- 1Department of Clinical Research and Epidemiology, Comprehensive Heart Failure Center, University Hospital Würzburg, Würzburg, Germany
- 2Department of Internal Medicine I, University Hospital Würzburg, Würzburg, Germany
- 3Department of Oto-Rhino-Laryngology, Head and Neck Surgery, University of Würzburg, Würzburg, Germany
- 4Institute of Clinical Epidemiology and Biometry, University of Würzburg, Würzburg, Germany
- 5Cosinuss GmBH, München, Germany
- 6ZANA Technologies GmbH, Karlsruhe, Germany
Acute heart failure (AHF) is a life-threatening condition and a common cause of hospitalization. The defining clinical feature of AHF is volume overload, leading to pulmonary and peripheral edema and consequently to weight gain. Vocal biomarkers have the potential to facilitate the early detection of worsening HF and the prevention of AHF episodes by offering a non-invasive, low-barrier monitoring tool. The AHF-Voice study is a prospective monocentric cohort study designed to investigate the trajectories of voice alterations during and after episodes of AHF, identify potential vocal biomarkers, and enhance the understanding of the pathophysiological mechanisms underlying these voice changes. It will examine the characteristics and determinants of vocal biomarkers, analyzing their correlations with patients' clinical status and comparing them to alternative clinical parameters in HF. Further, it aims to determine whether specific vocal biomarkers can accurately map different HF phenotypes and assess their association with patient trajectories. The study phenotypes patients hospitalized for AHF at admission and discharge, and follows them for a period of 6 months. During hospitalization, daily voice recordings are collected using a specially-designed smartphone app. Following discharge, patients are requested to continue daily voice recordings with their own smartphone for the subsequent six months the 6-month follow-up. Patient-reported outcome measures and body composition are assessed in the hospital and at follow-up visits. Sub-studies explore vocal fold oscillation through video-laryngostroboscopy and assess the feasibility of combining voice analysis with in-ear sensor technology for comprehensive digital phenotyping. A total of 131 patients were enrolled between April 2023 and November 2024: their mean age was 75 years (SD 10), 31% were women, 86% were in NYHA functional class III or IV, and 38% presented with de novo heart failure. Additionally, 59% of participants owned smartphones. The AHF-Voice study will provide insights into the potential of vocal biomarkers as reliable indicators of congestion, paving the way for innovative and accessible tools to support heart failure management.
Introduction
Rationale
Heart failure (HF) affects over 64 million individuals globally, with symptoms like breathlessness, fatigue, and edema (1). In Germany, acute decompensated HF is the most common reason for hospitalization (2). The in-hospital mortality of patients with acute HF (AHF) ranges between 4% and 10%, and mortality within the first year after discharge between 20% and 30% (3). Each hospitalization due to decompensated HF increases the risk of subsequent events (4). It is therefore imperative that incipient decompensation is promptly identified to prevent hospitalization (3, 5).
Patients with worsening HF usually experience a gradually increasing volume overload, accompanied by body weight gain and symptoms like dyspnea, peripheral edema and fatigue. Without adequate treatment, the patient will eventually require hospitalization due to further deterioration. The international HF guidelines recommend that patients assess their body weight daily as a primary non-invasive self-monitoring measure (3). In addition, non-invasive and invasive remote patient management (RPM) including hemodynamic monitoring of pulmonary artery pressure or self-monitoring weight measurement, has demonstrated beneficial effects (6–8). However, RPM require either invasive procedures, and/or the acquisition of additional hardware. There is a paucity of non-invasive alternatives for self-monitoring and reliable identification of incipient decompensation.
In recent years, advancements in digital technologies including artificial intelligence have facilitated the utilization of the human voice as a vocal biomarker for the diagnosis and management of diseases (9). Previous studies have reported the use of vocal biomarkers in neurodegenerative, cardiovascular and respiratory diseases (10–12). In the case of HF, Murton et al. showed that weight loss following diuretic therapy in patients hospitalized for AHF was associated with changes in specific voice features (13). Maor et al. demonstrated that vocal biomarkers can be generated from unstructured telephone-based recordings by crosslinking selected voice features with clinical outcomes. In their study, vocal biomarkers were associated with an increased risk of hospitalization and mortality in patients with congestive HF (14). The use of smartphone-based voice recordings and the automated generation of clinically meaningful vocal biomarkers reflective of changes in clinical status in patients with HF has been demonstrated by Amir et al. (15, 16). However, the evidence for vocal biomarkers in patients with HF is scarce and heterogeneous, with most studies encompassing small sample sizes and are limited to remote data collection after discharge.
Furthermore, the underlying pathophysiological mechanisms of voice alterations in patients with HF remain poorly understood. Several hypotheses are being discussed. (1) Murton et al. suggested that AHF-related volume overload leads to vocal fold edema that may cause changes in phonation, such as a hoarse voice with a limited vocal range, and leads to a changed fundamental and more irregular frequency (higher jitter) (13); (2) a general swelling of the vocal tract was proposed by Amir et al. (15, 16); that may influence formant frequencies (resonance characteristics) and contribute to reduced vocal clarity or shifts in frequency bands (17); (3) Reddy et al. showed that congestion alters sound pressure levels, which might be indicative for impaired lung function (18) relating to a reduced phonation duration and variability in intensity; (4) dysphonia has been observed in patients with left atrial enlargement, which may be caused by compression of the recurrent laryngeal nerve, known as cardiovocal or Ortner's syndrome, with the inability to control strengthening of the vocal folds and resulting in a hoarse and unstable voice (19, 20). Also, a combinations of these alterations are plausible mechanisms, as voice production involves an interplay between vocal fold oscillation and airflow (21).
The AHF-Voice study aims to improve the understanding of vocal biomarkers in HF by exploring the characteristics of voice alteration in patients with AHF via daily voice recordings throughout their in-hospital period of decompensation-recompensation and the post-discharge period thereafter. Additionally, it will assess the self-perceived measurements of voice alteration and anatomically visual differences in the state of decompensation and recompensation.
Main research questions
The “Acute Heart Failure Voice Analysis Prospective Cohort Study” (AHF-Voice study) aims to explore the relationship between congestion and voice alterations in patients with AHF, with a view to reflecting their clinical condition. The study will address the following key questions: (1) What are the characteristics and determinants of vocal alterations in patients with AHF? (2) To what extent do these vocal alterations correlate with the patient's clinical status during an AHF episode? (3) To what extent are vocal biomarkers sensitive to changes over time, and how do they compare to established clinical parameters in HF such as quality of life or NT-proBNP levels? (4) Can specific vocal biomarkers or combinations thereof be mapped to different HF phenotypes? (5) Are vocal biomarkers associated with patient prognosis? (6) Are voice alterations in patients with AHF associated with pathophysiological changes, such as vocal fold edema, that affect vocal fold oscillation?
Methods
Study design
The AHF-Voice study is designed as a prospective monocentric observational cohort study. The study is being conducted at the University Hospital Würzburg as part of the UNISONO project, which is funded by the German Federal Ministry of Education and Research (Grant #16SV8877). The study was approved by the local Ethics Committee (245/22-me), complies with the Declaration of Helsinki and adheres to the STROBE reporting guidelines (22). The AHF-Voice study was registered at ISRCTN registry (ISRCTN13093083).
Setting
All patients hospitalized at the Department Internal Medicine I of the University Hospital Würzburg are screened for study participation. The study period will be 6 months after index hospitalization, including structured follow-up visits after 6 weeks and 6 months. Figure 1 shows the study design. In addition to the primary study, two sub-studies will be conducted: the Strobo sub-study and the In-Ear sub-study.
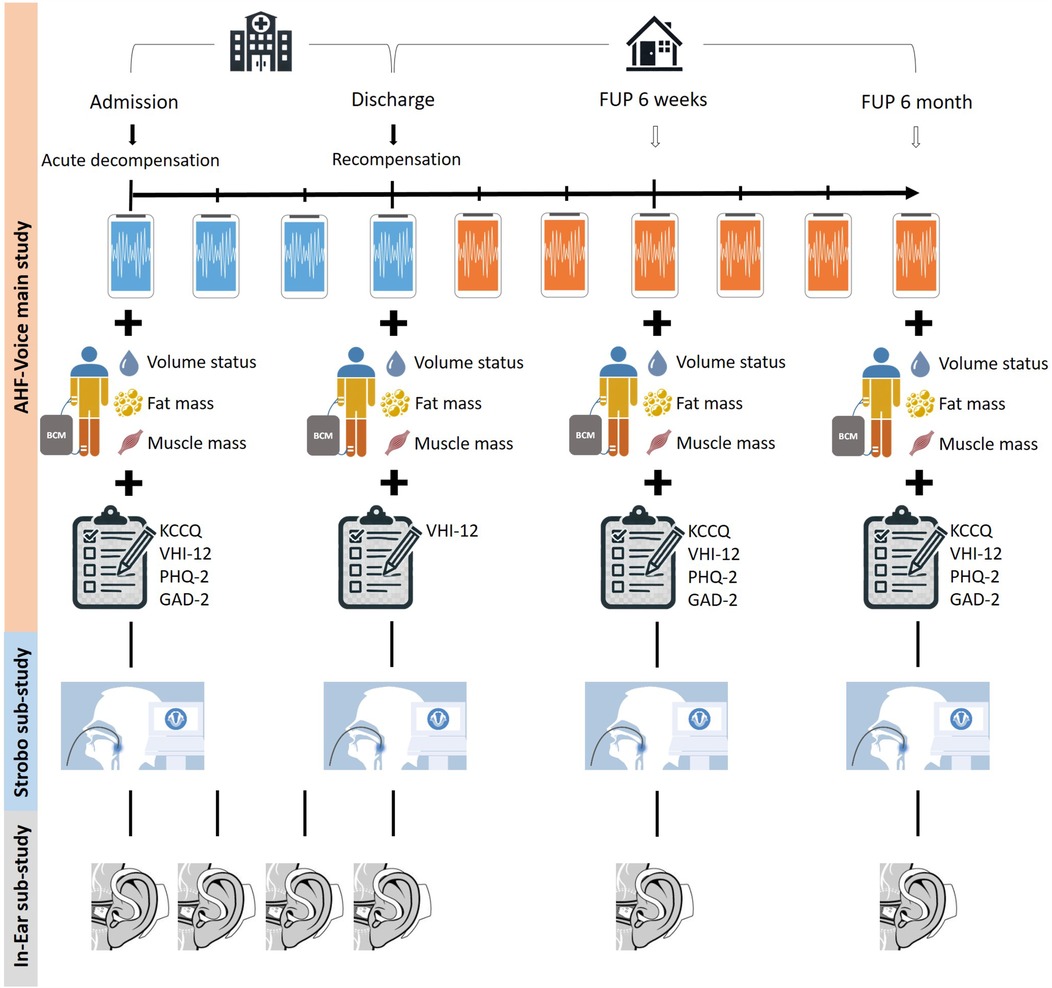
Figure 1. Design of the AHF-Voice main study and its two sub-studies. The figure shows the data collection over time. In the main study, daily voice recordings are performed during index hospitalization (blue smartphone), and voice recordings are continued at home on the patients’ own smartphones, if available (orange smartphone). In addition, body composition analyses and patient-related outcome measurements are carried out during admission, discharge, and follow-up visits. In the Strobo sub-study, a video-laryngostroboscopy and a voice field measurement will be performed on admission, discharge, and follow-up time points. As part of the In-ear sub-study, the in-ear sensor is used daily during index hospitalization, and at the 6-week and 6-month follow-up visits. BCM, body composition monitor; GAD-2, generalized anxiety disorder-2, KCCQ, Kansas City cardiomyopathy questionnaire; PHQ-2, patient health questionnaire-2.
Participants
Inclusion criteria are: hospitalization with AHF (diagnosis compatible with international guidelines including edema, dyspnea, fatigue, and/or signs of congestion in chest x-ray and elevated NT-proBNP levels) (3), age ≥18 years, life-expectancy ≥6 months, willingness to attend planned follow-up visits at the outpatient clinic, and written informed consent. Exclusion criteria are: high output HF, cardiogenic shock, high-urgency listing for heart transplant, left ventricular assist device implanted/planned, and history of vocal fold disorder or vocal fold surgery.
Index hospitalization
During the index hospitalization, detailed phenotyping of each patient will be conducted, including medical history, clinical data (e.g., transthoracic echocardiogram, electrocardiogram), and routine blood tests. At index hospitalization, patients will complete quality of life self-report questionnaires regarding health-related quality of life (23-item Kansas City Cardiomyopathy Questionnaire) (23), depression (2-item Patient Health Questionnaire) and anxiety (2-item Generalized Anxiety Disorder) (24). Additionally, a voice impairment-related questionnaire (12-item Voice Handicap Index) (25) will be conducted at admission and discharge. Daily voice recordings will be collected using a specifically designed smartphone app (ZANA Technologies GmbH, Karlsruhe, Germany) and will be performed under supervision of study staff (see Figure 2). A comprehensive assessment of body composition (Body Composition Monitor, Fresenius Medical Care, Bad Homburg, Germany) is conducted at admission and discharge.
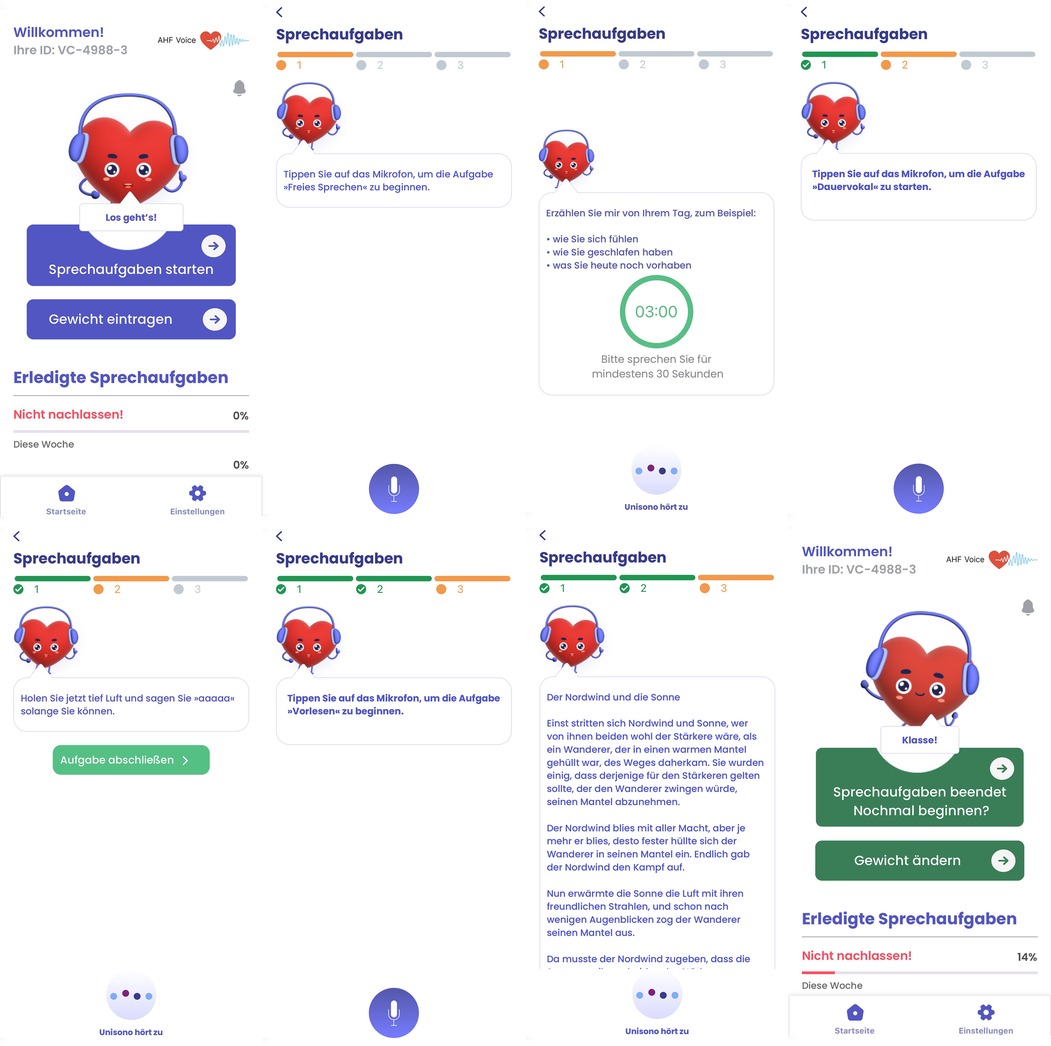
Figure 2. User interface of the specifically designed smartphone application in the AHF-Voice study. The application facilitates the recording of three distinct voice tasks: (1) spontaneous speech, (2) sustained vowel, and (3) reading of a text passage, one after the other. Additionally, the application allows the patient to maintain a weight diary. Screenshots from AHF Voice app, Zana Technologies GmbH.
In addition to the daily voice recordings, patients contributing to the In-Ear sub-study, will explore the feasibility of using novel in-ear sensor technology for comprehensive digital phenotyping in patients with HF. Therefore, patients will have an in-ear sensor inserted and will wear it for at least 5 min daily, during which vital parameters will be recorded (In-Ear sub-study). The Strobo sub-study will focus on visualizing the pathophysiological aspects of vocal changes during the AHF period. For this purpose, patients will undergo video-laryngostroboscopy (video nasopharyngoscope XN HD, Xion Medical, Berlin, Germany) and voice field measurements (Digital Video Archive Software DiVAS; Xion Medical, Berlin, Germany) at admission and discharge as well. The obtained video and audio materials will be analyzed by a blinded phoniatrist.
Follow-up period
All patients are asked to continue with the use of the mobile application for daily voice recordings after discharge on their own smartphone, if available. Six weeks and 6 months after the index hospitalization, patients are invited to the outpatient clinic of the Comprehensive Heart Failure Center at the University Hospital Würzburg (26). There, they will undergo standardized routine clinical re-evaluation including electrocardiogram, transthoracic echocardiogram, six-minute-walk test, pulmonary function tests, body composition analysis and video-laryngostroboscopy/voice field measurement (Strobo sub-study) and in-ear vital parameter measuring (In-Ear sub-study). Furthermore, participants will be asked to complete questionnaires regarding health-related quality of life (Kansas City Cardiomyopathy Questionnaire-23) (23), depression (Patient Health Questionnaire-2), anxiety (Generalized Anxiety Disorder-2) (24) and voice impairment (Voice-Handicap-Index-12) (25). Potential hospitalizations since the last study visit will be documented and tracked by requesting discharge letters. In case of death between follow-up visits, the cause will be clarified using death certificates, hospital letters, or reports from physicians and relatives. If patients do not attend follow-up visits, the study staff will conduct a standardized telephone-based interview to collect an abbreviated clinical data set.
Surrogates of congestion
AHF-Voice utilizes a number of surrogate markers to assess the level of congestion. Daily weight recordings are taken during hospitalization and follow-up visits. Patients with their own smartphone can log their daily weight through app-based entries. Routine laboratory parameters, including NT-proBNP levels, are measured at admission and discharge. Additionally, body composition assessments will be performed at admission, discharge, and during follow-up visits, providing insights into fluid overload.
Smartphone application
The smartphone application has been developed specifically for voice recording in the study. The app is available for iOS and Android systems and was developed through an iterative process that actively involved patients with HF in dedicated focus groups. Their feedback was incorporated into the design of the user interface to create a user-friendly and easy-to-use application (see Figure 2).
During index hospitalization, all patients are asked to perform daily voice recordings with a study smartphone (iPhone SE 2023, Apple Inc., Cupertino, USA) under the supervision of the study staff. Patients are then prompted through a voice dialogue interface on the smartphone app to perform the following set of speech tasks:
• Sustained vowel phonation (vowel/a:/)
• Spontaneous speech
• Reading standardized passage (“Northwind and Sun”)
During index hospitalization, study staff ensures that the same time of day is chosen for the daily voice recordings (e.g., 30 min–1 h after waking up). After discharge, patients will be asked to continue using the smartphone app on their own smartphone, if available. These patients will be instructed to install the smartphone app on their device prior to discharge, and will then be prompted to perform the speech tasks once a day. In addition, the daily weight measurements can be entered into the app. During the follow-up period, patients who do not own a smartphone will not collect voice recordings after discharge, but will contribute to the data set when attending the outpatient visits after 6 weeks and 6 months.
In-ear sensor
The in-ear sensor called c-med° alpha (Cosinuss GmbH, Munich, Germany) is comparable to a hearing aid and is inserted into the ear canal (Figure 3). It continuously and non-invasively measures body temperature, pulse rate, and blood oxygen levels in real time using optical sensors. It is approved as a Class IIa medical device (27, 28).
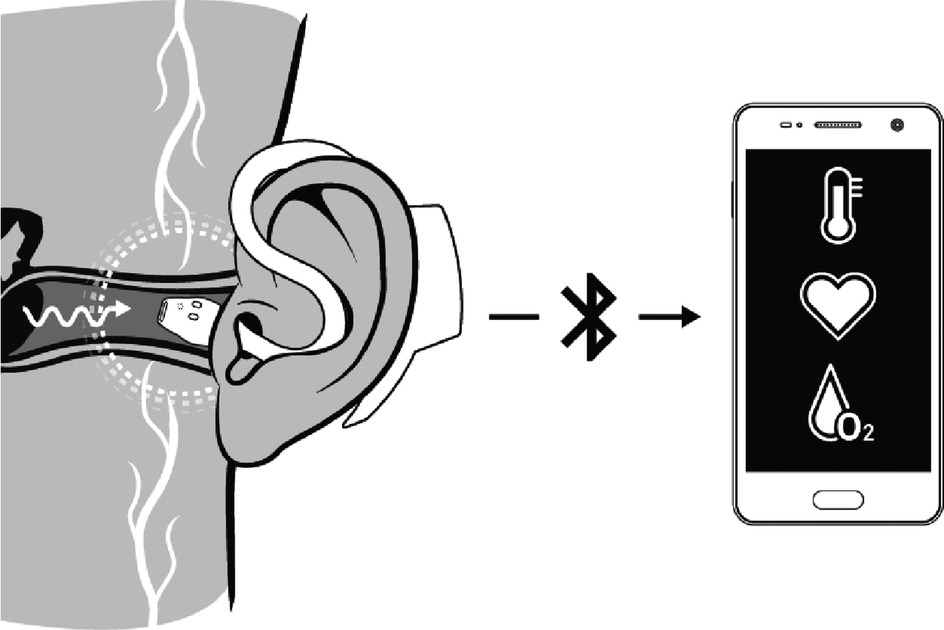
Figure 3. Application principle of the c-med° alpha in-ear sensor. The illustration shows the functionality of the in-ear sensor and illustrates its position within the ear canal. The data, which includes the pulse rate, oxygen saturation, and body temperature, is transmitted to a smartphone via bluetooth. Reproduced with permission from “Application principle of the c-med° alpha” by Cosinuss GmbH.
Premature termination of follow-up
Withdrawal of consent will trigger premature termination of follow-up. Reason for withdrawal will be documented, and efforts will be made to complete the clinical information for this last patient contact. In case a patient misses a follow-up visit, study staff contact the patient directly in order to motivate him/her to attend the clinical follow-up visit at the Comprehensive Heart Failure Center (CHFC). If a visit at the CHFC isn't possible, telephone follow-up assessments and/or completion of background information via the general practitioner or other care providers will be attempted.
Sample size calculation and power analysis
In general, there is no consensus about how to determine the sample size for clinical prediction models when applying AI methods (29). Also, the variability of sample size in the above-mentioned studies for voice analysis in patients with heart failure is high: whereas Amir et al. investigated voice alterations in 40 hospitalized patients for the association of vocal biomarkers with the binary status of congestion (“wet” vs. “dry”) (15), Maor et al. used the voice recordings of 10,583 patients (n = 8,316 for training cohort and n = 2,267 for test cohort) for the correlation of speech measures with hard clinical endpoints (i.e., hospitalization and death) (14). To conclude, the feasibility of vocal biomarker generation in patients with heart failure has been proven, but the optimal generation process is far from being well described.
The sample size calculation was performed using GPower software and based on the study of Amir et al. (16). In their study, the vocal biomarker (unitless value; mean value ± SD) of chronic heart failure patients undergoing hemodialysis treatment due to volume overload changed from 0.87 ± 0.17 before dialysis to 1.07 ± 0.15 after dialysis. The mean loss of body weight was 2 kg. From own results, we know that the volume reduction in hospitalized AHF patients is even greater (about 3 kg body weight) (30). Based on a repeated measures ANCOVA using the covariates left ventricular ejection fraction (LVEF ≥50% and <50%) and sex (♀, ♂) and assuming a conservative large variance (SD 0.30) and an effect size of f = 0.40, 111 patients are needed to achieve a power of 80% at alpha of 5%. Based on previous study experience, a drop-out rate of 10% was assumed. We therefore aim for a total sample size of 123 patients, which also allows for the analysis of changes in NT-proBNP using the methods described in Sahiti et al. (30). To this number were added those patients who withdrew from the study prematurely while still in hospital, resulting in a cohort of 131 patients.
Data analysis of baseline characteristics
Data are summarized using descriptive statistics. For continuous variables, normally distributed data are presented as mean and standard deviation, while non-normally distributed data are expressed as median and quartiles. Categorical variables are reported as absolute numbers and percentages.
Future statistical analyses
Data will be described using conventional methods according to the nature of the data. Repetitively sampled data will be investigated using generalized estimating equations (GEE) for repeated measures analysis (vocal feature) or repeated measures analysis of (co)variance (rANCOVA; e.g., for clinical data). To investigate the prognostic utility, Kaplan–Meier plots for graphical inspection and uni- and multivariable Cox proportional hazards regression analyses will be used. Subgroup analyses will include age, sex, de novo vs. decompensated chronic HF, heart failure with reduced ejection fraction (HFrEF) vs. heart failure with preserved ejection fraction (HFpEF). post-hoc comparisons of baseline findings and follow-up patient outcomes may be performed in the entire study and sub-populations of special interest. Appropriate adjustment for potential confounding and multiple testing will be considered for each research question separately.
AI-based methods, including machine learning and deep learning will be performed for the derivation of clinically meaningful vocal biomarker in patients with AHF applying primarily a binary approach (“decompensated” vs. “recompensated”).
Missing data
All patients of the AHF-Voice study will be included, possibly with censored observation times. All efforts will be taken to prevent missing values in subjects who are alive. Whenever there is the possibility of significant bias due to missing data, additional analyses using imputation techniques will be employed and used for supportive sensitivity analysis.
Results
Recruitment and characteristics of the AHF-voice study sample
Between April 2023 and November 2024, 131 patients were recruited for the AHF-Voice Study. 50 (38%) of the patients participated in the Strobo sub-study, and 31 (24%) in the In-Ear sub-study. Of 131 patients, 9 (7%) of patients withdrew their consent during the follow-up observation time. Non-participation of patients was primarily attributable to physical or mental limitations.
Table 1 summarizes the baseline characteristics of the study population. The mean age of participants was 75 ± 10 years, 31% were women, and 38% were diagnosed with de novo HF. The majority of patients were in New York Heart Association (NYHA) functional class III (57%) or IV (29%) and the median NT-proBNP level at admission was 5,215 [2,650; 13,558] pg/ml. The most frequent underlying cause of HF was an ischemic etiology (42%), the mean LVEF was 47 ± 17, and 47% exhibited HF with a preserved ejection fraction (HFpEF). The most common comorbidities were diabetes (38%), peripheral artery disease (17%), chronic obstructive pulmonary disease (18%), and 10% current-smokers and 50% former smokers. The median length of hospital stay was 10 [7; 15] days. The majority of patients (59%) had their own smartphone, which enabled them to continue with daily voice recordings after discharge.
Overall, 3,072 voice recordings (1,024 per voice task) were collected during the hospitalization period of all 131 patients, corresponding to a median of 21 [15; 30] voice recordings (7 [5; 10] per voice tasks) per patient.
Discussion
The AHF-Voice study has been designed to provide new insights into the etiology, characteristics, determinants, progression, and prognostic utility of vocal biomarkers in patients experiencing an episode of AHF. Smartphone-based daily voice recordings are used as a substrate to detect subtle vocal changes, which are then associated with conventional markers of congestion.
The AHF-Voice study cohort represents a well-phenotyped population of patients with AHF. It comprises a high proportion of patients with NYHA functional class III and IV at admission, indicating a substantial number of patients with significant congestion. The cohort includes a significant proportion of patients with de novo HF and an even distribution between heart failure with a reduced or mildly reduced ejection fraction and HFpEF. The profound differences between LVEF-based HF phenotypes (31) will allow for stratified analyses, thereby exploring potential voice-based differences between these HF subtypes. Furthermore, a substantial number of voice recordings were collected during the index hospitalization and will be collected during follow-up period, enabling the application of advanced artificial intelligence methodologies for predictive modeling.
Changes of vocal biomarkers over time and their determinants remain under-researched. Previous studies only suggested the existence of distinct vocal biomarker patterns in individuals exhibiting either congested or decongested states (13, 15). To address this gap, the AHF-Voice study will investigate the longitudinal trajectories of vocal biomarkers in patients with AHF through daily voice recordings, both during the course of their hospitalization and over a 6-month observation period. This approach will facilitate a comprehensive assessment of vocal changes in relation to disease progression and patient outcomes, thereby contributing to the development of more personalized and predictive care strategies for patient with AHF.
According to the European Laryngological Society (ELS) and the American Speech-Language-Hearing Association, a comprehensive voice assessment for general voice impairments in the field of phoniatrics is suggested (32, 33). The AHF-Voice study will be the first to comprehensively examine all four (auditory, visual, physician-reported, and patient-reported) dimensions of the human voice: (i) vocal characteristics are captured through the recording of various voice tasks; (ii) visual assessment of the vocal cords is conducted using video-laryngostroboscopy, with vocal fold description following the guidelines of the ELS (32); (iii) an independent phoniatrist evaluates the voice using the RBH (roughness, breathiness, hoarseness) scale; (iv) all patients provide self-reported outcomes via the Voice Handicap Index-12.
As previously stated, the pathophysiological reasons for voice alterations in patients with HF remain unclear. The considerable number of patients participating in the Strobo sub-study will enable the provision of innovative insights into the pathophysiological aspects of disturbed vocal cord function and its potential association with prognosis, including rehospitalization and mortality within six months. Furthermore, the quality of voice recordings can vary due to factors such as ambient noise, the distance between the mouth and microphone, and the type of smartphone used by the patient after discharge (34). To assess and compare these impacts, simultaneous voice recordings were collected using both a smartphone and the reference standard of voice field measurement.
The importance of digital health technology in clinical trials is increasing (35, 36). Our study encompasses the development of a dedicated mobile solution and deploys smartphone-based remote data collection, allowing for asynchronous and continuous data collection in both hospital and home settings. The results from the AHF-Voice study will inform future decentralized and virtual clinical trials. Furthermore, vocal biomarkers may serve as a novel digital endpoint in future HF trials.
The study has several limitations. First, selection bias may affect the generalizability of the findings, as only German-speaking participants were included, which limits the applicability of the results to non-German speakers. Further studies should be conducted in additional languages in order to gain a more comprehensive understanding of the impact of language. Alterations in voice quality resulting from upper respiratory infections during the study period could confound the analysis of vocal biomarkers. For this purpose, a longitudinal data collection approach was chosen, and clinical (e.g., fever) and laboratory parameters (e.g., C-reactive protein) of inflammation assessed at each study visit. Finally, it is possible that participants' increased awareness of potential voice disorders, due to their involvement in the study, may influence their self-assessments of their voice.
Conclusion
The AHF-Voice study provides a comprehensive framework deriving vocal biomarkers from voice recordings, covering all four dimensions of voice diagnostics: self-reported outcomes, physician-reported assessments, visual evaluation of the vocal cords, and acoustic voice analyses. The longitudinal design of the study permits the continuous monitoring of the voice over time, thereby offering valuable insights into the trajectories of voice alterations and their association with disease progression and prognosis in patients with HF experiencing an AHF episode. Moreover, the investigation aims to explore the potential of vocal biomarkers as a future tool for telemonitoring, with the objective to enable the early detection of decompensation in HF patients. In addition, the AHF-Voice study will inform on the utility of vocal biomarkers as digital endpoints for future HF trials.
Data availability statement
The original contributions presented in the study are included in the article/Supplementary Material, further inquiries can be directed to the corresponding author.
Ethics statement
The studies involving humans were approved by medical ethics committee, University Würzburg. The studies were conducted in accordance with the local legislation and institutional requirements. The participants provided their written informed consent to participate in this study.
Author contributions
FKerw: Writing – original draft, Writing – review & editing. MBaus: Writing – original draft, Writing – review & editing. MBaur: Writing – review & editing. FKrau: Writing – review & editing. CM: Writing – review & editing. RP: Writing – review & editing. KR: Writing – review & editing. SF: Writing – review & editing. MW: Writing – review & editing. JH: Writing – review & editing. SSt: Writing – review & editing, Writing – original draft.
Funding
The author(s) declare that financial support was received for the research and/or publication of this article. The authors declare that this study received funding from Federal Ministry of Education and Research (BMBF, #16SV8877) and was conducted within the UNISONO project. The funder was not involved in the study design, collection, analysis, interpretation of data, the writing of this article, or the decision to submit it for publication.
Conflict of interest
FKerw is supported through the clinician scientist-program UNION-CVD of the German Research Council (DFG, #413657723); he reports travel support from Novartis and Lilly, and research support from Novartis and Bayer, all unrelated to the study. MBaus reports travel support from Lilly, unrelated to the study. MBaur reports no funding and disclosures in relation to the study. FKrau reports no funding and disclosures in relation to the study. CM reports research cooperation with the University of Würzburg and Tomtec Imaging Systems funded by a research grant from the Bavarian Ministry of Economic Affairs, Regional Development and Energy, German Germany (MED-1811-0011 and LSM-2104-0002); she is supported by the German Research Foundation (DFG) within the Comprehensive Research Center 1525 “Cardio-immune interfaces” (453989101, project C5) and receives financial support from the Interdisciplinary Center for Clinical Research - IZKF Würzburg (advanced clinician-scientist program; AdvCSP 3). She further received advisory and speakers honoraria as well as travel grants from Tomtec, Edwards, Alnylam, Pfizer, Boehringer Ingelheim, Eli Lilly, SOBI, AstraZeneca, NovoNordisk, Alexion, Janssen, Bayer, Intellia, and EBR Systems; she serves as principal investigator in trials sponsored by Alnylam, Bayer, NovoNordisk, Intellia and AstraZeneca, all unrelated to the study. RP is a partner in Lenox UG, which has set itself the goal of translating scientific findings into digital health applications. Lenox UG holds shares in HealthStudyClub GmbH; he received consulting fees, reimbursements for congress attendance and travel expenses as well as payments for lectures in the context of diabetes topics and in connection with mobile health and e-mental health topics, all unrelated to the study. KR is supported by the German Research Council (DFG) and the Interdisciplinary Centre for Clinical Research (IZFK) Würzburg. He receives travel grants from MED-EL, Advance Bionics and Cochlear, all unrelated to the study. SF is supported by the German Research Council (DFG). He has received consultancy and lecture fees as well as support/ travel grants for meetings from Abbot, Abiomed, Amarin, Amgen, AstraZeneca, Bayer, Berlin-Chemie, Biotronik, Boehringer, Bristol-Myers Squibb, Boehringer, Daiichi Sankyo, Edwards, Lilly, Novartis, Novo Nordisk, Pfizer, Sanofi-Aventis, Siemens, Vifor, Zoll, all unrelated to the study. MW is an employee of Cosinuss GmbH. JH is CEO and cofounder of ZANA Technologies GmbH. She reports research funded by the German Federal Ministry of Education and Research (BMBF); she reports research supported from AstraZeneca and Boehringer Ingelheim, all unrelated to the study. SSt is supported by the German Federal Ministry of Education and Research (BMBF); he received honoraria as speaker or member of advisory boards by AstraZeneca, Bayer, Boehringer Ingelheim, Novartis, NovoNordisk, Pfizer, Servier, Vifor, all unrelated to the study; he reports research support from Alnylam, Akcea, Amgen, AstraZeneca, Bayer, Boehringer Ingelheim, Cytokinetics, Lilly, MSD, Novartis, NovoNordisk, Pfizer, all unrelated to the study.
Generative AI statement
The author(s) declare that no Generative AI was used in the creation of this manuscript.
Publisher's note
All claims expressed in this article are solely those of the authors and do not necessarily represent those of their affiliated organizations, or those of the publisher, the editors and the reviewers. Any product that may be evaluated in this article, or claim that may be made by its manufacturer, is not guaranteed or endorsed by the publisher.
Abbreviations
AHF, acute heart failure; ELS, European laryngological society; GAD-2, generalized anxiety disorder—2; KCCQ, Kansas City cardiomyopathy questionnaire; LVEF, left ventricular ejection fraction; NT-proBNP, N-terminal prohormone of brain natriuretic peptide; NYHA, New York Heart Association; PHQ-2, patient health questionnaire-2; RBH, roughness, breathiness, hoarseness; RPM, remote patient management.
References
1. Savarese G, Becher PM, Lund LH, Seferovic P, Rosano GMC, Coats AJS. Global burden of heart failure: a comprehensive and updated review of epidemiology. Cardiovasc Res. (2022) 118(17):3272–87. doi: 10.1093/cvr/cvac013
2. Dörr M, Riemer U, Christ M, Bauersachs J, Bosch R, Laufs U, et al. Hospitalizations for heart failure: still major differences between east and west Germany 30 years after reunification. ESC Heart Fail. (2021) 8(4):2546–55. doi: 10.1002/ehf2.13407
3. McDonagh TA, Metra M, Adamo M, Gardner RS, Baumbach A, Bohm M, et al. 2021 ESC guidelines for the diagnosis and treatment of acute and chronic heart failure. Eur Heart J. (2021) 42(36):3599–726. doi: 10.1093/eurheartj/ehab368
4. Setoguchi S, Stevenson LW, Schneeweiss S. Repeated hospitalizations predict mortality in the community population with heart failure. Am Heart J. (2007) 154(2):260–6. doi: 10.1016/j.ahj.2007.01.041
5. Arrigo M, Jessup M, Mullens W, Reza N, Shah AM, Sliwa K, et al. Acute heart failure. Nat Rev Dis Primers. (2020) 6(1):16. doi: 10.1038/s41572-020-0151-7
6. Scholte NTB, Gürgöze MT, Aydin D, Theuns D, Manintveld OC, Ronner E, et al. Telemonitoring for heart failure: a meta-analysis. Eur Heart J. (2023) 44(31):2911–26. doi: 10.1093/eurheartj/ehad280
7. Koehler F, Koehler K, Deckwart O, Prescher S, Wegscheider K, Kirwan BA, et al. Efficacy of telemedical interventional management in patients with heart failure (TIM-HF2): a randomised, controlled, parallel-group, unmasked trial. Lancet. (2018) 392(10152):1047–57. doi: 10.1016/S0140-6736(18)31880-4
8. Kerwagen F, Koehler K, Vettorazzi E, Stangl V, Koehler M, Halle M, et al. Remote patient management of heart failure across the ejection fraction spectrum: a pre-specified analysis of the TIM-HF2 trial. Eur J Heart Fail. (2023) 25(9):1671–81. doi: 10.1002/ejhf.2948
9. Fagherazzi G, Fischer A, Ismael M, Despotovic V. Voice for health: the use of vocal biomarkers from research to clinical practice. Digit Biomark. (2021) 5(1):78–88. doi: 10.1159/000515346
10. Tracy JM, Özkanca Y, Atkins DC, Hosseini Ghomi R. Investigating voice as a biomarker: deep phenotyping methods for early detection of Parkinson’s disease. J Biomed Inform. (2020) 104:103362. doi: 10.1016/j.jbi.2019.103362
11. Laguarta J, Hueto F, Subirana B. COVID-19 artificial intelligence diagnosis using only cough recordings. IEEE Open J Eng Med Biol. (2020) 1:275–81. doi: 10.1109/OJEMB.2020.3026928
12. Maor E, Sara JD, Orbelo DM, Lerman LO, Levanon Y, Lerman A. Voice signal characteristics are independently associated with coronary artery disease. Mayo Clin Proc. (2018) 93(7):840–7. doi: 10.1016/j.mayocp.2017.12.025
13. Murton OM, Hillman RE, Mehta DD, Semigran M, Daher M, Cunningham T, et al. Acoustic speech analysis of patients with decompensated heart failure: a pilot study. J Acoust Soc Am. (2017) 142(4):EL401. doi: 10.1121/1.5007092
14. Maor E, Perry D, Mevorach D, Taiblum N, Luz Y, Mazin I, et al. Vocal biomarker is associated with hospitalization and mortality among heart failure patients. J Am Heart Assoc. (2020) 9(7):e013359. doi: 10.1161/JAHA.119.013359
15. Amir O, Abraham WT, Azzam ZS, Berger G, Anker SD, Pinney SP, et al. Remote speech analysis in the evaluation of hospitalized patients with acute decompensated heart failure. JACC Heart Fail. (2022) 10(1):41–9. doi: 10.1016/j.jchf.2021.08.008
16. Amir O, Anker SD, Gork I, Abraham WT, Pinney SP, Burkhoff D, et al. Feasibility of remote speech analysis in evaluation of dynamic fluid overload in heart failure patients undergoing haemodialysis treatment. ESC Heart Fail. (2021) 8(4):2467–72. doi: 10.1002/ehf2.13367
18. Mittapalle KR, Pohjalainen H, Helkkula P, Kaitue K, Minkkinen M, Tolppanen H, et al. Glottal flow characteristics in vowels produced by speakers with heart failure. Speech Commun. (2022) 137:35–43. doi: 10.1016/j.specom.2021.12.001
19. Mulpuru SK, Vasavada BC, Punukollu GK, Patel AG. Cardiovocal syndrome: a systematic review. Heart Lung Circ. (2008) 17(1):1–4. doi: 10.1016/j.hlc.2007.04.007
20. Prada-Delgado O, Barge-Caballero E. Images in clinical medicine. Ortner’s syndrome. N Engl J Med. (2011) 365(10):939. doi: 10.1056/NEJMicm1014167
21. Zhang Z. Mechanics of human voice production and control. J Acoust Soc Am. (2016) 140(4):2614–35. doi: 10.1121/1.4964509
22. von Elm E, Altman DG, Egger M, Pocock SJ, Gøtzsche PC, Vandenbroucke JP. The strengthening the reporting of observational studies in epidemiology (STROBE) statement: guidelines for reporting observational studies. Lancet. (2007) 370(9596):1453–7. doi: 10.1016/S0140-6736(07)61602-X
23. Faller H, Steinbüchel T, Schowalter M, Spertus JA, Störk S, Angermann CE. The Kansas city cardiomyopathy questionnaire (KCCQ)—a new disease-specific quality of life measure for patients with chronic heart failure. Psychother Psychosom Med Psychol. (2005) 55(3–4):200–8. doi: 10.1055/s-2004-834597
24. Löwe B, Wahl I, Rose M, Spitzer C, Glaesmer H, Wingenfeld K, et al. A 4-item measure of depression and anxiety: validation and standardization of the patient health questionnaire-4 (PHQ-4) in the general population. J Affect Disord. (2010) 122(1–2):86–95. doi: 10.1016/j.jad.2009.06.019
25. Gantner S, Caffier P, Hulin P, Fuchs M, Kummer P, Lorenz A. Der singing voice handicap index-12: entwicklung und validierung einer deutschen Fassung [Singing voice handicap index-12 : development and validation of a German version]. HNO. (2022) 70(2):117–24. doi: 10.1007/s00106-021-01092-3
26. Angermann CE, Frantz S, Maack C. The comprehensive heart failure centre in würzburg, Germany. Eur Heart J. (2018) 39(20):1757–60. doi: 10.1093/eurheartj/ehy202
27. Wurzer D, Spielhagen P, Siegmann A, Gercekcioglu A, Gorgass J, Henze S, et al. Remote monitoring of COVID-19 positive high-risk patients in domestic isolation: a feasibility study. PLoS One. (2021) 16(9):e0257095. doi: 10.1371/journal.pone.0257095
28. Adams T, Wagner S, Baldinger M, Zellhuber I, Weber M, Nass D, et al. Accurate detection of heart rate using in-ear photoplethysmography in a clinical setting. Front Digit Health. (2022) 4:909519. doi: 10.3389/fdgth.2022.909519
29. Baeza-Delgado C, Cerdá Alberich L, Carot-Sierra JM, Veiga-Canuto D, Martínez de Las Heras B, Raza B, et al. A practical solution to estimate the sample size required for clinical prediction models generated from observational research on data. Eur Radiol Exp. (2022) 6(1):22. doi: 10.1186/s41747-022-00276-y
30. Sahiti F, Morbach C, Henneges C, Stefenelli U, Scholz N, Cejka V, et al. Dynamics of left ventricular myocardial work in patients hospitalized for acute heart failure. J Card Fail. (2021) 27(12):1393–403. doi: 10.1016/j.cardfail.2021.07.004
31. Savarese G, Stolfo D, Sinagra G, Lund LH. Heart failure with mid-range or mildly reduced ejection fraction. Nat Rev Cardiol. (2022) 19(2):100–16. doi: 10.1038/s41569-021-00605-5
32. Dejonckere PH, Bradley P, Clemente P, Cornut G, Crevier-Buchman L, Friedrich G, et al. A basic protocol for functional assessment of voice pathology, especially for investigating the efficacy of (phonosurgical) treatments and evaluating new assessment techniques. Guideline elaborated by the committee on phoniatrics of the European laryngological society (ELS). Eur Arch Otorhinolaryngol. (2001) 258(2):77–82. doi: 10.1007/s004050000299
33. Patel Rita R, Awan Shaheen N, Barkmeier-Kraemer J, Courey M, Deliyski D, Eadie T, et al. Recommended protocols for instrumental assessment of voice: American speech-language-hearing association expert panel to develop a protocol for instrumental assessment of vocal function. Am J Speech Lang Pathol. (2018) 27(3):887–905. doi: 10.1044/2018_AJSLP-17-0009
34. Awan SN, Shaikh MA, Awan JA, Abdalla I, Lim KO, Misono S. Smartphone recordings are comparable to “gold standard” recordings for acoustic measurements of voice. J Voice. (2023) 14. doi: 10.1016/j.jvoice.2023.01.031
35. Mittermaier M, Venkatesh KP, Kvedar JC. Digital health technology in clinical trials. NPJ Digit Med. (2023) 6(1):88. doi: 10.1038/s41746-023-00841-8
Keywords: heart failure, decompensation, digital health, telemonitoring, remote patient management, voice, vocal biomarker, study design
Citation: Kerwagen F, Bauser M, Baur M, Kraus F, Morbach C, Pryss R, Rak K, Frantz S, Weber M, Hoxha J and Störk S (2025) Vocal biomarkers in heart failure—design, rationale and baseline characteristics of the AHF-Voice study. Front. Digit. Health 7:1548600. doi: 10.3389/fdgth.2025.1548600
Received: 19 December 2024; Accepted: 26 March 2025;
Published: 2 May 2025.
Edited by:
Toshiyo Tamura, Waseda University, JapanReviewed by:
Tanmoy Sarkar Pias, Virginia Tech, United StatesSteffen Pauws, Tilburg University, Netherlands
Copyright: © 2025 Kerwagen, Bauser, Baur, Kraus, Morbach, Pryss, Rak, Frantz, Weber, Hoxha and Störk. This is an open-access article distributed under the terms of the Creative Commons Attribution License (CC BY). The use, distribution or reproduction in other forums is permitted, provided the original author(s) and the copyright owner(s) are credited and that the original publication in this journal is cited, in accordance with accepted academic practice. No use, distribution or reproduction is permitted which does not comply with these terms.
*Correspondence: Fabian Kerwagen, a2Vyd2FnZW5fZkB1a3cuZGU=
†These authors have contributed equally to this work and share first authorship