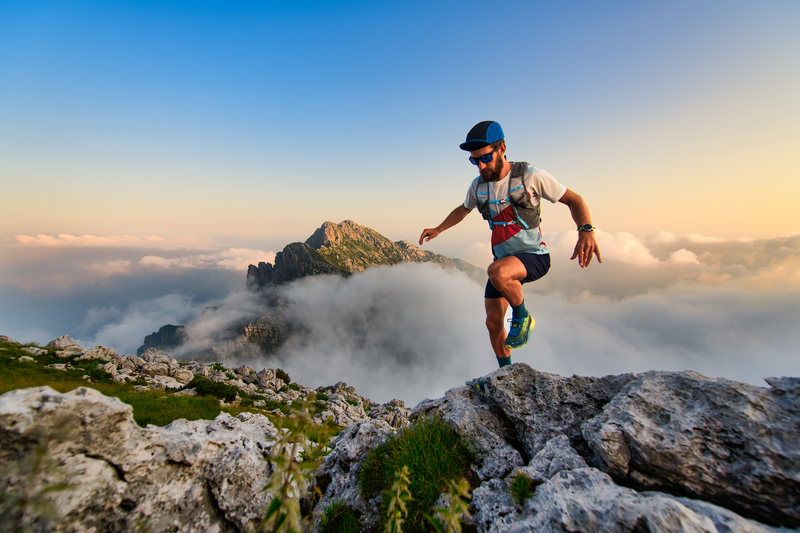
95% of researchers rate our articles as excellent or good
Learn more about the work of our research integrity team to safeguard the quality of each article we publish.
Find out more
ORIGINAL RESEARCH article
Front. Genet. , 16 May 2023
Sec. RNA
Volume 14 - 2023 | https://doi.org/10.3389/fgene.2023.1177259
Background: Long non-coding RNAs (lncRNAs), which are generally less functionally characterized or less annotated, evolve more rapidly than mRNAs and substantially possess fewer sequence conservation patterns than protein-coding genes across divergent species. People assume that the functional inference could be conducted on the evolutionarily conserved long non-coding RNAs as they are most likely to be functional. In the past decades, substantial progress has been made in discussions on the evolutionary conservation of non-coding genomic regions from multiple perspectives. However, understanding their conservation and the functions associated with sequence conservation in relation to further corresponding phenotypic variability or disorders still remains incomplete.
Results: Accordingly, we determined a highly conserved region (HCR) to verify the sequence conservation among long non-coding RNAs and systematically profiled homologous long non-coding RNA clusters in humans and mice based on the detection of highly conserved regions. Moreover, according to homolog clustering, we explored the potential function inference via highly conserved regions on representative long non-coding RNAs. On lncRNA XACT, we investigated the potential functional competence between XACT and lncRNA XIST by recruiting miRNA-29a, regulating the downstream target genes. In addition, on lncRNA LINC00461, we examined the interaction relationship between LINC00461 and SND1. This interaction or association may be perturbed during the progression of glioma. In addition, we have constructed a website with user-friendly web interfaces for searching, analyzing, and downloading to present the homologous clusters of humans and mice.
Conclusion: Collectively, homolog clustering via the highly conserved region definition and detection on long non-coding RNAs, as well as the functional explorations on representative sequences in our research, would provide new evidence for the potential function of long non-coding RNAs. Our results on the remarkable roles of long non-coding RNAs would presumably provide a new theoretical basis and candidate diagnostic indicators for tumors.
Comparative genomic analyses have identified that at least 5% of the human genome is under purifying selection or evolutionary constraint, with ∼1.5% of the constrained sequences corresponding to orthologous coding genes. In contrast, the remaining approximately 3.5% are conserved elements in non-coding regions (Leypold and Speicher, 2021). In recent years, the evolutionary conservation of non-coding genomic regions has been discussed with multiple dimensions, such as spatiotemporal expressions and chromatin structure (Diehl et al., 2020; Gorkin et al., 2020). Nowadays, accumulated evidence has proven that long non-coding RNAs (lncRNAs) are involved in increasing numbers of biological processes (Yao et al., 2019). However, concerns remain regarding their conservation and their impact on corresponding functions, as well as on further phenotypic variability or disorders.
It is known that lncRNAs evolve more rapidly than mRNAs and that most lncRNAs currently need to be functionally uncharacterized or less annotated (Yao et al., 2019). Although lncRNAs have substantially fewer sequence conservation patterns than protein-coding genes across species, it is believed that evolutionarily conserved lncRNAs are likely to be functional and emerge as important targets to investigate conserved lncRNAs undergoing conserved processing, localization, and functions. Studies have revealed that some lncRNA orthologs exhibit different subcellular localizations in human and mouse embryonic stem cells due to differential RNA processing, which leads to their functional divergence in pluripotency regulation (Guo et al., 2020). Meanwhile, some extremely conserved non-coding regions are non-randomly distributed across chromosomes and tend to cluster in the vicinity of genes with regulatory roles in multicellular development and differentiation (Katzman et al., 2007; Polychronopoulos et al., 2017). In addition, many regulatory roles could be inferred by sequence conservations, such as the transcription factor (TF) recognition sites and the DNase I hypersensitive sites (DHSs) (Stergachis et al., 2014; Vierstra et al., 2014). Moreover, there are emerging diagnostic approaches working on transcription start sites, TF-binding sites, and other non-coding regions (Ulz et al., 2019). Ribo-seq studies have documented that there are more RNA–protein interaction signals among human-conserved and mouse-conserved lncRNAs than non-conserved lncRNAs, suggesting the significant roles for these conserved non-coding sequences (Ruiz-Orera and Alba, 2019). Moreover, a series of neuronal tissue-specific lncRNAs between the human and Rhesus macaque was found to possess conserved expression patterns during prefrontal cortex development and maturation (He et al., 2014). Definitely, studies argue that conserved non-coding molecular structures or functions are not necessarily dependent on sequence constraint to some extent (Diederichs, 2014; Leypold and Speicher, 2021). However, most of the comparative analyses based on sequence conservation still provided direct explanations for the alterations which are associated with phenotypes such as cancer (Rheinbay et al., 2020), malformations, behavioral and neurological disorders, and autism (Turner et al., 2017; Dickel et al., 2018). The understanding of the conservation of lncRNAs and their impacts on the underlying mechanisms, associated phenotypes, and diseases remains incomplete.
Here, on lncRNA sequences, we determined a highly conserved region (HCR) to verify their conservations and conducted homologous lncRNA clustering in humans and mice based on the detection of HCRs. Additionally, on the corresponding homolog clustering, we explored the potential function inference via HCRs on representative lncRNAs. For lncRNA XACT, we investigated the potential functional competence between XACT and lncRNA XIST by recruiting miRNA-29a, regulating the downstream target genes. Furthermore, for lncRNA LINC00461, we examined the interaction relationship between LINC00461 and SND1, and the association may be perturbed during the progression of glioma. In addition, we constructed a website with user-friendly web interfaces for searching, analyzing, and downloading to present the homologous clusters of humans and mice.
In our study, we evaluated the conservation score of sequences using phastCons. Since the phyHMM algorithm built into phastCons relies on multiple sequence alignments and phylogenetic relationships among multiple sequences, our homologous sequence clustering was implemented through the following steps: 1) sequence search based on the Basic Local Alignment Search Tool (BLAST) (parameter: e-value < 1e-1) (Altschul et al., 1990); 2) multiple sequence alignment using MUSCLE (Edgar, 2004) and optimization of the alignment results based on trimAl (Capella-Gutiérrez et al., 2009) (parameter: -automated1); 3) building a phylogenetic tree based on the multiple sequence alignment results using FastTree (Price et al., 2010); 4) selecting a computational model using phyloFit based on the multiple sequence alignment results and phylogenetic analysis results (parameter: subst-mod HKY85); 5) obtaining the conservation score of the corresponding bases in the sequence using phastCons based on the multiple sequence alignment results and phylogenetic analysis results, as well as the model file generated by phyloFit (Siepel and Haussler, 2004); 6) identifying the HCRs based on the conservation scores along the sequence of each base. In our analysis, two criteria were set for identifying HCRs: (a) using a sliding window of 200 bp with a shift unit of 1 bp, we defined HCRs as contiguous segments with conservation scores of more than 60% sites> average conservation score of the whole sequence; (b) randomly selecting equal numbers of sequences from the HCR and non-HCR parts with a random length and satisfying the significance test of inter-group differences in conservation scores (p < 0.05). Furthermore, successful clustering of homologous lncRNAs in our study requires the capture of at least one HCR.
A total of 54,291 transcripts of human lncRNAs were obtained from the GENCODE version41 (http://www.gencodegenes.org/), and 25,419 transcripts of mouse lncRNAs were from GENCODE - Mouse Release M30 (gencodegenes.org), with the longest transcript of individual gene locus being collected. Homologous clustering was conducted individually in human lncRNAs, mouse lncRNAs, and their collection dataset.
In our study, a region including a lncRNA and its nearest upstream two gene loci and downstream two gene loci were used to assess the collinearity between two positions. If the total of the upstream and the downstream four genes exhibited to be orthologs (with reciprocal best BLAST hits; RBH) and the lncRNA pair exhibited to be homologs by the BLAST, these lncRNAs were defined as positionally conserved lncRNA pairs.
Repeat detection on the sequences was carried out by RepeatMasker (Chen, 2004). The subcellular localization predictions were conducted by iLoc-LncRNA (lin-group.cn) (Su et al., 2018). RNA–protein interaction pairs were predicted by catRAPID (Bellucci et al., 2011). RNAfold was utilized to predict the secondary structure (http://rna.tbi.univie.ac.at/cgi-bin/RNAWebSuite/RNAfold.cgi) using the complete sequences, with the minimum free energy structure, the thermodynamic ensemble of the RNA structure, and the centroid structure, together with the positional entropy for each position. Among them, the centroid is the structure in the entire ensemble that has the minimum total base-pair distance to the structures in a given set of structures, which acts as an efficient method for predicting the RNA secondary structure. The high peaks in the plot indicate the more stable RNA structures.
Potential binding sites were conducted by an online toolkit TargetScan (Agarwal et al., 2015) and miRanda (Enright et al., 2003). In addition, the expression of genes and lncRNAs was obtained and calculated from The Cancer Genome Atlas (TCGA; https://portal.gdc.cancer.gov; including 529 LGG samples and 173 GBM samples) and 1,152 normal brain cortex from the Genotype-Tissue Expression (GTEX) dataset (Consortium, 2013). The details are summarized in Supplementary Table S1. The batch correction was performed using the normalizeBetweenArrays function from the limma package (R package) (Ritchie et al., 2015; Chazarra-Gil et al., 2021).
The statistical significance of differences between the two groups was analyzed by the paired Student’s t-test. All reported p-values were two-sided, and p < 0.05 was considered statistically significant.
Our website was implemented based on Django (http://www.djangoproject.com). The web interfaces were developed by HTML5, CSS3, AJAX (Asynchronous JavaScript and XML), and in-house Python scripts.
To investigate homologous relationships, we carried out homolog clustering based on sequence conservation. The conservation scores were calculated to identify homologous sequences. The clusters with successful detection of HCR were defined as lncRNA homologous clusters. We performed homolog clustering individually in human lncRNAs, mouse lncRNAs, and their collection dataset. The size distribution of all detected HCRs showed that the peak length of mouse HCRs was approximately 220 bp, whereas there were two peak lengths of human HCRs at approximately 210 and 410 bp, respectively (Supplementary Figure S1).
In our analysis, a total of 5,287 homologous clusters involving 6,166 lncRNAs were obtained in Homo sapiens, and 514 homologous clusters involving 1,610 lncRNAs were achieved in Mus musculus. Moreover, 21 clusters were detected containing both human and mouse lncRNAs. The limited numbers of lncRNAs across these two species consistently indicate that lncRNAs evolve fairly rapidly. The homologous lncRNAs of humans tend to possess longer sequences with an average length of 105 bp (peak of ∼53,619 bp), with 104 bp in the mouse (peak of ∼8,279 bp) (Figure 1A). Meanwhile, on average, there are more sequences being clustered into a homologous cluster in H. sapiens than in M. musculus (Figure 1B). Furthermore, we examined the distribution of homologous lncRNAs on chromosomes and found that the homologs were scattered and distributed throughout the chromosomes (Figures 1C, D). In order to investigate the potential impact of highly repeated regions on the identification of HCR on lncRNAs, we examined the distribution of mobile elements on lncRNAs and found that 18.31% of human HCRs (25.13% of mouse HCRs) possess the repeat elements. After the removal of labeled repeat sequences, the length distribution of human and mouse sequences, and that of HCRs was examined (Supplementary Figure S2). Accordingly, we choose to add a warning label to such HCR regions, informing us of the presence of repetitive sequence feature elements in that region. Additionally, the vast majority of the sequences in homologous clusters come from different chromosomes predictably, and there are 943 human homologous clusters (17.8%) that possess neighbor lncRNAs (with an adjacent locus on the same strand) on the chromosome, and 38 mouse clusters (7.4%) possess neighbor lncRNAs (Figure 1E). Based on this result, we further detected the genome position conservations of lncRNAs between the human and mouse (Method) and distributed the percentage of sequence conservation clusters involving position conservation (Figure 1F). Although lncRNAs are less conserved than coding genes, we assumed that homologous lncRNAs possibly possess functional conservation related to HCRs. Accordingly, based on our HCR detection method, we explored the homologous lncRNAs between the human and mouse and obtained 21 homologous clusters with each cluster including at least one mouse lncRNA and one human lncRNA. These clusters are summarized in Table 1. We could examine the specific functional roles that are similar or related since at least one HCR has been detected in each individual cluster. Meanwhile, among them, there are 11 homologous clusters containing only one lncRNA from the human and one from the mouse. Another 10 clusters contain more than two sequences which indicate the duplications in the corresponding species. Moreover, due to the remarkable sequence length, we could find that some clusters have overlapped lncRNA sequences. For instance, snhg14 (with a length of 24,124 bp) and MALAT (with a length of 8,762 bp) both belong to two clusters, and XACT (with a length of 347,561 bp) belongs to seven clusters. As different clusters possess different HCRs, we chose to present the original clustering instead of integrating the overlapping clusters into a super cluster. Furthermore, in order to explore the spatial function characteristic of sequence-conserved lncRNAs, we retrieved sequence-conserved lncRNAs and compared their subcellular localization patterns (Figure 1G). The results show that most of the sequence-conserved lncRNAs in individual clusters possess similar subcellular localization patterns, inferring their conserved functional roles.
FIGURE 1. Distribution of divergent characteristics of human and mouse lncRNA clusters. (A) The average sequence length of homologous lncRNA clusters. (B) The average sequence number of homologous lncRNA clusters. (C) Scattered distributions of lncRNAs being clustered on human chromosomes. (D) Scattered distributions of lncRNAs being clustered on mouse chromosomes. (E) Percentage of the sequences in homologous clusters from different/same chromosomes and neighbor homologous lncRNAs (with an adjacent locus on the same strand) on the chromosome in humans and mice. (F) Percentage of sequences conservation clusters involving position conservation. (G) Subcellular localization patterns of sequence-conserved lncRNAs in individual clusters.
Our study aims to investigate the potential functional conservation of homologous lncRNAs by detecting HCRs. As a case study, we focused on XACT, a known X-linked lncRNA that has been reported to coat active X chromosomes in early human embryonic stages (Vallot et al., 2013). Previous studies have shown that XACT is weakly conserved across mammals and absent in mice, suggesting that it may have evolved to fulfill a primate-specific function (Vallot et al., 2013). To explore the potential functional conservation of XACT, we examined its sequence conservation and found that it belongs to seven homologous clusters. This suggests that XACT may have conserved functional roles in both humans and mice despite its weak sequence conservation. Our findings suggest that homologous lncRNAs detected by HCR analysis may have functional conservation and could provide a basis for further investigation of lncRNA functions.
In our analysis, we found that XACT has diverse HCRs with different transcripts of lncRNAs, including GAS5 (ENST00000702964.1; HCR location: 361∼767 bp), PRKAR2A-AS1 (ENST00000655796.1; HCR location:146,869∼149,088 bp), SNHG14 (ENST00000549804.7; HCR location: 8,498∼14,987 bp), TALAM1 (ENST00000698129.1; HCR location: 136∼2,060 bp), MEG3 (ENST00000522771.9; HCR location: 1,493∼8,653 bp), PAUPAR (ENST00000630360.1; HCR location: 48∼1,234 bp), and Gm20745 (ENSMUST00000216827.2; HCR location: 8∼3,778 bp), inferring its complicated roles suggesting that XACT may have related or supplementary functions with sequences in the same homologous clusters. Our analysis revealed that XACT belongs to seven clusters, each having its own HCR information and seven different mouse lncRNAs (Figure 2). However, we did not find any mouse lncRNA that belonged to seven or fewer different clusters like XACT. As a result, we could not detect the ortholog of XACT in mice consistently. In other words, XACT appears to be a primate-specific lncRNA that has weak conservation across mammals, as suggested by previous studies. Recently, a study reported that XACT and lncRNA XIST compete in controlling X chromosome activity during early human development, but the underlying mechanism remains unclear (Vallot et al., 2017). Previous research has shown that XIST regulates gene expression by recruiting miRNAs. Thus, we hypothesized that the regulatory roles of XACT and XIST may involve miRNAs. To investigate this, we examined a common HCR and found that miRNA-29a can bind to XIST at 4,424∼4,247 bp (Figure 2B). Additionally, predictive analysis suggests that miRNA-29a can also bind to XACT at 16,999 to 17,205 bp, indicating that both XIST and XACT may recruit miRNA-29a to regulate gene expression. Furthermore, we found that the binding region locates within the HCR of XACT and XIST (Figure 2C). For these two sequences, we presented the mountain plots of the MFE (minimum free energy) structure, the thermodynamic ensemble of the RNA structure, and the centroid structure, together with the positional entropy for each position (Figures 2D, E), and the binding regions exhibit to possess the most stable RNA structure. In addition, we found that CDK6, a kind of cyclin-dependent kinase, is the potential target gene of miRNA-29a, and CDK6 is a dominant gene playing a significant role in cell proliferation (Goel et al., 2022). Evidence from the relative expression profiles of human early development shows that the major burst of zygotic XIST and XACT expression occurred at the four-cell and the eight-cell stages (Vallot et al., 2017), inferring the potential associations between XIST/XACT and CDK6, through recruiting miR-29a.
FIGURE 2. Sequence exploration on XACT. (A) Different HCRs on XACT, with the representative sequence IDs from each homologous cluster. (B) Binding sites of miR-29a with XIST and XACT. (C) Detection of HCRs (with a gray background) on the transcripts of XIST and XACT and the binding region of miRNA-29a (with a steel blue background). (D, E) Mountain plots for (D) XIST and (E) XACT of the MFE (minimum free energy) structure, the thermodynamic ensemble of the RNA structure, and the centroid structure, together with the positional entropy for each position.
Although the structure–function relationship is well established for many proteins, the same cannot be said for most lncRNAs as they are still largely uncharacterized. Moreover, due to the lack of understanding of their functions, it is challenging to identify lncRNA biomarkers that are involved in cancer development. Based on the detection of HCRs of the homologous clusters, ENSMUST00000242216.1 (from mouse; C130071C03Rik) and ENST00000658935.1 (from human; ECONEXIN; LINC00461) are found to be in a homologous cluster. By comparing their subcellular localization results, we discovered that these two homologous lncRNAs both perform their functions in the cytoplasm. Two HCRs were found along the LINC00461 sequence (917∼1,715 bp and 2,449∼2,867 bp on ENST00000658935.1; Figure 3A; the region with a gray background color). Moreover, based on the examination of the binding ability of the lncRNAs, we found that SND1 was able to bind to LINC00461 and C130071C03Rik on the HCR region (Figure 3A, the region with a steel blue background) with highly reliable values (interaction propensity:96.08; z-score:3.6; Figures 3D, E), indicating the significantly associated roles of SND1 and these two lncRNAs. Furthermore, we hypothesized that, given the possibility of interactions, the binding region on the HCR should possess a stable spatial structure. To emphasize the key findings, we presented three types of structures (the minimum free energy, the thermodynamic ensemble, and the centroid structure) for the RNA molecule, along with the positional entropy data for each position (Figures 3B, C). By analyzing the data, we observed that the binding region (∼1,500 bp) exhibited the most stable RNA structure, which aligns with its presumed functional significance. Therefore, our results suggest a correlation between the stability of the RNA structure and its potential biological function in this specific context.
FIGURE 3. Functional prediction on the lncRNA LINC00461. (A) Detection of HCRs (with a gray background) on the transcript of LINC00461 and the binding regions of SND1 (with a steel blue background). (B, C) Mountain plots of the MFE (minimum free energy) structure, the thermodynamic ensemble of the RNA structure, and the centroid structure, together with the positional entropy for each position. (D) Distribution of the binding ability of SND1 to LINC00461 and (E) C130071C03Rik on an individual HCR region. (F) Comparison of the expression level of LINC00461 in samples of normal and two different types of gliomas (LGG and GBM). (G) Comparison of the expression level of SND1 in samples of normal and two different types of gliomas. (H–J) Correlation between expressions of SND1 and LINC00461 in (H) normal samples, (I) LGG samples, and (J) GBM samples. *** indicates p < 10−4.
Due to this fact, glioma is divided into lower-grade glioma (LGG) and high-grade glioma (e.g., glioblastoma, GBM), and LINC00461 is previously reported to be a regulator in glioblastoma (GBM) (Deguchi et al., 2017). In our analysis, we further detected the expression patterns of LINC00461 and SND1 among different types of gliomas and the normal brain cortex using the TCGA and GTEX datasets. The expressions of LINC00461 in GBM and LGG are significantly higher than those in normal samples (Figure 3F); the expressions of SND1 in GBM and LGG are also significantly higher than those in normal samples (Figure 3G), suggesting our predicted potential associated regulator roles of LINC00461 and SND1. Furthermore, considering the interaction possibility between LINC00461 and SND1, we explored the Pearson correlation relationships between expressions of these two sequences among different sample types. Intriguingly, we found that in normal samples, the expressions of SND1 and LINC00461 are positively correlated (Figure 3H; R = 0.32, p < 2.2e-16), whereas the correlation relationship is negatively correlated but exhibited to be not significant in LGG samples (Figure 3I; R = −0.014, p = 0.76). Meanwhile, the negative correlation relationship is enhanced in GBM samples (Figure 3J; R = −0.25, p < 8e-4). Collectively, our results indicate the interaction relationship between LINC00461 and SND1, and their interaction or association may be perturbed during the progression of glioma, which provides a candidate potential marker for glioma.
In order to present the homologous clusters of the human and mouse, we constructed a website with user-friendly web interfaces for searching, analyzing, and downloading (Figure 4). The website was implemented based on Django (http://www.djangoproject.com). The web interfaces were developed by HTML5, CSS3, AJAX (Asynchronous JavaScript and XML), and in-house Python scripts. It is freely available online at http://homolog.cn/. A user could search the homologous clusters and the detected HCR result by a lncRNA ID or by lncRNA sequences directly, and the website would return the related homologous cluster results, including the BLAST results, the phylogenetic trees among the homologs, the conservation scores along the sequences, and the HCR detection file. We will also provide clear instructions with a README file in the output file folders on how to interpret the results. In addition, a warning label to HCRs, informing users of the presence of repetitive sequence feature elements will be returned and displayed in the HCR results during sequence search. However, our website nowadays can only identify the lncRNA IDs from Ensembl transcripts. Furthermore, we have added the example inputs and outputs to guide the user. It is recommended that a user could paste the lncRNA sequences in the textbox or upload the lncRNA sequences by the “upload” button. If our website receives the uploaded sequences, the BLAST will be invoked and the related homologous cluster results of its best-hit lncRNA will be returned. We think the website will provide direct access for a biologist who is interested in sequence conservation for their corresponding functional inference in humans and mice.
Most of the conservations are just based on lncRNA sequence alignments by BLAST. We believe that the detection of highly conserved segments, such as HCR in our analysis, acts as an improvement of the conservation annotation, and the functional conservation could be inferred more relevantly. Among the 21 homologous clusters we identified, some lncRNAs were detected to be highly conserved between humans and mice (Table 1), such as MALAT, snhg1, Paupar, and Zeb2. The successful detection of these extremely conserved regions in these clusters would provide functional references. In our study, we only focused on XACT, which acts as an overlapped lncRNA in different clusters, due to its remarkable length, and discussed its possible functional roles by recruiting miRNA-29a on the HCR. XIST, which shares a common HCR with XACT, is reported to possess the binding sites of miRNA-29a on the HCR region, and the regulation of XIST and miR-29a was reported in the denatured dermis and human skin fibroblasts (HSFs) after thermal injury (Guo et al., 2018) but with limited documents on XCI. Taken together, our study provides a potential explanation of the competence of XACT and XIST in XCI. In addition, it is widely believed that lncRNA is closely associated with various diseases (Wang and Chang, 2011; Batista and Chang, 2013). In recent years, lines of evidence have accumulated that lncRNAs are involved in tumorigenesis and tumor metastasis, especially in cancer development (Taniue and Akimitsu, 2021), cancer immunity, cancer metabolism, and cancer metastasis (Jiang et al., 2019). Recent studies have shown that lncRNAs may also engage in remodeling the tumor microenvironment (Sang et al., 2018; Botti et al., 2019). Given the fact that cancer is difficult to cure, developing effective therapeutic approaches or markers to treat cancer is still important. Among the different types of cancers, gliomas are primary brain tumors derived from neuroglial stem or progenitor cells. Our study based on the detection of HCR and the functional exploration of this region offers a possibility that LINC00461 and SND1 could act as candidate markers in different types of gliomas. The correlations are obviously changed in LGG and GBM. We hypothesized that this could be a result of the binding ability between LINC00461 and SND1 during the progression of glioma. This finding could, to some extent, improve the diagnostics and classification systems. Certainly, our future experiment verification would be conducted in this field, and some substantial progress would be made based on this analysis in understanding the molecular pathogenesis of gliomas.
The data on lncRNAs serve as an important resource for public databases, and the number of lncRNAs is increasing. Many important databases were constructed for providing the sequence information and related aspects of functional characteristics of lncRNAs using omics datasets, such that LNCipedia (Volders et al., 2015) includes human lncRNA transcript sequences and their structure information; lncRNADisease (Bao et al., 2019) distributes the associations between lncRNAs and diseases; LncRNA2Target (Cheng et al., 2019) collects and provides with RNA-seq datasets before and after knockdown or overexpression of some specific lncRNAs; LncRNA2Function (Jiang et al., 2015) correlates lncRNAs with Gene Ontology (GO) terms and biological process; and lncRNASNP2 (Miao et al., 2018) relates lncRNAs with SNPs and TF2LncRNA (Jiang et al., 2014) with transcription factors similarly. Our website here is not an integrated database, just providing the homologous cluster search results. Considering that limited databases focus on sequence conservations and that our detection of highly conserved segments could act as an improvement on the conservation annotation, the highly conserved information will enhance our understanding of functional lncRNAs. Meanwhile, we admit that nowadays, the species are finite in humans and mice, and the related result is limited. As more lncRNAs are identified in different species, we will continue to integrate more lncRNAs from other species, provide more characteristics of lncRNAs, such as lncRNA–DNA/RNA interactions, and modification regulations, editing site information in these specially conserved regions, and perform comparisons of lncRNAs in a relatively large evolutionary timescale.
Publicly available datasets were analyzed in this study. Information for existing publicly accessible datasets is contained within the article.
Ethical review and approval was not required for the study of human participants in accordance with the local legislation and institutional requirements. Written informed consent from the patients/ participants or patients/participants’ legal guardian/next of kin was not required to participate in this study in accordance with the national legislation and the institutional requirements. Ethical review and approval was also not required for the study of animals in accordance with the local legislation and institutional requirements.
HY conceived the project, designed, and supervised this study. ZL, TG, YZ, ZY, and HL performed the analyses. ZY and HY wrote the manuscript. All authors contributed to the manuscript and approved the submitted version.
This work was supported by the Hainan Provincial Natural Science Foundation of China (321RC456) and the National Natural Science Foundation of China (31960299) and the startup fund from Hainan University.
We thank the reviewers for their valuable suggestions and comments.
The authors declare that the research was conducted in the absence of any commercial or financial relationships that could be construed as a potential conflict of interest.
All claims expressed in this article are solely those of the authors and do not necessarily represent those of their affiliated organizations, or those of the publisher, the editors, and the reviewers. Any product that may be evaluated in this article, or claim that may be made by its manufacturer, is not guaranteed or endorsed by the publisher.
The Supplementary Material for this article can be found online at: https://www.frontiersin.org/articles/10.3389/fgene.2023.1177259/full#supplementary-material
SUPPLEMENTARY FIGURE S1 | The length distribution of detected HCRs in human lncRNAs, mouse lncRNAs, and the collected dataset.
SUPPLEMENTARY FIGURE S2 | The length distribution of (A) human and mouse sequences and (B) the HCRs after the flagged repeats are removed.
Agarwal, V., Bell, G. W., Nam, J. W., and Bartel, D. P. (2015). Predicting effective microRNA target sites in mammalian mRNAs. Elife 4, e05005. doi:10.7554/eLife.05005
Altschul, S. F., Gish, W., Miller, W., Myers, E. W., and Lipman, D. J. (1990). Basic local alignment search tool. J Mol Biol. 215, 403–410. doi:10.1016/S0022-2836(05)80360-2
Bao, Z., Yang, Z., Huang, Z., Zhou, Y., Cui, Q., and Dong, D. (2019). LncRNADisease 2.0: An updated database of long non-coding RNA-associated diseases. Nucleic Acids Res. 47, D1034–D1037. doi:10.1093/nar/gky905
Batista, P. J., and Chang, H. Y. (2013). Long noncoding RNAs: Cellular address codes in development and disease. Cell 152, 1298–1307. doi:10.1016/j.cell.2013.02.012
Bellucci, M., Agostini, F., Masin, M., and Tartaglia, G. G. (2011). Predicting protein associations with long noncoding RNAs. Nat. Methods 8, 444–445. doi:10.1038/nmeth.1611
Botti, G., Scognamiglio, G., Aquino, G., Liguori, G., and Cantile, M. (2019). LncRNA HOTAIR in tumor microenvironment: What role? Int. J. Mol. Sci. 20, 2279. doi:10.3390/ijms20092279
Capella-Gutiérrez, S., Silla-Martínez, J. M., and Gabaldón, T. (2009). trimAl: a tool for automated alignment trimming in large-scale phylogenetic analyses. Bioinformatics 25, 1972–1973. doi:10.1093/bioinformatics/btp348
Chazarra-Gil, R., Van Dongen, S., Kiselev, V. Y., and Hemberg, M. (2021). Flexible comparison of batch correction methods for single-cell RNA-seq using BatchBench. Nucleic acids Res. 49, e42. doi:10.1093/nar/gkab004
Chen, N. (2004). Using Repeat Masker to identify repetitive elements in genomic sequences. Curr. Protoc. Bioinforma. 5, Unit 4.10. doi:10.1002/0471250953.bi0410s05
Cheng, L., Wang, P., Tian, R., Wang, S., Guo, Q., Luo, M., et al. (2019). LncRNA2Target v2.0: A comprehensive database for target genes of lncRNAs in human and mouse. Nucleic Acids Res. 47, D140–D144. doi:10.1093/nar/gky1051
Consortium, G. T. (2013). The genotype-tissue expression (GTEx) project. Nat. Genet. 45, 580–585. doi:10.1038/ng.2653
Deguchi, S., Katsushima, K., Hatanaka, A., Shinjo, K., Ohka, F., Wakabayashi, T., et al. (2017). Oncogenic effects of evolutionarily conserved noncoding RNA ECONEXIN on gliomagenesis. Oncogene 36, 4629–4640. doi:10.1038/onc.2017.88
Dickel, D. E., Ypsilanti, A. R., Pla, R., Zhu, Y., Barozzi, I., Mannion, B. J., et al. (2018). Ultraconserved enhancers are required for normal development. Cell 172, 491–499. doi:10.1016/j.cell.2017.12.017
Diederichs, S. (2014). The four dimensions of noncoding RNA conservation. Trends Genet. 30, 121–123. doi:10.1016/j.tig.2014.01.004
Diehl, A. G., Ouyang, N., and Boyle, A. P. (2020). Transposable elements contribute to cell and species-specific chromatin looping and gene regulation in mammalian genomes. Nat. Commun. 11, 1796. doi:10.1038/s41467-020-15520-5
Edgar, R. C. (1990). MUSCLE: a multiple sequence alignment method with reduced time and space complexity. BMC Bioinformatics 5, 113. doi:10.1186/1471-2105-5-113
Enright, A. J., John, B., Gaul, U., Tuschl, T., Sander, C., and Marks, D. S. (2003). MicroRNA targets in Drosophila. Genome Biol. 5, R1. doi:10.1186/gb-2003-5-1-r1
Goel, S., Bergholz, J. S., and Zhao, J. J. (2022). Targeting CDK4 and CDK6 in cancer. Nat. Rev. Cancer 22, 356–372. doi:10.1038/s41568-022-00456-3
Gorkin, D. U., Barozzi, I., Zhao, Y., Zhang, Y., Huang, H., Lee, A. Y., et al. (2020). An atlas of dynamic chromatin landscapes in mouse fetal development. Nature 583, 744–751. doi:10.1038/s41586-020-2093-3
Guo, C. J., Ma, X. K., Xing, Y. H., Zheng, C. C., Xu, Y. F., Shan, L., et al. (2020). Distinct processing of lncRNAs contributes to non-conserved functions in stem cells. Cell 181, 621–636. doi:10.1016/j.cell.2020.03.006
Guo, L., Huang, X., Liang, P., Zhang, P., Zhang, M., Ren, L., et al. (2018). Role of XIST/miR-29a/LIN28A pathway in denatured dermis and human skin fibroblasts (HSFs) after thermal injury. J. Cell Biochem. 119, 1463–1474. doi:10.1002/jcb.26307
He, Z., Bammann, H., Han, D., Xie, G., and Khaitovich, P. (2014). Conserved expression of lincRNA during human and macaque prefrontal cortex development and maturation. RNA 20, 1103–1111. doi:10.1261/rna.043075.113
Jiang, M. C., Ni, J. J., Cui, W. Y., Wang, B. Y., and Zhuo, W. (2019). Emerging roles of lncRNA in cancer and therapeutic opportunities. Am. J. Cancer Res. 9, 1354–1366.
Jiang, Q., Ma, R., Wang, J., Wu, X., Jin, S., Peng, J., et al. (2015). LncRNA2Function: A comprehensive resource for functional investigation of human lncRNAs based on RNA-seq data. BMC Genomics 16, S2. doi:10.1186/1471-2164-16-S3-S2
Jiang, Q., Wang, J., Wang, Y., Ma, R., Wu, X., and Li, Y. (2014). TF2LncRNA: Identifying common transcription factors for a list of lncRNA genes from ChIP-seq data. Biomed. Res. Int. 2014, 317642. doi:10.1155/2014/317642
Katzman, S., Kern, A. D., Bejerano, G., Fewell, G., Fulton, L., Wilson, R. K., et al. (2007). Human genome ultraconserved elements are ultraselected. Science 317, 915. doi:10.1126/science.1142430
Leypold, N. A., and Speicher, M. R. (2021). Evolutionary conservation in noncoding genomic regions. Trends Genet. 37, 903–918. doi:10.1016/j.tig.2021.06.007
Miao, Y. R., Liu, W., Zhang, Q., and Guo, A. Y. (2018). lncRNASNP2: an updated database of functional SNPs and mutations in human and mouse lncRNAs. Nucleic Acids Res. 46, D276–D280. doi:10.1093/nar/gkx1004
Polychronopoulos, D., King, J. W. D., Nash, A. J., Tan, G., and Lenhard, B. (2017). Conserved non-coding elements: Developmental gene regulation meets genome organization. Nucleic Acids Res. 45, 12611–12624. doi:10.1093/nar/gkx1074
Price, M. N., Dehal, P. S., and Arkin, A. P. (2010). FastTree 2–approximately maximum-likelihood trees for large alignments. PloS one 5, e9490. doi:10.1371/journal.pone.0009490
Rheinbay, E., Nielsen, M. M., Abascal, F., Wala, J. A., Shapira, O., Tiao, G., et al. (2020). Analyses of non-coding somatic drivers in 2,658 cancer whole genomes. Nature 578, 102–111. doi:10.1038/s41586-020-1965-x
Ritchie, M. E., Phipson, B., Wu, D., Hu, Y., Law, C. W., Shi, W., et al. (2015). Limma powers differential expression analyses for RNA-sequencing and microarray studies. Nucleic acids Res. 43, e47. doi:10.1093/nar/gkv007
Ruiz-Orera, J., and Alba, M. M. (2019). Conserved regions in long non-coding RNAs contain abundant translation and protein-RNA interaction signatures. Nar. Genom Bioinform 1, e2. doi:10.1093/nargab/lqz002
Sang, L. J., Ju, H. Q., Liu, G. P., Tian, T., Ma, G. L., Lu, Y. X., et al. (2018). LncRNA CamK-A regulates Ca(2+)-signaling-mediated tumor microenvironment remodeling. Mol. Cell 72, 601. doi:10.1016/j.molcel.2018.10.024
Siepel, A., and Haussler, D. (2004). Phylogenetic estimation of context-dependent substitution rates by maximum likelihood. Mol. Biol. Evol. 21, 468–488. doi:10.1093/molbev/msh039
Stergachis, A. B., Neph, S., Sandstrom, R., Haugen, E., Reynolds, A. P., Zhang, M., et al. (2014). Conservation of trans-acting circuitry during mammalian regulatory evolution. Nature 515, 365–370. doi:10.1038/nature13972
Su, Z. D., Huang, Y., Zhang, Z. Y., Zhao, Y. W., Wang, D., Chen, W., et al. (2018). iLoc-lncRNA: predict the subcellular location of lncRNAs by incorporating octamer composition into general PseKNC. Bioinformatics 34, 4196–4204. doi:10.1093/bioinformatics/bty508
Taniue, K., and Akimitsu, N. (2021). The functions and unique features of LncRNAs in cancer development and tumorigenesis. Int. J. Mol. Sci. 22, 632. doi:10.3390/ijms22020632
Turner, T. N., Coe, B. P., Dickel, D. E., Hoekzema, K., Nelson, B. J., Zody, M. C., et al. (2017). Genomic patterns of de novo mutation in simplex autism. Cell 171, 710–722. doi:10.1016/j.cell.2017.08.047
Ulz, P., Perakis, S., Zhou, Q., Moser, T., Belic, J., Lazzeri, I., et al. (2019). Inference of transcription factor binding from cell-free DNA enables tumor subtype prediction and early detection. Nat. Commun. 10, 4666. doi:10.1038/s41467-019-12714-4
Vallot, C., Huret, C., Lesecque, Y., Resch, A., Oudrhiri, N., Bennaceur-Griscelli, A., et al. (2013). XACT, a long noncoding transcript coating the active X chromosome in human pluripotent cells. Nat. Genet. 45, 239–241. doi:10.1038/ng.2530
Vallot, C., Patrat, C., Collier, A. J., Huret, C., Casanova, M., Liyakat Ali, T. M., et al. (2017). XACT noncoding RNA competes with XIST in the control of X chromosome activity during human early development. Cell Stem Cell 20, 102–111. doi:10.1016/j.stem.2016.10.014
Vierstra, J., Rynes, E., Sandstrom, R., Zhang, M., Canfield, T., Hansen, R. S., et al. (2014). Mouse regulatory DNA landscapes reveal global principles of cis-regulatory evolution. Science 346, 1007–1012. doi:10.1126/science.1246426
Volders, P. J., Verheggen, K., Menschaert, G., Vandepoele, K., Martens, L., Vandesompele, J., et al. (2015). An update on LNCipedia: A database for annotated human lncRNA sequences. Nucleic Acids Res. 43, D174–D180. doi:10.1093/nar/gku1060
Wang, K. C., and Chang, H. Y. (2011). Molecular mechanisms of long noncoding RNAs. Mol. Cell 43, 904–914. doi:10.1016/j.molcel.2011.08.018
Keywords: homolog clustering, HCR, XACT, LINC00461, functional inference
Citation: Liu Z, Guo T, Yin Z, Zeng Y, Liu H and Yin H (2023) Functional inference of long non-coding RNAs through exploration of highly conserved regions. Front. Genet. 14:1177259. doi: 10.3389/fgene.2023.1177259
Received: 01 March 2023; Accepted: 28 April 2023;
Published: 16 May 2023.
Edited by:
Nicholas Delihas, Stony Brook Medicine, United StatesReviewed by:
Ricardo Vialle, Rush University, United StatesCopyright © 2023 Liu, Guo, Yin, Zeng, Liu and Yin. This is an open-access article distributed under the terms of the Creative Commons Attribution License (CC BY). The use, distribution or reproduction in other forums is permitted, provided the original author(s) and the copyright owner(s) are credited and that the original publication in this journal is cited, in accordance with accepted academic practice. No use, distribution or reproduction is permitted which does not comply with these terms.
*Correspondence: Hongyan Yin, eWluaHlAaGFpbmFudS5lZHUuY24=
Disclaimer: All claims expressed in this article are solely those of the authors and do not necessarily represent those of their affiliated organizations, or those of the publisher, the editors and the reviewers. Any product that may be evaluated in this article or claim that may be made by its manufacturer is not guaranteed or endorsed by the publisher.
Research integrity at Frontiers
Learn more about the work of our research integrity team to safeguard the quality of each article we publish.