- 1Department of Epidemiology and Health Statistics, Public Health College, Qingdao University, Qingdao, China
- 2Qingdao Municipal Center for Disease Control and Prevention, Qingdao, China
- 3Epidemiology and Biostatistics, Department of Public Health, University of Southern Denmark, Odense, Denmark
- 4Unit of Human Genetics, Department of Clinical Research, University of Southern Denmark, Odense, Denmark
Previous genome-wide association studies on anthropometric measurements have identified more than 100 related loci, but only a small portion of heritability in obesity was explained. Here we present a bivariate twin study to look for the genetic variants associated with body mass index and waist-hip ratio, and to explore the obesity-related pathways in Northern Han Chinese. Cholesky decomposition model for 242 monozygotic and 140 dizygotic twin pairs indicated a moderate genetic correlation (r = 0.53, 95%CI: 0.42–0.64) between body mass index and waist-hip ratio. Bivariate genome-wide association analysis in 139 dizygotic twin pairs identified 26 associated SNPs with p < 10−5. Further gene-based analysis found 291 nominally associated genes (P < 0.05), including F12, HCRTR1, PHOSPHO1, DOCK2, DOCK6, DGKB, GLP1R, TRHR, MMP1, GPR55, CCK, and OR2AK2, as well as 6 enriched gene-sets with FDR < 0.05. Expression quantitative trait loci analysis identified rs2242044 as a significant cis-eQTL in both the normal adipose-subcutaneous (P = 1.7 × 10−9) and adipose-visceral (P = 4.4 × 10−15) tissue. These findings may provide an important entry point to unravel genetic pleiotropy in obesity traits.
Introduction
Obesity is a worldwide epidemic associated with increased morbidity and mortality, and greatly contributes to the global disease burden (Fall and Ingelsson, 2014). The evidence from family and twin studies have showed that around 40–70% (Sandholt et al., 2012) of the obesity variation in human was attributed to genetic factors. Searching for related susceptibility loci would help to better understand the molecular mechanisms and biological pathways of obesity.
Usually obesity is approximately assessed by anthropometric measurements including body mass index (BMI) and waist-hip ratio (WHR), because of its low cost of efficiency (Fall and Ingelsson, 2014). Previous genome-wide association studies (GWAS) on these anthropometric measurements using univariate analysis identified more than 100 related loci, but only a small portion of heritability in obesity was explained by those genetic variants (Fall and Ingelsson, 2014; Locke et al., 2015; Shungin et al., 2015). Since complex disease is contributed from many genetic factors, theoretically univariate GWAS is less powerful for identifying the potential genetic correlation in correlated traits such as BMI and WHR. Therefore, it is hard to identify the pleiotropic genes associated with a set of correlated phenotypes that play a central role in the pathogenesis (Allison et al., 1998; Lu et al., 2016). Bivariant or multivariate association studies, which consider two or more phenotypes simultaneously, could help to address this issue. They not only could find pleiotropic genes, but also could relieve multiple testing problems. These studies have successfully identified susceptibility genes for several complex traits, including Kashin-Beck disease, heart rate and low density lipoprotein subfractions (Melton et al., 2010; Shim et al., 2015; Hao et al, 2016). However, limited bivariate and multivariate GWA analyses of obesity-related phenotypes have been reported.
Human twins are two offspring produced during one pregnancy. Monozygotic (MZ) twin share 100% of their genetic background, while dizygotic (DZ) twin share only 50% (Zheng et al., 2013). Twins are particularly valuable for genetic studies due to their sharing of intrauterine and rearing environments, as well as the genetic similarity and dissimilarity (Tan et al., 2015). By applying structure equation model using MZ and DZ twin samples, the classical twin design is able to estimate heritability for an given phenotype (Duan et al., 2011; Wu et al., 2015). It is usually believed that using unrelated individuals in the GWAS of a quantitative phenotype is better than using related family members because related individuals could be “over-matched” for genotypes. However, researchers reported that for GWAS on a quantitative phenotype with related individuals, little power was lost whilst there are manifold additional advantages, including better quality control, more robust population stratification and fewer false positive (Visscher et al., 2008; Benyamin et al., 2009; Rosenthal et al., 2015).
Here we presented a bivariate twin study design to: (1) assess the genetic correlation between BMI and WHR; (2) determine the BMI-WHR jointly associated genetic variants; and (3) explore the obesity-related pathways in Northern Han Chinese.
Subjects and Methods
Study Population
The study participants were recruited from the Qingdao Twin Registry, China (Pang et al., 2006; Duan et al., 2013). All of the twins in the current study were taken from the latest wave of genetic epidemiology survey on aging phenotypes conducted from 2012 to 2013. Information was collected through questionnaire, extraction of blood, together with anthropometric and laboratory measurements by well-trained clinicians. Zygosity was identified by DNA markers at the laboratory in Qingdao Blood Center. Subjects were included with the following criteria: aged ≥30 years old; Han Chinese; ancestral home is in Shandong Province; free of heart failure, kidney failure, cancer, or severe mental disorder; not pregnant, breast feeding or taking weight-reducing medication within 1 month. A total of 242 pairs of MZ twins including 1 pair of triplet and 140 DZ twins have met the criteria and were included in the current sample. Signed consent forms were obtained from all participants and the study was approved by the Qingdao Center for Disease Control and Prevention Ethics Committee.
Phenotypes
Weight and standing height were measured for these subjects with lightweight clothes on and shoes removed. Waist circumference was measured at the midpoint between the iliac crest and the lower rib, and hip circumference was measured at the widest circumference over the gluteal muscles. BMI was defined as weight (kilogram) divided by height (meter) squared. WHR was defined as waist circumference (centimeter) divided by hip circumference (centimeter).
Heritability Calculation
A bivariate heritability analysis in 242 MZ and 140 DZ twins was conducted by using the structure equation modeling package Mx (Wu et al., 2011). The Cholesky decomposition model was fitted, with age and sex adjusted. Variations for both phenotype as well as their covariance were decomposed into sources of additive genetic (A), common (shared) environmental (C), and unique (non-shared) environmental (E) parameters. After fitting the full ACE model, the nested models were constructed by dropping the C (AE model) or the A (CE model). The likelihood ratio chi-square tests (-2LL; significant tests indicate significant deterioration in fit) were applied to compare the performances between the full model and its nested models. The index of Akaike's Information Criterion (AIC; parsimony fit index, with lower values indicating the more suitable model) was calculated to estimate the parsimony of the model.
Genotyping and GWAS
Genomic DNA was extracted from the whole peripheral blood of the subject using QIAamp DNA Blood Mini Kit (Qiagen, GmbH, Hilden, Germany). DNA quantification and integrity were determined by the Nanodrop spectrophotometer (Thermo Fisher Scientific Inc., Wilmington, DE USA) and the 1% agarose electrophoresis, respectively. Genotyping of 278 eligible DNA samples from 139 DZ twin pairs was performed at the BioMiao Biological Technology Company Limited (Beijing, China) using Infinium Omni2.5Exome-8v1.2 genotyping BeadChips (Illumina Inc, San Diego, USA), which covers 2,608,742 SNPs. Only the data from the autosomes were analyzed. The raw data were compiled and tested using GenomeStudio (Illumina) and PLINK tool. All samples have SNP call rates over 95%. Single SNP had to meet the following criteria: minor allele frequencies (MAF) > 0.01, Hardy-Weinberg equilibrium (HWE) significance > 1 × 10−4. Finally, 1,365,181 SNPs from 278 samples (139 pairs) were used for the current GWA analysis.
Bivariate GWA analysis of BMI-WHR for 139 DZ twin pairs was done using the program of genome-wide efficient mixed-model analysis (GEMMA) (Zhou and Stephens, 2012). Genetic relatedness matrix and Bayesian sparse linear mixed model were used with age and sex adjusted as covariates. Since our sample size was limited, we did not anticipate genome-wide significant findings (P < 0.05 × 10−8), and a suggestive evidence level of P < 1 × 10−5 was adopted (Ran et al., 2013; Loukola et al., 2014). Linkage disequilibrium (LD) block analysis was performed for the jointly significant SNPs located near the same gene by using Haploview system in the “Han Chinese in Beijing, China (CHB)” sample.
Gene-Based Analysis
Individual SNP results from the bivariate GWAS were aggregated to conduct a gene-based analysis by using the SNP-set association test implemented in Versatile Gene-based Association Study-2 (VEGAS2, Version of HPC Linux). “1000G East ASIAN” sample was selected. We set P < 2.82 × 10−6 (0.05/17,723) as genome-wide significant using Bonferroni correction. The P < 0.05 was considered as nominal significance level (Xu et al., 2017).
Gene Set Enrichment Analysis (GSEA)
Significant genes yielded by gene-based analysis were evaluated for enrichment of gene-sets in Reactome and KEGG pathways using GSEA (http://software.broadinstitute.org/gsea/index.jsp). False discovery rate (FDR), analog of hypergeometric P-value after correction for multiple hypothesis testing according to Benjamini and Hochberg was calculated to obtain the significant (FDR < 0.05) biological pathways.
Expression Quantitative Trait Loci (eQTL) Analysis
An eQTL search was conducted by submitting the list of suggestively significant SNPs generated by GWAS to HaploReg V4.1 (http://archive.broadinstitute.org/mammals/haploreg/haploreg.php). The GTEx project portal (https://www.gtexportal.org/home/) (as of February 2018) (Consortium, 2013; Loo et al., 2017) was used to identify cis-eQTLs in normal adipose-subcutaneous (n = 385), adipose-visceral (omentum) (n = 313) or muscle-skeletal (n = 491) for our top 20 genes from VEGAS2. Significant level was defined as FDR < 0.05.
Results
Heritability Analysis
The basic characteristics of the current sample were summarized in Table 1. The phenotypic correlation between BMI and WHR was 0.39 (P < 0.001) and further best fitting Cholesky decomposition model (AE) identified that the genetic correlation between the two phenotypes was 0.53 (95%CI: 0.42–0.64). The standardized path coefficients from best fitting Cholesky decomposition model were presented in Figure 1.
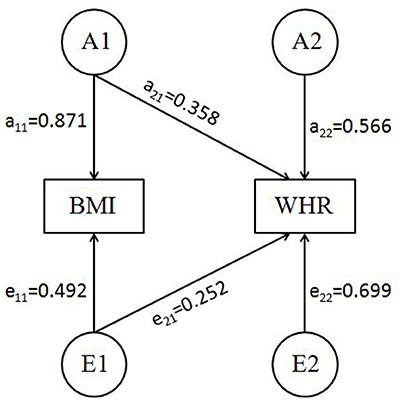
Figure 1. Best bivariate model (AE model) for BMI-WHR with standardized path coefficients (SEM pathway plot). A1, A2 = genetic variance components; E1, E2 = unique environmental variance components; a11 through a22 = genetic path coefficients of which a22 represents specific genetic influence on WHR; e11 through e22 = unique environmental path coefficients, of which e22 represents specific unique environmental influence on WHR.
Bivariate GWA Analysis
A bivariate GWA analysis on BMI-WHR in 139 DZ twin pairs was performed. The relationship between the observed and expected GWAS P-values was illustrated in the quantile-quantile plot (Figure 2). There was a slight deviation in the upper right tail from the null hypothesis, suggesting an evidence for weak association. A low level of λ-statistic (λ = 1.045) indicated no evidence of genomic inflation or bias from population stratification. As illustrated from the Manhattan plot (Figure 3), although none of the SNPs reached the genome-wide significance level, 26 SNPs involved in 10 genes that were suggestively associated with BMI-WHR (P < 10−5) were identified. The characteristics of the 26 SNPs were summarized in Table 2. Considering that several SNPs were mapped near the same gene with the similar beta value, LD block analyses were performed and identified that 11 SNPs near the LINC0034 gene (from r2 = 0.801 for rs7320405 and rs9586994 to r2 = 1 for rs2025924 and rs13378734), 4 SNPs near the LOC105375763 gene (r2 = 1 for each other), 2 SNPs near the DOCK2 gene (r2 = 1), 2 SNPs near the LOC101928945 gene (r2 = 1) were in the same block (the conventional threshold of r2 > 0.8). The SNP rs201664108 near the LOC105375763 gene was not available in Haploview. In addition, we mapped the genome-wide significant SNPs reported from previous meta-analyses, including 97 BMI loci (Locke et al., 2015), 49 WHR adjusted for BMI loci (Shungin et al., 2015) and 10 BMI loci in East Asian (Wen et al., 2012), to the region of 100 kilo-bases (kb) upstream and downstream from the 26 SNPs identified by our study. No overlap was found.
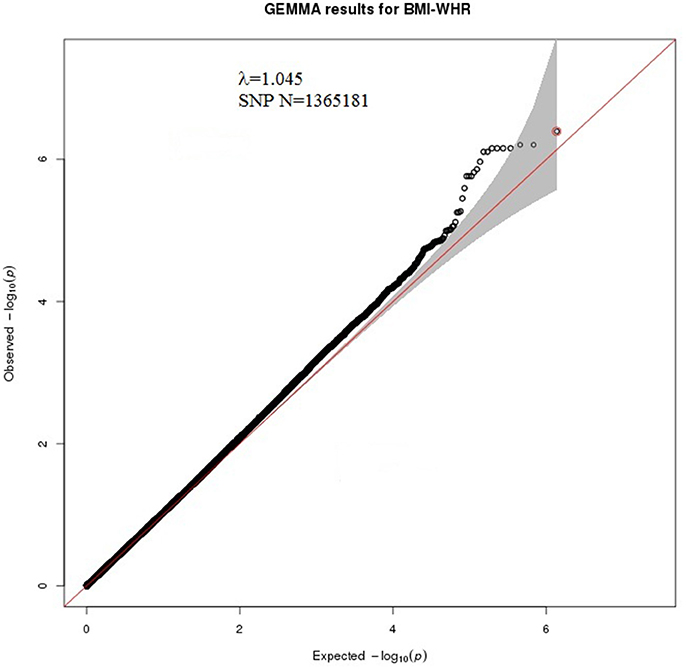
Figure 2. Quantile-quantile plot of χ2 test statistics for 1,365,181 SNPs from the bivariate GWA analysis of BMI-WHR, genomic inflation λ = 1.045. The x-axis indicates the expected-log10 P-values under the null hypothesis. The y-axis shows the observed-log10 P-values calculated by a bivariate linear mixed model. The red line represents y = x, which corresponds to the null hypothesis of no association. The red dot is the SNP with lowest P-value (rs2025924). The gray shaded area shows 95% confidence intervals of the null hypothesis.
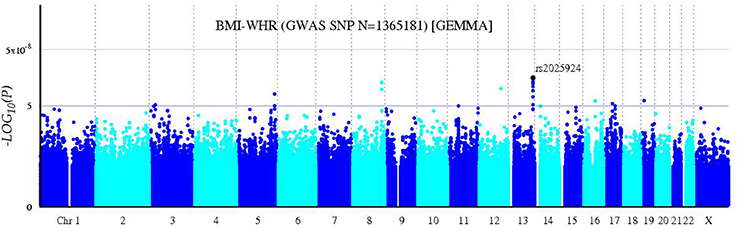
Figure 3. Bivariate Manhattan plot of BMI-WHR. The x-axis represents chromosomal positions and the y-axis represents –log10 P-values calculated by a bivariate linear mixed model. The blue and red horizontal lines indicate the suggestive (P = 1 × 10−5) and genome-wide (P = 5 × 10−8) significance levels, respectively.
Gene-Based Analysis
Results of gene-based analysis using VEGAS2 were reported in Supplementary Table 1. While no gene achieved genome-wide significance, a total of 291 genes were found to be nominally associated with BMI-WHR (P < 0.05), and we reported the top 20 genes ranked by their P-value in Table 3.
GSEA
Gene set enrichment analysis with 291 significant genes using GSEA reported 6 biological pathways (Table 4). In these 6 pathways, there were 5 REACTOME pathways which are involved in hemostasis, G protein-coupled receptor (GPCR) signaling, platelet production, GPCR ligand binding, and gastrin-CREB signaling. The only KEGG pathway was the olfactory transduction pathway.
eQTL Analysis
HaploReg presented all of the SNPs that are in LD with our 26 queried SNPs. Among them, rs2242044 from our GWAS and 2 other variants (rs1868910 and rs10838458) with r2 > 0.8 were identified as significant eQTLs in both the adipose-subcutaneous and adipose-visceral tissues. GTEx Portal showed that P-values of rs2242044 were 1.7 × 10−9 and 4.4 × 10−15 for normal adipose-subcutaneous and adipose-visceral tissue, respectively. We also queried our top 20 genes from VEGAS2 using the GTEx Portal, and it identified that 13 of them harbored statistically significant cis-eQTLs in at least one relevant tissue (Supplementary Table 2).
Discussion
This twin study documented four major findings. First, the Cholesky heritability model in 242 MZ and 140 DZ twin pairs suggested an important genetic component in the phenotypic correlation between BMI and WHR. Second, bivariate GWAS in 139 pairs of DZ twins and further gene-based analysis revealed several associated SNPs and gene-based loci supported by some previous reports. Third, GSEA identified pathways involved in hemostasis, platelet production, GPCR signaling, Gastrin-CREB signaling and aolfactory transduction, all of which were associated with BMI-WHR. Fourth, after querying suggestively significant SNPs, or SNPs in high LD with them by using HaploReg and GTEx Portal, we determined that rs2242044 was a significant cis-eQTL in both the normal adipose-subcutaneous and adipose-visceral tissue.
Our estimate of a moderate genetic correlation between BMI and WHR was similar to previous works in other populations (Li et al., 2006; Zabaneh et al., 2009) and it confirmed the necessity for further bivariate GWA analysis for discovering relevant pleiotropic gene variants. The current GWAS identified 26 SNPs with genome-wide-suggestive P-value cutoff 1 × 10−5 (Table 2). Most of them were near to the RNA genes that not yet characterized and/or their neighboring protein-coding genes that have not been previously identified to be associated with obesity. However, a connection between rs79817709 and obesity is biologically plausible. SNP rs79817709 lies in 3′UTR (utr variant 3 prime) of KEAP1 gene. KEAP1 protein is an inhibitor of nuclear factor (erythroid-derived 2)-like 2 (Nrf2) by blocking Nrf2 translocation to the nucleus and also promoting its degradation, and lack of Nrf2 can ameliorate insulin resistance, adipogenesis and adipocyte differentiation (Pi et al., 2010; Zhang et al., 2015).
The gene-based analysis focused on several associated chromosome regions, including 5q35.3, 17q11.2, 4p15.32, 1p35.2 and so on (Table 3). Our findings were supported by previous reports on the linkage of chromosome 5q35 to obesity-related phenotypes in Caucasians population (Zhao et al., 2007; He et al., 2008), the linkage of 1p35.2 to plasma adiponectin concentrations in Genetics of Lipid Lowering Drugs and Diet Network Study (Rasmussen-Torvik et al., 2009), as well as the linkage of 4p15.3 to hip circumference in post-menopausal women from a United States mid-western population (Kelemen et al., 2010). Although there was no direct evidence that the chromosome 17q11.2 region was linked to obesity traits, a case report should be noted that a 3-year-old girl with a microduplication at 17q11.2 have developed obesity within the first 6 months of life (White et al., 2013).
F12, our second top gene from VEGAS2 results (Table 3), encodes coagulation factor XII which circulates in blood as a zymogen. It has been showed that the activity of coagulation factor XII increased in the obese individual and has strong correlation with the measures of adiposity and insulin resistance in a number of observational studies (Bowles et al., 2003; Kotronen et al., 2011). The twelfth top gene, PHOSPHO1, is involved in the pathways of metabolism and glycerophospholipid biosynthesis. A previous study found that DNA methylation at the PHOSPHO1 was associated with type 2 diabetes (Chambers et al., 2015) and positively correlated with high density lipoprotein levels (Dayeh et al., 2016). HCRTR1, the twentieth top gene, encodes hypocretin receptor type 1, a protein belonging to the G-protein coupled receptor family. This protein binds with neuropeptide orexin A, and orexin neurons provide a critical link between peripheral energy balance and the central mechanisms that coordinate sleep/wakefulness and motivate behaviors such as food seeking (Kaewsutthi et al., 2016).
The results of GSEA indicated that 34 (Table 4) of the 291 significant genes (Supplementary Table 1) overlapped with 6 biological pathways. Apart from the aforementioned F12 and HCRTR1 genes, several other genes should be also noted, i.e., DOCK2, DOCK6, DGKB, GLP1R, TRHR, MMP1, GPR55, CCK, and OR2AK2. DOCK2 was the only gene identified both by our GWAS and GSEA. Dedicator of cytokinesis 2 (DOCK2) is involved in several inflammatory diseases. A recent experimental study indicated that DOCK2 deficiency might protect mice from high-fat-diet induced obesity by reducing adipose tissue inflammation and increasing energy expenditure (Guo et al., 2017). Our findings on the role of DOCK6 and DGKB in BMI-WHR were supported by two meta-analyses respectively which identified genome-wide significant signals near DOCK6 for total cholesterol in Hispanics (Below et al., 2016) and near DGKB for beta cell function in East Asians (Hong et al., 2014).
GLP1R gene encodes a 7-transmembrane protein that functions as a receptor for glucagon-like peptide 1 hormone, which stimulates glucose-induced insulin secretion. TRHR gene encodes the thyrotropin-releasing hormone receptor and regulates crucial biological functions, including the increase of basal cell metabolism, growth, thermogenesis, total energy expenditure, lipid and carbohydrate catabolism, as well as myogenesis in skeletal muscle (Costa-Urrutia et al., 2017). MMP1 gene encodes a member of the peptidase M10 family of matrix metalloproteinases which are involved in the breakdown of extracellular matrix in normal physiological processes. Previous candidate gene studies on obesity and its related traits have reported GLP1R in European Americans (Li et al., 2014), TRHR in Mexican-Mestizos (Costa-Urrutia et al., 2017) and MMP1 in Koreans (Nho et al., 2008). Our results further consolidated these associations in Northern Han Chinese.
Our observations on the significant associations of GPR55 and CCK with BMI-WHR were also supported by two interesting experimental studies: Compared to wild-type mice, GPR55-null mice exhibited significantly increased fat-mass and insulin resistance as well as decreased spontaneous locomotor activity and physical activity (Meadows et al., 2016); CCK knockout mice were resistant to high-fat diet-induced obesity (Lo et al., 2010). It suggested that these two genes might play important roles in human energy balance and metabolism. Besides, OR2AK2, a member of olfactory receptor genes family which located in chromosome 1q, involved in both GPCR signaling pathway and olfactory signaling pathway. It has been found that the predicted damaging missense variants in olfactory receptor genes on chromosome 1q and rare damaging variants in protocadherin beta-cluster genes on chromosome 5q31 co-localized in subjects with extreme obesity (Mariman et al., 2015). The functions of other genes in terms of obesity were unknown, whereas they may be interesting potential candidates for future research.
Our eQTL analysis showed that rs2242044 was a significant cis-eQTL in both the normal adipose-subcutaneous and adipose-visceral tissue. LOC399886, which is the closest gene to rs2242044, is an uncharacterized ncRNA. Whether or not rs2242044, as an eQTL, controls the expression of LOC399886 in regulating obesity requires further investigation.
We utilized a sample of adult Chinese twins with a homogenous genetic background to conduct a bivariate GWA analysis on BMI-WHR. Comparing with traditional univariate GWAS in unrelated population, this design has a stronger power in identifying genes with pleiotropic effect and may help reveal the interconnected pathophysiological networks for a spectrum of common human diseases such as obesity (Ran et al., 2013; Loukola et al., 2014). However, there are some potential limitations in our study. Firstly, in many cases, the loci identified by our GWAS harbor few, if any, annotated genes with clear connections to the biology of obesity. Our findings presented here still need to be further validated. Secondly, we couldn't replicate our bivariate GWAS results when compared with previously implicated meta-analyses with large sample sizes (Wen et al., 2012; Locke et al., 2015; Shungin et al., 2015), perhaps due to ethnic differences in genetic background and sample characteristics. Thirdly, although 13 of the top 20 genes harbored significant cis-eQTLs in adipose or muscle-skeletal tissue, it is not clear that whether the SNPs underlying the top enriched genes as well as their LD SNPs overlap with these cis-eQTLs.
In conclusion, this twin study identified several BMI-WHR jointly associated genetic loci, genomic regions and pathways that are biologically meaningful. It suggested that there are still many biological mechanisms related to obesity waiting to be discovered, and applying methods in this article on larger dataset may yields more genes and pathways that are biological meaningful.
Author Notes
The SNP data has been deposited in the European Variation Archive (EVA), (accession no. PRJEB23749).
Author Contributions
YW, ZP, DZ, and QT designed the study. HD, CX, XT, and ZP collected the data. YW, WW, WJ, and QT analyzed the data and interpreted the results. YW wrote the first version of the article. All authors reviewed and edited the manuscript and approved the final version.
Funding
This study was jointly supported by National Natural Science Foundation of China (grant number: 81773506), China Postdoctoral Science Foundation (grant number: 2016M602102) and Qingdao Postdoctoral Science Foundation (grant number: 2016064). The work of QT was supported by the Independent Research Fund Denmark (grant number DFF-6110-00114).
Conflict of Interest Statement
The authors declare that the research was conducted in the absence of any commercial or financial relationships that could be construed as a potential conflict of interest.
The reviewer LS and handling Editor declared their shared affiliation.
Acknowledgments
The authors are grateful to Dr. Gu Zhu for his excellent technical help. The authors thank Weilong Li for his valuable suggestions in the writing of manuscript.
Supplementary Material
The Supplementary Material for this article can be found online at: https://www.frontiersin.org/articles/10.3389/fgene.2018.00179/full#supplementary-material
References
Allison, D. B., Thiel, B., St Jean, P., Elston, R. C., Infante, M. C., and Schork, N. J. (1998). Multiple phenotype modeling in gene-mapping studies of quantitative traits: power advantages. Am. J. Hum. Genet. 63, 1190–1201. doi: 10.1086/302038
Below, J. E., Parra, E. J., Gamazon, E. R., Torres, J., Krithika, S., Candille, S., et al. (2016). Meta-analysis of lipid-traits in Hispanics identifies novel loci, population-specific effects, and tissue-specific enrichment of eQTLs. Sci. Rep. 6:19429. doi: 10.1038/srep19429
Benyamin, B., Visscher, P. M., and McRae, A. F. (2009). Family-based genome-wide association studies. Pharmacogenomics 10, 181–190. doi: 10.2217/14622416.10.2.181
Bowles, L. K., Cooper, J. A., Howarth, D. J., Miller, G. J., and MacCallum, P. K. (2003). Associations of haemostatic variables with body mass index: a community-based study. Blood Coagul. Fibrinol. 14, 569–573. doi: 10.1097/00001721-200309000-00009
Chambers, J. C., Loh, M., Lehne, B., Drong, A., Kriebel, J., Motta, V., et al. (2015). Epigenome-wide association of DNA methylation markers in peripheral blood from Indian Asians and Europeans with incident type 2 diabetes: a nested case-control study. Lancet Diabetes Endocrinol. 3, 526–534. doi: 10.1016/S2213-8587(15)00127-8
Consortium, G. T. (2013). The genotype-tissue expression (GTEx) project. Nat. Genet. 45, 580–585. doi: 10.1038/ng.2653
Costa-Urrutia, P., Abud, C., Franco-Trecu, V., Colistro, V., Rodríguez-Arellano, M. E., Vázquez-Pérez, J., et al. (2017). Genetic obesity risk and attenuation effect of physical fitness in mexican-mestizo population: a case-control study. Ann. Hum. Genet. 81, 106–116. doi: 10.1111/ahg.12190
Dayeh, T., Tuomi, T., Almgren, P., Perfilyev, A., Jansson, P. A., de Mello, V. D., et al. (2016). DNA methylation of loci within ABCG1 and PHOSPHO1 in blood DNA is associated with future type 2 diabetes risk. Epigenetics 11, 482–488. doi: 10.1080/15592294.2016.1178418
Duan, H., Ning, F., Zhang, D., Wang, S., Zhang, D., Tan, Q., et al. (2013). The qingdao twin registry: a status update. Twin Res. Hum. Genet. 16, 79–85. doi: 10.1017/thg.2012.113
Duan, H., Pang, Z., Zhang, D., Li, S., Kruse, T. A., Kyvik, K. O., et al. (2011). Genetic and environmental dissections of sub-phenotypes of metabolic syndrome in the Chinese population: a twin-based heritability study. Obes. Facts 4, 99–104. doi: 10.1159/000327735
Fall, T., and Ingelsson, E. (2014). Genome-wide association studies of obesity and metabolic syndrome. Mol. Cell. Endocrinol. 382, 740–757. doi: 10.1016/j.mce.2012.08.018
Guo, X., Li, F., Xu, Z., Yin, A., Yin, H., Li, C., et al. (2017). DOCK2 deficiency mitigates HFD-induced obesity by reducing adipose tissue inflammation and increasing energy expenditure. J. Lipid Res. 58, 1777–1784. doi: 10.1194/jlr.M073049
Hao, J., Wang, W., Wen, Y., Xiao, X., He, A., Guo, X., et al. (2016). A bivariate genome-wide association study identifies ADAM12 as a novel susceptibility gene for Kashin-Beck disease. Sci. Rep. 6:31792. doi: 10.1038/srep31792
He, L. N., Liu, Y. J., Xiao, P., Zhang, L., Guo, Y., Yang, T. L., et al. (2008). Genomewide linkage scan for combined obesity phenotypes using principal component analysis. Ann. Hum. Genet. 72, 319–326. doi: 10.1111/j.1469-1809.2007.00423.x
Hong, K. W., Chung, M., and Cho, S. B. (2014). Meta-analysis of genome-wide association study of homeostasis model assessment beta cell function and insulin resistance in an East Asian population and the European results. Mol. Genet. Genomics 289, 1247–1255. doi: 10.1007/s00438-014-0885-6
Kaewsutthi, S., Santiprabhob, J., Phonrat, B., Tungtrongchitr, A., Lertrit, P., and Tungtrongchitr, R. (2016). Exome sequencing in Thai patients with familial obesity. Genet. Mol. Res. 15. doi: 10.4238/gmr.15028311
Kelemen, L. E., Atkinson, E. J., de Andrade, M., Pankratz, V. S., Cunningham, J. M., Wang, A., et al. (2010). Linkage analysis of obesity phenotypes in pre- and post-menopausal women from a United States mid-western population. BMC Med. Genet. 11:156. doi: 10.1186/1471-2350-11-156
Kotronen, A., Joutsi-Korhonen, L., Sevastianova, K., Bergholm, R., Hakkarainen, A., Piätilainen, K. H., et al. (2011). Increased coagulation factor VIII, IX, XI and XII activities in non-alcoholic fatty liver disease. Liver Int. 31, 176–183. doi: 10.1111/j.1478-3231.2010.02375.x
Li, P., Tiwari, H. K., Lin, W. Y., Allison, D. B., Chung, W. K., Leibel, R. L., et al. (2014). Genetic association analysis of 30 genes related to obesity in a European American population. Int. J. Obes. 38, 724–729. doi: 10.1038/ijo.2013.140
Li, X., Quiñones, M. J., Wang, D., Bulnes-Enriquez, I., Jimenez, X., De La Rosa, R., et al. (2006). Genetic effects on obesity assessed by bivariate genome scan: the Mexican-American coronary artery disease study. Obesity 14, 1192–1200. doi: 10.1038/oby.2006.136
Lo, C. M., King, A., Samuelson, L. C., Kindel, T. L., Rider, T., Jandacek, R. J., et al. (2010). Cholecystokinin knockout mice are resistant to high-fat diet-induced obesity. Gastroenterology 138, 1997–2005. doi: 10.1053/j.gastro.2010.01.044
Locke, A. E., Kahali, B., Berndt, S. I., Justice, A. E., Pers, T. H., Day, F. R., et al. (2015). Genetic studies of body mass index yield new insights for obesity biology. Nature 518, 197–206. doi: 10.1038/nature14177
Loo, L. W. M., Lemire, M., and Le Marchand, L. (2017). In silico pathway analysis and tissue specific cis-eQTL for colorectal cancer GWAS risk variants. BMC Genomics 18:381. doi: 10.1186/s12864-017-3750-2
Loukola, A., Wedenoja, J., Keskitalo-Vuokko, K., Broms, U., Korhonen, T., Ripatti, S., et al. (2014). Genome-wide association study on detailed profiles of smoking behavior and nicotine dependence in a twin sample. Mol. Psychiatry 19, 615–624. doi: 10.1038/mp.2013.72
Lu, S., Zhao, L. J., Chen, X. D., Papasian, C. J., Wu, K. H., Tan, L. J., et al. (2016). Bivariate genome-wide association analyses identified genetic pleiotropic effects for bone mineral density and alcohol drinking in Caucasians. J. Bone Miner. Metab. 35, 649–658. doi: 10.1007/s00774-016-0802-7
Mariman, E. C., Szklarczyk, R., Bouwman, F. G., Aller, E. E., van Baak, M. A., and Wang, P. (2015). Olfactory receptor genes cooperate with protocadherin genes in human extreme obesity. Genes Nutr. 10:465. doi: 10.1007/s12263-015-0465-3
Meadows, A., Lee, J. H., Wu, C. S., Wei, Q., Pradhan, G., Yafi, M., et al. (2016). Deletion of G-protein-coupled receptor 55 promotes obesity by reducing physical activity. Int. J. Obes. 40, 417–424. doi: 10.1038/ijo.2015.209
Melton, P. E., Rutherford, S., Voruganti, V. S., Goring, H. H., Laston, S., Haack, K., et al. (2010). Bivariate genetic association of KIAA 1797 with heart rate in american indians: the strong heart family study. Hum. Mol. Genet. 19, 3662–3671. doi: 10.1093/hmg/ddq274
Nho, Y. K., Ha, E., Yu, K. I., Chung, J. H., Wook, N. C., Chung, I. S., et al. (2008). Matrix metalloproteinase-1 promoter is associated with body mass index in Korean population with aged greater or equal to 50 years. Clin. Chim. Acta 396, 14–17. doi: 10.1016/j.cca.2008.06.012
Pang, Z., Ning, F., Unger, J., Johnson, C. A., Wang, S., Guo, Q., et al. (2006). The qingdao twin registry: a focus on chronic disease research. Twin Res. Hum. Genet. 9, 758–762. doi: 10.1375/twin.9.6.758
Pi, J., Leung, L., Xue, P., Wang, W., Hou, Y., Liu, D., et al. (2010). Deficiency in the nuclear factor E2-related factor-2 transcription factor results in impaired adipogenesis and protects against diet-induced obesity. J. Biol. Chem. 285, 9292–9300. doi: 10.1074/jbc.M109.093955
Ran, S., Pei, Y. F., Liu, Y. J., Zhang, L., Han, Y. Y., Hai, R., et al. (2013). Bivariate genome-wide association analyses identified genes with pleiotropic effects for femoral neck bone geometry and age at menarche. PLoS ONE 8:e60362. doi: 10.1371/journal.pone.0060362
Rasmussen-Torvik, L. J., Pankow, J. S., Peacock, J. M., Borecki, I. B., Hixson, J. E., Tsai, M. Y., et al. (2009). Suggestion for linkage of chromosome 1p35.2 and 3q28 to plasma adiponectin concentrations in the GOLDN Study. BMC Med. Genet. 10:39. doi: 10.1186/1471-2350-10-39
Rosenthal, E., Blue, E., and Jarvik, G. P. (2015). Next-generation gene discovery for variants of large impact on lipid traits. Curr. Opin. Lipidol. 26, 114–119. doi: 10.1097/MOL.0000000000000156
Sandholt, C. H., Hansen, T., and Pedersen, O. (2012). Beyond the fourth wave of genome-wide obesity association studies. Nutr. Diabetes. 2:e37. doi: 10.1038/nutd.2012.9
Shim, H., Chasman, D. I., Smith, J. D., Mora, S., Ridker, P. M., Nickerson, D. A., et al. (2015). A multivariate genome-wide association analysis of 10 LDL subfractions, and their response to statin treatment, in 1868 Caucasians. PLoS ONE 10:e0120758. doi: 10.1371/journal.pone.0120758
Shungin, D., Winkler, T. W., Croteau-Chonka, D. C., Ferreira, T., Locke, A. E., Mägi, R., et al. (2015). New genetic loci link adipose and insulin biology to body fat distribution. Nature 518, 187–196. doi: 10.1038/nature14132
Tan, Q., Christiansen, L., von Bornemann Hjelmborg, J., and Christensen, K. (2015). Twin methodology in epigenetic studies. J. Exp. Biol. 218, 134–139. doi: 10.1242/jeb.107151
Visscher, P. M., Andrew, T., and Nyholt, D. R. (2008). Genome-wide association studies of quantitative traits with related individuals: little (power) lost but much to be gained. Eur. J. Hum. Genet. 16, 387–390. doi: 10.1038/sj.ejhg.5201990
Wen, W., Cho, Y. S., Zheng, W., Dorajoo, R., Kato, N., Qi, L., et al. (2012). Meta-analysis identifies common variants associated with body mass index in east Asians. Nat. Genet. 44, 307–311. doi: 10.1038/ng.1087
White, M., Conroy, J., Bullman, H., Lever, M., Daly, E., Betts, D. R., et al. (2013). Duplication of 17q11.2 and features of albright hereditary osteodystrophy secondary to methylation defects within the GNAS cluster: coincidence or causal? Case Rep. Genet. 2013:764152. doi: 10.1155/2013/764152
Wu, T., Snieder, H., Li, L., Cao, W., Zhan, S., Lv, J., et al. (2011). Genetic and environmental influences on blood pressure and body mass index in Han Chinese: a twin study. Hypertens. Res. 34, 173–179. doi: 10.1038/hr.2010.194
Wu, Y., Zhang, D., Pang, Z., Jiang, W., Wang, S., Li, S., et al. (2015). Multivariate modeling of body mass index, pulse pressure, systolic and diastolic blood pressure in Chinese twins. Twin Res. Hum. Genet. 18, 73–78. doi: 10.1017/thg.2014.83
Xu, C., Zhang, D., Wu, Y., Tian, X., Pang, Z., Li, S., et al. (2017). A genome-wide association study of cognitive function in Chinese adult twins. Biogerontology 18, 811–819. doi: 10.1007/s10522-017-9725-5
Zabaneh, D., Chambers, J. C., Elliott, P., Scott, J., Balding, D. J., and Kooner, J. S. (2009). Heritability and genetic correlations of insulin resistance and component phenotypes in Asian Indian families using a multivariate analysis. Diabetologia 52, 2585–2589. doi: 10.1007/s00125-009-1504-7
Zhang, Z., Zhou, S., Jiang, X., Wang, Y. H., Li, F., Wang, Y. G., et al. (2015). The role of the Nrf2/Keap1 pathway in obesity and metabolic syndrome. Rev. Endocr. Metab. Disord. 16, 35–45. doi: 10.1007/s11154-014-9305-9
Zhao, L. J., Xiao, P., Liu, Y. J., Xiong, D. H., Shen, H., Recker, R. R., et al. (2007). A genome-wide linkage scan for quantitative trait loci underlying obesity related phenotypes in 434 Caucasian families. Hum. Genet. 121, 145–148. doi: 10.1007/s00439-006-0286-y
Zheng, Y., Huang, W. Y., Zhang, J., and He, M. G. (2013). Phenotypic and genetic correlation of blood pressure and body mass index with retinal vascular caliber in children and adolescents: the guangzhou twin eye study. Invest. Ophthalmol. Vis. Sci. 54, 423–428. doi: 10.1167/iovs.12-9543
Keywords: obesity, signaling by G protein-coupled receptor, olfactory transduction, bivariate genome-wide association study, twin study
Citation: Wu Y, Duan H, Tian X, Xu C, Wang W, Jiang W, Pang Z, Zhang D and Tan Q (2018) Genetics of Obesity Traits: A Bivariate Genome-Wide Association Analysis. Front. Genet. 9:179. doi: 10.3389/fgene.2018.00179
Received: 19 October 2017; Accepted: 30 April 2018;
Published: 16 May 2018.
Edited by:
William Scott Bush, Case Western Reserve University, United StatesReviewed by:
Larry Parnell, Jean Mayer USDA Human Nutrition Research Center on Aging at Tufts University, United StatesLindsay Sausville, Case Western Reserve University, United States
Copyright © 2018 Wu, Duan, Tian, Xu, Wang, Jiang, Pang, Zhang and Tan. This is an open-access article distributed under the terms of the Creative Commons Attribution License (CC BY). The use, distribution or reproduction in other forums is permitted, provided the original author(s) and the copyright owner are credited and that the original publication in this journal is cited, in accordance with accepted academic practice. No use, distribution or reproduction is permitted which does not comply with these terms.
*Correspondence: Yili Wu, eWlsaXd1NzlAMTYzLmNvbQ==