- 1Department of Medical Oncology, The First Affiliated Hospital of Nanchang University, Nanchang, China
- 2Medical Innovation Center, The First Affiliated Hospital of Nanchang University, Nanchang, China
- 3Department of General Surgery, The First Affiliated Hospital of Nanchang University, Nanchang, China
- 4Human Genetic Resources Center, The First Affiliated Hospital of Nanchang University, Nanchang, China
Increasing studies have reported that circular RNAs (circRNAs) play critical roles in tumorigenesis and cancer progression. However, the underlying regulatory mechanisms of circRNA-related competing endogenous RNA (ceRNA) in liver hepatocellular carcinoma (LIHC) are still unclear. In the present study, we discovered dysregulated circRNAs through Gene Expression Omnibus (GEO) analysis and validated the expression of the top seven circRNAs with upregulated expression by qRT–PCR and Sanger sequencing. Then, the Cancer-Specific CircRNA Database (CSCD) was used to predict the downstream miRNAs of seven circRNAs, and expression and survival analyses through The Cancer Genome Atlas (TCGA) were performed to identify the key miRNA in LIHC. Thereafter, the hsa_circ_0017264-hsa-miR-195–5p subnetwork was successfully constructed. Subsequently, we predicted downstream target genes of hsa-miR-195–5p with TargetScan, miRDB, and mirtarbase and overlapped them with differentially expressed mRNAs to obtain 21 target genes. Gene ontology (GO) and Kyoto Encyclopedia of Genes and Genomes (KEGG) pathway enrichment analyses were performed to predict the biological and functional roles of these target genes. Finally, with Pearson correlation and prognostic value analysis, a survival-related hsa_circ_0017264-hsa-miR-195-5p-CHEK1/CDC25A/FOXK1 axis was established. Gene set enrichment analysis (GSEA) was performed to determine the function of CHEK1/CDC25A/FOXK1 in the ceRNA network. Moreover, immune infiltration analysis revealed that the ceRNA network was markedly associated with the levels of multiple immune cell infiltrates, immune cell biomarkers and immune checkpoints. Overall, the hsa_circ_0017264-hsa-miR-195-5p-CHEK1/CDC25A/FOXK1 network might provide novel insights into the potential mechanisms underlying LIHC onset and progression.
Introduction
Liver cancer is one of the most common malignant tumors; 75%–85% of liver cancer cases are classified as hepatocellular carcinoma (LIHC), and 10%–15% are classified as intrahepatic cholangiocarcinoma (CHOL) (Sung et al., 2021). The most common risk factors for LIHC are chronic infection with hepatitis B virus (HBV) or hepatitis C virus (HCV), aflatoxin-contaminated foods, heavy alcohol intake, excess body weight, type 2 diabetes, and smoking (Suh et al., 2018). Increasing evidence suggests that the occurrence and development of LIHC are associated with abnormal genetic changes and cancer-related signaling pathways, such as TERT, the WNT/β-catenin pathway, and the mTOR pathway (Li et al., 2020). Currently, due to the lack of available screening methods and noticeable early symptoms, many LIHC patients are diagnosed at an advanced stage, and the treatment outcomes are unsatisfactory (Ogunwobi et al., 2019; Marrero, 2020). Therefore, it is of great significance to explore the molecular mechanisms of LIHC to identify novel diagnostic and prognostic targets.
Circular RNAs (circRNAs) are a type of endogenous noncoding RNA (ncRNA) discovered by Sanger in viroids in 1976 (Sanger et al., 1976). With the development of bioinformatics and high-throughput sequencing technology, circRNAs have been widely observed in viruses, fungi, plants and animals (Wang et al., 2018). Salmena et al. (2011) first proposed the competitive endogenous RNA (ceRNA) hypothesis that circRNAs negatively regulate miRNA expression by interacting with miRNA response elements (MREs) and then regulate intracellular signaling pathways and downstream gene expression. Recently, several studies (Yao et al., 2019; He et al., 2021) have revealed that circRNAs play a pivotal role in the occurrence and development of LIHC through ceRNA networks. In addition, circRNAs can be used as diagnostic or prognostic markers for LIHC patients (Wang et al., 2019; Chen et al., 2020). Hence, we built a circRNA–miRNA–mRNA network to further clarify the detailed mechanism of circRNAs in LIHC.
In this study, we established a survival-related circRNA-miRNA-mRNA ceRNA regulatory network for LIHC. To achieve this goal, we identified differentially expressed circRNAs, miRNAs, and mRNAs in LIHC and predicted the binding relationship between circRNAs, miRNAs and mRNAs through multiple bioinformatic tools. Finally, combined with clinical information from The Cancer Genome Atlas (TCGA) database, we successfully constructed a new ceRNA network that is significantly related to the prognosis of LIHC patients. In addition, the Tumor Immune Estimation Resource (TIMER) database was used to estimate the correlation between the ceRNA network and the level of immune infiltration. This study will provide us with a new perspective on the pathogenesis of LIHC and improve the diagnosis and treatment of LIHC.
Materials and Methods
Dataset Retrieval
The NCBI Gene Expression Omnibus (GEO) database (http://www.ncbi.nlm.nih.gov/geo/) was used to find potential functional circRNAs in LIHC. Dataset selection was based on the following screening criteria: 1) datasets focused on human LIHC tissue samples; 2) datasets excluded LIHC cell lines or animal LIHC tissue samples; and 3) the number of samples in the selected dataset was more than 10. Hence, we chose the GSE164803 dataset for further research. Next, we obtained miRNA expression profiles, mRNA expression profiles, and clinical information of LIHC patients from the TCGA database (https://portal.gdc.Cancer.gov/). Detailed information on these datasets is shown in Table 1.
Differential Expression Analysis of circRNAs, miRNAs and mRNAs
After downloading data from the GEO and TCGA databases, we used Perl (version 5.32.1.1) to analyze and process the dataset. According to the annotation platform file of the expression profile, the probe names were converted to the corresponding gene names, and empty probes were removed. Then, the “limma” package (Ritchie et al., 2015) (version 3.50.0) of R (version 4.1.0)software was used to identify circRNAs, miRNAs and mRNAs that were differentially expressed between tumor tissues and adjacent tissues. Adjusted p < 0.05 and |logFoldChange| > 1 were the identification thresholds.
circBase and Cancer-Specific CircRNA Database Analysis
Circbase (Glažar et al., 2014) (http://www.circbase.org/) is a circRNA database that contains detailed information on circRNAs in humans, mice and many other species. We found the gene sequence of circRNAs through circBase and compared it with the results of Sanger sequencing. CSCD (Xia et al., 2018) (http://gb.whu.edu.cn/CSCD/) is an online tool for studying cancer-specific circRNAs and is used to visualize the circular structure of potential circRNAs and predict the binding relationship between circRNAs and miRNAs.
Clinical Specimens
Tumor and adjacent normal tissue specimens were collected from 10 HCC patients undergoing surgery at the First Affiliated Hospital of Nanchang University (Nanchang, China). The patients did not receive any radiotherapy or chemotherapy before surgical operation. Normal and tumor tissues from 10 HCC patients were immediately frozen in liquid nitrogen and then stored in −80°C to extract RNA. This study was approved by the Ethics Committee of the First Affiliated Hospital of Nanchang University.
Ribonucleic Acid Extraction and Quantitative Reverse Transcription–Polymerase Chain Reaction
Total RNA was extracted from the clinical samples and separated using TRIzol (Invitrogen) according to the manufacturer’s protocol. To quantify the expression of circRNAs, miRNAs and mRNAs, cDNA was synthesized using the PrimeScript™ RT reagent Kit (Takara, Dalian, China), and qRT–PCR was performed using TB Green™ Premix Ex Taq II (Takara, Dalian, China) in a Roche LightCycler 96 instrument. The qRT–PCR cycling conditions were as follows: 95°C for 30 s, 40 cycles at 95°C for 5 s, and 60°C for 20 s. The melt curve stage was set as follows: 95°C for 15 s, 60°C for 60 s, and 95°C for 15 s. The primers were designed and synthesized by Sangon Biotech (Shanghai, China). All primer sequences are listed in Table 2. GAPDH served as an endogenous control for circRNAs and mRNAs, and U6 was used as an internal control for miRNA. The relative level of gene expression was calculated using the 2−ΔCt method (Livak and Schmittgen, 2001).
Sanger Sequencing
CircRNA products amplified with divergent primers, including splicing sites, were validated by Tsingke Biological Technology (Beijing, China).
Target Gene Prediction and miRNA-mRNA Correlation Analysis
The miRDB (Chen and Wang, 2020) (http://www.mirdb.org/), TargetScan (Agarwal et al., 2015) (http://www.targetscan.org/), and mirtarbase (Chou et al., 2018) (https://mirtarbase.cuhk.edu.cn/∼miRTarBase/miRTarBase_2022/php/index.php) three databases were used to predict target genes of miRNAs. Venny2.1 (https://bioinfogp.cnb.csic.es/tools/venny) was used to compare two groups of molecules to obtain overlapping genes. The starbase (Li et al., 2014) (http://starbase.sysu.edu.cn/) database was used for Pearson correlation analysis between miRNAs and mRNAs.
Survival and Prognostic Analysis
To evaluate the value of the identified ceRNA network and determine the miRNAs and mRNAs related to prognosis, we downloaded the miRNA and mRNA expression profiles and clinical information of LIHC patients from the TCGA database. The “survival” package in R was used to analyze the prognostic values of miRNAs and mRNAs in LIHC.
Gene Ontology and Pathway Enrichment Analysis
The “clusterProfiler” (version 4.2.1) and “GOplot” packages (Yu et al., 2012; Walter et al., 2015) of R were used to perform GO annotation and Kyoto Encyclopedia of Genes and Genomes (KEGG) pathway analysis to evaluate the 21 selected mRNAs with prognostic value. p < 0.05 served as the cutoff criterion.
Immune Infiltration Level Analysis of the ceRNA Network
TIMER (Li et al., 2017) is a comprehensive database for investigating the molecular characterization of tumor–immune interactions (https://cistrome.shinyapps.io/timer/). We analyzed the correlation of CHEK1/CDC25A/FOXK1 expression with the abundance of immune infiltrates (B cells, CD4+ T cells, CD8+ T cells, neutrophils, macrophages, and dendritic cells) via gene modules. Gene expression levels against tumor purity are displayed in the left-most panel. In addition, correlations between CHEK1/CDC25A/FOXK1 expression and gene markers of tumor-infiltrating immune cells were explored via correlation modules.
Immune Checkpoint Expression Analysis of the ceRNA Network
Gene Expression Profiling Interactive Analysis (GEPIA) (http://gepia.cancer-pku.cn/) is a public database (Tang et al., 2017)) that enables pairwise gene correlation analysis for any given set of TCGA and/or GTEx expression data. The “correlation analysis” module was used to assess the correlation between the expression of genes in the ceRNA regulatory network and the expression of immune checkpoints.
Statistical Analysis
The qRT–PCR results were statistically analyzed using GraphPad Prism 7. Paired Student’s t-test was used to evaluate the differences in circRNAs, miRNA and mRNAs expression between the cancer group and the control group. p < 0.05 was considered to indicate a statistically significant difference.
Results
Prediction of the Differentially Expressed circRNAs in LIHC
To identify some potential circRNAs related to the progression of LIHC, we used the GSE164803 dataset in GEO to analyze the differential expression of liver cancer tissues and adjacent tissues based on the criterion |log2FC| > 1, adjusted p < 0.05. The results are displayed in Figure 1A. For more accurate analysis, we changed the screening criteria for differential circRNAs to |log2FC| > 3, adjusted p < 0.05. As shown in Figure 1B, 10 circRNAs, including nine upregulated circRNAs and one downregulated circRNA, were identified. The basic information of circRNAs is listed in Table 3. The data included circbase ID, parental gene, genomic length, spliced length, and genome location. In our current work, our goal is to explore prognostic biomarkers specifically increased in LIHC patients, which will be useful in clinical detection and contribute to future drug therapies. We selected nine upregulated circRNAs for further study. Then, we found eight circRNAs structural loop graphs through the CSCD. The graph for hsa_circ_0055605 was not available in the database, but the others are shown in Figure 2. It can be seen that these circRNAs all have miRNA response elements (MREs), which suggests that they may play a role in the progress of LIHC through the ceRNA mechanism.
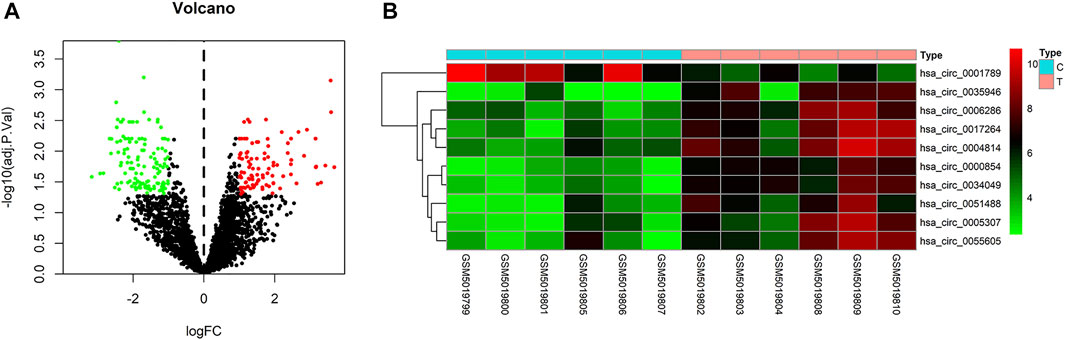
FIGURE 1. Differentially expressed circRNAs in GSE164803. (A) Volcano plot of differentially expressed circRNAs in LIHC from the GSE164803 dataset. The red dots and green dots represent significantly upregulated circRNAs and downregulated circRNAs, respectively (adjusted p < 0.05 and |logFoldChange| > 1). The black dots are circRNAs without significant changes. (B) Heatmap of 10 potential circRNAs (|logFoldChange| > 3). The intensity increased from green (relatively lower expression) to red (relatively higher expression). C, control tissues; T, tumor tissues.
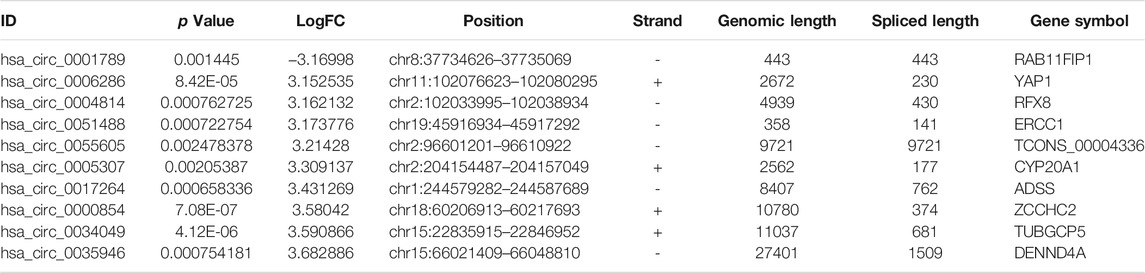
TABLE 3. Basic information for the top 10 differentially expressed circRNAs with a |logFoldChange| > 3.
Verification of the Expression of Candidate circRNAs in LIHC
To detect and verify the expression of the eight candidate circRNAs, we used divergent primers in clinical specimens. As shown in Figure 3, we found that the expression level of hsa_circ_0004814 was quite low and difficult to detect, and the levels of other circRNAs were consistent with our predictions in the bioinformatics analysis. In addition, we performed Sanger sequencing of the qRT–PCR products to further verify the specificity of these primers, and the results are displayed in Figure 4. Based on this, seven circRNAs (excluding hsa_circ_0004814) were considered the final candidate circRNAs in LIHC.
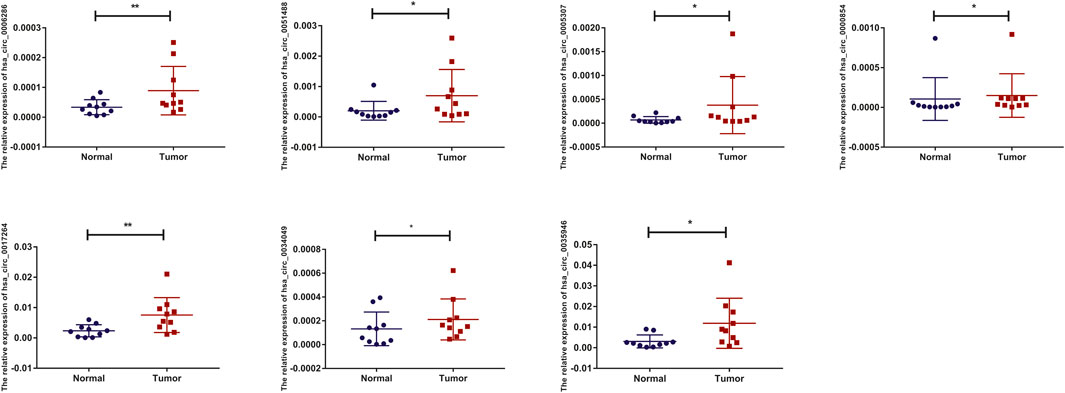
FIGURE 3. qRT–PCR validation of the expression of candidate circRNAs in LIHC tissues and adjacent normal tissues (n = 10).
Prediction and Analysis of Binding miRNAs of circRNAs in LIHC
To predict the binding miRNAs of these circRNAs, we predicted miRNAs that may bind to seven circRNAs through the CSCD database. We found that 366 miRNAs may be targets of the seven circRNAs. According to the ceRNA hypothesis, the expression of circRNAs should be negatively correlated with the expression of miRNAs. Then, we further analyzed the differential expression of miRNAs (DEmiRNAs) in LIHC in the TCGA database. As shown in Figure 5A and Supplementary Figure S1, 260 upregulated and 40 downregulated DEmiRNAs were found. As presented in Figure 5B, we utilized Venn diagrams to illustrate the intersection of the targeted miRNAs and the downregulated DEmiRNAs. Nine miRNAs (hsa-miR-195–5p, hsa-miR-490–3p, hsa-miR-1248, hsa-miR-335–5p, hsa-miR-5589–3p, hsa-miR-6502–5p, hsa-miR-326, hsa-miR-5589–5p, hsa-miR-424–5p) were identified. Moreover, we obtained the clinical information of LIHC patients in the TCGA database and performed survival analysis according to the expression of the nine miRNAs. As shown in Figures 5C–K, we recognized that two miRNAs (hsa-miR-195–5p and hsa-miR-326) were related to prognosis, and only low expression of hsa-miR-195–5p was related to a good prognosis. Besides, as presented in Figure 5L, we further validated the differential expression of hsa-miR-195–5p between the cancer and normal samples.
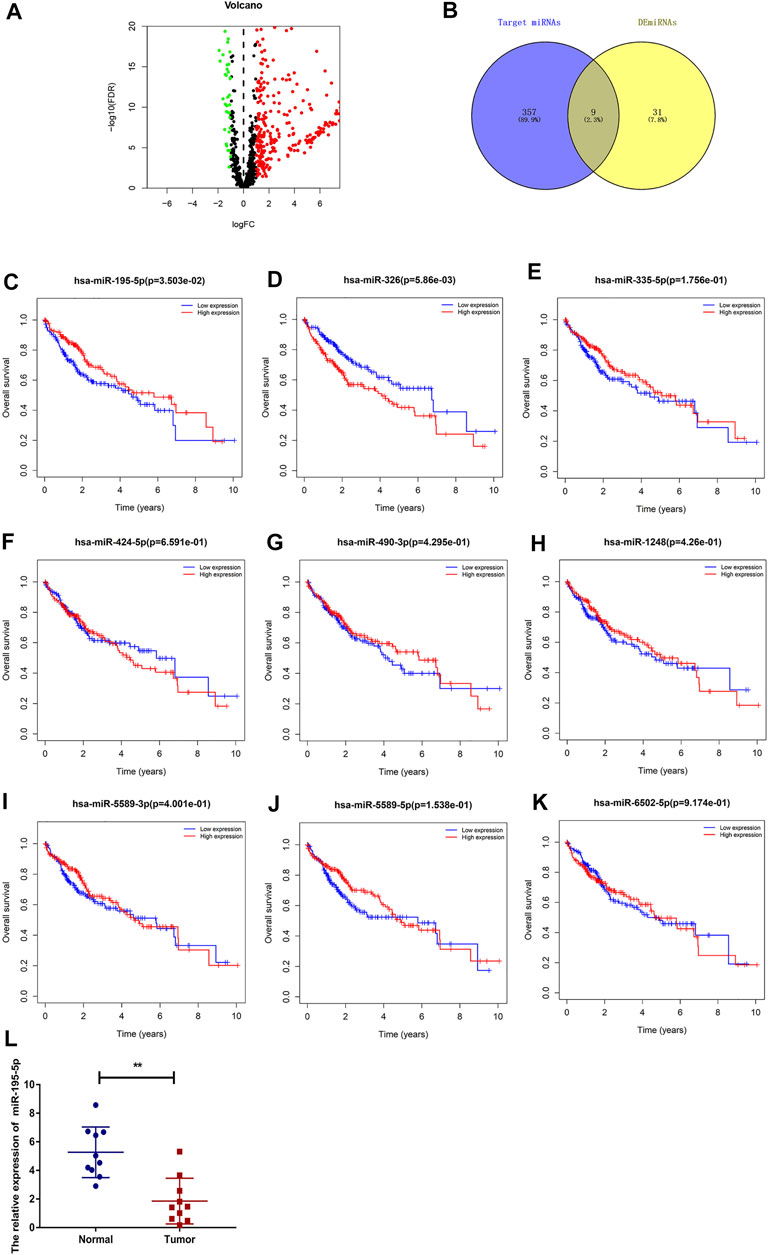
FIGURE 5. Prediction and analysis of the binding miRNAs of circRNAs in LIHC. (A) The volcano plot of DEmiRNAs in LIHC from the TCGA (adjusted p < 0.05 and |logFoldChange| > 1). (B) Venn diagram analysis of target miRNAs of the seven circRNAs and downregulated DEmiRNAs. (C–K) The prognostic value of nine miRNAs in LIHC. (L) The expression of hsa-miR-195–5p in LIHC tissues and adjacent normal tissues (n = 10).
Prediction and Analysis of Target Genes of hsa-miR-195-5p in LIHC
We predicted the target genes of hsa-miR-195–5p via three databases (miRDB, mirtarbase, and TargetScan). As shown in Figures 6A,B and Supplementary Figure S2, a total of 21 mRNAs (CCNE1, CDCA4, ITGA2, E2F7, CBX2, KIF23, CDC25A, CHEK1, RASEF, HOXA10, PHF19, AXIN2, PLAG1, OSBPL3, CEP55, CLSPN, TPM2, FASN, HOXA3, LAMC1, FOXK1) were identified from the intersection of target genes and upregulated differentially expressed mRNAs (DEmRNAs) of LIHC in the TCGA database. Then, we applied GO and KEGG pathway enrichment analyses to clarify the potential biological processes and signaling pathways of the 21 target genes. As shown in Figures 6C–F, GO analysis revealed that the 21 target genes were mainly enriched in hepatocyte differentiation, DNA replication, chromatin-mediated maintenance of transcription, positive regulation of cell cycle, DNA damage checkpoint, and DNA integrity checkpoint (adjusted p < 0.05). According to the results of the KEGG pathway analysis, the target genes were mainly enriched in the cell cycle, cellular senescence, microRNAs in cancer, the p53 signaling pathway, and the PI3K−Akt signaling pathway (adjusted p < 0.05).
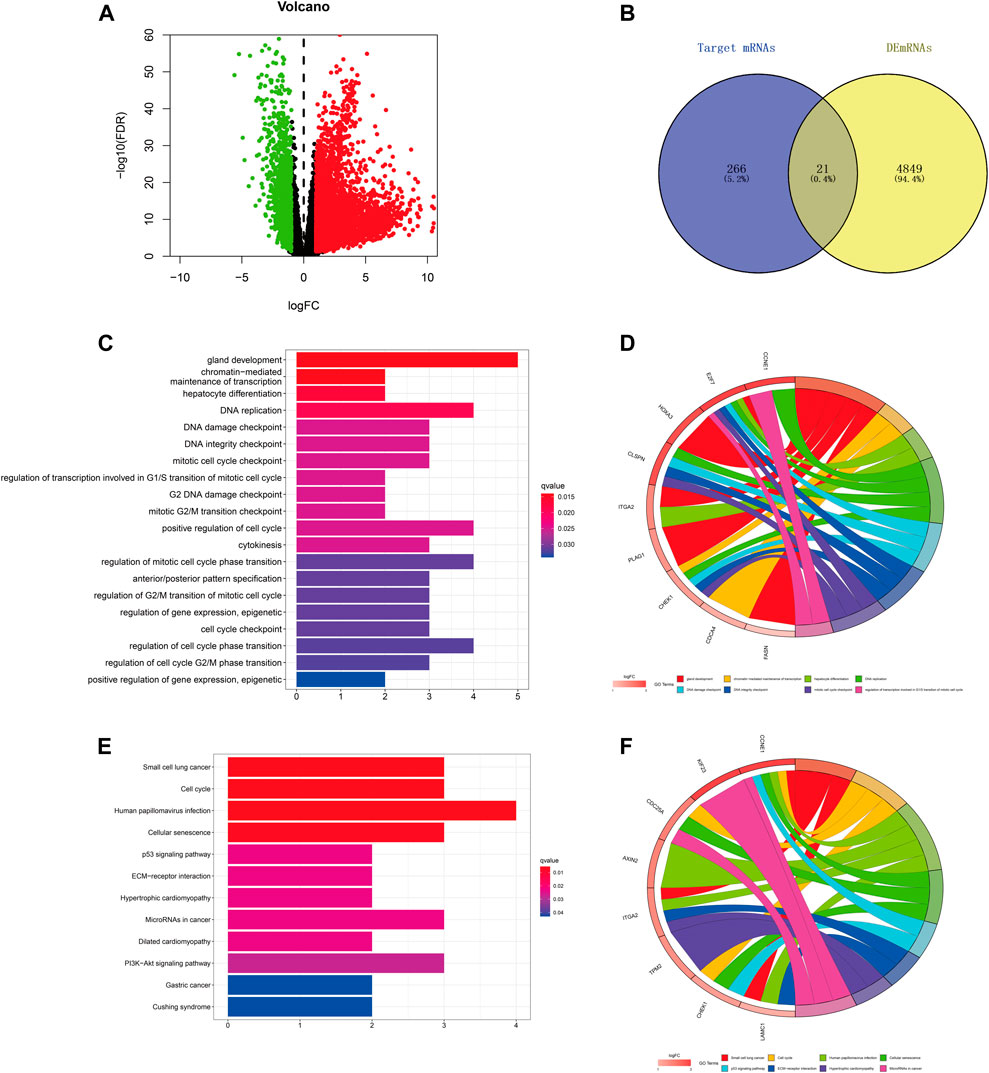
FIGURE 6. Prediction and analysis of target genes of hsa-miR-195–5p in LIHC. (A) The volcano plot of differentially expressed mRNAs in LIHC from TCGA (adjusted p < 0.05 and |logFoldChange| > 1). (B) Venn diagram analysis of the target mRNAs of hsa-miR-195–5p and upregulated DEmRNAs. (C–F) GO and KEGG pathway analyses of the 21 mRNAs.
Construction of a Survival-Related hsa_circ_0017264-hsa-miR-195-5p-CHEK1/CDC25A/FOXK1 ceRNA Network in LIHC
Based on our above analysis, we found that hsa-miR-195–5p has 21 target genes, which are the intersection of downstream target molecules predicted by three databases and showed significantly upregulated expression in the LIHC-TCGA dataset. As shown in Figures 7A–F, we observed that six target genes had a weak negative correlation with hsa-miR-195–5p but a significant linear relationship. Next, we evaluated the prognostic value of these six mRNAs in LIHC. As presented in Figures 8A–C, among these mRNAs, CHEK1, CDC25A and FOXK1 were negatively correlated with the survival time of patients with LIHC. Moreover, the expression levels of CHEK1, CDC25A and FOXK1 in 10 LIHC patients are presented in Figures 8D–F. Finally, we established a hsa_circ_0017264-hsa-miR-195-5p-CHEK1/CDC25A/FOXK1 ceRNA network, which may provide promising therapeutic targets in treating LIHC in the near future. Then, GSEA was used to study the potential role of CHEK1, CDC25A and FOXK1 in LIHC. As shown in Figures 8G–I, the three genes were positively correlated with the cell cycle, p53 signaling pathway, and WNT signaling pathway and were negatively correlated with primary bile acid biosynthesis.
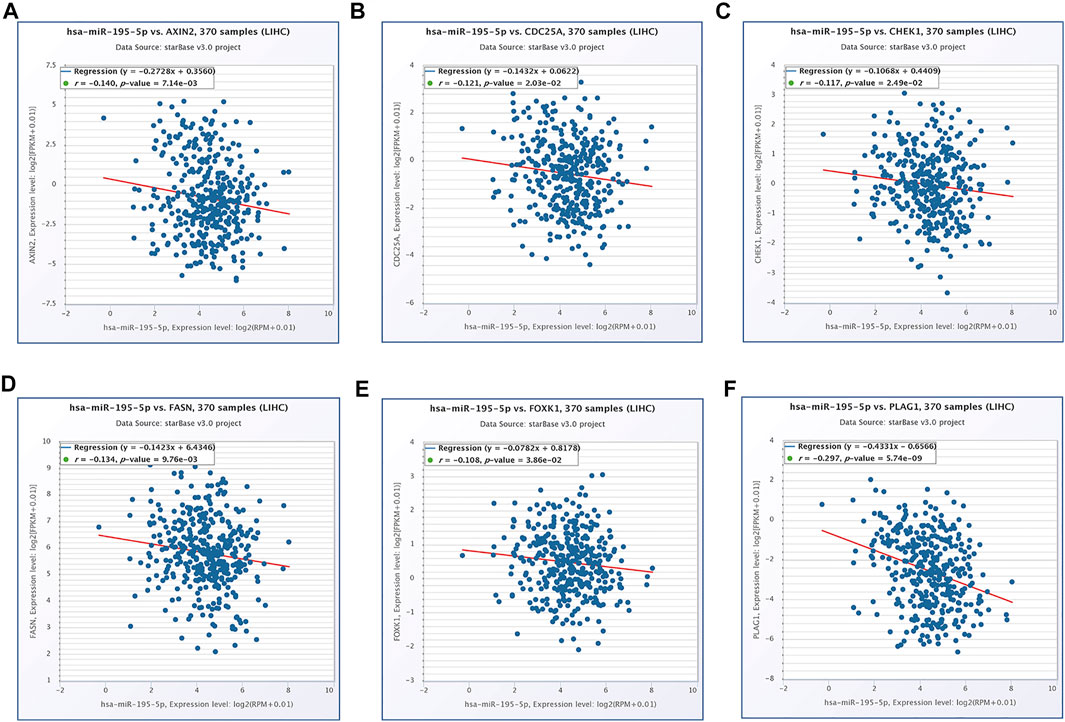
FIGURE 7. The expression correlation of hsa-miR-195–5p with AXIN2 (A), CDC25A (B), CHEK1 (C), FASN (D), FOXK1 (E), PLAG1 (F).
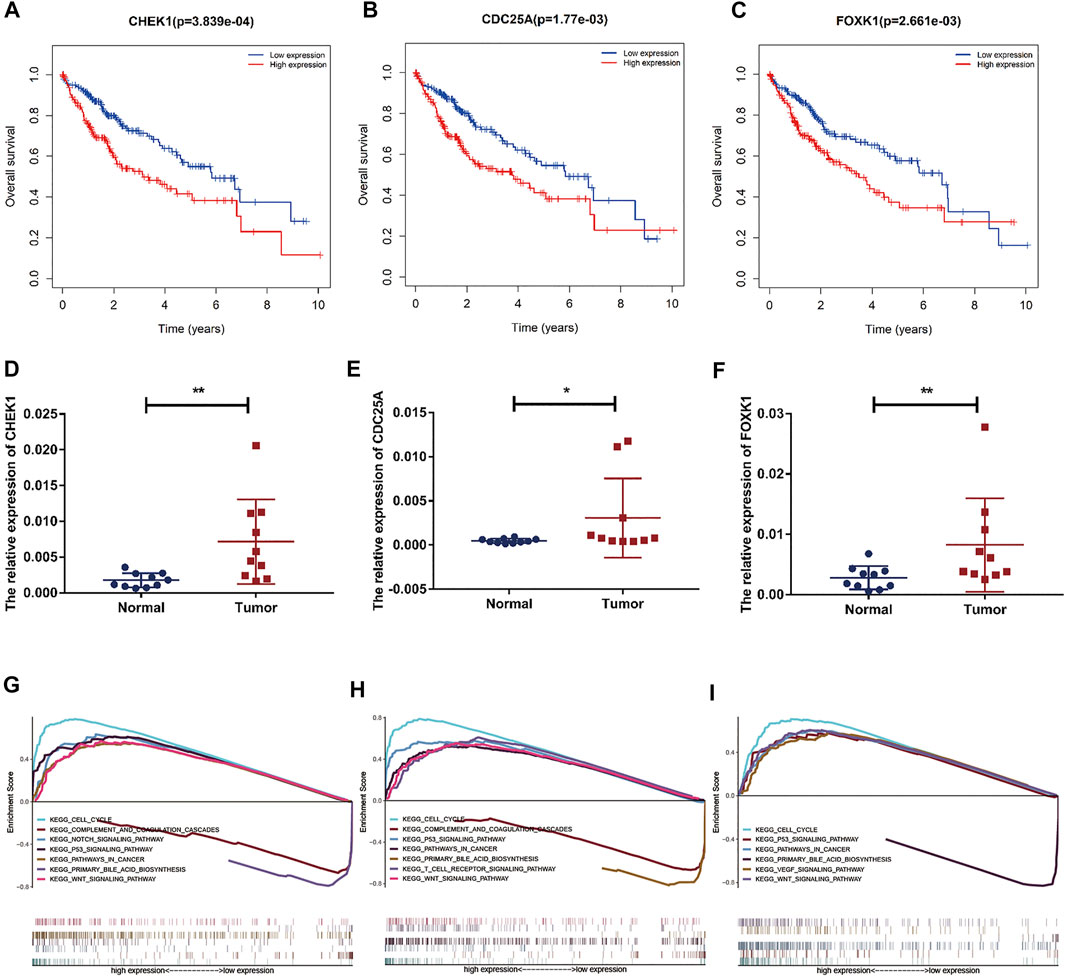
FIGURE 8. Prognostic assessment and GSEA of target genes. (A–C) The prognostic value of CHEK1, CDC25A, FOXK1 in LIHC. (D–F) mRNA expression of CHEK1, CDC25A and FOXK1 in LIHC tissues and adjacent normal tissues (n = 10) (G–I) GSEA of CHEK1, CDC25A and FOXK1 expressed in the ceRNA network.
Correlations of CHEK1/CDC25A/FOXK1 With Immune Infiltration Level in LIHC
The correlation between CHEK1, CDC25A, FOXK1 and tumor-infiltrating immune cells in the LIHC microenvironment was evaluated by using TIMER. As shown in Figures 9A–C, we found that the expression of genes in the ceRNA regulatory network was significantly related to the infiltration of immune cells (B cells, CD4+/CD8+ T cells, macrophages, neutrophils and dendritic cells) into the LIHC microenvironment. Furthermore, we explored the relationships between CHEK1/CDC25A/FOXK1 and immune markers by Spearman correlation analysis, and the results are displayed in Table 4.
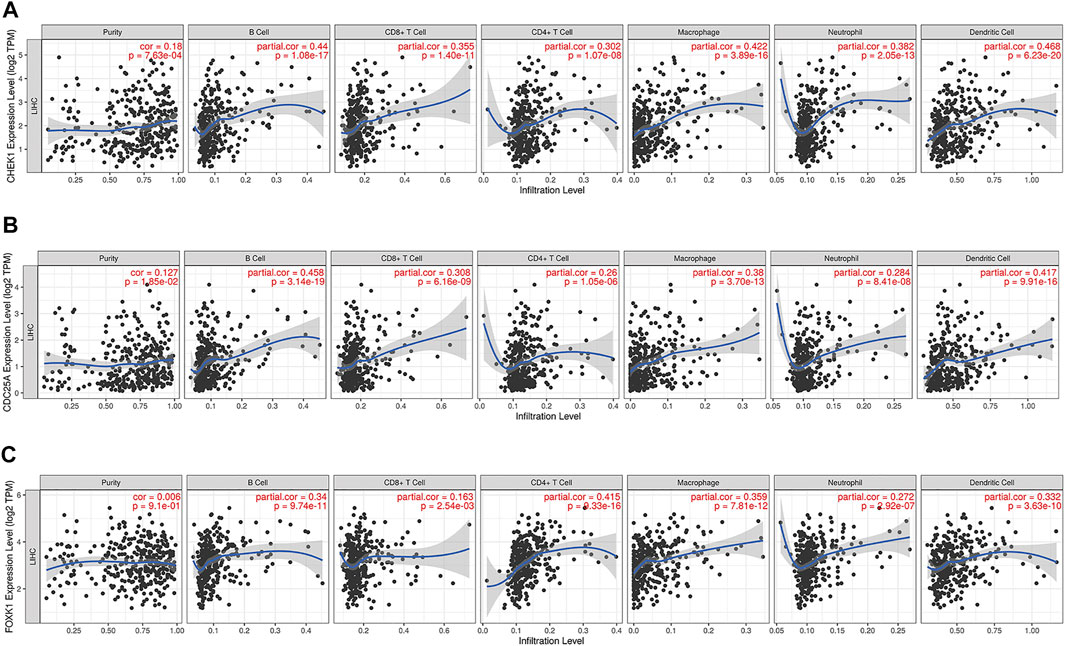
FIGURE 9. Associations of the expression of CHEK1 (A), CDC25A (B), FOXK1 (C) in the ceRNA network with immune infiltration levels in LIHC.
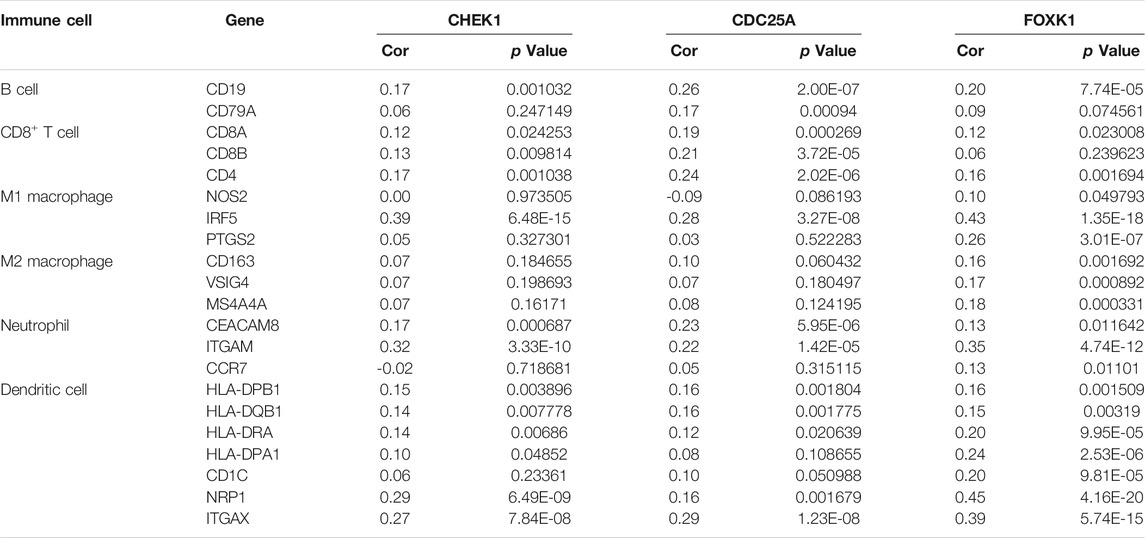
TABLE 4. Analysis of the correlations between the expression of mRNAs in the ceRNA network and that of immune cell biomarkers.
The Relationships Between CHEK1/CDC25A/FOXK1 and Immune Checkpoints
Programmed cell death protein 1 (PD-1), programmed cell death one ligand 1 (PD-L1) and cytotoxic T-lymphocyte-associated protein 4 (CTLA-4) are essential immune checkpoints for tumor immune escape. As shown in Figures 10A–I, the “R” indicates a weak positive correlation among CHEK1, CDC25A, FOXK1 and PD-1, PD-L1, and CTLA-4 in LIHC, with a significant linear relationship. These findings suggest that the hsa_circ_0017264-hsa-miR-195-5p-CHEK1/CDC25A/FOXK1 axis may be involved in facilitating immune escape in the LIHC microenvironment.
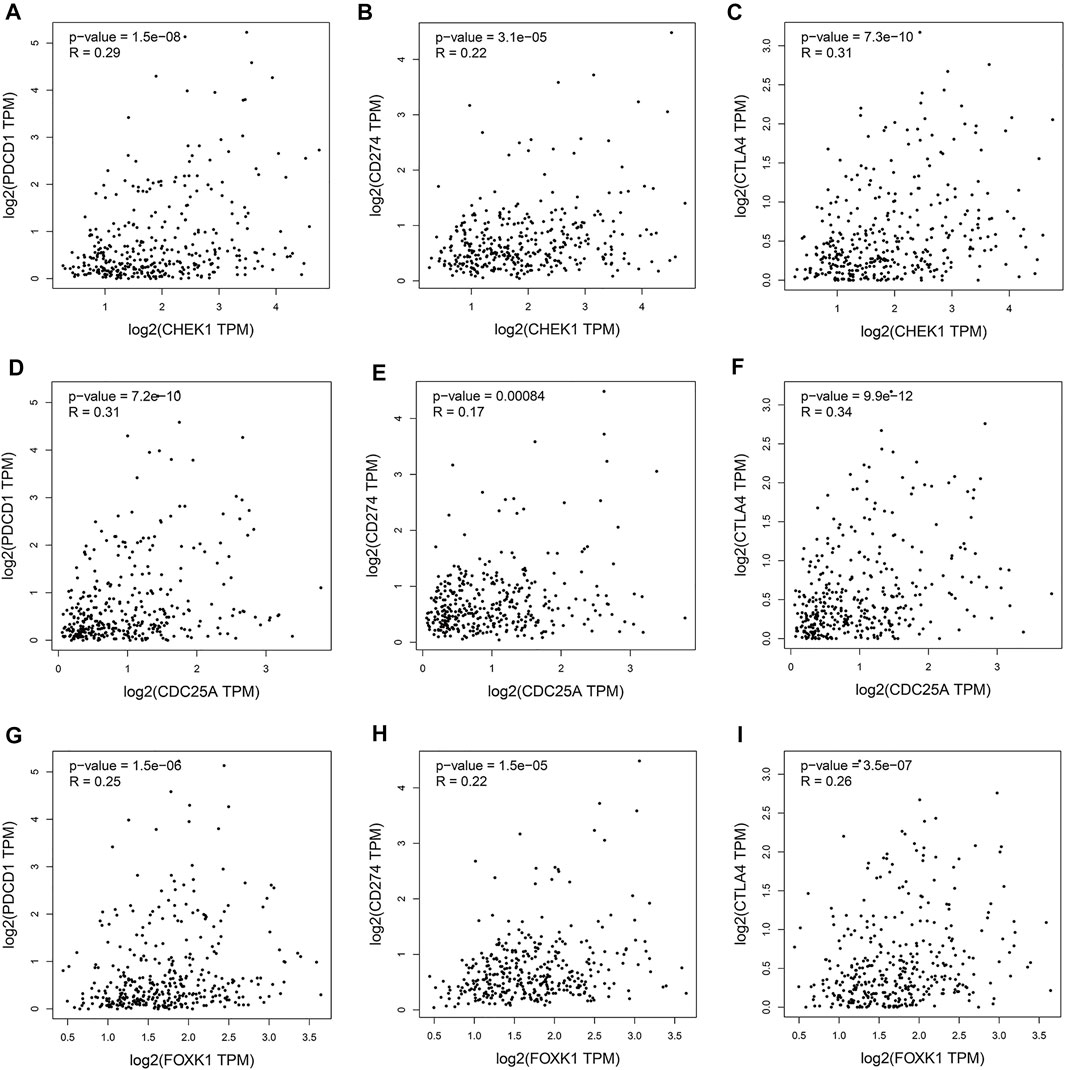
FIGURE 10. Associations of the expression of CHEK1 (A–C), CDC25A (D–F), FOXK1 (G–I) in the ceRNA network with the expression of immune checkpoints in LIHC.
Discussion
In recent years, emerging evidence (Kudo, 2010; Zhang et al., 2020a) has shown that circRNAs are involved in the occurrence and development of tumors through the regulation of parental gene expression, transcriptional translation, and protein modification, but the most crucial role is through the sponge mechanism. Previous research (Huang et al., 2020) indicated that hsa_circRNA_104348 might promote LIHC progression by targeting the miR-187–3p/RTKN2 axis and activating the Wnt/β-catenin pathway. Zhang et al. (2020b) suggested that circTMEM45A sponges miR-665 to promote LIHC progression. In addition, exosomal circUHRF1 (Zhang et al., 2020c) was reported to increase the expression of TIM-3 by sponging miR-449c-5p and inducing NK cell failure. Nevertheless, the molecular characteristics of circRNA-related ceRNA networks in LIHC have not been fully and deeply studied. Therefore, in this study, we constructed a survival-related circRNA-miRNA-mRNA network of LIHC, which will provide potential prognostic biomarkers and therapeutic targets for LIHC.
As a new family of ncRNA molecules, circRNAs affect the occurrence of tumors through glycolysis, metastasis and the cell cycle (Meng et al., 2017). In this study, we performed differential expression analysis on the GSE164803 dataset in the GEO database, identified seven circRNAs with significantly upregulated expression in liver cancer tissues compared with normal liver tissues and verified them in LIHC clinical specimens by qRT–PCR. Among them, one circRNA was previously reported to be closely related to cancer: hsa_circ_0051488 sponges miR-6717–5p, thereby regulating the expression of SATB2, which plays a vital role in lung squamous cell carcinoma (Xiao et al., 2021). However, there is no research focusing on the significance of the other circRNAs, and they are worthy of further study.
MiRNAs, a class of small molecules 21–25 nucleotides in length, are closely related to tumor occurrence, development, metastasis, and drug resistance (Santarpia et al., 2013; Bottai et al., 2014). In the present study, nine DEmiRNAs were identified in the first step. Then, to enhance the reliability of our research, we conducted prognostic analysis on these DEmiRNAs and finally selected hsa-miR-195–5p for further investigation. Studies have shown (Xu et al., 2015) that miR-195–5p inhibits the proliferation, migration and invasion of liver cancer cells by regulating PHF19. Furthermore, Cai et al. (2018) found that PRR11 is an important downstream mediator of the suppressive effects of miR-195 on prostate cancer progression. These investigations demonstrated that hsa-miR-195–5p plays a tumor suppressor role in various cancers, which partially increased the reliability of our results. To understand the downstream mechanism of hsa-miR-195–5p, we predicted its target genes by employing miRDB, mirtarbase, and TargetScan. Then, we overlapped these target genes with DEmRNAs, and 21 intersecting mRNAs were obtained. GO and KEGG analyses revealed that the 21 genes played a crucial role in many cancer-related biological functions and pathways. Next, we performed prognostic analysis and correlation analysis for the 21 mRNAs and screened the three mRNAs to establish the circRNA-miRNA-mRNA network (Figure 11). Moreover, CHEK1 (Bao et al., 2018; Gong et al., 2020), CDC25A (Liu et al., 2012; Liu et al., 2021), and FOXK1 (Chen et al., 2021; Meng et al., 2021) have been reported as oncogenes, which is consistent with our previous analysis. CHEK1 (Stelzer et al., 2016) is a serine/threonine-specific protein kinase that mediates cell cycle arrest when DNA damage occurs. It was reported (Wu et al., 2019) that CHEK1 overexpression leads to the development of human malignant tumors. As one of the cell cycle regulators, CDC25A participates in the radioresistance of various tumor cells (Ding et al., 2019)). FOXK1 (Feng et al., 2021) is a member of the FOX transcription factor family. The dysregulation of FOXK1 expression and subcellular localization leads to the uncontrolled development and progression of many tumors. Furthermore, GSEA revealed that CHEK1, CDC25A and FOXK1 were enriched in the cell cycle, pathways in cancer, and the P53 signaling pathway, which are correlated with cancer pathogenesis.
Accumulating studies (Jiang et al., 2021; Zhou et al., 2021) have found that tumor-infiltrating immune cells, including B cells, CD4+/CD8+ T cells, macrophages, neutrophils and dendritic cells, affect the efficacy of chemotherapy and immunotherapy and thus affect the prognosis of patients. Our work showed that the ceRNA regulatory network was significantly related to the infiltration of immune cells and the expression of immune checkpoints. Based on the above research, we speculated that the ceRNA regulatory network discovered in this study may be involved in the immune escape of tumor cells. The results will help improve the efficacy of immunotherapy for LIHC.
However, this study has some limitations that need to be considered. First, the construction of a circRNA–miRNA–mRNA regulatory network mainly relies on a series of bioinformatic analyses and databases, and its authenticity and accuracy need to be verified in more experiments. Second, we could not obtain all the clinicopathological data for each LIHC patient in the TCGA database, so the survival analysis may have lower statistical power. Finally, the sample size of the analyzed dataset from the GEO and TCGA was not large, and the number of paired samples was small.
In conclusion, we discovered the survival-related hsa_circ_0017264-hsa-miR-195-5p-CHEK1/CDC25A/FOXK1 regulatory network through a series of bioinformatics analyses. Furthermore, this regulatory network can alter the effect of immunotherapy by affecting tumor immune cell infiltration and immune checkpoint expression, which provides new therapeutic targets for clinical LIHC treatment.
Data Availability Statement
The original contributions presented in the study are included in the article/Supplementary Material, further inquiries can be directed to the corresponding author.
Ethics Statement
The studies involving human participants were reviewed and approved by Ethics Committee of the First Affiliated Hospital of Nanchang University. The patients/participants provided their written informed consent to participate in this study.
Author Contributions
LZ designed the research. WF processed the figures and tables. YG drew up the manuscript and contribution to the data analyses. YZ conducted molecular experiments. PW accomplished statistical analysis for the data. RS collected clinical specimens. All authors have browsed and agreed with the final manuscript.
Funding
This work was supported by the National Natural Science Foundation of China (grant number 81760431) and the Natural Science Foundation of Jiangxi Province, China (grant number 20161BAB205243).
Publisher’s Note
All claims expressed in this article are solely those of the authors and do not necessarily represent those of their affiliated organizations, or those of the publisher, the editors and the reviewers. Any product that may be evaluated in this article, or claim that may be made by its manufacturer, is not guaranteed or endorsed by the publisher.
Conflict of Interest
The authors declare that the research was conducted in the absence of any commercial or financial relationships that could be construed as a potential conflict of interest.
Supplementary Material
The Supplementary Material for this article can be found online at: https://www.frontiersin.org/articles/10.3389/fgene.2022.800537/full#supplementary-material
References
Agarwal, V., Bell, G. W., Nam, J.-W., and Bartel, D. P. (2015). Predicting Effective microRNA Target Sites in Mammalian mRNAs. Elife 4, 4. doi:10.7554/eLife.05005
Bao, J., Yu, Y., Chen, J., He, Y., Chen, X., Ren, Z., et al. (2018). MiR-126 Negatively Regulates PLK-4 to Impact the Development of Hepatocellular Carcinoma via ATR/CHEK1 Pathway. Cell Death Dis 9 (10), 1045. doi:10.1038/s41419-018-1020-0
Bottai, G., Pasculli, B., Calin, G. A., and Santarpia, L. (2014). Targeting the microRNA-Regulating DNA Damage/repair Pathways in Cancer. Expert Opin. Biol. Ther. 14 (11), 1667–1683. doi:10.1517/14712598.2014.950650
Cai, C., He, H., Duan, X., Wu, W., Mai, Z., Zhang, T., et al. (2018). miR-195 Inhibits Cell Proliferation and Angiogenesis in Human Prostate Cancer by Downregulating PRR11 Expression. Oncol. Rep. 39 (4), 1658–1670. doi:10.3892/or.2018.6240
Cancer Genome Atlas Research Network (2017). Comprehensive and Integrative Genomic Characterization of Hepatocellular Carcinoma. Cell 169 (7), 1327–e23. e23. doi:10.1016/j.cell.2017.05.046
Chen, W., Li, Y., Zhong, J., and Wen, G. (2021). Circ-PRKCI Targets miR-1294 and miR-186-5p by Downregulating FOXK1 Expression to Suppress Glycolysis in Hepatocellular Carcinoma. Mol. Med. Rep. 23 (6). doi:10.3892/mmr.2021.12103
Chen, W., Quan, Y., Fan, S., Wang, H., Liang, J., Huang, L., et al. (2020). Exosome-transmitted Circular RNA Hsa_circ_0051443 Suppresses Hepatocellular Carcinoma Progression. Cancer Lett. 475, 119–128. doi:10.1016/j.canlet.2020.01.022
Chen, Y., and Wang, X. (2020). miRDB: an Online Database for Prediction of Functional microRNA Targets. Nucleic Acids Res. 48 (D1), D127–D131. doi:10.1093/nar/gkz757
Chou, C.-H., Shrestha, S., Yang, C.-D., Chang, N.-W., Lin, Y.-L., Liao, K.-W., et al. (2018). miRTarBase Update 2018: a Resource for Experimentally Validated microRNA-Target Interactions. Nucleic Acids Res. 46 (D1), D296–D302. doi:10.1093/nar/gkx1067
Ding, F. N., Gao, B. H., Wu, X., Gong, C. W., Wang, W. Q., and Zhang, S. M. (2019). miR‐122‐5p Modulates the Radiosensitivity of Cervical Cancer Cells by Regulatingcell Division Cycle 25A(CDC25A). FEBS Open Bio 9 (11), 1869–1879. doi:10.1002/2211-5463.12730
Feng, Y., Bai, Z., Song, J., and Zhang, Z. (2021). FOXK1 Plays an Oncogenic Role in the Progression of Hilar Cholangiocarcinoma. Mol. Med. Rep. 23 (2). doi:10.3892/mmr.2020.11730
Glažar, P., Papavasileiou, P., and Rajewsky, N. (2014). circBase: a Database for Circular RNAs. RNA 20 (11), 1666–1670. doi:10.1261/rna.043687.113
Gong, D., Feng, P.-C., Ke, X.-F., Kuang, H.-L., Pan, L.-L., Ye, Q., et al. (2020). Silencing Long Non-coding RNA LINC01224 Inhibits Hepatocellular Carcinoma Progression via MicroRNA-330-5p-Induced Inhibition of CHEK1. Mol. Ther. - Nucleic Acids 19, 482–497. doi:10.1016/j.omtn.2019.10.007
He, Q., Tao, L., Xu, H., Xie, X., and Cheng, S. (2021). Circ_0072995 Promotes Proliferation and Invasion via Regulating miR-1253/EIF4A3 Signaling in HCC. Cmar Vol. 13, 5981–5987. doi:10.2147/CMAR.S316559
Huang, G., Liang, M., Liu, H., Huang, J., Li, P., Wang, C., et al. (2020). CircRNA hsa_circRNA_104348 Promotes Hepatocellular Carcinoma Progression through Modulating miR-187-3p/RTKN2 axis and Activating Wnt/β-Catenin Pathway. Cel Death Dis 11 (12), 1065. doi:10.1038/s41419-020-03276-1
Jiang, Q., Sun, J., Chen, H., Ding, C., Tang, Z., Ruan, Y., et al. (2021). Establishment of an Immune Cell Infiltration Score to Help Predict the Prognosis and Chemotherapy Responsiveness of Gastric Cancer Patients. Front. Oncol. 11, 650673. doi:10.3389/fonc.2021.650673
Kudo, M. (2010) Management Of Hepatocellular Carcinoma: From Prevention To Molecular Targeted Therapy. Oncology 78 (Suppl. 1), 1–6. doi:10.1159/000315222
Li, C., Zhang, W., Yang, H., Xiang, J., Wang, X., and Wang, J. (2020). Integrative Analysis of Dysregulated lncRNA-Associated ceRNA Network Reveals Potential lncRNA Biomarkers for Human Hepatocellular Carcinoma. PeerJ 8, e8758. doi:10.7717/peerj.8758
Li, J.-H., Liu, S., Zhou, H., Qu, L.-H., and Yang, J.-H. (2014). starBase v2.0: Decoding miRNA-ceRNA, miRNA-ncRNA and Protein-RNA Interaction Networks from Large-Scale CLIP-Seq Data. Nucl. Acids Res. 42 (Database issue), D92–D97. doi:10.1093/nar/gkt1248
Li, T., Fan, J., Wang, B., Traugh, N., Chen, Q., Liu, J. S., et al. (2017). TIMER: A Web Server for Comprehensive Analysis of Tumor-Infiltrating Immune Cells. Cancer Res. 77 (21), e108–e110. doi:10.1158/0008-5472.CAN-17-0307
Liu, P., Xia, P., Fu, Q., Liu, C., Luo, Q., Cheng, L., et al. (2021). miR-199a-5p Inhibits the Proliferation of Hepatocellular Carcinoma Cells by Regulating CDC25A to Induce Cell Cycle Arrest. Biochem. Biophysical Res. Commun. 571, 96–103. doi:10.1016/j.bbrc.2021.07.035
Liu, T., Yu, X., Li, G., Yuan, R., Wang, Q., Tang, P., et al. (2012). Rock2 Regulates Cdc25A through Ubiquitin Proteasome System in Hepatocellular Carcinoma Cells. Exp. Cel Res. 318 (16), 1994–2003. doi:10.1016/j.yexcr.2012.04.017
Livak, K. J., and Schmittgen, T. D. (2001). Analysis of Relative Gene Expression Data Using Real-Time Quantitative PCR and the 2−ΔΔCT Method. Methods 25 (4), 402–408. doi:10.1006/meth.2001.1262
Marrero, J. A. (2020). Surveillance for Hepatocellular Carcinoma. Clin. Liver Dis. 24 (4), 611–621. doi:10.1016/j.cld.2020.07.013
Meng, F., Liu, J., Lu, T., Zang, L., Wang, J., He, Q., et al. (2021). SNHG1 Knockdown Upregulates miR-376a and Downregulates FOXK1/Snail axis to Prevent Tumor Growth and Metastasis in HCC. Mol. Ther. - Oncolytics 21, 264–277. doi:10.1016/j.omto.2021.02.002
Meng, S., Zhou, H., Feng, Z., Xu, Z., Tang, Y., Li, P., et al. (2017). CircRNA: Functions and Properties of a Novel Potential Biomarker for Cancer. Mol. Cancer 16 (1), 94. doi:10.1186/s12943-017-0663-2
Ogunwobi, O. O., Harricharran, T., Huaman, J., Galuza, A., Odumuwagun, O., Tan, Y., et al. (2019). Mechanisms of Hepatocellular Carcinoma Progression. Wjg 25 (19), 2279–2293. doi:10.3748/wjg.v25.i19.2279
Ritchie, M. E., Phipson, B., Wu, D., Hu, Y., Law, C. W., Shi, W., et al. (2015). Limma powers Differential Expression Analyses for RNA-Sequencing and Microarray Studies. Nucleic Acids Res. 43 (7), e47. doi:10.1093/nar/gkv007
Salmena, L., Poliseno, L., Tay, Y., Kats, L., and Pandolfi, P. P. (2011). A ceRNA Hypothesis: the Rosetta Stone of a Hidden RNA Language. Cell 146 (3), 353–358. doi:10.1016/j.cell.2011.07.014
Sanger, H. L., Klotz, G., Riesner, D., Gross, H. J., and Kleinschmidt, A. K. (1976). Viroids Are Single-Stranded Covalently Closed Circular RNA Molecules Existing as Highly Base-Paired Rod-like Structures. Proc. Natl. Acad. Sci. 73 (11), 3852–3856. doi:10.1073/pnas.73.11.3852
Santarpia, L., Calin, G. A., Adam, L., Ye, L., Fusco, A., Giunti, S., et al. (2013). A miRNA Signature Associated with Human Metastatic Medullary Thyroid Carcinoma. Endocr. Relat. Cancer 20 (6), 809–823. doi:10.1530/ERC-13-0357
Stelzer, G., Rosen, N., Plaschkes, I., Zimmerman, S., Twik, M., Fishilevich, S., et al. (2016). The GeneCards Suite: From Gene Data Mining to Disease Genome Sequence Analyses. Curr. Protoc. Bioinformatics 54, 1 30 1–1 3. doi:10.1002/cpbi.5
Suh, J. K., Lee, J., Lee, J.-H., Shin, S., Tchoe, H. j., and Kwon, J.-W. (2018). Risk Factors for Developing Liver Cancer in People with and without Liver Disease. PLoS One 13 (10), e0206374. doi:10.1371/journal.pone.0206374
Sung, H., Ferlay, J., Siegel, R. L., Laversanne, M., Soerjomataram, I., Jemal, A., et al. (2021). Global Cancer Statistics 2020: GLOBOCAN Estimates of Incidence and Mortality Worldwide for 36 Cancers in 185 Countries. CA A. Cancer J. Clin. 71 (3), 209–249. doi:10.3322/caac.21660
Tang, Z., Li, C., Kang, B., Gao, G., Li, C., and Zhang, Z. (2017). GEPIA: a Web Server for Cancer and normal Gene Expression Profiling and Interactive Analyses. Nucleic Acids Res. 45 (W1), W98–W102. doi:10.1093/nar/gkx247
Walter, W., Sánchez-Cabo, F., and Ricote, M. (2015). GOplot: an R Package for Visually Combining Expression Data with Functional Analysis: Fig. 1. Bioinformatics 31 (17), 2912–2914. doi:10.1093/bioinformatics/btv300
Wang, M., Yu, F., and Li, P. (2018). Circular RNAs: Characteristics, Function and Clinical Significance in Hepatocellular Carcinoma. Cancers 10 (8), 258. doi:10.3390/cancers10080258
Wang, X., Wang, X., Li, W., Zhang, Q., Chen, J., and Chen, T. (2019). Up-Regulation of Hsa_circ_0000517 Predicts Adverse Prognosis of Hepatocellular Carcinoma. Front. Oncol. 9, 1105. doi:10.3389/fonc.2019.01105
Wu, M., Pang, J. S., Sun, Q., Huang, Y., Hou, J. Y., Chen, G., et al. (2019). The Clinical Significance of CHEK1 in Breast Cancer: a High-Throughput Data Analysis and Immunohistochemical Study. Int. J. Clin. Exp. Pathol. 12 (1), 1
Xia, S., Feng, J., Chen, K., Ma, Y., Gong, J., Cai, F., et al. (2018). CSCD: a Database for Cancer-specific Circular RNAs. Nucleic Acids Res. 46 (D1), D925–D929. doi:10.1093/nar/gkx863
Xiao, M., Cui, S., Zhang, L., Yu, T., Zhang, G., Li, L., et al. (2021). Benzo[a]pyrene Diol Epoxide‐induced Transformed Cells Identify the Significance of Hsa_circ_0051488, a ERCC1‐derived Circular RNA in Pulmonary Squamous Cell Carcinoma. Mol. Carcinogenesis 60, 684–701. doi:10.1002/mc.23335
Xu, H., Hu, Y.-W., Zhao, J.-Y., Hu, X.-M., Li, S.-F., Wang, Y.-C., et al. (2015). MicroRNA-195-5p Acts as an Anti-oncogene by Targeting PHF19 in Hepatocellular Carcinoma. Oncol. Rep. 34 (1), 175–182. doi:10.3892/or.2015.3957
Yao, Z., Xu, R., Yuan, L., Xu, M., Zhuang, H., Li, Y., et al. (2019). Circ_0001955 Facilitates Hepatocellular Carcinoma (HCC) Tumorigenesis by Sponging miR-516a-5p to Release TRAF6 and MAPK11. Cel Death Dis 10 (12), 945. doi:10.1038/s41419-019-2176-y
Yu, G., Wang, L.-G., Han, Y., and He, Q.-Y. (2012). clusterProfiler: an R Package for Comparing Biological Themes Among Gene Clusters. OMICS: A J. Integr. Biol. 16 (5), 284–287. doi:10.1089/omi.2011.0118
Zhang, P.-F., Gao, C., Huang, X.-Y., Lu, J.-C., Guo, X.-J., Shi, G.-M., et al. (2020). Cancer Cell-Derived Exosomal circUHRF1 Induces Natural Killer Cell Exhaustion and May Cause Resistance to Anti-PD1 Therapy in Hepatocellular Carcinoma. Mol. Cancer 19 (1), 110. doi:10.1186/s12943-020-01222-5
Zhang, T., Jing, B., Bai, Y., Zhang, Y., and Yu, H. (2020). Circular RNA circTMEM45A Acts as the Sponge of MicroRNA-665 to Promote Hepatocellular Carcinoma Progression. Mol. Ther. - Nucleic Acids 22, 285–297. doi:10.1016/j.omtn.2020.08.011
Zhang, X., Lu, N., Wang, L., Wang, Y., Li, M., Zhou, Y., et al. (2020). Circular RNAs and Esophageal Cancer. Cancer Cel Int 20, 362. doi:10.1186/s12935-020-01451-0
Keywords: liver hepatocellular carcinoma, competing endogenous RNA network, circular RNAs, bioinformatics, immune infiltration
Citation: Gan Y, Fang W, Zeng Y, Wang P, Shan R and Zhang L (2022) Identification of a Novel Survival-Related circRNA–miRNA–mRNA Regulatory Network Related to Immune Infiltration in Liver Hepatocellular Carcinoma. Front. Genet. 13:800537. doi: 10.3389/fgene.2022.800537
Received: 18 November 2021; Accepted: 03 February 2022;
Published: 02 March 2022.
Edited by:
Tao Huang, Shanghai Institute of Nutrition and Health (CAS), ChinaReviewed by:
Petar Ozretić, Rudjer Boskovic Institute, CroatiaWei-Lin Wang, Zhejiang University, China
Copyright © 2022 Gan, Fang, Zeng, Wang, Shan and Zhang. This is an open-access article distributed under the terms of the Creative Commons Attribution License (CC BY). The use, distribution or reproduction in other forums is permitted, provided the original author(s) and the copyright owner(s) are credited and that the original publication in this journal is cited, in accordance with accepted academic practice. No use, distribution or reproduction is permitted which does not comply with these terms.
*Correspondence: Ling Zhang, bmR5ZnkxMzA2QG5jdS5lZHUuY24=