- 1Animal Genetics Division, ICAR-Indian Veterinary Research Institute, Izatnangar, Bareilly, India
- 2Livestock Production and Management, Indian Council of Agricultural Research (ICAR)-Indian Veterinary Research Institute, Bareilly, India
- 3Animal Biotechnology, Indian Council of Agricultural Research (ICAR)-Indian Veterinary Research Institute, Bareilly, India
India is home to a large and diverse buffalo population. The Murrah breed of North India is known for its milk production, and it has been used in breeding programs in several countries. Selection signature analysis yield valuable information about how the natural and artificial selective pressures have shaped the genomic landscape of modern-day livestock species. Genotype information was generated on six buffalo breeds of India, namely, Murrah, Bhadawari, Mehsana, Pandharpuri, Surti, and Toda using ddRAD sequencing protocol. Initially, the genotypes were used to carry out population diversity and structure analysis among the six breeds, followed by pair-wise comparisons of Murrah with the other five breeds through XP-EHH and FST methodologies to identify regions under selection in Murrah. Admixture results showed significant levels of Murrah inheritance in all the breeds except Pandharpuri. The selection signature analysis revealed six regions in Murrah, which were identified in more than one pair-wise comparison through both XP-EHH and FST analyses. The significant regions overlapped with QTLs for milk production, immunity, and body development traits. Genes present in these regions included SLC37A1, PDE9A, PPBP, CXCL6, RASSF6, AFM, AFP, ALB, ANKRD17, CNTNAP2, GPC5, MYLK3, and GPT2. These genes emerged as candidates for future polymorphism studies of adaptability and performance traits in buffaloes. The results also suggested ddRAD sequencing as a useful cost-effective alternative for whole-genome sequencing to carry out diversity analysis and discover selection signatures in Indian buffalo breeds.
Introduction
Water buffalo is considered as an important livestock resource in tropical and sub-tropical countries due to its high milk production ability along with adaptability to hot and humid environment, and high feed conversion efficiency (Kumar et al., 2019). Buffaloes are the major contributors of milk production in India accounting for 49.2% of 187.7 million tons of total milk production (DAHD&F, 2018). India possesses a remarkably large and diverse buffalo population with 109.85 million buffaloes and 17 registered breeds (DAHD&F, 2018; NBAGR Karnal, 2021).
Murrah is the most important buffalo breed of India, constituting about 44.3% of the total buffalo population of the country. The main breeding area of this breed is the northern states of India, namely Punjab, Haryana, and Western Uttar Pradesh. Due to its high milk potential in varied environmental conditions, the germplasm of the breed has been extensively used throughout the country. It has also been imported in several countries like China, Brazil, Vietnam, Egypt, Bangladesh, etc., due to its higher milk production potential (Zhang et al., 2020). As part of the breed improvement schemes, Murrah buffalo has been selected for improved milk production for the past 30 years, and the process is going on. By investigation of selection sweeps in the Murrah genome, we may gain insights into the genes and genomic regions related to important economic traits in buffaloes. Recently, Dutta et al. (2020) identified selection sweeps in seven Indian riverine buffaloes and compared patterns of between-species selective sweeps with different cattle breeds using whole-genome sequencing (WGS) data. Since WGS is a costly process, several workers have proposed reduced representation genotyping techniques such as the double digest restriction site-associated DNA sequencing (ddRAD-seq) as a useful alternative to WGS for genotyping Indian buffaloes (Surya et al., 2019; Mishra et al., 2020). For the present study, the genotype data of six Indian buffalo breeds (Murrah, Surti, Mehsana, Bhadawari, Pandharpuri, and Toda) was generated using ddRAD sequencing.
This study aimed to assess the genetic diversity and population structure among the six Indian buffalo breeds using ddRAD data. Furthermore, we attempted to unravel signatures of positive selection in Murrah by comparing it with other reference Indian breeds (Surti, Mehsana, Bhadawari, Pandharpuri, and Toda) through cross-population extended haplotype homozygosity (XP-EHH) and cross-population fixation index (FST) approaches.
Material and Methods
Sample Collection and Generation of Double Digest Restriction Site-Associated DNA Data
Ninety-six samples were collected from six breeds of riverine buffalo from different parts of India. These breeds are diverse in terms of physical features, milk production, and adaptation. Selection of the animals was done in a way to cover the genepool of the respective breeds. So the animals of all the breeds in the present study were chosen randomly from their respective institutional farms (except animals of the Toda breed of buffalo for which random samples were collected from its breeding tracts in the Nilgiri Hills area of Tamilnadu state of India). As the Murrah breed is mainly found in the northern part of India, the random samples were collected from three institutional farms of the area, i.e., the Livestock Research Station (LRS) ICAR-IVRI situated in Izatnagar, Bareilly (Uttar Pradesh), the Buffalo Farm at livestock research station of GBPUA and T, Pantnagar (Uttarakhand), and the Livestock Farm, GADVASU Ludhiana. The samples of Bhadawari buffalo were collected from the Buffalo Farm, ICAR-IGFRI, Jhansi (Uttar Pradesh), Mehsana buffalo samples were collected from the Livestock Research Station, SDAU, SK Nagar (Gujarat), Surti buffalo samples were collected from the Livestock Research Station, CVAS, Udaipur (Rajasthan), and Pandharpuri buffalo samples were collected from the Buffalo Farm, Zonal Agriculture Research Station, Kolhapur (Maharashtra). All these farms are situated in their respective breeding tract, and animals were randomly selected from these institution farms as to cover substantially the genepool of the population. The breed-wise details of sample numbers and location are also provided in Supplementary Table S1. Whole-blood samples were collected from the jugular vein of the animals in 10-ml vacutainers under aseptic condition, and genomic DNA was extracted using the standard phenol–chloroform method (Sambrook and Russell, 2006). The concentration and purity of the DNA were measured using agarose gel electrophoresis and NanoDrop spectrophotometer. Following the ddRAD protocol (Peterson et al., 2012), the double digestion of genomic DNA was carried out using Sph I and MluC I enzymes as mentioned in Kumar et al. (2020), and the samples were sequenced on Illumina Hi-seq 2000 platform to generate 150-bp reads.
Quality Control and Variant Calling
The reads were quality checked using FastQC (Andrews, 2010). Trimming of Illumina universal adapters and quality filtering was performed by the process_radtags function of the STACKS v2 software (Rochette et al., 2019). Reads were examined using a sliding window spanning 15% of the read length, and the reads having average phred score of <15 were discarded. The barcode of the reads was removed using Cutadapt 2.10 (Martin, 2011).
The paired reads were aligned to the Bubalus bubalis assembly UOA_WB_1 downloaded from NCBI (Low et al., 2019; https://www.ncbi.nlm.nih.gov/assembly/GCF_003121395.1/) using BWA-MEM 0.7.17 (Li, 2013) with default settings. The percentage of reads aligning to the reference genome was determined by Samtools (v1.7) flagstats (Li et al., 2009) function. Variant calling was performed through the bcftools mpileup utility of the Samtoolsv1.7 suite in a multi-sample mode as recommended by Wright et al. (2019). SNPs with quality score greater than 30 and a read depth of 10 were retained for further analysis.
The structural and functional annotation of the retained SNPs was performed using SnpEff v4.3 (Cingolani et al., 2012). Quality filtering of the annotated variants was performed by removing unmapped and non-autosomal SNPs. SNPs missing in more than 25% of the individuals and below the minor allele frequency (MAF) threshold of 0.01 were also filtered out using PLINK 1.9 (Purcell et al., 2007). Genotype imputation of sporadically missing genotypes was done using Beagle 4.1 (Browning and Browning, 2016).
Genetic Diversity and Population Structure Analysis
Linkage disequilibrium (LD) pruning of the SNPs was carried out using the indep-pairwise command parameters (indep-pairwise 50 5 0.2) of the PLINK software. The observed (Ho) and expected (He) heterozygosities for different buffalo breeds were estimated using PLINK 1.9. Furthermore, admixture analysis was performed on the LD pruned data for K values ranging from K = 2 to K = 6 using ADMIXTURE 1.3 software (Alexander et al., 2009). The results of the admixture analysis were visualized using PONG (Behr et al., 2016). A genomic relationship matrix was prepared in GCTA (Yang et al., 2011), and the first 10 principal components were extracted. The top principal components were plotted in R (R Core team, 2018) to visualize population clustering. A maximum-likelihood phylogram was constructed using TREEMIX (Pickrell and Pritchard, 2012) to infer the ancestral relationships and migration patterns between the breeds.
Analysis of Selection Signatures
Cross-population selection signatures between Murrah buffalo and five other Indian water buffalo breeds (Bhadawari, Surti, Mehsana, Pandharpuri, and Toda) were derived using XP-EHH (Sabeti et al., 2007) and FST (Weir and Cockerham, 1984) methodologies. The genotypic data of all the breeds were phased using BEAGLE v5.1 (Browning et al., 2018) using default settings (burnin = 6; iterations = 12; and phase-states = 280). The XP-EHH scores of the Murrah buffalo were calculated for each breed comparison using the R package rehh (Gautier et al., 2017), taking the other water buffalo breeds in the study as the reference populations. To detect positive selection, average XP-EHH scores were computed for 100-kb regions with a 50-kb overlap. Regions with absolute XP-EHH scores of four or above were considered as putative candidate regions in Murrah.
The pairwise FST estimates between the Murrah and other buffalo breeds were calculated with VCFTOOLS (Danecek et al., 2011), with a sliding window of 100 kb and a 50-kb step size. Windows belonging to the top 0.1% of the FST values were considered as potential regions under selection (Singh et al., 2020).
The candidate genes in the selected regions were annotated using the GTF (gene transfer format) file supplied with the UOA_WB_1 assembly, using BEDTools (Quinlan and Hall, 2010) intersect function. Each putative selected region was cross-referenced with the literature to find previously detected regions of functional importance.
Results
In the present study, total 397.8 million paired-end reads of 150-bp length were obtained for the 96 buffalo breeds, averaging 4.14 million reads per sample. After initial quality control, a total of 367.2 million reads (92.3% of the total reads) of average 135-bp length were retained. The average alignment rate of the reads was 99.82% with the reference genome. Sample-wise alignment percentages are given in Supplementary Table S2. A total of 569,535 variants were identified, out of which 502,476 were SNPs and 67,059 were indels. A total of 551,458 variants were present on autosomes, 15,315 on the X chromosome and 12 on the mtDNA, and 2,750 variants were located on unmapped contigs (Supplementary Table S3). A variant was discovered for every 4,637 bp of the genome length. The total number of SNPs and indels of each buffalo breed at read depth 10 is mentioned in Supplementary Table S4. The highest number of SNPs was found for the Mehsana (489,738) buffalo followed by the Murrah buffalo (484,449), and lowest for the Toda buffalo (448,714). After quality control and imputation of sporadically missing genotypes, a total of 237,762 SNPs, which were common across all the breeds, were used for downstream analysis.
Genome-wide Annotation of SNPs in Water Buffalo Breeds
Based on the sequence ontology terms, a greater number of identified SNPs were located within the intronic regions (66.57%), followed by the intergenic regions (22.13%), and 0.34% of SNPs were found to be located in the transcript region (Supplementary Figure S1). The impact-wise and region-wise distribution of variant effects, as generated by SNPeff, are given in Supplementary Table S5.
About 71.89% of the annotated SNPs were identified as transitions (Ts) while 28.10% as transversions (Tv) with a TS/TV ratio of 2.5578. The Ts/Tv ratio serves as a quality control indicator of high-throughput sequencing data. Our values are consistent with previous reports of targeted sequencing methods in buffalo (Surya et al., 2019; Kumar et al., 2020).
Genetic Diversity
For the genetic diversity and population structure analyses, we used a subset of 67,798 SNPs after pruning the SNPs in LD. The average observed heterozygosity (Ho) and expected heterozygosity (He) of all breeds in the study are presented in Table 1. The Ho and He was found highest for the Murrah (0.237 and 0.246) and lowest for the Toda (0.215 and 0.211). The genetic distances (FST) of the Murrah with the Bhadawari, Mehsana, Surti, Pandharpuri, and Toda were 0.11, 0.17, 0.09, 0.15, and 0.13, respectively.
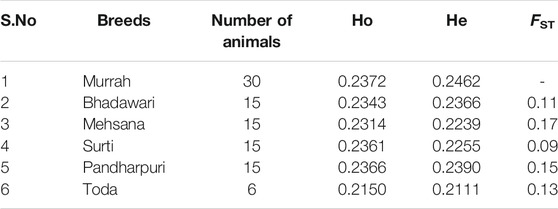
TABLE 1. Number of animals, means of observed (HO) and expected heterozygosity (HE), and differentiation (FST) between each breed and the Murrah.
Population Structure
The population structure of the Indian water buffalo breeds was identified using PCA. The first and second principal component (PC) explained 3.4 and 2.86% of the total variance. PC1 separated the crossbred Mehsana individuals from the rest of the breeds, while PC2 separated the Pandharpuri, Surti, and Toda from the Murrah and Bhadawari (Figure 1A). PC3 explained 2.71% of the total variation and showed clear separation between the Murrah and Bhadawari (Figure 1B).
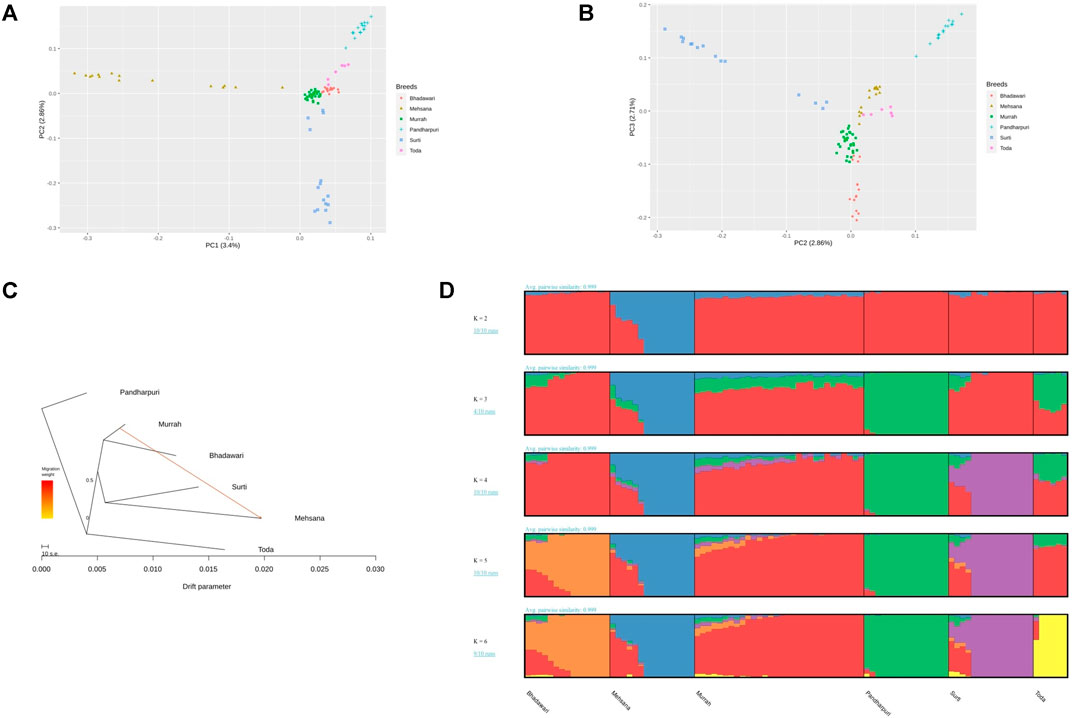
FIGURE 1. (A) Plot of the first two principal components (PC1 and PC2) of the genomic relationship matrix of the 96 animals under study. (B) Plot of PC2 and PC3. (C) Treemix phylogram showing one migration path. (D) Admixture results from K = 2 to K = 6.
The maximum-likelihood phylogram constructed with Treemix also displayed a similar tree (Figure 1C). The addition of one migration path in Treemix revealed the introgression of the Murrah inheritance in the Mehsana buffaloes. This tree explained 99.6% of the covariance observed between populations, whereas the tree without any migration events included explained only 98.3% of the covariance.
As seen with PC1, the Mehsana was separated from the rest of the breeds at K = 2 in the admixture analysis. K = 3 separated the Pandharpuri as a distinct population from the rest of the breeds, which gives credence to the results of the phylogenetic analyses. The Toda samples in our study showed a mixture of Pandharpuri and Murrah inheritance. At K = 6, all the breeds were assigned to their own clusters, with varying levels of Murrah ancestry appearing in other breeds (Bhadawari, Mehsana, Surti, and Toda) (Figure 1D).
Cross-Population Signatures of Selection (XP-EHH and FST)
The distribution of XP-EHH scores for the Murrah buffalo (positive values) against other water buffalo breeds in the study is visualized in Figure 2. A total of 164 putative selection regions for the Murrah buffalo were identified in comparison with the reference breeds (Supplementary Table S6). Ten selection sweeps were detected in comparisons of the Murrah with more than one breed (Table 2).
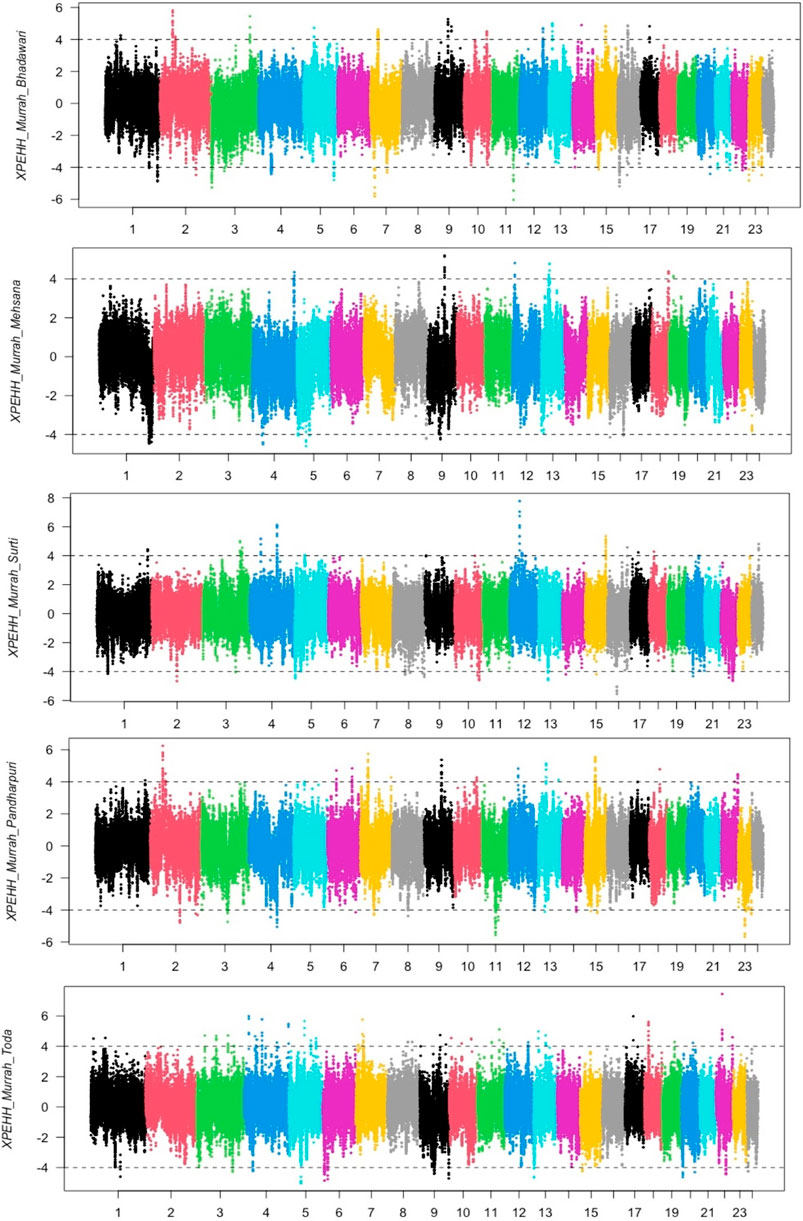
FIGURE 2. Cross-population extended haplotype heterozygote (XP-EHH) plot of the Murrah in comparison with the Bhadawari, Mehsana, Surti, Pandharpuri, and Toda.
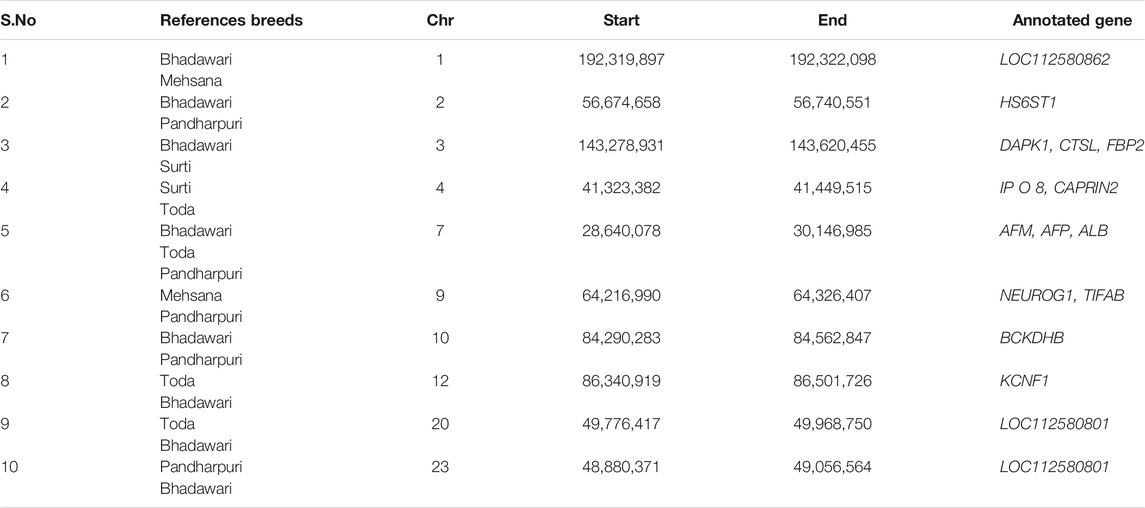
TABLE 2. Common selection sweeps identified by cross-population extended haplotype homozygosity (XP-EHH) in two or more pairwise comparisons involving the Murrah.
The Manhattan plot for pairwise FST across all comparisons are shown in Figure 3. A total of 58 positive regions were identified from all comparisons. The selection sweeps were located on all autosomes except for chromosome 5, 14, and 21. The highest number of selected regions were identified on chromosome 8 (seven regions), followed by chromosomes 1, 9, and 10 from all pairwise comparisons (Supplementary Table S7).
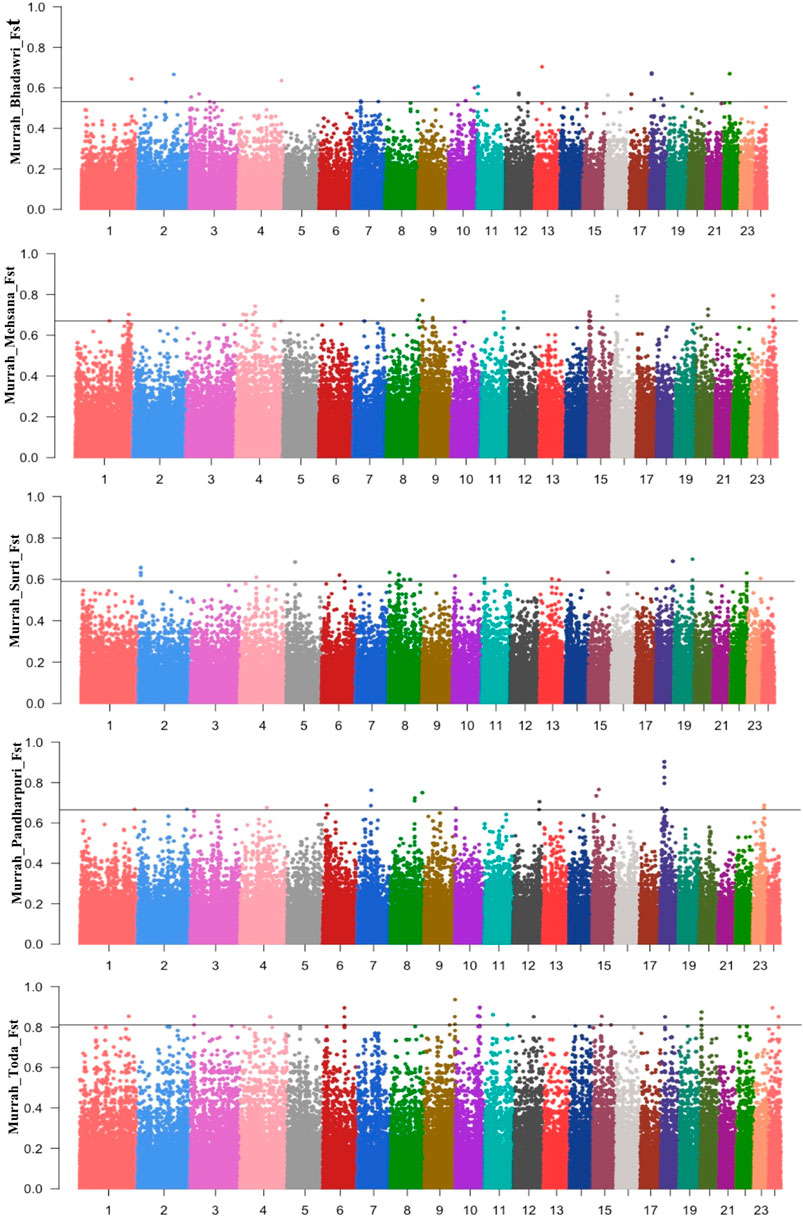
FIGURE 3. Manhattan plot for FST between the Murrah in comparison with the Bhadawari, Mehsana, Surti, Pandharpuri, and Toda.
A total of six fully or partially overlapping selection sweeps were identified from both the approaches XP-EHH and FST (Table 3). These regions were distributed on chromosomes 1, 7, 8, 13, 15, and 18.
Discussion
In the present study, ddRAD sequencing was used to identify genetic variants in six water buffalo breeds of India. The average heterozygosity levels ranged from 0.215 to 0.237, which were lower compared with a previous study (Kumar et al., 2006). However, they used microsatellite data, which suffers from ascertainment bias due to the most polymorphic microsatellite markers being studied, resulting in inflated heterozygosity estimates (Fischer et al., 2017). The population structure analysis separated the six breeds under study. Our findings confirmed two existing notions about the Indian buffaloes. First, it has been traditionally believed that the Mehsana breed is of the Murrah and Surti lineage (Patel et al., 2017; Sathwara et al., 2020). The maximum-likelihood phylogram constructed using Treemix in our study showed the Mehsana and Surti emerging from the same node in the phylogenetic tree, with introgression of the Murrah germplasm into the Mehsana, which supports the anecdotal knowledge about this breed. The admixture analyses also showed varying levels of Murrah inheritance into the Mehsana breed. Second, the western Indian buffalo, the Pandharpuri, formed a separate lineage from the rest of the breeds and appeared free of any Murrah inheritance, which was in agreement with previous studies (Kumar et al., 2006). However, in our study, the geographically distinct semi-wild Toda breed clustered with the Murrah. Admixture analysis showed all the Toda samples to contain significant levels of Murrah inheritance, which is a cause for concern. The samples were collected from the hamlets of the Toda tribes, situated in the jungles in and around Nilgiris district. In the 1990s, some of the Murrah bulls were introduced in Toda hamlets near small towns. This may be one of the reasons for the inheritance of the Murrah in Toda, which is reflected predominantly due to only six samples taken in the study.
The second objective of this study was to identify positive signatures of selection in the Murrah buffaloes. Humans have exerted strong artificial selection on different breeds of buffalo for similar traits since domestication (Dutta et al., 2020). Probably milk production formed the basis of selection and breeding, which resulted in the evolution of the dairy breeds of the farmers of riverine buffalo like the Murrah, Bhadawari, Mehsana, Surti, Pandharpuri, etc. (CIRB, Hisar, 2017). The Toda, on the other end is a semi wild breed purposely used for religious values from the past in the Nilgiri hills. These breeds may share mutations in the same gene(s) or regulatory region and, consequently, may have selective sweeps in the same area of the genome. However, the scope of selective sweeps may differ among breeds sharing mutations in the same genes because of differences in breed history, effective population size, and mutation rate (Pollinger et al., 2005), and also, differences may be caused by large environmental variations and different managemental practices throughout the country.
The positive signatures of selection in the Murrah buffaloes were identified using XP-EHH and FST approaches. Several fully or partially overlapping candidate regions in Murrah were identified through XP-EHH comparisons against more than one breed, which indicated recent artificial selection in the Murrah, given the characteristics of the XP-EHH test (Cheruiyot et al., 2018). Many of these regions overlap with previous reports in the Murrah.
On chromosome 1, a region was identified around the 192.2 Mb position against the Bhadawari, Mehsana, and Toda, which was in agreement with Dutta et al. (2020). This region includes UPK1B (Uroplakin 1 B), B4GALT4 (Beta-1,4-Galactosyltransferase), and ARHGAP31 (Rho GTPase-activating protein 31) genes, which could be putative candidate genes undergoing selection in the Murrah. The UPK1B and ARHGAP31genes have previously been linked with growth and carcass traits in cattle breeds (Kim et al., 2012; Medeiros de Oliveira Silva et al., 2017). Another partly overlapping region (17.4–17.5 Mb) in agreement with Dutta et al. (2020) was identified on chromosome two against the Pandharpuri. The region includes FABP3 (fatty acid-binding protein 3) gene, which is involved in the synthesis of long-chain fatty acid and, thus, regulates milk fat composition (Li et al., 2014).
A selection sweep (28.5–29.1 Mb) on chromosome seven in comparisons of the Murrah with the Pandharpuri, Toda, and Bhadawari also confirms a previously reported selection sweep (chromosome 7, 26.5–30.5 Mb) in the Murrah genome by Dutta et al. (2020). This region contains ALB, AFP, and AFM belonging to the family of albumin genes. The ALB (albumin) gene encodes albumin protein, which is involved in the transportation of varied endogenous molecules. ALB was reported to be significantly associated with total milk yield, milk fat, and protein percentage in the Holstein cattle (Seo et al., 2016) and obesity in humans (Kunej et al., 2013).
In agreement with Dutta et al. (2020), two regions on chromosome 13 (23.4–24.9 Mb) and chromosome 18 (14.6–14.9 Mb) were identified in our study. The region on chromosome 13 included GPC5 (glypican 5) gene, which is linked with fatty acid composition (Li et al., 2014), fertility traits (Purfield et al., 2019), and feed efficiency (Serão et al., 2013) in cattle. The MYLK3 (myosin light chain kinase 3) and GPT2 (glutamic pyruvic transaminase 2) genes on chromosome 18 are involved in muscle cell development (Silva-Vignato et al., 2019; Cheng et al., 2020) and Ca+2 signaling pathway in contraction of striated muscles (Zhang et al., 2009).
In addition, several novel regions of positive selection were also identified. These regions contain candidate genes, which are associated with the phenotypes that are under selection in the Murrah buffalo, including milk production and fat metabolism (HS6ST1, FBP2, and PDE9A), immunity-related pathways (DAPK1), stature (CTSL), and fertility traits (KCNF1 and CNTNAP2) (Jiang et al., 2011; Abo-Ismail et al., 2017; Guan et al., 2020). The regions included HS6ST1 (heparin sulfate 6-O sulfotransferase 1) gene located on chromosome 2, which plays a pivotal role in heparin metabolism pathway and regulates the fatty acid composition (Jiang et al., 2011). Another region on chromosome 3 contains DAPK1 (death-associated protein kinase 1), CTSL (cathepsin L), and FBP2 (fructose bisphophatase 2) genes, which are involved in various metabolic processes such as immunity and milk production (Vineeth et al., 2019; Guan et al., 2020). The KCNF1 (potassium voltage-gated channel modifier subfamily F member 1) gene on chromosome 12 has been previously reported to be associated with fertility traits in buffaloes (de Araujo Neto et al., 2020). Another candidate region spanning 280 kb on chromosome 1, which was detected by both approaches, contains PDE9A gene (phosphodiesterase 9A). This gene is involved in the signaling pathway, which regulates the level of cGMP inside the cell. Yang et al. (2015) has reported the strong association of PDE9A gene with milk production in Chinese Holstein cattle. On chromosome 8, CNTNAP2 (contactin-associated protein 2) gene was present in a significant region. This gene has been reported to be associated with immunity and growth traits in cattle (Abo-Ismail et al., 2017). CNTNAP2 gene is also reported to play an important role in milk synthesis pathway in water buffalo (Mishra et al., 2020). These positively selected genes may create the observed differences in the Murrah buffaloes from the rest of the buffalo breeds included in the study and makes the Murrah as one of the high milk-producing buffalo breed with high fertility and immunity.
Conclusion
The genetic diversity and population structure analysis revealed varying levels of the Murrah inheritance in the Bhadawari, Mehsana, Surti, and Toda buffalo breeds. The selection signature analysis provides several genomic regions as selection signature in the Murrah, which is the prominent milch breed in India. Using reduced representation ddRAD data, our results confirm many regions, which have been previously identified as selection sweeps in the Murrah genome using WGS data. In addition, novel regions were also identified, which are involved in several biological pathways. The candidate genes, found to be positively selected, are involved in milk production (ALB, FBP2, PDE9A, and GPC5), immunity-related traits (DAPK1), muscle cell development (MYLK3 and GPT2), and fertility traits (KCNF1 and CNTNAP2). These genes are suitable candidates for future polymorphism studies to detect causative variants associated with these phenotypes in buffaloes.
Data Availability Statement
The genotypes of the 96 individuals under study have been uploaded to Figshare under the DOI https://doi.org/10.6084/m9.figshare.14130389.v1. The data will be made public upon acceptance of the article.
Ethics Statement
The study was carried out in accordance with recommendation of Institute Animal Ethics Committee of ICAR-IVRI, Bareilly India.
Author Contributions
AP and AK conceived and designed the experiments. ST performed the experiments. AM and AS analyzed the data and wrote the manuscript. TD and BM contributed reagents/materials/analysis tools. ST, AK and AP edited the manuscript.
Funding
This project work was supported by the CAAST-ACLH project of NAHEP, and institute funding of ICAR-IVRI.
Conflict of Interest
The authors declare that the research was conducted in the absence of any commercial or financial relationships that could be construed as a potential conflict of interest.
Publisher’s Note
All claims expressed in this article are solely those of the authors and do not necessarily represent those of their affiliated organizations, or those of the publisher, the editors and the reviewers. Any product that may be evaluated in this article, or claim that may be made by its manufacturer, is not guaranteed or endorsed by the publisher.
Acknowledgments
The authors acknowledge the logistic and laboratory support rendered by the Director ICAR-IVRI. We also thank Dr. P.P Dubey and Dr. Puneet Malhotra (GADVASU), Dr. B.N. Sahi (GBPUA&T), Dr. B.P. Kushwaha (ICAR-IGFRI, Jhansi), Dr. Mitesh Gaur (LRS, Vallabhnagar), Director of Research (SDAU, SK Nagar, Gujarat), Dr. R.S. Kataria (ICAR-NBAGR), Dr. Jayakumar S. (ICAR-Directorate of Poultry Research), and Dr. A. P. Fernandes (MPKV Rahuri) for their contribution in providing the samples used in this study. We also wish to acknowledge Prof. Hubert Pausch (Animal Genomics, ETH Zurich), for his inputs regarding the SNP calling pipeline.
Supplementary Material
The Supplementary Material for this article can be found online at: https://www.frontiersin.org/articles/10.3389/fgene.2021.673697/full#supplementary-material
References
Abo-Ismail, M. K., Brito, L. F., Miller, S. P., Sargolzaei, M., Grossi, D. A., Moore, S. S., et al. (2017). Genome-Wide Association Studies and Genomic Prediction of Breeding Values for Calving Performance and Body Conformation Traits in Holstein Cattle. Genet. Sel. Evol. 49, 1–29. doi:10.1186/s12711-017-0356-8
Alexander, D. H., Novembre, J., and Lange, K. (2009). Fast Model-Based Estimation of Ancestry in Unrelated Individuals. Genome Res. 19, 1655–1664. doi:10.1101/gr.094052.109
Andrews, S. (2010). FastQC: A Quality Control Tool for High Throughput Sequence Data. Available at: at: http://www.bioinformatics.babraham.ac.uk/projects/fastqc/
Behr, A. A., Liu, K. Z., Liu-Fang, G., Nakka, P., and Ramachandran, S. (2016). Pong: Fast Analysis and Visualization of Latent Clusters in Population Genetic Data. Bioinformatics 32, 2817–2823. doi:10.1093/bioinformatics/btw327
Browning, B. L., and Browning, S. R. (2016). Genotype Imputation with Millions of Reference Samples. Am. J. Hum. Genet. 98, 116–126. doi:10.1016/j.ajhg.2015.11.020
Browning, B. L., Zhou, Y., and Browning, S. R. (2018). A One-Penny Imputed Genome from Next-Generation Reference Panels. Am. J. Hum. Genet. 103, 338–348. doi:10.1016/j.ajhg.2018.07.015
Cheng, J., Cao, X., Hanif, Q., Pi, L., Hu, L., Huang, Y., et al. (2020). Integrating Genome-Wide CNVs into QTLs and High Confidence GWAScore Regions Identified Positional Candidates for Sheep Economic Traits. Front. Genet. 11, 569. doi:10.3389/fgene.2020.00569
Cheruiyot, E. K., Bett, R. C., Amimo, J. O., Zhang, Y., Mrode, R., and Mujibi, F. D. N. (2018). Signatures of Selection in Admixed Dairy Cattle in Tanzania. Front. Genet. 9, 607. doi:10.3389/fgene.2018.00607
Cingolani, P., Platts, A., Wang, L. L., Coon, M., Nguyen, T., Wang, L., et al. (2012). A Program for Annotating and Predicting the Effects of Single Nucleotide Polymorphisms, SnpEff. Fly 6, 80–92. doi:10.4161/fly.19695
CIRB, Hisar (2017). Available at: https://cirb.icar.gov.in/history/. (Accessed February 25, 2021).
DAHD&F (2018). Department of Animal Husbandry Dairying & Fisheries. New Delhi: Ministry of Fisheries, Animal Husbandry & Dairying.
Danecek, P., Auton, A., Abecasis, G., Albers, C. A., Banks, E., DePristo, M. A., et al. (2011). The Variant Call Format and VCFtools. Bioinformatics 27, 2156–2158. doi:10.1093/bioinformatics/btr330
de Araujo Neto, F. R., Takada, L., Santos, D. J. A., Aspilcueta‐Borquis, R. R., Cardoso, D. F., Nascimento, A. V., et al. (2020). Identification of Genomic Regions Related to Age at First Calving and First Calving Interval in Water buffalo Using Single‐Step GBLUP. Reprod. Dom Anim. 55, 1565–1572. doi:10.1111/rda.13811
Dutta, P., Talenti, A., Young, R., Jayaraman, S., Callaby, R., Jadhav, S. K., et al. (2020). Whole Genome Analysis of Water Buffalo and Global Cattle Breeds Highlights Convergent Signatures of Domestication. Nat. Commun. 11, 1–13. doi:10.1038/s41467-020-18550-1
Fischer, M. C., Rellstab, C., Leuzinger, M., Roumet, M., Gugerli, F., Shimizu, K. K., et al. (2017). Estimating Genomic Diversity and Population Differentiation - an Empirical Comparison of Microsatellite and SNP Variation in Arabidopsis Halleri. BMC Genomics 18, 1–15. doi:10.1186/s12864-016-3459-7
Gautier, M., Klassmann, A., and Vitalis, R. (2017). rehh2.0: A Reimplementation of the R Packagerehhto Detect Positive Selection from Haplotype Structure. Mol. Ecol. Resour. 17, 78–90. doi:10.1111/1755-0998.12634
Guan, D., Landi, V., Luigi-Sierra, M. G., Delgado, J. V., Such, X., Castelló, A., et al. (2020). Analyzing the Genomic and Transcriptomic Architecture of Milk Traits in Murciano-Granadina Goats. J. Anim. Sci Biotechnol 11, 1–19. doi:10.1186/s40104-020-00435-4
Jiang, Z., Michal, J. J., Wu, X.-L., Pan, Z., and MacNeil, M. D. (2011). The Heparan and Heparin Metabolism Pathway Is Involved in Regulation of Fatty Acid Composition. Int. J. Biol. Sci. 7, 659–663. doi:10.7150/ijbs.7.659
Kim, K. S., Kim, S. W., Raney, N. E., and Ernst, C. W. (2012). Evaluation of BTA1 and BTA5 QTL Regions for Growth and Carcass Traits in American and Korean Cattle. Asian Australas. J. Anim. Sci. 25, 1521–1528. doi:10.5713/ajas.2012.12218
Kumar, M., Dahiya, S. P., Ratwan, P., Kumar, S., and Chitra, A. (2019). Status, Constraints and Future Prospects of Murrah Buffaloes in India. Indian J. Anim. Sci. 89, 1291–1302.
Kumar, S., Gupta, J., Kumar, N., Dikshit, K., Navani, N., Jain, P., et al. (2006). Genetic Variation and Relationships Among Eight Indian Riverine Buffalo Breeds. Mol. Ecol. 15, 593–600. doi:10.1111/j.1365-294X.2006.02837.x
Kunej, T., Skok, D. J., Zorc, M., Ogrinc, A., Michal, J. J., Kovac, M., et al. (2013). Obesity Gene Atlas in Mammals. J. Genomics 1, 45–55. doi:10.7150/jgen.3996
Li, C., Sun, D., Zhang, S., Wang, S., Wu, X., Zhang, Q., et al. (2014). Genome Wide Association Study Identifies 20 Novel Promising Genes Associated with Milk Fatty Acid Traits in Chinese Holstein. PLoS One 9, e96186. doi:10.1371/journal.pone.0096186
Li, H. (2013). Aligning Sequence Reads, Clone Sequences and Assembly Contigs with BWA-MEM. arXiv preprint arXiv:1303.3997
Li, H., Handsaker, B., Wysoker, A., Fennell, T., Ruan, J., Homer, N., et al. (2009). The Sequence Alignment/Map Format and SAMtools. Bioinformatics 25, 2078–2079. doi:10.1093/bioinformatics/btp352
Low, W. Y., Tearle, R., Bickhart, D. M., Rosen, B. D., Kingan, S. B., Swale, T., et al. (2019). Chromosome-Level Assembly of the Water Buffalo Genome Surpasses Human and Goat Genomes in Sequence Contiguity. Nat. Commun. 10, 1–11. doi:10.1038/s41467-018-08260-0
Martin, M. (2011). Cutadapt Removes Adapter Sequences from High-Throughput Sequencing Reads. EMBnet j. 17, 10–12. doi:10.14806/ej.17.1.200
Medeiros de Oliveira Silva, R., Bonvino Stafuzza, N., de Oliveira Fragomeni, B., Miguel Ferreira de Camargo, G., Matos Ceacero, T., Noely dos Santos Gonçalves Cyrillo, J., et al. (2017). Genome-wide Association Study for Carcass Traits in an Experimental Nelore Cattle Population. PLoS One 12, e0169860. doi:10.1371/journal.pone.0169860
Mishra, D. C., Sikka, P., Yadav, S., Bhati, J., Paul, S. S., Jerome, A., et al. (2020). Identification and Characterization of Trait-Specific SNPs Using ddRAD Sequencing in Water Buffalo. Genomics 112, 3571–3578. doi:10.1016/j.ygeno.2020.04.012
NBAGR Karnal (2021). Breed Profiles. Available at: https://nbagr.icar.gov.in/en/registered-buffalo/(Accessed July 04, 2021).
Patel, S., Thakkar, J., Koringa, P., Jakhesara, S., Patel, A., De, S., et al. (2017). Evolution and Diversity Studies of Innate Immune Genes in Indian Buffalo (Bubalus Bubalis) Breeds Using Next Generation Sequencing. Genes Genom 39, 1237–1247. doi:10.1007/s13258-017-0585-9
Peterson, B. K., Weber, J. N., Kay, E. H., Fisher, H. S., and Hoekstra, H. E. (2012). Double Digest RADseq: An Inexpensive Method for De Novo SNP Discovery and Genotyping in Model and Non-Model Species. PLoS One 7, e37135. doi:10.1371/journal.pone.0037135
Pickrell, J., and Pritchard, J. (2012). Inference of Population Splits and Mixtures from Genome-Wide Allele Frequency Data. PLoS Genet. 8 (11), e1002967. doi:10.1371/journal.pgen.1002967
Pollinger, J. P., Bustamante, C. D., Fledel-Alon, A., Schmutz, S., Gray, M. M., and Wayne, R. K. (2005). Selective Sweep Mapping of Genes with Large Phenotypic Effects. Genome Res. 15, 1809–1819. doi:10.1101/gr.4374505
Purcell, S., Neale, B., Todd-Brown, K., Thomas, L., Ferreira, M. A. R., Bender, D., et al. (2007). PLINK: A Tool Set for Whole-Genome Association and Population-Based Linkage Analyses. Am. J. Hum. Genet. 81, 559–575. doi:10.1086/519795
Purfield, D. C., Evans, R. D., Carthy, T. R., and Berry, D. P. (2019). Genomic Regions Associated with Gestation Length Detected Using Whole-Genome Sequence Data Differ between Dairy and Beef Cattle. Front. Genet. 10, 1068. doi:10.3389/fgene.2019.01068
Quinlan, A. R., and Hall, I. M. (2010). BEDTools: A Flexible Suite of Utilities for Comparing Genomic Features. Bioinformatics 26, 841–842. doi:10.1093/bioinformatics/btq033
R Core Team (2018). R: A Language and Environment for Statistical Computing. Vienna: R Foundation for Statistical Computing.
Ravi Kumar, D., Joel Devadasan, M., Surya, T., Vineeth, M. R., Choudhary, A., Sivalingam, J., et al. (2020). Genomic Diversity and Selection Sweeps Identified in Indian Swamp Buffaloes Reveals It's Uniqueness with Riverine Buffaloes. Genomics 112, 2385–2392. doi:10.1016/j.ygeno.2020.01.010
Rochette, N. C., Rivera‐Colón, A. G., and Catchen, J. M. (2019). Stacks 2: Analytical Methods for Paired‐end Sequencing Improve RADseq‐based Population Genomics. Mol. Ecol. 28, 4737–4754. doi:10.1111/mec.15253
Sabeti, P. C., Varilly, P., Varilly, P., Fry, B., Lohmueller, J., Hostetter, E., et al. (2007). Genome-Wide Detection and Characterization of Positive Selection in Human Populations. Nature 449, 913–918. doi:10.1038/nature06250
Sambrook, J., and Russell, D. W. (2006). Purification of Nucleic Acids by Extraction with Phenol: Chloroform. Cold Spring Harbor Protoc. 2006, pdb-prot4455. doi:10.1101/pdb.prot4455
Sathwara, R. N., Gupta, J. P., Chaudhari, J. D., Parmar, G. A., Prajapati, B. M., Srivastava, A. K., et al. (2020). Analysis of Association between Various Fertility Indicators and Production Traits in Mehsana Buffaloes. Trop. Anim. Health Prod. 52, 2585–2592. doi:10.1007/s11250-020-02288-5
Seo, M., Kim, K., Yoon, J., Jeong, J. Y., Lee, H.-J., Cho, S., et al. (2016). RNA-seq Analysis for Detecting Quantitative Trait-Associated Genes. Sci. Rep. 6, 24375. doi:10.1038/srep24375
Serão, N. V., González-Peña, D., Beever, J. E., Faulkner, D. B., Southey, B. R., and Rodriguez-Zas, S. L. (2013). Single Nucleotide Polymorphisms and Haplotypes Associated with Feed Efficiency in Beef Cattle. BMC Genet. 14, 1–20. doi:10.1186/1471-2156-14-94
Silva-Vignato, B., Coutinho, L. L., Poleti, M. D., Cesar, A. S. M., Moncau, C. T., Regitano, L. C. A., et al. (2019). Gene Co-Expression Networks Associated with Carcass Traits Reveal New Pathways for Muscle and Fat Deposition in Nelore Cattle. BMC Genomics 20, 32. doi:10.1186/s12864-018-5345-y
Singh, A., Mehrotra, A., Gondro, C., Romero, A. R. d. S., Pandey, A. K., Karthikeyan, A., et al. (2020). Signatures of Selection in Composite Vrindavani Cattle of India. Front. Genet. 11, 589496. doi:10.3389/fgene.2020.589496
Surya, T., Vineeth, M. R., Sivalingam, J., Tantia, M. S., Dixit, S. P., Niranjan, S. K., et al. (2019). Genomewide Identification and Annotation of SNPs in Bubalus Bubalis. Genomics 111, 1695–1698. doi:10.1016/j.ygeno.2018.11.021
Vineeth, M. R., Surya, T., Sivalingam, J., Kumar, A., Niranjan, S. K., Dixit, S. P., et al. (2019). Genome-Wide Discovery of SNPs in Candidate Genes Related to Production and Fertility Traits in Sahiwal Cattle. Trop. Anim. Health Pro 52 (4), 1707–1715. doi:10.1007/s11250-019-02180-x
Weir, B. S., and Cockerham, C. C. (1984). Estimating F-Statistics for the Analysis of Population Structure. Evolution 38, 1358–1370. doi:10.2307/2408641
Wright, B., Farquharson, K. A., McLennan, E. A., Belov, K., Hogg, C. J., and Grueber, C. E. (2019). From Reference Genomes to Population Genomics: Comparing Three Reference-Aligned Reduced-Representation Sequencing Pipelines in Two Wildlife Species. BMC Genomics 20, 1–10. doi:10.1186/s12864-019-5806-y
Yang, J., Lee, S. H., Goddard, M. E., and Visscher, P. M. (2011). GCTA: a Tool for Genome-Wide Complex Trait Analysis. Am. J. Hum. Genet. 88, 76–82. doi:10.1016/j.ajhg.2010.11.011
Yang, S.-H., Bi, X.-J., Xie, Y., Li, C., Zhang, S.-L., Zhang, Q., et al. (2015). Validation of PDE9A Gene Identified in GWAS Showing strong Association with Milk Production Traits in Chinese Holstein. Int.J. Mol.Sci. 16, 26530–26542. doi:10.3390/ijms161125976
Zhang, S.-Z., Xu, Y., Xie, H.-Q., Li, X.-Q., Wei, Y.-Q., and Yang, Z.-M. (2009). The Possible Role of Myosin Light Chain in Myoblast Proliferation. Biol. Res. 42, 121–132. doi:10.4067/S0716-97602009000100013
Keywords: ddRAD, genotypes, bubalus, Fst, XP-EHH
Citation: Tyagi SK, Mehrotra A, Singh A, Kumar A, Dutt T, Mishra BP and Pandey AK (2021) Comparative Signatures of Selection Analyses Identify Loci Under Positive Selection in the Murrah Buffalo of India. Front. Genet. 12:673697. doi: 10.3389/fgene.2021.673697
Received: 28 February 2021; Accepted: 17 September 2021;
Published: 19 October 2021.
Edited by:
Yang Zhou, Huazhong Agricultural University, ChinaReviewed by:
Pablo Fonseca, University of Guelph, CanadaMonika Sodhi, National Bureau of Animal Genetic Resources (NBAGR), India
Copyright © 2021 Tyagi, Mehrotra, Singh, Kumar, Dutt, Mishra and Pandey. This is an open-access article distributed under the terms of the Creative Commons Attribution License (CC BY). The use, distribution or reproduction in other forums is permitted, provided the original author(s) and the copyright owner(s) are credited and that the original publication in this journal is cited, in accordance with accepted academic practice. No use, distribution or reproduction is permitted which does not comply with these terms.
*Correspondence: Ashwni K. Pandey, YXNod25pLnBhbmRleUBnbWFpbC5jb20=