- Department of Geriatric Medicine, Xiangya Hospital, Central South University, Changsha, China
Background: Previous studies have indicated that the gut microbiota (GM) is associated with coronary artery disease (CAD), but the causality of these associations remains unestablished due to confounding factors and reverse causality. We conducted Mendelian randomization study (MR) to determine the causal effect of the specific bacterial taxa on CAD/myocardial infarction (MI) and identify the mediating factors involved.
Methods: Two-sample MR, multivariable MR (MVMR) and mediation analysis were performed. Inverse-variance weighting (IVW) was the main method used to analyze causality, and sensitivity analysis was used to verify the reliability of the study. Causal estimates from CARDIoGRAMplusC4D and FinnGen databases were combined using the meta-analysis method, and repeated validation was conducted based on the UK Biobank (UKB) database. Confounders that may affect the causal estimates were corrected by MVMP and the potential mediation effects were investigated by using mediation analysis.
Results: The study suggested that increased abundance of the RuminococcusUCG010 genus leads to a lower risk of CAD (OR, 0.88; 95% CI, 0.78, 1.00; p = 2.88 × 10−2) and MI (OR, 0.88; 95% CI, 0.79, 0.97; p = 1.08 × 10−2), with consistent results in both meta-analysis (CAD: OR, 0.86; 95% CI, 0.78, 0.96; p = 4.71 × 10−3; MI: OR, 0.82; 95% CI, 0.73, 0.92; p = 8.25 × 10−4) and repeated analysis of the UKB dataset (CAD: OR, 0.99; 95% CI, 0.99, 1.00, p = 2.53 × 10−4; MI: OR, 0.99; 95% CI, 0.99, 1.00, p = 1.85 × 10–11). Based on multiple databases, T2DM was proved as a mediating factor in the causal effect of RuminococcusUCG010 and CAD/MI, with an average mediation effect proportion of 20% on CAD and 17% on MI, respectively.
Conclusion: This MR study provided suggestive genetic evidence that the higher the RuminococcusUCG010 abundance is, the lower the risk of CAD and MI, with T2DM playing a mediating effect. This genus may become a novel target in strategies for treating and preventing CAD and MI.
1 Introduction
Cardiometabolic diseases refer to a clinical syndrome primarily caused by metabolic disorders, with atherosclerotic cardiovascular disease as the main manifestation, except for structural cardiovascular pathologies, arrhythmias and cardiomyopathies (Zhu, 2021). There is an explicit causal relationship between metabolic abnormalities and cardiovascular diseases. Intervention in metabolic disorders can effectively improve prognosis (Saxon et al., 2020). Coronary artery disease (CAD), the main type of cardiometabolic disease, is the leading cause of morbidity and mortality worldwide, accounting for approximately nine million deaths in 2017, and the prevalence of CAD is projected to increase to more than 1,845 cases per 100,000 individuals, with an upper confidence estimate of 1,917 cases per 100,000 individuals, by 2030 (Mozaffarian et al., 2015; Khan et al., 2020). Cardiometabolic risk factors, such as type 2 diabetes, dyslipidaemia, obesity, and insulin resistance, are related to an increased risk of CAD. In recent decades, treatment targeting these traditional risk factors has significantly reduced cardiovascular mortality. However, despite recent advances, residual risk of CVD remains (Kotseva et al., 2016; Kotseva et al., 2017). As the prevalence of CAD increases globally under the current control measures, efficient new strategies are necessary to target CAD and modifiable risk factors. The gastrointestinal tract is where nutrients the body requires are digested and absorbed and where the greatest number and diversity of microbiota are found; the gut microbiota (GM) is a key regulator of metabolism and exhibits relative stability and resilience over time (Rinninella et al., 2019; Afzaal et al., 2022). Accumulating evidence has revealed that the GM and metabolites are involved in human metabolism, immunity and inflammation processes and are involved in the development and progression of CAD (Kazemian et al., 2020; Novakovic et al., 2020; Xu et al., 2020).
Trimethylamine-N-oxide (TMAO) is an intestinal microbiota-dependent metabolite from dietary choline, betaine, and L-carnitine that is metabolized by trimethylamine lyases to form trimethylamine (TMA) and catalyzed by the action of flavin monooxygenases in the liver (Bennett et al., 2013). TMAO inhibits reverse cholesterol transport, which augments cholesterol accumulation within macrophages and promotes foam cell formation to form atherosclerotic plaques. In addition, TMAO has a proinflammatory effect (Yamashita et al., 2021). Studies have demonstrated a critical role of TMAO in pro-atherogenesis in germ-free mice (Wang et al., 2011). In several large independent clinical cohorts, plasma TMAO levels were shown to be associated with the risk of CAD morbidity and long-term mortality and thus predictors of cardiovascular risk (Tang et al., 2013; Wang et al., 2014). However, in the MR study, there is no direct causal relationship between TMAO and CAD, but a suggestive association of genetically increased choline with a higher risk of T2DM (Jia et al., 2019) and the same effect of TMAO and carnitine with systolic BP (Wang et al., 2022). Butyrate is a short-chain fatty acid (SCFA) produced by the metabolism of intestinal microflora, which has been proved to be a protective metabolite for CAD in contrast to TAMO (Hu et al., 2022). However, there is a lack of MR studies. In a comparative study of CAD patients and controls, patients exhibited characteristic changes in the composition and abundance of the GM. A relative reduction in Bacteroides and Prevotella and enrichment in Streptococcus, Escherichia, and Collinsella were observed in patients (Karlsson et al., 2012; Jie et al., 2017). Stephan J’s study confirmed the presence of bacteria in atherosclerotic lesions in 2006 with a high overall bacterial diversity of more than 50 different species (Ott et al., 2006). Later, in Omry Koren’s and Ju Seung Kwun’s study, the bacterial taxa isolated from atherosclerotic plaques were found to exist in the gut of the same individuals and the amount of bacterial DNA correlated with a amount of leukocytes in the atherosclerotic plaque, which indicates that the amount of bacteria contributes to the inflammatory status of the atherosclerotic plaque (Koren et al., 2011; Kwun et al., 2020). These observations suggest that the bacteria in plaques are homologous to the GM. Therefore, the GM potentially promotes plaque formation. Based on these findings, more research has been performed to investigate the mechanisms and potential therapeutic targets of the GM in CAD. Jill Gregory et al. found that atherosclerosis susceptibility could be transferred to ApoE null mice through transplantation of a high/low TMAO-producing strain of the GM (Gregory et al., 2015). A novel Bowman-Birk type major trypsin inhibitor (FMB-BBTI) was shown to markedly restrain atherosclerosis progression by remodeling the structure of the GM, as verified in a mouse model (Shan et al., 2022). In a randomized controlled study of 44 CAD patients, probiotic supplementation (Lactobacillus rhamnosus GG, LGG) had beneficial effects on metabolic endotoxemia, and mega inflammation in patients. The levels of biomarkers of inflammation and metabolic endotoxemia, such as IL-1 beta and hs-CRP, decreased significantly in the LGG group compared to the placebo group (Moludi et al., 2021). Increasing evidence indicates that the GM may represent a novel therapeutic target for CAD, and GM-based CAD therapies include gut flora transplantation, probiotic supplementation of specific taxa, and other modulation of the GM (Warmbrunn et al., 2020).
However, current studies on the correlation between the GM and CAD still have limitations. Small study samples may lead to biased results and underestimate some important predictors. Whether the mutual association is causal remains undetermined according to traditional epidemiological studies due to residual confounding factors and reverse causation bias. In addition, randomized controlled trials, as the gold standard for inferring a causal association, have ethical restrictions and are impractical. Mendelian randomization (MR), however, is a method for assessing causal associations by using genetic variants as instrumental variables (IVs). MR can prevent the possible effects of residual confounding and reverse causality because genetic variants are assorted randomly and defined at conception (Smith and Ebrahim, 2003; Zoccali et al., 2006; Verduijn et al., 2010). Therefore, we conducted a two-sample MR study to explore the causal associations of the GM with CAD/myocardial infarction (MI) and identify specific causal bacterial taxa as potential prevention and treatment targets. Confounding factors that may affect the causal estimates were corrected by multivariable MR (MVMR) and the potential mediation effects were investigated by using mediation analysis.
2 Materials and methods
2.1 Study design
MR is used to identify and estimate a causal effect using genetic instrumental variables. Two-sample MR assesses two independent populations (same ethnicity) using single nucleotide polymorphisms (SNPs) as IVs (Lawlor, 2016). It is generally not associated with confounders of conventional observational studies, such as postnatal environmental factors, socioeconomic status, behavior and reverse causality, because the genotypes of germline genetic variation are defined at conception (Smith and Ebrahim, 2003; Smith et al., 2007). The MR approach follows Mendel’s law of inheritance, which is considered analogous to randomized controlled trials. There are three critical assumptions of MR analysis (Figure 1). First, the relevance assumption is that IVs are robustly associated with exposure. Second, the independence assumption is that IVs are absolutely independent of any confounders that may affect the exposure and outcomes. Third, the exclusion restriction assumption is that the association between the IVs and outcomes is exclusively dominated by exposure instead of alternative mechanisms (Emdin et al., 2017). In this two-sample MR study, we made use of publicly accessible datasets from large-sample genome-wide association studies (GWASs) for both GM (as a risk factor) and CAD/MI (as a disease outcome) to estimate the causal inferences. Sensitivity analysis was used to verify the reliability of the causal association results. As a protective metabolite of CAD/MI, butyrate may play a potential mediating effect between GM and CAD/MI. Therefore, we conducted synchronously MR studies of butyrate and CAD/MI. MVMR is an extension of univariate MR, which takes into account the pleiotropy of genetic variants that the same genetic variation may dominate multiple phenotypes (Burgess and Thompson, 2015). The causal effect of exposure on outcomes was direct or indirect depending on the role of confounding factors that as a risk for outcome may have overlapping effect with exposure. MVMP can be used to verify and correct the confounders. Mediation analysis is based on the framework of two-sample MR to assess the mediating effect of significant confounders in the causal association that exposure X leads to a change in mediator Z, which in turn results in a change in outcome Y (Figure 2) (Burgess et al., 2014). Our MR study followed the MR-STROBE guidance to strengthen the Reporting of Observational Studies in Epidemiology using MR (Skrivankova et al., 2021).
2.2 Data sources
In the two-sample MR, the SNPs that were used as IVs were extracted from the MiBioGen consortium, the largest and latest GM-GWAS, involving 18,473 participants in 25 cohorts dominated by European descent. The GWAS generated 122,110 variant sites from 211 taxa at 5 levels (from genus to phylum) (Kurilshikov et al., 2021). We obtained summary-level data for CAD/MI from the CARDIoGRAMplusC4D and FinnGen databases. The CARDIoGRAMplusC4D 1000 Genomes-based GWAS is a meta-analysis of CAD-GWASs involving 60,801 CAD (43,676 MI cases) cases and 123,504 controls, nearly 77% individuals with European ancestry (Nikpay et al., 2015). The FinnGen consortium is a large genomic database of half a million Finns, established in 2017 (https://www.finngen.fi/en), including 25,707 CAD cases (defined by the International Classification of Diseases (ICD)−8, code 410 or 4110; ICD−9, code 410 or 4110 and ICD−10, code I20, I21 or I22) and 15,787 MI cases (defined by ICD−8, code 410, ICD−9, code 410 and ICD−10, code I21 or I22) (FinnGen, 2022). Additionally, we validated the analyses using UK Biobank (UKB) database, a general population prospective cohort study recruiting approximately 500,000 participants from the United Kingdom. The purpose of that study was to assess the genetic and lifestyle-related risk factors for various chronic diseases, and the database included 10,157 CAD cases and 7,018 MI cases (Sudlow et al., 2015). Plasma butyrate levels are challenging to measure in current assays, so it is difficult to obtain the direct genetic estimation of butyrate in a large population cohort. PWY-502 is a microbial pathway involved in 4-aminobutanoate (GABA) degradation, the abundance of which was used as a proxy for butyrate production in the intestine. The summary-level data of PWY-502 is based on the Life Lines DEEP (LL-DEEP) queue, that is a subset of the largest Lifelines biobank consisting of 1,539 individuals from Northern Netherlands. (Bonder et al., 2016; Sanna et al., 2019). In the MVMP and Mediation analysis, the summary-level data of type 2 diabetes (T2DM) were extracted from FinnGen and a meta-analysis of three GWAS data: Diabetes Genetics Replication and Meta-analysis (DIAGRAM), Genetic Epidemiology Research on Aging (GERA) and UKB (Xue et al., 2018). Essential hypertension was obtained from FinnGen and UKB. Global Lipid Genetics Consortium (GLGC) as a source of genetic data for Low-density lipoprotein cholesterol (LDL-C), involved in 188,578 European-ancestry individuals (Willer et al., 2013).
2.3 Genetic instrumental variables
In our study, SNPs associated with the GM at 5 taxonomic levels (phylum, class, order, family, genus, and species) were screened from the MiBioGen study as IVs. A range of filtering criteria were used to select qualified IVs. First, SNPs associated with the GM were extracted at a p-value less than the locus-wide significance level (1 × 10−5). Second, Linkage disequilibrium (LD) refers to the non-random association of alleles at different loci that means the probability of two alleles located on the same chromosome simultaneously is greater than the probability of random distribution in the population. To ensure the independence of each SNP from distinct bacterial taxa, the clumping process was performed with reference to the European samples from the 1000 Genomes Project, with an R2 < 0.001 and a window size = 10,000 k. (Sved and Hill, 2018). Third, SNPs with a minor allele frequency (MAF) less than 0.01 were removed to avoid statistical bias. Fourth, the SNPs located on sex chromosome 23 were removed. Fifth, SNPs associated with potential confounders were excluded across PhenoScanner V2 (http://www.phenoscanner.medschl.cam.ac.uk/) to ensure the second core assumption of MR. Sixth, if selected SNPs were not available in the outcome dataset, proxy SNPs with a high linkage disequilibrium (LD) (r2>0.80) were identified by using SNiPa (http://snipa.helmholtz muenchen. de/snipa3/) as an alternative. Seventh, the MR Steiger filtering test was conducted to identify the third key hypothesis. This approach examined whether exposure is directional to the causality of the outcome and eliminated SNPs with reverse causality. Eighth, the F statistic was defined as
2.4 Statistical analysis
2.4.1 Two-sample MR
Inverse-variance weighting (IVW), MR Egger, weighted median estimator (WME), and simple median analyses were performed to infer the causal inferences. IVW was the main analysis method, and the intercept was constrained to zero. The meta-analysis of IVW combined the Wald estimates for each SNP (Burgess et al., 2013). The point estimates of IVW were equivalent to a weighted linear regression of SNP-outcome associations on SNP-exposure associations (Bowden et al., 2017). Fixed and random effects IVW approaches were used. MR‒Egger analysis was performed by a simple modification to the weighted linear regression of IVW. MR‒Egger analysis differs from IVW in that it does not set the intercept term to zero, and the term is estimated to assess pleiotropy as part of the analysis (Bowden et al., 2015). If the intercept term is close to zero or not statistically significant, then the slope term in MR‒Egger regression represents the estimated causal effect of exposure on the outcome, which is close to the IVW estimate; otherwise, it indicates the existence of horizontal pleiotropy. However, in contrast to the traditional methods for estimating the causal effects of MR, MR‒Egger analysis violates the InSIDE hypothesis that the pleiotropic effects of the genetic variants in the analysis are uncorrelated with the associations of the variants with the risk factor, which have been shown to lead to increased bias and type 1 error rate inflation (Burgess and Thompson., 2017; Lin et al., 2022). Moreover, the results of MR‒Egger regression are susceptible to outlying variants, which can lead to reversal of the horizontal pleiotropy test and causal effect estimates (Burgess and Thompson., 2017). The simple median estimator is the median ratio estimate, which is equivalent to a weighted median estimator with equal weights. When less than 50% of the genetic variants are invalid, the simple median can provide a consistent estimate of the causal effect (Han, 2008). The WME estimate is the median of a weighted empirical density function of the ratio estimates, which can provide a consistent estimation of the causal effect even when more than 50% of the weight comes from invalid IVs. WME is more accurate than the simple median when individual estimates differ greatly in accuracy (Bowden et al., 2016).
The sensitivity analysis in this study was conducted in three aspects. We tested for multiplicity of validity by MR Egger and Mendelian randomized multiplicity residuals and outliers (MR-PRESSO). As described above, when the MR Egger intercept term was not close to zero or statistically significant, it indicates the presence of horizontal multiplicity (Bowden et al., 2015). When the proportion of multiplicity IVs is less than 50%, MR-PRESSO can be used as an effective method for identifying horizontal multiplicity outliers in MR analyses and contains three main functions. First, the MR-PRESSO global test is used to test for the presence of horizontal pleiotropy. Second, the MR-PRESSO outlier test is used to identify and remove pleiotropic outliers and recalculate relatively unbiased estimates. Third, the MR-PRESSO distortion test provides significant differences in the causal estimates before and after correction for outliers (Verbanck et al., 2018). The heterogeneity of the SNPs included was tested by Cochran’s Q test and I2 statistics in the IVW model. Cochran’s Q test can be used to test the heterogeneity of each independent study. If the results were statistically significant, significant heterogeneity was demonstrated. The I2 statistic is calculated as I2 = [(Q–df)/Q] x 100%, reflecting the proportion of the heterogeneous component in the total variance (Higgins and Thompson., 2002). Mild heterogeneity was considered when the I2 statistic ranged from 0% to 25%, moderate heterogeneity when it ranged from 25% to 50%, and high heterogeneity when it was greater than 50%. The leave-one-out sensitivity test identifies strongly influential SNPs by removing individual SNPs one by one to re-estimate the total effect with IVW and determine the degree of influence of single SNPs on the association estimates by the stability of the effect estimates. All statistical analyses were conducted in R version 4.0.5 using the Two-Sample MR (https://github.com/MRCIEU/TwoSampleMR), MR-PRESSO (https://github.com/rondolab/MR-PRESSO), Forestplot (https://rdrr.io/cran/forestplot/f/vignettes/forestplot.Rmd). Power calculations were performed by the online tool mRnd (https://shiny.cnsgenomics.com/mRnd/) with the minimum effect >80% power. Sample size, Type-I error rate, proportion of cases, the odds ratios (ORs) and R2 were included in the calculation as the main parameters. In multiple testing, Bonferroni-corrected p values of <0.05/2 were considered statistically significant.
2.4.2 Multivariable MR
Based on the two-sample MR, confounders with the same causal effect as GM were included in the MVMR. IVs extracted in MVMP is a combination of multiple exposures associated with at least one of the GM and confounders. IVW was also the main analysis method. The analysis was conducted using the packages Two-Sample MR and Mendelian Randomization (https://cran.r-project.org/package=MendelianRandomization) in R.
2.4.3 Mediation analysis
The mediating effect is calculated as
3 Results
3.1 The two-sample MR
The MR-STROBE guidance is shown in Supplementary Table S1. Table 1 provides details of the studies and samples used in the MR analyses. The majority of all study participants were adults of European ancestry. Bacterial taxa were analyzed in the MR study at five levels, including 9 phyla, 16 classes, 20 orders, 35 families and 131 genera. A distinct taxon was defined as a feature. Detailed information is shown in Supplementary Table S2. RuminococcusUCG010 was the only genus that maintained consistent positive results in multiple databases and meta-analyses (Table 2; Figure 3). Twenty SNPs closely related to the genus RuminococcusUCG010 were screened from the MBG. database (p < 1 × 10−5). Twelve no independent SNPs (“rs34668774", "rs686403", "rs10281901", "rs591790", "rs686246", "rs592858", "rs8056635", "rs73218806", "rs886017", "rs2773827", "rs3088274", "rs7661237") were removed from the LD test (r2<0.001, Kb = 10,000), and 2 palindromic SNPs (rs35506912, rs7935775) were removed in the harmonization process. The remaining 6 SNPs were all present in the outcome database as IVs. These six SNPs were not found to be associated with other confounders in the PhenoScanner, and all had MAFs greater than 0.01 and F values well above 10. Supplementary Table S3 provides information about the SNPs, including chromosome, effect allele, non-effect allele, effect allele frequency, etc. The results of the MR Steiger filtering test are presented in Supplementary Table S4. The six IVs explained more genetic variation in the GM than CAD or MI, indicating causality in the expected direction. These SNPs could explain 10.06% of the phenotypic variance in the genus RuminococcusUCG010 (Supplementary Table S5).
The MR estimates from different methods of assessing the causal effect of RuminococcusUCG010 with CAD/MI are shown in Table 2. In this study, according to IVW as the primary causal assessment method, the risk of CAD or MI was reduced by 12% when the abundance of RuminococcusUCG010 doubled the standard deviation in the random effect IVW model based on the CARDIoGRAMplusC4D dataset (CAD: OR, 0.88; 95% CI, 0.78, 1.00; p = 2.88 × 10−2; MI: OR, 0.88; 95% CI, 0.79, 0.97; p = 1.08 × 10−2). All analyses based on the FinnGen dataset were positive, except for MR‒Egger analysis. The meta-analysis of CARDIoGRAMPlusC4D and FinnGen data maintained consistent protection (CAD: OR, 0.86; 95% CI, 0.78, 0.96; p = 4.71 × 10−3; MI: OR, 0.82; 95% CI, 0.73, 0.92; p = 8.25 × 10−4; Figure 3). To further verify the consistency of the association of the abundance of RuminococcusUCG010 with CAD or MI, the analysis was repeated based on the UKB. The above causal effects persisted in the MR analysis (CAD: random-effects IVW model: OR, 0.99; 95% CI, 0.99, 1.00, p = 2.53 × 10−4; Weighted median: OR, 0.99; 95% CI, 0.99, 1.00, p = 3.97 × 10−2; Simple median analysis: OR, 0.99; 95% CI, 0.99, 1.00, p = 3.81 × 10−2; MI: Fixed-effects IVW model: OR, 0.99; 95% CI, 0.99, 1.00, p = 5.63 × 10−3; random-effects IVW model: OR, 0.99; 95% CI, 0.99, 1.00, p = 1.85 × 10–11). Nevertheless, caution is needed here, as several associations did not reach the Bonferroni-corrected p-value of 0.0125. The scatter plots for each outcome were shown in Figure 4.
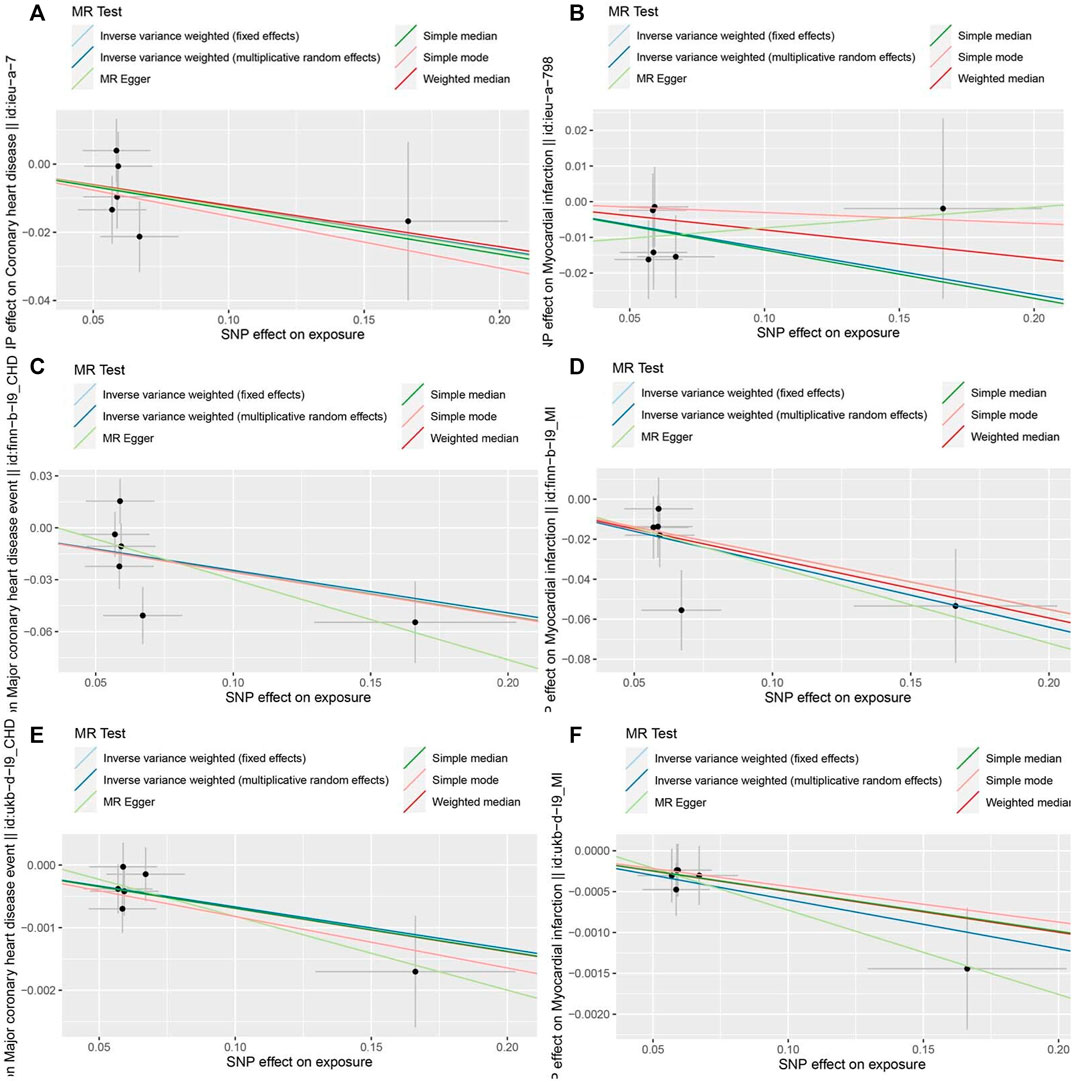
FIGURE 4. Scatter plots of the MR estimates for the association of RuminococcusUCG010 on CAD/MI. (A). RuminococcusUCG010-CAD based on CARDIoGRAMplusC4D. (B). RuminococcusUCG010-MI based on CARDIoGRAMplusC4D. (C). RuminococcusUCG010-CAD based on FinnGen. (D). RuminococcusUCG010-MI based on FinnGen. (E). RuminococcusUCG010-CAD based on UKB. (F). RuminococcusUCG010-MI based on UKB.
In the Cochran Q and I2 tests, there was a certain heterogeneity in the SNPs associated with the GM and FinnGen (P Cochran’s Q = 0.05, I2 = 54%), with no heterogeneity in the rest (Table 3). There was no horizontal pleiotropy detected in either the MR‒Egger intercept P or MR-PRESSO global test P tests (P intercept >0.0125; P global test >0.0125, Table 3). In addition, no outlier SNP to be corrected was found in the MR−PRESSO outlier test, and no potential SNP driving causality was found in the leave-one-out analysis (Figure 5). This study had over 80% statistical power for detecting the associations between the GM and CAD/MI based on the CARDIoGRAMplusC4D and FinnGen studies, respectively (Supplementary Table S5). However, the statistical power based on the UKB was restrictive, because of the shortage of CAD/MI cases or the deficiency variance in the GM explained by the selected IVs.
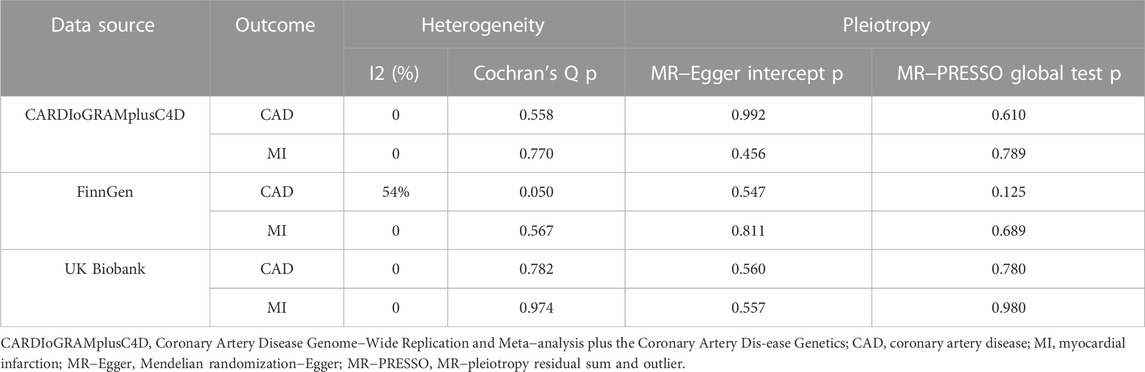
TABLE 3. Evaluation of heterogeneity and directional pleiotropy using different methods on RuminococcusUCG010 with CAD/MI.
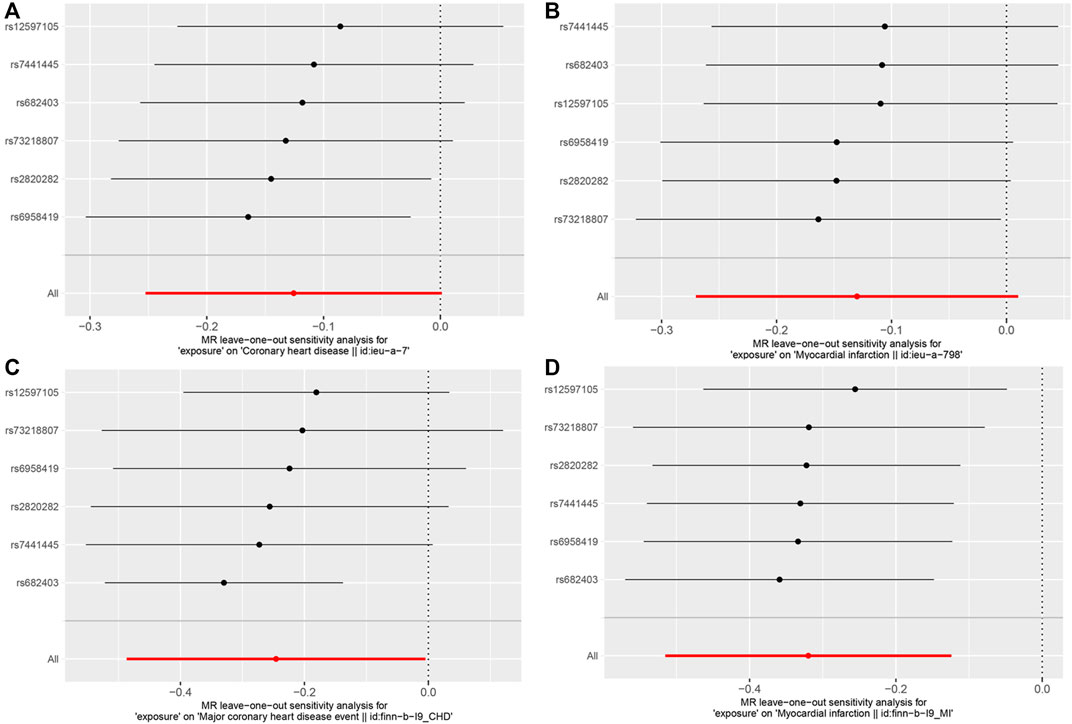
FIGURE 5. Results of the leave-one-out analysis. (A). The leave-one-out analysis for RuminococcusUCG010 on CAD based on CARDIoGRAMplusC4D. (B). The leave-one-out analysis for RuminococcusUCG010 on MI based on CARDIoGRAMplusC4D. (C). The leave-one-out analysis for RuminococcusUCG010 on CAD based on FinnGen. (D). The leave-one-out analysis for RuminococcusUCG010 on MI based on FinnGen.
3.2 MVMR study and mediation analysis
A suggestively association was observed for a higher genetically predicted abundance of RuminococcusUCG010 with T2DM risk based on both the DIAGRAMplusGERAplusUKB (OR: 0.81, 95% CI: 0.71, 0.93, p = 1.75 × 10−3) and FinnGen (OR: 0.79, 95% CI: 0.69, 0.90, p = 3.27 × 10−4). There was no obvious causal effect between RuminococcusUCG010 and LDL-C, essential hypertension. The results of MR estimates are shown in Supplementary Table S6. There was no heterogeneity in the Cochran Q and I2 tests (Supplementary Table S7).
Nine genetic predictors for PWY-5022 was screened out as IVs to calculate association (Supplementary Table S8). We did not detect any causal relationships between the abundance of PWY-502 and CAD/MI (Supplementary Table S9). There was a certain heterogeneity between PWY-5022 and CAD based on the FinnGen (P Cochran’s Q = 0.05, I2 = 36%), with no heterogeneity in the rest (Supplementary Table S10).
T2DM and RuminococcusUCG010 was included as exposures for MVMR. The association of genetically predicted RuminococcusUCG010 with CAD/MI disappeared after adjusting for T2DM in the MVMR. There was no causal effect of RuminococcusUCG010 on CAD or MI in the correction model (Table 4). The mediation effect of T2DM was presented in Table 5 with an average proportion of 20% on CAD and 17% on MI, respectively.
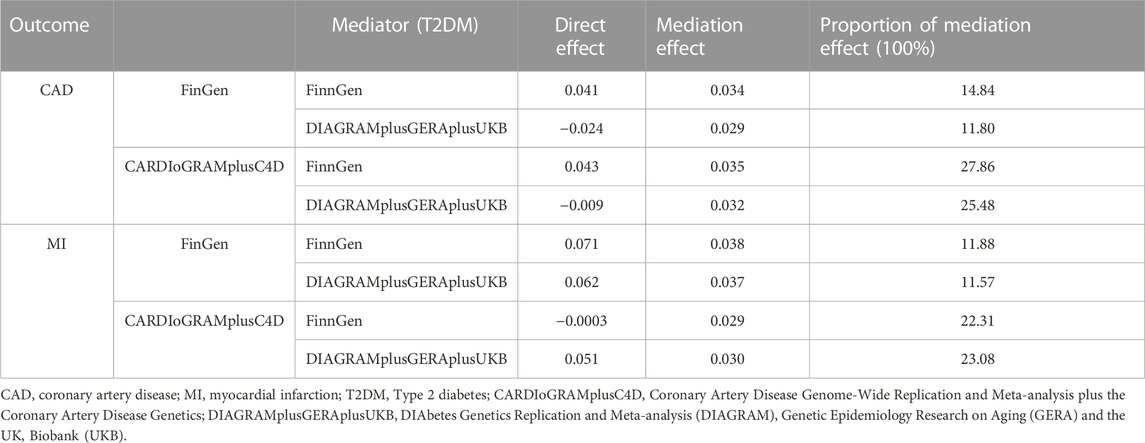
TABLE 5. Mediation analysis of the mediation effect between RuminococcaceaeUCG010 and CAD/MI via T2DM.
4 Discussion
Metabolic risk factors such as diabetes, dyslipidaemia and obesity often converge and interact with each other to significantly increase the risk of cardiometabolic diseases, which is an important strategy for the primary prevention of CAD. The occurrence of one metabolic disease is associated with a significantly increased risk of the second. In a cohort of 0.5 million Chinese adults, the multimorbidity rate was 15.8%. The highest mortality is caused by cardiometabolic multimorbidity, and any combination of cardiometabolic multimorbidity has a multiplicative mortality risk (Fan et al., 2022). The gut–heart axis has been proposed on the basis of accumulating evidence that gut dysbiosis plays a crucial part in the progression of atherosclerosis. Consequently, the composition and function of the GM is a potential target to improve prognosis (Chen et al., 2020; Bianchi et al., 2022). Our study aimed to evaluate the causal effect between the GM and CAD/MI based on the current correlation study, and to provide a new metabolic intervention target for CAD.
In this study, we conducted MR analysis at different levels of GM bacterial taxa. At the phylum, class, order and species levels, no association between the GM taxa and CAD or MI risk was found in any database. However, the results suggested that increased abundance of the RuminococcusUCG010 genus reduced the risk of CAD and MI with consistent results in both meta-analysis and repeated analysis of the UKB dataset. No horizontal pleiotropy was detected in our study. In MVMR and mediator analysis, we further found that T2DM was involved as a mediator in the protective effect of RumicocusUCG010 on CAD/MI. Our findings provide novel ideas for future approaches to the prophylaxis and treatment of CAD or MI, that is, targeted modulation of dysbiosis of specific GM taxa.
The GM comprises several species of microorganisms, including bacteria, yeast, and viruses. Taxonomically, bacteria are classified by phyla, classes, orders, families, genera, and species. The dominant microbes of the GM belong to five phyla (Bacteroidetes, Firmicutes, Actinobacteria, Proteobacteria and Cerrucomicrobia), with Bacteroidetes and Firmicutes accounting for 90% (Arumugam et al., 2011). Ruminococcus (family Oscillospiraceae, phylum Firmicutes) is a gut-associated butyrate-producing bacterial genus. Butyrate, a short-chain fatty acid (SCFA), has powerful anti-inflammatory properties that inhibit proinflammatory responses by intestinal macrophages (Louis et al., 2007; Arpaia and Rudensky., 2014). Increased inflammation has been confirmed to play a role in the onset of atherosclerotic CVD. Experimentally induced acute endotoxemia can aggregate inflammatory factors and lead to vascular endothelial damage, while chronic endotoxemia is related to metabolic syndrome (MetS) (Boutagy et al., 2016). Kazuyuki and Kang et al. reported that butyrate and butyrate producing Ruminococcus can reduce the development of endotoxemia and atherosclerosis in mice (Kang et al., 2017; Kasahara et al., 2018). In a population cohort study, involving 161 CAD patients and 40 healthy controls, a co-abundance bacterial group containing Lachnospiraceae and Ruminococcaceae was negatively associated with CAD development (Liu et al., 2019). However, there is no significant causal effect between butyrate and CAD/MI in our study. It should be noted that PWY-5022 represents as a proxy for intestinal butyrate production, but not be directly related to the amount of butyrate absorbed by the host. The species of bacteria most associated with the abundance of PWY-5022 are Eubacterium rectale and Roseburia integralis, and the metabolic pathway correlated with RuminococcusUCG010 needs to be found to improve the reliability of the results. Arterial stiffness is known as an early manifestation of atherosclerosis, and carotid-femoral pulse velocity (cfPWV) is considered the gold standard for measuring arterial stiffness (Katsiki et al., 2016). A recent study involving 617 women from the TwinsUK cohort revealed a negative correlation between arterial stiffness and the abundance of the Ruminococcaceae family (Menni et al., 2018). As the primary risk factor for cardiovascular disease, diabetes has been an important target for the prevention and control of CAD. It has been found that daggligin and metformin produce hypoglycaemic effects by improving the structure of the microbiota. Ruminococcaceae was the dominant enterotype in two groups of medicated mice, which indicates that Ruminococcaceae plays a potential role in regulating blood glucose (Yang et al., 2020). Our study further confirmed the mediation effect of T2DM on the causal effect of RuminococcusUCG010 and CAD/MI that RuminococcusUCG010 can reduce the risk of CAD by reducing the incidence of T2DM. The above study findings were consistent with our conclusions. Interestingly, another study found that Ruminococcus was enriched in stable angioma (SA) and acute myocardial infarction (AMI) through faecal gene sequencing of 261 patients with CAD (Zhang et al., 2022). In fact, in human ecology, the GM exists as functional groups named ‘guilds’, with pivotal members of each guild growing or declining together in response to changes in the physiological environment. In clinical cohort studies, the composition of the GM also changes dynamically with long-term development of CAD (Faust and Raes., 2012). In conclusion, our data suggested that, overall, a high abundance of RuminococcusUCG110 has a long-term protective effect against CAD or MI, in which T2DM plays an important mediating effect.
The MR study design is a major merit of this study. The two-sample MR explored the linear relationship between exposure and outcome and MVMR and Mediation analysis was conducted to investigate a potential non-linear association. Genetic variation is a stable and measurable exposure factor that diminishes confounding factors and reverse causality and therefore reinforces causal inference. Based on an adequate sample size of data, stringent quality control conditions and analysis methods were adopted. We used multiple models to evaluate causal effects and conduct repeated validation in different databases. In addition, several sensitivity analyses were performed in our study. The research results were reliable and stable. Compared to a single exposure MR study, the exposure factor, namely, the GM, in this study is complex and massive with a huge workload and analysis challenges. There were several limitations to this study that need to be taken into account. First, two-sample MR requires two independent samples from the same ethnicity but without overlap. This study aimed to ensure that both databases were from European populations as much as possible to avoid ethnic bias. However, in the absence of individual data, the independence of the two samples cannot be strictly guaranteed. Second, the statistical power for the UK Biobank data analysis did not reach the 80% threshold, which may be caused by slight variance in the GM explained by the selected IVs. Third, this study focused on European populations, and subsequent comprehensive studies will need to be carried out across different ethnic groups.
5 Conclusion
This MR study provided suggestive genetic evidence that the higher the RuminococcusUCG010 abundance is, the lower the risk of CAD and MI, with T2DM playing a mediating effect. This genus may become a novel target in strategies for treating and preventing CAD and MI.
Data availability statement
The original contributions presented in the study are included in the article/Supplementary Material, further inquiries can be directed to the corresponding author.
Ethics statement
Ethical review and approval was not required for the study on human participants in accordance with the local legislation and institutional requirements. The patients/participants provided their written informed consent to participate in this study.
Author contributions
Conceptualization, YL, DW; Methodology, YL, DW; Formal analysis, DW, XC; Software, DW, ZL; Data Curation, DW and XC; Investigation, DW, XC, and ZL; Resources, DW; Visualization, DW and ZL; Supervision, YL and DW; Writing-original draft, DW, XC; Writing-review and editing, YL; Funding acquisition, YL; Project administration, YL and DW All authors have read and agreed to the published version of the manuscript.
Funding
This study was funded by the Natural Science Foundation of Hunan Province (No. 2022JJ30947).
Acknowledgments
The authors sincerely thank the UK Biobank, CARDIoGRAMplusC4D, FinnGen and the MiBioGen consortium for providing summary statistics.
Conflict of interest
The authors declare that the research was conducted in the absence of any commercial or financial relationships that could be construed as a potential conflict of interest.
Publisher’s note
All claims expressed in this article are solely those of the authors and do not necessarily represent those of their affiliated organizations, or those of the publisher, the editors and the reviewers. Any product that may be evaluated in this article, or claim that may be made by its manufacturer, is not guaranteed or endorsed by the publisher.
Supplementary material
The Supplementary Material for this article can be found online at: https://www.frontiersin.org/articles/10.3389/fgene.2023.1158293/full#supplementary-material
References
Afzaal, M., Saeed, F., Shah, Y. A., Hussain, M., Rabail, R., Socol, C. T., et al. (2022). Human gut microbiota in health and disease: Unveiling the relationship. Front. Microbiol. 13, 999001. doi:10.3389/fmicb.2022.999001
Arpaia, N., and Rudensky, A. Y. (2014). Microbial metabolites control gut inflammatory responses. Proc. Natl. Acad. Sci. U. S. A. 111 (6), 2058–2059. doi:10.1073/pnas.1323183111
Arumugam, M., Raes, J., Pelletier, E., Le Paslier, D., Yamada, T., Mende, D. R., et al. (2011). Enterotypes of the human gut microbiome. Nature 473 (7346), 174–180. doi:10.1038/nature09944
Bennett, B. J., de Aguiar Vallim, T. Q., Wang, Z., Shih, D. M., Meng, Y., Gregory, J., et al. (2013). Trimethylamine-N-oxide, a metabolite associated with atherosclerosis, exhibits complex genetic and dietary regulation. Cell Metab. 17 (1), 49–60. doi:10.1016/j.cmet.2012.12.011
Bianchi, F., Cappella, A., Gagliano, N., Sfondrini, L., and Stacchiotti, A. (2022). Polyphenols-Gut-Heart: An impactful relationship to improve cardiovascular diseases. Antioxidants (Basel) 11 (9), 1700. doi:10.3390/antiox11091700
Bonder, M. J., Kurilshikov, A., Tigchelaar, E. F., Mujagic, Z., Imhann, F., Vila, A. V., et al. (2016). The effect of host genetics on the gut microbiome. Nat. Genet. 48 (11), 1407–1412. doi:10.1038/ng.3663
Boutagy, N. E., McMillan, R. P., Frisard, M. I., and Hulver, M. W. (2016). Metabolic endotoxemia with obesity: Is it real and is it relevant? Biochimie 124, 11–20. doi:10.1016/j.biochi.2015.06.020
Bowden, J., Davey Smith, G., and Burgess, S. (2015). Mendelian randomization with invalid instruments: Effect estimation and bias detection through egger regression. Int. J. Epidemiol. 44 (2), 512–525. doi:10.1093/ije/dyv080
Bowden, J., Davey Smith, G., Haycock, P. C., and Burgess, S. (2016). Consistent estimation in mendelian randomization with some invalid instruments using a weighted median estimator. Genet. Epidemiol. 40 (4), 304–314. doi:10.1002/gepi.21965
Bowden, J., Del Greco, M. F., Minelli, C., Davey Smith, G., Sheehan, N., and Thompson, J. (2017). A framework for the investigation of pleiotropy in two-sample summary data Mendelian randomization. Stat. Med. 36 (11), 1783–1802. doi:10.1002/sim.7221
Burgess, S., Butterworth, A., and Thompson, S. G. (2013). Mendelian randomization analysis with multiple genetic variants using summarized data. Genet. Epidemiol. 37 (7), 658–665. doi:10.1002/gepi.21758
Burgess, S., Daniel, R. M., Butterworth, A. S., and Thompson, S. G.EPIC-InterAct consortium. (2014). Network mendelian randomization: Using genetic variants as instrumental variables to investigate mediation in causal pathways. Int. J. Epidemiol. 44, 484–495. doi:10.1093/ije/dyu176
Burgess, S., and Thompson, S. G.CRP CHD Genetics Collaboration (2011). Avoiding bias from weak instruments in Mendelian randomization studies. Int. J. Epidemiol. 40 (3), 755–764. doi:10.1093/ije/dyr036
Burgess, S., and Thompson, S. G. (2017). Interpreting findings from Mendelian randomization using the MR-Egger method. Eur. J. Epidemiol. 32 (5), 377–389. doi:10.1007/s10654-017-0255-x
Burgess, S., and Thompson, S. G. (2015). Multivariable mendelian randomization: The use of pleiotropic genetic variants to estimate causal effects. Am. J. Epidemiol. 181 (4), 251–260. doi:10.1093/aje/kwu283
Chen, W., Zhang, S., Wu, J., Ye, T., Wang, S., and Wang, P. (2020). Butyrate-producing bacteria and the gut-heart axis in atherosclerosis. Clin. Chim. Acta 507, 236–241. doi:10.1016/j.cca.2020.04.037
Emdin, C. A., Khera, A. V., and Kathiresan, S. (2017). Mendelian randomization. JAMA 318 (19), 1925–1926. doi:10.1001/jama.2017.17219
Fan, J., Sun, Z., Yu, C., Guo, Y., Pei, P., Yang, L., et al. (2022). Multimorbidity patterns and association with mortality in 0.5 million Chinese adults. Chin. Med. J. Engl. 135 (6), 648–657. doi:10.1097/CM9.0000000000001985
Faust, K., and Raes, J. (2012). Microbial interactions: From networks to models. Nat. Rev. Microbiol. 10 (8), 538–550. doi:10.1038/nrmicro2832
FinnGen (2022). Consortium FinnGen data freeze 6. Available online: https://www.finngen.fi/.[Accessed 10 February 2022].
Gregory, J. C., Buffa, J. A., Org, E., Wang, Z., Levison, B. S., Zhu, W., et al. (2015). Transmission of atherosclerosis susceptibility with gut microbial transplantation. J. Biol. Chem. 290 (9), 5647–5660. doi:10.1074/jbc.M114.618249
Han, C. (2008). Detecting invalid instruments using L1-GMM. Econ. Lett. 101.3, 285–287. doi:10.1016/j.econlet.2008.09.004
Higgins, J. P., and Thompson, S. G. (2002). Quantifying heterogeneity in a meta-analysis. Stat. Med. 14, 1539–1558. doi:10.1002/sim.1186
Hu, T., Wu, Q., Yao, Q., Jiang, K., Yu, J., and Tang, Q. (2022). Short-chain fatty acid metabolism and multiple effects on cardiovascular diseases. Ageing Res. Rev. 81, 101706. doi:10.1016/j.arr.2022.101706
Jia, J., Dou, P., Gao, M., Kong, X., Li, C., and Liu, Z. (2019). Assessment of causal direction between gut microbiota-dependent metabolites and cardiometabolic health: A bidirectional mendelian randomization analysis. Diabetes 68 (9), 1747–1755. doi:10.2337/db19-0153
Jie, Z., Xia, H., Zhong, S. L., Feng, Q., Li, S., Liang, S., et al. (2017). The gut microbiome in atherosclerotic cardiovascular disease. Nat. Commun. 8, 845. doi:10.1038/s41467-017-00900-1
Kang, C., Wang, B., Kaliannan, K., Wang, X., Lang, H., Hui, S., et al. (2017). Gut microbiotamediates the protective effects of dietary capsaicin against chronic low-grade inflammation and associated obesity induced by high-fat diet. mBio 8 (3), e00470-17. doi:10.1128/mBio.00470-17
Karlsson, F. H., Fak, F., Nookaew, I., Tremaroli, V., Fagerberg, B., and Petranovic, D. (2012). Symptomatic atherosclerosis is associated with an altered gut metagenome. Nat. Commun. 3, 1245. doi:10.1038/ncomms2266
Kasahara, K., Krautkramer, K. A., Org, E., Romano, K. A., Kerby, R. L., Vivas, E. I., et al. (2018). Interactions between Roseburia intestinalis and diet modulate atherogenesis in a murine model. Nat. Microbiol. 3 (12), 1461–1471. doi:10.1038/s41564-018-0272-x
Katsiki, N., Kollari, E., Dardas, S., Dardas, P., Haidich, A. B., and Athyros, V. G. (2016). Is there an association between carotid-femoral pulse wave velocity and coronary heart disease in patients with coronary artery disease: A pilot study. Open Cardiovasc Med. J. 10, 64–68. doi:10.2174/1874192401610010064
Kazemian, N., Mahmoudi, M., Halperin, F., Wu, J. C., and Pakpour, S. (2020). Gut microbiota and cardiovascular disease: Opportunities and challenges. Microbiome 8 (1), 36. doi:10.1186/s40168-020-00821-0
Khan, M. A., Hashim, M. J., Mustafa, H., Baniyas, M. Y., Al suwaidi, S., AlKatheeri, R., et al. (2020). Global epidemiology of ischemic heart disease: Results from the global burden of disease study. Cureus. 12(7), e9349. doi:10.7759/cureus.9349
Koren, O., Spor, A., Felin, J., Fak, F., Stombaugh, J., Tremaroli, V., et al. (2011). Human oral, gut, and plaque microbiota in patients with atherosclerosis. Proc. Natl. Acad. Sci. U. S. A. 1, 4592–4598. doi:10.1073/pnas.1011383107
Kotseva, K., De Bacquer, D., Jennings, C., Gyberg, V., De Backer, G., Rydén, L., et al. (2017). Time trends in lifestyle, risk factor control, and use of evidence-based medications in patients with coronary heart disease in europe: Results from 3 EUROASPIRE surveys, 1999-2013. Glob. Heart 12 (4), 315–322.e3. doi:10.1016/j.gheart.2015.11.003
Kotseva, K., Wood, D., De Bacquer, D., De Backer, G., Rydén, L., Jennings, C., et al. (2016). Euroaspire IV: A European society of cardiology survey on the lifestyle, risk factor and therapeutic management of coronary patients from 24 European countries. Eur. J. Prev. Cardiol. 23 (6), 636–648. doi:10.1177/2047487315569401
Kurilshikov, A., Medina-Gomez, C., Bacigalupe, R., Radjabzadeh, D., Wang, J., Demirkan, A., et al. (2021). Large-scale association analyses identify host factors influencing human gut microbiome composition. Nat. Genet. 53 (2), 156–165. doi:10.1038/s41588-020-00763-1
Kwun, J. S., Kang, S. H., Lee, H. J., Park, H. K., Lee, W. J., Yoon, C. H., et al. (2020). Comparison of thrombus, gut, and oral microbiomes in Korean patients with ST-elevation myocardial infarction: A case-control study. Exp. Mol. Med. 52 (12), 2069–2079. doi:10.1038/s12276-020-00543-1
Lawlor, D. A. (2016). Commentary: Two-sample mendelian randomization: Opportunities and challenges. Int. J. Epidemiol. 45 (3), 908–915. doi:10.1093/ije/dyw127
Lin, Z., Pan, I., and Pan, W. (2022). A practical problem with Egger regression in Mendelian randomization. PLoS Genet. 18 (5), e1010166. doi:10.1371/journal.pgen.1010166
Liu, H., Chen, X., Hu, X., Niu, H., Tian, R., Wang, H., et al. (2019). Alterations in the gut microbiome and metabolism with coronary artery disease severity. Microbiome 7 (1), 68. doi:10.1186/s40168-019-0683-9
Louis, P., Scott, K. P., Duncan, S. H., and Flint, H. J. (2007). Understanding the effects of diet on bacterial metabolism in the large intestine. J. Appl. Microbiol. 102, 1197–1208. doi:10.1111/j.1365-2672.2007.03322.x
Menni, C., Lin, C., Cecelja, M., Mangino, M., Matey-Hernandez, M. L., Keehn, L., et al. (2018). Gut microbial diversity is associated with lower arterial stiffness in women. Eur. Heart J. 39 (25), 2390–2397. doi:10.1093/eurheartj/ehy226
Moludi, J., Kafil, H. S., Qaisar, S. A., Gholizadeh, P., Alizadeh, M., and Vayghyan, H. J. (2021). Effect of probiotic supplementation along with calorie restriction on metabolic endotoxemia, and inflammation markers in coronary artery disease patients: A double blind placebo controlled randomized clinical trial. Nutr. J. 20 (1), 47. doi:10.1186/s12937-021-00703-7
Mozaffarian, D., Benjamin, E. J., Go, A. S., Arnett, D. K., Blaha, M. J., Cushman, M., et al. (2015). Heart disease and stroke statistics-2015 update: A report from the American heart association. Circulation 131 (4), e29–e322. doi:10.1161/CIR.0000000000000152
Nikpay, M., Goel, A., Won, H. H., Hall, L. M., Willenborg, C., Hall, L. M., et al. (2015). A comprehensive 1,000 Genomes-based genome-wide association meta-analysis of coronary artery disease. Nat. Genet. 47 (10), 1121–1130. doi:10.1038/ng.3396
Novakovic, M., Rout, A., Kingsley, T., Kirchoff, R., Singh, A., Verma, V., et al. (2020). Role of gut microbiota in cardiovascular diseases. World J. Cardiol. 12 (4), 110–122. doi:10.4330/wjc.v12.i4.110
Ott, S. J., El Mokhtari, N. E., Musfeldt, M., Hellmig, S., Freitag, S., Rehman, A., et al. (2006). Detection of diverse bacterial signatures in atherosclerotic lesions of patients with coronary heart disease. Circulation 113 (7), 929–937. doi:10.1161/CIRCULATIONAHA.105.579979
Relton, C. L., and Davey Smith, G. (2012). Two-step epigenetic mendelian randomization: A strategy for establishing the causal role of epigenetic processes in pathways to disease. Int. J. Epidemiol. 41 (1), 161–176. doi:10.1093/ije/dyr233
Rinninella, E., Raoul, P., Cintoni, M., Franceschi, F., Miggiano, G. A. D., and Gasbarrini, A. (2019). What is the healthy gut microbiota composition? A changing ecosystem across age, environment, diet, and diseases. Microorganisms 7 (1), 14. doi:10.3390/microorganisms7010014
Sanna, S., van Zuydam, N. R., Mahajan, A., Kurilshikov, A., Vich Vila, A., Võsa, U., et al. (2019). Causal relationships among the gut microbiome, short-chain fatty acids and metabolic diseases. Nat. Genet. 51(4):600–605. doi:10.1038/s41588-019-0350-x
Saxon, D. R., Reiter-Brennan, C., Blaha, M. J., and Eckel, R. H. (2020). Cardiometabolic medicine: Development of a new subspecialty. J. Clin. Endocrinol. Metab. 105, dgaa261. doi:10.1210/clinem/dgaa261
Shan, S., Yin, R., Shi, J., Zhang, L., Liu, F., Qiao, Q., et al. (2022). Bowman-birk major type trypsin inhibitor derived from foxtail millet bran attenuate atherosclerosis via remodeling gut microbiota in ApoE-/- mice. J. Agric. Food Chem. 70 (2), 507–519. doi:10.1021/acs.jafc.1c05747
Skrivankova, V. W., Richmond, R. C., Woolf, B. A. R., Yarmolinsky, J., Davies, N. M., Swanson, S. A., et al. (2021). Strengthening the reporting of observational studies in epidemiology using mendelian randomization: The STROBE-MR statement. JAMA 326 (16), 1614–1621. doi:10.1001/jama.2021.18236
Smith, G. D., and Ebrahim, S. (2003). 'Mendelian randomization': Can genetic epidemiology contribute to understanding environmental determinants of disease? Int. J. Epidemiol. 32 (1), 1–22. doi:10.1093/ije/dyg070
Smith, G. D., Lawlor, D. A., Harbord, R., Timpson, N., Day, I., and Ebrahim, S. (2007). Clustered environments and randomized genes: A fundamental distinction between conventional and genetic epidemiology. PLoS Med. 4 (12), e352. doi:10.1371/journal.pmed.0040352
Sudlow, C., Gallacher, J., Allen, N., Beral, V., Burton, P., Danesh, J., et al. (2015). UK biobank: An open access resource for identifying the causes of a wide range of complex diseases of middle and old age. PLoS Med. 12 (3), e1001779. doi:10.1371/journal.pmed.1001779
Sved, J. A., and Hill, W. G. (2018). One hundred years of linkage disequilibrium. Genetics 209 (3), 629–636. doi:10.1534/genetics.118.300642
Tang, W. H., Wang, Z., Levison, B. S., Koeth, R. A., Britt, E. B., Fu, X., et al. (2013). Intestinal microbial metabolism of phosphatidylcholine and cardiovascular risk. N. Engl. J. Med. 368 (17), 1575–1584. doi:10.1056/NEJMoa1109400
Verbanck, M., Chen, C. Y., Neale, B., and Do, R. (2018). Detection of widespread horizontal pleiotropy in causal relationships inferred from Mendelian randomization between complex traits and diseases. Nat. Genet. 50 (5), 693–698. doi:10.1038/s41588-018-0099-7
Verduijn, M., Siegerink, B., Jager, K. J., Zoccali, C., and Dekker, F. W. (2010). Mendelian randomization: Use of genetics to enable causal inference in observational studies. Nephrol. Dial. Transpl. 25 (5), 1394–1398. doi:10.1093/ndt/gfq098
Wang, H., Luo, Q., Ding, X., Chen, L., and Zhang, Z. (2022). Trimethylamine N-oxide and its precursors in relation to blood pressure: A mendelian randomization study. Front. Cardiovasc Med. 9, 922441. doi:10.3389/fcvm.2022.922441
Wang, Z., Klipfell, E., Bennett, B. J., Koeth, R., Levison, B. S., Dugar, B., et al. (2011). Gut flora metabolism of phosphatidylcholine promotes cardiovascular disease. Nature 472 (7341), 57–63. doi:10.1038/nature09922
Wang, Z., Tang, W. H., Buffa, J. A., Fu, X., Britt, E. B., Koeth, R. A., et al. (2014). Prognostic value of choline and betaine depends on intestinal microbiota-generated metabolite trimethylamine-N-oxide. Eur. Heart J. 35 (14), 904–910. doi:10.1093/eurheartj/ehu002
Warmbrunn, M. V., Herrema, H., Aron-Wisnewsky, J., Soeters, M. R., Van Raalte, D. H., and Nieuwdorp, M. (2020). Gut microbiota: A promising target against cardiometabolic diseases. Expert Rev. Endocrinol. Metab. 15 (1), 13–27. doi:10.1080/17446651.2020.1720511
Willer, C. J., Schmidt, E. M., Sengupta, S., Peloso, G. M., Gustafsson, S., Kanoni, S., et al. (2013). Discovery and refinement of loci associated with lipid levels. Nat. Genet. 45 (11), 1274–1283. doi:10.1038/ng.2797
Xu, H., Wang, X., Feng, W., Liu, Q., Zhou, S., and Liu, Q. (2020). The gut microbiota and its interactions with cardiovascular disease. Microb. Biotechnol. 13, 637–656. doi:10.1111/1751-7915.13524
Xue, A., Wu, Y., Zhu, Z., Zhang, F., Kemper, K. E., Zheng, Z., et al. (2018). Genome-wide association analyses identify 143 risk variants and putative regulatory mechanisms for type 2 diabetes. Nat. Commun. 9 (1), 2941. doi:10.1038/s41467-018-04951-w
Yamashita, T., Yoshida, N., Emoto, T., Saito, Y., and Hirata, K. I. (2021). Two gut microbiota-derived toxins are closely associated with cardiovascular diseases: A review. Toxins (Basel) 13 (5), 297. doi:10.3390/toxins13050297
Yang, M., Shi, F. H., Liu, W., Zhang, M. C., Feng, R. L., Qian, C., et al. (2020). Dapagliflozin modulates the fecal microbiota in a type 2 diabetic rat model. Front. Endocrinol. (Lausanne) 11, 635. doi:10.3389/fendo.2020.00635
Zhang, T., Ren, H., Du, Z., Zou, T., Guang, X., and Zhang, Y. (2022). Diversified shifts in the cross talk between members of the gut microbiota and development of coronary artery diseases. Microbiol. Spectr. 27, e0280422. doi:10.1128/spectrum.02804-22
Zhu, Z. M. (2021). Cardiometabolic diseases: Concept, challenge and clinical practice. Chin. J. Cardiol. 49 (7), 650–655. doi:10.3760/cma.j.cn112148-20210506-00395
Keywords: Mendelian randomization, gut microbiota, coronary artery disease, myocardial infarction, causality
Citation: Wang D, Chen X, Li Z and Luo Y (2023) Association of the gut microbiota with coronary artery disease and myocardial infarction: A Mendelian randomization study. Front. Genet. 14:1158293. doi: 10.3389/fgene.2023.1158293
Received: 03 February 2023; Accepted: 29 March 2023;
Published: 11 April 2023.
Edited by:
Tomohiro Hayashi, Kobe University, JapanReviewed by:
Kei Hang Katie Chan, City University of Hong Kong, Hong Kong SAR, ChinaSatya Dash, University Health Network (UHN), Canada
Copyright © 2023 Wang, Chen, Li and Luo. This is an open-access article distributed under the terms of the Creative Commons Attribution License (CC BY). The use, distribution or reproduction in other forums is permitted, provided the original author(s) and the copyright owner(s) are credited and that the original publication in this journal is cited, in accordance with accepted academic practice. No use, distribution or reproduction is permitted which does not comply with these terms.
*Correspondence: Ying Luo, 13873190858@163.com