- 1Pediatric Research Center, Children’s Hospital, Helsinki University Hospital, University of Helsinki, Helsinki, Finland
- 2Division of Micro and Nanosystems, School of Electrical Engineering and Computer Science, KTH Royal Institute of Technology, Stockholm, Sweden
- 3Champions Oncology, Hackensack, NJ, United States
- 4Istituto di Ricerca Pediatrica, Padova, Italy
- 5XenTech, Evry, France
- 6Department of Developmental Biology, Washington University School of Medicine, St. Louis, MO, United States
- 7Department of Pediatrics, Washington University School of Medicine, St. Louis Children’s Hospital, St. Louis, MO, United States
- 8Faculty of Medicine and Health Technology, Center for Child, Adolescent and Maternal Health Research, Tampere University, Tampere, Finland
Hepatoblastoma (HB) is the most common malignant liver tumor among children. To gain insight into the pathobiology of HB, we performed RNA sequence analysis on 5 patient-derived xenograft lines (HB-243, HB-279, HB-282, HB-284, HB-295) and 1 immortalized cell line (HUH6). Using cultured hepatocytes as a control, we found 2,868 genes that were differentially expressed in all of the HB lines on mRNA level. The most upregulated genes were ODAM, TRIM71, and IGDCC3, and the most downregulated were SAA1, SAA2, and NNMT. Protein-protein interaction analysis identified ubiquitination as a key pathway dysregulated in HB. UBE2C, encoding an E2 ubiquitin ligase often overexpressed in cancer cells, was markedly upregulated in 5 of the 6 HB cell lines. Validation studies confirmed UBE2C immunostaining in 20 of 25 HB tumor specimens versus 1 of 6 normal liver samples. The silencing of UBE2C in two HB cell models resulted in decreased cell viability. RNA sequencing analysis showed alterations in cell cycle regulation after UBE2C knockdown. UBE2C expression in HB correlated with inferior patient survival. We conclude that UBE2C may hold prognostic utility in HB and that the ubiquitin pathway is a potential therapeutic target in this tumor.
1 Introduction
Hepatoblastoma (HB) is the most common malignant pediatric liver neoplasm with an annual incidence of 1.9/1,000,000 (Aronson and Meyers, 2016; Feng et al., 2019) that has been increasing over the past decades (Linabery and Ross, 2008). The etiology of most cases of HB remains unknown, but preterm birth, birthweight less than 2500 g, and certain genetic conditions such as Familial Adenomatous Polyposis and Beckwith-Wiedemann syndrome are associated with increased risk of HB (Spector and Birch, 2012; Paquette et al., 2019). Wnt/β-catenin signaling has been identified as one of the pathways altered in the majority of HB tumors, but other molecular pathways involved in HB pathogenesis are not yet fully understood (Udatsu et al., 2001; Armengol et al., 2011). Current treatment of HB includes complete surgical resection combined with doxorubicin and cisplatin- or carboplatin-based neoadjuvant and adjuvant chemotherapy (Zsíros et al., 2010). These forms of chemotherapy are effective but often lead to serious long-term side effects including cardiotoxicity, ototoxicity, and nephrotoxicity (Spector and Birch, 2012; Volkova and Russell, 2012). Although the prognosis of HB has improved over the years, 20%–30% of HB patients still respond poorly to current treatment modalities (Sivaprakasam et al., 2011), so new therapeutic targets are needed.
Metabolic reprogramming is one of the hallmarks of cancer (Faubert et al., 2020). Highly proliferating tumor cells need to adapt to conditions such as hypoxia and lack of nutrients and thus require metabolic reprogramming to enhance their survival. Many of the oncogenes and tumor suppressors are participating in dysregulation of metabolic pathways in cancer (Nong et al., 2023). Also, genes coding for metabolic enzymes have been described to be mutated or aberrantly expressed in several tumor types (Sreedhar and Zhao, 2018). Major changes include alterations in glucose metabolism, known as Warburg effect, as well as in amino acid and lipid metabolism (Counihan et al., 2018). Alterations in genes regulating ubiquitination and deubiquitination and their role as modulators of the metabolic changes of tumor cells are also known to be essential in cancer progression (Sun et al., 2020).
Some changes in metabolic genes have already been demonstrated to be present in HB. In HB tumors, activating mutations in the Wnt/β-catenin pathway genes lead to altered glucose metabolism mediated by upregulation of GLUT3 (Crippa et al., 2017). Immortalized HepG2 cells, originally derived from a HB, exhibit deranged bile acid metabolism (Kullak-Ublick et al., 1996) due in part to and downregulated SLC10A1 (Wang et al., 2020). In the same study, the authors also found that downregulation of SLC10A1 resulted in upregulated adenosine metabolism. Retinol metabolism and cytochrome P450 pathway have both been demonstrated to be downregulated in HB (Sekiguchi et al., 2020). Despite these findings, the gene expression behind the metabolic alterations taking place in HB still remain poorly understood.
The objective of this study was to characterize the landscape of metabolic genes in HB using RNA sequencing data and bioinformatics analyses. Our overarching goal was to identify potential treatment targets and novel biomarkers in HB.
2 Materials and methods
2.1 RNA sequencing and microarray datasets
Raw RNA sequencing datasets from previously published studies were obtained from Gene Expression Omnibus (GEO) database of National Center for Biotechnology Information (NCBI) (http://www.ncbi.nlm.nih.gov/geo/) and European Genome-phenome Archive (EGA) (https://ega-archive.org/). Accession numbers were as follows: EGAS00001004827/EGAD00001006621 (HB-282, HB-295, HB-279, HB-284, HB-243), GSE140520 (PHH-D3, 1–3), GSE83518 (1HUH6HB, 2HUH6HB). Raw microarray data of gene expression in 53 HB tissue samples and 14 noncancerous liver tissue samples collected from the HB patients at the time of surgery were acquired from GEO, accession number GSE131329.
2.2 Identification of differentially expressed genes and differentially expressed metabolic genes
RNA sequencing dataset files were analyzed with Chipster software (https://chipster.rahtiapp.fi/) (Kallio et al., 2011). Reads were preprocessed using Trimmomatic and aligned to human reference genome (GRCh38) using HISAT2 (Kim et al., 2015). Reads per genes were counted with HTseq (Anders et al., 2015). Differential expression analysis was conducted with the edgeR package (Robinson et al., 2009). Differentially expressed genes (DEGs) were then filtered using cut-off criteria adjusted to p-value <0.05 and |log2FC|≥1.0.
Microarray data were analyzed with Chipster plus the normalization tool for Affymetrix gene arrays (Li, 2001; Irizarry et al., 2003). Statistical tests were conducted using the “Two group tests” tool (empirical Bayes as test and BH as p-value adjustment method) (Smyth, 2004).
Human metabolic genes were obtained from The Virtual Metabolic Human database (VMH) (Supplementary Table S1) (Noronha et al., 2019). A list of DEGs in each cell line was compared to a list of human metabolic genes to further filter the results. Duplicates were removed from the list leaving 3,285 unique genes.
2.3 Protein-protein interaction (PPI) network construction
PPI networks of differentially expressed metabolic genes in each cell line were constructed with the online tool of STRING database (Szklarczyk et al., 2019) using 0.7 as minimum required interaction score. Results were visualized with Cytoscape (Shannon et al., 2003). Highly interconnected areas (clusters) of these interaction maps were identified and scored using the Cytoscape plugin Molecular Complex Detection (MCODE) (Bader and Hogue, 2003) using the following criteria: degree cut-off: 2; haircut: yes; fluff: no; node score cut-off: 0.2; K-core: 2; max. Depth: 100. Based on this score, highest-ranking cluster of each cell line was chosen to be investigated more thoroughly. PPI network of UBE2C was constructed using STRING with 0.4 as minimum required interaction score.
2.4 Kyoto encyclopedia of genes and genomes (KEGG) and gene ontology (GO) pathway enrichment analyses
Genes in each cell line’s highest-scoring cluster were uploaded to Enrichr (Chen et al., 2013; Kuleshov et al., 2016; Xie et al., 2021). Results of KEGG pathway and GO Biological Processes term enrichment analyses were imported from the website. Results with adjusted p-value of <0.05 were considered significant. The top 5 terms with lowest adjusted p-values from both KEGG and GO were chosen for each HB cell line. Python programming language (Python Software Foundation) with Matplotlib (Hunter, 2007), NumPy (Harris et al., 2020), and pandas (McKinney, 2010) libraries were used for handling and plotting this data.
2.5 Statistical analysis of clinical variables
Student’s t-test, Mann-Whitney U test, and receiver operating characteristic (ROC) curves were used to analyze microarray gene expression data and clinical variables provided in GSE131329 dataset. Statistical significance was set to p-value <0.05. Analyses were conducted with R software (v. 4.0.3) or GraphPad Prism (v. 8.4.2; GraphPad, San Diego, CA, United States).
2.6 Gene co-expression analysis
An online tool for gene co-expression analysis, GeneFriends v 5.0 (Raina et al., 2022), was used to analyze the gene co-expression of UBE2C. Following input parameters were used: Species; Homo Sapiens, Data Source; SRA, Tissue: All tissue types, Object type; Gene, Seed gene: UBE2C, Pearson correlation threshold: 0.75.
2.7 Patient samples
Formalin-fixed paraffin-embedded (FFPE) HB tumor samples [n = 24, median age in years 3.37 (range 0.23–11.75)] and normal liver control samples (NL, n = 6, median age in years 12.5 (range 3–26)) were obtained from the Helsinki Biobank at Helsinki University Hospital. HB samples were originally collected at the time of surgical treatment from patients treated in Children’s Hospital, Helsinki University Hospital between 1 January 1991, and 31 December 2017. Prior to resection, majority of patients had received preoperative chemotherapy. NL samples were collected from liver transplantation donors in Helsinki University Hospital. This study was approved by Helsinki University Hospital institutional ethical committee (HUS/3319/2018) and conducted in accordance with Finnish bylaws. Informed consent was obtained when samples were deposited to the Helsinki Biobank.
2.8 Immunohistochemistry
Samples were heated for 30 min at 60°C oven and deparaffinized with NeoClear (Merck-Millipore, Darmstadt, Germany). Target retrieval was performed using pH 9.0 target retrieval solution for 30 min at + 98°C (Dako, Glostrup, Denmark). Novolink Polymer Detection System Kit (Leica, Newcastle, United Kingdom) was used to block endogenous peroxidase activity and nonspecific binding. Samples were incubated with UBE2C polyclonal antibody at +4°C overnight (dilution 1:1,500; #PA5-102791; Invitrogen, Thermo Fisher Scientific). A polymerized reporter enzyme staining system (Novolink Polymer Detection System Kit) was used to visualize the bound antibody. UBE2C immunoreactivity was scored as strong nuclear staining (positive) or negative by two separate observers. Images were generated using 3DHISTECH Pannoramic 250 FLASH II digital slide scanner at Genome Biology Unit supported by HiLIFE and the Faculty of Medicine, University of Helsinki, and Biocenter Finland.
2.9 HB in vitro models
HB cell line HUH6 was obtained from Japanese Collection of Research Bioresources Cell Bank (Osaka, Japan). Cells were maintained in Dulbecco’s modified Eagle’s medium (DMEM)-glutaMAX (Gibco) supplemented with 10% FBS (Gibco), 100 U/mL penicillin (Gibco), and 100 μg/mL streptomycin sulfate (Gibco, Waltham, MA, United States). HB cell line HB-243 from patient-derived xenograft (PDX) was provided by XenTech (Evry, France) (Kats et al., 2019). HB-243 cells were cultured in Advanced DMEM/F12 (Gibco, Waltham, MA, United States) supplemented with 8% fetal bovine serum (FBS) (Gibco), 2 mM glutaMAX (Gibco), 100 U/mL penicillin (Gibco), 100 μg/mL streptomycin sulfate (Gibco) and 20 μM rock kinase inhibitor Y-27632 (S1049; SelleckChem, Houston, TX, United States). Absence of mycoplasma was regularly confirmed with PCR-based method (PromoCell, Heidelberg, Germany).
2.10 UBE2C silencing
UBE2C expression in HUH6 and HB-243 cell models was silenced by small interfering RNA (siRNA) transfection. The cells were exposed to 25 nM UBE2C ON-TARGETplus SMARTpool siRNA (cat# L-004693-00-0005) or ON-TARGETplus non-targeting (NT) control siRNA (cat# D-001810-10-05; both purchased from Horizon Discovery, Cambridge, United Kingdom). Dharmafect 4 (Horizon Discovery) was used to deliver the siRNAs into the HUH6 cells using the protocol provided. Knockdown efficacy was evaluated at mRNA and at protein level 48 h after transfection.
2.11 RNA and protein extraction
Total RNA and protein were extracted from cultured HUH6 and HB-243 cell models using NucleoSpin RNA/Protein extraction kit (Macherey-Nagel, Düren, Germany). Instructions provided by the manufacturer were followed.
2.12 Quantitative real-time polymerase chain reaction
Reverse transcription was carried out using iScript cDNA Synthesis Kit (Bio-Rad, Hercules, CA, United States). Quantitative polymerase chain reaction (qPCR) was performed using PowerUp SYBR Green Master Mix (Thermo Fisher Scientific, Fremont, CA, United States). The geometric mean of B2M and PPIG served as a reference. Primer sequenced were designed as follows: B2M 5′- GAT GAG TAT GCC TGC CGT GT—3′ (forward), 5′- CTG CTT ACA TGT CTT GAT CCC A- 3′ (reverse); PPIG 5′ -CAA TGG CCA ACA GAG GGA AG—3′(forward), 5′—CCA AAA ACA TGA TGC CCA—3′ (reverse); UBE2C 5′- CCG CCC GTA AAG G—3′ (forward), 5′- CTC AGG TCT TCA TAT ACT GTT CCA G -3′ (reverse).
2.13 Western blotting
Equal amounts of protein (10 µg) were loaded into Mini-Protein TGX stain-free gels (Bio-Rad) and separated using gel electrophoresis. Proteins were transferred to polyvinyl-fluoride membrane and 5% skimmed milk in Tris-buffered saline-Tween20 was utilized to block unspecific binding. Primary antibody incubation was performed at room temperature for overnight (UBE2C at dilution 1:500; #14234S; Cell Signaling Technology Inc., Danvers, MA, United States). Secondary antibody incubation was carried out at room temperature for 1 h (goat anti-rabbit IgG at dilution 1:10,000; #111-035-144, Jackson ImmunoResearch, West Grove, PA, United States). Protein bands were visualized using Enhanced Chemiluminescence detection kit (Amersham ECL reagent; GE Healthcare, Barrington, IL, United States). Protein quantification was performed with Image Lab software (version 6.0, Bio-Rad) by normalizing UBE2C band intensities to amount of total protein in corresponding lane utilizing stain-free technology (Gürtler et al., 2013).
2.14 RNA sequencing of UBE2C silenced HUH6 cells
HUH6 cells were cultured and treated with either UBE2C or non-targeting siRNAs. RNA and protein extraction were conducted as described above. Prior to sequencing, RNA concentration, quality, and integrity were assessed using Qubit fluorometer (Thermo Fisher Scientific, Waltham, MA, United States) and the TapeStation system (Agilent, Glostrup, Denmark). After quality assessment, RNA libraries were constructed applying polyA selection, and Illumina compatible cDNA libraries were prepared by GENEWIZ (Leipzig, Germany). Samples were then sequenced on Illumina NovaSeq 6,000 yielding 2 × 150 bp paired end reads (GENEWIZ). Processing of RNA sequencing data was done using Chipster software as described above. The cut-off criteria were set to adjusted p-value <0.1. No cut-off criteria were used for logFC. Enrichr was used to identify enriched pathways and ontologies.
2.15 Cell viability assays
Cell viability after UBE2C knockdown was evaluated with cell proliferation agent WST-1 and clonogenic survival assay. The WST-1 assay (Roche Diagnostics GmbH, Basel, Switzerland) was performed according to the manufacturer’s instructions at timepoint of 48 h. For the clonogenic assay, HUH6 cells transfected with UBE2C or NT siRNAs were disaggregated into single-cell suspension and seeded at low density into 12-well plates. After culturing for 72 h, the cells were washed with phosphate-buffered saline (PBS), fixed with 4% paraformaldehyde, permeabilized with 100% methanol, stained with crystal violet and rinsed with dH2O. The area occupied by cell colonies in each well was calculated using Cell Profiler (McQuin et al., 2018).
2.16 Migration assay
Cell migration was assessed using transwell migration inserts (8 um pore size; Merck Millipore, Darmstadt, Germany). The bottom of each insert was pre-coated with collagen I (0.1 mg/mL; Sigma Aldrich, St. Louis, MO, United States) and placed into 24-well plates containing cell culture medium (10% serum). UBE2C or NT siRNA transfected cells were seeded to upper side of membrane in starvation medium (serum-free) (seeding density 5 × 105 cells per well). After culturing for 42 h, cells were fixed with 4% paraformaldehyde, permeabilized with 100% methanol, and stained with crystal violet. Non-migrated cells were removed from upper side of membrane with a cotton swab. In each insert, images were captured from five randomly chosen fields with Eclipse TS100 microscope supplemented with DS-Fi1 digital imaging system (magnification ×10; Nikon, Tokyo, Japan). The number of migrated cells was calculated with ImageJ software.
3 Results
3.1 Genes differentially expressed in HB cell lines vs. primary hepatocytes
The workflow is outlined in Figure 1. Five of the cell lines used in this study were established from aggressive HB tumors; the sixth was the immortalized human HB cell line, HUH6. Details of these cell lines are shown in Table 1 (Doi, 1976; Kats et al., 2019). RNA-sequencing analysis of these six cell lines identified approximately 9,000 differentially expressed genes (DEGs) in each cell line compared to primary hepatocytes (Figure 2A; Supplementary Table S2). Of these, approximately half were upregulated and half downregulated. Venn analysis showed that 2,868 of DEGs were shared among all 6 HB cell lines (Figure 2B). The top 20 most upregulated and most downregulated genes are shown in Figure 2C. The most upregulated genes were ODAM, TRIM71, and IGDCC3, while the most downregulated genes were SAA1, SAA2, and NNMT. Among the most upregulated genes were GPC3, DLK1, and SP8, previously connected to aggressive HB, underscoring the robustness of the analysis pipeline (Cairo et al., 2008; Zynger et al., 2008; Wagner et al., 2020).
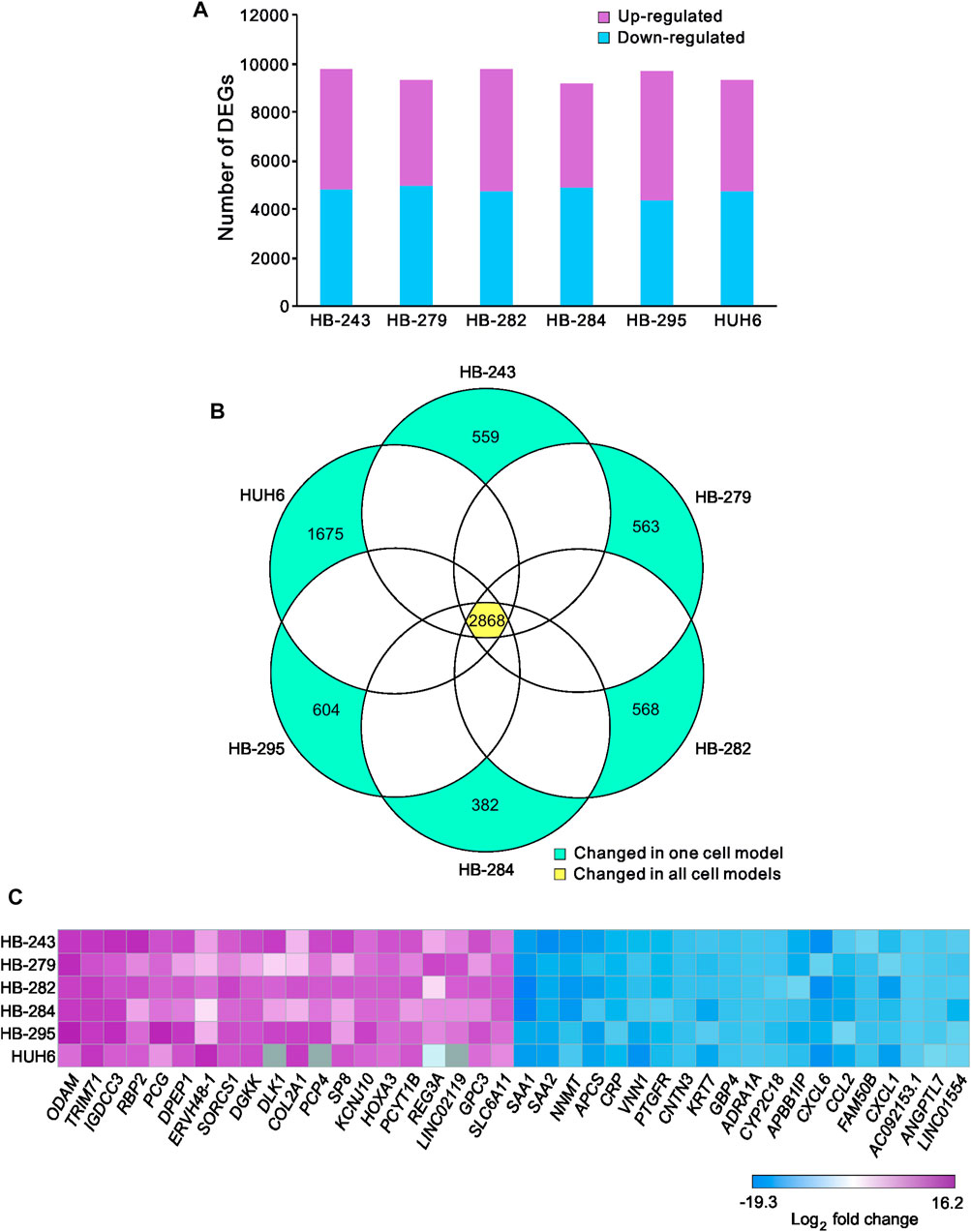
FIGURE 2. Differentially expressed genes in HB cell lines compared to primary hepatocytes Upregulated (pink) and downregulated (blue) genes in each HB cell line (A). Venn diagram showing the number of significant differentially expressed genes compared to primary hepatocytes that were shared among all six HB cell models (B). Heatmap of the 20 most downregulated and the 20 most upregulated differentially expressed genes sorted by log2FC (C). Genes in gray = change not statistically significant. Differential expression analysis was conducted using the edgeR package.
3.2 Differentially expressed metabolic genes
A list of known human metabolic genes was obtained from Virtual Human Metabolomics (Supplementary Table S1). Venn analysis showed that 490 of these metabolic genes were shared among all 6 HB cell lines (Figure 3A; Supplementary Table S4). Approximately 1,400 DEGs in each HB cell line were classified as metabolic genes (Figure 3B; Supplementary Table S3). The 20 most upregulated and most downregulated DEGs overlapping with metabolic genes are listed in Figure 3C. The most upregulated metabolism-associated genes were RBP2, DPEP1, and PCYT1B, and the most downregulated genes were NNMT, VNN1, and CYP2C18. Out of these six genes, DPEP1, responsible for hydrolysis of several dipeptides, and nicotinamide N-methyltransferase NNMT have been demonstrated to play a role in HB pathogenesis in previous studies (Cui et al., 2019; Rivas et al., 2020). Other genes were not previously reported in HB. These genes are involved in regulating oxidative phosphorylation (RBP2, VNN1), phosphatidylcholine biosynthesis (PCYT1B), and xenobiotic and retinoid metabolism (CYP2C18, RBP2) (Chen and Goldstein, 2012; Grinde et al., 2014; Váraljai et al., 2015; Giessner et al., 2018; Blaner et al., 2020).
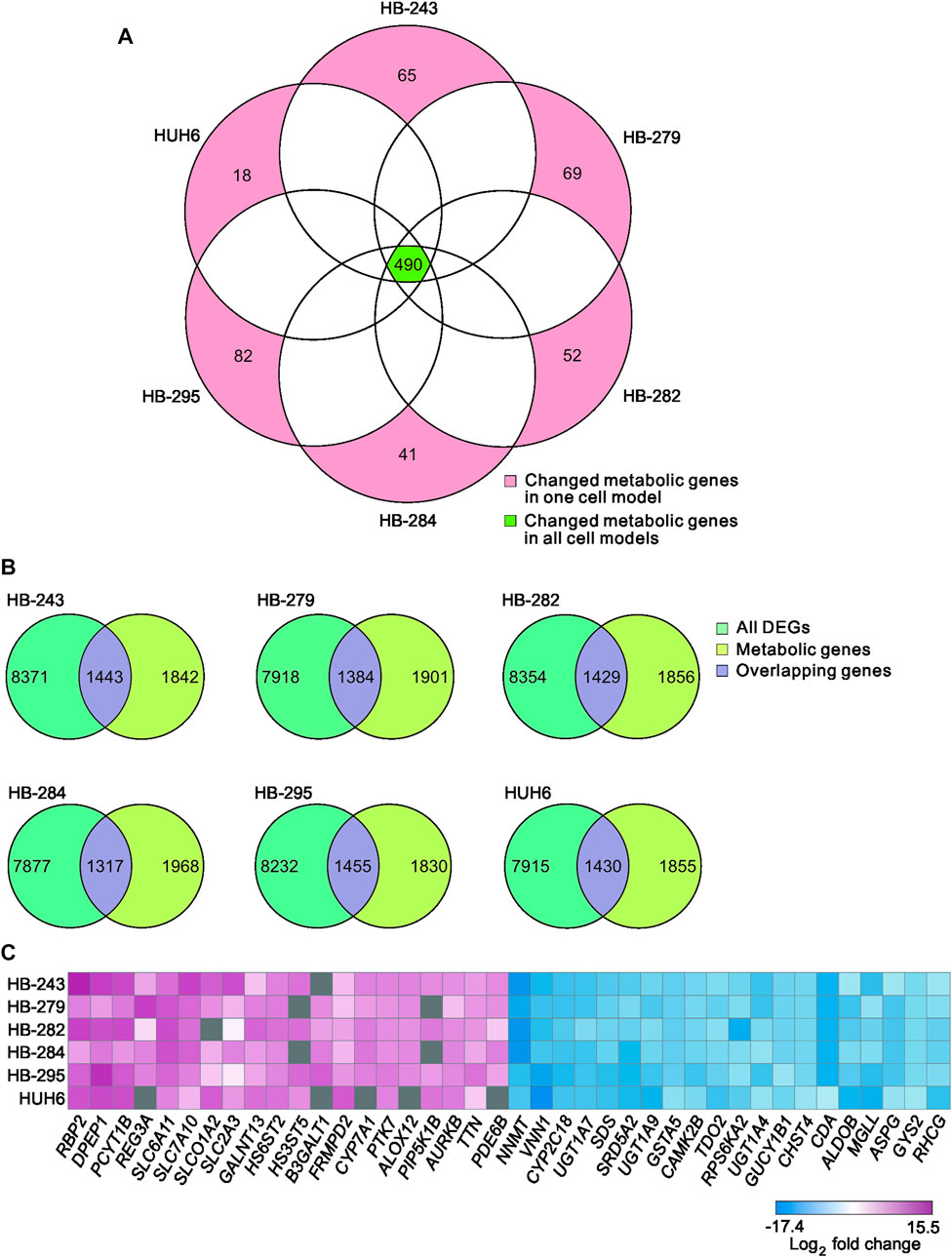
FIGURE 3. Differentially expressed metabolic genes in HB cell models Venn diagram showing the number of shared metabolic genes among the 6 HB cell lines (A). Overlap of differentially expressed genes in each cell line and the list of all metabolic genes (B). Heatmap of the 20 most up- and the 20 most downregulated metabolic genes in HB cell models (C). Genes in gray = change not statistically significant.
3.3 PPI-network construction and clustering
Protein-to-protein interaction (PPI) networks describe the physical contact of proteins within cells. PPI networks were constructed to better understand the changes in cell physiology represented by transcriptome analysis in HB. Lists of differentially expressed metabolic genes in each cell line (Supplementary Table S3) were used to construct the PPI networks using the STRING database. Highly interconnected areas (clusters) in each PPI-network were identified, scored, and ranked on size and density. The highest-scoring cluster of each cell line is shown in Figures 4A–F. The scores of the top clusters were 34 (HB-243), 24 (HB-279), 32 (HB-282), 29 (HB-284), 33 (HB-295) and 28 (HUH6). Upregulated genes found in one or more of these clusters included UBE2C, UBE2D1, UBE2N, UBE2O, UBE2Q1, UBE2Q2, UBE2R2, UBE2S, DZIP3, HACE1, MGRN1, MIB2, MYLIP, RBBP6, RNF126, RNF138, RNF182, SIAH2, SMURF2, WWP1, ZNRF1, and ZNRF2. Downregulated genes included CBLB, HECTD2, HECTD3, HECW2, HERC3, HERC4, LRSAM1, MKRN1, NEDD4, NEDD4L, PJA1, RCHY1, RNF115, RNF130, RNF14, RNF144B, RNF19A, RNF19B, RNF217, SH3RF1, SIAH1, SMURF1, STUB1, TRAF7, TRIM32, TRIP12, UBA1, UBA7, UBE2A, UBE2D4, UBE2E1, UBE2E2, UBE2E3, UBE2H, UBE2J1, UBE2J2, UBE2L6 UBE3C, UBR1, and UBR2.
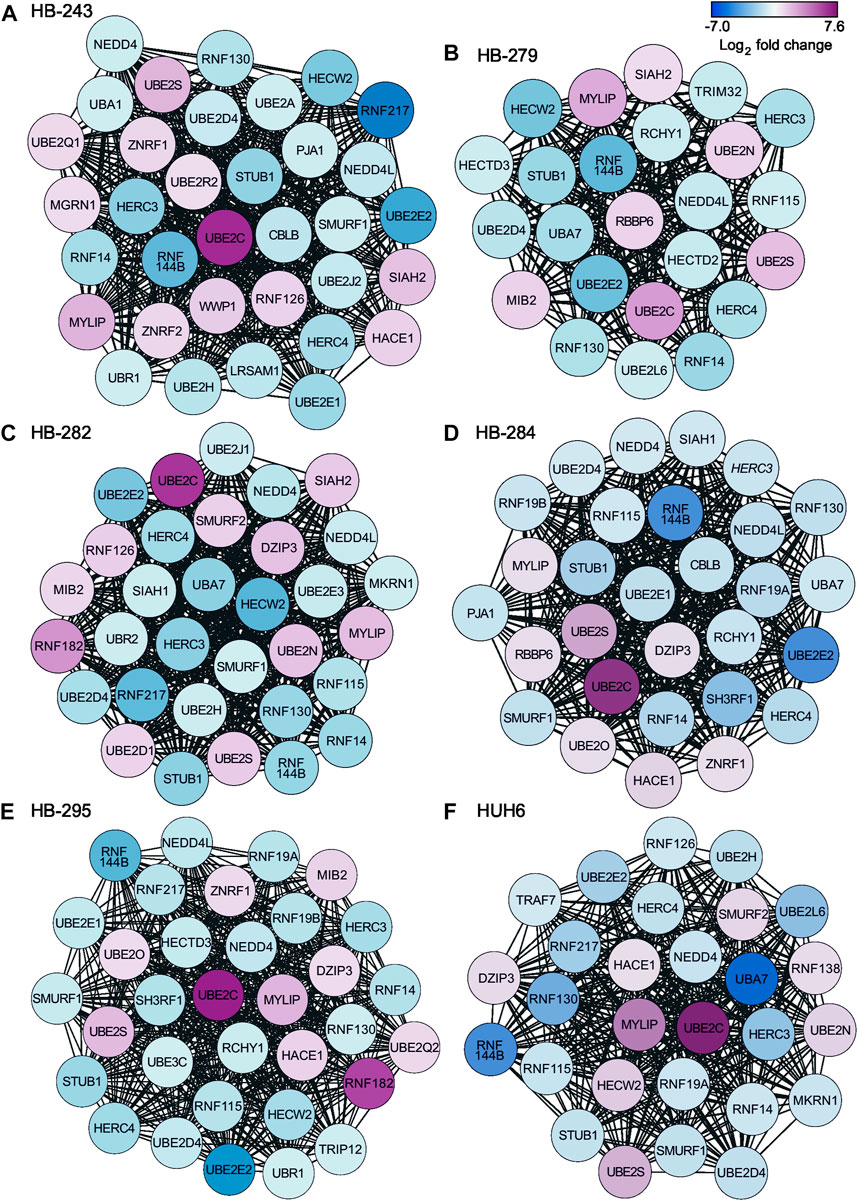
FIGURE 4. Highest-scoring clusters found in PPI networks of differentially expressed metabolic genes in each cell line. Clusters were ranked based on their score using MCODE plugin of Cytoscape. HB-243 (A), HB-279 (B), HB-282 (C), HB-284 (D), HB-295 (E), and HUH6 (F).
3.4 KEGG and GO analyses of the highest-ranking clusters
Next, to identify enriched pathways and gene sets, the list of protein-coding genes in each cell line’s highest-scoring PPI cluster were uploaded to the online tool Enrichr and the results ranked by p-value. The top 5 most statistically significant GO-terms in the highest-ranking clusters were protein ubiquitination, protein polyubiquitination, protein modification by small protein conjugation, modification-dependent protein catabolic processes, and protein ubiquitination involved in ubiquitin-dependent protein catabolic processes (Figure 5A). The corresponding KEGG-terms were ubiquitin mediated proteolysis, endocytosis, protein processing in endoplasmic reticulum, Hedgehog signaling pathway, TGF-β signaling pathway, and Parkinson’s disease (Figure 5B).

FIGURE 5. KEGG and GO analyses for enriched pathways. Genes in each cell line’s top-ranking PPI clusters were analyzed to find common enriched pathways. Statistically significant (p < 0.05) GO biological processes (A) and KEGG pathways (B) are shown for each cell line.
3.5 Validation of findings with HB patient microarray dataset
Genes present in all six of the highest-scoring clusters in the PPI-network were RNF130, UBE2E2, UBE2C, RNF14, HERC3, HERC4, STUB1, UBE2S, RNF144B, MYLIP, and UBE2D4. Of these, four genes—RNF130, UBE2C, HERC3, and RNF144B—were found to be significantly altered in the GSE131329 microarray dataset. This dataset includes 53 HB tissue samples and 14 noncancerous liver (NCL) tissue samples collected at the time of surgery from HB patients. RNF144B, RNF130, and HERC3 mRNA expression was downregulated compared to the normal liver samples (log2FC −0.84, adj. p-value 1.10*10−5; log2FC −0.6, adj. p-value 3.0*10−06, and log2FC −0.62, adj. p-value 0.00186, respectively), whereas UBE2C mRNA expression was upregulated in HB samples (log2FC 1.0; adj. p-value 0.0001). RNF144B, RNF130, and HERC3 act as a ubiquitin ligases and UBE2C is an ubiquitin conjugating enzyme. Expression of these 4 genes in both HB and NCL groups is shown in Figures 6A–D.
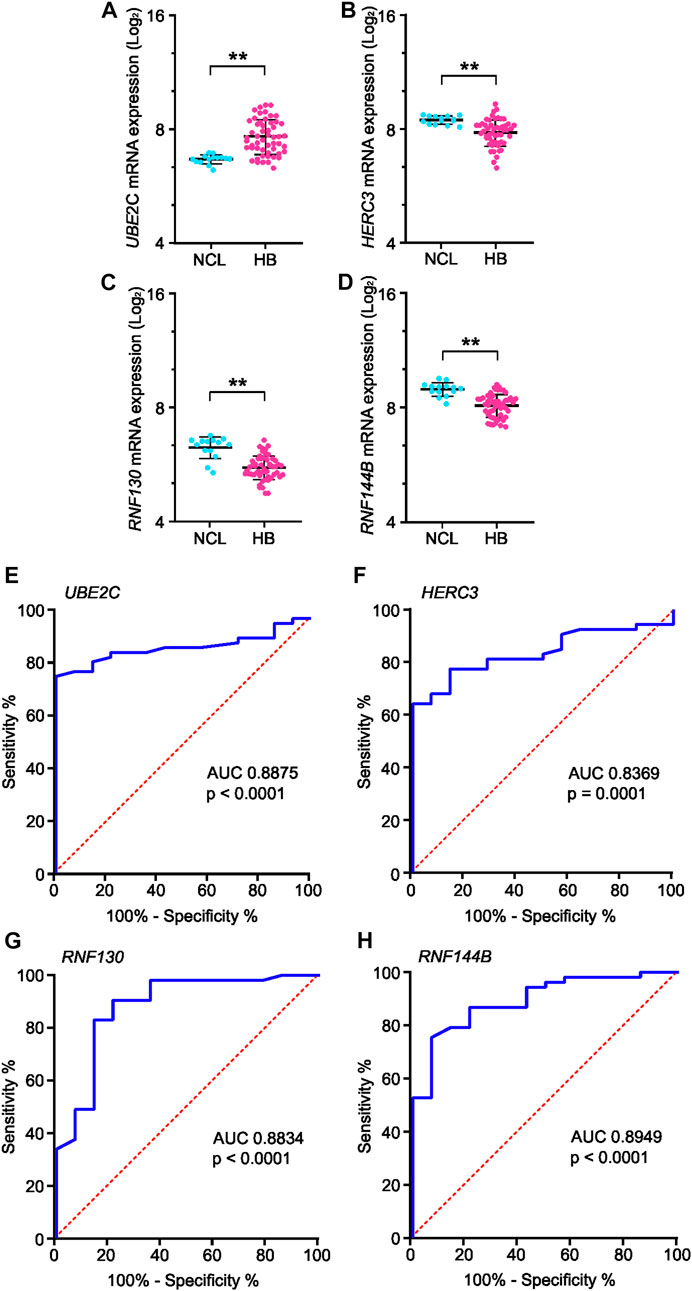
FIGURE 6. mRNA expression and ROC curve analysis of the four key genes in GSE131329 dataset. Expression of UBE2C (A), HERC3 (B), RNF130 (C) and RNF144B (D) in HB samples compared to noncancerous liver samples on mRNA level. ROC curve analysis of UBE2C (E), HERC3 (F), RNF130 (G) and RNF144B (H) assessing the suitability of each gene for discrimination of noncancerous liver (NCL) and HB. ** = p < 0.01. Grey dots represent gene expression of independent patients, the whiskers represent the first and third quartile, and the thick solid line is median (A–D).
3.6 Clinical analyses of potential key genes UBE2C, RNF130, HERC3, and RNF144B
To analyze the suitability of each selected gene (UBE2C, RNF130, HERC3, and RNF144B) to discriminate normal control liver samples (NCL) and HB, we performed ROC curve analysis. The area under curve (AUC) was 0.8875 for UBE2C (Figure 6E, 95% CI 0.81–0.96), 0.8369 for HERC3 (Figure 6F, 95% CI 0.74–0.93), 0.8834 for RNF130 (Figure 6G, 95% CI 0.77–0.99), and 0.8949 for RNF144B (Figure 6H, 95% CI 0.81–0.98). Next, we assessed the association of each of these genes with distant metastasis status, occurrence of events, and overall survival. High UBE2C mRNA expression was linked with distant metastasis (p-value <0.01), events (p-value <0.05), and death (p-value <0.01) (Table 2). Downregulation of HERC3 and RNF144B was associated with occurrence of events (p-values <0.05) (Table 2). RNF130 expression did not show a statistically significant association with any of the studied variables (Table 2). Clinical information adapted from GSE131329 dataset is summarized in Table 3.
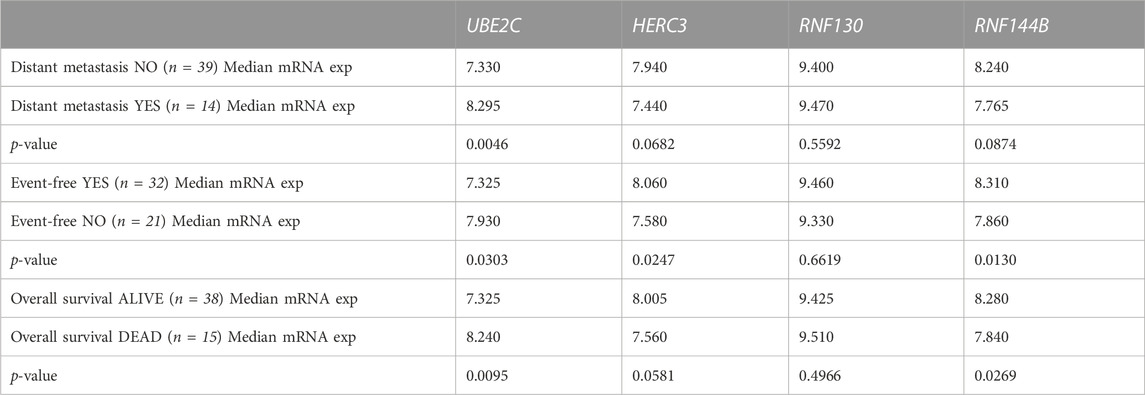
TABLE 2. Association of UBE2C, HERC3, RNF130, and RNF144B mRNA expression (log2) with clinical course of the disease assessed with Mann-Whitney U test.
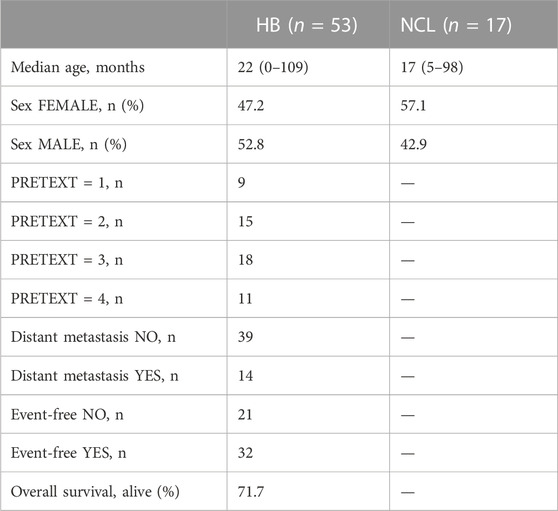
TABLE 3. Clinical information of GSE131329 dataset. (HB = hepatoblastoma; NCL = non-cancerous liver).
3.7 UBE2C associated protein interactions and gene co-expression analysis
To analyze the functional enrichment of UBE2C specific protein interactions in general, STRING network analysis was carried out. UBE2C was used as the input protein. Thirty proteins were interacting with UBE2C when the cut-off was set to 0.4 (Figure 7A). Next, we assessed the level of differential RNA expression of these UBE2C interacting proteins in our RNA sequencing data of the six HB cell models. Of these 30 proteins, fourteen were found to be significantly differentially expressed on RNA level in all 6 HB models (Figure 7B).
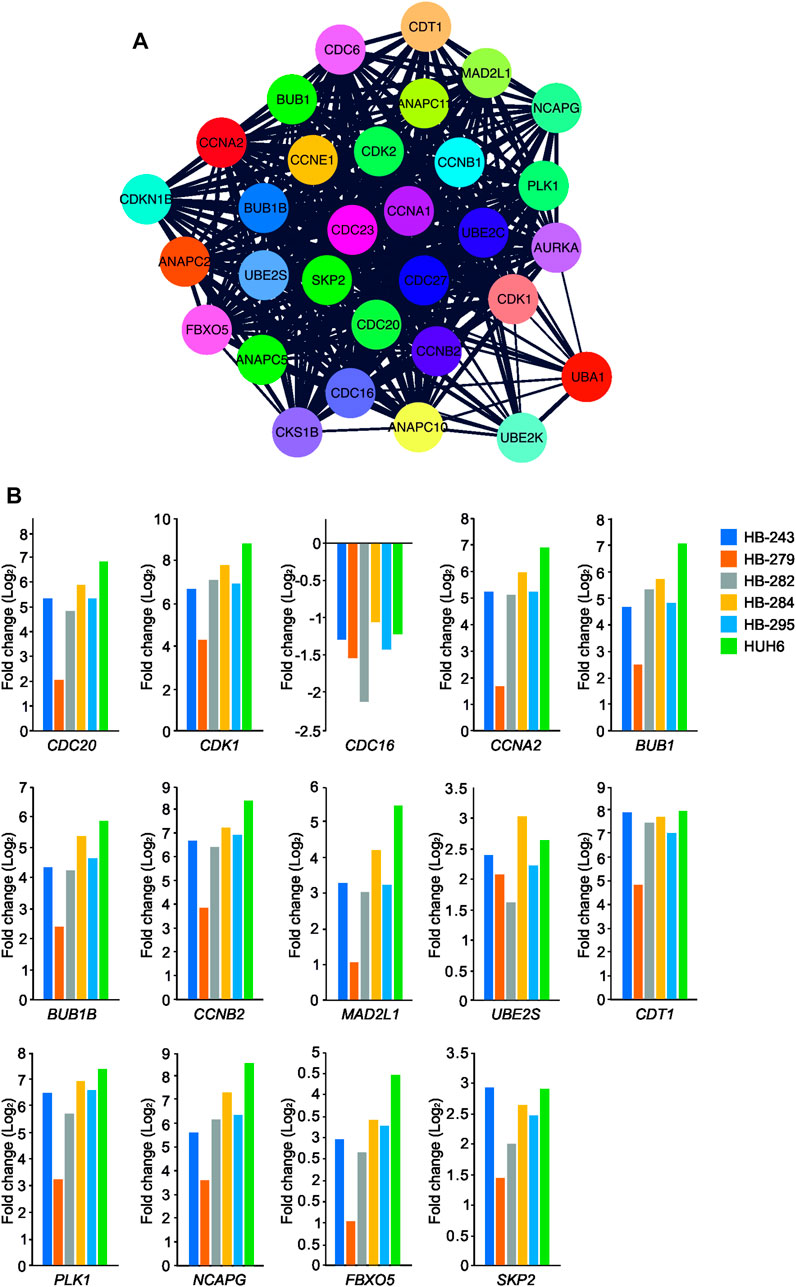
FIGURE 7. UBE2C protein-protein interaction network. UBE2C associated protein-protein interactions (A). The fold change of UBE2C interacting DEGs (in relation to primary hepatocyte) emerging from our RNA sequencing analysis (B).
Gene co-expression analysis was conducted for UBE2C using the online tool GeneFriends to further explore the relationship between UBE2C and related genes. Using UBE2C as the seed gene, top 10 co-expressed genes with the highest Pearson correlation values were CCNB2, TOP2A, NUSAP1, CKS2, NUF2, CDK1, NEK2, PTTG1, CKS1B, and lncRNA RP11-102C16.3 (Supplementary Figure S1A–B). When comparing these protein interactions and gene co-expression results of UBE2C, three genes/proteins, CKS1B, CCNB2 and CDK1, were present in both networks.
3.8 Immunohistochemical staining of UBE2C in HB patient samples
To validate UBE2C protein expression in HB patient samples, immunohistochemical staining was performed on 6 NL (Table 4) and 25 HB (Table 5) samples. One of the 6 NL samples showed positive UBE2C staining on the cell membranes (Figures 8A, B). Of the HB samples, 5 were considered UBE2C-negatives (Figures 8C, D) and 20 UBE2C positive (Figures 8E, F). Compared to NL samples, staining for UBE2C in HB cells appeared stronger and localized to nuclei rather than the cell membrane.
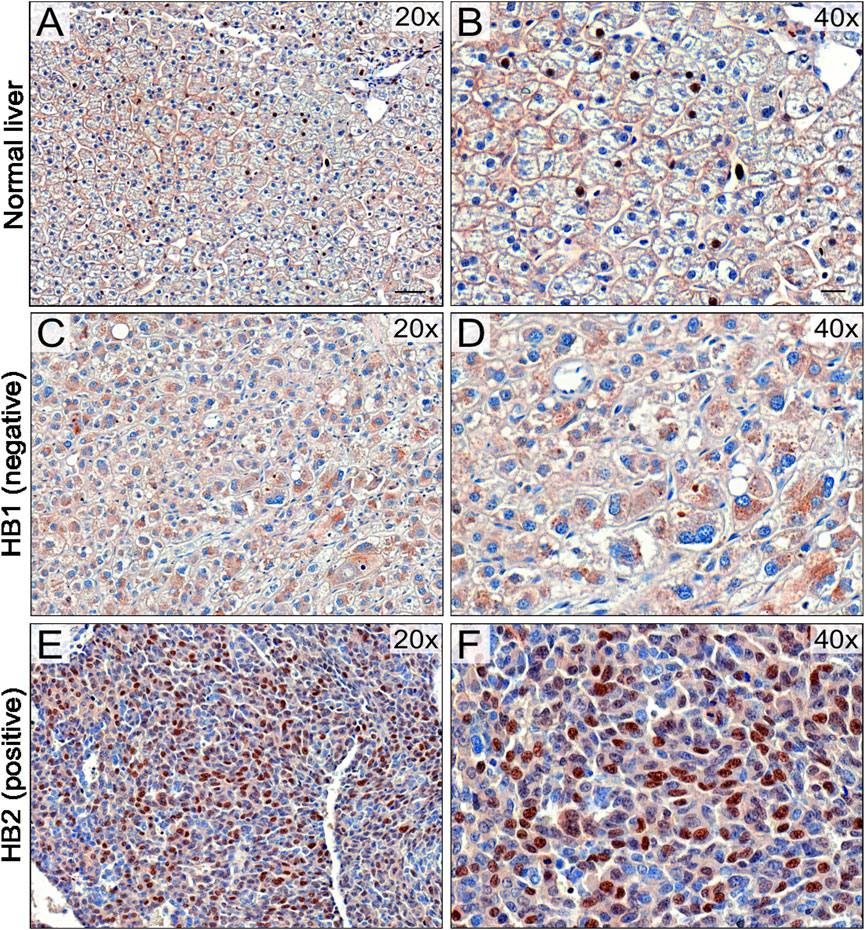
FIGURE 8. UBE2C protein expression in HB tissues. Immunohistochemical staining of UBE2C in 6 normal liver (NL) samples and 25 hepatoblastoma (HB) samples was done. Representative image of normal liver stained with UBE2C with ×20 (A) and ×40 (B) magnification. Representative image of HB liver staining negative for UBE2C with ×20 (C) and ×40 (D) magnification. Representative sample of HB liver staining positive for UBE2C ×20 (E) and ×40 (F) magnification. Scale bars = 50 µm (B,D) and 20 µm (C,E).
3.9 UBE2C silencing decreases cell viability and migration in HUH6 and HB-243 HB cell models
To explore UBE2C function in vitro, UBE2C was silenced in HUH6 and HB-243 cell lines using siRNA transfection. Non-targeting (NT) siRNAs were used as a control. After siRNA transfections, UBE2C expression was reduced 95% at mRNA and 80% at protein level in HUH6 (Figures 9A, B) and 98% at mRNA and 80% at protein level in HB-243. (Figures 9D, E). The effect of UBE2C knockdown on cell viability was evaluated using WST-1 assay. Relative cell viability decreased 24% in HUH6 (Figure 9C) and 44% in HB-243 (Figure 9F).
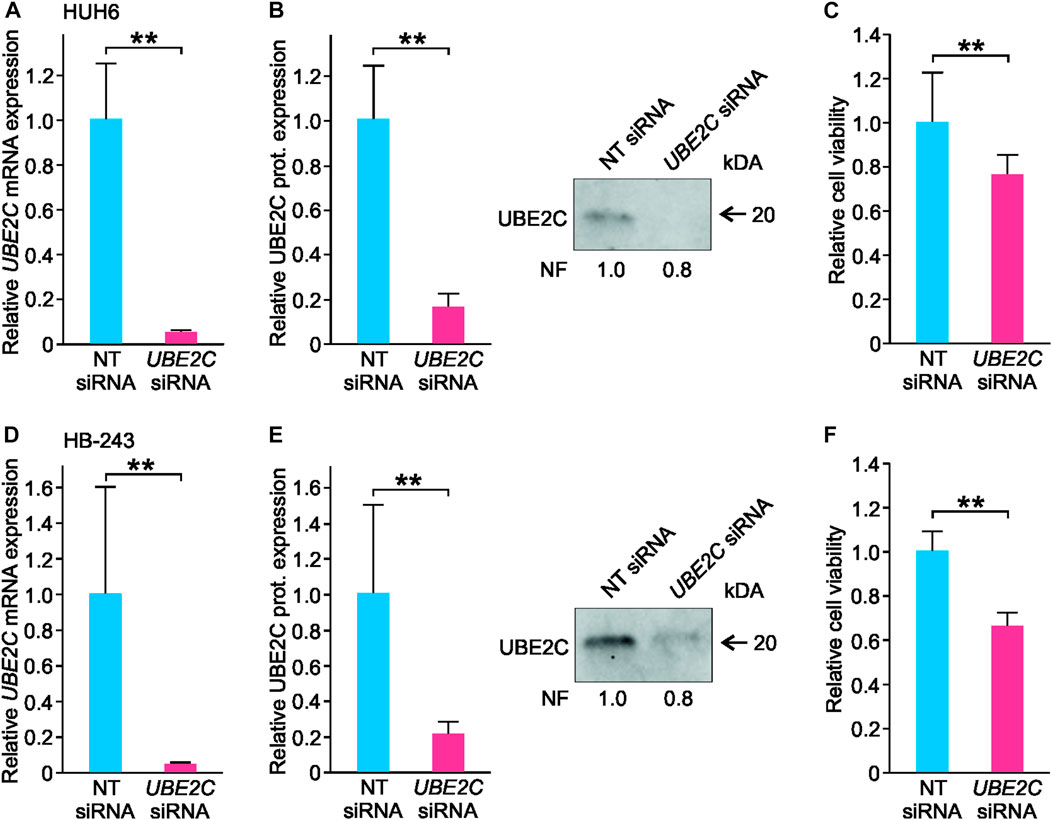
FIGURE 9. Knockdown of UBE2C and its effect on HUH6 and HB-243 cell viability. Following the siRNA transfection, UBE2C mRNA expression in HUH6 was reduced by 95% (A) and protein expression by 80% (B) compared to cells transfected with non-targeting (NT) siRNA. In HB-243, UBE2C mRNA expression was reduced by 98% (D) and protein expression by 80% (E). WST-1 assay showed a 24% decrease in HUH6 (C) and 44% in HB-243 (F) in cell viability after UBE2C silencing. Bar plots are presented as relative values of mean of three independent experiments ± RSD. **p-value <0.01, NT = non-targeting. Normalization factor (NF) describing the amount of total protein in lane in relation to other lanes is given beneath the bands (B, E).
RNA sequencing of UBE2C silenced HUH6 cells showed 111 differentially expressed genes (Supplementary Table S5). Top 5 pathways and ontologies matching these genes included cell cycle related processes such as p53 regulation, DNA damage response, and G1/S checkpoint (Figures 10A–F).
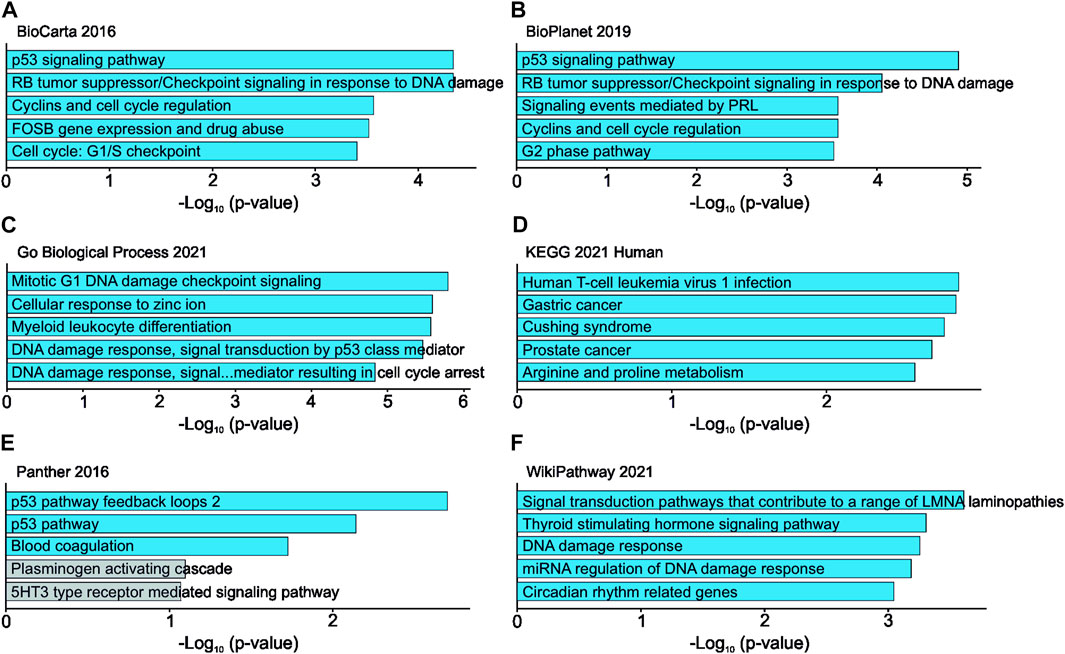
FIGURE 10. Effects of UBE2C knockdown in HUH6 cells on RNA level. UBE2C knockdown is linked with alterations in RNA expression of genes connected to cell cycle regulation and p53 signaling pathway. Top 5 pathways and ontologies ranked by p-value (A–F).
Effects of UBE2C knockdown in HUH6 cells were further evaluated using a clonogenic assay, which showed a statistically significant fall in cell number in UBE2C silenced cells with the well area covered by cells decreasing 35% (Supplementary Figure S2A–C). The effect of UBE2C silencing on HUH6 cell migration was assessed with transwell assay, which demonstrated a statistically significant 65% decrease in the number of migrated cells compared to NT siRNA treated cells (Supplementary Figure S2D–F).
4 Discussion
Metabolic derangements have been associated with enhanced tumorigenesis and cancer progression in several tumor entities (Faubert et al., 2020), and these tumor-specific changes can be exploited to develop targeted therapies (Sullivan et al., 2016). In our RNA sequencing analysis of HB cell models, ubiquitination emerged as the most significantly altered metabolic pathway. The expression of three ubiquitin ligases (HERC3, RNF130, and RNF144A) and one ubiquitin conjugating enzyme (UBE2C) was significantly dysregulated in all studied HB models. These four genes were assessed more thoroughly in a HB patient dataset to validate their significance in the clinical setting. We noticed a remarkable association between high UBE2C expression and aggressive disease in the particular HB patient cohort.
Ubiquitination is a crucial mechanism for the degradation of short-lived proteins like those involved in cell cycle regulation (Guo et al., 2019; Zhang et al., 2021). In addition to protein degradation, ubiquitination regulates DNA repair, translation, and inflammation (Miranda and Sorkin, 2007). The three main steps in ubiquitination are activation (performed by ubiquitin-activating enzymes, E1s), conjugation (ubiquitin-conjugating enzymes, E2s), and ligation (ubiquitin-ligating enzymes, E3s) (Komander and Rape, 2012). Ubiquitination and deubiquitination are known to be modulated during cancer progression (Sun et al., 2020), and high UBE2C expression portends poor survival in various cancers including node-positive breast cancer (Loussouarn et al., 2009) and ovarian carcinomas (Berlingieri et al., 2007). The magnitude of UBE2C mRNA overexpression in HB cell lines was striking (up to 128-fold higher than primary hepatocytes), and UBE2C expression in HB clinical specimens was associated with increased risk of distant metastasis, events, and death. Our findings echo a recent study showing that UBE2C expression may be used as a diagnostic biomarker in hepatocellular carcinoma, the most frequent liver cancer in adults (Gao et al., 2021).
In HB tissue samples, we observed predominantly nuclear localization of UBE2C protein. In other cancers, both nuclear and cytoplasmic UBE2C immunoreactivity have been observed, but the functional significance of this is unclear (Shen et al., 2013; Ma et al., 2016; Palumbo et al., 2016). Kraft et al. showed that strong nuclear expression of UBE2C was linked with higher mitosis rate in melanoma suggesting that UBE2C localization in nuclei may be at least partially related to its role in the regulation of cell cycle associated proteins (Kraft et al., 2017).
It has previously been shown that in cancer cells UBE2C plays an important role in facilitation of protein degradation and dysregulation of the cell cycle (Sun et al., 2020). UBE2C overexpressing cells have the ability to override mitotic spindle checkpoints, which may lead to loss of genomic stability, a characteristic of cancer (Reddy et al., 2007). UBE2C is also suggested to be a potential oncogene enhancing migration and invasion in hepatocellular carcinoma (Xiong et al., 2019). Consistently, we demonstrated that knockdown of UBE2C resulted in a decrease in HB viability, and preliminary results suggest that it could also have a negative effect on HB cell migration. Our RNA sequencing results supported the hypothesis that UBE2C participates in cell cycle regulation in HB. After UBE2C knockdown, we observed alterations in mRNA expression of CDKN1A, CDK, PIK3C2B, PIDD1, and E2F2 genes which are known to participate in cell cycle regulation and the p53 signaling pathway. Changes in mRNA expression, however, were rather subtle. Effects of UBE2C knockdown on cell cycle were, however, not assessed with in vitro experiments in this article. Given the role of UBE2C as a post-translational factor rather than a direct regulator of gene expression, proteomics analysis could be conducted in future experiments. UBE2C overexpression has also previously been linked to increased ubiquitination and subsequent degradation of the tumor suppressor p53 in endometrial cancer (Liu et al., 2020). A novel therapy aimed at enhancing p53 activity has been suggested to be a potential treatment alternative for HB (Woodfield et al., 2021). If UBE2C participates in post-translational regulation of p53 expression in HB, its inhibition could lead to reactivation of p53.
Aurora Kinase A (AURKA), a serine/threonine kinase, has a critical role in regulating cell cycle and mitosis (Du et al., 2021). Expression of AURKA has been shown to be significantly higher in HB than in normal liver (Tian et al., 2021). In our study, AURKA was significantly upregulated in all studied HB cell lines. Treatment modalities targeting AURKA, such as alisertib, have shown promising results in preclinical studies of HB (Tan et al., 2020). Interestingly, increased AURKA expression has been demonstrated to correlate with upregulated UBE2C in cancer cells (http://gepia.cancer-pku.cn) (Naso et al., 2021). Furthermore, inhibition of UBE2C expression was shown to reduce the level of phosphorylation of AURKA and impair cell viability in gastric adenocarcinoma cells (Wang et al., 2017).
UBE2C links to another key HB gene, cyclin-dependent kinase 1 (CDK1) (Aghajanzadeh et al., 2020; Sun et al., 2021; Tian et al., 2021). CDK1 functions as a serine/threonine kinase and, like AURKA, plays an important role in cell cycle regulation. CDK1 has been reported to be upregulated in various cancers including hepatocellular carcinoma (Zhou et al., 2019). Consistent with previous studies, our RNA-sequencing results showed that CDK1 was highly upregulated in HB cell models. CDK1 siRNA knockdown was shown to inhibit the growth and invasiveness of HUH6 HB cells (Tian et al., 2021). A study of ovarian cancer cells showed that high UBE2C expression correlated with expression of CDK1. Knockdown of UBE2C induced G2/M arrest in the cells, which led to decreased CDK1 expression (Li et al., 2020). In our study, knockdown of UBE2C in HUH6 cells increased cyclin-dependent kinase inhibitor 1 (CDKN1A) expression and decreased cyclin-dependent kinase 2 (CDK2) expression at mRNA level, both of these having a role in G1/S transition. CDK1 expression was not significantly altered.
There is some evidence that UBE2C overexpression impacts chemoresistance. Downregulation of UBE2C reversed resistance to cisplatin in ovarian cancer cell models (Li et al., 2020). UBE2C inhibition has been shown to increase doxorubicin sensitivity in breast cancer cells in vitro (Rawat et al., 2013). Cisplatin and doxorubicin are both widely used in HB management. In addition to targeted treatment, UBE2C expression status could thus be utilized in the evaluation of treatment resistance to conventional chemotherapy in HB. The proteasome inhibitor bortezomib slows HB progression in vitro and in vivo (Hooks et al., 2018). In colorectal carcinoma, bortezomib treatment has been demonstrated to downregulate UBE2C expression leading to decreased cell viability via stabilizing mitotic cyclins and inhibiting cell cycle progression (Bavi et al., 2011). Thus, high UBE2C expression could identify HB patients who may benefit from bortezomib treatment.
There are some limitations to this study. The reader should note that in this article, both mRNA- and protein expression of the genes in question are being used. While changes in mRNA-expression often correlate with changes in protein expression, this is not always the case given the several factors affecting the translation process and the final amount of protein in the tissue. This should be kept in mind while interpretating the results. In this study we have used immunohistochemistry and Western blotting to determine the protein expression levels of UBE2C in HB tissues. More extensive proteomics would, however, be required to further elucidate the actual protein expression levels of all the genes related to UBE2C. Liver matures throughout childhood, and the use of primary hepatocytes from adult donor as control cells in RNA sequencing analyses of the PDX models may have impacted our results. The noted effects of UBE2C silencing on mRNA expression level of cell cycle regulating genes should be further validated with in vitro and in vivo experiments in order to properly assess the effects on cell cycle. The number of HB patient samples available for this study limited clinical analyses and conclusions. This is unfortunately the case with most studies concerning HB, since the prevalence of HB is low and the availability of samples therefore limited.
Given the promising role of ubiquitin system as a target of new cancer treatments, the role and function of UBE2C in HB progression should be investigated further. The possible role of UBE2C and other ubiquitination-mediating enzymes in drug resistance is also intriguing. One possible technique that could be utilized is single-cell RNA sequencing (scRNAseq). Previously, Bondoc et al. have characterized HB tumor cell populations and identified driver tumor cell clusters using scRNAseq (Bondoc et al., 2021). Given the advantages of the technique, analysis of scRNAseq could provide new insight.
Taken together, we found that metabolic alterations taking place in HB tumors are diverse and that ubiquitination-related factors may have a significant role in HB progression. Notably, UBE2C expression was highly upregulated in all six HB cell lines as well as in patient samples at both mRNA and protein level. In vitro knockdown of UBE2C resulted in decreased cell division and motility. Moreover, high UBE2C expression was associated with inferior patient survival. These findings may be brought to the clinic to identify the high-risk HB patients for earlier treatment interventions.
Data availability statement
The datasets presented in this study can be found in online repositories. The names of the repository/repositories and accession number (s) can be found at https://www.ncbi.nlm.nih.gov/geo/ (GSE140520, GSE83518, GSE131329) and https://ega-archive.org/ (EGAS00001004827/EGAD00001006621).
Ethics statement
The studies involving human participants were reviewed and approved by the Helsinki University Hospital institutional ethics committee. Written informed consent to participate in this study was provided by the participants’ legal guardian/next of kin.
Author contributions
Conceptualization, KE and MP; formal analysis, RN and KE; funding acquisition, MH and MP; investigation, RN and KE; methodology, RN, KE, NI, and MP; project administration, KE and MP; resources, SC and MH; software, NI; supervision, KE, DW, MH, and MP; validation, RN; visualization, NI and MP; writing—original draft, RN; writing—review and editing, RN, KE, NI, SC, DW, MH, and MP. All authors contributed to the article and approved the submitted version.
Funding
This study was supported by Biomedicum Helsinki Foundation Grants, Finska Läkaresällskapet, Helsinki University Central Hospital Research Grants, Päivikki and Sakari Sohlberg Foundation, Sigrid Jusélius Foundation, and Väre Foundation for Pediatric Cancer Research.
Conflict of interest
Author SC is employed by Champions Oncology and was previously employed by XenTech.
The remaining authors declare that the research was conducted in the absence of any commercial or financial relationships that could be construed as a potential conflict of interest.
Publisher’s note
All claims expressed in this article are solely those of the authors and do not necessarily represent those of their affiliated organizations, or those of the publisher, the editors and the reviewers. Any product that may be evaluated in this article, or claim that may be made by its manufacturer, is not guaranteed or endorsed by the publisher.
Supplementary material
The Supplementary Material for this article can be found online at: https://www.frontiersin.org/articles/10.3389/fgene.2023.1170940/full#supplementary-material
References
Aghajanzadeh, T., Tebbi, K., and Talkhabi, M. (2020). Identification of potential key genes and miRNAs involved in Hepatoblastoma pathogenesis and prognosis. J. Cell. Commun. Signal 151 (15), 131–142. doi:10.1007/S12079-020-00584-1
Anders, S., Pyl, P. T., and Huber, W. (2015). HTSeq-a Python framework to work with high-throughput sequencing data. Bioinformatics 31, 166–169. doi:10.1093/bioinformatics/btu638
Armengol, C., Cairo, S., Fabre, M., and Buendia, M. A. (2011). Wnt signaling and hepatocarcinogenesis: The hepatoblastoma model. Int. J. Biochem. Cell. Biol. 43, 265–270. doi:10.1016/j.biocel.2009.07.012
Aronson, D. C., and Meyers, R. L. (2016). Malignant tumors of the liver in children. Semin. Pediatr. Surg. 25, 265–275. doi:10.1053/j.sempedsurg.2016.09.002
Bavi, P., Uddin, S., Ahmed, M., Jehan, Z., Bu, R., Abubaker, J., et al. (2011). Bortezomib stabilizes mitotic cyclins and prevents cell cycle progression via inhibition of UBE2C in colorectal carcinoma. Am. J. Pathol. 178, 2109–2120. doi:10.1016/j.ajpath.2011.01.034
Bader, G. D., and Hogue, C. W. V. (2003). An automated method for finding molecular complexes in large protein interaction networks. BMC Bioinforma. 4, 2. doi:10.1186/1471-2105-4-2
Berlingieri, M. T., Pallante, P., Guida, M., Nappi, C., Masciullo, V., Scambia, G., et al. (2007). UbcH10 expression may be a useful tool in the prognosis of ovarian carcinomas. Oncogene 26 (14), 2136–2140. doi:10.1038/sj.onc.1210010
Blaner, W. S., Brun, P. J., Calderon, R. M., and Golczak, M. (2020). Retinol-binding protein 2 (RBP2): Biology and pathobiology. Crit. Rev. Biochem. Mol. Biol. 55 (2), 197–218. doi:10.1080/10409238.2020.1768207
Bondoc, A., Glaser, K., Jin, K., Lake, C., Cairo, S., Geller, J., et al. (2021). Identification of distinct tumor cell populations and key genetic mechanisms through single cell sequencing in hepatoblastoma. Commun. Biol. 4, 1049. doi:10.1038/S42003-021-02562-8
Cairo, S., Armengol, C., Reyniès, A. D., Wei, Y., Thomas, E., Renard, C. A., et al. (2008). Hepatic stem-like phenotype and interplay of Wnt/beta-catenin and Myc signaling in aggressive childhood liver cancer. Cancer Cell. 14, 471–484. doi:10.1016/J.CCR.2008.11.002
Chen, E. Y., Tan, C. M., Kou, Y., Duan, Q., Wang, Z., Meirelles, G. V., et al. (2013). Enrichr: Interactive and collaborative HTML5 gene list enrichment analysis tool. BMC Bioinforma. 14, 128. doi:10.1186/1471-2105-14-128
Chen, Y., and Goldstein, J. (2012). The transcriptional regulation of the human CYP2C genes. Curr. Drug Metab. 10, 567–578. doi:10.2174/138920009789375397
Counihan, J. L., Grossman, E. A., and Nomura, D. K. (2018). Cancer metabolism: Current understanding and therapies. Chem. Rev. 118, 6893–6923. doi:10.1021/ACS.CHEMREV.7B00775
Crippa, S., Ancey, P., Vazquez, J., Angelino, P., Rougemont, A. L., Guettier, C., et al. (2017). Mutant CTNNB 1 and histological heterogeneity define metabolic subtypes of hepatoblastoma. EMBO Mol. Med. 9, 1589–1604. doi:10.15252/emmm.201707814
Cui, X., Liu, X., Han, Q., Zhu, J., Li, J., Ren, Z., et al. (2019). DPEP1 is a direct target of miR-193a-5p and promotes hepatoblastoma progression by PI3K/Akt/mTOR pathway. Cell. Death Dis. 10, 701–716. doi:10.1038/s41419-019-1943-0
Doi, I. (1976). Establishment of a cell line and its clonal sublines from a patient with hepatoblastoma. Gan 67, 1–10.
Du, R., Huang, C., Liu, K., Li, X., and Dong, Z. (2021). Targeting AURKA in cancer: Molecular mechanisms and opportunities for cancer therapy. Mol. Cancer 20, 15. doi:10.1186/s12943-020-01305-3
Faubert, B., Solmonson, A., and DeBerardinis, R. J. (2020). Metabolic reprogramming and cancer progression. Science 80, eaaw5473. doi:10.1126/science.aaw5473
Feng, J., Polychronidis, G., Heger, U., Frongia, G., Mehrabi, A., and Hoffmann, K. (2019). Incidence trends and survival prediction of hepatoblastoma in children: A population-based study. Cancer Commun. 39, 62. doi:10.1186/s40880-019-0411-7
Gao, S., Gang, J., Yu, M., Xin, G., and Tan, H. (2021). Computational analysis for identification of early diagnostic biomarkers and prognostic biomarkers of liver cancer based on GEO and TCGA databases and studies on pathways and biological functions affecting the survival time of liver cancer. BMC Cancer 21 (1), 791. doi:10.1186/S12885-021-08520-1
Giessner, C., Millet, V., Mostert, K. J., Gensollen, T., Vu Manh, T. P., Garibal, M., et al. (2018). Vnn1 pantetheinase limits the Warburg effect and sarcoma growth by rescuing mitochondrial activity. Life Sci. Alliance 1, e201800073. doi:10.26508/LSA.201800073
Grinde, M. T., Skrbo, N., Moestue, S. A., Rødland, E. A., Borgan, E., Kristian, A., et al. (2014). Interplay of choline metabolites and genes in patient-derived breast cancer xenografts. Breast Cancer Res. 16, R5. doi:10.1186/BCR3597
Guo, J., Wang, M., Wang, J-P., and Wu, C-X. (2019). Ubiquitin-conjugating enzyme E2T knockdown suppresses hepatocellular tumorigenesis via inducing cell cycle arrest and apoptosis. World J. Gastroenterol. 25, 6386–6403. doi:10.3748/WJG.V25.I43.6386
Gürtler, A., Kunz, N., Gomolka, M., Hornhardt, S., Friedl, A. A., McDonald, K., et al. (2013). Stain-Free technology as a normalization tool in Western blot analysis. Anal. Biochem. 433, 105–111. doi:10.1016/j.ab.2012.10.010
Harris, C. R., Millman, K. J., van der Walt, S. J., Gommers, R., Virtanen, P., Cournapeau, D., et al. (2020). Array programming with NumPy. Nat 585, 357–362. doi:10.1038/s41586-020-2649-2
Hooks, K. B., Audoux, J., Fazli, H., Lesjean, S., Ernault, T., Dugot-Senant, N., et al. (2018). New insights into diagnosis and therapeutic options for proliferative hepatoblastoma. Hepatology 68, 89–102. doi:10.1002/hep.29672
Hunter, J. D. (2007). Matplotlib: A 2D graphics environment. Comput. Sci. Eng. 9, 90–95. doi:10.1109/MCSE.2007.55
Irizarry, R. A., Hobbs, B., Collin, F., Beazer-Barclay, Y. D., Antonellis, K. J., Scherf, U., et al. (2003). Exploration, normalization, and summaries of high density oligonucleotide array probe level data. Biostatistics 4, 249–264. doi:10.1093/biostatistics/4.2.249
Kallio, M. A., Tuimala, J. T., Hupponen, T., Klemelä, P., Gentile, M., Scheinin, I., et al. (2011). Chipster: User-friendly analysis software for microarray and other high-throughput data. BMC Genomics 12, 507. doi:10.1186/1471-2164-12-507
Kats, D., Ricker, C. A., Berlow, N. E., Noblet, B., Nicolle, D., Mevel, K., et al. (2019). Volasertib preclinical activity in high-risk hepatoblastoma. Oncotarget 10, 6403–6417. doi:10.18632/oncotarget.27237
Kim, D., Langmead, B., and Salzberg, S. L. (2015). Hisat: A fast spliced aligner with low memory requirements. Nat. Methods 12, 357–360. doi:10.1038/nmeth.3317
Komander, D., and Rape, M. (2012). The ubiquitin code. Ubiquitin Code 81, 203–229. doi:10.1146/annurev-biochem-060310-170328
Kraft, S., Moore, J. B., Muzikansky, A., Scott, K. L., and Duncan, L. M. (2017). Differential UBE2C and HOXA1 expression in melanocytic nevi and melanoma. J. Cutan. Pathol. 44, 843–850. doi:10.1111/CUP.12997
Kuleshov, M. V., Jones, M. R., Rouillard, A. D., Fernandez, N. F., Duan, Q., Wang, Z., et al. (2016). Enrichr: A comprehensive gene set enrichment analysis web server 2016 update. Nucleic Acids Res. 44, W90–W97. doi:10.1093/nar/gkw377
Kullak-Ublick, G. A., Beuers, U., and Paumgartner, G. (1996). Molecular and functional characterization of bile acid transport in human hepatoblastoma HepG2 cells. Hepatology 23, 1053–1060. doi:10.1002/hep.510230518
Li, C., and Wong, W. H. (2001). Model-based analysis of oligonucleotide arrays: Expression index computation and outlier detection. Proc. Natl. Acad. Sci. 98, 31–36. doi:10.1073/pnas.011404098
Li, J., Zhi, X., Shen, X., Chen, C., Yuan, L., Dong, X., et al. (2020). Depletion of UBE2C reduces ovarian cancer malignancy and reverses cisplatin resistance via downregulating CDK1. Biochem. Biophys. Res. Commun. 523, 434–440. doi:10.1016/j.bbrc.2019.12.058
Linabery, A. M., and Ross, J. A. (2008). Trends in childhood cancer incidence in the U.S. Cancer 112, 416–432. doi:10.1002/cncr.23169
Liu, Y., Zhao, R., Chi, S., Zhang, W., Xiao, C., Zhou, X., et al. (2020). UBE2C is upregulated by estrogen and promotes epithelial–mesenchymal transition via p53 in endometrial cancer. Mol. Cancer Res. 18, 204–215. doi:10.1158/1541-7786.MCR-19-0561
Loussouarn, D., Campion, L., Leclair, F., Campone, M., Charbonnel, C., Ricolleau, G., et al. (2009). Validation of UBE2C protein as a prognostic marker in node-positive breast cancer. Br. J. Cancer 101, 166–173. doi:10.1038/sj.bjc.6605122
Ma, R., Kang, X., Zhang, G., Fang, F., Du, Y., and Lv, H. (2016). High expression of UBE2C is associated with the aggressive progression and poor outcome of malignant glioma. Oncol. Lett. 11, 2300–2304. doi:10.3892/OL.2016.4171
McKinney, W. (2010). “Data structures for statistical computing in Python,” in Proceedings of the 9th Python in Science Conference, Austin, TX, June 28- July 3, 2010, 56–61.
McQuin, C., Goodman, A., Chernyshev, V., Kamentsky, L., Cimini, B. A., Karhohs, K. W., et al. (2018). CellProfiler 3.0: Next-generation image processing for biology. PLoS Biol. 16, e2005970. doi:10.1371/JOURNAL.PBIO.2005970
Miranda, M., and Sorkin, A. (2007). Regulation of receptors and transporters by ubiquitination: New insights into surprisingly similar mechanisms. Mol. Interv. 7, 157–167. doi:10.1124/MI.7.3.7
Naso, F. D., Boi, D., Ascanelli, C., Pamfil, G., Lindon, C., Paiardini, A., et al. (2021). Nuclear localisation of aurora-A: Its regulation and significance for aurora-A functions in cancer. Oncogene 40, 3917–3928. doi:10.1038/s41388-021-01766-w
Nong, S., Han, X., Xiang, Y., Qian, Y., Wei, Y., Zhang, T., et al. (2023). Metabolic reprogramming in cancer: Mechanisms and therapeutics. MedComm 4, e218. doi:10.1002/MCO2.218
Noronha, A., Modamio, J., Jarosz, Y., Guerard, E., Sompairac, N., Preciat, G., et al. (2019). The Virtual Metabolic Human database: Integrating human and gut microbiome metabolism with nutrition and disease. Nucleic Acids Res. 47, D614–D624. doi:10.1093/nar/gky992
Palumbo, A., Costa, N. M. D., De Martino, M., Sepe, R., Pellecchia, S., de Sousa, V. P. L., et al. (2016). UBE2C is overexpressed in ESCC tissues and its abrogation attenuates the malignant phenotype of ESCC cell lines. Oncotarget 7, 65876–65887. doi:10.18632/ONCOTARGET.11674
Paquette, K., Coltin, H., Boivin, A., Amre, D., Nuyt, A. M., and Luu, T. M. (2019). Cancer risk in children and young adults born preterm: A systematic review and meta-analysis. PLoS One 14, e0210366. doi:10.1371/journal.pone.0210366
Raina, P., Guinea, R., Chatsirisupachai, K., Lopes, I., Farooq, Z., Guinea, C., et al. (2022). GeneFriends: Gene co-expression databases and tools for humans and model organisms. Nucleic Acids Res. 51, D145–D158. doi:10.1093/nar/gkac1031
Rawat, A., Gopal, G., Selvaluxmy, G., and Rajkumar, T. (2013). Inhibition of ubiquitin conjugating enzyme UBE2C reduces proliferation and sensitizes breast cancer cells to radiation, doxorubicin, tamoxifen and letrozole. Cell. Oncol. 36, 459–467. doi:10.1007/s13402-013-0150-8
Reddy, S. K., Rape, M., Margansky, W. A., and Kirschner, M. W. (2007). Ubiquitination by the anaphase-promoting complex drives spindle checkpoint inactivation. Nature 446, 921–925. doi:10.1038/nature05734
Rivas, M. P., Aguiar, T. F. M., Maschietto, M., Lemes, R. B., Caires-Júnior, L. C., Goulart, E., et al. (2020). Hepatoblastomas exhibit marked NNMT downregulation driven by promoter DNA hypermethylation. Tumor Biol. 42, 1010428320977124. doi:10.1177/1010428320977124
Robinson, M. D., McCarthy, D. J., and Smyth, G. K. (2009). edgeR: A Bioconductor package for differential expression analysis of digital gene expression data. Bioinformatics 26, 139–140. doi:10.1093/bioinformatics/btp616
Sekiguchi, M., Seki, M., Kawai, T., Yoshida, K., Yoshida, M., Isobe, T., et al. (2020). Integrated multiomics analysis of hepatoblastoma unravels its heterogeneity and provides novel druggable targets. npj Precis. Oncol. 4, 20–12. doi:10.1038/s41698-020-0125-y
Shannon, P., Markiel, A., Ozier, O., Baliga, N. S., Wang, J. T., Ramage, D., et al. (2003). Cytoscape: A software environment for integrated models of biomolecular interaction networks. Genome Res. 13, 2498–2504. doi:10.1101/gr.1239303
Shen, Z., Jiang, X., Zeng, C., Zheng, S., Luo, B., Zeng, Y., et al. (2013). High expression of ubiquitin-conjugating enzyme 2C (UBE2C) correlates with nasopharyngeal carcinoma progression. BMC Cancer 13, 192. doi:10.1186/1471-2407-13-192
Sivaprakasam, P., Gupta, A. A., Greenberg, M. L., Capra, M., and Nathan, P. C. (2011). Survival and long-term outcomes in children with hepatoblastoma treated with continuous infusion of cisplatin and doxorubicin. J. Pediatr. Hematol. Oncol. 33, e226–e230. doi:10.1097/MPH.0b013e31821f0eaf
Smyth, G. K. (2004). Linear models and empirical bayes methods for assessing differential expression in microarray experiments. Stat. Appl. Genet. Mol. Biol. 3, 1027. doi:10.2202/1544-6115.1027
Spector, L. G., and Birch, J. (2012). The epidemiology of hepatoblastoma. Pediatr. Blood Cancer 59, 776–779. doi:10.1002/pbc.24215
Sreedhar, A., and Zhao, Y. (2018). Dysregulated metabolic enzymes and metabolic reprogramming in cancer cells. Biomed. Rep. 8, 3–10. doi:10.3892/br.2017.1022
Sullivan, L. B., Gui, D. Y., and Heiden, M. G. Vander (2016). Altered metabolite levels in cancer: Implications for tumour biology and cancer therapy. Nat. Rev. Cancer 16 (11), 680–693. doi:10.1038/nrc.2016.85
Sun, R., Li, S., Zhao, K., Diao, M., and Li, L. (2021). Identification of ten core hub genes as potential biomarkers and treatment target for hepatoblastoma. Front. Oncol. 11, 591507. doi:10.3389/FONC.2021.591507
Sun, T., Liu, Z., and Yang, Q. (2020). The role of ubiquitination and deubiquitination in cancer metabolism. Mol. Cancer 19 (1), 146. doi:10.1186/s12943-020-01262-x
Szklarczyk, D., Gable, A. L., Lyon, D., Junge, A., Wyder, S., Huerta-Cepas, J., et al. (2019). STRING v11: Protein-protein association networks with increased coverage, supporting functional discovery in genome-wide experimental datasets. Nucleic Acids Res. 47, D607–D613. doi:10.1093/nar/gky1131
Tan, J., Xu, W., Lei, L., Liu, H., Wang, H., Cao, X., et al. (2020). Inhibition of aurora kinase a by alisertib reduces cell proliferation and induces apoptosis and autophagy in HuH-6 human hepatoblastoma cells. Onco Targets Ther. 13, 3953–3963. doi:10.2147/OTT.S228656
Tian, L., Chen, T., Lu, J., Yan, J., Zhang, Y., Qin, P., et al. (2021). Integrated protein-protein interaction and weighted gene Co-expression network analysis uncover three key genes in hepatoblastoma. Front. Cell. Dev. Biol. 9, 631982. doi:10.3389/fcell.2021.631982
Udatsu, Y., Kusafuka, T., Kuroda, S., Miao, J., and Okada, A. (2001). High frequency of β-catenin mutations in hepatoblastoma. Pediatr. Surg. Int. 17, 508–512. doi:10.1007/s003830000576
Váraljai, R., Islam, A. B. M. M. K., Beshiri, M. L., Rehman, J., Lopez-Bigas, N., and Benevolenskaya, E. V. (2015). Increased mitochondrial function downstream from KDM5A histone demethylase rescues differentiation in pRB-deficient cells. Genes. Dev. 29, 1817–1834. doi:10.1101/GAD.264036.115
Volkova, M., and Russell, R. (2012). Anthracycline cardiotoxicity: Prevalence, pathogenesis and treatment. Curr. Cardiol. Rev. 7, 214–220. doi:10.2174/157340311799960645
Wagner, A. E., Schwarzmayr, T., Häberle, B., Vokuhl, C., Schmid, I., von Schweinitz, D., et al. (2020). SP8 promotes an aggressive phenotype in hepatoblastoma via FGF8 activation. Cancers 12, 2294. doi:10.3390/CANCERS12082294
Wang, J., Tian, R., Shan, Y., Li, J., Gao, H., Xie, C., et al. (2020). Metabolomics study of the metabolic changes in hepatoblastoma cells in response to NTCP/SLC10A1 overexpression. Int. J. Biochem. Cell. Biol. 125, 105773. doi:10.1016/j.biocel.2020.105773
Wang, R., Song, Y., Liu, X., Wang, Q., Wang, Y., Li, L., et al. (2017). UBE2C induces EMT through Wnt/β-catenin and PI3K/Akt signaling pathways by regulating phosphorylation levels of Aurora-A. Int. J. Oncol. 50, 1116–1126. doi:10.3892/ijo.2017.3880
Woodfield, S. E., Shi, Y., Patel, R. H., Chen, Z., Shah, A. P., Srivastava, R. K., et al. (2021). MDM4 inhibition: A novel therapeutic strategy to reactivate p53 in hepatoblastoma. Sci. Rep. 11 (1), 2967. doi:10.1038/s41598-021-82542-4
Xie, Z., Bailey, A., Kuleshov, M. V., Clarke, D. J. B., Evangelista, J. E., Jenkins, S. L., et al. (2021). Gene set knowledge discovery with Enrichr. Curr. Protoc. 1, e90. doi:10.1002/cpz1.90
Xiong, Y., Lu, J., Fang, Q., Lu, Y., Xie, C., Wu, H., et al. (2019). UBE2C functions as a potential oncogene by enhancing cell proliferation, migration, invasion, and drug resistance in hepatocellular carcinoma cells. Biosci. Rep. 39. doi:10.1042/BSR20182384
Zhang, R-Y., Liu, Z-K., Wei, D., Yong, Y. L., Lin, P., Li, H., et al. (2021). UBE2S interacting with TRIM28 in the nucleus accelerates cell cycle by ubiquitination of p27 to promote hepatocellular carcinoma development. Signal Transduct. Target Ther. 61 (6), 64–12. doi:10.1038/s41392-020-00432-z
Zhou, Z., Li, Y., Hao, H., Wang, Y., Zhou, Z., Wang, Z., et al. (2019). Screening hub genes as prognostic biomarkers of hepatocellular carcinoma by bioinformatics analysis. Cell. Transpl. 28, 76S–86S. doi:10.1177/0963689719893950
Zsíros, J., Maibach, R., Shafford, E., Brugieres, L., Brock, P., Czauderna, P., et al. (2010). Successful treatment of childhood high-risk hepatoblastoma with dose-intensive multiagent chemotherapy and surgery: Final results of the SIOPEL-3HR study. J. Clin. Oncol. 28, 2584–2590. doi:10.1200/JCO.2009.22.4857
Zynger, D. L., Gupta, A., Luan, C., Chou, P. M., Yang, G. Y., and Yang, X. J. (2008). Expression of glypican 3 in hepatoblastoma: An immunohistochemical study of 65 cases. Hum. Pathol. 39, 224–230. doi:10.1016/j.humpath.2007.06.006
Glossary
Keywords: hepatoblastoma, liver tumor, pediatric cancer, ubiquitin, UBE2C
Citation: Nousiainen R, Eloranta K, Isoaho N, Cairo S, Wilson DB, Heikinheimo M and Pihlajoki M (2023) UBE2C expression is elevated in hepatoblastoma and correlates with inferior patient survival. Front. Genet. 14:1170940. doi: 10.3389/fgene.2023.1170940
Received: 21 February 2023; Accepted: 29 May 2023;
Published: 12 June 2023.
Edited by:
Junlin Xu, Hunan University, ChinaReviewed by:
Qiaoming Liu, Harbin Institute of Technology, ChinaSabine Colnot, Ligue Nationale Contre le Cancer, France
Copyright © 2023 Nousiainen, Eloranta, Isoaho, Cairo, Wilson, Heikinheimo and Pihlajoki. This is an open-access article distributed under the terms of the Creative Commons Attribution License (CC BY). The use, distribution or reproduction in other forums is permitted, provided the original author(s) and the copyright owner(s) are credited and that the original publication in this journal is cited, in accordance with accepted academic practice. No use, distribution or reproduction is permitted which does not comply with these terms.
*Correspondence: Katja Eloranta, a2F0amEuZWxvcmFudGFAaGVsc2lua2kuZmk=
†These authors have contributed equally to this work and share first authorship