- 1Atherogenomics Laboratory, University of Ottawa Heart Institute, Ottawa, ON, Canada
- 2Ruddy Canadian Cardiovascular Genetics Centre, University of Ottawa Heart Institute, Ottawa, ON, Canada
- 3Center for Public Health Genomics, University of Virginia, Charlottesville, VA, United States
- 4Children Hospital of Eastern Ontario Research Institute, Ottawa, ON, Canada
- 5Department of Biochemistry, Microbiology and Immunology, University of Ottawa, Ottawa, ON, Canada
- 6Department of Medicine, University of Ottawa Heart Institute, Ottawa, ON, Canada
Genome-wide association studies have identified several genetic loci linked to coronary artery disease (CAD) most of them located in non-protein coding regions of the genome. One such locus is the CAD Associated Region between MFGE8 and ABHD2 (CARMA), a ∼18 kb haplotype that was recently shown to regulate vicinal protein coding genes. Here, we further investigate the region by examining a long non-coding RNA gene locus (CARMAL/RP11-326A19.4/AC013565) abutting the CARMA region. Expression-genotype correlation analyses of public databases indicate that CARMAL levels are influenced by CAD associated variants suggesting that it might have cardioprotective functions. We found CARMAL to be stably expressed at relatively low levels and enriched in the cytosol. CARMAL function was investigated by several gene targeting approaches in HEK293T: inactive CRISPR fusion proteins, antisense, overexpression and inactivation by CRISPR-mediated knock-out. Modest increases in CARMAL (3–4×) obtained via CRISPRa using distinct single-guided RNAs did not result in consistent transcriptome effects. By contrast, CARMAL deletion or reduced CARMAL expression via CRISPRi increased MFGE8 levels, suggesting that CARMAL is contributing to reduce MFGE8 expression under basal conditions. While future investigations are required to clarify the mechanism(s) by which CARMAL acts on MFGE8, integrative bioinformatic analyses of the transcriptome of CARMAL deleted cells suggest that this locus may also be involved in leucine metabolism, splicing, transcriptional regulation and Shwachman-Bodian-Diamond syndrome protein function.
Introduction
Genome-Wide Association Studies (GWAS) have identified genomic variants that statistically partition with numerous diseases. With regard to cardiovascular disease (CAD) more than 160 loci tagged by distinct single nucleotide polymorphisms (SNPs) distributed throughout the genome have been shown to associate with CAD risk (p < 10–8). Since the vast majority of these SNPs are situated in regions distant from genes, understanding of their role in disease processes will necessitate extensive follow-up mechanistic inquiries. Recently, we examined the role of a specific CAD associated 18 kb region in HuH-7 hepatoma cells and showed that it could regulate 2 distinct genes situated ∼150 kb away (MFGE8 and HAPLN3) (Soubeyrand et al., 2019b). Both proteins are secreted and involved in cell adhesion. MFGE8 (milk fat globule-EGFP factor 8) encodes for lactadherin, which has been implicated in several physiological and cellular processes (Raymond et al., 2009). The physiological role of HAPLN3 (hyaluronan and proteoglycan link protein 3) is less clear, although it has been linked to height (UK Biobank data) and cancer (Møller et al., 2017).
Our previous study focused on validated protein coding genes in cis, but in the course of the study the presence of a long non-coding RNA (lncRNA) gene, RP11-326A19.4, in close proximity to the CAD associated region was noted. Long non-coding RNAs are relatively long transcripts that resemble traditional mRNA but lack protein coding potential (Ransohoff et al., 2017). Recent estimates suggest that they outnumber protein coding genes and are expressed pervasively throughout the genome. Compared to protein coding genes they tend to be less conserved, more spliced, expressed at lower levels and enriched in the nucleus (Derrien et al., 2012).
A current controversy is the relative importance of the transcription product per se versus the underlying chromatin remodeling giving rise to the nascent transcript. This contentious issue stems in part from the low expression and conservation of lncRNA sequences, which beyond complicating functional inquiries, have been interpreted to signify a lack of transcript function. As the function of lncRNAs is presumed to require folding, conservation of structure and function may be retained despite low nucleotide conservation (Diederichs, 2014). An analysis of a subset of lncRNA loci suggests that in most cases, cis effects were mediated by chromatin remodeling itself (Engreitz et al., 2016). For some lncRNA loci however, considerable evidence support a role for the transcript itself (e.g., Xist, MALAT1, NEAT1) (Böhmdorfer and Wierzbicki, 2015). Finally, some lncRNAs operate outside the nucleus; indeed lncRNAs originating from the nucleus have been located in the cytosol and mitochondria (Noh et al., 2018).
Preliminary attempts to characterize the function of this lncRNA revealed a putative regulatory role in IL6 expression, which we ultimately showed to be the result of an off-target effect by the CRISPRa system through intermediates that remain to be clarified (Soubeyrand et al., 2019a). Here we have characterized the RP11-326A19.4 transcript in an effort to uncover its biological role. Mining publicly available data and presenting novel functional data, we provide evidence that this lncRNA, CARMAL (CAD Associated Region between MFGE8 and ABHD2 LncRNA), is expressed in the vasculature, indicating that the locus is active and potentially functionally important. Functional investigations point to both genome-wide and local roles, via the repression of MFGE8 expression through mechanisms that remain to be clarified.
Results
Identification of a Long Non-coding RNA Enriched in Arterial Tissues
We recently characterized a “gene desert” region linked to CAD through a combination of fine-mapping approaches and functional assays (Soubeyrand et al., 2019b). The investigation revealed that the region could regulate various genes in cis, primarily MFGE8, a gene located 130 kb away, as a likely CAD relevant downstream mediator. While the analysis focused on well characterized genes, we also noted the presence of an annotated but otherwise uncharacterized gene classified as a long non-coding RNA (lncRNA) immediately abutting the region (Supplementary Figure S1). This putative lncRNA (RP11-326A19.4/AC013565.1) is expressed in a haplotype dependent manner, with the protective CAD allele being associated with reduced expression in the vasculature (Supplementary Figure S2). This last feature hinted at a possible vascular role, an interpretation further substantiated by its enriched expression in the vascular bed relative to other tissues (Supplementary Figure S3). To reflect the physical integration of this lncRNA within the CAD associated region between ABHD2 and MFGE8 (CARMA) gene hub, the lncRNA is denoted here as CARMAL (CARMA Associated lncRNA).
CARMAL Is Stably Expressed at Low Levels in HEK293T and Coronary Models
Biological role is to a large extent tributary of expression level. For instance, enzymes which can undergo multiple rounds of catalysis (e.g., MAPK3) are considerably less abundant than structural proteins (e.g., TUBB) (Wang et al., 2015). Similarly more plentiful lncRNA are predicted to operate as structural scaffolds (e.g., NEAT1) or miRNA sponges (e.g., DANCR) whereas lower abundance RNA may point to more local roles (e.g., ANRIL and transcription regulation in cis). An early validation of the RP11-326A19.4 transcript, described previously, confirmed its presence in HEK293T but its abundance was not investigated (Soubeyrand et al., 2019a). Expression levels of CARMAL were measured by droplet digital RT-PCR (ddPCR) in HEK293T and two primary coronary models using a pair of oligonucleotides targeting exon 1 and 2, shared by all reported splice variants of CARMAL. Expression was generally low, ranging from ∼50 copies per cell in HEK293T to only a few copies in the vascular models; consistent (relative) values were obtained by qRT-PCR after normalization to the housekeeper gene Peptidyl-prolyl cis-trans isomerase A (PPIA) (Figure 1).
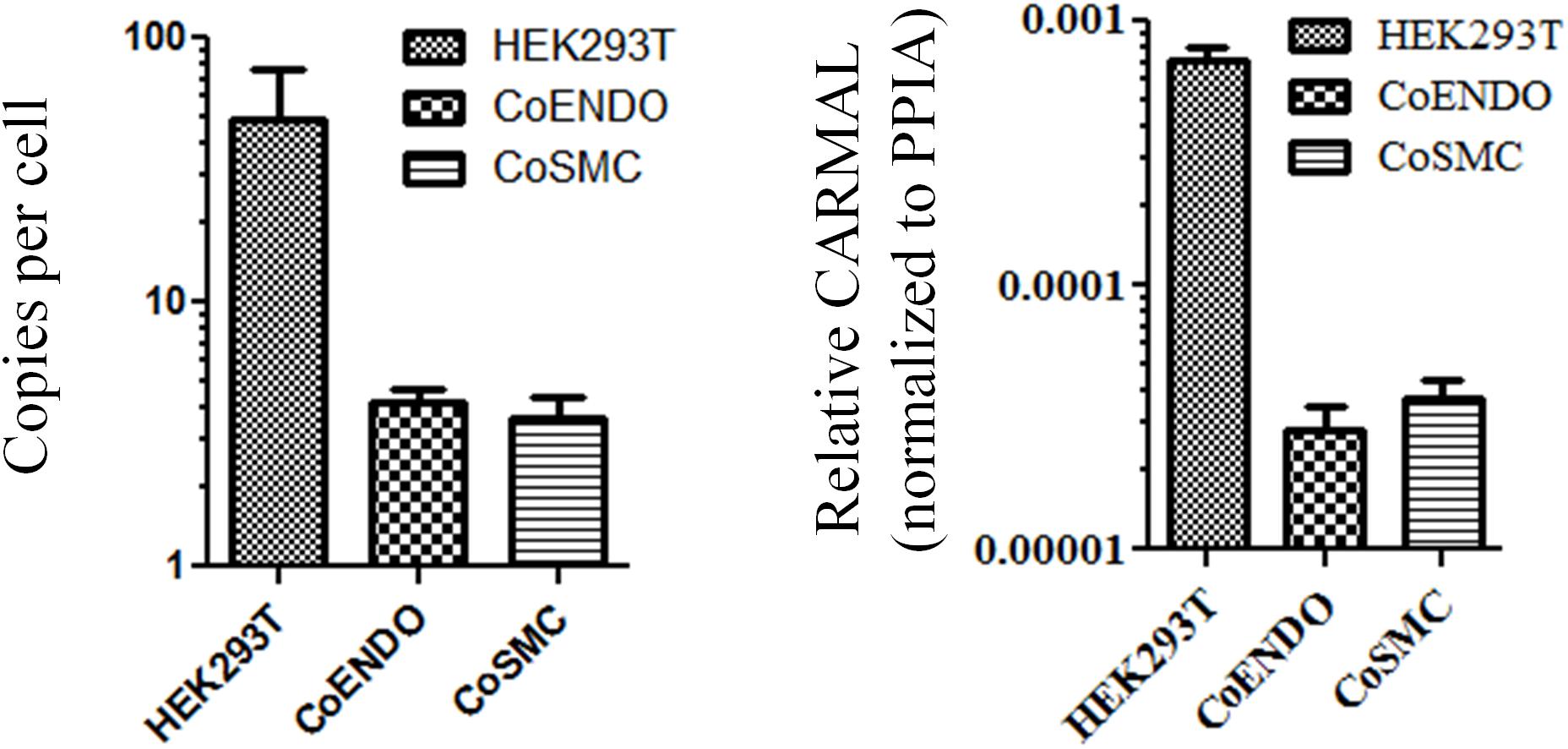
Figure 1. CARMAL is expressed at low levels. Copy number of CARMAL per cell (left) was estimated by droplet PCR while expression relative to PPIA (right) was determined by qRT-PCR. Data represent the mean of three biological replicates (±SD). CoENDO and coSMC refer to coronary endothelial cells and coronary smooth muscle cells, respectively.
CARMAL Is Enriched in the Cytoplasm and Is Polyadenylated
As biological function is contingent on localization, we next determined CARMAL subcellular distribution. Fractionation assays were performed on HEK293T cells as well as primary smooth muscle cells. In both cell types CARMAL fractionated similarly to PPIA, a mature (cytosolic) mRNA, in showing a pattern distinct from U1, a spliceosome (nuclear) RNA (Figure 2). Attempts to support our fractionation findings via complementary approaches, MS2-GFP binding and FISH (Stellaris), were unsuccessful as the first approach revealed a non-specific (i.e., similar to the reverse-complement transcript) enrichment in nuclear speckles and the second, no detectable signal. As an alternative, we took advantage of algorithms that have been engineered to predict lncRNA localization. One such tool, LncLocator is a recently available tool that utilizes a deep learning approach on a large set of experimentally validated lncRNAs to predict subcellular localization (Cao et al., 2018; Gudenas and Wang, 2018). Subjecting CARMAL’s sequence alongside various lncRNA of known distribution to the algorithm predicts a cytoplasmic localization, in line with our findings (Supplementary Table S1). Interestingly, the likelihood of nuclear localization remained substantial, on par with MALAT1, a lncRNA that was reported to shuttle between the nucleus and cytoplasm in a cell cycle dependent manner (Yang et al., 2013; Zhang et al., 2017).
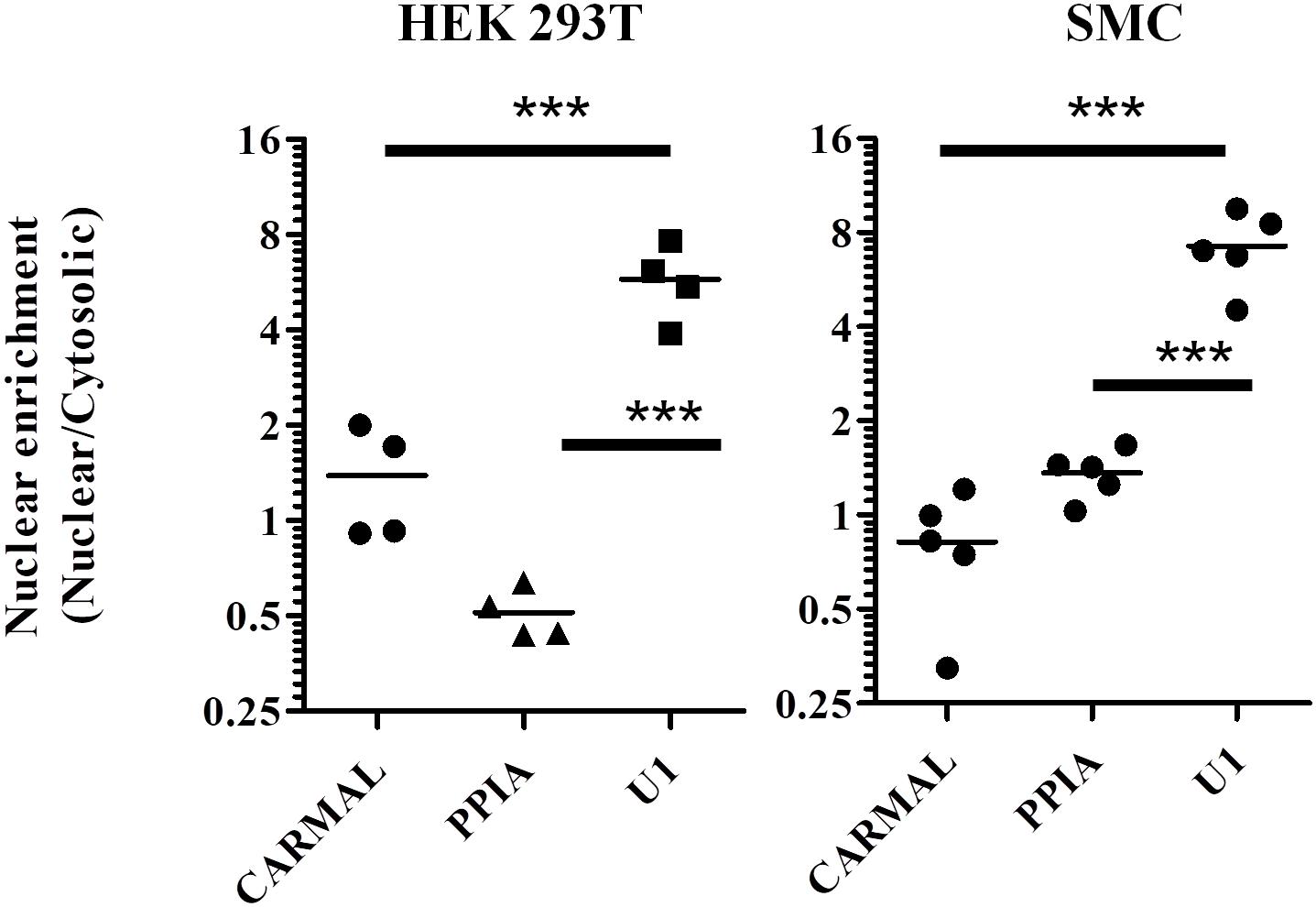
Figure 2. PPIA and CARMAL display similar cell fractionation profiles. Relative transcript enrichment in fractionated HEK293T and arterial smooth muscle (SMC) cells. Cells were fractionated sequentially into cytosolic and nuclear fractions, and the indicated RNAs within were quantified by qRT-PCR. Nuclear enrichment is defined as the ratio of nuclear to cytosolic signals. Individual data points represent independent fractionations. Statistically significant differences were tested using one-way ANOVA and Tukey’s post hoc test. *** indicates p < 0.005.
Presence of a poly(A) tail was tested next as some lncRNA, particularly those arising from transcriptional “noise” or enhancer sequences have been reported to contain no poly(A) tail (Liu, 2017). While GTEx data were derived from RNA enriched by poly(A) bead isolation, the relatively low abundance reported by GTEx could reflect a substantial loss of a non-poly(A) population during isolation. To directly test for the presence of a poly(A) tail in CARMAL, cDNA synthesis reactions performed in the presence of either oligo(dT) anchors or random hexamers were performed; CARMAL does not contain internal poly(A) stretches that could promote internal primer binding and extension. Similar conversion to cDNA was obtained with oligodT anchor or random hexamers, pointing to the presence of a poly(A) tail in the bulk of the population (Figure 3). Addition of a poly(A) tail occurs co-transcriptionally with RNA polymerase II transcription, which is sensitive to Actinomycin D (ActD). Inclusion of Act D (for up to 5 h) did not reduce, indeed in some cells slightly increased, CARMAL levels, indicating that the RNA is basally stable and might be further increased via post-transcriptional processes upon ActD inhibition (e.g., by interfering with miRNA-mediated destabilization).
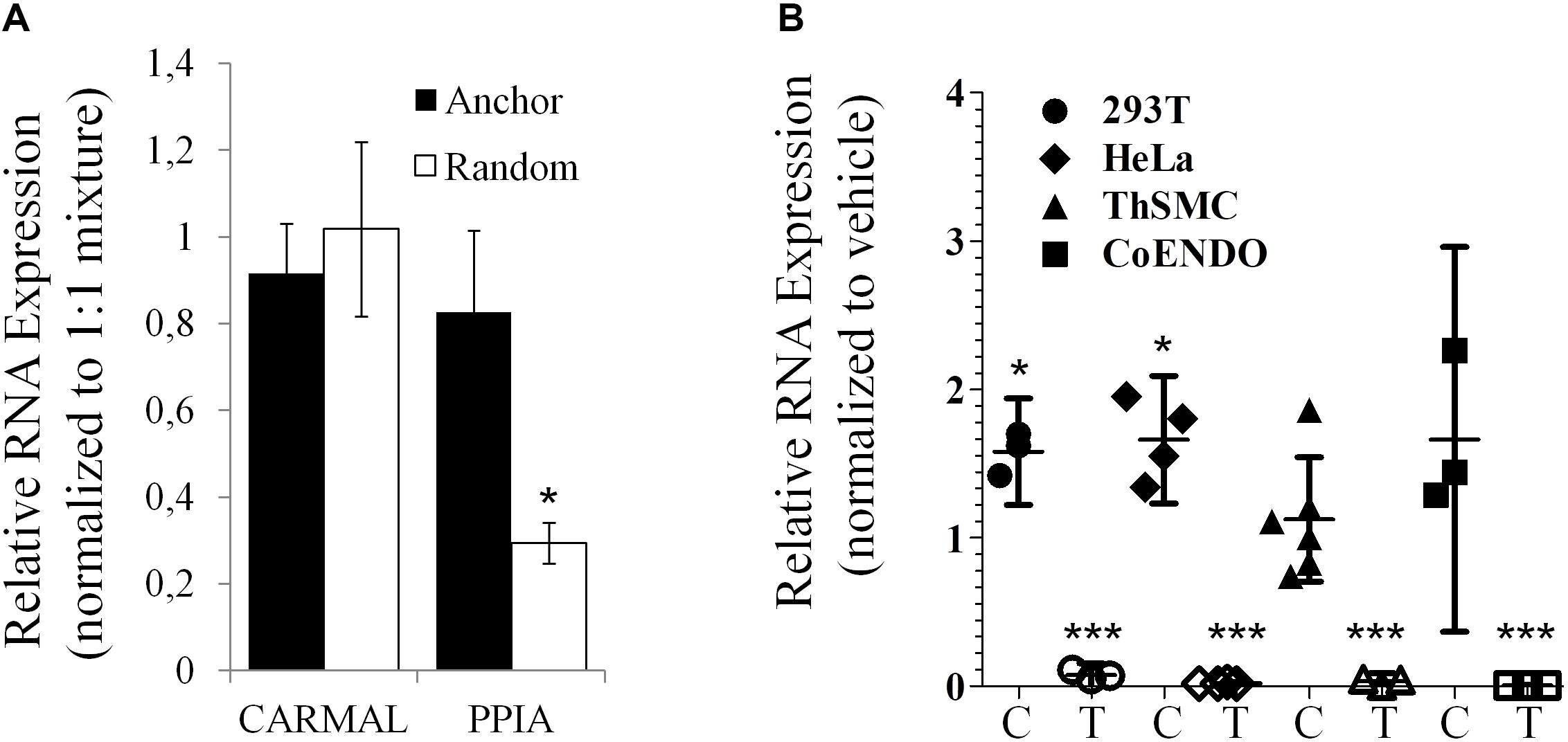
Figure 3. CARMAL is polyadenylated and stable. (A) CARMAL and PPIA levels were determined on cDNA prepared from HEK293T RNA in the presence of either random hexamer primer (poly(A) tail independent), oligodT anchor primer (poly(A) tail dependent) or a 1:1 mixture of both (standard condition), to which values are normalized. Results represent the mean of 3 biological replicates (±SD). (B) CARMAL (filled symbols; C) and TRIB1 (an unstable mRNA control; matching empty symbols; T) expression levels measured in cells treated with either Actinomycin D or vehicle DMSO) only. Values represent the ratio of the relative RNA abundance (relative to PPIA) in Actinomycin D treated cells over the vehicle treated cells. Data from three to five biological replicates are shown (±95% CI). *p < 0.05. Changes for TRIB1 were all highly significant (***p < 0.005).
Deletion of CARMAL Affects Flanking Gene Expression
Next, CARMAL requirement was directly addressed by CRISPR mediated KO targeting of a ∼420 bp deletion spanning part of the promoter region as well as exon 1 of CARMAL in HEK293T cells. We previously demonstrated that the region proximal to the transcription initiation region acted as a promoter in a reporter assay (Soubeyrand et al., 2019a). Abrogation of CARMAL expression was first confirmed by qPCR using primers targeting distinct exon combinations (Supplementary Figure S4). The local impact of the disruption was examined first since we previously demonstrated effects of the abutting CARMA region on HAPLN3 and MFGE8 in HuH-7 (Soubeyrand et al., 2019a). Deletion of the CARMAL exon 1 region resulted in increased MFGE8/HAPLN3 and reduced ABHD2 consistent with a local regulatory function for CARMAL (Figure 4). Thus CARMAL may, together with the abutting CARMA genomic region, be involved in regulating MFGE8 levels.
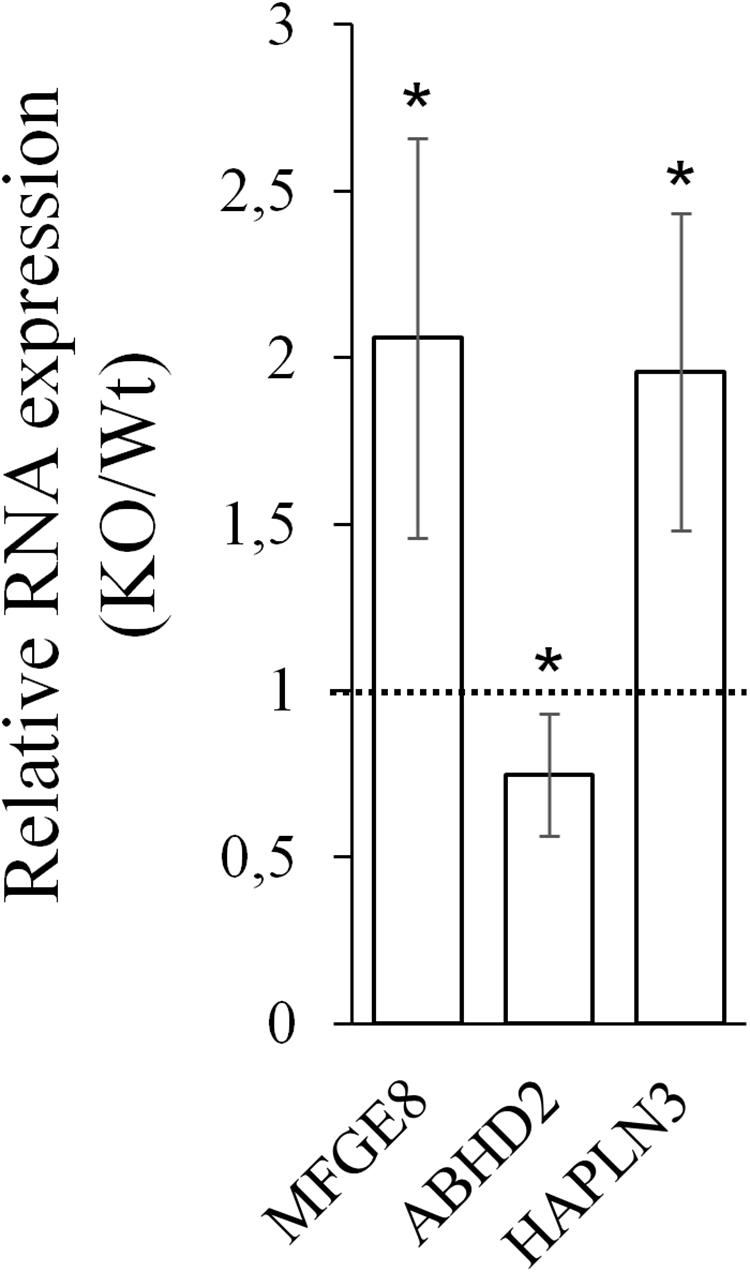
Figure 4. CARMAL deletion leads to increased MFGE8 and HAPLN3 but reduced ABHD2. RNA isolated from HEK293T, either Wt or harboring a CRISPR-Cas9-mediated CARMAL deletion (KO) were isolated and analyzed by qRT-PCR. Bars represent the average of eight (MFGE8/ABHD2) or four (HAPLN3) biological replicates (±95% CI). All changes between the KO and Wt were statistically significant (p < 0.05).
Suppression of CARMAL Leads to a Modest Increase in MFGE8
The above findings pointed to a functional link between CARMAL and MFGE8, with CARMAL possibly contributing to the suppression of MFGE8 under basal conditions. This could arise either as a result of loss of normal CARMAL expression or disruption of the gene locus per se. To test the contribution of the transcript to MFGE8 expression, CARMAL was first targeted using antisense oligonucleotides. This analysis proved inconclusive as CARMAL was resistant to ASO treatment (Supplementary Figure S5). As an alternative approach, CRISPRi was used. We previously demonstrated that a KRAB derivatized inactive (dCRISPR) version had no measurable impact on local gene expression when targeted to the promoter region of CARMAL, despite achieving a ∼30% reduction in CARMAL expression (Soubeyrand et al., 2019a). Recently however, novel KRAB derivatives with improved inhibitory potential have been reported (Yeo et al., 2018). Using three distinct repressors (including KRAB for comparison) in combination with one of three distinct gRNA designed against intron 1 of CARMAL, we achieved 40–60% suppression of CARMAL. Interestingly, there was a statistically significant, albeit modest, increase in MFGE8 when the most potent repressor (dCas9-KRAB-MeCP2) was used (Figure 5). Of note, with the exception of CARMAL, among the transcripts tested, only MFGE8 was significantly affected relative to SRP14.
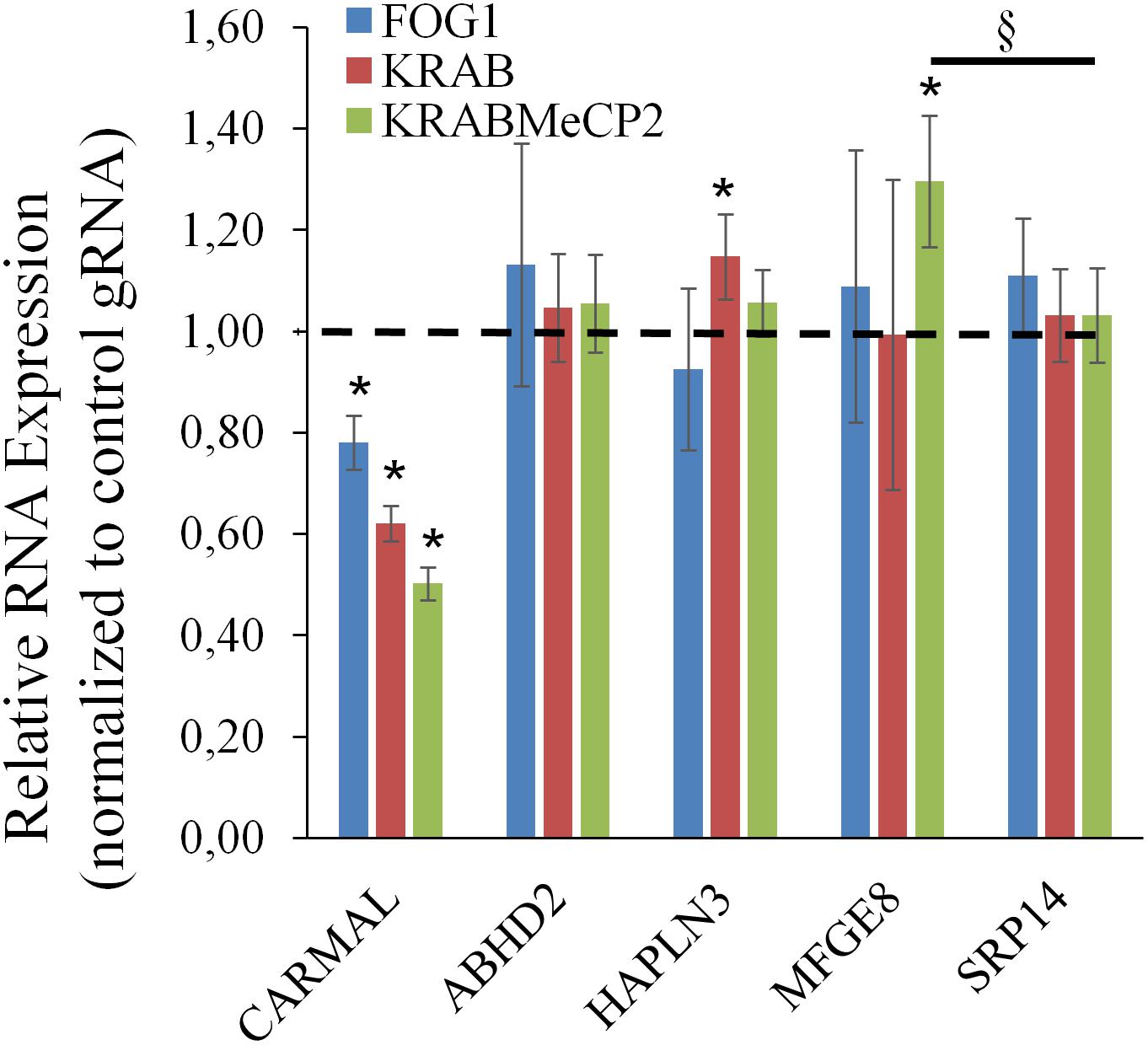
Figure 5. CARMAL suppression by KRABMeCP2-dCas9 is associated with increased MFGE8 levels. HEK293T cells were transfected for 72 h with the indicated CRISPRi fusion constructs together with one of three distinct gRNA or a control gRNA (tracrRNA + linker only) expressing constructs. For each biological replicate, inhibition (vs trcrRNA control) values obtained using each gRNA were averaged. Values represent the average of three distinct biological replicates (±95% CI) *, <0.05 vs CRISPRi/trcrRNA only; §p < 0.05 vs normalized SRP14 values (one-way ANOVA).
Increased CARMAL Abundance and Expression Do Not Affect MFGE8
Having examined the effects of CARMAL reduction, overexpression was investigated next. We previously demonstrated that a mild (∼4 X) upregulation of CARMAL via CRISPRa (VP160) had no effects on local gene expression, including HAPLN3 and MFGE8 (Soubeyrand et al., 2019a). These findings were confirmed in a new set of CRISPRa experiments using a more potent activator (SP-dCas9-VPR) and two distinct gRNAs (data is publicly available as GSE142097). In contrast to CRISPRa, transducing CARMAL led to a strong increase in CARMAL abundance (Figure 6B) which nonetheless failed to impact MFGE8 or ABHD2 (Figure 6A). Thus, these results agree with the CRISPRa data insofar that they demonstrate that higher CARMAL levels affect neither MFGE8 nor ABDH2 in Wt cells. In addition, the observation that the introduction of CARMAL increases neither transcript in CARMAL KO cells suggests that the CARMAL transcript is neither needed nor sufficient to maintain a steady-state level of MFGE8/ABHD2. Lastly, since the introduction of CARMAL does not reduce MFGE8/ABHD2 levels in either cell type, the MFGE8 upregulation seen in the KO and the CRISPRi experiments cannot be explained by the removal or reduction of a destabilizing influence of CARMAL on MFGE8.
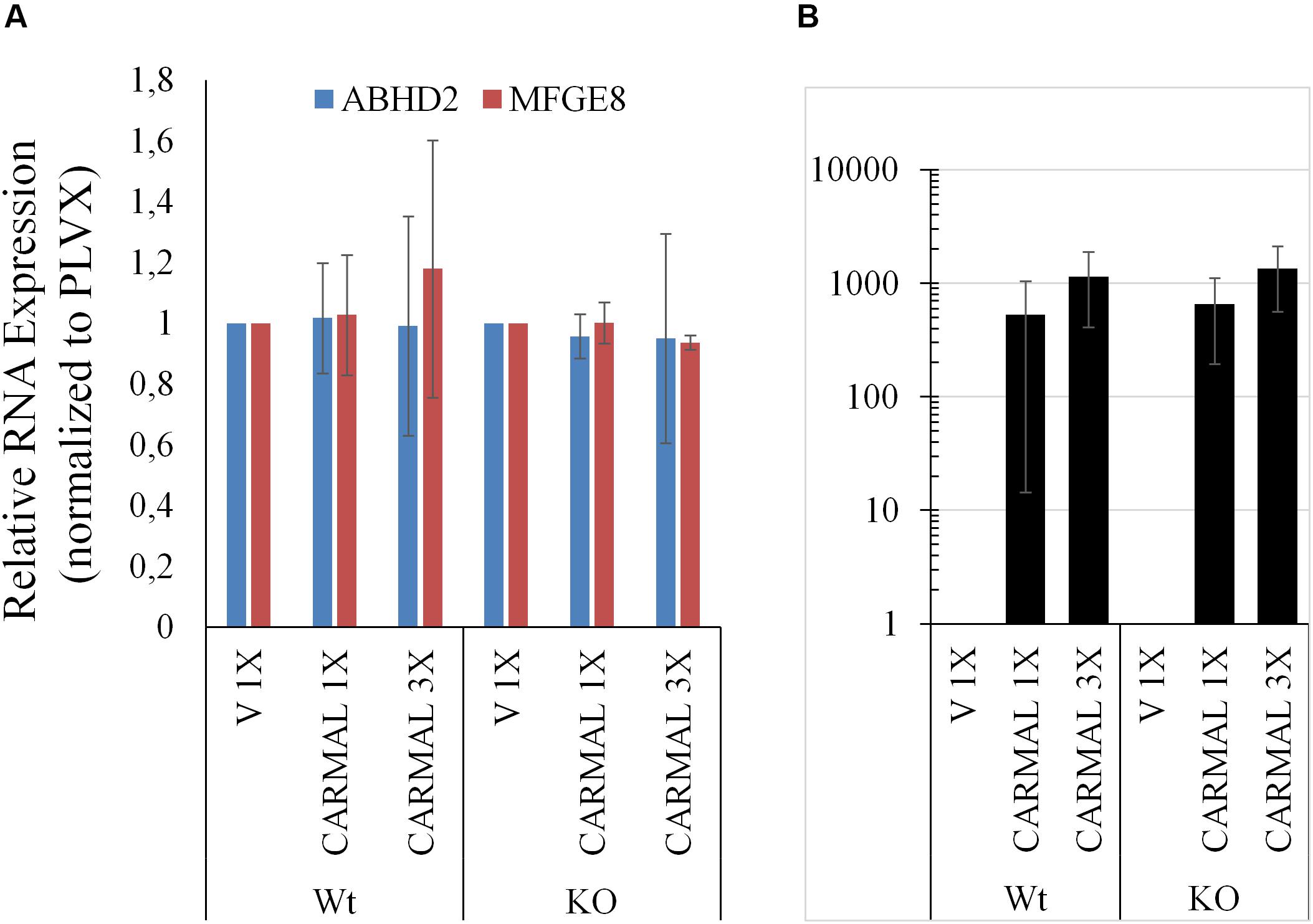
Figure 6. CARMAL overexpression affects neither ABHD2 nor MFGE8 in HEK293T. (A) Cells Wt or deleted for CARMAL (KO), were transduced with lentiviruses expressing CARMAL or control vector (V) for 48 h, were processed for qRT-PCR analysis. (B) CARMAL expression relative to vector infected (V = 1) controls. Results represent the means of three experiments (±SD).
Transcriptome-Wide Impacts of CARMAL Deletion
Analyses thus far pointed to a likely role of the chromatin region in the regulation of local targets, but did not examine putative genome-wide roles of the CARMAL locus. Indeed, any impact CARMAL transcripts might have on local transcription is likely to be mediated indirectly via a feed-back loop involving the cytoplasm since our fractionation results are more in line with a cytosolic role. With the rational that this guilt-by-association approach might provide biological insight, transcription array results from CARMAL-deleted and control cells were contrasted, looking for emerging genome-wide patterns. CARMAL deletion resulted in changes in the abundance of 1.6% (Linear fold change >1.5, p < 0.05) of several transcripts, with a slight non-significant (Fisher’s p = 0.10) bias toward upregulation (433 vs 366). In line with the earlier qPCR results, CARMAL deletion led to an increase in MFGE8 (1.34-fold, p = 0.02) and a reduction in ABHD2 (−1.67-fold, p = 0.003). While HAPLN3 levels increased on average, in agreement with the qPCR results, changes did not reach statistical significance (1.12-fold, p = 0.29).
Over-Representation Analysis of Transcripts Impacted by CARMAL Deletion
To help pinpoint a possible transcriptome-wide trend, over-representation analyses, which hinge on the enrichment of a subset of genes (significantly impacted array hits) within a larger list (entire array list) were first performed. The analysis, focusing on nominally significant hits (p < 0.05, >1.5-liner fold change) and the Reactome and KEGG pathway databases [via WebGestalt (Subramanian et al., 2005; Liao et al., 2019)], failed to detect FDR significant enrichment. The analysis was repeated with a more stringent hit list (FDR significant hits only; 64 unique Entrez Gene IDs) with similar results. This analysis relied on pathway information as defined by Reactome and KEGG and did not examine other informative gene properties (biochemical features, protein modification etc…). To obtain a more comprehensive view of the genes impacted by the deletion, nominally significant hits were subjected to DAVID (Database for Annotation, Visualization and Integrated Discovery), specifically looking for additional properties. Several (9) categories were significantly (FDR <0.05) enriched within this list, including several UniProt tags (UP_KEYWORDS) (Table 1). Interestingly, alternative splicing was the most statistically significant hit by far, indicating that CARMAL deletion disproportionally affects alternatively spliced transcripts although the overall enrichment was low (1.11-fold). In addition, similar numbers of upregulated and downregulated genes were observed in the enriched population (Fisher’s = 0.52). A putative role in splicing was interrogated via a reporter assay consisting of luciferase constructs harboring an internal intron and its matching control (Younis et al., 2010). The assay indeed revealed a difference between Wt and CARMAL KO cell (Figure 7).
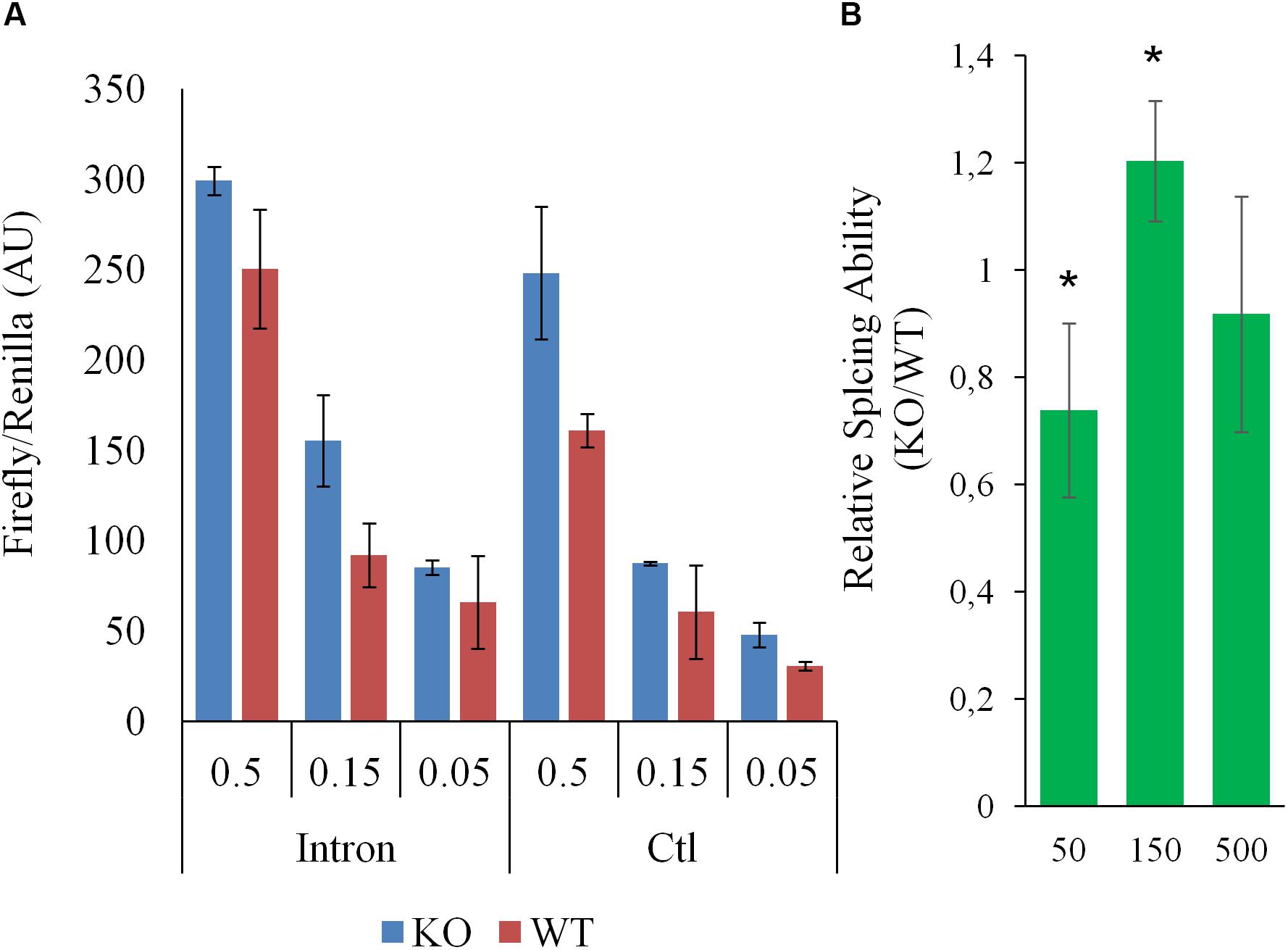
Figure 7. Splicing in WT and CARMAL deleted HEK293T differ. Wild-type (WT) or CARMAL deleted cells in 24 well plates were transfected for 24 h with variable amounts (μg) of either an intron-containing luciferase construct or an intronless control [CMV-LUC2CP/intron/ARE and CMV2-LU2CP/ARE (Addgene)], together with 1% of Renilla internal control. Four independent experiments were performed over a 2 week period. (A) Representative experiment is shown (average of two distinct DNA preparations ± SD). (B) Average ability to process intron (intron/intronless values) of the CARMAL deleted cells relative to the Wt controls, over four experiments (±SD). Values per dose (in ng per well) of transfected DNA are shown.
Gene Set Enrichment, PADOG and Ingenuity Pathway Analysis
One limitation of over-representation analysis is that by focusing on statistically changed genes, it ignores the underlying magnitude and direction of the changes, which carry important biological information. To capture the dynamics of the affected pathways, two ranking analyses were used. First, Gene Set Enrichment Analysis (GSEA) of the entire ranked (fold-change) dataset was performed, again using Reactome mapped pathways via the WebGestalt interface (Table 2 and Supplementary Figure S6). This inquiry revealed that deletion of CARMAL was associated with a possible enrichment in chromatin remodeling in deleted cells, with an FDR significant signal for histone acetylation. To further mine the dataset, PADOG (Pathway Analysis with Down-weighting of Overlapping Genes) was used (Tarca et al., 2012). A unique feature of PADOG is that it weighs genes according to their presence across multiple pathways, favoring less “frequent” (i.e., more pivotal) genes. The analysis resulted in the identification of 28 nominally (P < 0.05) and 11 FDR (q < 0.05) significant KEGG pathways, albeit with no salient uniting theme (Supplementary Table S2).
To help pin down affected pathways, a topology-based analysis was used. Ingenuity (IPA) uses positional information within curated pathways to garner further information, including possible regulators. Ingenuity also uses its own curated “canonical” pathways that were interrogated first. The analysis identified multiple pathways that were nominally affected although none reached FDR (B-H corrected) significance (Supplementary Table S3). Interestingly the top identified pathway (Leucine Degradation I) overlapped with a PADOG FDR significant hit (valine, leucine and isoleucine degradation). The negative z-score suggests a reduced ability to degrade leucine in CARMAL deleted cells. Upstream Regulator Analysis (URA) was then performed to predict candidate regulators compatible with the (nominally) differentially expressed genes. This analysis identified patterns consistent with the implication of several regulators or conditions (Table 3 and Supplementary Table S4). Of particular interest, changes resembled those ensuing from the treatment with three inhibitors (tazemetostat, decitabine, SP2509) targeting (de)methylation processes, indicative of possible repercussions of CARMAL deletion on genome-wide methylation. The most statistically significant regulator was SBDS (Shwachman-Bodian-Diamond Syndrome), a regulator of ribosomal maturation (Weis et al., 2015). Transcription array data however, indicated that SDBS was not significantly affected at the transcript level. Possible post-transcriptional regulation was assessed by Western blotting, that revealed no significant difference in SBDS level across both cell populations (Supplementary Figure S7).
Discussion
This work examines a reported but largely uncharacterized lncRNA locus, CARMAL/RP11-326A19.4. In a previous study, we showed the corresponding transcript to be somewhat conserved but only superficially explored its function. The current study explores the functional aspects of CARMAL, both locally and genome-wide. At the local level, we show that transcript levels of gene neighbors are affected by targeting the CARMAL locus, although the mechanisms require further clarification. We demonstrate that CARMAL is enriched in the cytosol; while attempts to support our CARMAL fractionation data via microscopic approaches were unsuccessful, cytosolic localization was supported by bioinformatic evidence. Of note, a recent large scale bioinformatic analysis indicates that lncRNA subcellular localization is largely conserved across cell types (Gudenas and Wang, 2018). Notwithstanding its classification as a lncRNA, the possibility remains that the CARMAL transcript encodes bioactive short peptides, which in turn may regulate nuclear function. Indeed, examination of ribosome profiling data from GWIPS-viz (Michel et al., 2014) indicated a weak ribosome association to the identified exons in HEK293T cells, particularly around exon 1 (Supplementary Figure S8). However, in accordance with its classification as a lncRNA, Protein BLAST or the Web CD-Search tool1 failed to identify any sequence or domain pattern in the predicted amino acid sequences, casting doubt on the biological relevance of any putative peptide output (Supplementary Figure S8).
Primary differentiated cell models are inadequate as experimental models for CRISPR-related experiments due to their poor transfectability and finite proliferative capacity. Our choice of HEK293T to explore CARMAL function was based on several criteria: a human origin, significant expression of CARMAL, euploidy over the entire CARMA region (as per ENCODE/HAIB data), high transfection efficiency compatible with large CRISPR plasmids, a successful history of CRISPR editing, as well as robust growth. Use of HEK293T comes at the expense of a reduced a priori translatability to normal tissues and some findings will have to be validated in cells more pertinent to CAD. No cellular model is perfect however. For one, the normal arterial vasculature consists of several cell types and while smooth muscle cells constitute the bulk of the cell mass, other cells populations may contribute to CARMAL expression than their relative abundance (Roostalu and Wong, 2018). In addition, smooth muscle cells are phenotypically plastic and adapt to their environment by adjusting their transcriptional profile to ex vivo conditions (Alexander and Owens, 2012).
CARMAL was expressed at relatively low levels in HEK293T and in two vascular cell models. These results are in line with GTEx quantification in arteries (in the low transcripts per million range), indicating that low expression is a feature of its biological role. Low expression contrasts with the observation that the CARMAL promoter region is active in promoter trapping assays (Soubeyrand et al., 2019a) suggesting that the promoter region is under tight repressive control in its native environment. Interestingly common genetic variants (e.g., rs2083460) that are expression quantitative trait loci (eQTL) for CARMAL show eQTL effects for MFGE8 expression in coronary vessels (GTEx), indicating that the region is under concerted regulation in vascular tissues. Thus polymorphisms that associate with MFGE8 expression may exert this effect, in part, by altering CARMAL expression. Since reducing CARMAL expression increases MFGE8, a normal function of CARMAL might be to moderate MFGE8 expression, which we previously demonstrated correlated with CAD (Soubeyrand et al., 2019b). One related question of interest is the impact of CAD and its progression on vascular expression of CARMAL and MFGE8. As CARMAL is not conserved in rodent models, answering that question will require interrogating diseased and control coronary tissue from human cohorts.
Upregulation of MFGE8 required substantial suppression of the CARMAL locus, achieved by one of the 3 types of KRAB derivatives tested. As all three CRISPRi effectors are targeted to the same (intronic) regions of CARMAL, and thus are expected to similarly perturb local chromatin, additional factors are likely to be at work. One simple interpretation is that CARMAL must be reduced by >∼60% in order to affect MFGE8, and thus that a minimal level of CARMAL >∼40% is needed for its expression. This latter explanation however, fails to account for the inability of transduced CARMAL to increase (i.e., restore) MFGE8 in KO cells. Alternatively, the type of KRAB derivative may impart changes to the region more conducive to MFGE8 transcription, independent of its impact on CARMAL expression although it is unclear how a repressor such as KRAB-MeCP2 could activate local transcription directly. In summary, these findings indicate that CARMAL transcription represses MFGE8 under basal conditions and point to the importance of the CARMAL locus proper, rather than its transcript, in this process.
Ontology analysis uncovers potential differences in RNA splicing following CARMAL deletion. This finding is further supported by direct evidence from a reporter assay demonstrating a non-linear difference in the ability of these cells to effect splicing. Unfortunately the transcript arrays used to interrogate our differential expression samples do not carry splicing variants information. Beyond supporting a possible role in splicing, how this dose-dependence in splicing ability translates to the natural splicing environment is unclear. Additional experiments will be needed to first ascertain whether CARMAL is directly involved or this represents a cell adaptation to the genetic editing or a stochastic genetic drift.
Impact analysis (URA) reveals expression changes consistent with a contribution of SBDS as a result of CARMAL deletion. Through URA we previously identified HNF4A as downstream effector of TRIB1 (Soubeyrand et al., 2017). The evidence for SBDS involvement is derived from RNA expression data derived from SDBS siRNA experiments performed in HEK293T, the main cell type used in this study. Given that our expression array data indicate that SBDS levels are unaffected by CARMAL deletion, CARMAL may regulate SBDS post-transcriptionally. Although we did not find a significant impact on SBDS protein level, the absence of CARMAL may affect its function via changes in post-translational modifications (PTM). Multiple PTM within SBDS have been identified via proteome-wide analyses, but further validation and functional studies are required2. Dysfunctional SBDS can lead to the Shwachman-Bodian-Diamond syndrome, a recessive disease defined by a complex suite of clinical features including pancreatic dysfunction, short stature and leukemia (Boocock et al., 2003; Warren, 2018). In human cohorts, an intronic variant in SBDS (rs12667745) is associated with peripheral vascular disease and respiratory disorders (UKBB data accessed through phenoscanner3). At the cellular level, SBDS has been identified as a key regulator of late (cytoplasmic) ribosomal assembly and has been reported to shuttle across the nuclear membrane (Finch et al., 2011; Orelio et al., 2011; Weis et al., 2015). Moreover SBDS may play multiple roles. For instance, nuclear forms of SBDS appear to contribute to the maintenance of telomere integrity (Liu et al., 2018). Given its low expression, CARMAL is not expected to play a major role in ribosomal maturation. A putative functional interaction between CARMAL and SBDS is likely restricted to other processes but remains an intriguing possibility that we are currently exploring.
Methods
Cell Culture, Transfection and Transductions
Lentiviral particles were generated using HEK293FT using pLVX, pSPAX2 and pMD2. G (from Addgene). Supernatants were filtered through 0.4 μM filters, titered using qPCR assays of viral cDNAs and stored at −80°C. HEK239T cells were transfected with expression plasmids using Lipofectamine 3000 [1:2:3 ratio of DNA (μg): P3000 (μl):lipofectamine 3000 (μl) reagent] or RNAiMax. Unless specified, 0.5 μg DNA was used per 200 mm2 and 70% confluent cells. Oligonucleotide transfection was performed using lipofectamine RNAiMax (ThermoFisher) and 10 nM final concentration.
CRISPR-Mediated KO of CARMAL
Deletion was performed on HEK293T cells using gRNA flanking the promoter and exon 1 of CARMAL. The gRNA vector was derived from pCR2.0 by insertion of a U6 promoter-tracrRNA cassette from pX330-U6-Chimeric_BB-CBh-hSpCas9 (Cong et al., 2013). Single clones were obtained by fluorescent assisted cell sorting, were expanded and screened using a step-wise approach. Deletions were first screened by real time PCR looking for loss of signal over the deleted region, followed by a positive selection using primers spanning the deletion. Out of 100 screened clonal populations, bi-allelic deletion was observed in a single clone. Sanger sequencing of the clone revealed that one allele was deleted as intended while the other retained a short (∼80 bp) promoter fragment that was reintroduced in the reverse orientation. See Supplementary Data Sheet 3 for sgRNA sequence information.
CRISPRi and CRISPRa
Plasmids used for CRISPRa and CRISPRi were obtained from Addgene. Specifically dCas9-KRAB-MeCP2 (#110821), dCas9-FOG1[N + C] (#100085), dCas9-KRAB (#110820); CRISPRa was a VPR derivative (SP-dCas9-VPR; #63798) (Chavez et al., 2015; O’Geen et al., 2015; Yeo et al., 2018). For a typical experiment, the dCAS9 plasmid was co-transfected at a mass ratio of 7:3 together with a sgRNA plasmid (pCRU6) expressing the single-guide RNA driven by a U6 promoter; for a 12-well plate format, wells were transfected with either 0.5 μg (CRISPRa) or 1 μg (CRISPRi). Transfections were performed for 48–72 h as indicated. Guide sequence information are detailed in Supplementary Data Sheet 3.
PCR and qRT-PCR
For standard PCR analysis, DNA samples were analyzed using Terra PCR direct for 35–40 cycles as suggested by the supplier (Takara Bio). Quantitative (RT-) PCR analyses were used using the SYBR Green I Master Mix on a LightCycler 480 (Roche) and a 65–55°C touchdown over 45 cycles (30 s extension). For cDNA synthesis, mRNA was first isolated using the High Pure RNA Isolation Kit (Roche), followed by cDNA was synthesis using the Transcriptor First Strand cDNA Synthesis Kit (Roche) according to the supplier’s protocol, including a 5 min 65°C denaturation/annealing step and a 50°C (1 h) reverse transcription step. Unless mentioned otherwise, a typical reaction included 0.1–0.5 μg of RNA and a 1:1 mixture of an anchored oligo(dT)18 oligomer and random hexamers. For Droplet Digital RT-PCR, cDNA was first generated according to the standard RT protocol and mixed with QX200 ddPCR EvaGreen Supermix (Biorad) following the manufacturer’s protocol. Droplets were prepared using QX200 droplet generation oil on a QX200 Droplet Generator (Biorad) and subjected to PCR using a C1000 thermocycler (BioRad) as follows: 95°C for 5 min, followed by 45 cycles of 95°C for 30 s at a ramp rate of 2°C/s and 60°C 1 min extensions at a ramp rate of 2°C/s. Positive/negative droplets were counted by a QX200 Droplet Reader (BioRad). All primers are described in Supplementary Data Sheet 3.
Transcriptomics
Transcriptome analyses were performed at the TCAG (Toronto) using Human ST 2.0 arrays. All mRNAs were purified via High Pure RNA isolation spin columns (Roche). For Knock-out analysis, three distinct wild type populations were mixed and compared with the CARMAL deleted clone. RNAs harvested over four passages were processed individually for array analysis, for a total of eight analyzed samples. For CRISPRa, three independent transfections were analyzed. DataSets and experimental details can be found at the Gene Expression Omnibus: GSE142097 and GSE142098 for the activation and deletion datasets, respectively.
RNA Fractionation Analysis
Samples were fractionated essentially as described previously (Rinn et al., 2007) and RNA therein isolated (High Pure RNA isolation kit, Roche). RNA (0.2–0.5 μg) was then converted to cDNA (First Strand RNA Transcriptor, Roche) and quantified by qRT-PCR. Nuclear enrichment was then quantified using the deltaCt method, subtracting the cytosolic from the nuclear signal Ct values (deltaCt). Nuclear enrichment was then 2ExpdeltaCt, with values over 1 reflecting respectively nuclear enrichment and below 1, cytosolic enrichment.
Bioinformatic Analysis
Multiple approaches were used with the rational that coherent results emerging from complementary approaches should be more robust findings. DAVID (Huang et al., 2009) was used using default settings with the inclusion of Reactome and UP_Tissue datasets. The whole array list (Human ST 2.0) was used as background. WebGestalt GSEA analyses were performed using the default settings. GSEA is a rank based method that utilizes a priori defined gene set information (pathways) to identify the most impacted biological processes (Subramanian et al., 2005). Although related to GSEA (ranked and enrichment based), PADOG uses a weighting function that favors genes that tend to be enriched in particular gene sets and downweights genes that are shared across gene sets, with the rational that these genes play more central roles and are therefore more likely to impact any given pathway when perturbed. Moreover, PADOG uses a different gene-scoring method. The approach is more sensitive, possibly at the expense of reduced specificity (Nguyen et al., 2019). PADOG (3.1) was run in R (3.6) using default settings. For IPA (Ingenuity), a list of 48227 Affymetrix IDs were submitted, which were mapped to 30067 IPA addresses. Using a nominal (0.05 cutoff) filter resulted in 3035 molecules that were analyzed by core analysis using the default settings.
Data Availability Statement
The datasets generated for this study can be found in the Gene Expression Omnibus (GEO): GSE142097 and GSE142098.
Author Contributions
SS and RM planned and designed the research. SS, PL, H-DH, and AT conducted the experiments. TA provided logistical assistance. MN conducted the PADOG analysis. SS and RM wrote the manuscript. All authors contributed to the article and approved the submitted version.
Funding
This work was made possible by the Canadian Institutes of Health Research through a Foundation grant (FRN:154308) held by RM.
Conflict of Interest
The authors declare that the research was conducted in the absence of any commercial or financial relationships that could be construed as a potential conflict of interest.
Supplementary Material
The Supplementary Material for this article can be found online at: https://www.frontiersin.org/articles/10.3389/fgene.2020.00631/full#supplementary-material
Footnotes
- ^ https://www.ncbi.nlm.nih.gov/Structure/bwrpsb/bwrpsb.gi
- ^ https://www.phosphosite.org/proteinAction?id=17958&showAllSites=true
- ^ http://www.phenoscanner.medschl.cam.ac.uk/
References
Alexander, M. R., and Owens, G. K. (2012). Epigenetic control of smooth muscle cell differentiation and phenotypic switching in vascular development and disease. Annu. Rev. Physiol. 74, 13–40. doi: 10.1146/annurev-physiol-012110-142315
Böhmdorfer, G., and Wierzbicki, A. T. (2015). Control of chromatin structure by long noncoding RNA. Trends Cell Biol. 25, 623–632. doi: 10.1016/j.tcb.2015.07.002
Boocock, G. R. B., Morrison, J. A., Popovic, M., Richards, N., Ellis, L., Durie, P. R., et al. (2003). Mutations in SBDS are associated with Shwachman-Diamond syndrome. Nat. Genet. 33, 97–101. doi: 10.1038/ng1062
Cao, Z., Pan, X., Yang, Y., Huang, Y., and Shen, H. B. (2018). The lncLocator: a subcellular localization predictor for long non-coding RNAs based on a stacked ensemble classifier. Bioinformatics 34, 2185–2194. doi: 10.1093/bioinformatics/bty085
Chavez, A., Scheiman, J., Vora, S., Pruitt, B. W., Tuttle, M., Iyer, E. P. R., et al. (2015). Highly efficient Cas9-mediated transcriptional programming. Nat. Methods 12, 326–328. doi: 10.1038/nmeth.3312
Cong, L., Ran, F. A., Cox, D., Lin, S., Barretto, R., Habib, N., et al. (2013). Multiplex genome engineering using CRISPR/Cas systems. Science 339, 819–823. doi: 10.1126/science.1231143
Derrien, T., Johnson, R., Bussotti, G., Tanzer, A., Djebali, S., Tilgner, H., et al. (2012). The GENCODE v7 catalog of human long noncoding RNAs: analysis of their gene structure, evolution, and expression. Genome Res. 22, 1775–1789. doi: 10.1101/gr.132159.111
Diederichs, S. (2014). The four dimensions of noncoding RNA conservation. Trends Genet. 30, 121–123. doi: 10.1016/j.tig.2014.01.004
Engreitz, J. M., Haines, J. E., Perez, E. M., Munson, G., Chen, J., Kane, M., et al. (2016). Local regulation of gene expression by lncRNA promoters, transcription and splicing. Nature 539, 452–455. doi: 10.1038/nature20149
Finch, A. J., Hilcenko, C., Basse, N., Drynan, L. F., Goyenechea, B., Menne, T. F., et al. (2011). Uncoupling of GTP hydrolysis from eIF6 release on the ribosome causes shwachman-diamond syndrome. Genes Dev. 25, 917–929. doi: 10.1101/gad.623011
Gudenas, B. L., and Wang, L. (2018). Prediction of LncRNA subcellular localization with deep learning from sequence features. Sci. Rep. 8:16385. doi: 10.1038/s41598-018-34708-w
Huang, D. W., Sherman, B. T., and Lempicki, R. A. (2009). Systematic and integrative analysis of large gene lists using DAVID bioinformatics resources. Nat. Protoc. 4, 44–57. doi: 10.1038/nprot.2008.211
Liao, Y., Wang, J., Jaehnig, E. J., Shi, Z., and Zhang, B. (2019). WebGestalt 2019: gene set analysis toolkit with revamped UIs and APIs. Nucleic Acids Res. 47, W199–W205. doi: 10.1093/nar/gkz401
Liu, F. (2017). Enhancer-derived RNA: a primer. Genomics Proteomics Bioinformatics 15, 196–200. doi: 10.1016/j.gpb.2016.12.006
Liu, Y., Liu, F., Cao, Y., Xu, H., Wu, Y., Wu, S., et al. (2018). Shwachman-diamond syndrome protein SBDS maintains human telomeres by regulating telomerase recruitment. Cell Rep. 22, 1849–1860. doi: 10.1016/j.celrep.2018.01.057
Michel, A. M., Fox, G., Kiran, A. M., De Bo, C., O’Connor, P. B. F., Heaphy, S. M., et al. (2014). GWIPS-viz: development of a ribo-seq genome browser. Nucleic Acids Res. 42, D859–D864. doi: 10.1093/nar/gkt1035
Møller, M., Strand, S. H., Mundbjerg, K., Liang, G., Gill, I., Haldrup, C., et al. (2017). Heterogeneous patterns of DNA methylation-based field effects in histologically normal prostate tissue from cancer patients. Sci. Rep. 7:40636. doi: 10.1038/srep40636
Nguyen, T.-M., Shafi, A., Nguyen, T., and Draghici, S. (2019). Identifying significantly impacted pathways: a comprehensive review and assessment. Genome Biol. 20:203. doi: 10.1186/s13059-019-1790-4
Noh, J. H., Kim, K. M., McClusky, W. G., Abdelmohsen, K., and Gorospe, M. (2018). Cytoplasmic functions of long noncoding RNAs. Wiley Interdiscip. Rev. RNA 9:e1471. doi: 10.1002/wrna.1471
O’Geen, H., Henry, I. M., Bhakta, M. S., Meckler, J. F., and Segal, D. J. (2015). A genome-wide analysis of Cas9 binding specificity using ChIP-seq and targeted sequence capture. Nucleic Acids Res. 43, 3389–3404. doi: 10.1093/nar/gkv137
Orelio, C., van der Sluis, R. M., Verkuijlen, P., Nethe, M., Hordijk, P. L., van den Berg, T. K., et al. (2011). Altered intracellular localization and mobility of SBDS protein upon mutation in Shwachman-Diamond syndrome. PLoS One 6:e20727. doi: 10.1371/journal.pone.0020727
Ransohoff, J. D., Wei, Y., and Khavari, P. A. (2017). The functions and unique features of long intergenic non-coding RNA. Nat. Rev. Mol. Cell Biol. 19, 143–157. doi: 10.1038/nrm.2017.104
Raymond, A., Ensslin, M. A., and Shur, B. D. (2009). SED1/MFG-E8: a bi-motif protein that orchestrates diverse cellular interactions. J. Cell. Biochem. 106, 957–966. doi: 10.1002/jcb.22076
Rinn, J. L., Kertesz, M., Wang, J. K., Squazzo, S. L., Xu, X., Brugmann, S. A., et al. (2007). Functional demarcation of active and silent chromatin domains in human HOX loci by noncoding RNAs. Cell 129, 1311–1323. doi: 10.1016/j.cell.2007.05.022
Roostalu, U., and Wong, J. K. (2018). Arterial smooth muscle dynamics in development and repair. Dev. Biol. 435, 109–121. doi: 10.1016/j.ydbio.2018.01.018
Soubeyrand, S., Lau, P., Peters, V., and McPherson, R. (2019a). Off-target effects of CRISPRa on interleukin-6 expression. PLoS One 14:e0224113. doi: 10.1371/journal.pone.0224113
Soubeyrand, S., Martinuk, A., and McPherson, R. (2017). TRIB1 is a positive regulator of hepatocyte nuclear factor 4-Alpha. Sci. Rep. 7:5574. doi: 10.1038/s41598-017-05768-1
Soubeyrand, S., Nikpay, M., Turner, A., Dang, A.-T., Herfkens, M., Lau, P., et al. (2019b). Regulation of MFGE8 by the intergenic coronary artery disease locus on 15q26.1. Atherosclerosis 284, 11–17. doi: 10.1016/j.atherosclerosis.2019.02.012
Subramanian, A., Tamayo, P., Mootha, V. K., Mukherjee, S., Ebert, B. L., Gillette, M. A., et al. (2005). Gene set enrichment analysis: a knowledge-based approach for interpreting genome-wide expression profiles. Proc. Natl. Acad. Sci. U.S.A. 102, 15545–15550. doi: 10.1073/pnas.0506580102
Tarca, A. L., Draghici, S., Bhatti, G., and Romero, R. (2012). Down-weighting overlapping genes improves gene set analysis. BMC Bioinformatics 13:136. doi: 10.1186/1471-2105-13-136
Wang, M., Herrmann, C. J., Simonovic, M., Szklarczyk, D., and von Mering, C. (2015). Version 4.0 of PaxDb: protein abundance data, integrated across model organisms, tissues, and cell-lines. Proteomics 15, 3163–3168. doi: 10.1002/pmic.201400441
Warren, A. J. (2018). Molecular basis of the human ribosomopathy Shwachman-diamond syndrome. Adv. Biol. Regul. 67, 109–127. doi: 10.1016/j.jbior.2017.09.002
Weis, F., Giudice, E., Churcher, M., Jin, L., Hilcenko, C., Wong, C. C., et al. (2015). Mechanism of eIF6 release from the nascent 60S ribosomal subunit. Nat. Struct. Mol. Biol. 22, 914–919. doi: 10.1038/nsmb.3112
Yang, F., Yi, F., Han, X., Du, Q., and Liang, Z. (2013). MALAT-1 interacts with hnRNP C in cell cycle regulation. FEBS Lett. 587, 3175–3181. doi: 10.1016/j.febslet.2013.07.048
Yeo, N. C., Chavez, A., Lance-Byrne, A., Chan, Y., Menn, D., Milanova, D., et al. (2018). An enhanced CRISPR repressor for targeted mammalian gene regulation. Nat. Methods 15, 611–616. doi: 10.1038/s41592-018-0048-5
Younis, I., Berg, M., Kaida, D., Dittmar, K., Wang, C., and Dreyfuss, G. (2010). Rapid-response splicing reporter screens identify differential regulators of constitutive and alternative splicing. Mol. Cell. Biol. 30, 1718–1728. doi: 10.1128/mcb.01301-09
Keywords: lncRNA, CARMAL, RP11-326A19.4, MFGE8, gene expression, transcription, SBDS
Citation: Soubeyrand S, Nikpay M, Lau P, Turner A, Hoang H-D, Alain T and McPherson R (2020) CARMAL Is a Long Non-coding RNA Locus That Regulates MFGE8 Expression. Front. Genet. 11:631. doi: 10.3389/fgene.2020.00631
Received: 10 February 2020; Accepted: 26 May 2020;
Published: 17 June 2020.
Edited by:
Graziano Pesole, National Research Council, ItalyReviewed by:
Claudio Santoro, University of Eastern Piedmont, ItalyEmmanuel Dias-Neto, A.C.Camargo Cancer Center, Brazil
Copyright © 2020 Soubeyrand, Nikpay, Lau, Turner, Hoang, Alain and McPherson. This is an open-access article distributed under the terms of the Creative Commons Attribution License (CC BY). The use, distribution or reproduction in other forums is permitted, provided the original author(s) and the copyright owner(s) are credited and that the original publication in this journal is cited, in accordance with accepted academic practice. No use, distribution or reproduction is permitted which does not comply with these terms.
*Correspondence: Sébastien Soubeyrand, ssoubeyrand@ottawaheart.ca; Ruth McPherson, rmcpherson@ottawaheart.ca