1007/BF03392260
33. Bhatt V, Chakraborty S. Real-time healthcare monitoring using smart systems: a step towards healthcare service orchestration smart systems for futuristic healthcare. 2021 International Conference on Artificial Intelligence and Smart Systems (ICAIS). Coimbatore, India: IEEE (2021). p. 772–7. Available at: https://ieeexplore.ieee.org/document/9396029/
34. Chan AHY, Horne R, Hankins M, Chisari C. The medication adherence report scale: a measurement tool for eliciting patients’ reports of nonadherence. Br J Clin Pharmacol. (2020) 86(7):1281–8. doi: 10.1111/bcp.14193
35. Gadkari AS, McHorney CA. Unintentional non-adherence to chronic prescription medications: how unintentional is it really? BMC Health Serv Res. (2012) 12(1):98. doi: 10.1186/1472-6963-12-98
Keywords: prescriptions, machine learning, artificial intelligence, directive counseling, medication adherence
Citation: Reis ZSN, Pereira GMV, Dias CdS, Lage EM, de Oliveira IJR and Pagano AS (2025) Artificial intelligence-based tools for patient support to enhance medication adherence: a focused review. Front. Digit. Health 7:1523070. doi: 10.3389/fdgth.2025.1523070
Received: 5 November 2024; Accepted: 9 April 2025;
Published: 29 April 2025.
Edited by:
Sean Lawley, The University of Utah, United StatesReviewed by:
Teresa Gibson, Rochester Institute of Technology (RIT), United StatesRuth Jeminiwa, Thomas Jefferson University, United States
Copyright: © 2025 Reis, Pereira, Dias, Lage, de Oliveira and Pagano. This is an open-access article distributed under the terms of the Creative Commons Attribution License (CC BY). The use, distribution or reproduction in other forums is permitted, provided the original author(s) and the copyright owner(s) are credited and that the original publication in this journal is cited, in accordance with accepted academic practice. No use, distribution or reproduction is permitted which does not comply with these terms.
*Correspondence: Zilma Silveira Nogueira Reis, emlsbWFAdWZtZy5icg==