- 1Genomics Core Facility, VetCore, University of Veterinary Medicine, Vienna, Austria
- 2Garvan Institute of Medical Research, Sydney, NSW, Australia
- 3Lowy Cancer Research Centre, School of Biomedical Sciences, University of New South Wales, Sydney, NSW, Australia
- 4Institute of Psychology, Karl-Franzens-University Graz, Graz, Austria
- 5Department of Behavioral and Cognitive Biology, University of Vienna, Vienna, Austria
- 6Department of Microbiology, Immunobiology and Genetics, University of Vienna, Vienna, Austria
We aimed at extending the repertoire of high-quality miRNA normalizers for reverse transcription-quantitative PCR (RT-qPCR) of human plasma with special emphasis on the extremely guanine-cytosine-rich portion of the miRNome. For high-throughput selection of stable candidates, microarray technology was preferred over small-RNA sequencing (sRNA-seq) since the latter underrepresented miRNAs with a guanine-cytosine (GC) content of at least 75% (p = 0.0002, n = 2). miRNA abundances measured on the microarray were ranked for consistency and uniformity using nine normalization approaches. The eleven most stable sequences included miRNAs of moderate, but also extreme GC content (45%–65%: miR-320d, miR-425-5p, miR-185-5p, miR-486-5p; 80%–95%: miR-1915-3p, miR-3656-5p, miR-3665-5p, miR-3960-5p, miR-4488-5p, miR-4497 and miR-4787-5p). In contrast, the seven extremely GC-rich miRNAs were not found in the two plasma miRNomes screened by sRNA-seq. Stem-loop RT-qPCR was employed for stability verification in 32 plasma samples of healthy male Caucasians (age range: 18–55 years). The lowest inter-individual variance of miRNA abundance was determined for miR-3665 and miR-1915-3p [coefficient of variation (CV) values: 0.08 and 0.50, respectively]. The eight most stable sequences included four extremely GC-rich miRNAs (miR-1915-3p, miR-3665, miR-4787-5p and miR-4497). The best-performing duo normalization factor (NF) for the condition of human plasma, miR-320d and miR-4787-5p, also included a GC-extreme miRNA. In summary, the identification of extremely guanine-cytosine-rich plasma normalizers will help to increase accuracy of PCR-based miRNA quantification, thus raise the potential that miRNAs become markers for psychological stress reactions or early and precise diagnosis of clinical phenotypes. The novel miRNAs might also be useful for orthologous contexts considering their conservation in related animal genomes.
Introduction
miRNAs are non-coding, post-transcriptional regulators of gene expression. They are rather uniform in size (18–25 nucleotides (Sen, 2014)), but differ vastly in GC content, base and backbone modifications (reviewed in (Bryzgunova et al., 2021)) as well as in expression depending on time and tissue (Diener et al., 2022). Out of the 2,300 mature miRNAs extrapolated for human cells (Alles et al., 2019), a significant fraction appears to be highly conserved in other animals (Penso-Dolfin et al., 2018). miRNAs fine-tune a broad diversity of molecular functions and processes by post-transcriptional repression of mRNAs and other transcripts (Bartel, 2018) or by exerting unconventional regulatory functions outside of this paradigm (Dragomir et al., 2018). Extracellular miRNAs such as body-fluid miRNAs widely vary in half-life, but are highly stable if associated with extracellular vesicles (Coenen-Stass et al., 2019). Pronounced resistance against degradation can be provided by complexing with proteins, lipoproteins, supramolecular complexes, and by packaging in membrane-free particles such as high-density lipoprotein particles (Vickers et al., 2011) or membranous extracellular vesicles (EVs) that comprise exosomes (Bryzgunova et al., 2021), shedding microvesicles (Hunter et al., 2008), and apoptotic bodies (Zernecke et al., 2009). These “shields” are likely responsible for the remarkable stability of cell-free miRNAs over prolonged storage at room temperature and multiple freeze-thaw cycles (Chen et al., 2008; Mitchell et al., 2008; Zampetaki et al., 2012; Rao et al., 2013). The half-life of extracellular mature miRNAs positively correlates with GC content (Coenen-Stass et al., 2019). Moreover, when a mature miRNA is “free”, not knotted into the RISC complex, folding into hairpin secondary structures can convey nuclease resistance (Belter et al., 2014). Features such as non-invasiveness, stability, and repeatability, combined with the ability of EV-shuttled miRNAs to cross the blood-brain barrier (Banks et al., 2020), makes circulating plasma/serum miRNAs an ideal diagnostic signature for brain-related contexts. These phenotypes range from cancer (Dai et al., 2020), stroke (Wang et al., 2018), neurodegenerative disorders (Wang and Zhang, 2020) and their early stages (Sheinerman et al., 2012) over depression (Chen et al., 2020; Li et al., 2021) up to stress and stress-associated diseases (Crigna et al., 2020).
A miRNA can increase its impact by targeting a set of genes that are involved in the same pathway or protein complex (Linsley et al., 2007), hence even relatively small changes of its abundance can cause an amplified biological effect (Calin and Croce, 2006). From the point of view of accurate miRNA quantification, intolerance towards poor normalization is especially important since alteration of miRNA abundance tends to be subtle, typically a few fold (Zhang et al., 2012). Technical noise reduction of qPCR-based measurement of a particular miRNA would be essential and can be leveraged by up-to-date data normalization with two to three stable endogenous controls appropriately selected for the experimental context of interest (Pagacz et al., 2020).
The identification of miRNAs that are stably abundant in human plasma, serum and extracellular vesicles was a research topic for more than a decade (Kroh et al., 2010; Meyer et al., 2010) up to the present (Faraldi et al., 2018). At the onset of the qPCR-based era of quantitative miRNA analysis, small nucleolar RNAs or small nuclear RNAs were frequently used for normalization (Song et al., 2012; Dufourd et al., 2019). Considering their low abundance in body fluid samples (Mitchell et al., 2008; Turchinovich et al., 2011; Qi et al., 2012; Zhang et al., 2016), higher variability (Wotschofsky et al., 2011; Schwarzenbach et al., 2015) and different class assignation, they were soon replaced by miRNA spike-ins (Roberts et al., 2014) and/or specific miRNAs (Zhang et al., 2016; Hakansson et al., 2018; Faraldi et al., 2019). Favourably, the normalizer in low-throughput profiling of extracellular miRNAs should come from the same molecule class, should be amenable to miRNA assay design, exhibit similar extraction efficiency, degradation and turnover regulation (Zhang et al., 2012) and shuttling. There are several layers requiring attention in the identification process of normalizers. Their extraction can be biased across samples and studies resulting from different extraction chemistries (Tan et al., 2015; Parker et al., 2021). The comparison of miRNA stability across different cohorts can be hampered by various physiological parameters impairing their abundance such as subject’s age (Noren Hooten et al., 2013; Ameling et al., 2015; Fehlmann et al., 2020; Maffioletti et al., 2020; Wang et al., 2020), gender (Duttagupta et al., 2011; Ameling et al., 2015), pregnancy (Tay et al., 2017) or ethnicity (Patel et al., 2019). Moreover, levels of circulating miRNAs can be impacted by alcohol consumption (Ignacio et al., 2015), dietary habit (Tarallo et al., 2014; Liu et al., 2020), poor sleep quality (Baek et al., 2021), obesity parameters (body mass index (Ameling et al., 2015), levels of visceral and subcutaneous adipose tissue, or body-fat percentage (Munetsuna et al., 2018)), settlement (e.g. high-altitude hypoxic environment (Yan et al., 2015)), psychological activity (Dal Lin et al., 2021), exercise (Wardle et al., 2015; Li et al., 2020) including training regime (Wardle et al., 2015) and cigarette smoking (Takahashi et al., 2013; Banerjee et al., 2015). The latter deregulated nine of the miRNA normalizers formerly recommended, namely let-7b, miR-16, miR-93-5p, miR-126-3p, miR-185, miR-188-5p, miR-191, miR-345 and miR-425-5p (Takahashi et al., 2013), thus representing a striking example for the importance of physiological context.
There are various methodologies for the selection of miRNA normalizers (Mathew et al., 2020). When choosing the profiling platform for undirected selection of stable miRNAs, the specific strengths and weaknesses of a technique have to be considered together with the experimental aim and setting (Mestdagh et al., 2014). For example, extreme base compositions of DNA or RNA such as AT- or GC-rich sequences, have caused an uneven coverage or even no coverage of reads on Illumina’s platform for next-generation sequencing (NGS) (Aird et al., 2011; Chen et al., 2013; Backes et al., 2016).
Aiming at the selection of high-quality normalizers for human plasma, our study considered the positive correlation between half-life and GC content of intra- and extracellular miRNAs (Kakimoto et al., 2016; Coenen-Stass et al., 2019). For high-throughput selection of stable miRNAs we preferred microarray analysis over NGS to avoid bias against highly GC-rich miRNAs. We also aimed at inspiring future research on miRNA markers related to psychological stress reactions by devising normalizers for the condition of cognitive stress-coping styles self-assessed using the Mainz Coping Inventory (MCI).
Materials and methods
Study design and participants
For microarray-based selection of stable miRNA normalizers (phase 1) and their verification by qPCR (phase 2), separate sets of male Caucasians were recruited. Grouping of participants in the two study phases was based on the dimensions of cognitive avoidance and vigilance scored by answers to the MCI self-report instrument. This theory-based, self-administered stimulus-response inventory is based on the participant’s stated behaviour in hypothetical physical or self-esteem harming situations (Krohne and Egloff, 1999). The model postulates four characteristic modes of coping with threat, sensitization (low cognitive avoidance and high vigilance), repression (high avoidance, low vigilance), anxiety (high scores on both dimensions), and non-defensiveness (low scores on both dimensions). Completed MCI surveys were evaluated using the statistical software platform IBM® SPSS® Statistics version 20 (SPSS Inc, Chicago, IL, United States of America).
In phase 1, a participant was assigned to one of the four MCI groups applying a threshold of ± 2 of the median scores for coping dimensions of cognitive avoidance and vigilance calculated for the cohort of all study participants (n = 64). Considering this “spacing zone”, blood donors of study phase-1 were selected when exhibiting score ranges of cognitive avoidance and vigilance of ≤23 to ≥27 and ≤16 to ≥20, respectively. To meet the required threshold of total RNA input requested by the manufacturer of the miRNA expression microarray, sample pooling had to be performed occasionally (Supplementary Table S1).
In phase 2, eight probands were selected per coping group without considering a “spacing zone” (n = 32; age range: 18–55 years; Supplementary Table S2).
Plasma RNAs subjected to sRNA-seq were derived from a proband that was also listed as participant of study phase-2 and from an independent adult male of Caucasian decent.
Plasma preparation
Participants reporting to be in good physical health donated ∼9 mL of venous blood that was collected into EDTA tubes (Vacuette® K2EDTA tubes; Greiner BioOne International AG, Kremsmünster, Austria). To exclude regulation of miRNA expression by the circadian rhythm (Shende et al., 2011), blood was taken around the same day time (between 8 and 11 a.m.). Cells and cellular debris were pelleted within 30 min after blood donation (3,000 × g, 10 min, room temperature). Aliquots of the supernatant were stored at −80°C until RNA extraction.
Extraction of plasma RNA
Efficiency of RNA extraction, synthesis of complementary DNA (cDNA) and PCR amplification was monitored using a synthetic spike-in control (McAlexander et al., 2013; Tan et al., 2015). It represented a miRNA-like sequence termed “spike-A” (Androvic et al., 2019) that was predicted to be linear. RNA was extracted using silica-membrane spin-column technology (Qiagen, Hilden, Germany) supplied by the miRNeasy Serum/Plasma Kit (phase 1) or the miRNeasy Serum/Plasma Advanced Kit (phase 2). In phase 2, 2 μg of glycogen carrier (Thermo Fisher Scientific GmbH, Vienna, Austria) was added per 200 μl plasma for improving recovery and reproducibility (Androvic et al., 2019). An aliquot of 200 μl plasma thawed on ice was mixed with lysis solution containing 2.0 × 10−5 spike copies. The concentration of the RNA extracted was slightly increased by reducing the volume of the eluant (45 μl instead of 50 μl RNase-free water). To exclude elevated levels of free oxyhaemoglobin stemming from sample haemolysis, spectrophotometric absorbance was measured at 414 nm applying a threshold of less than 0.2 (Kirschner et al., 2011).
Body-fluid RNA can rarely be assessed for integrity based on an RNA integrity number (RIN) value, as the RNA concentration is usually too low. When these samples have a high enough RNA concentration to detect a RIN value, it would be low. This is due to the fact that plasma/serum samples would contain short fragments of RNA (<1,000 nucleotides) being perceived by the microfluidic-measurement system as degraded RNA. Usually, ribosomal RNAs are not detected in these samples (Lam et al., 2012). Since RNA concentration extracted from plasma/serum volumes of ≤200 μl is at the limit of accurate quantification by spectrophotometric or microfluidic measurement (Schlosser et al., 2015; Marabita et al., 2016), normalization based on a fixed amount of starting RNA was considered impracticable and replaced by normalization to a fixed volume of template (Marabita et al., 2016). RNA was stored at −80°C until use.
sRNA-seq analysis
Small-RNA libraries were prepared without experimental size-selection (Faridani et al., 2016). The protocol included the use of unique molecular identifiers (UMIs) to counteract PCR stochasticity and to enable counting of RNA molecules. Briefly, a 3′ adaptor was ligated to total RNA using T4 RNA Ligase 2, truncated KQ (New England Biolabs, Ipswich, MA, United States). Then, free 3′ adaptors were removed enzymatically using 5′ deadenylase and Lambda exonuclease (New England Biolabs). Next, the 5′ adaptor containing a UMI was ligated using T4 RNA ligase 1 (New England Biolabs). The use of UMIs was reported to mitigate RT and amplification biases, hence to improve accuracy and reliability of miRNA quantification by sRNA-seq (Wright et al., 2019). Note that only sRNAs can be ligated to 5′ adaptor and mRNA ligation is inhibited due to the existence of the cap structure. RT was performed using Invitrogen™ SuperScript™ II Reverse Transcriptase (Thermo Fisher Scientific) followed by PCR using indexed primers and KAPA HiFi polymerase (Roche Diagnostics International AG, Rotkreuz, Switzerland). Final cDNA libraries were cleaned by AMPure XP beads (Beckman Coulter AB, Bromma, Sweden), checked by Bioanalyzer High Sensitivity DNA Kit (Agilent Technologies Sweden AB, Sundbyberg, Sweden) and subjected to NGS.
Sequencing-by-synthesis and mapping of reads were performed by the Core Facility for Next-Generation Sequencing of the University of Vienna (www.viennabiocenter.org/vbcf/next-generation-sequencing, part of Vienna BioCenter Core Facilities, Austria). Sequencing was performed on the HiSeq 2500 System (Illumina Inc, San Diego, CA, United States) using SR50 for a read length of 50 bp and the High Output Run Mode (v4). The computational pipeline used for read annotation (Faridani et al., 2016) is available at https://github.com/eyay/smallseq. First, the 8-bp unique molecular identifiers were removed from the 5′ end of the raw reads using scripts of the Computational Genomics Analysis Tools (CGAT) collection https://cgat.readthedocs.io/en/latest/cgat.html. Second, adapters and the CA spacer preceding each read were removed using the adapter trimmer Cutadapt (Martin, 2011). UMI sequences were added to the header of the reads for the ease of later processing. Third, reads were mapped against the human reference genome (ncbi38_hg20) using the Spliced Transcripts Alignment to a Reference (STAR) aligner ((Dobin et al., 2013); https://github.com/alexdobin/STAR/releases; version 2.7.3a) run with the settings outSAMstrandField: intronMotif, outFilterMultimapNmax: 50, outFilterScoreMinOverLread: 0, outFilterMatchNmin: 18, outFilterMatchNminOverLread: 0, and outFilterMismatchNoverLmax: 0.04, alignIntronMax: 1. This disabled spliced alignment and mismatching within the first 25 bp of the read while allowing a single mismatch in total. PCR duplicates were removed by deduplicating and counting the sequences using UMI tools (https://github.com/CGATOxford/UMI-tools), thereby collapsing the reads based on the adjacency network approach. In the last step, the biotypes of the remaining reads were assigned based on the annotation of mirBase (www.mirbase.org; version 21), the Genomic tRNA Database (GtRNAdb; (Chan and Lowe, 2016); http://gtrnadb.ucsc.edu) and the GENCODE project (www.gencodegenes.org; release 22). Sequence molecules mapping to multiple genomic locations were assigned by a weighting approach (molecule number/number of annotated locations). miRNAs expressed from different genomic locations were collapsed.
miRNA profiling with expression microarray
Plasma abundance of human miRNAs was measured on the GeneChip® miRNA 3.0 Array (Thermo Fisher Scientific; formerly Affymetrix, Santa Clara, CA, United States) that requires a minimum input of 130 ng RNA according to the manufacturer. Three biological replicates were measured per coping mode using a template amount of 176 ng RNA. To overcome limitations in sample quantity and to reduce variance, template RNA was pooled from three to eight individuals (sample set 1, Supplementary Table S1). Microarray expression analysis was outsourced to a commercial service provider (AROS Applied Biotechniques A/S, Aarhus, Denmark, www.arosab.com; part of Eurofins Genomics, https://eurofinsgenomics.eu/) and analysed as follows. Two unimodal signal distributions, background noise caused by false-positive hybridization and the actual signal of an expressed miRNA, and their superimposition were observed in the signal number per intensity plot. To separate the two signal modes, a cut-off was manually set and only miRNAs expressed above this threshold were further analysed. Targets not called present by the Detection Above Average (DABG) algorithm implemented by Affymetrix were excluded. Consistency of miRNA expression was evaluated following normalization with nine approaches (global mean normalization, generalized procrustean analysis, loess, cyclic loess, modified loess, quantile normalization, variance stabilization normalization, variance stabilization normalization based on invariant miRNAs, and Z-score normalization). If not implemented in a normalization workflow, background correction was performed by applying the R function “bg.correct.rma” included in the R package ‘affy’ (Gautier et al., 2004). For each expressed miRNA, the occurrences of top-ten ranks across the nine normalization approaches were counted. Consistency of abundance was assumed if a miRNA was called present on at least eleven of the twelve arrays. To rank the stabilities of the consistently expressed miRNA set, their signal intensity values were log2 transformed and treated similarly to cycle-of-quantification (Cq) values of RT-qPCR. Expression stability was assessed with four common statistical algorithms, geNorm (Vandesompele et al., 2002), Normfinder (Andersen et al., 2004), BestKeeper (Pfaffl et al., 2004), and the ΔCt method (Silver et al., 2006) accessed at the comprehensive platform RefFinder ((Xie et al., 2012); www.heartcure.com.au/reffinder/). The ranks of the resulting four lists were aggregated using the R package “RankAggreg” (Pihur et al., 2009) that assigns an appropriate weight to an individual gene and calculates the geometric mean of their weights for the overall final ranking.
Design of stem-loop RT-qPCR oligonucleotides
Specificity of the stem-loop RT-qPCR assays was achieved by the stem-loop RT primer and the forward PCR primer except of the assay against miR-93-5p. In this case, specificity was derived solely from a specific 3′ end of the forward PCR primer (Supplementary Table S3). Assays used two versions of a universal reverse amplification primer and dye-based monitoring of product accumulation.
Considering that in silico tools such as Primer-BLAST ((Ye et al., 2012); https://www.ncbi.nlm.nih.gov/tools/primer-blast/) or Human BLAT Search (Kent, 2002); https://genome.ucsc.edu/cgi-bin/hgBlat) could not easily be adapted to predict the specificity of oligonucleotides designed for miRNA detection, e.g. of those employed by stem-loop RT-qPCR, their specificity was evaluated as follows. FASTA-formatted, 5′ to 3′ sequences of all mature human miRNAs were downloaded from miRBase (release 22 March 2018 (Kozomara and Griffiths-Jones, 2014); https://www.mirbase.org) and saved in a Microsoft Excel spreadsheet. The 5′ to 3′ sequences and their reverse counterparts were sorted alphabetically to facilitate assessment of the cross-hybridization potential of the forward PCR primer and the stem-loop RT primer, respectively (Supplementary Table S3). Based on this alignment, for certain extremely GC-rich miRNAs the common single-stranded specific stretch of six-nucleotide at the 3′ end of the stem-loop RT primer (Varkonyi-Gasic and Hellens, 2011) had to be extended by one to three nucleotides.
All stem-loop RT primers were designed for optional application of a universal short hydrolysis probe modified with locked nucleic acid (LNA) bases (Tm: ∼70°C; https://geneglobe.qiagen.com/us/tools/tm-prediction). In the 8-mer probe sequence 5′-T+G+G+C+T+C+T+G all nucleotides except the last at the 5′ terminus were LNA-modified. This was to allow cleavage by the 5′ to 3′ nuclease activity of Taq DNA polymerase (Echwald et al., 2013).
Quantification of mature miRNAs by dye-based stem-loop RT-qPCR
Plasma abundance of mature miRNAs was quantified using stem-loop RT-qPCR that discriminates between mature and pre- or pri-miRNA by 100-fold (Kramer, 2011). Before stem-loop RT-qPCR, the structure of the stem-loop RT primer was re-constituted by denaturing at 95°C for 10 min and reassociation achieved by reducing the temperature to 75°C slowly at a speed of 1°C/s, 1 h incubations at 75, 68, 65 and 62°C, and incubation at 60°C for several hours (Kramer, 2011). For pre-RT annealing, 2 μl template RNA was mixed with 1 μl of 1 μM RT primer and 3.25 μl RNase-free water, and heated to 65°C for 1 min to remove secondary structures. After the annealing step performed for 30 min at the specific temperature requested by the particular RT primer (24°C–45°C), the mixture was immediately placed on ice. Subsequently, it was supplemented with 1 mM dNTP mix (Solis BioDyne, Tartu, Estonia), 1 × RT-Buffer, 10 U RiboLock RNase Inhibitor and 100 U RevertAid Reverse Transcriptase (all Thermo Fisher Scientific) to obtain a final RT volume of 10 μl. Following pulsed RT (60 cycles: 30°C for 30 s, 42°C for 30 s, 50°C for 2 s) performed to minimise unspecific interaction and increase detection sensitivity (Chen et al., 2005), cDNA synthesis was terminated by enzyme inactivation at 85°C for 5 min. Incubation steps of RT were performed on a MJ Research PTC-200 Thermal Cycler (Bio-Rad, Hercules, CA, United States). To monitor the impact of putative DNA contamination, a mock-RT reaction was carried out for each experimental RNA.
The signal of stem-loop RT-qPCR was generated using the SYTO™ 82 Orange Fluorescent Nucleic Acid Stain (Thermo Fisher Scientific). It produces a signal-to-noise ratio of ∼40 (https://www.gene-quantification.de/syto-dyes.pdf) and a narrow peak during amplicon melting (Gudnason et al., 2007), is without AT- or GC preference (Giglio et al., 2003; Gudnason et al., 2007) and not inhibitory to the PCR (Gudnason et al., 2007), binds DNA mainly dependent on charge and primarily in the minor groove (Eischeid, 2011), and exhibits lower nucleic-acid affinity compared to the common SYBR Green I (Eischeid, 2011), hence, has less potential to form primer hybrids (Gudnason et al., 2007).
The 15-μl qPCR contained 1.2 mM dye, 0.2 μM of each primer (Sigma-Aldrich, St. Louis, MO, United States), 1 × B2 buffer (Tris-HCl (NH4)2SO4 and Tween 20), 0.2 mM of each dNTP, 2.5 or 3.0 mM MgCl2 depending on assay, 1 U of the hot-start Taq DNA polymerase HOT FIREPol® (all Solis BioDyne) and 4 µl cDNA that was eight-fold diluted in 1 × TE buffer (10 mM Tris-HCl, 1 mM EDTA, pH 8). Amplification reactions were carried out in a 96 well-plate format and scored using the LightCycler® 96 Instrument operated by the LightCycler® 96 application version 1.1.0.1320 (Roche Diagnostics GmbH, Vienna, Austria). Following template denaturation and activation of the polymerase at 95°C for 15 min, amplification was performed over 40 cycles (denaturation for 20 s at 95°C, annealing for 20 s at 59°C, 60°C, 61°C or 62°C, and elongation for 10 s at 72°C). Fluorescence was monitored during the extension step. The specificity of each primer set was verified by melt-curve analysis. Following initial denaturation at 95°C for 10 s and renaturation at 65°C for 60 s, amplicons were dissociated by ramping from 65 to 97°C in 0.2°C increments per second. Fluorescence signals were continuously acquired at each increment.
S-poly(T) miRNA method for quantification of miR-1469
The S-Poly(T) miRNA method (Kang et al., 2012) comprises miRNA polyadenylation and RT with a S-Poly(T) primer that contains sites for binding of a universal reverse PCR primer and a universal probe, of an oligo (dT)11 sequence and of several miRNA-specific bases at its 3′ end. RT of miR-1469 was primed with 5′-CAGTGGAGGGTCGGAGGTCAGTGGCTCTGTTTTTTTTTTTGGAGCC, where the underlined nucleotides are target specific. The 8-mer presented in bold designates the arbitrary sequence of the hydrolysis probe 5′-T+G+G+C+T+C+T+G used to monitor amplification (see above). Amplification was primed with the universal reverse primer 5′-CAGTGGAGGGTCGGAGGT and the specific forward primer 5′-ataatttaCTCGGCGCGGG, where lower-case letters designate the arbitrary Tm-enhancing tail.
Efficiency of qPCR amplification, adjustment of measured Cq values and outlier treatment
Amplification efficiency was calculated from the exponential phase of the amplification curve. In detail, not baseline-corrected (raw) fluorescence values exported from the qPCR system’s operating software in the Real-time PCR Data Markup Language were imported into Real-time PCR Miner 4.0 ((Zhao and Fernald, 2005); http://miner.ewindup.cn/miner/) run under default settings. Cq values measured at Efi < 1 were corrected according to the term Cq × log10 (Efi + 1)/log102) (Kubista et al., 2007), where Efi represents the mean of the individual amplification efficiencies determined for the assayed cDNAs.
A qPCR replicate was treated as outlier and discarded when it caused a standard deviation of more than 0.5 calculated for the quadruplicate Cq values of an experimental sample (qPCR duplicates for each of the two cDNA replicates). If the means of the qPCR duplicates run for each cDNA replicates differed by more than one cycle, the cDNA replicate that exhibited the highest deviation from the global average of the 32-sample cohort was removed from analysis.
Assessment of miRNA abundance stability
CV analysis
The CV value (ratio of standard deviation to the mean) was used as a quality control metrics reflecting the variation of a reference gene across all samples. It was used as the simplest parameter to compare the variation of the sequence abundances independent from their actual levels (Boda et al., 2009).
Efficiency adjustment of Cq values
Efficiency-adjusted, not log-transformed Cq values were used as input to determine the optimal number of reference sequences for appropriate RT-qPCR normalization and to rank the expression stability of single miRNAs and duos or trios of miRNAs.
Determination of the optimal number of sequences for RT-qPCR normalization
The optimal number of miRNAs required for appropriate normalization was concluded based on the pairwise variation V value of the geNorm tool. The software determined the optimal number of normalizers based on pairwise variation (Vn/Vn+1) between two sequential factors, NFn and NFn+1. The common cut-off value of Vn/Vn+1 < 0.15 determined the lowest number of genes for accurate normalisation (Vandesompele et al., 2002).
Abundance stability of single normalizers
The stability of single miRNAs across the four stress-coping modes was assessed using the statistical algorithms, geNorm (Vandesompele et al., 2002; Hellemans et al., 2007) incorporated in the qbase + software 3.2 (Biogazelle, Ghent, Belgium; (Hellemans et al., 2007); www.qbaseplus.com), and the Excel-based programs NormFinder (Andersen et al., 2004) and BestKeeper (Pfaffl et al., 2004). The resulting three rank scores were compiled into a comprehensive rank using the ComprFinder algorithm (Zhang et al., 2020). In contrast to the geometric averaging applied by the RefFinder platform, ComprFinder maintains the dimension of an individual stability gain or loss by standardising the range of the stability score across the individual statistical algorithms.
Systematic assessment of normalizer combinations
The abundance stability of all possible NFs calculated as the geometric mean of two or three miRNAs, was compared using NormiRazor ((Grabia et al., 2020); https://norm.btm.umed.pl), a tool that includes the geNorm, NormFinder and BestKeeper algorithms.
Statistical analysis
Statistical analysis was performed using GraphPad Prism 9.2.0 software (GraphPad Software Inc, San Diego, CA, United States), Microsoft Excel 2010 (Microsoft Corporation, Redmond, United States) or The R Project for Statistical Computing (https://www.r-project.org) if not otherwise stated.
Pearson’s correlation between the two best-ranked miRNA normalizers for the condition of plasma irrespective of copying style, of their efficiency-adjusted Cq values was assessed at https://astatsa.com/CorrelationTest/using default settings (two-sided, true correlation: non-zero).
The CV value (relative standard deviation) was used to reflect the extent of variability in miRNA abundance. Pearson’s correlation between miRNA’s CV and minimal free energy (MFE) values was calculated using the Correlation Coefficient Calculator (Statistics Kingdom, Melbourne, Australia; https://www.statskingdom.com/correlation-calculator.html).
MFE of folding calculated for miRNA sets with moderate or extremely high GC contents (below or above 64%) was evaluated for statistical significance using the Mann-Whitney U test Calculator with default settings (https://www.statskingdom.com/170median_mann_whitney.html).
Normality of miRNA’s Cq values reflecting their plasma abundances was examined by the Shapiro-Wilk test. Since data were not always normally distributed, the non-parametric Mann-Whitney U test was used to assess differential abundance of a miRNA across a stress-coping dimension (low versus high scores of cognitive avoidance or low versus high vigilance).
Results
Nowadays, stably expressed miRNAs can be selected from miRNomes by various approaches including RNA-seq and the historically older microarray technology. Due to its low amount of RNA, profiling plasma by RNA-seq would involve an exponential amplification step during construction of the sequencing library. However, common protocols for NGS cause under-representation of highly GC-rich sequences owing to inefficient amplification by PCR. Therefore, we asked whether miRNA profiling by sRNA-seq that does not involve measures against GC-rich sequence bias (Faridani et al., 2016) would similarly be challenged. Expression data measured on a miRNA microarray platform were used for comparison.
Bias of small RNA-seq against extremely GC-rich miRNAs
GC bias is inherent to any PCR-amplified RNA-seq library preparation (Shi et al., 2021; Santacruz et al., 2022). For example, transcript regions with a GC content above 70% can strongly be underrepresented (Sendler et al., 2011). Here, we asked whether this limited ability to capture the true sequence representation would also affect our sRNA-seq workflow. Exemplarily, we profiled two plasma samples from probands exhibiting low or high MCI scores for vigilance. While both the set of mature human miRNAs contained in the miRBase repository and the two NGS-derived plasma miRNomes did not follow a normal distribution (p < 0.001, Supplementary Table S4), the sRNA-seq workflow was biased from false-negative detection of extremely GC-rich miRNAs (≥75% G/C, p = 0.0002, Figure 1 and Supplementary Table S5). Therefore, microarray expression analysis was subsequently employed to select stable miRNAs at high throughput.
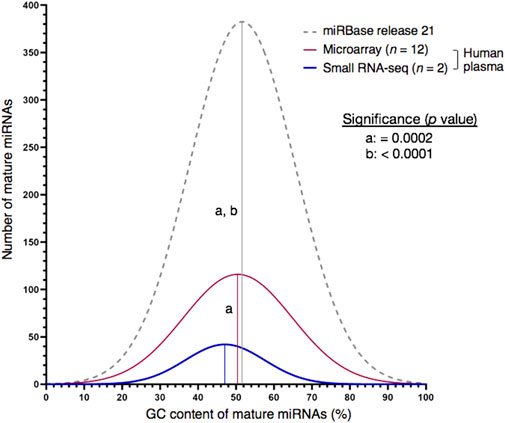
FIGURE 1. Choice of high-throughput profiling technique may cause detection bias for miRNA targets with very high GC content (≥75%). Whereas the GC plot of miRNAs contained in the miRBase database was similar with the one of miRNAs detected by miRNA microarray analysis on the Affymetrix GeneChip® platform, small RNA-seq produced a left-skewed distribution as indicated by Shapiro-Wilk test for normality. Numbers of miRNAs annotated by miRBase database or detected by expression analysis in blood plasma of human males: 2,654 (miRBase, release 22), 837 (miRNA microarray, n = 12) and 226 (sRNA-seq, n = 2). Mean GC proportions are depicted by bell-shaped curves: miRBase database (grey), miRNA microarray (red) and sRNA-seq (blue). Non-significant and significant differences between two means are depicted by the same or a different letter, respectively. Significance was analysed with Kruskal–Wallis test followed by Dunn’s test using a threshold of p < 0.01.
Selection of stable miRNAs from microarray expression data
When profiling human plasma for miRNAs, it is important to remember that it contains traces of bacterial and viral RNA in addition to miRNAs and other transcripts of the host (Semenov et al., 2012). The miRNA microarray data produced for our human plasma samples contained a high fraction of miRNAs with low expression (Supplementary Figure S1). We argued that the bimodal distribution observed in the signal number per signal intensity plot is caused by a mixture of two unimodal distributions with either high or low signal intensities. Whereas the former reflects the distribution of actually expressed miRNAs, the latter is derived from extremely low expressed or even unexpressed miRNAs as well as from artefacts due to cross hybridization of various RNA fragments (Semenov et al., 2012) or other background noise. The exclusion of low-intensity signals resulted in a list of 103 mature human miRNAs (Supplementary Table S6). Constitutive expression across the experimental samples was assumed if a miRNA could be detected on at least eleven of the twelve arrays evaluated by the DABG algorithm. This request was supposed to compensate for possible cases of false-negative detection by the algorithm. A set of 37 mature miRNAs passing this criterion (Supplementary Table S7) were analysed by nine approaches for microarray data normalization (Supplementary Table S8). The particular effects of the normalizations on the distribution and balancing of individual hybridization intensities were illustrated in Supplementary Figure S2. Application of the nine approaches identified 24 miRNAs with at least one top-ten call (Table 1). For each of the nine normalization workflows, we assessed their expression stability using the RefFinder tool that delivered a comprehensive ranking based on the four common statistical algorithms geNorm, NormFinder, BestKeeper and ΔCt method. Finally, a list of putative reference miRNAs was derived by aggregating the individual ranks (Supplementary Table S9).
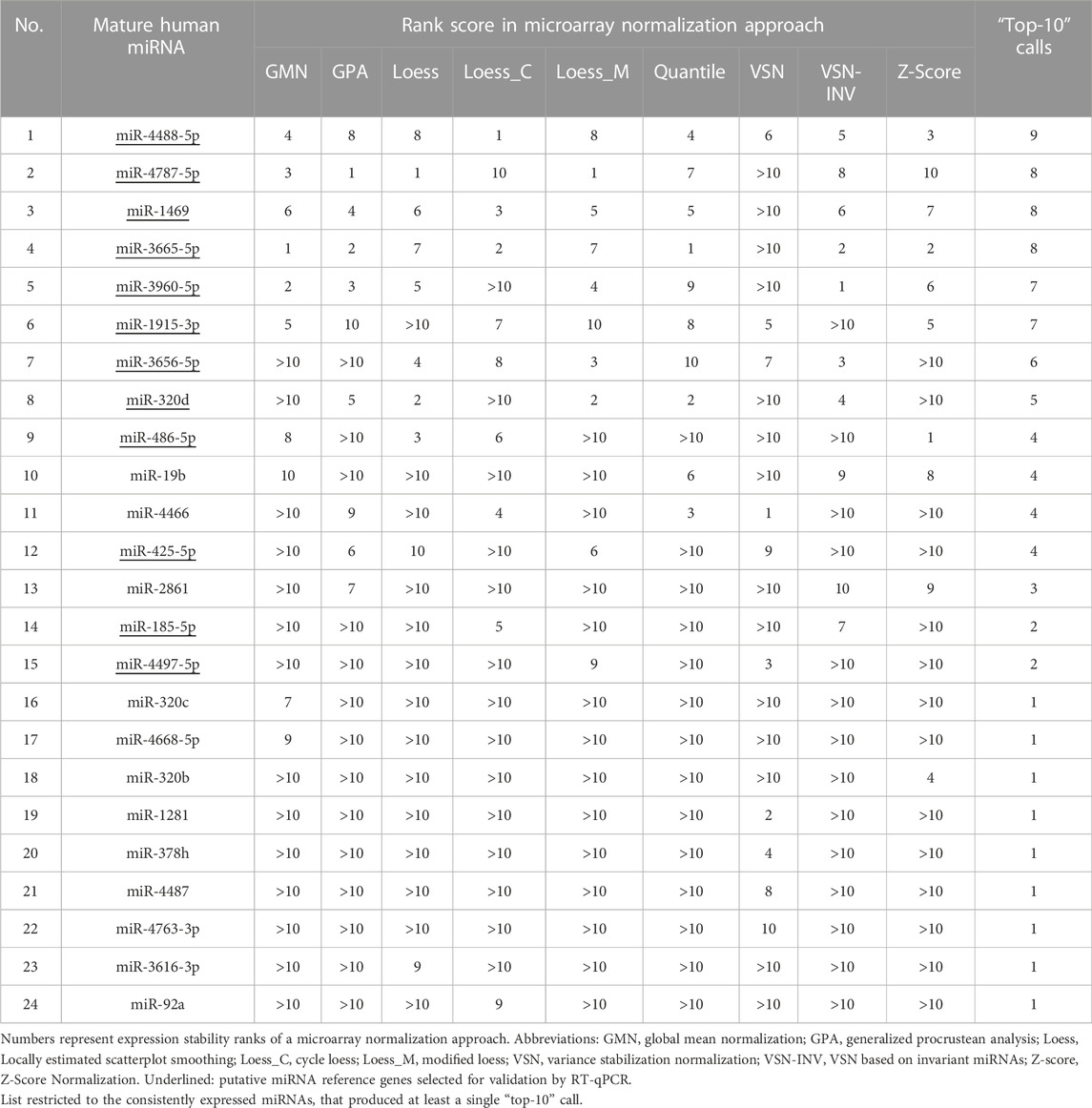
TABLE 1. Number of top-ten ranks of consistently expressed human plasma miRNAs across nine approaches for microarray data normalization.
Set-up of stem-loop RT-qPCR assays
From the consensus list of the nine approaches for microarray data normalization, twelve constitutively and stably abundant miRNAs were chosen to validate their plasma stability by stem-loop RT-qPCR (Table 2). The set covered inter- and intragenic miRNAs with a wide range of chromosomal locations, GC contents and secondary structures including several well-formed hairpins with very negative MFE values of up to −8 kcal/mol (Supplementary Table S9 and Figure 2). Each of these normalizer candidates was (also) found to be residing within circulating exosomes of the blood (Supplementary Table S9).
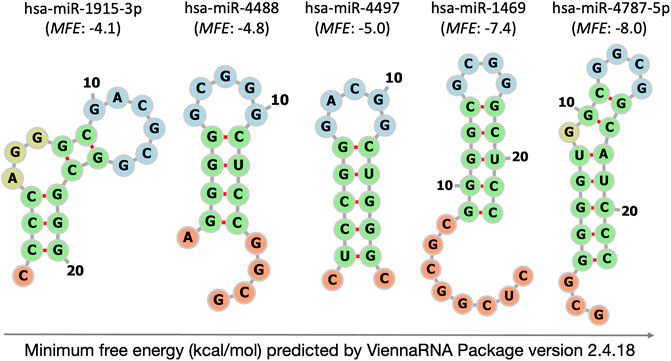
FIGURE 2. Self-complementarity of extremely GC-rich mature miRNAs at 37°C predicted by ViennaRNA Package version 2.4.18. The illustration of intramolecular hybridisation is limited to the five microarray-selected miRNAs with most negative ΔG values. MFE: minimum free energy (kcal/mol). Structural details depicted by colour are loop (blue), stem (green), single-stranded tail (orange) and bulge or internal loop (olive).
For comparison, we tested the plasma normalizers miR-126-3p (Faraldi et al., 2019) and miR-93-5p (Liu et al., 2014; Niu et al., 2016; Zalewski et al., 2017). Both are among the seven potential miRNA reference genes included in Qiagen’s Serum/Plasma Focus miRNA PCR Panels (Qiagen, 2022).
Stem-loop RT-qPCR assays were established for 13 of the 14 miRNAs of interest (except of miR-1469, see below). Positive RNA templates for assay set-up were isolated from the human cancer cell lines HepG2, MDA-MB-231 and TR146 (Supplementary File S1). The latter cell line used for most of the assays (n = 9), was subjected to genotype-based authentication at a commercial service provider. The profiling of eight autosomal short tandem repeat (STR) core markers and the gender marker amelogenin confirmed its identity (Supplementary File S1 and Supplementary Table S10). Only one allele differed from the publicly available STR profile.
Specificity of stem-loop RT-qPCR assays was concluded from melt-curve analysis and gel electrophoresis (Supplementary Figure S3 and Supplementary File S1). The level and stability of miRNA plasma abundances was determined on a sample set collected from 32 probands classified into the four stress-coping styles based on the self-report instrument MCI. They represented high-anxious and non-defensive stress copers as well as sensitizers and repressors (n = 8 per group; Supplementary Table S2). In general, the miRNAs targeted were consistently detected by stem-loop RT-qPCR with the exception of the sporadically appearing miR-4488 (two positives).
The abundance of miR-1469 was quantified using the S-Poly(T) method (Supplementary Figure S4). It was found to be consistently abundant across the 32 plasma samples, although at highly diverse and mostly rather low levels (∆Cq: 12, median Cq: 30.1, respectively).
Consequentially, miR-4488 and miR-1469 were excluded from further analysis of abundance stability due to sporadic occurrence or poor stability.
Stability assessment of the selected miRNA reference candidates
The remaining twelve miRNA sequences were consistently detected (Table 3; Figure 3 and Supplementary Table S11). Their abundance levels differed considerably ranging from low-to medium in case of miR-486-5p and miR-320d (Cq values of 27.5 and 27.2, respectively) up to very high in case of miR-3960 and miR-4787-5p (Cq: 12.6). They differed considerably in abundance stability ranging from poor to high or extraordinarily high (CV range: 2.76 down to 0.50 and 0.08; Figure 3, Table 3, and Supplementary Table S12) and showed mostly low inter- and intragroup variability assessed with the NormFinder algorithm (Figure 4).
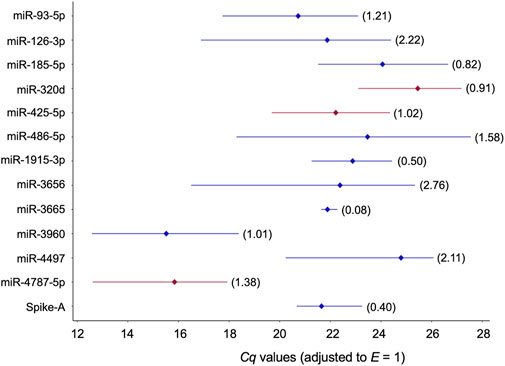
FIGURE 3. Profiles of Cq values of 13 candidate reference miRNAs across 32 blood plasmas derived from probands with different modes of cognitive stress coping. Cq values were corrected with the amplification efficiency value determined for the respective qPCR assay (Table 2). The median value is depicted by the diamond. The percent coefficient of variation (CV) is given in parentheses. miRNAs composing the two-gene NFs either recommended for plasma or the two stress-coping dimensions are highlighted in red.
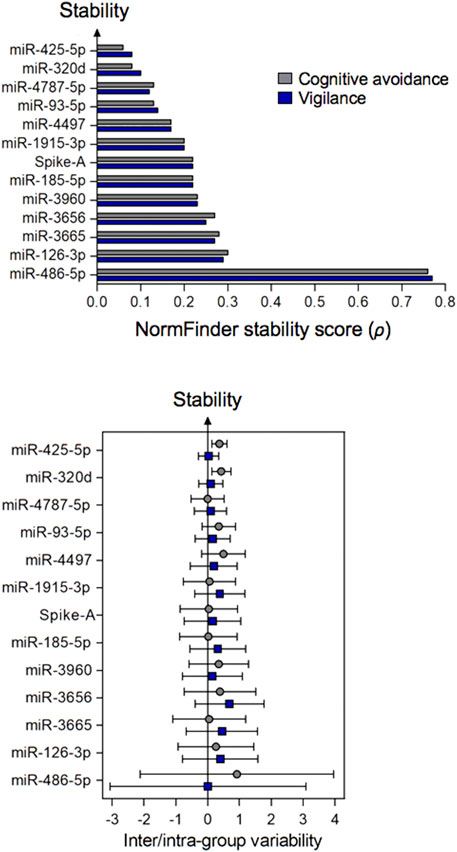
FIGURE 4. Stability of plasma miRNAs (top figure) and their inter- and intra-group variation (bottom figure) calculated by the NormFinder algorithm. Samples were grouped according to the stress-coping dimensions of cognitive avoidance or vigilance. The circles or squares of the bottom figure relate to inter- or intra-group analysis, respectively. Error bars reflect the extent of variability. Minimal intergroup variation (close to zero) and small error bars are indicative for a stable miRNA. miRNAs are ranked in descending order based on the stability scores of NormFinder.
While these putative normalizers were not differently abundant between phase-2 participants with either low or high vigilance scores (p > 0.15), the analogous comparison for the coping dimension of cognitive avoidance yielded only a single case of different abundance (miR-3665: p = 0.040 without adjusting for multiple testing). Correcting the significance levels according to the method of (Benjamini and Hochberg, 1995) recommended for multiple comparisons rendered this relationship non-significant. However, it has to be noted that while the p-value adjustment decreases the probability of a type-I error, it might favour a type-II error, thus reduces the power of the test. Taken together, we concluded that in general, intergroup variation of the twelve consistently abundant miRNAs would likely not impair quantification of a miRNA of interest in the experimental setting.
Since knotting the ends of mature miRNAs into a hairpin-stem structure can extend their half-life (Belter et al., 2014), we asked whether miRNA’s plasma stability would be related to the extent of secondary-structure formation. However, MFE of folding was not related to the CV of miRNA’s plasma abundance (Pearson’s correlation coefficient r = 0.03, p = 0.93, n = 12). This clearly implicated that the steady state of miRNA abundance is regulated in a more complex manner going beyond the mere influence of secondary structure.
Folding of spike-in miRNA controls
A synthetic, unstructured miRNA-like arbitrary sequence was used as spike-in process control to monitor RNA extraction, cDNA synthesis, and qPCR technical quality. Its uniformity of recovery was good based on low CV (0.40) and was only outperformed by miR-3665 (Table 3). We are unaware of attempts to further increase the uniformity of spike recovery by using miRNAs with knotty secondary structure that were reported to convey nuclease resistance outside of the RNA-induced silencing complex (Belter et al., 2014). Disclosed sequences of miRNA spike-ins currently in use for mammalian gene expression analysis are either linear (MFE: 0.0 kcal/mol) or form hairpins of low to moderate stability that did not involve both ends of the miRNA spike (MFE: 0.4 to −3.5 kcal/mol, Figure 2, Supplementary Table S13). Future experimentation should address, whether the recovery of spike-in controls can be improved by using worm (Caenorhabditis elegans), plant (Arabidopsis thaliana or Oryza sativa), artificial or other miRNAs that form hairpins of elevated stability similar to those predicted for our extremely GC-rich miRNAs (MFE ≤ −4.1 kcal/mol, Figure 2).
Stability of single miRNAs and their duo and trio combinations
The stability of single miRNAs across the plasma samples of healthy male Caucasians was ranked using the mainstream algorithms geNorm, NormFinder, and BestKeeper. In line with various other reports, differences were observed between the three resulting lists (Table 3). To compile a final ranking, we applied ComprFinder, a novel tool that maintains the dimension of an individual stability gain or loss by standardising the range of the stability score across the individual statistical algorithms. The top-eight ranks were occupied by a mixture of miRNAs with moderate or extreme GC content (moderate: miR-425-5p, miR-320d, miR-93-5p, and miR-185-5p; extreme: miR-1915-3p, miR-3665, miR-4787-5p, and miR-4497, respectively; Table 3). Ranking miR-93-5p at position three, confirmed a former report that recommended this miRNA as normalizer for plasma or serum samples of healthy individuals (Supplementary Table S14).
The intrinsic variability that a single reference shows across individual samples and/or experimental conditions, can be minimised by using multiple internal controls (Chervoneva et al., 2010). Often, studies based on high-throughput transcriptome data found a combination of just two normalizers to be sufficient for quantitative accuracy. This minimises the number of assays to be processed for each sample, template input and costs, hence increases practicality. The minimal/optimal number of reference genes for adequate normalization of qPCR data is commonly determined using the geNorm’s pairwise variation coefficient Vn/Vn+1 calculated between the sequential normalization factors NFn and NFn+1. A Vn/Vn+1 value of less than 0.15 indicates that n reference genes/sequences would be the best compromise between accuracy and expenditure compared with n + 1 genes/sequences. For the condition of human plasma, just two miRNAs were sufficient for accurate normalization and adding another normalizer sequence would not significantly improve reliability (Supplementary Figure S5). Meeting the cut-off of V2/3 < 0.15 as in our case of undirected high-throughput selection, indicates that high-quality references were selected for the particular experimental condition. Therefore, all possible sequence duos, but also trio combinations were assessed for stability assessment. Screening was performed using NormiRazor, a web tool that integrates the established normalization algorithms geNorm, NormFinder and BestKeeper. It best-ranked the combination of miR-320d and miR-4787-5p for all 32 plasma samples, i.e. without consideration of coping-style stratification (Table 4, Supplementary Table S15). For either of the stress-coping dimensions of cognitive avoidance or vigilance, the duo NF composed of miR-425p and miR-4787-5p was identified as best performing (Table 4).
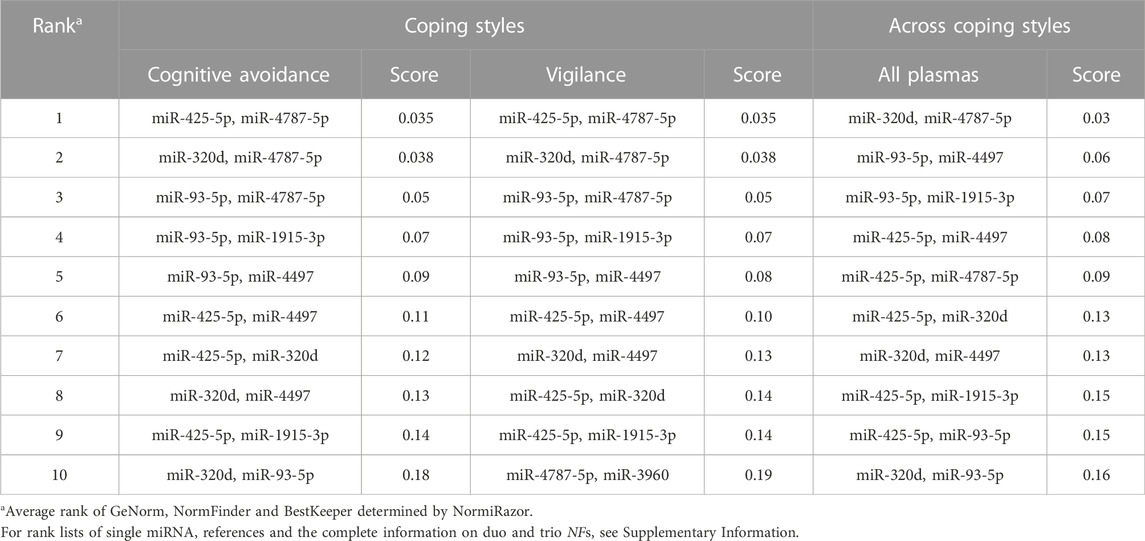
TABLE 4. Stability scores of miRNA reference gene pairs in plasma of male Caucasians differing in their mode of cognitive stress coping.
Central to choosing multiple reference genes for normalization of RT-qPCR expression data is to avoid the possibility of co-regulation. To minimise the chance for this bias, we assured that the NF-composing miRNAs were neither members of the same miRNA family (miR family members are often co-expressed, either from the same pri-miR transcript or from distinct but co-regulated loci) nor were they clustered on the genome (genomic distance: >10 kb). A Pearson’s correlation coefficient of r ≥ 0.9 was considered as threshold to highlight the rather poor effectiveness of a combination of two miRNA normalizers (Babion et al., 2017). In our case, this critical level of correlation was not reached, neither for miR-320d & miR-4787-5p that were found to be most appropriate for all 32 plasma samples (r = 0.69, p = 0.000017), nor for the combination of miR-425-5p & miR-4787-5p being most stable under the conditions of cognitive avoidant or vigilant stress-coping (r = 0.66 to 0.82, p = 0.02–0.11).
Associations between the mRNA targets and pathways of the paired miRNAs were either absent (miR-320d and miR-4787-5p) or minor (miR-425-5p and miR-4787-5p) according to the miRNA Pathway Dictionary Database (Supplementary File S1). Mostly, they targeted different signalling or metabolic pathways (Supplementary Figure S6, Supplementary Tables S16, S17).
Impaired ability of sRNA-seq to detect the novel extremely GC-rich miRNA normalizers
Here we return to the bias of sRNA-seq against detection of miRNAs with high and extreme GC contents (see above) providing a focused analysis for the novel extremely GC-rich miRNA normalizers (Supplementary Table S18). While our sRNA-seq protocol successfully detected the sequences of moderate GC-content such as miR-93-5p, miR-126-3p, miR-185-5p, miR-320d, miR-425-5p, and miR-486-5p (GC content: 45%–65%), it caused false-negative detection of the extremely GC-rich miRNAs miR-1915-3p, miR-3656-5p, miR-3665-5p, miR-3960-5p, miR-4488-5p, miR-4497 and miR-4787-5p (GC content: 82%–95%; Table 2). In contrast to the miRNAs with moderate GC contents (<64%), they were significantly more prone to intramolecular hybridization as indicated by more negative ΔG values (medians of −0.7 and −4.5 kcal/mol, respectively, Supplementary Table S9; p = 0.012). This confirmed the expectation that sequences of elevated GC content are likely to have more stable secondary structure (Chan et al., 2009).
Discussion
The complexity of biological systems contradicts the hypothetical concept of a single-best normalization strategy fitting all physiological contexts. For the condition of plasma sampled from healthy Caucasian men and additionally stratifying for modes of cognitive stress-coping, this study combined expression analysis on a miRNA microarray and stem-loop RT-qPCR to select and verify stable miRNAs, respectively (Figure 5). Expression analysis on a miRNA microarray is still a contemporary technique (Pimentel et al., 2015) that is superior to sRNA-seq in targeting the highly GC-rich fraction of the miRNome (Figure 1 and see below) and well balances precision, accuracy, sample input, expediency and costs.
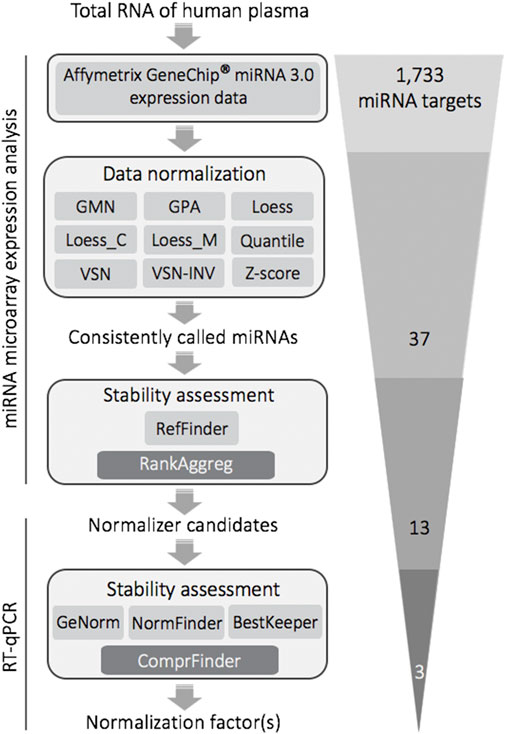
FIGURE 5. Selection and validation of stable reference miRNAs for RT-qPCR-based quantitative expression analysis of plasma donated by human males differing in cognitive stress coping styles: methodological flow chart and filtering steps. The final trio of stable miRNAs was composed of miR-320d, miR-4787-5p (duo NF for plasma of Caucasian males) and miR-425-5p (composing the duo NF together with miR-4787-5p when plasma samples were stratified into cognitive avoidant or vigilant copers). Abbreviations: GMN, Global Mean Normalization; GPA, Generalized Procrustes Analysis; Loess, locally estimated scatterplot smoothing; Loess_C, Cyclic Loess; Loess_M, Modified Loess; VSN, Variance Stabilization Normalization; VSN-INV, VSN based on invariant miRNAs; Z-score, Z-Score Normalization; RefFinder, comprehensive analysis of stability based on the common statistical algorithms geNorm, NormFinder, BestKeeper, and ΔCt method; RankAggreg, software package for weighted rank aggregation; ComprFinder, comprehensive stability ranking algorithm based on weighted standardisation.
Our strategy resulted in an extended miRNA repertoire for context-optimised RT-qPCR normalization (n = 8). The set of normalizers included miR-3960-5p, the tumour suppressors miR-185-5p, miR-3665 and miR-4787-5p, an oncogenic miRNA, miR-425-5p, and the Janus-faced tumour molecules miR-93-5p, miR-320d and miR-1915-3p that fulfil either tumour-suppressive oroncogenic functions depending on the cellular context and the downstream targets they affect (Han et al., 2020). Their key characteristics are summarised in Supplementary Table S17. All circulating miRNA candidates of this study might (also) appear as extracellular exosomal miRNAs (exomiRs) in human plasma/serum (Supplementary Table S9). The lipid-bilayer enclosing of exosomes might add to their stability in the cell-free circulation by protecting against nucleolytic degradation. Moreover, ordered secondary structures can provide nuclease resistance for miRNAs residing outside of the RNA-induced silencing complex (Gangemi et al., 2020), thus, can further add to their superior stability. At least in silico, the extremely GC-rich mature miRNAs were more structured as indicated by more negative ΔG values (p = 0.013, Supplementary Table S9), thus confirming the assumption that a sequence of higher GC content is likely to have more stable folding.
The high quality of the novel miRNA normalizers is indicated by the following. First, by the suggestion of geNorm to use just two sequences for adequate normalization. Second, stability evaluation using CV analysis identified two miRNAs that showed extraordinary or high uniformity of abundance across the plasma samples of the 32 human males, namely, miR-3665 and miR-1915-3p (CVs: 0.08 and 0.50, respectively). Third, we confirmed the status of stable abundance for circulating miR-320d and miR-425-5p. While miR-320d was formerly identified as a suitable reference gene for the plasma of healthy human males (Faraldi et al., 2019), miR-425-5p was reported to be stable in plasma exosomes of healthy donors likely of Chinese descent (Han et al., 2020). However, an ethnicity issue might limit the use of the latter miRNA. miR-425-5p that is among the seven potential miRNA reference genes of Qiagen’s Serum/Plasma Focus miRNA PCR Panels (Qiagen, 2022), is ∼8-fold more abundant in the blood of black compared to white individuals (Patel et al., 2019). It is currently unknown how this ethnicity difference translates to plasma or serum samples of other ethnic groups.
The disclosure of assay oligonucleotides (Table 2) allows to estimate the putative impact of heterogeneity resulting from isomiRs— 5′, 3′ or internal modifications of a canonical miRNA regulated post-transcriptionally (Tomasello et al., 2021). For example, the recommended plasma/serum normalizer hsa-miR-425-5p ((Qiagen, 2019); Supplementary Table S14 and this study) is subject of dynamic regulation of 3′ isomiRs resulting from nucleotide addition, deletion or substitution of a canonical miRNA (Dhanoa et al., 2019). Since 3′-addition or -trimming variants are relatively widespread (Nejad et al., 2018), the canonical length of a miRNA, currently defined in the miRBase based on a landmark study of miRNA cloning, has to be taken with caution.
This study and earlier reports encountered the difficulty or even failure to detect our GC-extreme miRNA normalizer candidates in human plasma by sRNA-seq (Supplementary Table S19, Figure 1). This is in line with the common underrepresentation of GC-extreme sequences in NGS libraries (Browne et al., 2020) and especially applies to miRNome sequencing of biofluids such as plasma where the poor sensitivity of NGS meets low template input (Raabe et al., 2014; Backes et al., 2016; Coenen-Stass et al., 2018; Shi et al., 2021). Hence, these types of sample material require a minimum number of PCR cycles (e.g., 4–8) to produce sufficient library yield. In addition to GC bias of PCR amplification (Wright et al., 2019) implemented into common sRNA-seq protocols (Burgos et al., 2013; Backes et al., 2016; Yagi et al., 2017), coverage bias can be caused by various steps of the library preparation such as adapter ligation (Barberan-Soler et al., 2018), RT (Wright et al., 2019), and other sample-handling procedures (Browne et al., 2020). For example, bias of PCR amplification can be mitigated or even resolved by various means (Shi et al., 2021) including automation of library preparation (Santacruz et al., 2022), fine-tuning of cycling conditions, the use of PCR additives and/or outperforming polymerases (and master mixes) that reduce the impact of secondary structure (Wright et al., 2019).
In summary, a detailed understanding of the source and nature of the under-coverage of extremely GC-rich miRNAs by sRNA-seq is essential to interpret the sequencing outcome and to avoid or at least reduce the bias, especially for low input materials (reviewed by (Shi et al., 2021)). Alternatively, the complementary use of methods might help to reveal the full spectrum of miRNA abundances.
Conclusion
Each expression study set-up requires adequate selection of multiple, preferably same-class normalizers to reduce technical noise, hence to minimise masking or exaggerating biologically meaningful changes. Here, we expanded the panel of putative miRNA normalizers for the context of human (and possibly also animal) plasma by adding miR-3665, miR-1915-3p, miR-185-5p, miR-320d, miR- 3960-5p, miR-425-5p, miR-93-5p, and miR-4787-5p. For the GC-moderate miR-320d of this panel, we confirmed its status of a plasma normalizer reported before. In addition, we identified two extraordinarily stable miRNAs, miR-3665, miR-1915-3p, that were extremely GC-rich (>80%, Supplementary Table S9). Another extremely GC-rich miRNA normalizer, miR-4787-5p, was part of the most appropriate two-gene NF recommended for healthy male Caucasians classified as either cognitive-avoidant or vigilant stress copers according to the self-report instrument MCI. The novel normalizers can serve as a tool for identifying miRNA plasma biomarkers related to cognitive stress-coping and other (patho)physiological human (or animal) conditions.
Last but not least, we enhanced assay reliability by providing information on putative cross-hybridization of the designed stem-loop RT-qPCR oligonucleotides and by disclosing their sequences as requested by the original minimum information for the publication of quantitative PCR experiments (MIQE) guidelines (Bustin et al., 2009). This would also facilitate cost reduction compared to a commercial solution.
Data availability statement
The datasets presented in this study can be found in online repositories. The names of the repository/repositories and accession number(s) can be found below: https://www.ncbi.nlm.nih.gov/geo/, GSE59359; https://www.ncbi.nlm.nih.gov/geo/, GSE209568.
Ethics statement
The studies involving human participants were reviewed and approved by Ethics Committee at the University of Graz and by the Research Ethics Committee of the Austrian Armed Forces. The participants provided their written informed consent to participate in this study.
Author contributions
Conceptualisation: AS and RS; resources: BW; funding: AS, OF and RS; determination of coping styles: AS; construction of sRNA-seq library: OF; assay design: VB and RS; qPCR data: VB; stability assessment: A-TA; data curation: A-TA; visualisation: VB, A-TA and RS; project administration and supervision: VB and RS; preparation of manuscript draft: RS. All authors approved the manuscript and agreed to be held accountable for the content therein.
Funding
This study was supported by resources of the University of Graz (Austria), the Karolinska Institutet Stockholm (Sweden), and the University of Veterinary Medicine Vienna (Austria).
Acknowledgments
We are very grateful to Moritz Staltner for the various normalizations of miRNA microarray expression data. We thank Eva-Maria Messner for making us familiar with the coping style issue, Claudia Stocsits for mapping the reads of small RNA-seq, Claus Vogl for valuable discussion, Anna Orlova, Daniela Allmer, Benjamin Siart and Manuela Sophie Koller for technical assistance as well as Georg Mair, Gerald Eder, Martin Hofer, Christoph Kreitzer, and Lukas Tombor for support. Winfried Neuhaus and Haider Sami are gratefully acknowledged for gifting the cancer cells.
Conflict of interest
The authors declare that the research was conducted in the absence of any commercial or financial relationships that could be construed as a potential conflict of interest.
Publisher’s note
All claims expressed in this article are solely those of the authors and do not necessarily represent those of their affiliated organizations, or those of the publisher, the editors and the reviewers. Any product that may be evaluated in this article, or claim that may be made by its manufacturer, is not guaranteed or endorsed by the publisher.
Supplementary material
The Supplementary Material for this article can be found online at: https://www.frontiersin.org/articles/10.3389/fgene.2022.1058668/full#supplementary-material
Abbreviations
Cq, cycle of quantification; CV, coefficient of variation; E, PCR efficiency; EVs, extracellular vesicles; GC content, guanine-cytosine content; LNA, locked nucleic acid; MCI, Mainz Coping Inventory; MFE, minimal free energy; miRNA, micro RNA; NF, normalization factor; NGS, next-generation sequencing; RT-qPCR, reverse-transcription quantitative PCR; sRNA-seq, small RNA sequencing; STR, short tandem repeat; UMI, unique molecular identifier.
References
Aird, D., Ross, M. G., Chen, W. S., Danielsson, M., Fennell, T., Russ, C., et al. (2011). Analyzing and minimizing PCR amplification bias in Illumina sequencing libraries. Genome Biol. 12, R18. doi:10.1186/gb-2011-12-2-r18
Alles, J., Fehlmann, T., Fischer, U., Backes, C., Galata, V., Minet, M., et al. (2019). An estimate of the total number of true human miRNAs. Nucleic Acids Res. 47, 3353–3364. doi:10.1093/nar/gkz097
Ameling, S., Kacprowski, T., Chilukoti, R. K., Malsch, C., Liebscher, V., Suhre, K., et al. (2015). Associations of circulating plasma microRNAs with age, body mass index and sex in a population-based study. BMC Med. Genomics 8, 61. doi:10.1186/s12920-015-0136-7
Andersen, C. L., Jensen, J. L., and Orntoft, T. F. (2004). Normalization of real-time quantitative reverse transcription-PCR data: A model-based variance estimation approach to identify genes suited for normalization, applied to bladder and colon cancer data sets. Cancer Res. 64, 5245–5250. doi:10.1158/0008-5472.CAN-04-0496
Androvic, P., Romanyuk, N., Urdzikova-Machova, L., Rohlova, E., Kubista, M., and Valihrach, L. (2019). Two-tailed RT-qPCR panel for quality control of circulating microRNA studies. Sci. Rep. 9, 4255. doi:10.1038/s41598-019-40513-w
Babion, I., Snoek, B. C., De Wiel, M. A., Wilting, S. M., and Steenbergen, R. D. M. (2017). A strategy to find suitable reference genes for miRNA quantitative PCR analysis and its application to cervical specimens. J. Mol. Diagn. 19, 625–637. doi:10.1016/j.jmoldx.2017.04.010
Backes, C., Sedaghat-Hamedani, F., Frese, K., Hart, M., Ludwig, N., Meder, B., et al. (2016). Bias in high-throughput analysis of miRNAs and implications for biomarker studies. Anal. Chem. 88, 2088–2095. doi:10.1021/acs.analchem.5b03376
Baek, S. J., Ban, H. J., Park, S. M., Lee, B., Choi, Y., Baek, Y., et al. (2021). Circulating microRNAs as potential diagnostic biomarkers for poor sleep quality. Nat. Sci. Sleep. 13, 1001–1012. doi:10.2147/NSS.S311541
Banerjee, A., Waters, D., Camacho, O. M., and Minet, E. (2015). Quantification of plasma microRNAs in a group of healthy smokers, ex-smokers and non-smokers and correlation to biomarkers of tobacco exposure. Biomarkers 20, 123–131. doi:10.3109/1354750X.2014.1000970
Banks, W. A., Sharma, P., Bullock, K. M., Hansen, K. M., Ludwig, N., and Whiteside, T. L. (2020). Transport of extracellular vesicles across the blood-brain barrier: Brain pharmacokinetics and effects of inflammation. Int. J. Mol. Sci. 21, 4407. doi:10.3390/ijms21124407
Barberan-Soler, S., Vo, J. M., Hogans, R. E., Dallas, A., Johnston, B. H., and Kazakov, S. A. (2018). Decreasing miRNA sequencing bias using a single adapter and circularization approach. Genome Biol. 19, 105. doi:10.1186/s13059-018-1488-z
Belter, A., Gudanis, D., Rolle, K., Piwecka, M., Gdaniec, Z., Naskret-Barciszewska, M. Z., et al. (2014). Mature MiRNAs form secondary structure, which suggests their function beyond RISC. Plos One 9, e113848. doi:10.1371/journal.pone.0113848
Benjamini, Y., and Hochberg, Y. (1995). Controlling the false discovery rate - a practical and powerful approach to multiple testing. J. R. Stat. Soc. Ser. B 57, 289–300. doi:10.1111/j.2517-6161.1995.tb02031.x
Boda, E., Pini, A., Hoxha, E., Parolisi, R., and Tempia, F. (2009). Selection of reference genes for quantitative real-time RT-PCR studies in mouse brain. J. Mol. Neurosci. 37, 238–253. doi:10.1007/s12031-008-9128-9
Browne, P. D., Nielsen, T. K., Kot, W., Aggerholm, A., Gilbert, M. T. P., Puetz, L., et al. (2020). GC bias affects genomic and metagenomic reconstructions, underrepresenting GC-poor organisms. Gigascience 9, giaa008. doi:10.1093/gigascience/giaa008
Bryzgunova, O., Konoshenko, M., Zaporozhchenko, I., Yakovlev, A., and Laktionov, P. (2021). Isolation of cell-free miRNA from biological fluids: Influencing factors and methods. Diagn. (Basel) 11, 865. doi:10.3390/diagnostics11050865
Burgos, K. L., Javaherian, A., Bomprezzi, R., Ghaffari, L., Rhodes, S., Courtright, A., et al. (2013). Identification of extracellular miRNA in human cerebrospinal fluid by next-generation sequencing. RNA 19, 712–722. doi:10.1261/rna.036863.112
Bustin, S. A., Benes, V., Garson, J. A., Hellemans, J., Huggett, J., Kubista, M., et al. (2009). The MIQE guidelines: Minimum information for publication of quantitative real-time PCR experiments. Clin. Chem. 55, 611–622. doi:10.1373/clinchem.2008.112797
Calin, G. A., and Croce, C. M. (2006). MicroRNA-cancer connection: The beginning of a new tale. Cancer Res. 66, 7390–7394. doi:10.1158/0008-5472.CAN-06-0800
Chan, C. Y., Carmack, C. S., Long, D. D., Maliyekkel, A., Shao, Y., Roninson, I. B., et al. (2009). A structural interpretation of the effect of GC-content on efficiency of RNA interference. BMC Bioinforma. 10, S33. doi:10.1186/1471-2105-10-S1-S33
Chan, P. P., and Lowe, T. M. (2016). GtRNAdb 2.0: An expanded database of transfer RNA genes identified in complete and draft genomes. Nucleic Acids Res. 44, D184–D189. doi:10.1093/nar/gkv1309
Chen, C., Ridzon, D. A., Broomer, A. J., Zhou, Z., Lee, D. H., Nguyen, J. T., et al. (2005). Real-time quantification of microRNAs by stem-loop RT-PCR. Nucleic Acids Res. 33, e179. doi:10.1093/nar/gni178
Chen, X., Ba, Y., Ma, L. J., Cai, X., Yin, Y., Wang, K. H., et al. (2008). Characterization of microRNAs in serum: A novel class of biomarkers for diagnosis of cancer and other diseases. Cell Res. 18, 997–1006. doi:10.1038/cr.2008.282
Chen, Y. C., Liu, T., Yu, C. H., Chiang, T. Y., and Hwang, C. C. (2013). Effects of GC bias in next-generation-sequencing data on de novo genome assembly. PLoS One 8, e62856. doi:10.1371/journal.pone.0062856
Chen, Y., Shi, J. B., Liu, H. Y., Wang, Q., Chen, X. X., Tang, H., et al. (2020). Plasma microRNA array analysis identifies overexpressed miR-19b-3p as a biomarker of bipolar depression distinguishing from unipolar depression. Front. Psychiatry 11, 757. doi:10.3389/fpsyt.2020.00757
Chervoneva, I., Li, Y., Schulz, S., Croker, S., Wilson, C., Waldman, S. A., et al. (2010). Selection of optimal reference genes for normalization in quantitative RT-PCR. BMC Bioinforma. 11, ARTN253. doi:10.1186/1471-2105-11-253
Coenen-Stass, A. M. L., Magen, I., Brooks, T., Ben-Dov, I. Z., Greensmith, L., Hornstein, E., et al. (2018). Evaluation of methodologies for microRNA biomarker detection by next generation sequencing. RNA Biol. 15, 1133–1145. doi:10.1080/15476286.2018.1514236
Coenen-Stass, A. M. L., Pauwels, M. J., Hanson, B., Martin Perez, C., Conceição, M., Wood, M. J. A., et al. (2019). Extracellular microRNAs exhibit sequence-dependent stability and cellular release kinetics. RNA Biol. 16, 696–706. doi:10.1080/15476286.2019.1582956
Crigna, A. T., Samec, M., Koklesova, L., Liskova, A., Giordano, F. A., Kubatka, P., et al. (2020). Cell-free nucleic acid patterns in disease prediction and monitoring-hype or hope? EPMA J. 11, 603–627. doi:10.1007/s13167-020-00226-x
Dai, J., Su, Y., Zhong, S., Cong, L., Liu, B., Yang, J., et al. (2020). Exosomes: Key players in cancer and potential therapeutic strategy. Signal Transduct. Target. Ther. 5, 145. doi:10.1038/s41392-020-00261-0
Dal Lin, C., Marinova, M., Brugnolo, L., Rubino, G., Plebani, M., Iliceto, S., et al. (2021). Rapid changes of miRNAs-20, -30, -410, -515, -134, and -183 and telomerase with psychological activity: A one year study on the relaxation response and epistemological considerations. J. Tradit. Complement. Med. 11, 409–418. doi:10.1016/j.jtcme.2021.02.005
Dhanoa, J. K., Verma, R., Sethi, R. S., Arora, J. S., and Mukhopadhyay, C. S. (2019). Biogenesis and biological implications of isomirs in mammals-a review. ExRNA 1, 3. doi:10.1186/s41544-018-0003-8
Diener, C., Keller, A., and Meese, E. (2022). Emerging concepts of miRNA therapeutics: From cells to clinic. Trends Genet. 38, 613–626. doi:10.1016/j.tig.2022.02.006
Dobin, A., Davis, C. A., Schlesinger, F., Drenkow, J., Zaleski, C., Jha, S., et al. (2013). Star: Ultrafast universal RNA-seq aligner. Bioinformatics 29, 15–21. doi:10.1093/bioinformatics/bts635
Donati, S., Ciuffi, S., and Brandi, M. L. (2019). Human circulating miRNAs real-time qRT-PCR-based analysis: An overview of endogenous reference genes used for data normalization. Int. J. Mol. Sci. 20, 4353. ARTN 4353. doi:10.3390/ijms20184353
Dragomir, M. P., Knutsen, E., and Calin, G. A. (2018). SnapShot: Unconventional miRNA functions. Cell 174, 1038–1038 e1. doi:10.1016/j.cell.2018.07.040
Dufourd, T., Robil, N., Mallet, D., Carcenac, C., Boulet, S., Brishoual, S., et al. (2019). Plasma or serum? A qualitative study on rodents and humans using high-throughput microRNA sequencing for circulating biomarkers. Biol. Methods Protoc. 4, bpz006. doi:10.1093/biomethods/bpz006
Duttagupta, R., Jiang, R., Gollub, J., Getts, R. C., and Jones, K. W. (2011). Impact of cellular miRNAs on circulating miRNA biomarker signatures. PLoS One 6, e20769. doi:10.1371/journal.pone.0020769
Echwald, S. M., Andreasen, D., and Mouritzen, P. (2013). “LNA™: Adding new functionality to PCR,” in PCR technology: Current innovations, third edition. Editors T. NOLAN, and S. A. BUSTIN (Taylor & Francis).
Eischeid, A. C. (2011). SYTO dyes and EvaGreen outperform SYBR Green in real-time PCR. BMC Res. Notes 4, 263. doi:10.1186/1756-0500-4-263
Faraldi, M., Gomarasca, M., Banfi, G., and Lombardi, G. (2018). Free circulating miRNAs measurement in clinical settings: The still unsolved issue of the normalization. Adv. Clin. Chem. 87, 113–139. doi:10.1016/bs.acc.2018.07.003
Faraldi, M., Gomarasca, M., Sansoni, V., Perego, S., Banfi, G., and Lombardi, G. (2019). Normalization strategies differently affect circulating miRNA profile associated with the training status. Sci. Rep. 9, 1584. doi:10.1038/s41598-019-38505-x
Faridani, O. R., Abdullayev, I., Hagemann-Jensen, M., Schell, J. P., Lanner, F., and Sandberg, R. (2016). Single-cell sequencing of the small-RNA transcriptome. Nat. Biotechnol. 34, 1264–1266. doi:10.1038/nbt.3701
Fehlmann, T., Lehallier, B., Schaum, N., Hahn, O., Kahraman, M., Li, Y., et al. (2020). Common diseases alter the physiological age-related blood microRNA profile. Nat. Commun. 11, 5958. doi:10.1038/s41467-020-19665-1
Gangemi, C. M. A., Alaimo, S., Pulvirenti, A., Garcia-Vinuales, S., Milardi, D., Falanga, A. P., et al. (2020). Endogenous and artificial miRNAs explore a rich variety of conformations: A potential relationship between secondary structure and biological functionality. Sci. Rep. 10, 453. ARTN 453. doi:10.1038/s41598-019-57289-8
Gautier, L., Cope, L., Bolstad, B. M., and Irizarry, R. A. (2004). Affy - analysis of Affymetrix GeneChip data at the probe level. Bioinformatics 20, 307–315. doi:10.1093/bioinformatics/btg405
Giglio, S., Monis, P. T., and Saint, C. P. (2003). Demonstration of preferential binding of SYBR Green I to specific DNA fragments in real-time multiplex PCR. Nucleic Acids Res. 31, e136. doi:10.1093/nar/gng135
Grabia, S., Smyczynska, U., Pagacz, K., and Fendler, W. (2020). NormiRazor: Tool applying GPU-accelerated computing for determination of internal references in microRNA transcription studies. BMC Bioinforma. 21, 425. doi:10.1186/s12859-020-03743-8
Gudnason, H., Dufva, M., Bang, D. D., and Wolff, A. (2007). Comparison of multiple DNA dyes for real-time PCR: Effects of dye concentration and sequence composition on DNA amplification and melting temperature. Nucleic Acids Res. 35, e127. doi:10.1093/nar/gkm671
Hakansson, K. E. J., Sollie, O., Simons, K. H., Quax, P. H. A., Jensen, J., and Nossent, A. Y. (2018). Circulating small non-coding RNAs as biomarkers for recovery after exhaustive or repetitive exercise. Front. Physiol. 9, 1136. ARTN 1136. doi:10.3389/fphys.2018.01136
Han, Z. J., Li, Y. Y., Zhang, J., Guo, C. Y., Li, Q., Zhang, X., et al. (2020). Tumor-derived circulating exosomal miR-342-5p and miR-574-5p as promising diagnostic biomarkers for early-stage Lung Adenocarcinoma. Int. J. Med. Sci. 17, 1428–1438. doi:10.7150/ijms.43500
Hellemans, J., Mortier, G., De Paepe, A., Speleman, F., and Vandesompele, J. (2007). qBase relative quantification framework and software for management and automated analysis of real-time quantitative PCR data. Genome Biol. 8, R19. doi:10.1186/gb-2007-8-2-r19
Hunter, M. P., Ismail, N., Zhang, X., Aguda, B. D., Lee, E. J., Yu, L., et al. (2008). Detection of microRNA expression in human peripheral blood microvesicles. PLoS One 3, e3694. doi:10.1371/journal.pone.0003694
Ignacio, C., Hicks, S. D., Burke, P., Lewis, L., Szombathyne-Meszaros, Z., and Middleton, F. A. (2015). Alterations in serum microRNA in humans with alcohol use disorders impact cell proliferation and cell death pathways and predict structural and functional changes in brain. BMC Neurosci. 16, 55. ARTN 55. doi:10.1186/s12868-015-0195-x
Kakimoto, Y., Tanaka, M., Kamiguchi, H., Ochiai, E., and Osawa, M. (2016). MicroRNA stability in FFPE tissue samples: Dependence on GC content. PLoS One 11, e0163125. doi:10.1371/journal.pone.0163125
Kang, K., Zhang, X., Liu, H., Wang, Z., Zhong, J., Huang, Z., et al. (2012). A novel real-time PCR assay of microRNAs using S-Poly(T), a specific oligo(dT) reverse transcription primer with excellent sensitivity and specificity. PloS One 7, e48536. doi:10.1371/journal.pone.0048536
Kent, W. J. (2002). BLAT-the BLAST-like alignment tool. Genome Res. 12, 656–664. doi:10.1101/gr.229202
Kirschner, M. B., Kao, S. C., Edelman, J. J., Armstrong, N. J., Vallely, M. P., Van Zandwijk, N., et al. (2011). Haemolysis during sample preparation alters microRNA content of plasma. PLoS One 6, e24145. doi:10.1371/journal.pone.0024145
Kozomara, A., and Griffiths-Jones, S. (2014). miRBase: annotating high confidence microRNAs using deep sequencing data. Nucleic Acids Res. 42, D68–D73. doi:10.1093/nar/gkt1181
Kramer, M. F. (2011). Stem-loop RT-qPCR for miRNAs. Curr. Protoc. Mol. Biol. Chapter 15, Unit 15.10. Chapter 15, Unit 15.10. doi:10.1002/0471142727.mb1510s95
Kroh, E. M., Parkin, R. K., Mitchell, P. S., and Tewari, M. (2010). Analysis of circulating microRNA biomarkers in plasma and serum using quantitative reverse transcription-PCR (qRT-PCR). Methods (San Diego, Calif.) 50, 298–301. doi:10.1016/j.ymeth.2010.01.032
Krohne, H.-W., and Egloff, B. (1999). Das angstbewältigungs-inventar (ABI). Frankfurt a.M: Swets Test Services.
Kubista, M., Sindelka, R., Tichopad, A., Bergkvist, A., Lindh, D., and Forootan, A. (2007). The prime technique: Real-time PCR data analysis. G.I.T. Laboratory J., 33–35. Available at: https://www.gene-quantification.de/kubista-bioinf-2007.pdf.
Lam, B., Simkin, M., Rghei, N., and Haj-Ahmad, Y. (2012). Revised guidelines for RNA quality assessment for diverse biological sample input (application note 47). Application note. Thorold, ontario, Canada: Centre for biotechnology. St. Catharines, Ontario, Canada: Brock University.
Li, F., Bai, M., Xu, J., Zhu, L., Liu, C., and Duan, R. (2020). Long-term exercise alters the profiles of circulating micro-RNAs in the plasma of young women. Front. Physiol. 11, 372. doi:10.3389/fphys.2020.00372
Li, L. D., Naveed, M., Du, Z. W., Ding, H., Gu, K., Wei, L. L., et al. (2021). Abnormal expression profile of plasma-derived exosomal microRNAs in patients with treatment-resistant depression. Hum. Genomics 15, 55. doi:10.1186/s40246-021-00354-z
Linsley, P. S., Schelter, J., Burchard, J., Kibukawa, M., Martin, M. M., Bartz, S. R., et al. (2007). Transcripts targeted by the microRNA-16 family cooperatively regulate cell cycle progression. Mol. Cell. Biol. 27, 2240–2252. doi:10.1128/MCB.02005-06
Liu, T., Gatto, N. M., Chen, Z., Qiu, H., Lee, G., Duerksen-Hughes, P., et al. (2020). Vegetarian diets, circulating miRNA expression and healthspan in subjects living in the Blue Zone. Precis. Clin. Med. 3, 245–259. doi:10.1093/pcmedi/pbaa037
Liu, X., Zhang, L., Cheng, K., Wang, X., Ren, G., and Xie, P. (2014). Identification of suitable plasma-based reference genes for miRNAome analysis of major depressive disorder. J. Affect. Disord. 163, 133–139. doi:10.1016/j.jad.2013.12.035
Maffioletti, E., Milanesi, E., Ansari, A., Zanetti, O., Galluzzi, S., Geroldi, C., et al. (2020). miR-146a plasma levels are not altered in alzheimer’s disease but correlate with age and illness severity. Front. Aging Neurosci. 11, 366. doi:10.3389/fnagi.2019.00366
Marabita, F., De Candia, P., Torri, A., Tegner, J., Abrignani, S., and Rossi, R. L. (2016). Normalization of circulating microRNA expression data obtained by quantitative real-time RT-PCR. Brief. Bioinform. 17, 204–212. doi:10.1093/bib/bbv056
Martin, M. (2011). Cutadapt removes adapter sequences from high-throughput sequencing reads. EMBnet. J. 17, 10–12. doi:10.14806/ej.17.1.200
Mathew, R., Mattei, V., Al Hashmi, M., and Tomei, S. (2020). Updates on the current Technologies for microRNA profiling. Microrna 9, 17–24. doi:10.2174/2211536608666190628112722
Mcalexander, M. A., Phillips, M. J., and Witwer, K. W. (2013). Comparison of methods for miRNA extraction from plasma and quantitative recovery of RNA from cerebrospinal fluid. Front. Genet. 4, 83. doi:10.3389/fgene.2013.00083
Mestdagh, P., Hartmann, N., Baeriswyl, L., Andreasen, D., Bernard, N., Chen, C., et al. (2014). Evaluation of quantitative miRNA expression platforms in the microRNA quality control (miRQC) study. Nat. Methods 11, 809–815. doi:10.1038/nmeth.3014
Meyer, S. U., Pfaffl, M. W., and Ulbrich, S. E. (2010). Normalization strategies for microRNA profiling experiments: A 'normal' way to a hidden layer of complexity? Biotechnol. Lett. 32, 1777–1788. doi:10.1007/s10529-010-0380-z
Mitchell, P. S., Parkin, R. K., Kroh, E. M., Fritz, B. R., Wyman, S. K., Pogosova-Agadjanyan, E. L., et al. (2008). Circulating microRNAs as stable blood-based markers for cancer detection. Proc. Natl. Acad. Sci. U. S. A. 105, 10513–10518. doi:10.1073/pnas.0804549105
Munetsuna, E., Yamada, H., Ando, Y., Yamazaki, M., Tsuboi, Y., Kondo, M., et al. (2018). Association of subcutaneous and visceral fat with circulating microRNAs in a middle-aged Japanese population. Ann. Clin. Biochem. 55, 437–445. doi:10.1177/0004563217735124
Nejad, C., Pillman, K. A., Siddle, K. J., Pepin, G., Anko, M. L., Mccoy, C. E., et al. (2018). miR-222 isoforms are differentially regulated by type-I interferon. RNA 24, 332–341. doi:10.1261/rna.064550.117
Niu, Y., Wu, Y., Huang, J., Li, Q., Kang, K., Qu, J., et al. (2016). Identification of reference genes for circulating microRNA analysis in colorectal cancer. Sci. Rep. 6, 35611. doi:10.1038/srep35611
Noren Hooten, N., Fitzpatrick, M., Wood, W. H., 3Rd, De, S., Ejiogu, N., Zhang, Y., et al. (2013). Age-related changes in microRNA levels in serum. Aging (Albany NY) 5, 725–740. doi:10.18632/aging.100603
Pagacz, K., Kucharski, P., Smyczynska, U., Grabia, S., Chowdhury, D., and Fendler, W. (2020). A systemic approach to screening high-throughput RT-qPCR data for a suitable set of reference circulating miRNAs. BMC Genomics 21, 111. doi:10.1186/s12864-020-6530-3
Parker, V. L., Cushen, B. F., Gavriil, E., Marshall, B., Waite, S., Pacey, A., et al. (2021). Comparison and optimisation of microRNA extraction from the plasma of healthy pregnant women. Mol. Med. Rep. 23, 1. doi:10.3892/mmr.2021.11897
Patel, N., Russell, G. K., Musunuru, K., Gutierrez, O. M., Halade, G., Kain, V., et al. (2019). Race, natriuretic peptides, and high-carbohydrate challenge: A clinical trial. Circ. Res. 125, 957–968. doi:10.1161/CIRCRESAHA.119.315026
Penso-Dolfin, L., Moxon, S., Haerty, W., and Di Palma, F. (2018). The evolutionary dynamics of microRNAs in domestic mammals. Sci. Rep. 8, 17050. doi:10.1038/s41598-018-34243-8
Pfaffl, M. W., Tichopad, A., Prgomet, C., and Neuvians, T. P. (2004). Determination of stable housekeeping genes, differentially regulated target genes and sample integrity: BestKeeper-Excel-based tool using pair-wise correlations. Biotechnol. Lett. 26, 509–515. doi:10.1023/b:bile.0000019559.84305.47
Pihur, V., Datta, S., and Datta, S. (2009). RankAggreg, an R package for weighted rank aggregation. BMC Bioinforma. 10, 62. doi:10.1186/1471-2105-10-62
Pimentel, F., Bonilla, P., Ravishankar, Y. G., Contag, A., Gopal, N., Lacour, S., et al. (2015). Technology in MicroRNA profiling: Circulating MicroRNAs as noninvasive cancer biomarkers in breast cancer. J. Lab. Autom. 20, 574–588. doi:10.1177/2211068214561788
Qi, R. Q., Weiland, M., Gao, X. H., Zhou, L., and Mi, Q. S. (2012). Identification of endogenous normalizers for serum MicroRNAs by microarray profiling: U6 small nuclear RNA is not a reliable normalizer. Hepatology 55, 1640–1642. doi:10.1002/hep.25558
QIAGEN (2019). Guidelines for profiling biofluid miRNAs. Hilden: Qiagen. [Online] Available: https://www.qiagen.com/-/media/project/qiagen/qiagen-home/content-worlds/pcr/l2-real-time-qpcr/guidelines_for_profiling_biofluid_mirnas.pdf ([Accessed].
QIAGEN (2022). miRCURY LNA miRNA Focus PCR panels. Hilden: Qiagen. [Online]. Available: www.qiagen.com/us/products/discovery-and-translational-research/pcr-qpcr-dpcr/qpcr-assays-and-instruments/mirna-qpcr-assay-and-panels/mircury-lna-mirna-focus-pcr-panels/ ([Accessed].
Raabe, C. A., Tang, T. H., Brosius, J., and Rozhdestvensky, T. S. (2014). Biases in small RNA deep sequencing data. Nucleic Acids Res. 42, 1414–1426. doi:10.1093/nar/gkt1021
Rao, P., Benito, E., and Fischer, A. (2013). MicroRNAs as biomarkers for CNS disease. Front. Mol. Neurosci. 6, 39. ARTN 39. doi:10.3389/fnmol.2013.00039
Roberts, T. C., Coenen-Stass, A. M., and Wood, M. J. (2014). Assessment of RT-qPCR normalization strategies for accurate quantification of extracellular microRNAs in murine serum. PLoS One 9, e89237. doi:10.1371/journal.pone.0089237
Santacruz, D., Enane, F. O., Fundel-Clemens, K., Giner, M., Wolf, G., Onstein, S., et al. (2022). Automation of high-throughput mRNA-seq library preparation: A robust, hands-free and time efficient methodology. SLAS Discov. 27, 140–147. doi:10.1016/j.slasd.2022.01.002
Schlosser, K., Mcintyre, L. A., White, R. J., and Stewart, D. J. (2015). Customized internal reference controls for improved assessment of circulating MicroRNAs in disease. PLoS One 10, e0127443. doi:10.1371/journal.pone.0127443
Schwarzenbach, H., Da Silva, A. M., Calin, G., and Pantel, K. (2015). Data normalization strategies for MicroRNA quantification. Clin. Chem. 61, 1333–1342. doi:10.1373/clinchem.2015.239459
Semenov, D. V., Baryakin, D. N., Brenner, E. V., Kurilshikov, A. M., Vasiliev, G. V., Bryzgalov, L. A., et al. (2012). Unbiased approach to profile the variety of small non-coding RNA of human blood plasma with massively parallel sequencing technology. Expert Opin. Biol. Ther. 12, S43–S51. doi:10.1517/14712598.2012.679653
Sendler, E., Johnson, G. D., and Krawetz, S. A. (2011). Local and global factors affecting RNA sequencing analysis. Anal. Biochem. 419, 317–322. doi:10.1016/j.ab.2011.08.013
Sheinerman, K. S., Tsivinsky, V. G., Crawford, F., Mullan, M. J., Abdullah, L., and Umansky, S. R. (2012). Plasma microRNA biomarkers for detection of mild cognitive impairment. Aging (Albany NY) 4, 590–605. doi:10.18632/aging.100486
Shende, V. R., Goldrick, M. M., Ramani, S., and Earnest, D. J. (2011). Expression and rhythmic modulation of circulating microRNAs targeting the clock gene Bmal1 in mice. PLoS One 6, e22586. doi:10.1371/journal.pone.0022586
Shi, H., Zhou, Y., Jia, E., Pan, M., Bai, Y., and Ge, Q. (2021). Bias in RNA-seq library preparation: Current challenges and solutions. Biomed. Res. Int. 2021, 6647597. doi:10.1155/2021/6647597
Silver, N., Best, S., Jiang, J., and Thein, S. L. (2006). Selection of housekeeping genes for gene expression studies in human reticulocytes using real-time PCR. BMC Mol. Biol. 7, 33. Artn 33. doi:10.1186/1471-2199-7-33
Song, J., Bai, Z., Han, W., Zhang, J., Meng, H., Bi, J., et al. (2012). Identification of suitable reference genes for qPCR analysis of serum microRNA in gastric cancer patients. Dig. Dis. Sci. 57, 897–904. doi:10.1007/s10620-011-1981-7
Takahashi, K., Yokota, S., Tatsumi, N., Fukami, T., Yokoi, T., and Nakajima, M. (2013). Cigarette smoking substantially alters plasma microRNA profiles in healthy subjects. Toxicol. Appl. Pharmacol. 272, 154–160. doi:10.1016/j.taap.2013.05.018
Tan, G. W., Khoo, A. S., and Tan, L. P. (2015). Evaluation of extraction kits and RT-qPCR systems adapted to high-throughput platform for circulating miRNAs. Sci. Rep. 5, 9430. doi:10.1038/srep09430
Tarallo, S., Pardini, B., Mancuso, G., Rosa, F., Di Gaetano, C., Rosina, F., et al. (2014). MicroRNA expression in relation to different dietary habits: A comparison in stool and plasma samples. Mutagenesis 29, 385–391. doi:10.1093/mutage/geu028
Tay, J. W., James, I., Hughes, Q. W., Tiao, J. Y., and Baker, R. I. (2017). Identification of reference miRNAs in plasma useful for the study of oestrogen-responsive miRNAs associated with acquired Protein S deficiency in pregnancy. BMC Res. Notes 10, 312. doi:10.1186/s13104-017-2636-3
Tomasello, L., Distefano, R., Nigita, G., and Croce, C. M. (2021). The MicroRNA family gets wider: The IsomiRs classification and role. Front. Cell Dev. Biol. 9, 668648. doi:10.3389/fcell.2021.668648
Turchinovich, A., Weiz, L., Langheinz, A., and Burwinkel, B. (2011). Characterization of extracellular circulating microRNA. Nucleic Acids Res. 39, 7223–7233. doi:10.1093/nar/gkr254
Vandesompele, J., De Preter, K., Pattyn, F., Poppe, B., Van Roy, N., De Paepe, A., et al. (2002). Accurate normalization of real-time quantitative RT-PCR data by geometric averaging of multiple internal control genes. Genome Biol. 3, RESEARCH0034. doi:10.1186/gb-2002-3-7-research0034
Varkonyi-Gasic, E., and Hellens, R. P. (2011). Quantitative stem-loop RT-PCR for detection of microRNAs. Methods Mol. Biol. 744, 145–157. doi:10.1007/978-1-61779-123-9_10
Vickers, K. C., Palmisano, B. T., Shoucri, B. M., Shamburek, R. D., and Remaley, A. T. (2011). MicroRNAs are transported in plasma and delivered to recipient cells by high-density lipoproteins. Nat. Cell Biol. 13, 423–433. doi:10.1038/ncb2210
Wang, H., Zhou, Y., Yin, Z. N., Chen, L., Jin, L., Cui, Q. H., et al. (2020). Transcriptome analysis of common and diverged circulating miRNAs between arterial and venous during aging. Aging (Albany NY) 12, 12987–13004. doi:10.18632/aging.103385
Wang, L., and Zhang, L. J. (2020). Circulating exosomal miRNA as diagnostic biomarkers of neurodegenerative diseases. Front. Mol. Neurosci. 13, 53. ARTN 53. doi:10.3389/fnmol.2020.00053
Wang, Z. F., Lu, G., Sze, J., Liu, Y., Lin, S., Yao, H., et al. (2018). Plasma miR-124 is a promising candidate biomarker for human intracerebral hemorrhage stroke. Mol. Neurobiol. 55, 5879–5888. doi:10.1007/s12035-017-0808-8
Wardle, S. L., Bailey, M. E. S., Kilikevicius, A., Malkova, D., Wilson, R. H., Venckunas, T., et al. (2015). Plasma MicroRNA levels differ between endurance and strength athletes. Plos One 10, e0122107. doi:10.1371/journal.pone.0122107
Wotschofsky, Z., Meyer, H. A., Jung, M., Fendler, A., Wagner, I., Stephan, C., et al. (2011). Reference genes for the relative quantification of microRNAs in renal cell carcinomas and their metastases. Anal. Biochem. 417, 233–241. doi:10.1016/j.ab.2011.06.009
Wright, C., Rajpurohit, A., Burke, E. E., Williams, C., Collado-Torres, L., Kimos, M., et al. (2019). Comprehensive assessment of multiple biases in small RNA sequencing reveals significant differences in the performance of widely used methods. BMC Genomics 20, 513. ARTN 513. doi:10.1186/s12864-019-5870-3
Xie, F., Xiao, P., Chen, D., Xu, L., and Zhang, B. (2012). miRDeepFinder: a miRNA analysis tool for deep sequencing of plant small RNAs. Plant Mol. Biol. 80, 75–84. doi:10.1007/s11103-012-9885-2
Yagi, Y., Ohkubo, T., Kawaji, H., Machida, A., Miyata, H., Goda, S., et al. (2017). Next-generation sequencing-based small RNA profiling of cerebrospinal fluid exosomes. Neurosci. Lett. 636, 48–57. doi:10.1016/j.neulet.2016.10.042
Yan, Y., Shi, Y., Wang, C., Guo, P., Wang, J., Zhang, C. Y., et al. (2015). Influence of a high-altitude hypoxic environment on human plasma microRNA profiles. Sci. Rep. 5, 15156. doi:10.1038/srep15156
Ye, J., Coulouris, G., Zaretskaya, I., Cutcutache, I., Rozen, S., and Madden, T. L. (2012). Primer-BLAST: A tool to design target-specific primers for polymerase chain reaction. BMC Bioinforma. 13, 134. doi:10.1186/1471-2105-13-134
Zalewski, K., Misiek, M., Kowalik, A., Bakula-Zalewska, E., Kopczynski, J., Zielinska, A., et al. (2017). Normalizers for microRNA quantification in plasma of patients with vulvar intraepithelial neoplasia lesions and vulvar carcinoma. Tumour Biol. 39, 1010428317717140–1010428317717148. doi:10.1177/1010428317717140
Zampetaki, A., Willeit, P., Drozdov, I., Kiechl, S., and Mayr, M. (2012). Profiling of circulating microRNAs: From single biomarkers to re-wired networks. Cardiovasc. Res. 93, 555–562. doi:10.1093/cvr/cvr266
Zernecke, A., Bidzhekov, K., Noels, H., Shagdarsuren, E., Gan, L., Denecke, B., et al. (2009). Delivery of microRNA-126 by apoptotic bodies induces CXCL12-dependent vascular protection. Sci. Signal. 2, ra81. doi:10.1126/scisignal.2000610
Zhang, J., Deng, C., Li, J., and Zhao, Y. (2020). Transcriptome-based selection and validation of optimal house-keeping genes for skin research in goats (Capra hircus). BMC Genomics 21, 493. doi:10.1186/s12864-020-06912-4
Zhang, Y. J., Tang, W. X., Peng, L., Tang, J. Q., and Yuan, Z. K. (2016). Identification and validation of microRNAs as endogenous controls for quantitative polymerase chain reaction in plasma for stable coronary artery disease. Cardiol. J. 23, 694–703. doi:10.5603/CJ.2016.0109
Zhang, Z., Qin, Y. W., Brewer, G., and Jing, Q. (2012). MicroRNA degradation and turnover: Regulating the regulators. Wiley Interdiscip. Rev. RNA 3, 593–600. doi:10.1002/wrna.1114
Keywords: miRNA expression microarray, small-RNA sequencing, stem-loop reverse-transcription quantitative PCR, human plasma miRNAs, miRNA reference genes, cognitive stress-coping, qPCR normalization
Citation: Baumann V, Athanasiou A-T, Faridani OR, Schwerdtfeger AR, Wallner B and Steinborn R (2023) Identification of extremely GC-rich micro RNAs for RT-qPCR data normalization in human plasma. Front. Genet. 13:1058668. doi: 10.3389/fgene.2022.1058668
Received: 30 September 2022; Accepted: 02 December 2022;
Published: 04 January 2023.
Edited by:
Amaresh Chandra Panda, Institute of Life Sciences (ILS), IndiaReviewed by:
Gopal Pandi, Madurai Kamaraj University, IndiaKotb Abdelmohsen, National Institute on Aging (NIH), United States
Copyright © 2023 Baumann, Athanasiou, Faridani, Schwerdtfeger, Wallner and Steinborn. This is an open-access article distributed under the terms of the Creative Commons Attribution License (CC BY). The use, distribution or reproduction in other forums is permitted, provided the original author(s) and the copyright owner(s) are credited and that the original publication in this journal is cited, in accordance with accepted academic practice. No use, distribution or reproduction is permitted which does not comply with these terms.
*Correspondence: Ralf Steinborn, cmFsZi5zdGVpbmJvcm5AZ214LmF0
†ORCID: Ralf Steinborn, orcid.org/orcid.org/0000-0001-9287-5039