- 1Department of Chemical and Biomolecular Engineering (BK21 four), Korea Advanced Institute of Science and Technology (KAIST), Daejeon, South Korea
- 2KAIST Institute for Artificial Intelligence, KAIST, Daejeon, South Korea
- 3BioProcess Engineering Research Center and BioInformatics Research Center KAIST, Daejeon, South Korea
Chemotherapy is a mainstream cancer treatment, but has a constant challenge of drug resistance, which consequently leads to poor prognosis in cancer treatment. For better understanding and effective treatment of drug-resistant cancer cells, omics approaches have been widely conducted in various forms. A notable use of omics data beyond routine data mining is to use them for computational modeling that allows generating useful predictions, such as drug responses and prognostic biomarkers. In particular, an increasing volume of omics data has facilitated the development of machine learning models. In this mini review, we highlight recent studies on the use of multi-omics data for studying drug-resistant cancer cells. We put a particular focus on studies that use computational models to characterize drug-resistant cancer cells, and to predict biomarkers and/or drug responses. Computational models covered in this mini review include network-based models, machine learning models and genome-scale metabolic models. We also provide perspectives on future research opportunities for combating drug-resistant cancer cells.
Introduction
Drug resistance has been a major obstacle for a successful treatment of cancers, as manifested by over 90% mortality of cancer patients that appeared to be associated with drug resistance (Bukowski et al., 2020). Drug resistance is a phenotypic state that arises as a result of a complex interplay between genetic and non-genetic mechanisms (Marine et al., 2020). Such genetic and non-genetic reprogramming consequently leads to drug resistance through various mechanisms (Gatti and Zunino, 2005; Housman et al., 2014; Zheng, 2017; Lim and Ma, 2019; Vasan et al., 2019; Bukowski et al., 2020), including: drug inactivation, for example by an excessive level of glutathione that detoxifies xenobiotics (Jiang et al., 2017; De Luca et al., 2019); alteration of a drug target by mutations or changes in an expression level (Likhite et al., 2006; Costa et al., 2008); drug efflux by transporters (Giddings et al., 2021); enhanced DNA damage repair system (Harte et al., 2014); development of resistance via dysregulated autophagy (Martin et al., 2017; Cai et al., 2019); epithelial-mesenchymal transition (EMT) (Fischer et al., 2015; Zheng et al., 2015); or heterogeneity of a cancer cell population having cancer stem cells (Seth et al., 2019; Zhao et al., 2021). A state of drug resistance is indeed a highly complex phenotype that requires multidimensional approaches.
Omics technologies have now become indispensable for characterizing mechanisms of cancer progression, and for identifying effective biomarkers and treatment targets for cancers. For this reason, large-scale projects have been launched to generate omics data of various cancer cells. A recent representative example is the Pan-Cancer Analysis of Whole Genomes (PCAWG) Consortium of the International Cancer Genome Consortium (ICGC) and The Cancer Genome Atlas (TCGA), which has allowed advanced studies on gene mutations and gene expression profiles across cancers (Consortium, 2020). The resulting various datasets from such large-scale efforts have been found to be useful for studying drug-resistant cancer cells. Relevant representative datasets include the NCI-60 Human Tumor Cell Lines Screen (Shoemaker, 2006), the Genomics of Drug Sensitivity in Cancer (GDSC) (Yang et al., 2013), TCGA (Cancer Genome Atlas Research et al., 2013), the Cancer Therapeutic Response Portal (CTRP) (Seashore-Ludlow et al., 2015), L1000 profiles from The Library of Integrated Network-Based Cellular Signatures (LINCS) Program (Subramanian et al., 2017), the Cancer Cell Line Encyclopedia (CCLE) (Ghandi et al., 2019), and the Catalogue Of Somatic Mutations In Cancer (COSMIC) (Tate et al., 2019). All these datasets have served as a source of novel insights that help characterize and overcome drug-resistant cancer cells. In particular, it is expected that an increasing volume of such large-scale datasets will facilitate development of various computational models that will better systematize our approaches to studying drug-resistant cancer cells.
We here review recent studies that utilized multi-omics and computational modeling approaches to better understand mechanisms associated with the progression of drug resistance, and to identify biomarkers and/or drug responses (Figure 1 and Table 1). Especially, we put more focus on computational modeling that makes predictions for various scenarios for the treatment of drug-resistant cancer cells. We also provide an outlook for further advances on the use of computational models for studying drug-resistant cancer cells.
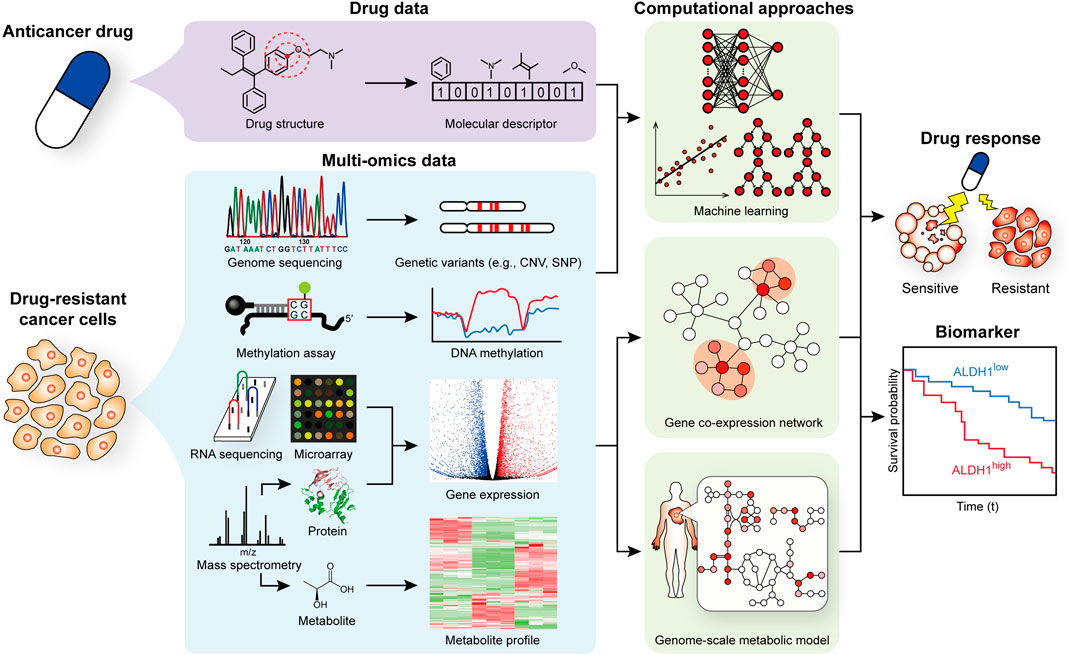
FIGURE 1. Scheme of omics data generation and computational modeling to better understand and treat drug-resistant cancer cells.
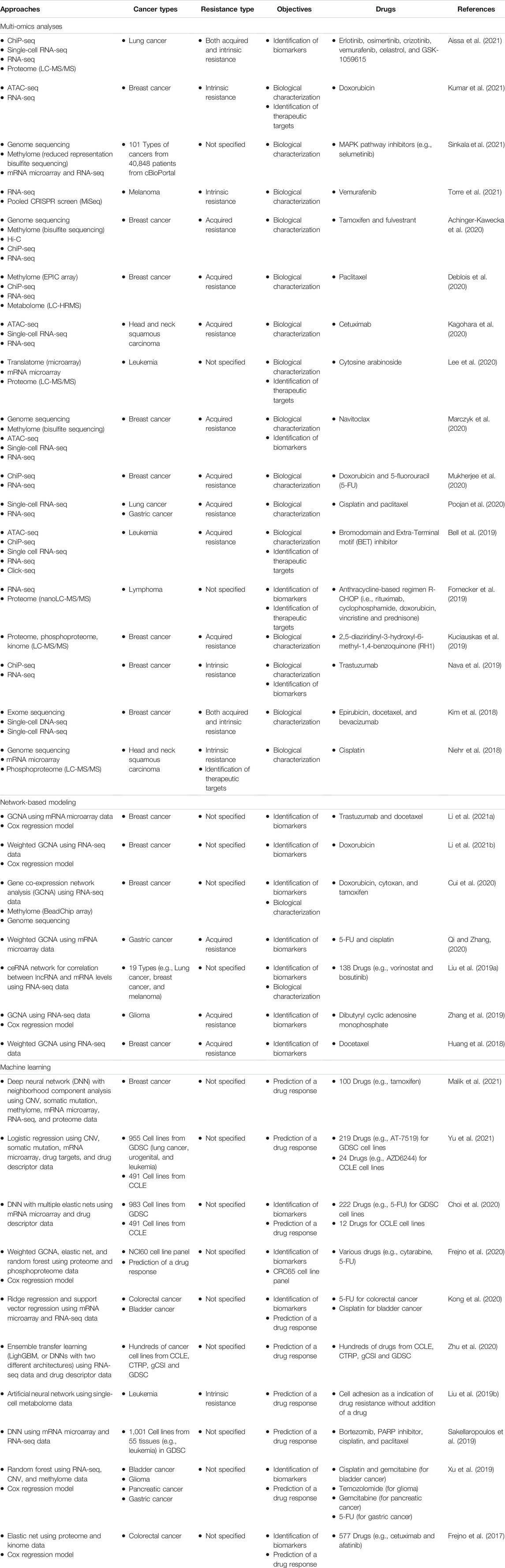
TABLE 1. Recent studies on the use of omics data and computational models to better understand and treat drug-resistant cancer cells.
Multi-Omics Analyses
Multiple omics data are often generated to examine various biological aspects of drug-resistant cancer cells (Figure 1). Target genotypes and phenotypes examined using omics data (Table 1) include: cancer-associated mutations (Niehr et al., 2018; Marczyk et al., 2020; Sinkala et al., 2021); changes in the expression level of specific genes (Niehr et al., 2018; Nava et al., 2019; Kagohara et al., 2020; Marczyk et al., 2020; Poojan et al., 2020; Sinkala et al., 2021); changes in chromosome structure (Kagohara et al., 2020; Marczyk et al., 2020; Aissa et al., 2021); epigenetic alterations (e.g., methylation or acetylation states of histone proteins) (Nava et al., 2019; Kagohara et al., 2020; Marczyk et al., 2020; Poojan et al., 2020; Sinkala et al., 2021); and the presence of heterogeneity of a cell population (Niehr et al., 2018), often increasingly examined at a single-cell resolution (Kagohara et al., 2020; Aissa et al., 2021). In a recent study for cell line heterogeneity, for example, application of single-cell DNA and RNA sequencing (RNA-seq) to 20 triple-negative breast cancer (TNBC) patients revealed that rare pre-existing clones having genotypes associated with chemoresistance were adaptively selected in response to neoadjuvant chemotherapy, which subsequently led to acquired transcriptional reprogramming (Kim et al., 2018). For epigenetic alteration, chromosome conformation capture (Hi-C) along with additional omics analyses were conducted for estrogen receptor positive (ER+) breast cancer, which showed that resistance development to endocrine therapy was accompanied with notable 3-dimensional (3D) epigenome alterations (Achinger-Kawecka et al., 2020). Application of multi-omics analyses has also been extended to examine biological processes in quiescent cancer cells that show drug resistance (Lee et al., 2020; Kumar et al., 2021).
Understanding the biology of drug resistance often helps devise effective treatment strategies for drug-resistant cancer cells. Relevant examples (Table 1) include targeting: cancer stem cell phenotypes, in particular stem cell factor receptor c-KIT, for TNBC cells resistant to an anticancer agent RH1 that is currently under clinical trials (Kuciauskas et al., 2019); a range of biological pathways (e.g., metabolism), microenvironment as well as proliferation, migration and invasion of cells, which are all associated with drug resistance for diffuse large B-cell lymphoma patients (Fornecker et al., 2019); zinc finger MYND domain-containing protein 8 (ZMYND8), a putative chromatin reader that appeared to suppress tumorigenic potential and drug resistance induced by doxorubicin (Mukherjee et al., 2020); and EZH2 responsible for histone methylation in taxane-resistant TNBC (Deblois et al., 2020).
As representative examples of overcoming drug resistance on the basis of omics analyses, recent studies additionally conducted CRISPR-Cas9-based genetic screens to examine cellular plasticity, which was suggested as a therapeutic target for drug-resistant cancer cells (Bell et al., 2019; Torre et al., 2021). Cellular plasticity describes non-genetic transformation of a cellular state into a drug-resistant state by reprogramming gene expression profiles. In a study by Torre et al., CRISPR-Cas9 genetic screens were implemented for melanoma cells to identify genes that affect cell fate decisions by altering cellular plasticity (Torre et al., 2021). In particular, modulating the cellular plasticity was demonstrated for vemurafenib inhibiting B-Raf, encoded by a proto-oncogene, in melanoma. Interestingly, inhibiting DOT1L, associated with the onset of melanoma, before the B-Raf inhibition showed more drug resistance than simultaneous inhibition of DOT1 and B-Raf using pinometostat and vemurafenib, respectively. Subsequent transcriptome analysis of knockout cell lines generated clues for non-genetic mechanisms of drug resistance. Another study by Bell et al. focused on acute myeloid leukemia patients that showed non-genetic drug resistance (Bell et al., 2019). Single-cell RNA-seq, followed by CRISPR-Cas9 screening, led to the identification of genes responsible for transcriptional plasticity that triggered epigenetic resistance. Among the genes identified was Lsd1, the inhibition of which was shown to overcome non-genetic drug resistance. As demonstrated by these two recent studies, implementation of genome engineering in addition to omics analyses provides compelling evidence for targets that can help overcome drug resistance.
COMPUTATIONAL MODELING APPROACHES
While various bioinformatic analyses are available for analyzing omics data, such as enrichment analyses, gene co-expression networks (GCNs) (Cui et al., 2020; Qi and Zhang, 2020) and their variants (e.g., a network of long non-coding RNAs and mRNAs) (Huang et al., 2018; Liu H. et al., 2019) as well as dimensionality reduction (e.g., t-SNE and UMAP), omics data have also been subjected to computational modeling to make predictions for discovering novel mechanisms and devising treatment strategies for drug-resistant cancers (Figure 1). Use of survival analysis in combination with GCNs, and development of a gene regulatory network (GRN) model using a set of ordinary differential equations (ODEs), machine learning models, and genome-scale metabolic models (GEMs) are representative computational modeling approaches that have recently been considered for studying drug-resistant cancer cells (Table 1).
Network-Based Modeling
GCN has been a popular analysis for understanding gene expression patterns from transcriptome data. GCN is an undirected graph that can be constructed from transcriptome data (e.g., RNA-seq), and connects pairs of genes (nodes in a GCN) with an edge if each pair of genes shows significant co-expression patterns across the transcriptome data. GCN analysis, such as identifying hub genes and/or modules, allows prioritizing candidate genes that may be highly associated with drug resistance of cancer cells. Weighted GCN additionally considers the level of significance in the co-expression relationship between genes in a pair. Often, outcomes from (weighted) GCN analysis are further subjected to other computational analyses, for example survival analysis, to validate the biological and/or clinical significance of the candidate genes. As a recent example, Li et al. focused on PPP2R2B, encoding serine/threonine-protein phosphatase 2A 55 kDa regulatory subunit B beta isoform, as a potential prognostic biomarker for TNBC on the basis of a series of bioinformatic analyses involving a GCN (Li Z. et al., 2021). Kaplan-Meier survival analysis for this gene revealed that patients with a low expression level of PPP2R2B showed shorter survival time than those with a high expression level of PPP2R2B. Interestingly, PPP2R2B upregulation could attenuate the resistance of TNBC cells to doxorubicin. Likewise, Cox proportional hazards regression model (Cox regression model) was used for genes selected from GCNs to predict prognostic biomarkers for breast cancer, and to suggest genes (e.g., CCNE2 and KIF14) that may help overcome drug resistance (Li Y.-K. et al., 2021).
While GCNs can provide clinically important information when combined with additional predictive models, such as survival analysis above, they have limitations in generating clues on a molecular mechanism associated with development of drug resistance, in particular dynamic interactions between genes. To address this problem, Zhang et al. developed a time-course RNA-seq data-driven computational framework (DryNetMC) to construct GRNs that help elucidate dynamic interactions between genes, and identify key genes associated with mechanisms of drug resistance (Zhang et al., 2019). DryNetMC involves a set of ODEs, a regularized regression method as well as a series of network analyses. Using DryNetMC, GRNs were constructed for dbcAMP-sensitive and dbcAMP-resistant glioma cells based on their time-course RNA-seq data. These differential GRNs were subsequently subjected to a systematic characterization to identify their unique network properties (e.g., node importance) that helped identify key genes (e.g., KIF2C, CCNA2, NDC80, KIF11, and KIF23) that are predictive of a cancer cell’s drug response. Because network-based models, either by using a GCN or other methods (e.g., ODEs), can visualize a biological context (e.g., association between genes), they will continue to be actively used in the analysis of omics data, and likely along with additional predictive models.
Machine Learning
Increasing availability of omics data for drug-resistant cancer cells has also provided unprecedented opportunities for building machine learning models. In general, machine learning models perform classification or regression, depending on a given problem. Recently, prediction of anticancer drug response was attempted by using various types of machine learning methods, such as logistic regression (Frejno et al., 2017; Yu et al., 2021), random forest (Xu et al., 2019) and deep neural network (DNN; e.g., multilayer perceptron) (Malik et al., 2021) on the basis of a range of omics and drug response data (Table 1). When developing these machine learning models, transcriptome (RNA-seq or mRNA microarray) was the most frequently adopted dataset, but other types of datasets were also considered, including genome (e.g., gene mutations) (Yu et al., 2021), proteome (Frejno et al., 2020), epigenome (Xu et al., 2019), mass spectrometry data (Liu R. et al., 2019) and molecular features of a target drug (Zhu et al., 2020).
In a recent study by Kong et al., a machine learning model was developed that can predict a patient’s drug response on the basis of the analysis of protein-protein interaction (PPI) network and pharmacogenomic data from 3D organoid culture models (Kong et al., 2020). Specifically, potential biomarkers were first inferred from the PPI network analysis, and their corresponding expression profiles along with drug response data (IC50) were used to train a machine learning model (e.g., ridge regression). The resulting drug responses were validated using survival analysis by focusing on colorectal and bladder cancer patients treated with 5-fluorouracil and cisplatin, respectively. The predicted drug responses also appeared to be consistent with transcriptome profiles from drug-sensitive and drug-resistant isogenic cancer cell lines as well as data on somatic mutations associated with already known biomarkers. In this study, consideration of the network analysis not only helped improve the performance of the developed machine learning model, but also facilitated the interpretation of model prediction outcomes. Likewise, in another study, elastic net and random forest regression were used to predict drug responses from abundance data of proteins and their phosphorylation sites in cancer cell lines (Frejno et al., 2020).
Among machine learning methods, DNNs are increasingly used for various predictions, and they have also been used to predict drug responses. Sakellaropoulos et al. developed a DNN model by using GDSC datasets (i.e., transcriptomic data for 1,001 cancer cell lines and IC50 values of 251 drugs) to predict drug responses (Sakellaropoulos et al., 2019). Across several datasets tested, the DNN model showed consistently better performance than elastic net and random forest models. The DNN model was validated by conducting survival analyses for the model-predicted IC50 values, which split patients based on their drug responsiveness. Importantly, pathway enrichment analysis using information from the DNN model (i.e., weights that connect the input layer and the first hidden layer) appeared to associate specific biological pathways with mechanisms of action for drugs. In a more recent study, predicting drug response was also attempted by using a DNN model combined with multiple elastic nets (Choi et al., 2020), referred to as Reference Drug-based Neural Network (RefDNN). RefDNN was developed more in the context of drug resistance, which predicts whether a given cell line is resistant to a target drug by processing gene expression profiles and molecular structure of a drug. RefDNN was also shown to help identify biomarker genes associated with drug resistance, and explore a novel anticancer drug via drug repositioning.
Despite its demonstrated performance, machine learning is often challenged with the limited availability of training datasets for many technical fields. This challenge can be addressed to a certain extent by employing transfer learning as recently demonstrated (Zhu et al., 2020). Zhu et al. demonstrated that ensemble transfer learning can improve the prediction of drug responses in the context of drug repositioning (i.e., use of a drug for another cancer that is already known), precision oncology (i.e., use of a drug for a new cancer that has never been treated before) and new drug development (i.e., use of a new drug for already known cancer). In this particular study, LightGBM (Light Gradient Boosting Machine) and two different DNN models were considered for ensemble transfer learning; larger datasets from the CTRP and GDSC were used as source data for initial training of models, and smaller datasets from CCLE and the Genentech Cell Line Screening Initiative (gCSI) served as target data for further refinement and testing of the models. It was shown that ensemble transfer learning-based models almost always outperformed models that were not developed using transfer learning. This study suggests the use of transfer learning for other drug-resistant cancer cells where a training dataset is sufficiently not available.
Genome-Scale Metabolic Modeling
GEM is a computational model that describes gene-protein-reaction (GPR) associations, and can be simulated to predict genome-scale metabolic flux distributions (Gu et al., 2019). GEMs are now available for an increasing number of organisms that are important in biotechnology and biomedicine. Several versions of human GEMs (Ryu et al., 2017; Brunk et al., 2018; Robinson et al., 2020) are currently available, which have been used to examine a target cell’s metabolism, and to predict biomarkers and drug targets for various diseases (Cook and Nielsen, 2017; Gu et al., 2019). For a medical application, a generic human GEM, covering all the known GPR associations in human metabolism, is initially integrated with omics data, often transcriptome (e.g., RNA-seq), to build a context-specific GEM, a GEM that is specific to a target cell or tissue (Ryu et al., 2015; Opdam et al., 2017). The resulting context-specific GEM is then simulated for various metabolic studies.
Human GEMs have recently been used to study radiation-resistant tumors (Lewis et al., 2021; Lewis and Kemp, 2021), but not drug-resistant cancer cells, to the best of our knowledge. Lewis et al. newly constructed GEMs for radiation-sensitive and radiation-resistant tumors through multi-omics integration (i.e., transcriptome data, mutational data, kinetic data and thermodynamic data) (Lewis et al., 2021). These context-specific GEMs were used to identify changes in redox cofactor production that give resistance to radiation therapy. In the other study, ensemble machine learning classifiers were developed to predict whether an individual is responsive or resistant to a radiation therapy by considering data of metabolite production rates predicted from context-specific GEMs as well as mutation data, transcriptome data and clinical data from TCGA (Lewis and Kemp, 2021). These two studies obviously suggest that GEM-based approaches can also be considered to identify metabolic signatures of drug-resistant cancer cells, and to predict effective drug targets for these cancer cells.
Outlook
Understanding genotype-phenotype associations in drug-resistant cancer cells is a highly complex problem, and therefore use of multi-omics data has been considered to capture various aspects of these troubling cancer cells. In particular, multi-omics analyses along with additional tools, such as genome engineering (e.g., CRISPR-Cas9), will continue to play an important role in thorough characterization of drug-resistant cancer cells. Also, an increasing volume of omics data will facilitate development of various types of computational models. As a consequence, prediction outcomes from computational models will allow more systematically designing experiments for drug-resistant cancer cells.
Despite the promises of omics data and computational models, technical challenges exist. First, current coverage of multi-omics data is not sufficient for thoroughly studying a range of drug-resistant cancer cells. In particular, generation of a consistent set of multi-omics data from each single cell is necessary for in-depth study of a target cancer cell and comparison of different types of cancer cells. Also, it will be interesting to examine the effects of using datasets obtained from patients having a specific disease instead of publicly available datasets (e.g., GDSC and CTRP). While currently available machine learning models have been rigorously validated by using public datasets, they might reveal previously unnoticed limitations in a clinical setting because the public datasets are often generated in a highly controlled condition. In particular, additional consideration of non-genetic factors (e.g., age, gender, and lifestyle) may help reveal new insights on drug-resistant cancer cells. Use of patient-specific datasets will allow more widespread use of the state-of-the-art computational models in a clinical setting.
For network-based modeling, including both GCN and GRN, a breakthrough is needed that allows efficiently developing a cell-specific large-scale GRN that can be simulated under various conditions (e.g., gene perturbation). For machine learning, despite its high predictive performance, there is always a challenge of avoiding overfitting and achieving explainability. Explainability in terms of biological processes is particularly important in the field of biomedicine in order to explain prediction outcomes and make medical decisions. In case of human GEMs, because patient-specific omics data (e.g., RNA-seq) are available to a certain extent, human GEMs should be more actively considered to systematically examine metabolism of drug-resistant cancer cells. Availability of multi-omics data will be particularly useful for interpreting human GEMs and their prediction outcomes; because human GEMs only cover a metabolic network, use of multi-omics data can help explain a complex interplay between metabolic and regulatory networks. Prediction outcomes from the simulation of human GEMs will in turn help explain the insights reaped from omics analyses.
Taken together, advances in omics technologies and computational modeling will bring about positive impacts in understanding and treating drug-resistant cancer cells. Feedback from clinicians and biomedical researchers will be additionally useful for the successful development and clinical application of computational models.
Author Contributions
HUK, HDJ and YJS wrote the manuscript. HDJ and YJS prepared the figure and table, and critically reviewed the manuscript. All authors contributed to the article and approved the submitted version.
Funding
This work was supported by the Bio-Synergy Research Project (NRF-2018M3A9C4076475) and the KAIST Cross-Generation Collaborative Lab project of the Ministry of Science and ICT through the National Research Foundation of Korea. This work was also supported by Kwon Oh-Hyun Assistant Professor fund of the KAIST Development Foundation.
Conflict of Interest
The authors declare that the research was conducted in the absence of any commercial or financial relationships that could be construed as a potential conflict of interest.
Publisher’s Note
All claims expressed in this article are solely those of the authors and do not necessarily represent those of their affiliated organizations, or those of the publisher, the editors and the reviewers. Any product that may be evaluated in this article, or claim that may be made by its manufacturer, is not guaranteed or endorsed by the publisher.
References
Achinger-Kawecka, J., Valdes-Mora, F., Luu, P.-L., Giles, K. A., Caldon, C. E., Qu, W., et al. (2020). Epigenetic Reprogramming at Estrogen-Receptor Binding Sites Alters 3D Chromatin Landscape in Endocrine-Resistant Breast Cancer. Nat. Commun. 11 (1), 320. doi:10.1038/s41467-019-14098-x
Aissa, A. F., Islam, A. B. M. M. K., Ariss, M. M., Go, C. C., Rader, A. E., Conrardy, R. D., et al. (2021). Single-cell Transcriptional Changes Associated with Drug Tolerance and Response to Combination Therapies in Cancer. Nat. Commun. 12 (1), 1628. doi:10.1038/s41467-021-21884-z
Bell, C. C., Fennell, K. A., Chan, Y.-C., Rambow, F., Yeung, M. M., Vassiliadis, D., et al. (2019). Targeting Enhancer Switching Overcomes Non-genetic Drug Resistance in Acute Myeloid Leukaemia. Nat. Commun. 10 (1), 2723. doi:10.1038/s41467-019-10652-9
Brunk, E., Sahoo, S., Zielinski, D. C., Altunkaya, A., Dräger, A., Mih, N., et al. (2018). Recon3D Enables a Three-Dimensional View of Gene Variation in Human Metabolism. Nat. Biotechnol. 36 (3), 272–281. doi:10.1038/nbt.4072
Bukowski, K., Kciuk, M., and Kontek, R. (2020). Mechanisms of Multidrug Resistance in Cancer Chemotherapy. Ijms 21 (9), 3233. doi:10.3390/ijms21093233
Cai, Q., Wang, S., Jin, L., Weng, M., Zhou, D., Wang, J., et al. (2019). Long Non-coding RNA GBCDRlnc1 Induces Chemoresistance of Gallbladder Cancer Cells by Activating Autophagy. Mol. Cancer 18 (1), 82. doi:10.1186/s12943-019-1016-0
Choi, J., Park, S., and Ahn, J. (2020). RefDNN: a Reference Drug Based Neural Network for More Accurate Prediction of Anticancer Drug Resistance. Sci. Rep. 10 (1), 1861. doi:10.1038/s41598-020-58821-x
Collisson, E. A., Weinstein, J. N., Weinstein, J. N., Mills, G. B., Shaw, K. R. M., Ozenberger, B. A., et al. (2013). The Cancer Genome Atlas Pan-Cancer Analysis Project. Nat. Genet. 45 (10), 1113–1120. doi:10.1038/ng.2764
Consortium, I. T. P.-C. A. o. W. G. (2020). Pan-cancer Analysis of Whole Genomes. Nature 578 (7793), 82–93. doi:10.1038/s41586-020-1969-6
Cook, D. J., and Nielsen, J. (2017). Genome-scale Metabolic Models Applied to Human Health and Disease. Wires Syst. Biol. Med. 9 (6), e1393. doi:10.1002/wsbm.1393
Costa, D. B., Nguyen, K.-S. H., Cho, B. C., Sequist, L. V., Jackman, D. M., Riely, G. J., et al. (2008). Effects of Erlotinib in EGFR Mutated Non-small Cell Lung Cancers with Resistance to Gefitinib. Clin. Cancer Res. 14 (21), 7060–7067. doi:10.1158/1078-0432.CCR-08-1455
Cui, H., Kong, H., Peng, F., Wang, C., Zhang, D., Tian, J., et al. (2020). Inferences of Individual Drug Response-Related Long Non-coding RNAs Based on Integrating Multi-Omics Data in Breast Cancer. Mol. Ther. - Nucleic Acids 20, 128–139. doi:10.1016/j.omtn.2020.01.038
De Luca, A., Parker, L. J., Ang, W. H., Rodolfo, C., Gabbarini, V., Hancock, N. C., et al. (2019). A Structure-Based Mechanism of Cisplatin Resistance Mediated by Glutathione Transferase P1-1. Proc. Natl. Acad. Sci. USA 116 (28), 13943–13951. doi:10.1073/pnas.1903297116
Deblois, G., Tonekaboni, S. A. M., Grillo, G., Martinez, C., Kao, Y. I., Tai, F., et al. (2020). Epigenetic Switch-Induced Viral Mimicry Evasion in Chemotherapy-Resistant Breast Cancer. Cancer Discov. 10 (9), 1312–1329. doi:10.1158/2159-8290.CD-19-1493
Fischer, K. R., Durrans, A., Lee, S., Sheng, J., Li, F., Wong, S. T. C., et al. (2015). Epithelial-to-mesenchymal Transition Is Not Required for Lung Metastasis but Contributes to Chemoresistance. Nature 527 (7579), 472–476. doi:10.1038/nature15748
Fornecker, L.-M., Muller, L., Bertrand, F., Paul, N., Pichot, A., Herbrecht, R., et al. (2019). Multi-omics Dataset to Decipher the Complexity of Drug Resistance in Diffuse Large B-Cell Lymphoma. Sci. Rep. 9 (1), 895. doi:10.1038/s41598-018-37273-4
Frejno, M., Meng, C., Ruprecht, B., Oellerich, T., Scheich, S., Kleigrewe, K., et al. (2020). Proteome Activity Landscapes of Tumor Cell Lines Determine Drug Responses. Nat. Commun. 11 (1), 3639. doi:10.1038/s41467-020-17336-9
Frejno, M., Zenezini Chiozzi, R., Wilhelm, M., Koch, H., Zheng, R., Klaeger, S., et al. (2017). Pharmacoproteomic Characterisation of Human colon and Rectal Cancer. Mol. Syst. Biol. 13 (11), 951. doi:10.15252/msb.20177701
Gatti, L., and Zunino, F. (2005). Overview of Tumor Cell Chemoresistance Mechanisms. Methods Mol. Med. 111, 127–148. doi:10.1385/1-59259-889-7:127
Ghandi, M., Huang, F. W., Jané-Valbuena, J., Kryukov, G. V., Lo, C. C., Barretina, J., et al. (2019). Next-generation Characterization of the Cancer Cell Line Encyclopedia. Nature 569 (7757), 503–508. doi:10.1038/s41586-019-1186-3
Giddings, E. L., Champagne, D. P., Wu, M.-H., Laffin, J. M., Thornton, T. M., Valenca-Pereira, F., et al. (2021). Mitochondrial ATP Fuels ABC Transporter-Mediated Drug Efflux in Cancer Chemoresistance. Nat. Commun. 12 (1), 2804. doi:10.1038/s41467-021-23071-6
Gu, C., Kim, G. B., Kim, W. J., Kim, H. U., and Lee, S. Y. (2019). Current Status and Applications of Genome-Scale Metabolic Models. Genome Biol. 20 (1), 121. doi:10.1186/s13059-019-1730-3
Harte, M. T., Gorski, J. J., Savage, K. I., Purcell, J. W., Barros, E. M., Burn, P. M., et al. (2014). NF-κB Is a Critical Mediator of BRCA1-Induced Chemoresistance. Oncogene 33 (6), 713–723. doi:10.1038/onc.2013.10
Housman, G., Byler, S., Heerboth, S., Lapinska, K., Longacre, M., Snyder, N., et al. (2014). Drug Resistance in Cancer: an Overview. Cancers 6 (3), 1769–1792. doi:10.3390/cancers6031769
Huang, P., Li, F., Li, L., You, Y., Luo, S., Dong, Z., et al. (2018). lncRNA Profile Study Reveals the mRNAs and lncRNAs Associated with Docetaxel Resistance in Breast Cancer Cells. Sci. Rep. 8 (1), 17970. doi:10.1038/s41598-018-36231-4
Jiang, Y., Cheng, J., Yang, C., Hu, Y., Li, J., Han, Y., et al. (2017). An Ultrasensitive Fluorogenic Probe for Revealing the Role of Glutathione in Chemotherapy Resistance. Chem. Sci. 8 (12), 8012–8018. doi:10.1039/c7sc03338a
Kagohara, L. T., Zamuner, F., Davis-Marcisak, E. F., Sharma, G., Considine, M., Allen, J., et al. (2020). Integrated Single-Cell and Bulk Gene Expression and ATAC-Seq Reveals Heterogeneity and Early Changes in Pathways Associated with Resistance to Cetuximab in HNSCC-Sensitive Cell Lines. Br. J. Cancer 123 (1), 101–113. doi:10.1038/s41416-020-0851-5
Kim, C., Gao, R., Sei, E., Brandt, R., Hartman, J., Hatschek, T., et al. (2018). Chemoresistance Evolution in Triple-Negative Breast Cancer Delineated by Single-Cell Sequencing. Cell 173 (4), 879–893. doi:e813doi:10.1016/j.cell.2018.03.041
Kong, J., Lee, H., Kim, D., Han, S. K., Ha, D., Shin, K., et al. (2020). Network-based Machine Learning in Colorectal and Bladder Organoid Models Predicts Anti-cancer Drug Efficacy in Patients. Nat. Commun. 11 (1), 5485. doi:10.1038/s41467-020-19313-8
Kuciauskas, D., Dreize, N., Ger, M., Kaupinis, A., Zemaitis, K., Stankevicius, V., et al. (2019). Proteomic Analysis of Breast Cancer Resistance to the Anticancer Drug RH1 Reveals the Importance of Cancer Stem Cells. Cancers 11 (7), 972. doi:10.3390/cancers11070972
Kumar, S., Nandi, A., Singh, S., Regulapati, R., Li, N., Tobias, J. W., et al. (2021). Dll1+ Quiescent Tumor Stem Cells Drive Chemoresistance in Breast Cancer through NF-Κb Survival Pathway. Nat. Commun. 12 (1), 432. doi:10.1038/s41467-020-20664-5
Lee, S., Micalizzi, D., Truesdell, S. S., Bukhari, S. I. A., Boukhali, M., Lombardi-Story, J., et al. (2020). A post-transcriptional Program of Chemoresistance by AU-Rich Elements and TTP in Quiescent Leukemic Cells. Genome Biol. 21 (1), 33. doi:10.1186/s13059-020-1936-4
Lewis, J. E., Forshaw, T. E., Boothman, D. A., Furdui, C. M., and Kemp, M. L. (2021). Personalized Genome-Scale Metabolic Models Identify Targets of Redox Metabolism in Radiation-Resistant Tumors. Cel Syst. 12 (1), 68–81. doi:e11doi:10.1016/j.cels.2020.12.001
Lewis, J. E., and Kemp, M. L. (2021). Integration of Machine Learning and Genome-Scale Metabolic Modeling Identifies Multi-Omics Biomarkers for Radiation Resistance. Nat. Commun. 12 (1), 2700. doi:10.1038/s41467-021-22989-1
Li, Y.-K., Hsu, H.-M., Lin, M.-C., Chang, C.-W., Chu, C.-M., Chang, Y.-J., et al. (2021a). Genetic Co-expression Networks Contribute to Creating Predictive Model and Exploring Novel Biomarkers for the Prognosis of Breast Cancer. Sci. Rep. 11 (1), 7268. doi:10.1038/s41598-021-84995-z
Li, Z., Li, Y., Wang, X., and Yang, Q. (2021b). PPP2R2B Downregulation Is Associated with Immune Evasion and Predicts Poor Clinical Outcomes in Triple-Negative Breast Cancer. Cancer Cel Int 21 (1), 13. doi:10.1186/s12935-020-01707-9
Likhite, V. S., Stossi, F., Kim, K., Katzenellenbogen, B. S., and Katzenellenbogen, J. A. (2006). Kinase-specific Phosphorylation of the Estrogen Receptor Changes Receptor Interactions with Ligand, Deoxyribonucleic Acid, and Coregulators Associated with Alterations in Estrogen and Tamoxifen Activity. Mol. Endocrinol. 20 (12), 3120–3132. doi:10.1210/me.2006-0068
Lim, Z.-F., and Ma, P. C. (2019). Emerging Insights of Tumor Heterogeneity and Drug Resistance Mechanisms in Lung Cancer Targeted Therapy. J. Hematol. Oncol. 12 (1), 134. doi:10.1186/s13045-019-0818-2
Liu, H., Wang, S., Zhou, S., Meng, Q., Ma, X., Song, X., et al. (2019a). Drug Resistance-Related Competing Interactions of lncRNA and mRNA across 19 Cancer Types. Mol. Ther. - Nucleic Acids 16, 442–451. doi:10.1016/j.omtn.2019.03.011
Liu, R., Zhang, G., and Yang, Z. (2019b). Towards Rapid Prediction of Drug-Resistant Cancer Cell Phenotypes: Single Cell Mass Spectrometry Combined with Machine Learning. Chem. Commun. 55 (5), 616–619. doi:10.1039/c8cc08296k
Malik, V., Kalakoti, Y., and Sundar, D. (2021). Deep Learning Assisted Multi-Omics Integration for Survival and Drug-Response Prediction in Breast Cancer. BMC Genomics 22 (1), 214. doi:10.1186/s12864-021-07524-2
Marczyk, M., Patwardhan, G. A., Zhao, J., Qu, R., Li, X., Wali, V. B., et al. (2020). Multi-Omics Investigation of Innate Navitoclax Resistance in Triple-Negative Breast Cancer Cells. Cancers 12 (9), 2551. doi:10.3390/cancers12092551
Marine, J.-C., Dawson, S.-J., and Dawson, M. A. (2020). Non-genetic Mechanisms of Therapeutic Resistance in Cancer. Nat. Rev. Cancer 20 (12), 743–756. doi:10.1038/s41568-020-00302-4
Martin, S., Dudek-Peric, A. M., Garg, A. D., Roose, H., Demirsoy, S., Van Eygen, S., et al. (2017). An Autophagy-Driven Pathway of ATP Secretion Supports the Aggressive Phenotype of BRAFV600E Inhibitor-Resistant Metastatic Melanoma Cells. Autophagy 13 (9), 1512–1527. doi:10.1080/15548627.2017.1332550
Mukherjee, S., Adhikary, S., Gadad, S. S., Mondal, P., Sen, S., Choudhari, R., et al. (2020). Suppression of Poised Oncogenes by ZMYND8 Promotes Chemo-Sensitization. Cell Death Dis 11 (12), 1073. doi:10.1038/s41419-020-03129-x
Nava, M., Dutta, P., Farias-Eisner, R., Vadgama, J. V., and Wu, Y. (2019). Utilization of NGS Technologies to Investigate Transcriptomic and Epigenomic Mechanisms in Trastuzumab Resistance. Sci. Rep. 9 (1), 5141. doi:10.1038/s41598-019-41672-6
Niehr, F., Eder, T., Pilz, T., Konschak, R., Treue, D., Klauschen, F., et al. (2018). Multilayered Omics-Based Analysis of a Head and Neck Cancer Model of Cisplatin Resistance Reveals Intratumoral Heterogeneity and Treatment-Induced Clonal Selection. Clin. Cancer Res. 24 (1), 158–168. doi:10.1158/1078-0432.CCR-17-2410
Opdam, S., Richelle, A., Kellman, B., Li, S., Zielinski, D. C., and Lewis, N. E. (2017). A Systematic Evaluation of Methods for Tailoring Genome-Scale Metabolic Models. Cel Syst. 4 (3), 318–329. doi:10.1016/j.cels.2017.01.010
Poojan, S., Bae, S.-H., Min, J.-W., Lee, E. Y., Song, Y., Kim, H. Y., et al. (2020). Cancer Cells Undergoing Epigenetic Transition Show Short-Term Resistance and Are Transformed into Cells with Medium-Term Resistance by Drug Treatment. Exp. Mol. Med. 52 (7), 1102–1115. doi:10.1038/s12276-020-0464-3
Qi, W., and Zhang, Q. (2020). Gene's Co-expression Network and Experimental Validation of Molecular Markers Associated with the Drug Resistance of Gastric Cancer. Biomarkers Med. 14 (9), 761–773. doi:10.2217/bmm-2019-0504
Robinson, J. L., Kocabaş, P., Wang, H., Cholley, P.-E., Cook, D., Nilsson, A., et al. (2020). An Atlas of Human Metabolism. Sci. Signal. 13 (624), eaaz1482. doi:10.1126/scisignal.aaz1482
Ryu, J. Y., Kim, H. U., and Lee, S. Y. (2017). Framework and Resource for More Than 11,000 Gene-Transcript-Protein-Reaction Associations in Human Metabolism. Proc. Natl. Acad. Sci. USA 114 (45), E9740–E9749. doi:10.1073/pnas.1713050114
Ryu, J. Y., Kim, H. U., and Lee, S. Y. (2015). Reconstruction of Genome-Scale Human Metabolic Models Using Omics Data. Integr. Biol. (Camb.) 7 (8), 859–868. doi:10.1039/c5ib00002e
Sakellaropoulos, T., Vougas, K., Narang, S., Koinis, F., Kotsinas, A., Polyzos, A., et al. (2019). A Deep Learning Framework for Predicting Response to Therapy in Cancer. Cel Rep. 29 (11), 3367–3373. doi:e3364doi:10.1016/j.celrep.2019.11.017
Seashore-Ludlow, B., Rees, M. G., Cheah, J. H., Cokol, M., Price, E. V., Coletti, M. E., et al. (2015). Harnessing Connectivity in a Large-Scale Small-Molecule Sensitivity Dataset. Cancer Discov. 5 (11), 1210–1223. doi:10.1158/2159-8290.CD-15-0235
Seth, S., Li, C.-Y., Ho, I.-L., Corti, D., Loponte, S., Sapio, L., et al. (2019). Pre-existing Functional Heterogeneity of Tumorigenic Compartment as the Origin of Chemoresistance in Pancreatic Tumors. Cel Rep. 26 (6), 1518–1532. doi:e1519doi:10.1016/j.celrep.2019.01.048
Shoemaker, R. H. (2006). The NCI60 Human Tumour Cell Line Anticancer Drug Screen. Nat. Rev. Cancer 6 (10), 813–823. doi:10.1038/nrc1951
Sinkala, M., Nkhoma, P., Mulder, N., and Martin, D. P. (2021). Integrated Molecular Characterisation of the MAPK Pathways in Human Cancers Reveals Pharmacologically Vulnerable Mutations and Gene Dependencies. Commun. Biol. 4 (1), 9. doi:10.1038/s42003-020-01552-6
Subramanian, A., Narayan, R., Corsello, S. M., Peck, D. D., Natoli, T. E., Lu, X., et al. (2017). A Next Generation Connectivity Map: L1000 Platform and the First 1,000,000 Profiles. Cell 171 (6), 1437–1452. doi:e1417doi:10.1016/j.cell.2017.10.049
Tate, J. G., Bamford, S., Jubb, H. C., Sondka, Z., Beare, D. M., Bindal, N., et al. (2019). COSMIC: the Catalogue of Somatic Mutations in Cancer. Nucleic Acids Res. 47 (D1), D941–D947. doi:10.1093/nar/gky1015
Torre, E. A., Arai, E., Bayatpour, S., Jiang, C. L., Beck, L. E., Emert, B. L., et al. (2021). Genetic Screening for Single-Cell Variability Modulators Driving Therapy Resistance. Nat. Genet. 53 (1), 76–85. doi:10.1038/s41588-020-00749-z
Vasan, N., Baselga, J., and Hyman, D. M. (2019). A View on Drug Resistance in Cancer. Nature 575 (7782), 299–309. doi:10.1038/s41586-019-1730-1
Xu, Y., Dong, Q., Li, F., Xu, Y., Hu, C., Wang, J., et al. (2019). Identifying Subpathway Signatures for Individualized Anticancer Drug Response by Integrating Multi-Omics Data. J. Transl. Med. 17 (1), 255. doi:10.1186/s12967-019-2010-4
Yang, W., Soares, J., Greninger, P., Edelman, E. J., Lightfoot, H., Forbes, S., et al. (2013). Genomics of Drug Sensitivity in Cancer (GDSC): a Resource for Therapeutic Biomarker Discovery in Cancer Cells. Nucleic Acids Res. 41 (Database issue), D955–D961. doi:10.1093/nar/gks1111
Yu, L., Zhou, D., Gao, L., and Zha, Y. (2021). Prediction of Drug Response in Multilayer Networks Based on Fusion of Multiomics Data. Methods 192, 85–92. doi:10.1016/j.ymeth.2020.08.006
Zhang, J., Zhu, W., Wang, Q., Gu, J., Huang, L. F., and Sun, X. (2019). Differential Regulatory Network-Based Quantification and Prioritization of Key Genes Underlying Cancer Drug Resistance Based on Time-Course RNA-Seq Data. Plos Comput. Biol. 15 (11). e1007435 doi:10.1371/journal.pcbi.1007435
Zhao, Y., Li, Z. X., Zhu, Y. J., Fu, J., Zhao, X. F., Zhang, Y. N., et al. (2021). Single‐Cell Transcriptome Analysis Uncovers Intratumoral Heterogeneity and Underlying Mechanisms for Drug Resistance in Hepatobiliary Tumor Organoids. Adv. Sci. 8 (11). 2003897 doi:10.1002/advs.202003897
Zheng, H.-C. (2017). The Molecular Mechanisms of Chemoresistance in Cancers. Oncotarget 8 (35), 59950–59964. doi:10.18632/oncotarget.19048
Zheng, X., Carstens, J. L., Kim, J., Scheible, M., Kaye, J., Sugimoto, H., et al. (2015). Epithelial-to-mesenchymal Transition Is Dispensable for Metastasis but Induces Chemoresistance in Pancreatic Cancer. Nature 527 (7579), 525–530. doi:10.1038/nature16064
Keywords: cancer, drug resistance, omics, computational modeling, network-based model, machine learning, genome-scale metabolic model
Citation: Jung HD, Sung YJ and Kim HU (2021) Omics and Computational Modeling Approaches for the Effective Treatment of Drug-Resistant Cancer Cells. Front. Genet. 12:742902. doi: 10.3389/fgene.2021.742902
Received: 16 July 2021; Accepted: 20 September 2021;
Published: 06 October 2021.
Edited by:
Alessio Martino, National Research Council (CNR), ItalyReviewed by:
Adil Mardinoglu, King’s College London, United KingdomSatyakam Dash, The Pennsylvania State University (PSU), United States
Copyright © 2021 Jung, Sung and Kim. This is an open-access article distributed under the terms of the Creative Commons Attribution License (CC BY). The use, distribution or reproduction in other forums is permitted, provided the original author(s) and the copyright owner(s) are credited and that the original publication in this journal is cited, in accordance with accepted academic practice. No use, distribution or reproduction is permitted which does not comply with these terms.
*Correspondence: Hyun Uk Kim, ZWh1a2ltQGthaXN0LmFjLmty