- 1School of Public Health, Li Ka Shing Faculty of Medicine, The University of Hong Kong, Hong Kong, China
- 2Key Laboratory of Carcinogenesis and Translational Research (Ministry of Education/Beijing), Department of Radiation Oncology, Peking University Cancer Hospital and Institute, Beijing, China
Background: Lack of definitive evidence supports the putative hypothesis that gut microbiota dysbiosis is associated with Barrett’s esophagus (BE). We conducted a two-sample Mendelian randomization study to assess the associations of 21 genera of human gut microbiota with BE.
Methods: We identified independent genetic instruments for 21 genera of gut microbiota (including nine dominant genera, four core genera among individuals of European ancestry, and eight esophagus-specific genera of gut microbiota) from MiBioGen (up to 18,340 participants). We applied them to summary statistics from the largest publicly available genome-wide association study on BE (9,680 cases and 31,211 controls). We obtained the causal estimates of genetically predicted higher genera of gut microbiota and BE using the inverse variance weighting method. Sensitivity analyses included weighted median, MR-Egger, MR-RAPS, and MR-PRESSO.
Results: We found that genetically predicted higher Actinomyces (OR: 0.76 per unit increase in log odds of having BE, 95% CI: 0.70–0.83) and higher Ruminiclostridium (OR: 0.75, 95% CI: 0.63–0.90) were significantly associated with a lower risk of BE. No associations of other genera of gut microbiota with BE were noted, apart from suggestive associations of higher Alistipes (OR: 0.77; 95% CI: 0.61–0.99), higher Eubacterium (OR: 0.89; 95% CI: 0.80–0.99), and higher Veillonella (OR: 0.76; 95% CI: 0.56–1.02) with a lower risk of BE, and higher Faecalibacterium (OR: 1.15; 95% CI: 0.99–1.33) with a higher risk of BE.
Conclusion: This study suggests that higher Actinomyces and higher Ruminiclostridium might protect against BE.
Introduction
The International Agency for Research on Cancer reported that around 15%–20% of cancer cases were attributable to microbial, particularly commensal microbiota (IARC Working Group on the Evaluation of Carcinogenic Risks to Humans, 2012). The human gastrointestinal (GI) tract, harboring up to ten thousand billion individual non–site-specific bacteria and three million bacterial genes, has recently become the focus of great interest as target interventions to combat Barrett’s esophagus (BE) and alleviate the side effects of therapeutics on GI cancer (Bhatt et al., 2017; Gillespie et al., 2021). Generally, dysbiosis changing the composition and function of gut microbiota appears to manipulate the host immune system and produce metabolites, which have been implicated in the development of BE and esophageal adenocarcinoma (EAC) (Bhatt et al., 2017; Levy et al., 2017). Several possible underlying mechanisms support such a hypothesis, for example, the loss of tolerance by the host immune system, modulating inflammation, inducing DNA damage, producing metabolites involved in tumor initiation and progression, and maladaptation of the host’s gut environment (Turnbaugh et al., 2007; Tozun and Vardareli, 2016; Bhatt et al., 2017; Levy et al., 2017). However, randomized controlled trials investigating the association of gut microbiota with BE remain scarce.
Observationally, several gut microbiomes have been reported to associate with BE. For example, a small-scale case-control study (ten BE cases and ten controls) showed that a decreased Streptococcus and increased Prevotella, Veillonella, and Leptotrichia were associated with a high BE risk (Lopetuso et al., 2020). The consistent result of a decreased Streptococcus on BE risk was also observed by another small-size study (Yang et al., 2009). In addition, increased Proteobacteria, Enterobacteriaceae, and Akkermansia were reported to associate with high-grade dysplasia risk (Snider et al., 2019). However, these results are usually difficult to interpret and cannot distinguish effects of changes in genera of gut microbiota from effects of confounding (e.g., diet and obesity), despite differences in sample collections, analysis methods, and study populations.
Mendelian randomization (MR) studies provide a valuable framework to investigate their roles in BE as it resists confounding by utilizing genetic variants randomly allocated at conception to proxy the exposure of interest (Smith and Ebrahim, 2003; Lawlor et al., 2008). More importantly, no MR study has been conducted to assess the possible gut microbiota–BE associations. To this end, we conducted a two-sample MR study using summary statistics from the largest publicly available genome-wide association study (GWAS) of gut microbiota and BE (An et al., 2019; Kurilshikov et al., 2021) in this study. We conducted a narrowed systematic review (Pei et al., 2004; Zilberstein et al., 2007; Yang et al., 2009; Blackett et al., 2013; Elliott et al., 2017; Dong et al., 2018; Hughes et al., 2020; Peter et al., 2020) and considered thirteen genera of human gut microbiota (i.e., Alistipes, Bacteroides, Blautia, Dorea, Faecalibacterium, Lachnoclostridium, Roseburia, Ruminococcus, Subdoligranulum, Ruminiclostridium, Fusicatenibacter, Butyricicoccus, and Eubacterium, in which Ruminiclostridium, Fusicatenibacter, Butyricicoccus, and Eubacterium are the core genera in the European descent) and eight esophagus-specific genera of gut microbiota (i.e., Actinomyces, Bifidobacterium, Haemophilus, Peptococcus, Lactobacillus, Prevotella, Streptococcus, and Veillonella) as shown in Table 1.
Methods
Study Design
This is a two-sample MR study that rests on three key assumptions for inferring causality (Smith and Ebrahim, 2003; Lawlor et al., 2008). First, the genetic instruments strongly predict the exposure of interest (i.e., the relevance assumption). Second, the genetic instruments are independent of confounders of the exposure–outcome association (i.e., the independence assumption). Third, the genetic instruments affect the outcome only via the exposure of interest (i.e., the exclusion–restriction assumption).
Exposure GWAS: 21 Genera of Gut Microbiota
We extracted genetic instruments, i.e., single-nucleotide polymorphism (SNP), for 21 genera of gut microbiota from summary statistics in MiBioGen, which is the largest and latest available 16S fecal microbiome data (up to 18,340 participants, including 16,632 adolescents and 1,708 children) on gut microbiota of individuals dominated by of European descent (∼72.3%, 13,266 participants) (Kurilshikov et al., 2021). We selected instruments suggestively [i.e., p < 5 × 10–6, which is commonly used to highlight “suggestive” genetic variants (Manolio, 2010)] and independently (i.e., r2 < 0.01, based on the 1000G European population reference panel) associated with each genus of gut microbiota. The median age of participants in MiBioGen was ∼46.5 years (range: 4–89 years) with ∼44.2% (∼8,073 participants) of males. The genetic effects on genera of gut microbiota were meta-analyzed using either the abundance levels (mbQTL, i.e., samples with zero abundance were truncated) or a binary trait coding presence/absence (mbBTL, i.e., presence versus absence of the bacterial genus), depending on their absolute abundance levels across sub-cohorts. These microbiome GWASs adjusted for age, sex, study-specific covariates, and the top genetic principal components for population stratification. Additional file 1: Supplementary Table S1 shows the list of genetic instruments for genera of gut microbiota included in this study.
Outcome GWAS: BE
We extracted summary statistics of BE from the most recent meta-analyzed BE GWAS (access via study accession GCST90000515 (Ong et al., 2021), including 3,513 cases and 14,052 controls in UK Biobank (An et al., 2019); and 6,167 cases and 17,159 controls in a sub-meta BE GWAS (Gharahkhani et al., 2016)) based on the rs number of the identified genetic instruments for 21 genera. Specifically, for UK Biobank, BE and EAC diagnosed with ICD-10 codes (i.e., K22.7 for BE and C15 for EAC) based on self-report and clinical diagnosis were combined as one phenotype mainly because BE is the premalignant precursor of EAC and has a very high genetic correlation with EAC (An et al., 2019). For the sub-meta BE GWAS of all individuals of European descent, patients with BE were identified by the histopathological diagnosis of intestinal metaplasia (Gharahkhani et al., 2016). The meta-analyzed BE GWAS adjusted for age, sex, study-specific covariates, and the first 10 principal components. Additional file 1: Supplementary Table S1 shows the genetic instrument associations with BE.
Pleiotropic Effects
Given that previous studies (including MR and systematic reviews) showed potential roles of obesity (Thrift et al., 2014), depression (Ong et al., 2021), years of schooling (Ong et al., 2021), and cigarette smoking (Andrici et al., 2013; Cook et al., 2012), we further explored associations of the genetic instruments strongly predicted genera of gut microbiota in respective GWASs with obesity proxied by the waist–hip ratio (GIANT and UK Biobank, n = 697,924 participants) (Pulit et al., 2019), cigarette smoking proxied by cigarettes smoked per day (a meta-analyzed GWAS, n = 337,334 participants) (Liu et al., 2019), depression (a meta-analyzed GWAS, n = 246,363 cases and 561,191 controls) (Howard et al., 2019), and years of schooling (SSGAC, up to 1,131,881 participants) (Lee et al., 2018). Details about study participants included in these GWASs are presented in Additional file 1: Supplementary Table S2. We excluded the instrument associated with any of these phenotypes aforementioned at genome-wide significance (p < 5 × 10−8) to reduce the possibility of pleiotropy. Here, we did not consider the potential pleiotropic effects of GERD on BE because they shared considerable genetic variants, responding for ∼91% (An et al., 2019). In addition, we cross-checked the associations of the selected instruments with any causes of death using the comprehensively genotype-to-phenotype cross-reference PhenoScanner at p < 5 × 10−8 to reduce other sources of pleiotropy and the risk of selection bias (Kamat et al., 2019; Schooling et al., 2020; Yang et al., 2021). Additional file 1: Supplementary Table S3 shows the excluded instruments and the corresponding pleiotropic effects.
Statistical Analysis
We assessed the instrument strength using the F statistic, whose value less than 10 indicated a higher likelihood of weak instrument bias (Bowden et al., 2016a). We used the multiplicative random-effects inverse-variance weighted method to estimate the association of genetically predicted higher genus of gut microbiota on BE by pooling the Wald estimator [i.e., the ratio between the SNP-outcome effect and the SNP-exposure effect with its standard error being approximated using the first-order weights (Bowden et al., 2017)] of each SNP. We reported the heterogeneity of the Wald estimator using the Cochrane Q statistics (Bowden et al., 2017), with the potential directional pleiotropy indicated by p<0.05 for MR-Egger intercept (Bowden et al., 2015).
We also conducted the sensitivity analysis using a weighted median estimator (Bowden et al., 2016b), MR-Egger (Bowden et al., 2015; Burgess and Thompson, 2017), MR-PRESSO (Verbanck et al., 2018), and MR robust adjusted profile score (MR-RAPS) (Zhao et al., 2020). Specifically, the weighted median method allows for up to 50% of weights from invalid instruments and produces a consistent causal estimate (Bowden et al., 2016b). MR-Egger detects the directional pleiotropy using the p value for the intercept and provides causal estimates after adjusting for pleiotropic effects with an additional assumption about the instrument strength independent of the dependent effect (i.e., InSIDE assumption) (Bowden et al., 2015; Burgess and Thompson, 2017). The MR-PRESSO detects the outlying instruments and provides consistent causal estimates after removing possible outliers with an additional assumption of no invalid instruments (Verbanck et al., 2018). The MR-RAPS allows the inclusion of weak instruments and the presence of systematic and idiosyncratic pleiotropy and provides consistent causal estimates using an adjusted profile likelihood estimator (Zhao et al., 2020).
Power Analysis
We approximated the variance of each genus of gut microbiota explained by the included instruments using well-established methods for continuous and binary exposures (Lee et al., 2012; Yarmolinsky et al., 2018). We assessed the power of our MR analyses using the online calculator mRnd (https://shiny.cnsgenomics.com/mRnd/) (Brion et al., 2013). Additional file 1: Supplementary Table S1 presents the estimated variance of each genus explained by the instruments. Additional file: Supplementary Table S4 shows the power of the estimated gut microbiota–BE associations.
All analyses were performed using R Version 3.6.2 (R Core Team (2019). R: A language and environment for statistical computing (R Foundation for Statistical Computing, Vienna, Austria. https://www.R-project.org/), with the R package “TwoSampleMR” (Hemani et al., 2018). We reported a two-sided p value at the Bonferroni-corrected threshold of 0.05/22 = 0.002, and the p value between 0.002 and 0.05 was considered suggestive of causation. We adhere to STROBE-MR: Guidelines for strengthening the reporting of observational studies in epidemiological studies using Mendelian randomization for reporting our results (Skrivankova et al., 2021).
Ethics Approval
This analysis of publicly available data does not require ethical approval.
Results
Up to 15 genetic instruments for Alistipes, 12 instruments for Bacteroides, 13 instruments for Blautia, 12 instruments for Dorea, 13 instruments for Faecalibacterium, 15 instruments for Lachnoclostridium, 18 instruments for Roseburia, 14 instruments for Ruminococcus, 14 instruments for Subdoligranulum, 9 instruments for Butyricicoccus, 20 instruments for Fusicatenibacter, 15 instruments for Ruminiclostridium, 19 instruments for Eubacterium, 8 instruments for Actinomyces, 18 instruments for Bifidobacterium, 14 instruments for Haemophilus, 12 instruments for Lactobacillus, 17 instruments for Peptococcus, 20 instruments for Prevotella, 18 instruments for Streptococcus, and 11 instruments for Veillonella were used in this study, as shown in Table 1. All instruments had F statistics (ranging from 17.0 to 88.4) greater than 10, implying a less likely weak instrument bias. Two genetic instruments associated with obesity (rs182549) and depression (rs17708276) were identified and excluded from the MR analysis (Additional file 1: Supplementary Table S3).
Figure 1 shows the associations of genetically predicted genera of gut microbiota with BE, with complete results presented in Additional file 1: Supplementary Table S4. No genera of gut microbiota–BE associations were observed after adjusting for multiplicity. However, suggestive associations of genetically predicted higher Alistipes with a lower BE risk and higher Faecalibacterium with a higher BE risk were noted, with no heterogeneity identified by the Cochran’s Q statistics test. The MR-Egger intercept indicated no horizontal pleiotropy. Furthermore, MR analyses had adequate power (i.e., 80%) to detect a significant association of a genus with BE at α = 0.05 level, given the true association exists. However, the corresponding estimates from MR-Egger regression were not always consistent with the main results, with wide confidence intervals and even reversed causal directions.
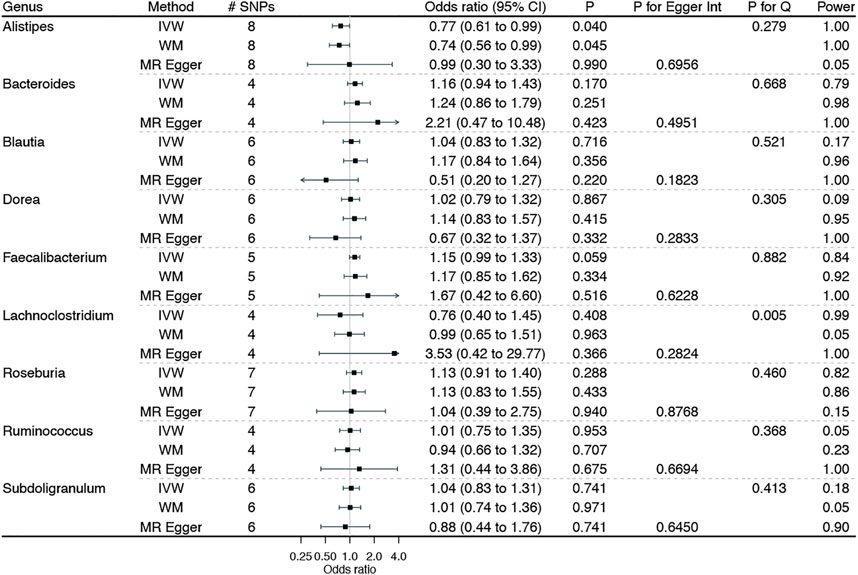
FIGURE 1. Associations of genetically predicted genera of gut microbiota on Barrett’s esophagus using Mendelian randomization. IVW: inverse variance weighted method with multiplicative random-effects; WM: weighted median estimator.
Figure 2 shows the associations of genetically predicted genera of gut microbiota dominated among individuals from European ancestry with BE, with complete results presented in Additional file 1: Supplementary Table S4. Genetically predicted higher Ruminiclostridium was significantly associated with a lower BE risk. The Cochran’s Q statistics test indicated no heterogeneity, and the MR Egger intercept suggested no horizontal pleiotropy. Similarly, higher Eubacterium was suggestively associated with a lower BE risk, with no identified heterogeneity and horizontal pleiotropy. Consistent results were also noted from sensitivity analyses, although not reach the Bonferroni-corrected significance.
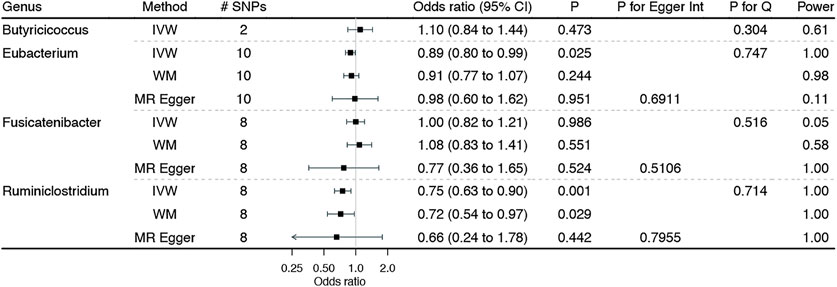
FIGURE 2. Associations of genetically predicted genera of gut microbiota dominated among individuals of European ancestry on Barrett’s esophagus. IVW: inverse variance weighted method with multiplicative random effects expect for Butyricicoccus using the fixed-effect inverse variance weighted method due to the inclusion of two genetic instruments; WM: weighted median estimator.
Figure 3 shows the associations of genetically predicted esophagus-specific genera of gut microbiota with BE, with complete results presented in Additional file 1: Supplementary Table S4. Genetically predicted higher Actinomyces was significantly associated with lower BE risk, with no heterogeneity and horizontal pleiotropy but adequate power. In addition, higher Veillonella was suggestively associated with lower BE risk, with no heterogeneity and horizontal pleiotropy. Though sensitivity analyses yield similar results, none of them reached the Bonferroni-corrected significance.
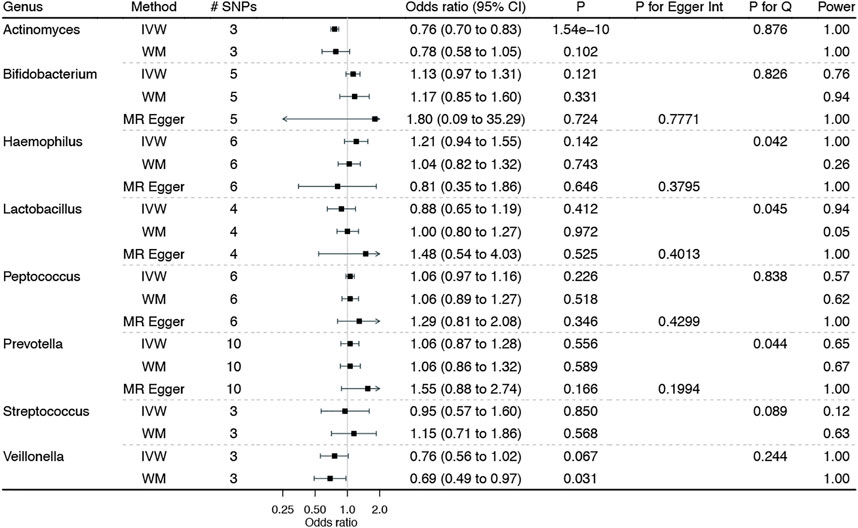
FIGURE 3. Associations of genetically predicted esophagus-specific genera of gut microbiota on Barrett’s esophagus. IVW: inverse variance weighted method with multiplicative random-effects; WM: weighted median estimator. Notably, the corresponding estimates from MR-Egger regression for Actinomyces, Streptococcus, and Veillonella were not showed herein due to the wide confidence intervals.
Discussion
Principal Findings
This MR study, taking advantage of the largest publicly available GWAS on gut microbiota and BE, was the first extensive analysis investigating the potential roles of a broad range of the dominant genera of human gut microbiota in BE. Our study found that genetically predicted higher Actinomyces and higher Ruminiclostridium appeared to protect against BE. Our study also found that genetically predicted higher Alistipes, higher Eubacterium, and higher Veillonella were suggestively associated with lower BE risk, while higher Faecalibacterium was associated with higher BE risk.
Comparison With Other Studies
In particular, there was no evidence supporting an association of Streptococcus with BE in our study, in which Streptococcus was thought to be the dominant BE-specific microbiota (Yang et al., 2009; Gall et al., 2015). This finding is inconsistent with that of previous observational studies showing that higher Streptococcus was positively associated with BE (Lopetuso et al., 2020). However, the discrepancies between previous findings and our study may be due to the chance finding caused by the small sample size and the unmeasured confounding caused by other factors, such as obesity and health status.
Our findings were consistent with those of previous studies showing that higher Actinomyces and higher Veillonella were inversely associated with BE (Elliott et al., 2017; Snider et al., 2019; Zhou et al., 2020), although the Veillonella-BE association became less evident after adjusting for multiplicity. However, our findings did not show any association of Prevotella with BE risk, which was inconsistent with previous observational studies showing a possible association of Prevotella with BE/EAC (Elliott et al., 2017; Snider et al., 2019; Lopetuso et al., 2020). Such discrepancies may be the consequence of confounding bias in observational studies arising from the diet, environmental factors, or medication (Rothschild et al., 2018; Hills et al., 2019), which were thought to shape the composition and abundance of genera of gut microbiota in humans in real-time, probably through mediations of metabolites and inflammatory cytokines (e.g., IL-8) (Munch et al., 2019).
Our findings also suggested that higher Alistipes appeared to protect against BE, which was not reported in previous studies. Nevertheless, from a biological perspective, higher Alistipes (i.e., a recently discovered gram-negative and anaerobic genus of the Bacteroidetes phylum in mostly healthy human GI tract) acts as a potential pathogen, contributing to the beneficial immunomodulation in cancer [e.g., colorectal cancer (Moschen et al., 2016)]. Second, Alistipes has been reported to modulate the tumor microenvironment and gut inflammation (Parker et al., 2020), and thus might have a role in cancer immunotherapy. For instance, manipulating the tumor microenvironment by reducing tumor necrosis factor produced myeloid cells using antibiotics appeared to decrease the tumor eradication rate (Iida et al., 2013). Furthermore, patients with non–small-cell lung cancer who responded to nivolumab (a checkpoint inhibitor for PD-1) tended to have an elevated Alistipes (Jin et al., 2019). Third, Alistipes-related dysbiosis has already been implicated in several other diseases (e.g., liver fibrosis (Rau et al., 2018), cardiovascular diseases (Zuo et al., 2019), and mood disorder (Bangsgaard Bendtsen et al., 2012)), possibly via inflammation.
In addition, our findings suggested that higher Faecalibacterium [i.e., a gram-positive and anaerobic bacterium that is one of the most abundant and critical commensal bacteria of human gut microbiota in the intestine (Miquel et al., 2013; Bag et al., 2017)] appeared to increase BE, possibly due to its role in boosting the immune system (Miquel et al., 2013) and improving gut barrier function (Stenman et al., 2016). Similar effects were also reported in Crohn’s disease (Wright et al., 2015). Finally, our findings suggested that higher Eubacterium (a gram-positive genus of the Eubacteriaceae family) and higher Ruminiclostridium may also have roles in reducing BE risk. However, further research studies using randomized controlled trials remain required to verify such findings.
Limitations
Although MR provides less confounded estimates of the gut microbiota–BE associations, limitations still exist. First, MR estimates rest on stringent assumptions (e.g., the independence and exclusion–restriction assumptions), which are always untestable (Glymour et al., 2012). However, in this study, we selected genetic instruments that strongly predicted gut microbiota from the largest publicly available GWAS, with an F statistic being greater than 10 to reduce the possibility of weak instrument bias (Bowden et al., 2016a). Furthermore, we selected valid genetic instruments at a “suggestive” threshold of p < 5 × 10–6, instead of the traditional genome-wide significance (i.e., p < 5 × 10–8), which may induce weak instrument bias. However, the sensitivity analyses, particularly MR-RAPS allowing the inclusion of weak genetic instruments (Zhao et al., 2020), yielded consistent results, indicating a less likely weak instrument bias. We excluded instruments associated with any potential confounders of the gut microbiota–BE associations and any known pleiotropic effects to reduce the risk of violating the independence and exclusion–restriction assumptions. We also conducted Cochran’s Q statistic test to detect the potential heterogeneity of the causal estimates and MR-Egger regression to examine the possible pleiotropic effects. No heterogeneity and pleiotropy were noted, as shown in Figures 1–3 and Additional file 1: Supplementary Table S4.
Second, the effects of genetic instruments on gut microbiota composition and abundance vary considerably across GWAS studies, and seldom do replications across these studies be available (Kurilshikov et al., 2017). As such, population-specific microbiota compositions may exist, which may result in a broad uncertainty and a lack of reproduction of our findings. However, the use of the largest publicly available GWAS of MiBioGen and extensive sensitivity analyses may reduce the risk of such issues. Furthermore, genetic instruments typically explain a small variation in a specific genus of gut microbiota, inducing underpowered MR estimates. However, our power analysis shows adequate power (i.e., 80%) to detect a positive association at the α = 0.05 level, given the true association exists.
Third, we limited MR analyses to participants of mainly European ancestry to reduce the risk of population stratification. Thus, our findings may not extend to other populations, although causations are always consistent across populations (Lopez et al., 2019). In addition, summary statistics obtained from GWAS on genera of gut microbiota had partially overlapping sets of participants from UK Biobank, which may bias our estimates with uncertain magnitudes and directions (Burgess et al., 2016). However, such an impact seemed small as MR-RAPS robust to the overlapping sample issue yielded similar results (Zhao et al., 2020).
Fourth, canalization buffering genetic factors may also exist. However, its impact on our estimates remains unknown. Fifth, our causal estimates reflected the natural genetic variation in lifelong exposure of genera of gut microbiota on BE, which might be different from the effects of short-term interventions with antibiotics.
Public Health Implications
Nevertheless, our findings provide genetic evidence for the associations of several genera of human gut microbiota with the risk of developing BE. Replication using different populations when the large-scale GWAS becomes available remains valuable to cross-validate our findings. Furthermore, manipulation of the abundance of Actinomyces and Ruminiclostridium may be helpful in preventing BE. However, Actinomyces are opportunistic pathogens in humans and may cause the infection of actinomycosis (Kononen and Wade, 2015), which should be considered. In addition, future research involving the associations of gut microbiota and BE might prioritize these four genera of gut microbiota (i.e., Alistipes, Eubacterium, Veillonella, and Faecalibacterium).
Conclusion
Our MR study showed that higher Actinomyces and higher Ruminiclostridium might have roles in preventing BE. Our study also suggested the potential benefit of higher Alistipes, Eubacterium, and Veillonella, and lower Faecalibacterium in preventing BE. However, a better understanding of their etiological roles in BE could provide additional insights and be valuable to reduce the burden of BE and EAC worldwide.
Data Availability Statement
Publicly available datasets were analyzed in this study. The names of the repository/repositories and accession number(s) can be found in the article/Supplementary Material; further inquiries can be directed to the corresponding author.
Ethics Statement
Each study has been specifically approved by the Ethical Committees of the original study and all the participants provided a written informed consent. This analysis of publicly data does not require ethical approval.
Author Contributions
ZY and WW designed the study. ZY wrote the analysis plan and interpreted the results. ZY performed the MR analyses with feedback from RY, WD, and WW. ZY and WD wrote the first draft of the manuscript with critical feedback and revisions from RY and WW. All authors read and approved the final manuscript.
Funding
This work was supported by the Beijing Municipal Science and Technology Commission (Z181100001718192), the National Natural Science Foundation (82073333), and the Science Foundation of Peking University Cancer Hospital (2022-19).
Conflict of Interest
The authors declare that the research was conducted in the absence of any commercial or financial relationships that could be construed as a potential conflict of interest.
Publisher’s Note
All claims expressed in this article are solely those of the authors and do not necessarily represent those of their affiliated organizations, or those of the publisher, the editors, and the reviewers. Any product that may be evaluated in this article, or claim that may be made by its manufacturer, is not guaranteed or endorsed by the publisher.
Acknowledgments
We thank the MiBioGen consortium (https://mibiogen.gcc.rug.nl/) for making the summary statistics of 16S fecal microbiome data publicly available. We thank the authors of “multitrait genetic association analysis identifies 50 new risk loci for gastro-esophageal reflux, seven new loci for Barrett’s oesophagus and provides insights into clinical heterogeneity in reflux diagnosis” for making the meta-analyzed summary statistics publicly available (study access GCST90000515). We thank the UK Biobank and the GIANT consortium for making the summary statistics of obesity proxied by the waist–hip ratio publicly available (https://zenodo.org/record/1251813#.YXPD155ByUk). We thank the GSCAN consortium (https://genome.psych.umn.edu/index.php/GSCAN) for making summary statistics of smoking proxied by cigarettes per day (MR-base id “ieu-b-25”) and drinking proxied by drinks per week (MR-base id “ieu-b-73”) publicly available (https://conservancy.umn.edu/handle/11299/201564). We thank the Psychiatric Genomics Consortium for making the meta-analyzed summary statistics of depression publicly available (https://datashare.ed.ac.uk/handle/10283/3203). We thank the SSGAC consortium (https://www.thessgac.org/) for making summary statistics of years of schooling (MR-base id “ieu-a-1239”) publicly available.
Supplementary Material
The Supplementary Material for this article can be found online at: https://www.frontiersin.org/articles/10.3389/fgene.2022.894900/full#supplementary-material
References
An, J., Gharahkhani, P., Law, M. H., Ong, J. S., Han, X., Olsen, C. M., et al. (2019). Gastroesophageal Reflux GWAS Identifies Risk Loci that Also Associate with Subsequent Severe Esophageal Diseases. Nat. Commun. 10 (1), 4219. Epub 2019/09/19PubMed PMID: 31527586; PubMed Central PMCID: PMCPMC6746768. doi:10.1038/s41467-019-11968-2
Andrici, J., Cox, M. R., and Eslick, G. D. (2013). Cigarette Smoking and the Risk of Barrett's Esophagus: a Systematic Review and Meta-Analysis. J. Gastroenterol. Hepatol. 28 (8), 1258–1273. Epub 2013/04/25PubMed PMID: 23611750. doi:10.1111/jgh.12230
Bag, S., Ghosh, T. S., and Das, B. (2017). Complete Genome Sequence of Faecalibacterium Prausnitzii Isolated from the Gut of a Healthy Indian Adult. Genome announc. 5 (46). Epub 2017/11/18PubMed PMID: 29146862; PubMed Central PMCID: PMCPMC5690339. doi:10.1128/genomeA.01286-17
Bangsgaard Bendtsen, K. M., Krych, L., Sorensen, D. B., Pang, W., Nielsen, D. S., Josefsen, K., et al. (2012). Gut Microbiota Composition Is Correlated to Grid Floor Induced Stress and Behavior in the BALB/c Mouse. PLoS One 7 (10), e46231. Epub 2012/10/12PubMed PMID: 23056268; PubMed Central PMCID: PMCPMC3462757. doi:10.1371/journal.pone.0046231
Bhatt, A. P., Redinbo, M. R., and Bultman, S. J. (2017). The Role of the Microbiome in Cancer Development and Therapy. CA Cancer J. Clin. 67 (4), 326–344. Epub 2017/05/10PubMed PMID: 28481406; PubMed Central PMCID: PMCPMC5530583. doi:10.3322/caac.21398
Blackett, K. L., Siddhi, S. S., Cleary, S., Steed, H., Miller, M. H., Macfarlane, S., et al. (2013). Oesophageal Bacterial Biofilm Changes in Gastro-Oesophageal Reflux Disease, Barrett's and Oesophageal Carcinoma: Association or Causality? Aliment. Pharmacol. Ther. 37 (11), 1084–1092. Epub 2013/04/23PubMed PMID: 23600758. doi:10.1111/apt.12317
Bowden, J., Davey Smith, G., and Burgess, S. (2015). Mendelian Randomization with Invalid Instruments: Effect Estimation and Bias Detection through Egger Regression. Int. J. Epidemiol. 44 (2), 512–525. Epub 2015/06/08PubMed PMID: 26050253; PubMed Central PMCID: PMCPMC4469799. doi:10.1093/ije/dyv080
Bowden, J., Davey Smith, G., Haycock, P. C., and Burgess, S. (2016). Consistent Estimation in Mendelian Randomization with Some Invalid Instruments Using a Weighted Median Estimator. Genet. Epidemiol. 40 (4), 304–314. Epub 2016/04/12PubMed PMID: 27061298; PubMed Central PMCID: PMCPMC4849733. doi:10.1002/gepi.21965
Bowden, J., Del Greco, M. F., Minelli, C., Davey Smith, G., Sheehan, N., and Thompson, J. (2017). A Framework for the Investigation of Pleiotropy in Two-Sample Summary Data Mendelian Randomization. Stat. Med. 36 (11), 1783–1802. Epub 2017/01/24PubMed PMID: 28114746; PubMed Central PMCID: PMCPMC5434863. doi:10.1002/sim.7221
Bowden, J., Del Greco, M. F., Minelli, C., Davey Smith, G., Sheehan, N. A., and Thompson, J. R. (2016). Assessing the Suitability of Summary Data for Two-Sample Mendelian Randomization Analyses Using MR-Egger Regression: the Role of the I2 Statistic. Int. J. Epidemiol. 45 (6), 1961–1974. Epub 2016/09/13PubMed PMID: 27616674; PubMed Central PMCID: PMCPMC5446088. doi:10.1093/ije/dyw220
Brion, M. J., Shakhbazov, K., and Visscher, P. M. (2013). Calculating Statistical Power in Mendelian Randomization Studies. Int. J. Epidemiol. 42 (5), 1497–1501. Epub 2013/10/26PubMed PMID: 24159078; PubMed Central PMCID: PMCPMC3807619. doi:10.1093/ije/dyt179
Burgess, S., Davies, N. M., and Thompson, S. G. (2016). Bias Due to Participant Overlap in Two-Sample Mendelian Randomization. Genet. Epidemiol. 40 (7), 597–608. Epub 2016/10/19PubMed PMID: 27625185; PubMed Central PMCID: PMCPMC5082560. doi:10.1002/gepi.21998
Burgess, S., and Thompson, S. G. (2017). Interpreting Findings from Mendelian Randomization Using the MR-Egger Method. Eur. J. Epidemiol. 32 (5), 377–389. Epub 2017/05/21PubMed PMID: 28527048; PubMed Central PMCID: PMCPMC5506233. doi:10.1007/s10654-017-0255-x
Cook, M. B., Shaheen, N. J., Anderson, L. A., Giffen, C., Chow, W. H., Vaughan, T. L., et al. (2012). Cigarette Smoking Increases Risk of Barrett's Esophagus: an Analysis of the Barrett's and Esophageal Adenocarcinoma Consortium. Gastroenterology 142 (4), 744–753. Epub 2012/01/17PubMed PMID: 22245667; PubMed Central PMCID: PMCPMC3321098. doi:10.1053/j.gastro.2011.12.049
Dong, L., Yin, J., Zhao, J., Ma, S. R., Wang, H. R., Wang, M., et al. (2018). Microbial Similarity and Preference for Specific Sites in Healthy Oral Cavity and Esophagus. Front. Microbiol. 9, 1603. Epub 2018/08/02PubMed PMID: 30065718; PubMed Central PMCID: PMCPMC6056649. doi:10.3389/fmicb.2018.01603
Elliott, D. R. F., Walker, A. W., O'Donovan, M., Parkhill, J., and Fitzgerald, R. C. (2017). A Non-endoscopic Device to Sample the Oesophageal Microbiota: a Case-Control Study. Lancet Gastroenterol. Hepatol. 2 (1), 32–42. Epub 2017/04/14PubMed PMID: 28404012; PubMed Central PMCID: PMCPMC5656094. doi:10.1016/S2468-1253(16)30086-3
Gall, A., Fero, J., McCoy, C., Claywell, B. C., Sanchez, C. A., Blount, P. L., et al. (2015). Bacterial Composition of the Human Upper Gastrointestinal Tract Microbiome Is Dynamic and Associated with Genomic Instability in a Barrett's Esophagus Cohort. PLoS One 10 (6), e0129055. Epub 2015/06/16PubMed PMID: 26076489; PubMed Central PMCID: PMCPMC4468150. doi:10.1371/journal.pone.0129055
Gharahkhani, P., Fitzgerald, R. C., Vaughan, T. L., Palles, C., Gockel, I., Tomlinson, I., et al. (2016). Genome-wide Association Studies in Oesophageal Adenocarcinoma and Barrett's Oesophagus: a Large-Scale Meta-Analysis. Lancet Oncol. 17 (10), 1363–1373. Epub 2016/08/17PubMed PMID: 27527254; PubMed Central PMCID: PMCPMC5052458. doi:10.1016/S1470-2045(16)30240-6
Gillespie, M. R., Rai, V., Agrawal, S., and Nandipati, K. C. (2021). The Role of Microbiota in the Pathogenesis of Esophageal Adenocarcinoma. Biol. (Basel) 10 (8). Epub 2021/08/28PubMed PMID: 34439930; PubMed Central PMCID: PMCPMC8389269. doi:10.3390/biology10080697
Glymour, M. M., Tchetgen Tchetgen, E. J., and Robins, J. M. (2012). Credible Mendelian Randomization Studies: Approaches for Evaluating the Instrumental Variable Assumptions. Am. J. Epidemiol. 175 (4), 332–339. Epub 2012/01/17PubMed PMID: 22247045; PubMed Central PMCID: PMCPMC3366596. doi:10.1093/aje/kwr323
Hemani, G., Zheng, J., Elsworth, B., Wade, K. H., Haberland, V., Baird, D., et al. (2018). The MR-Base Platform Supports Systematic Causal Inference across the Human Phenome. Elife 7. Epub 2018/05/31PubMed PMID: 29846171; PubMed Central PMCID: PMCPMC5976434. doi:10.7554/eLife.34408
Hills, R. D., Pontefract, B. A., Mishcon, H. R., Black, C. A., Sutton, S. C., and Theberge, C. R. (2019). Gut Microbiome: Profound Implications for Diet and Disease. Nutrients 11 (7). Epub 2019/07/19PubMed PMID: 31315227; PubMed Central PMCID: PMCPMC6682904. doi:10.3390/nu11071613
Howard, D. M., Adams, M. J., Clarke, T. K., Hafferty, J. D., Gibson, J., Shirali, M., et al. (2019). Genome-wide Meta-Analysis of Depression Identifies 102 Independent Variants and Highlights the Importance of the Prefrontal Brain Regions. Nat. Neurosci. 22 (3), 343–352. Epub 2019/02/06PubMed PMID: 30718901; PubMed Central PMCID: PMCPMC6522363. doi:10.1038/s41593-018-0326-7
Hughes, D. A., Bacigalupe, R., Wang, J., Ruhlemann, M. C., Tito, R. Y., Falony, G., et al. (2020). Genome-wide Associations of Human Gut Microbiome Variation and Implications for Causal Inference Analyses. Nat. Microbiol. 5 (9), 1079–1087. Epub 2020/06/24PubMed PMID: 32572223; PubMed Central PMCID: PMCPMC7610462. doi:10.1038/s41564-020-0743-8
IARC Working Group on the Evaluation of Carcinogenic Risks to Humans (2012). Biological Agents Volume 100 B A Review of Human Carcinogens. IARC Monogr. Eval. Carcinog. Risks Hum. 100 (Pt B), 1–441. Epub 2012/11/30. PubMed PMID: 23189750; PubMed Central PMCID: PMCPMC4781184.
Iida, N., Dzutsev, A., Stewart, C. A., Smith, L., Bouladoux, N., Weingarten, R. A., et al. (2013). Commensal Bacteria Control Cancer Response to Therapy by Modulating the Tumor Microenvironment. Science 342 (6161), 967–970. Epub 2013/11/23PubMed PMID: 24264989; PubMed Central PMCID: PMCPMC6709532. doi:10.1126/science.1240527
Jin, Y., Dong, H., Xia, L., Yang, Y., Zhu, Y., Shen, Y., et al. (2019). The Diversity of Gut Microbiome Is Associated with Favorable Responses to Anti-programmed Death 1 Immunotherapy in Chinese Patients with NSCLC. J. Thorac. Oncol. 14 (8), 1378–1389. Epub 2019/04/27PubMed PMID: 31026576. doi:10.1016/j.jtho.2019.04.007
Kamat, M. A., Blackshaw, J. A., Young, R., Surendran, P., Burgess, S., Danesh, J., et al. (2019). PhenoScanner V2: an Expanded Tool for Searching Human Genotype-Phenotype Associations. Bioinformatics. Epub 2019/06/25PubMed PMID: 31233103. doi:10.1093/bioinformatics/btz469
Kononen, E., and Wade, W. G. (2015). Actinomyces and Related Organisms in Human Infections. Clin. Microbiol. Rev. 28 (2), 419–442. doi:10.1128/CMR.00100-14
Kurilshikov, A., Medina-Gomez, C., Bacigalupe, R., Radjabzadeh, D., Wang, J., Demirkan, A., et al. (2021). Large-scale Association Analyses Identify Host Factors Influencing Human Gut Microbiome Composition. Nat. Genet. 53 (2), 156–165. Epub 2021/01/20PubMed PMID: 33462485. doi:10.1038/s41588-020-00763-1
Kurilshikov, A., Wijmenga, C., Fu, J., and Zhernakova, A. (2017). Host Genetics and Gut Microbiome: Challenges and Perspectives. Trends Immunol. 38 (9), 633–647. Epub 2017/07/04PubMed PMID: 28669638. doi:10.1016/j.it.2017.06.003
Lawlor, D. A., Harbord, R. M., Sterne, J. A., Timpson, N., and Davey Smith, G. (2008). Mendelian Randomization: Using Genes as Instruments for Making Causal Inferences in Epidemiology. Stat. Med. 27 (8), 1133–1163. Epub 2007/09/22PubMed PMID: 17886233. doi:10.1002/sim.3034
Lee, J. J., Wedow, R., Okbay, A., Kong, E., Maghzian, O., Zacher, M., et al. (2018). Gene Discovery and Polygenic Prediction from a Genome-wide Association Study of Educational Attainment in 1.1 Million Individuals. Nat. Genet. 50 (8), 1112–1121. Epub 2018/07/25PubMed PMID: 30038396; PubMed Central PMCID: PMCPMC6393768. doi:10.1038/s41588-018-0147-3
Lee, S. H., Goddard, M. E., Wray, N. R., and Visscher, P. M. (2012). A Better Coefficient of Determination for Genetic Profile Analysis. Genet. Epidemiol. 36 (3), 214–224. Epub 2012/06/21PubMed PMID: 22714935. doi:10.1002/gepi.21614
Levy, M., Kolodziejczyk, A. A., Thaiss, C. A., and Elinav, E. (2017). Dysbiosis and the Immune System. Nat. Rev. Immunol. 17 (4), 219–232. Epub 2017/03/07PubMed PMID: 28260787. doi:10.1038/nri.2017.7
Liu, M., Jiang, Y., Wedow, R., Li, Y., Brazel, D. M., Chen, F., et al. (2019). Association Studies of up to 1.2 Million Individuals Yield New Insights into the Genetic Etiology of Tobacco and Alcohol Use. Nat. Genet. 51 (2), 237–244. Epub 2019/01/16PubMed PMID: 30643251; PubMed Central PMCID: PMCPMC6358542. doi:10.1038/s41588-018-0307-5
Lopetuso, L. R., Severgnini, M., Pecere, S., Ponziani, F. R., Boskoski, I., Larghi, A., et al. (2020). Esophageal Microbiome Signature in Patients with Barrett's Esophagus and Esophageal Adenocarcinoma. PLoS One 15 (5), e0231789. Epub 2020/05/06PubMed PMID: 32369505; PubMed Central PMCID: PMCPMC7199943. doi:10.1371/journal.pone.0231789
Lopez, P. M., Subramanian, S. V., and Schooling, C. M. (2019). Effect Measure Modification Conceptualized Using Selection Diagrams as Mediation by Mechanisms of Varying Population-Level Relevance. J. Clin. Epidemiol. 113, 123–128. Epub 2019/05/24PubMed PMID: 31121303. doi:10.1016/j.jclinepi.2019.05.005
Manolio, T. A. (2010). Genomewide Association Studies and Assessment of the Risk of Disease. N. Engl. J. Med. 363 (2), 166–176. Epub 2010/07/22PubMed PMID: 20647212. doi:10.1056/NEJMra0905980
Miquel, S., Martin, R., Rossi, O., Bermudez-Humaran, L. G., Chatel, J. M., Sokol, H., et al. (2013). Faecalibacterium Prausnitzii and Human Intestinal Health. Curr. Opin. Microbiol. 16 (3), 255–261. Epub 2013/07/09PubMed PMID: 23831042. doi:10.1016/j.mib.2013.06.003
Moschen, A. R., Gerner, R. R., Wang, J., Klepsch, V., Adolph, T. E., Reider, S. J., et al. (2016). Lipocalin 2 Protects from Inflammation and Tumorigenesis Associated with Gut Microbiota Alterations. Cell Host Microbe 19 (4), 455–469. Epub 2016/04/15PubMed PMID: 27078067. doi:10.1016/j.chom.2016.03.007
Munch, N. S., Fang, H. Y., Ingermann, J., Maurer, H. C., Anand, A., Kellner, V., et al. (2019). High-fat Diet Accelerates Carcinogenesis in a Mouse Model of Barrett's Esophagus via Interleukin 8 and Alterations to the Gut Microbiome. Gastroenterology 157 (2), 492–506. e2Epub 2019/04/19PubMed PMID: 30998992; PubMed Central PMCID: PMCPMC6662596. doi:10.1053/j.gastro.2019.04.013
Ong, J. S., An, J., Han, X., Law, M. H., Nandakumar, P., Schumacher, J., et al. (2021). Multitrait Genetic Association Analysis Identifies 50 New Risk Loci for Gastro-Oesophageal Reflux, Seven New Loci for Barrett's Oesophagus and Provides Insights into Clinical Heterogeneity in Reflux Diagnosis. Gut. Epub 2021/07/01PubMed PMID: 34187846. doi:10.1136/gutjnl-2020-323906
Parker, B. J., Wearsch, P. A., Veloo, A. C. M., and Rodriguez-Palacios, A. (2020). The Genus Alistipes: Gut Bacteria with Emerging Implications to Inflammation, Cancer, and Mental Health. Front. Immunol. 11, 906. Epub 2020/06/26PubMed PMID: 32582143; PubMed Central PMCID: PMCPMC7296073. doi:10.3389/fimmu.2020.00906
Pei, Z., Bini, E. J., Yang, L., Zhou, M., Francois, F., and Blaser, M. J. (2004). Bacterial Biota in the Human Distal Esophagus. Proc. Natl. Acad. Sci. U. S. A. 101 (12), 4250–4255. Epub 2004/03/16PubMed PMID: 15016918; PubMed Central PMCID: PMCPMC384727. doi:10.1073/pnas.0306398101
Peter, S., Pendergraft, A., VanDerPol, W., Wilcox, C. M., Kyanam Kabir Baig, K. R., Morrow, C., et al. (2020). Mucosa-Associated Microbiota in Barrett's Esophagus, Dysplasia, and Esophageal Adenocarcinoma Differ Similarly Compared with Healthy Controls. Clin. Transl. Gastroenterol. 11 (8), e00199. Epub 2020/09/22PubMed PMID: 32955191; PubMed Central PMCID: PMCPMC7473866. doi:10.14309/ctg.0000000000000199
Pulit, S. L., Stoneman, C., Morris, A. P., Wood, A. R., Glastonbury, C. A., Tyrrell, J., et al. (2019). Meta-analysis of Genome-wide Association Studies for Body Fat Distribution in 694 649 Individuals of European Ancestry. Hum. Mol. Genet. 28 (1), 166–174. Epub 2018/09/22PubMed PMID: 30239722; PubMed Central PMCID: PMCPMC6298238. doi:10.1093/hmg/ddy327
Rau, M., Rehman, A., Dittrich, M., Groen, A. K., Hermanns, H. M., Seyfried, F., et al. (2018). Fecal SCFAs and SCFA-Producing Bacteria in Gut Microbiome of Human NAFLD as a Putative Link to Systemic T-Cell Activation and Advanced Disease. United Eur. Gastroenterol. J. 6 (10), 1496–1507. Epub 2018/12/24. doi:10.1177/2050640618804444
Rothschild, D., Weissbrod, O., Barkan, E., Kurilshikov, A., Korem, T., Zeevi, D., et al. (2018). Environment Dominates over Host Genetics in Shaping Human Gut Microbiota. Nature 555 (7695), 210–215. Epub 2018/03/01PubMed PMID: 29489753. doi:10.1038/nature25973
Schooling, C. M., Lopez, P. M., Yang, Z., Zhao, J. V., Au Yeung, S. L., and Huang, J. V. (2020). Use of Multivariable Mendelian Randomization to Address Biases Due to Competing Risk before Recruitment. Front. Genet. 11, 610852. Epub 2021/02/02PubMed PMID: 33519914; PubMed Central PMCID: PMCPMC7845663. doi:10.3389/fgene.2020.610852
Skrivankova, V. W., Richmond, R. C., Woolf, B. A. R., Yarmolinsky, J., Davies, N. M., Swanson, S. A., et al. (2021). Strengthening the Reporting of Observational Studies in Epidemiology Using Mendelian Randomization: The STROBE-MR Statement. JAMA 326 (16), 1614–1621. Epub 2021/10/27PubMed PMID: 34698778. doi:10.1001/jama.2021.18236
Smith, G. D., and Ebrahim, S. (2003). Mendelian Randomization': Can Genetic Epidemiology Contribute to Understanding Environmental Determinants of Disease? Int. J. Epidemiol. 32 (1), 1–22. Epub 2003/04/12PubMed PMID: 12689998. doi:10.1093/ije/dyg070
Snider, E. J., Compres, G., Freedberg, D. E., Khiabanian, H., Nobel, Y. R., Stump, S., et al. (2019). Alterations to the Esophageal Microbiome Associated with Progression from Barrett's Esophagus to Esophageal Adenocarcinoma. Cancer Epidemiol. Biomarkers Prev. 28 (10), 1687–1693. Epub 2019/08/31PubMed PMID: 31466948; PubMed Central PMCID: PMCPMC6774849. doi:10.1158/1055-9965.EPI-19-0008
Stenman, L. K., Burcelin, R., and Lahtinen, S. (2016). Establishing a Causal Link between Gut Microbes, Body Weight Gain and Glucose Metabolism in Humans - towards Treatment with Probiotics. Benef. Microbes 7 (1), 11–22. Epub 2015/11/14PubMed PMID: 26565087. doi:10.3920/BM2015.0069
Thrift, A. P., Shaheen, N. J., Gammon, M. D., Bernstein, L., Reid, B. J., Onstad, L., et al. (2014). Obesity and Risk of Esophageal Adenocarcinoma and Barrett's Esophagus: a Mendelian Randomization Study. J. Natl. Cancer Inst. 106 (11). Epub 2014/10/02PubMed PMID: 25269698; PubMed Central PMCID: PMCPMC4200028. doi:10.1093/jnci/dju252
Tozun, N., and Vardareli, E. (2016). Proceedings from the 8th Probiotics, Prebiotics & New Foods for Microbiota and Human Health Meeting Held in Rome. Gut Microbiome Gastrointest. Cancer Les liaisons Dangereuses J Clin GastroenterolItaly Sept. 13-15, S191–S6. Epub 2016/10/16PubMed PMID: 27741173. doi:10.1097/MCG.0000000000000714
Turnbaugh, P. J., Ley, R. E., Hamady, M., Fraser-Liggett, C. M., Knight, R., and Gordon, J. I. (2007). The Human Microbiome Project. Nature 449 (7164), 804–810. doi:10.1038/nature06244
Verbanck, M., Chen, C. Y., Neale, B., and Do, R. (2018). Detection of Widespread Horizontal Pleiotropy in Causal Relationships Inferred from Mendelian Randomization between Complex Traits and Diseases. Nat. Genet. 50 (5), 693–698. Epub 2018/04/25PubMed PMID: 29686387; PubMed Central PMCID: PMCPMC6083837. doi:10.1038/s41588-018-0099-7
Wright, E. K., Kamm, M. A., Teo, S. M., Inouye, M., Wagner, J., and Kirkwood, C. D. (2015). Recent Advances in Characterizing the Gastrointestinal Microbiome in Crohn's Disease: a Systematic Review. Inflamm. Bowel Dis. 21 (6), 1219–1228. Epub 2015/04/07PubMed PMID: 25844959; PubMed Central PMCID: PMCPMC4450900. doi:10.1097/MIB.0000000000000382
Yang, L., Lu, X., Nossa, C. W., Francois, F., Peek, R. M., and Pei, Z. (2009). Inflammation and Intestinal Metaplasia of the Distal Esophagus Are Associated with Alterations in the Microbiome. Gastroenterology 137 (2), 588–597. Epub 2009/04/28PubMed PMID: 19394334; PubMed Central PMCID: PMCPMC2963147. doi:10.1053/j.gastro.2009.04.046
Yang, Z., Schooling, C. M., and Kwok, M. K. (2021). Credible Mendelian Randomization Studies in the Presence of Selection Bias Using Control Exposures. Front. Genet. 12, 729326. Epub 2021/12/14PubMed PMID: 34899831; PubMed Central PMCID: PMCPMC8652250. doi:10.3389/fgene.2021.729326
Yarmolinsky, J., Bonilla, C., Haycock, P. C., Langdon, R. J. Q., Lotta, L. A., Langenberg, C., et al. (2018). Circulating Selenium and Prostate Cancer Risk: A Mendelian Randomization Analysis. J. Natl. Cancer Inst. 110 (9), 1035–1038. Epub 2018/05/23PubMed PMID: 29788239; PubMed Central PMCID: PMCPMC6136927. doi:10.1093/jnci/djy081
Zhao, Q., Wang, J., Hemani, G., Bowden, J., and Small, D. S. (2020). Statistical Inference in Two-Sample Summary-Data Mendelian Randomization Using Robust Adjusted Profile Score. Ann. Statistics 48 (3), 1742–1769. doi:10.1214/19-aos1866
Zhou, J., Shrestha, P., Qiu, Z., Harman, D. G., Teoh, W. C., Al-Sohaily, S., et al. (2020). Distinct Microbiota Dysbiosis in Patients with Non-erosive Reflux Disease and Esophageal Adenocarcinoma. J. Clin. Med. 9 (7). Epub 2020/07/12PubMed PMID: 32650561; PubMed Central PMCID: PMCPMC7408827. doi:10.3390/jcm9072162
Zilberstein, B., Quintanilha, A. G., Santos, M. A., Pajecki, D., Moura, E. G., Alves, P. R., et al. (2007). Digestive Tract Microbiota in Healthy Volunteers. Clin. (Sao Paulo) 62 (1), 47–54. Epub 2007/03/06PubMed PMID: 17334549. doi:10.1590/s1807-59322007000100008
Keywords: Barrett’s esophagus, causal estimates, gut microbiota, Mendelian randomization, MiBioGen
Citation: Yang Z, Yu R, Deng W and Wang W (2022) Roles of 21 Genera of Human Gut Microbiota in Barrett’s Esophagus Risk: A Mendelian Randomization Study. Front. Genet. 13:894900. doi: 10.3389/fgene.2022.894900
Received: 12 March 2022; Accepted: 25 April 2022;
Published: 09 June 2022.
Edited by:
Samuel Antwi, Mayo Clinic Florida, United StatesReviewed by:
Zhiyong Cui, Peking University Third Hospital, ChinaYaohua Yang, Vanderbilt University Medical Center, United States
Copyright © 2022 Yang, Yu, Deng and Wang. This is an open-access article distributed under the terms of the Creative Commons Attribution License (CC BY). The use, distribution or reproduction in other forums is permitted, provided the original author(s) and the copyright owner(s) are credited and that the original publication in this journal is cited, in accordance with accepted academic practice. No use, distribution or reproduction is permitted which does not comply with these terms.
*Correspondence: Weihu Wang, d2FuZ3dlaWh1ODhAMTYzLmNvbQ==
†These authors have contributed equally to the work