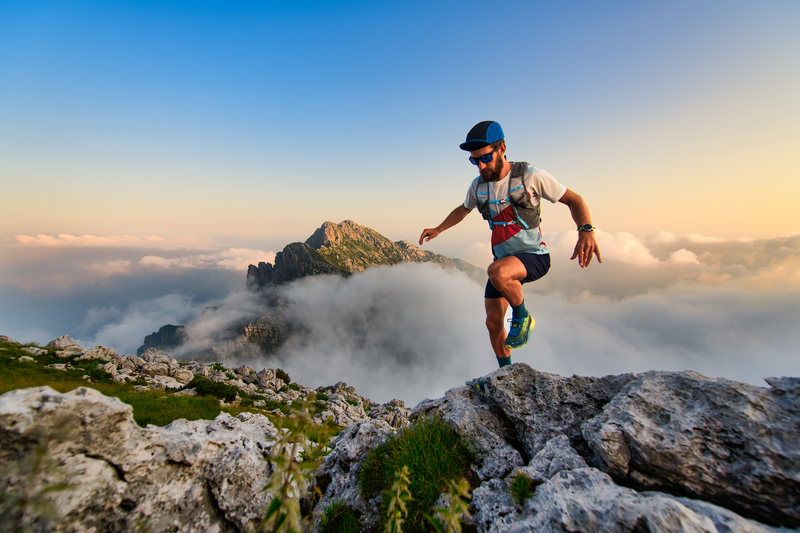
95% of researchers rate our articles as excellent or good
Learn more about the work of our research integrity team to safeguard the quality of each article we publish.
Find out more
ORIGINAL RESEARCH article
Front. Genet. , 04 February 2020
Sec. Computational Genomics
Volume 11 - 2020 | https://doi.org/10.3389/fgene.2020.00017
This article is part of the Research Topic Advanced Interpretable Machine Learning Methods for Clinical NGS Big Data of Complex Hereditary Diseases View all 24 articles
Lung cancer is a common malignant cancer. Kirsten rat sarcoma oncogene (KRAS) mutations have been considered as a key driver for lung cancers. KRAS p.G12C mutations were most predominant in NSCLC which was comprised about 11–16% of lung adenocarcinomas (p.G12C accounts for 45–50% of mutant KRAS). But it is still not clear how the KRAS mutation triggers lung cancers. To study the molecular mechanisms of KRAS mutation in lung cancer. We analyzed the gene expression profiles of 156 KRAS mutation samples and other negative samples with two stage feature selection approach: (1) minimal Redundancy Maximal Relevance (mRMR) and (2) Incremental Feature Selection (IFS). At last, 41 predictive genes for KRAS mutation were identified and a KRAS mutation predictor was constructed. Its leave one out cross validation MCC was 0.879. Our results were helpful for understanding the roles of KRAS mutation in lung cancer.
Lung cancer, known as a malignant cancer which defined as the overgrowth of uncontrolled cell in lung tissues, has proved be a key cause of cancer death. Each year, 1.3 million people die of lung cancer (Jemal et al., 2006; Jemal et al., 2011). Non-small-cell lung cancer (NSCLC) accounts for more than 85% of diagnosed lung cancer patients (Morgensztern et al., 2010). NSCLC can be further divided into adenocarcinoma, squamous cell carcinoma (SCC), and large cell carcinoma (Sandler et al., 2006; Morgensztern et al., 2010).
At present, the pathogenesis of lung cancer is not very clear, but is generally believed that one of the most important reason is the accumulation of mutations including single nucleotide transformation, small fragments of insertions and deletions, the changes of copy number, and chromosome rearrangement. Moreover, these mutations are closed with cell proliferation, invasion, metastasis, and apoptosis (Scagliotti et al., 2008; Liu et al., 2012). So, studying mutations in living systems will be helpful to understand how mutations are associated with lung-cancer biological processes.
In the last decade, researchers have uncovered the source of one of the important mutations is called as Kirsten rat sarcoma oncogene (KRAS) mutations in lung cancers using molecular studies (Gautschi et al., 2007). KRAS is the principal isoform of RAS. KRAS p.G12C mutations were most predominant in NSCLC which was comprised about 11–16% of lung adenocarcinomas (p.G12C accounts for 45–50% of mutant KRAS) (Cox et al., 2014). Other common KRAS mutations in lung cancer are G12V and G12D. In other cancers, such as pancreatic cancer and colorectal cancer, KRAS mutations are also frequent. Based on the TCGA data in cBioPortal (Gao et al., 2013), the most frequent KRAS mutations in pancreatic cancer are G12D, G12V, and G12R; the most frequent KRAS mutations in colorectal cancer are G12D, G12V, and G13D. KRAS may be a good lung cancer therapeutic target for searching potential drugs.
As above mentioned, mutations in KRAS is the most usual mutations that occur in lung cancer, especially in NSCLC (Mao et al., 1994; Mills et al., 1995; Nakamoto et al., 2001). KRAS mutation is more frequent in Caucasians than in Asians. Moreover, smokers may have more KRAS mutations than nonsmokers (Westcott and To, 2013; Ferrer et al., 2018). Single amino acid substitutions in codon 12 were most common KRAS mutations in NSCLC (Graziano et al., 1999). Therefore, the search for how the KRAS mutations affected the gene in lung cancer has been a long-standing goal in cancer biology.
In this study, to study the functional effects of key driver KRAS mutations on gene expression in lung cancer, we analyzed the gene expression profiles of 156 lung cancer cell lines with KRAS mutations and other 3,582 lung cancer cell lines without KRAS mutations. Forty-one discriminative genes for KRAS mutations were identified using two stage feature selection approach: (1) minimal Redundancy Maximal Relevance (mRMR) and (2) Incremental Feature Selection (IFS).
To identify the key genes that distinguishes key driver KRAS mutations from other mutations, we downloaded the gene expression profiles of 156 lung cancer cell lines with KRAS mutations as positive samples and other 3,582 lung cancer cell lines without KRAS mutations as negative samples from publicly available Gene Expression Omnibus (GEO) database under accession number of GSE83744 (Berger et al., 2016). The expression levels of 978 representative genes from Broad Institute Human L1000 landmark were measured. The L1000 landmark was derived from the Connectivity Map (CMap) project (Subramanian et al., 2017). CMap is a large gene-expression dataset of human cells perturbed with many chemicals and genetic reagents (Lamb et al., 2006). These 1,000 genes were sensitive to perturbations and can reflect 81% of non-measured transcripts (Subramanian et al., 2017).
We applied two stage feature selection approach to select the biomarker genes. First, the genes were ranked based on not only their relevance with mutation samples, but also their redundancy among genes using the mRMR algorithm (Peng et al., 2005). It had a wide range of applications in bioinformatics for feature selection (Chen et al., 2018c; Chen et al., 2019e; Li and Huang, 2018; Li et al., 2019b; Wang and Huang, 2019a). As the equation shown below, Ωs, Ωt and Ω were the set of m selected genes, n to-be-selected genes, and all m+n genes, respectively. We use mutual information (I) to measure the relevance of the expression levels of gene g from Ωt with KRAS mutation status t (Huang and Cai, 2013):/>
Meanwhile, the redundancy R of the gene g with the selected genes in Ωs can be calculated as below:
The optimal gene gj from Ωt with max relevance with KRAS mutation status t and min redundancy with the selected genes in Ωs can be selected by maximizing mRMR function listed below
With N round evaluations, genes can be ranked as
The top ranked genes were associated with KRAS mutation status, and had little redundancy with other genes. Such genes were suitable for biomarkers. The top 200 genes were further analyzed at the second stage.
The second stage was to determine the number of selected genes using the IFS method (Chen et al., 2018b; Chen et al., 2019b; Chen et al., 2019c; Chen et al., 2019d; Chen et al., 2019f; Li et al., 2019a; Pan et al., 2019a; Pan et al., 2019b; ). To do so, 200 classifiers were constructed using top 1, top 2, top 200 genes. The LOOCV (leave-one-out cross validation) MCC (Mathew’s correlation coefficient) of the top k-gene classifier was calculated each time.
We tried several different classifiers: (1) SVM (Support Vector Machine) (Jiang et al., 2019; Yan et al., 2019; Chen et al., 2019a; Li et al., 2019a; Pan et al., 2019a; Wang and Huang, 2019b; Chen et al., 2019d), (2) 1NN (1 Nearest Neighbor) (Lei et al., 2013; Chen et al., 2016; Wang et al., 2017a), (3) 3NN (3 Nearest Neighbors), (4) 5NN (5 Nearest Neighbors), (5) Decision Tree (DT) (Huang et al., 2008; Huang et al., 2011; Chen et al., 2015), (6) Neural Network (NN) (Liu et al., 2017; Pan et al., 2018; Chen et al., 2019e). The function svm from R package e1071, function knn from R package class, function rpart from R package rpart, function nnet from R package nnet were used to apply these classification algorithms.
Based on the IFS curve in which x-axis was the number of genes and y-axis was the corresponding LOOCV MCC, we can decide the best gene combinations we should select. The peak of the curve was the optimal selection.
As we mentioned before, the prediction performance of each classifier was evaluated with leave-one-out cross validation (LOOCV) (Cui et al., 2013; Yang et al., 2014). It will go through N rounds and each sample will be tested during the N rounds. In each round, one sample will be tested using the model trained with the other N-1 samples. It can objectively evaluate all samples (Chou, 2011).
The performance metrics, including Sensitivity (Sn), Specificity (Sp), Accuracy (ACC), and Mathew’s correlation coefficient (MCC) were all calculated:
where TP, TN, FP, and FN stand for the number of true positive samples, true negative samples, false positive samples, and false negative samples, respectively. Since the sizes of KRAS mutation + samples and KRAS mutation - samples were imbalance and MCC can trade-off sensitivity and specificity (Chen et al., 2018a; Li et al., 2018; Pan et al., 2018; Pan et al., 2019a; Pan et al., 2019b), MCC was used as the main performance metric.
The top 200 most informative genes for KRAS mutations were identified using the mRMR method which has been widely used in bioinformatics filed (Zhao et al., 2013; Zhang et al., 2016). The C/C++ version software written by Peng et al. (Peng et al., 2005; Best et al., 2017) (http://home.penglab.com/proj/mRMR/) was used to apply the mRMR algorithm. Unlike the traditional statistical test based univariate feature selection methods, mRMR considers the relevance between gene expression and KRAS mutation status, and the redundancy among genes.
After genes were ranked by mRMR, the IFS procedure was applied to find the optimal number of genes to be selected. The IFS curve in Figure 1 showed the relationship between the number of genes and their MCCs. The peak LOOCV MCCs of SVM, 1NN, 3NN, 5NN, DT, and NN were 0.858 with 8 genes, 0.853 with 48 genes, 0.879 with 41 genes, 0.878 with 59 genes, 0.871 with 69 genes, 0.842 with 174 genes. 3NN performed best. The corresponding 41 genes were shown in Table 1.
Figure 1 The IFS curves of six different classifiers. The x-axis was the number of genes and the y-axis was the then leave one out cross validation (LOOCV) MCC. The red, blue, brown, black, orange, and purple curves were the IFS results of SVM, 1NN, 3NN, 5NN, DT, and NN, respectively. Peak LOOCV MCCs of SVM, 1NN, 3NN, 5NN, DT, and NN were 0.858 with 8 genes, 0.853 with 48 genes, 0.879 with 41 genes, 0.878 with 59 genes, 0.871 with 69 genes, 0.842 with 174 genes. 3NN performed best. Therefore, the corresponding 41 genes were finally selected.
The 41 genes were chosen with two stage feature selection methods: mRMR and IFS. To more carefully evaluate their prediction power, we checked their confusion matrix which showed the overlaps between actual KRAS mutation status and predicted KRAS mutation status using 3NN (Table 2). The LOOCV sensitivity, specificity, accuracy, and MCC were 0.840, 0.997, 0.991, and 0.879, respectively.
We searched KRAS and the eight genes in STRING database Version: 11.0 (https://string-db.org) and Figure 2 showed their functional association networks. It can be seen that 20 out of 41 genes (CCND3, CDK19, CEBPA, CEBPD, CSNK1E, CTSL, DUSP6, GRB10, HMGA2, MMP1, MTHFD2, NR3C1, PAK4, PMAIP1, RAP1GAP, SDHB, STX1A, TP53, TRIB3, UBE2L6) had direct interactions with KRAS. The STRING network results supported that most of the 41 genes had direct interactions with KRAS.
Figure 2 The functional association network of KRAS and the selected genes based on STRING database. Twenty out of 41 genes (CCND3, CDK19, CEBPA, CEBPD, CSNK1E, CTSL, DUSP6, GRB10, HMGA2, MMP1, MTHFD2, NR3C1, PAK4, PMAIP1, RAP1GAP, SDHB, STX1A, TP53, TRIB3, UBE2L6) had direct interactions with KRAS. Each line represented an interaction supported by different evidences. The skype-blue, purple, green, red, blue, grass green, black, and navy-blue edges were interactions from curated databases, experiment, gene neighborhood, gene fusions, gene co-occurrence, text mining, co-expression, and protein homology, respectively. For more detailed explanations, please refer to STRING database (https://string-db.org).
As mentioned earlier, we used mRMR algorithm and IFS program to screen out 41 genes which may be molecular markers for identifying KARS mutations. Subsequently, we reviewed studies of these genes in lung cancer and other cancers with high frequency of KARS mutations such as colorectal and pancreatic cancer. In the study of Zhang X et al., Tribbles-3 (TRIB3) pseudokinase can activate the β-catenin signal pathway, which in turn promotes the proliferation and migration of NSCLC cells (Zhang et al., 2019). In addition, blocking the activity of TRIB3 may be one of the mechanisms for the treatment of lung cancer (Ding et al., 2018). Wang X et al. have found that PAK4 is significantly associated with poor prognosis of NSCLC (Wang et al., 2016b), and LIMK1 phosphorylation mediated by it regulates the migration and invasion of NSCLC. Therefore, PAK4 may be an important prognostic indicator and a potential molecular target for treatment of NSCLC (Cai et al., 2015). HMGA2 affects apoptosis and is highly expressed in metastatic LUAD through Caspase 3/9 and Bcl-2. It is also considered to be a biomarker and potential therapeutic target for lung cancer therapy (Kumar et al., 2014; Gao et al., 2017b). A meta-analysis of lung cancer showed that metallo-proteinase 1 (MMP1)-16071G/2G polymorphism was a risk factor for lung cancer in Asians (Li et al., 2015). In addition, DUSP6 rs2279574 gene polymorphism is thought to predict the survival time of NSCLC patients after chemotherapy (Wang et al., 2016a). Cyclin D3 gene (CCND3) is a key cell cycle gene of NSCLC, which can promote the growth of LUAD (Zhang et al., 2017). Casein kinase I epsilon (CSNK1E), a circadian rhythm gene, whose genetic variation has a very significant correlation with the risk of lung cancer (Ortega and Mas-Oliva, 1986). CEPBA, can be used as a new tumor suppressor factor, Lu H et al. through clinical experiments, it was found that up-regulation of CEBPA is an effective method for the treatment of human NSCLC (Halmos et al., 2002; Lu et al., 2015). In addition, a comprehensive analysis of lung cancer genes by, Lv M shows that CEPBD may be involved in the development of lung cancer (Lv and Wang, 2015). TP53 mutation is very common in NSCLC and is considered to be a marker of poor prognosis and a prognostic indicator of lung cancer (Gao et al., 2017a; Labbe et al., 2017). Methylenetetrahydrofolate dehydrogenase 2 (MTHFD2) has redox homeostasis and can be used in the treatment of lung cancer (Nishimura et al., 2019). NR3C1 is reported to be involved in the pathways related to the biological process of lung cancer, and as a gene marker has a significant correlation with the survival of LUAD (Zhao et al., 2015; Luo et al., 2018). Cathepsin L1, as a protein was encoded by the CTSL1 gene, could reduce the cellular matrix and proteolytic cascades which resulting to promote invasion or metastatic activity (Duffy, 1996; Turk et al., 2012). Elevated expression of extracellular Cathepsin L was related with cancer progression of lung cancer cells (Okudela et al., 2016). Moreover, Cathepsin L is viewed as a downstream target of oncogenic KRAS mutations.
The above genes have not only been proved to be closely related to the prognosis, diagnosis, and treatment of lung cancer, but also have a direct interaction with KRAS. Some of the 41 selected genes have no direct interaction with KRAS, but are considered to be involved in the occurrence and development of lung cancer. RBM6 protein is located at 3p21.3, and its expression changes regulate many of the most common abnormal splicing events in lung cancer (Sutherland et al., 2010; Coomer et al., 2019). The double up-regulation of RGS2 gene is related to the poor overall survival rate of patients with lung adenocarcinoma (Yin et al., 2016). Epigenetic silencing of BAMBI has been identified as a marker of NSCLC, and overexpression of BAMBI may become a new target for the treatment of this cancer (Marwitz et al., 2016; Wang et al., 2017b). Overexpression of PAFA-H1B1 can lead to the occurrence and poor prognosis of lung cancer (Lo et al., 2012). Collagen alpha-1(IV) chain (COL4A1), encoded by the COL4A1 gene, was found previously to play a crucial role in the coordinating alveolar morphogenesis and formatting the epithelium vasculature lung tissue (Abe et al., 2017).
KRAS related genes are likely to be diagnostic, prognostic markers and therapeutic targets of lung cancer. We also looked for studies of these genes and KRAS high-frequency mutations in other cancers, mainly in colorectal and pancreatic cancer. According to Hua F et al., TRIB 3 gene knockout can reduce the occurrence of colon tumors in mice, reduce the migration of colorectal cancer cells, and reduce their growth in mouse transplanted tumors. The strategy of blocking the activity of TRIB3 can be used to treat colorectal cancer (Hua et al., 2019). Tyagi N et al. have found that PAK4 can maintain the stem cell phenotype of pancreatic cancer cells by activating STAT3 signal, which can be used as a new therapeutic target (Tyagi et al., 2016). TP53 mutation is associated with early stage of colorectal cancer (Laurent et al., 2011). There was a significant correlation between MMP1 and colon cancer mortality (Slattery and Lundgreen, 2014).
We downloaded the blood gene expression profiles of 156 KRAS mutations as positive samples and other 3582 mutations as negative samples from publicly available GEO (Gene Expression Omnibus) under accession number of GSE83744.
JZha conceived and designed the study. HH and SX performed data analysis. HJ wrote the paper. JZhu, EC and ZH reviewed and edited the manuscript. JZha approved final version of the manuscript. All authors read and approved the manuscript.
This study was supported by the Funds from Science Technology Department of Zhejiang Province (LGF19H010010), Medical and Health Research Foundation of Zhejiang Province (2016ZDB005, 2017ZD020), China, WU JIEPING MEDICAL foundation (320.6750.19092-12), Beijing Xisike Clinical Oncology Research Foundation (Y-HS2017-037) and Medical Health and Scientific Technology Project of Zhejiang Province (2019RC182).
The authors declare that the research was conducted in the absence of any commercial or financial relationships that could be construed as a potential conflict of interest.
Abe, Y., Matsuduka, A., Okanari, K., Miyahara, H., Kato, M., Miyatake, S., et al. (2017). A severe pulmonary complication in a patient with COL4A1-related disorder: a case report. Eur. J. Med. Genet. 60 (3), 169–171. doi: 10.1016/j.ejmg.2016.12.008
Berger, A. H., Brooks, A. N., Wu, X., Shrestha, Y., Chouinard, C., Piccioni, F., et al. (2016). High-throughput phenotyping of lung cancer somatic mutations. Cancer Cell 30 (2), 214–228. doi: 10.1016/j.ccell.2016.06.022
Best, M. G., Sol, N., In ‘t Veld, S., Vancura, A., Muller, M., Niemeijer, A. N., et al. (2017). Swarm intelligence-enhanced detection of non-small-cell lung cancer using tumor-educated platelets. Cancer Cell 32 (2), 238–252.e239. doi: 10.1016/j.ccell.2017.07.004
Cai, S., Ye, Z., Wang, X., Pan, Y., Weng, Y., Lao, S., et al. (2015). Overexpression of P21-activated kinase 4 is associated with poor prognosis in non-small cell lung cancer and promotes migration and invasion. J. Exp. Clin. Cancer Res. 34, 48. doi: 10.1186/s13046-015-0165-2
Chen, L., Chu, C., Huang, T., Kong, X., Cai, Y. D. (2015). Prediction and analysis of cell-penetrating peptides using pseudo-amino acid composition and random forest models. Amino Acids 47 (7), 1485–1493. doi: 10.1007/s00726-015-1974-5
Chen, L., Zhang, Y. H., Huang, T., Cai, Y. D. (2016). Gene expression profiling gut microbiota in different races of humans. Sci. Rep. 6, 23075. doi: 10.1038/srep23075
Chen, L., Li, J., Zhang, Y. H., Feng, K., Wang, S., Zhang, Y., et al. (2018a). Identification of gene expression signatures across different types of neural stem cells with the Monte-Carlo feature selection method. J. Cell Biochem. 119 (4), 3394–3403. doi: 10.1002/jcb.26507
Chen, L., Zhang, Y.-H., Pan, X., Liu, M., Wang, S., Huang, T., et al. (2018b). Tissue Expression difference between mRNAs and lncRNAs. Int. J. Mol. Sci. 19 (11), 3416. doi: 10.3390/ijms19113416
Chen, L., Zhang, Y. H., Huang, G., Pan, X., Wang, S., Huang, T., et al. (2018c). Discriminating cirRNAs from other lncRNAs using a hierarchical extreme learning machine (H-ELM) algorithm with feature selection. Mol. Genet. Genomics 293 (1), 137–149. doi: 10.1007/s00438-017-1372-7
Chen, L., Pan, X., Zeng, T., Zhang, Y., Huang, T., Cai, Y. (2019a). Identifying essential signature genes and expression rules associated with distinctive development stages of early embryonic cells. IEEE Access 7, 128570–128578. doi: 10.1109/ACCESS.2019.2939556
Chen, L., Pan, X., Zhang, Y.-h., Hu, X., Feng, K., Huang, T., et al. (2019b). Primary tumor site specificity is preserved in patient-derived tumor xenograft models. Front. In Genet. doi: 10.3389/fgene.2019.00738
Chen, L., Pan, X., Zhang, Y.-H., Huang, T., Cai, Y.-D. (2019c). Analysis of gene expression differences between different pancreatic cells. ACS Omega 4 (4), 6421–6435. doi: 10.1021/acsomega.8b02171
Chen, L., Pan, X., Zhang, Y.-H., Kong, X., Huang, T., Cai, Y.-D. (2019d). Tissue differences revealed by gene expression profiles of various cell lines. J. Cell. Biochem. 120 (5), 7068–7081. doi: 10.1002/jcb.27977
Chen, L., Pan, X., Zhang, Y.-H., Liu, M., Huang, T., Cai, Y.-D. (2019e). Classification of widely and rarely expressed genes with recurrent neural network. Comput. Struct. Biotechnol. J. 17, 49–60. doi: 10.1016/j.csbj.2018.12.002
Chen, L., Zhang, S., Pan, X., Hu, X., Zhang, Y. H., Yuan, F., et al. (2019f). HIV infection alters the human epigenetic landscape. Gene Ther. 26 (1-2), 29–39. doi: 10.1038/s41434-018-0051-6
Chou, K. C. (2011). Some remarks on protein attribute prediction and pseudo amino acid composition. J. Theor. Biol. 273 (1), 236–247. doi: 10.1016/j.jtbi.2010.12.024
Coomer, A. O., Black, F., Greystoke, A., Munkley, J., Elliott, D. J. (2019). Alternative splicing in lung cancer. Biochim. Biophys. Acta Gene Regul. Mech. 1862 (11-12), 194388. doi: 10.1016/j.bbagrm.2019.05.006
Cox, A. D., Fesik, S. W., Kimmelman, A. C., Luo, J., Der, C. J. (2014). Drugging the undruggable RAS: mission possible? Nat. Rev. Drug Discovery 13 (11), 828–851. doi: 10.1038/nrd4389
Cui, W., Chen, L., Huang, T., Gao, Q., Jiang, M., Zhang, N., et al. (2013). Computationally identifying virulence factors based on KEGG pathways. Mol. Biosyst. 9 (6), 1447–1452. doi: 10.1039/c3mb70024k
Ding, C. Z., Guo, X. F., Wang, G. L., Wang, H. T., Xu, G. H., Liu, Y. Y., et al. (2018). High glucose contributes to the proliferation and migration of non-small cell lung cancer cells via GAS5-TRIB3 axis. Biosci. Rep. 38 (2), BSR20171014. doi: 10.1042/BSR20171014
Duffy, M. J. (1996). PSA as a marker for prostate cancer: a critical review. Ann. Clin. Biochem. 33 (Pt 6), 511–519. doi: 10.1177/000456329603300604
Ferrer, I., Zugazagoitia, J., Herbertz, S., John, W., Paz-Ares, L., Schmid-Bindert, G. (2018). KRAS-Mutant non-small cell lung cancer: From biology to therapy. Lung Cancer 124, 53–64. doi: 10.1016/j.lungcan.2018.07.013
Gao, J., Aksoy, B. A., Dogrusoz, U., Dresdner, G., Gross, B., Sumer, S. O., et al. (2013). Integrative analysis of complex cancer genomics and clinical profiles using the cBioPortal. Sci. Signal 6 (269), pl1. doi: 10.1126/scisignal.2004088
Gao, W., Jin, J., Yin, J., Land, S., Gaither-Davis, A., Christie, N., et al. (2017a). KRAS and TP53 mutations in bronchoscopy samples from former lung cancer patients. Mol. Carcinog. 56 (2), 381–388. doi: 10.1002/mc.22501
Gao, X., Dai, M., Li, Q., Wang, Z., Lu, Y., Song, Z. (2017b). HMGA2 regulates lung cancer proliferation and metastasis. Thorac. Cancer 8 (5), 501–510. doi: 10.1111/1759-7714.12476
Gautschi, O., Huegli, B., Ziegler, A., Gugger, M., Heighway, J., Ratschiller, D., et al. (2007). Origin and prognostic value of circulating KRAS mutations in lung cancer patients. Cancer Lett. 254 (2), 265–273. doi: 10.1016/j.canlet.2007.03.008
Graziano, S. L., Gamble, G. P., Newman, N. B., Abbott, L. Z., Rooney, M., Mookherjee, S., et al. (1999). Prognostic significance of K-ras codon 12 mutations in patients with resected stage I and II non-small-cell lung cancer. J. Clin. Oncol. 17 (2), 668–675. doi: 10.1200/JCO.1999.17.2.668
Halmos, B., Huettner, C. S., Kocher, O., Ferenczi, K., Karp, D. D., Tenen, D. G. (2002). Down-regulation and antiproliferative role of C/EBPalpha in lung cancer. Cancer Res. 62 (2), 528–534.
Hua, F., Shang, S., Yang, Y. W., Zhang, H. Z., Xu, T. L., Yu, J. J., et al. (2019). TRIB3 Interacts with beta-Catenin and TCF4 to increase stem cell features of colorectal cancer stem cells and tumorigenesis. Gastroenterology 156 (3), 708–721.e715. doi: 10.1053/j.gastro.2018.10.031
Huang, T., Cai, Y. D. (2013). An information-theoretic machine learning approach to expression QTL analysis. PloS One 8 (6), e67899. doi: 10.1371/journal.pone.0067899
Huang, T., Tu, K., Shyr, Y., Wei, C. C., Xie, L., Li, Y. X. (2008). The prediction of interferon treatment effects based on time series microarray gene expression profiles. J. Trans. Med. 6 (1), 44. doi: 10.1186/1479-5876-6-44
Huang, T., Chen, L., Liu, X. J., Cai, Y. D. (2011). Predicting triplet of transcription factor - mediating enzyme - target gene by functional profiles. Neurocomputing 74 (17), 3677–3681. doi: 10.1016/j.neucom.2011.07.019
Jemal, A., Siegel, R., Ward, E., Murray, T., Xu, J., Smigal, C., et al. (2006). Cancer Statistics, 2006. CA: A Cancer J. Clin. 56 (2), 106–130. doi: 10.3322/canjclin.56.2.106
Jemal, A., Bray, F., Center, M. M., Ferlay, J., Ward, E., Forman, D. (2011). Global cancer statistics. CA Cancer J. Clin. 61 (2), 69–90. doi: 10.3322/caac.20107
Jiang, Y., Pan, X., Zhang, Y., Huang, T., Gao, Y. (2019). Gene expression difference between primary and metastatic renal cell carcinoma using patient-derived xenografts. IEEE Access 7, 142586–142594. doi: 10.1109/ACCESS.2019.2944132
Kumar, M. S., Armenteros-Monterroso, E., East, P., Chakravorty, P., Matthews, N., Winslow, M. M., et al. (2014). HMGA2 functions as a competing endogenous RNA to promote lung cancer progression. Nature 505 (7482), 212–217. doi: 10.1038/nature12785
Labbe, C., Cabanero, M., Korpanty, G. J., Tomasini, P., Doherty, M. K., Mascaux, C., et al. (2017). Prognostic and predictive effects of TP53 co-mutation in patients with EGFR-mutated non-small cell lung cancer (NSCLC). Lung Cancer 111, 23–29. doi: 10.1016/j.lungcan.2017.06.014
Lamb, J., Crawford, E. D., Peck, D., Modell, J. W., Blat, I. C., Wrobel, M. J., et al. (2006). The connectivity map: using gene-expression signatures to connect small molecules, genes, and disease. Science 313 (5795), 1929–1935. doi: 10.1126/science.1132939
Laurent, C., Svrcek, M., Flejou, J. F., Chenard, M. P., Duclos, B., Freund, J. N., et al. (2011). Immunohistochemical expression of CDX2, beta-catenin, and TP53 in inflammatory bowel disease-associated colorectal cancer. Inflammation Bowel Dis. 17 (1), 232–240. doi: 10.1002/ibd.21451
Lei, C., Wei-Ming, Z., Yu-Dong, C., Tao, H. (2013). Prediction of metabolic pathway using graph property, chemical functional group and chemical structural set. Curr. Bioinf. 8 (2), 200–207. doi: 10.2174/1574893611308020008
Li, J., Huang, T. (2018). Predicting and analyzing early wake-up associated gene expressions by integrating GWAS and eQTL studies. Biochim. Biophys. Acta 1864 (6 Pt B), 2241–2246. doi: 10.1016/j.bbadis.2017.10.036
Li, H., Liang, X., Qin, X., Cai, S., Yu, S. (2015). Association of matrix metalloproteinase family gene polymorphisms with lung cancer risk: logistic regression and generalized odds of published data. Sci. Rep. 5, 10056. doi: 10.1038/srep10056
Li, J., Lan, C.-N., Kong, Y., Feng, S.-S., Huang, T. (2018). Identification and analysis of blood gene expression signature for osteoarthritis with advanced feature selection methods. Front. Genet. 9, 246. doi: 10.3389/fgene.2018.00246
Li, J., Lu, L., Zhang, Y.-H., Xu, Y., Liu, M., Feng, K., et al. (2019a). Identification of leukemia stem cell expression signatures through Monte Carlo feature selection strategy and support vector machine. Cancer Gene Ther. doi: 10.1038/s41417-019-0105-y
Li, J., Lu, L., Zhang, Y. H., Liu, M., Chen, L., Huang, T., et al. (2019b). Identification of synthetic lethality based on a functional network by using machine learning algorithms. J. Cell Biochem. 120 (1), 405–416. doi: 10.1002/jcb.27395
Liu, P., Morrison, C., Wang, L., Xiong, D., Vedell, P., Cui, P., et al. (2012). Identification of somatic mutations in non-small cell lung carcinomas using whole-exome sequencing. Carcinogenesis 33 (7), 1270–1276. doi: 10.1093/carcin/bgs148
Liu, C., Cui, P., Huang, T. (2017). Identification of cell cycle-regulated genes by convolutional neural network. Comb. Chem. High Throughput Screen 20 (7), 603–611. doi: 10.2174/1386207320666170417144937
Lo, F. Y., Chen, H. T., Cheng, H. C., Hsu, H. S., Wang, Y. C. (2012). Overexpression of PAFAH1B1 is associated with tumor metastasis and poor survival in non-small cell lung cancer. Lung Cancer 77 (3), 585–592. doi: 10.1016/j.lungcan.2012.05.105
Lu, H., Yu, Z., Liu, S., Cui, L., Chen, X., Yao, R. (2015). CUGBP1 promotes cell proliferation and suppresses apoptosis via down-regulating C/EBPalpha in human non-small cell lung cancers. Med. Oncol. 32 (3), 82. doi: 10.1007/s12032-015-0544-8
Luo, J., Shi, K., Yin, S. Y., Tang, R. X., Chen, W. J., Huang, L. Z., et al. (2018). Clinical value of miR-182-5p in lung squamous cell carcinoma: a study combining data from TCGA, GEO, and RT-qPCR validation. World J. Surg. Oncol. 16 (1), 76. doi: 10.1186/s12957-018-1378-6
Lv, M., Wang, L. (2015). Comprehensive analysis of genes, pathways, and TFs in nonsmoking Taiwan females with lung cancer. Exp. Lung Res. 41 (2), 74–83. doi: 10.3109/01902148.2014.971472
Mao, L., Hruban, H. R., Boyle, J. O., Ms, T., Sidransky, D. (1994). Detection of oncogene mutations in sputum precedes diagnosis of lung cancer. 54 (7), 1634–1637.
Marwitz, S., Depner, S., Dvornikov, D., Merkle, R., Szczygiel, M., Muller-Decker, K., et al. (2016). Downregulation of the TGFbeta pseudoreceptor bambi in non-small cell lung cancer enhances TGFbeta signaling and invasion. Cancer Res. 76 (13), 3785–3801. doi: 10.1158/0008-5472.CAN-15-1326
Mills, N. E., Fishman, C. L., Scholes, J., Anderson, S. E., Rom, W. N., Jacobson, D. R. (1995). Detection of K-ras oncogene mutations in bronchoalveolar lavage fluid for lung cancer diagnosis. JNCI: J. Natl. Cancer Institute 87 (14), 1056–1060. doi: 10.1093/jnci/87.14.1056
Morgensztern, D., Ng, S. H., Gao, F., Govindan, R. (2010). Trends in stage distribution for patients with non-small cell lung cancer: a national cancer database survey. J. Thoracic Oncol. 5 (1), 29–33. doi: 10.1097/JTO.0b013e3181c5920c
Nakamoto, M., Teramoto, H., Matsumoto, S., Igishi, T., Shimizu, E. (2001). K-ras and rho A mutations in malignant pleural effusion. Int. J. Oncol. 19 (5), 971–976. doi: 10.3892/ijo.19.5.971
Nishimura, T., Nakata, A., Chen, X., Nishi, K., Meguro-Horike, M., Sasaki, S., et al. (2019). Cancer stem-like properties and gefitinib resistance are dependent on purine synthetic metabolism mediated by the mitochondrial enzyme MTHFD2. Oncogene 38 (14), 2464–2481. doi: 10.1038/s41388-018-0589-1
Okudela, K., Mitsui, H., Woo, T., Arai, H., Suzuki, T., Matsumura, M., et al. (2016). Alterations in cathepsin L expression in lung cancers. Pathol. Int. 66 (7), 386–392. doi: 10.1111/pin.12424
Ortega, A., Mas-Oliva, J. (1986). Direct regulatory effect of cholesterol on the calmodulin stimulated calcium pump of cardiac sarcolemma. Biochem. Biophys. Res. Commun. 139 (3), 868–874. doi: 10.1016/S0006-291X(86)80258-3
Pan, X., Hu, X., Zhang, Y. H., Feng, K., Wang, S. P., Chen, L., et al. (2018). Identifying Patients with atrioventricular septal defect in down syndrome populations by using self-normalizing neural networks and feature selection. Genes (Basel) 9 (4). doi: 10.3390/genes9040208
Pan, X., Chen, L., Feng, K. Y., Hu, X. H., Zhang, Y. H., Kong, X. Y., et al. (2019a). Analysis of Expression Pattern of snoRNAs in Different Cancer Types with Machine Learning Algorithms. Int. J. Mol. Sci. 20 (9). doi: 10.3390/ijms20092185
Pan, X., Hu, X., Zhang, Y.-H., Chen, L., Zhu, L., Wan, S., et al. (2019b). Identification of the copy number variant biomarkers for breast cancer subtypes. Mol. Genet. Genomics 294 (1), 95–110. doi: 10.1007/s00438-018-1488-4
Peng, H., Long, F., Ding, C. (2005). Feature selection based on mutual information: criteria of max-dependency, max-relevance, and min-redundancy. IEEE Trans. Pattern Anal. Mach. Intell. 27 (8), 1226–1238. doi: 10.1109/TPAMI.2005.159
Sandler, A., Gray, R., Perry, M. C., Brahmer, J., Schiller, J. H., Dowlati, A., et al. (2006). Paclitaxel-carboplatin alone or with bevacizumab for non-small-cell lung cancer. N. Engl. J. Med. 355 (24), 2542–2550. doi: 10.1056/NEJMoa061884
Scagliotti, G. V., Parikh, P., von Pawel, J., Biesma, B., Vansteenkiste, J., Manegold, C., et al. (2008). Phase III study comparing cisplatin plus gemcitabine with cisplatin plus pemetrexed in chemotherapy-naive patients with advanced-stage non-small-cell lung cancer. J. Clin. Oncol. 26 (21), 3543–3551. doi: 10.1200/JCO.2007.15.0375
Slattery, M. L., Lundgreen, A. (2014). The influence of the CHIEF pathway on colorectal cancer-specific mortality. PloS One 9 (12), e116169. doi: 10.1371/journal.pone.0116169
Subramanian, A., Narayan, R., Corsello, S. M., Peck, D. D., Natoli, T. E., Lu, X., et al. (2017). A next generation connectivity map: L1000 platform and the first 1,000,000 profiles. Cell 171 (6), 1437–1452.e1417. doi: 10.1016/j.cell.2017.10.049
Sutherland, L. C., Wang, K., Robinson, A. G. (2010). RBM5 as a putative tumor suppressor gene for lung cancer. J. Thorac. Oncol. 5 (3), 294–298. doi: 10.1097/JTO.0b013e3181c6e330
Turk, V., Stoka, V., Vasiljeva, O., Renko, M., Sun, T., Turk, B., et al. (2012). Cysteine cathepsins: from structure, function and regulation to new frontiers. Biochim. Biophys. Acta 1824 (1), 68–88. doi: 10.1016/j.bbapap.2011.10.002
Tyagi, N., Marimuthu, S., Bhardwaj, A., Deshmukh, S. K., Srivastava, S. K., Singh, A. P., et al. (2016). p-21 activated kinase 4 (PAK4) maintains stem cell-like phenotypes in pancreatic cancer cells through activation of STAT3 signaling. Cancer Lett. 370 (2), 260–267. doi: 10.1016/j.canlet.2015.10.028
Wang, S.-B., Huang, T.J.M.B.R. (2019a). The early detection of asthma based on blood gene expression 46, 1, 217–223. doi: 10.1007/s11033-018-4463-6
Wang, S. B., Huang, T. (2019b). The early detection of asthma based on blood gene expression. Mol. Biol. Rep. 46 (1), 217–223. doi: 10.1007/s11033-018-4463-6
Wang, T. L., Song, Y. Q., Ren, Y. W., Zhou, B. S., Wang, H. T., Bai, L., et al. (2016a). Dual Specificity Phosphatase 6 (DUSP6) Polymorphism Predicts Prognosis of Inoperable Non-Small Cell Lung Cancer after Chemoradiotherapy. Clin. Lab. 62 (3), 301–310. doi: 10.7754/Clin.Lab.2015.150432
Wang, X., Lu, Y., Feng, W., Chen, Q., Guo, H., Sun, X., et al. (2016b). A two kinase-gene signature model using CDK2 and PAK4 expression predicts poor outcome in non-small cell lung cancers. Neoplasma 63 (2), 322–329. doi: 10.4149/220_150817N448
Wang, S., Zhang, Y. H., Zhang, N., Chen, L., Huang, T., Cai, Y. D. (2017a). Recognizing and predicting thioether bridges formed by lanthionine and beta-methyllanthionine in lantibiotics using a random forest approach with feature selection. Comb. Chem. High Throughput Screen 20 (7), 582–593. doi: 10.2174/1386207320666170310115754
Wang, X., Li, M., Hu, M., Wei, P., Zhu, W. (2017b). BAMBI overexpression together with beta-sitosterol ameliorates NSCLC via inhibiting autophagy and inactivating TGF-beta/Smad2/3 pathway. Oncol. Rep. 37 (5), 3046–3054. doi: 10.3892/or.2017.5508
Westcott, P. M., To, M. D. (2013). The genetics and biology of KRAS in lung cancer. Chin. J. Cancer 32 (2), 63–70. doi: 10.5732/cjc.012.10098
Yan, X., Yu-Hang, Z., JiaRui, L., Xiaoyong, P., Tao, H., Yu-Dong, C. (2019). New computational tool based on machine-learning algorithms for the identification of rhinovirus infection-related genes. Combinatorial Chem. High Throughput Screening 22, 1–1. doi: 10.2174/1386207322666191129114741
Yang, J., Chen, L., Kong, X., Huang, T., Cai, Y. D. (2014). Analysis of tumor suppressor genes based on gene ontology and the KEGG pathway. PloS One 9 (9), e107202. doi: 10.1371/journal.pone.0107202
Yin, H., Wang, Y., Chen, W., Zhong, S., Liu, Z., Zhao, J. (2016). Drug-resistant CXCR4-positive cells have the molecular characteristics of EMT in NSCLC. Gene 594 (1), 23–29. doi: 10.1016/j.gene.2016.08.043
Zhang, N., Wang, M., Zhang, P., Huang, T. (2016). Classification of cancers based on copy number variation landscapes. Biochim. Biophys. Acta 1860 (11 Pt B), 2750–2755. doi: 10.1016/j.bbagen.2016.06.003
Zhang, K., Wang, J., Tong, T. R., Wu, X., Nelson, R., Yuan, Y. C., et al. (2017). Loss of H2B monoubiquitination is associated with poor-differentiation and enhanced malignancy of lung adenocarcinoma. Int. J. Cancer 141 (4), 766–777. doi: 10.1002/ijc.30769
Zhang, X., Zhong, N., Li, X., Chen, M. B. (2019). TRIB3 promotes lung cancer progression by activating beta-catenin signaling. Eur. J. Pharmacol. 863, 172697. doi: 10.1016/j.ejphar.2019.172697
Zhao, T. H., Jiang, M., Huang, T., Li, B. Q., Zhang, N., Li, H. P., et al. (2013). A novel method of predicting protein disordered regions based on sequence features. BioMed. Res. Int. 2013, 414327. doi: 10.1155/2013/414327
Keywords: Kirsten rat sarcoma oncogene (KRAS), mutation, lung cancer, predictor, gene expression
Citation: Zhang J, Hu H, Xu S, Jiang H, Zhu J, Qin E, He Z and Chen E (2020) The Functional Effects of Key Driver KRAS Mutations on Gene Expression in Lung Cancer. Front. Genet. 11:17. doi: 10.3389/fgene.2020.00017
Received: 11 October 2019; Accepted: 07 January 2020;
Published: 04 February 2020.
Edited by:
Tao Huang, Shanghai Institutes for Biological Sciences (CAS), ChinaReviewed by:
Jing Feng, Tianjin Medical University General Hospital, ChinaCopyright © 2020 Zhang, Hu, Xu, Jiang, Zhu, Qin, He and Chen. This is an open-access article distributed under the terms of the Creative Commons Attribution License (CC BY). The use, distribution or reproduction in other forums is permitted, provided the original author(s) and the copyright owner(s) are credited and that the original publication in this journal is cited, in accordance with accepted academic practice. No use, distribution or reproduction is permitted which does not comply with these terms.
*Correspondence: Zhengfu He, aGV6aGVuZ2Z1QHpqdS5lZHUuY24=; Enguo Chen, MzE5NTAyNEB6anUuZWR1LmNu
Disclaimer: All claims expressed in this article are solely those of the authors and do not necessarily represent those of their affiliated organizations, or those of the publisher, the editors and the reviewers. Any product that may be evaluated in this article or claim that may be made by its manufacturer is not guaranteed or endorsed by the publisher.
Research integrity at Frontiers
Learn more about the work of our research integrity team to safeguard the quality of each article we publish.