- 1Center for Precision Health, McWilliams School of Biomedical Informatics, The University of Texas Health Science Center at Houston, Houston, TX, United States
- 2Department of Epidemiology, Human Genetics and Environmental Sciences, School of Public Health, The University of Texas Health Science Center at Houston, Houston, TX, United States
- 3Faillace Department of Psychiatry and Behavioral Sciences, McGovern Medical School, The University of Texas Health Science Center at Houston, Houston, TX, United States
Introduction: Frailty is the most common medical condition affecting the aging population, and its prevalence increases in the population aged 65 or more. Frailty is commonly diagnosed using the frailty index (FI) or frailty phenotype (FP) assessments. Observational studies have indicated the association of frailty with Alzheimer’s disease (AD). However, the shared genetic and biological mechanism of these comorbidity has not been studied.
Methods: To assess the genetic relationship between AD and frailty, we examined it at single nucleotide polymorphism (SNP), gene, and pathway levels.
Results: Overall, 16 genome-wide significant loci (15 unique loci) (pmeta-analysis < 5 × 10−8) and 22 genes (21 unique genes) were identified between AD and frailty using cross-trait meta-analysis. The 8 shared loci implicated 11 genes: CLRN1-AS1, CRHR1, FERMT2, GRK4, LINC01929, LRFN2, MADD, RP11-368P15.1, RP11-166N6.2, RNA5SP459, and ZNF652 between AD and FI, and 8 shared loci between AD and FFS implicated 11 genes: AFF3, C1QTNF4, CLEC16A, FAM180B, FBXL19, GRK4, LINC01104, MAD1L1, RGS12, ZDHHC5, and ZNF521. The loci 4p16.3 (GRK4) was identified in both meta-analyses. The colocalization analysis supported the results of our meta-analysis in these loci. The gene-based analysis revealed 80 genes between AD and frailty, and 4 genes were initially identified in our meta-analyses: C1QTNF4, CRHR1, MAD1L1, and RGS12. The pathway analysis showed enrichment for lipoprotein particle plasma, amyloid fibril formation, protein kinase regulator, and tau protein binding.
Conclusion: Overall, our results provide new insights into the genetics of AD and frailty, suggesting the existence of non-causal shared genetic mechanisms between these conditions.
Introduction
Alzheimer’s disease (AD) and frailty are two profound health concerns that exert a substantial impact on the aging population. AD is characterized by a relentless neurodegenerative process resulting in cognitive deterioration, memory loss, and alterations in behavior and physical capabilities. It affects approximately 6.7 million Americans aged 65 and above (Alzheimers Dement, 2023). Concurrently, frailty represents a clinically identifiable state of increased vulnerability and decreased physiological reserves in older adults in everyday life (Chen et al., 2014). There are several frailty definitions; the most common ones are the frailty phenotype (FP) (Fried et al., 2001) and the frailty index (FI) (Mitnitski et al., 2001). FP is diagnosed as a clinical syndrome predicated upon the presence of three of five physical components, namely, weakness, slow walking speed, inadequate physical activity, exhaustion, and unexpected weight loss. On the contrary, FI is rooted in accumulating various health deficits throughout an individual’s lifetime.
The pathophysiological underpinnings of AD are characterized by intricate and multifactorial processes involving beta-amyloid plaques and neurofibrillary tangles. Studies have illuminated the pivotal roles of inflammation and oxidative stress in the initiation and progression of AD and frailty (Di Bona et al., 2010; Chen et al., 2014; Sargent et al., 2018). Furthermore, these conditions share common risk factors such as age, cognitive decline, slow walking, depression, and diabetes. Notably, frailty is a recognized risk factor for AD (Buchman et al., 2007; Ward et al., 2022). With improvements in lifestyle and advanced therapeutics, the extended life span of the population has amplified the prevalence of both AD and frailty. AD prevalence increases with age, being 5.0% from age 65 to 74, 13.1% from age 75 to 84, and 33.3% from age 85 and older, respectively (Rajan et al., 2021). Estimating the prevalence of FI is challenging, but it is estimated to be 18% worldwide in the population aged 60 or above (Siriwardhana et al., 2018). Consequently, many studies used the FP, which is easier to ascertain in large population studies, to assess the prevalence of frailty. In the United States, for the population older than 65 years, the frailty prevalence ranges from 6% to 12% and increases from 3.9% in the 65–74 years age group to 25% in those older than 85 years (Fried et al., 2004). The comorbidity of AD and frailty can further impair quality of life (Mhaolain et al., 2012) and amplify healthcare costs (Butler et al., 2016).
A systematic review and meta-analysis of frailty in mild to moderate AD suggested an increased prevalence of frailty in AD (Kojima et al., 2017), and a randomized clinical trial showed a greater prevalence of frailty in AD compared to mild cognitive impairment (MCI) (Wightman et al., 2023). Although there are no genetic studies directly related to AD and frailty, some epidemiological studies have found an association between AD and frailty (Gomez-Gomez and Zapico, 2019; Alvarado et al., 2021; Sabbatinelli et al., 2021), and AD biomarkers and frailty (Koch et al., 2013; Wallace et al., 2018; Canevelli et al., 2021). Genome-wide association studies (GWAS) for AD and frailty have identified single nucleotide polymorphism (SNPs), genes, and susceptibility loci. Findings from these GWAS have suggested shared genetic underpinnings between the risk factors common to both AD and frailty, such as body mass index (BMI), cardiovascular diseases, depression, and smoking. To the best of our knowledge, no genetic studies have investigated potential pleiotropy or shared underlying mechanisms between AD and frailty using either SNP or gene-level information from GWAS of these two traits.
In the current study, we carried out comprehensive analyses with the goal of exploring the genetic and potential causal relationship between AD and frailty. Our investigation revealed a nuanced genetic overlap with minimal genetic correlation between AD and frailty. Furthermore, we employed cross-trait meta-analyses to identify shared loci between AD and frailty. Our causality analysis provides no substantial evidence for a causal relationship between these two conditions. However, in gene-based association analysis, we found common genes attaining genome-wide cutoff for AD and frailty. Lastly, pathway analyses revealed a notable enrichment of lipoprotein particle plasma, amyloid fibril formation, protein kinase regulator, and tau protein binding for those genes common to AD and frailty.
Materials and methods
The general workflow of the study is summarized in Figure 1.
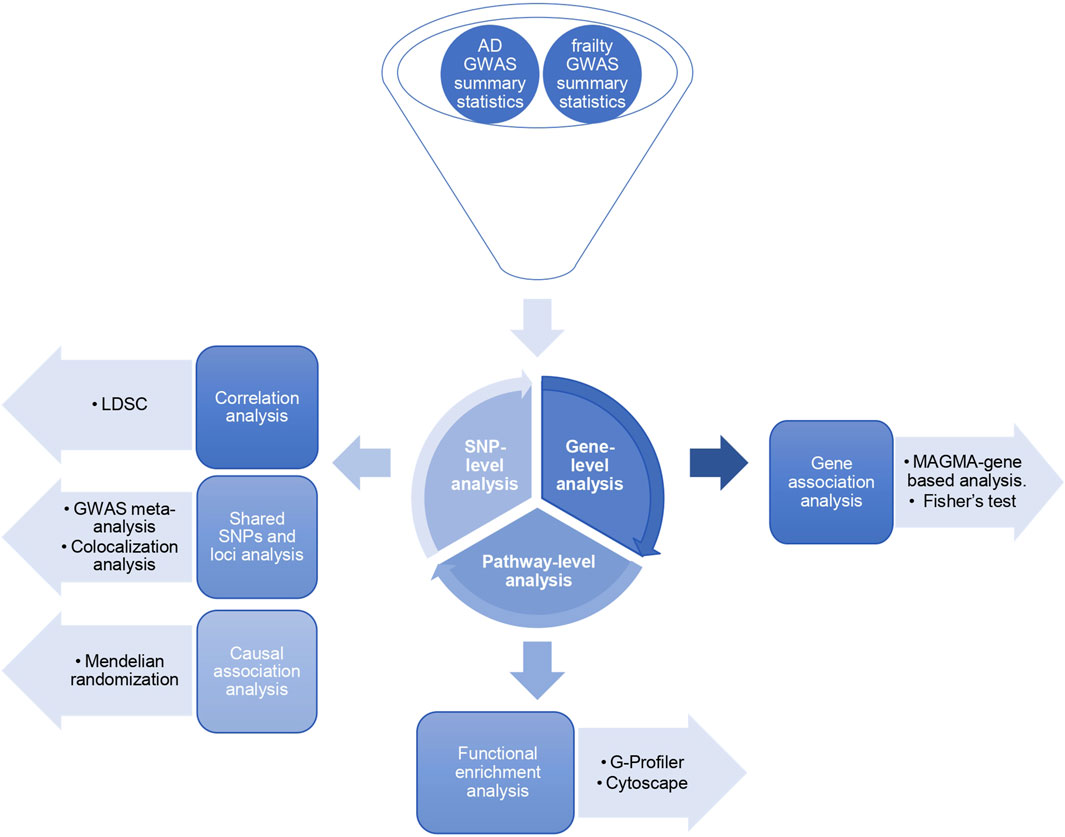
Figure 1. Study design. Three levels of SNP-based, gene-based, and pathway-based analyses exploring the shared genetic architecture between Alzheimer’s disease and frailty.
GWAS summary statistics
We obtained GWAS summary statistics data for AD, which included only diagnosed AD individuals (n = 398,058) (Wightman et al., 2021) (GWAS data with AD cases only, excluding AD proxies from UK Biobank, was made available by the authors upon request). Separately, GWAS summary statistics on FI (n = 164,610) (Atkins et al., 2021) and FP (n = 386,565) (Ye et al., 2023) analyzing participants from the UK Biobank were obtained. All individuals were of European ancestry, and the summary statistics were built on the genome reference GRCh37. There was no sample overlap between AD and each of the frailty assessments (FI and FP) GWAS datasets (Supplementary Table S1).
Linkage disequilibrium score regression (LDSC) between AD and frailty
To estimate the genetic correlation between AD and each of the frailty assessments, we employed the cross-trait linkage disequilibrium score regression (LDSC) method (Bulik-Sullivan et al., 2015). To ensure the robustness of our analysis, we utilized pre-estimated linkage disequilibrium (LD) scores provided by the developers of LDSC. These LD scores were derived from the 1,000 Genomes European reference population. We preprocessed the summary statistics using LDSC munge_sumstats.py and used the z-score as the signed summary statistic. To minimize the potential for bias arising from variations in LD structure, we conducted the genetic correlation calculations by incorporating HapMap3 SNPs in conjunction with LD reference panel SNPs. We excluded genomic regions covering the major histocompatibility complex (MHC, chr6: 25,119,106–33,854,733) and apolipoprotein E (APOE, chr19:44,000,000-47,000,000) from our analysis, considering their complex LD structure and known large genetic effect in AD, respectively.
GWAS cross-trait meta-analysis
We conducted cross-trait meta-analyses of AD and each of the frailty assessments GWAS data to identify shared pleiotropic SNPs and loci between both traits. The fixed effect (FE) and the modified random effect (RE2) (Han and Eskin, 2011) models were implemented in our meta-analyses using the METASOFT program (http://genetics.cs.ucla.edu/meta/). The RE2 model operates under the assumption that SNP effects vary and that it computes p values via a likelihood ratio test, in contrast to the FE model, which assumes uniform effect sizes across studies. The FE derives the p values using the inverse variance weighted (IVW) effect size methodology, which may be inadequate in the presence of heterogeneity. Our primary objective with this approach was to unveil the SNPs that, while not initially meeting the genome-wide significance threshold (5 × 10−8 < pGWAS-SNP < 0.05), attained this level of significance following each meta-analysis (pmeta-analysis < 5 × 10−8).
To further evaluate the shared SNPs between AD and each of the frailty assessments, we used the posterior probability (m-value) scores (Han and Eskin, 2011) from the meta-analysis results, which predict the effect size estimate in each of the studies under heterogeneity. An effect was predicted to exist if the posterior probability was greater than 0.9, no effect when the posterior probability was below 0.1, and an ambiguous effect when it was between 0.1 and 0.9 (Han and Eskin, 2011).
Genomic loci definition and functional annotation
For AD and each of the frailty meta-analyses, independent SNPs and loci were identified based on the FUMA protocol, an online tool for functional mapping of genetic variants (http://fuma.ctglab.nl/) (Watanabe et al., 2017). As a result of our meta-analysis, SNPs that reached genome-wide significance (pmeta-analysis < 5 × 10−8) with linkage disequilibrium (LD) r2 < 0.6 with each other were recognized as independently significant SNPs. A subgroup of these independent SNPs with LD r2 < 0.1 was then considered as the lead SNPs. The genomic locus boundaries were identified by detecting all the candidate SNPs that were in LD (r2 ≥ 0.6) with the lead SNP. If the distance between two loci was smaller than 250 kb, they were merged. Some loci may have multiple LD-independent (r2 < 0.1) lead SNPs within the locus. All candidate SNPs located in these distinct locations were regarded as one independent genomic locus. The LD calculations were based on the 1000 Genomes Project (Clarke et al., 2017) reference panel for European ancestry.
We annotated the genes to the lead SNPs based on positional mapping using ANNOtate VARiation (ANNOVAR) (Wang et al., 2010) in FUMA. Additionally, these SNPs were annotated with Combined Annotation Dependent Depletion (CADD) scores (Rentzsch et al., 2019) to predict how certain the SNP effect is on protein structure or function and possible contribution to genetic disease. Similarly, we used RegulomeDB (RDB version 1.1) scores (Boyle et al., 2012) to predict the likelihood of regulatory functionality and chromatin states to predict transcription and regulatory effects from chromatin states at the SNP locus. RDB ranks the SNPs with a score from 1 (1a to 1f) to 6. The SNPs with strong evidence of being a regulatory variant are given a score of 1, and the ones with the least evidence are scored 6 (Boyle et al., 2012).
Enrichment control and assessment of SNP novelty
SNPs within the MHC region (defined as chr6: 25,119,106 - 33,854,733) and APOE gene (chr19:44,000,000-47,000,000) were excluded from the analyses due to known association to AD (Lambert et al., 2010; Scheltens et al., 2016) or a very complex LD structure (de Bakker and Raychaudhuri, 2012). For the meta-analysis, we used a modified random effect (RE2) p-value and the GWAS p-value, i.e., pmeta-analysis < 5 × 10−8 and 5 × 10−8 < pGWAS-SNP < 0.05 cutoff to identify the novel, previously unidentified shared SNPs between two traits in their original GWAS.
To assess their novelty, we examined our identified loci in previously reported GWAS associations in the National Human Genome Research Institute (NHGRI-EBI) GWAS Catalog (Sollis et al., 2023). We further identified if the gene was novel to any of our traits based on the traits reported in the GWAS Catalog.
Colocalization analysis
To further investigate and discover genomic regions shared by each of the frailty assessment and AD, we conducted a colocalization study using the Pairwise GWAS approach (Pickrell et al., 2016) (GWAS-PW) (https://github.com/joepickrell/gwas-pw). The Bayesian pleiotropy association test, which reveals genomic regions that affect both traits, is the foundation of GWAS-PW. Additionally, we employed this technique to determine whether AD and frailty confidently share the same loci attaining genome-wide cutoff in our GWAS meta-analyses. The summary statistics of AD and each of the frailty assessments were combined using the GWAS-PW, and the posterior probability of association (PPA) of a pre-specified genomic region was calculated. GWAS-PW estimates four PPAs: i) the probability that the locus is associated with AD only (PPA1), ii) the probability that the locus is associated with frailty only (PPA2), iii) the probability that the locus is associated with both AD and frailty (PPA3) and iv) the probability that the locus is associated with both AD and frailty but through different causal variants (PPA4). The shared SNPs and regions (PPA3 and PPA4) were selected if their PPA >0.5 in the models.
Causal relationship
We evaluated the potential causal relationship between AD and each of the frailty assessments using Mendelian randomization (MR) analyses. MR uses SNPs as instrument variables (IV) to assess the causality, and these IVs are defined by three assumptions (Davies et al., 2018). First, the selected IVs are significantly associated (pGWAS < 5 × 10−8) with the exposure variable. Second, the IVs are independent between the exposure and the outcome. Third, the effect of IVs on the outcome must precede the exposure. We evaluated the bidirectional association between AD and each of the frailty assessments using the two-sample MR method (https://mrcieu.github.io/TwoSampleMR/articles/introduction.html). Initially, independent (r2 < 0.001) genome-wide significant SNPs (pGWAS < 5 × 10−8) associated with exposure (AD) were considered as instrumental variables (IVs) and assessed against outcome variables (FI and FP) and vice versa. We used the 5 MR methods (MR Egger, weighted median, inverse variance weighted (IVW), simple mode, and weighted mode) implemented in the 2SMR R package (Hemani et al., 2018).
Gene-based association analysis
We carried out gene-based association analyses to find genome-wide significant genes shared between AD and each of the frailty assessments. By combining the effects of multiple SNPs, this methodology enhances SNP-based studies and increases the power for detecting genetic risk variations. It additionally addresses the problem of smaller effect sizes or correlations amongst SNPs. We used the multi-marker analysis of genomic annotation (MAGMA) (de Leeuw et al., 2015) software (https://ctg.cncr.nl/software/magma) to conduct the gene-based association analysis for overlapping SNPs between AD and each of the frailty assessments. To annotate the SNPs present within the gene region and to carry out precise gene-based testing, we used the gene boundary length as within ‘±0 kb outside the gene’ downstream and upstream.
Genome-wide significant genes for AD and FI were defined at a corrected p-value <2.79 × 10−6 (Bonferroni correction for qualified 17,919 genes, 0.05/17,919), based on the MAGMA results. With an adjusted p-value of 2.83 × 10−6 (Bonferroni correction for qualified 17,683 genes (0.05/17,683), we also discovered genome-wide significant genes for AD and FP. Additionally, we retrieved their overlapping genes at gene-level p-value <0.1 (pgene < 0.1) to find the genes shared between AD and frailty. By implementing the Fisher’s combined p-value (FCP) analysis (Adewuyi et al., 2022) approach, we merged the FCP values for AD and frailty. We identified the common genes with genome-wide significance for AD and frailty at FCP <0.05.
Pathway-based analysis
To explore the underlying biological mechanisms between AD and frailty, we performed overrepresentation analysis (ORA) using the g:GOSt tool from the g-profiler software (Raudvere et al., 2019), a web-based functional pathway analysis tool. To perform analysis using user specified genes, the g:GOSt tool includes databases Gene Ontology, Human Protein Atlas, Wikipathways, Human Phenotype Ontology, CORUM, Kyoto Encyclopedia of Genes (KEGG), and Reactome. We attempted to interpret gene functionality between AD and frailty based on these findings. We chose the genes that were the output of the gene-based association analysis as input for pathway analysis. We used the default functional parameter in the tool (Reimand et al., 2019) for our analysis. The range of acceptable functional category term sizes was 5–1,000. We used the default ‘g: SCS threshold’ (set counts and sizes) for multiple testing correction and reported the significantly enriched pathways at the multiple testing adjusted p-value <0.05. We used the enrichment map from Cytoscape (Shannon et al., 2003) to visualize the pathways with FDR <10−3 and auto-annotate cluster to annotate the pathways.
Results
The workflow of our study is shown in Figure 1. We performed the analysis at three levels: SNP, gene, and pathway-based analysis (Adewuyi et al., 2022). First, in the SNP-based analysis, we computed the SNP-heritability and genetic correlation based on the LDSC method. The AD heritability was approximately 1.5% (h2SNP = 0.0155, SE = 0.0023), 12% for FI (h2SNP = 0.1169, SE = 0.0053), and 6% for FP (h2SNP = 0.0623, SE = 0.0027). There was no significant correlation between AD and FI (rg = −0.045, SE = 0.05, p = 0.3) and between AD and FP (rg = 0.01, SE = 0.04, p = 0.8). FI and FP have shown significant genetic correlation (rg = 0.75, SE = 0.02, p = 8.7e-309). Next, we performed GWAS meta-analyses to identify shared SNPs and loci associated with AD and frailty. We also used the pairwise GWAS colocalization method across the predefined genomic loci to identify the loci with shared genetic influence between AD and frailty. We assessed for any probable causal relationship between AD and frailty using MR. Finally, we conducted gene and pathway-level-based analyses to find the genes reaching genome-wide significance and the biological pathways between AD and frailty.
Cross-trait meta-analysis: shared and novel SNPs, loci, and genes between AD and frailty
We conducted cross-trait meta-analyses of AD and frailty utilizing GWAS summary statistics. We used a random effect meta-analysis to find SNPs that were not genome-wide significant in the individual AD or frailty GWAS (i.e., 5 × 10−8 < pGWAS < 0.05) but reached statistical significance in our analysis (pmeta-analysis < 5 × 10−8). Our meta-analyses found shared SNPs, some of which were novel for AD or/and frailty (Table 1). In our cross-trait meta-analyses, the SNPs with genome-wide significance in these loci were not reported to be significant in their original GWAS.
A total of 9,090,769 overlapping SNPs in the AD and FI GWAS were meta-analyzed in the combined 562,668 individuals. A total of 55 SNPs attained a genome-wide significance (Supplementary Table S2). To identify the independent and lead SNPs, we filtered them at LD r2 < 0.6 and subsequently at r2 < 0.1 in FUMA. Any overlapping SNPs within a 250 kb region were merged into a single locus, and the SNP with the least p-value was considered the lead SNP. We identified 8 genomic loci, each with a lead SNP. Of these 8 loci, 5 loci (3q25.1, 4p16.3, 6p21.2, 14q22.1, 18q21.2) were novel for both AD and FI, and 3 loci (11p11.2, 17q21.31, 17q21.33) were novel for FI (Table 1; Figure 2) and had pleiotropy association with AD. Based on the posterior probability (m-value), the effect exists for AD in all the SNPs, and, for FI, rs11039165 had no effect on FI, and rs28483960 had an ambiguous effect. These 8 lead SNPs were located in 11 genes (CLRN1-AS1, CRHR1, FERMT2, GRK4, LINC01929, LRFN2, MADD, RP11-368P15.1, RP11-166N6.2, RNA5SP459, and ZNF652), are either in their intronic (66.3%) or intergenic (33.7%) regions. All these genes are novel to FI. Five genes (CLRN1-AS1, GRK4, LINC01929, RP11-368P15.1, RP11-166N6.2) are novel to both AD and FI, and 3 (FERMT2, LRFN2, ZNF652) of them have been previously associated with AD or a family history of AD (Lambert et al., 2013; Marioni et al., 2018; Schwartzentruber et al., 2021; Wightman et al., 2021). The three RNA genes, CLRN1-AS1, RP11-166N6.2, and RP11-368P15.1, were not previously reported for any AD-related phenotype in the EBI GWAS Catalog.
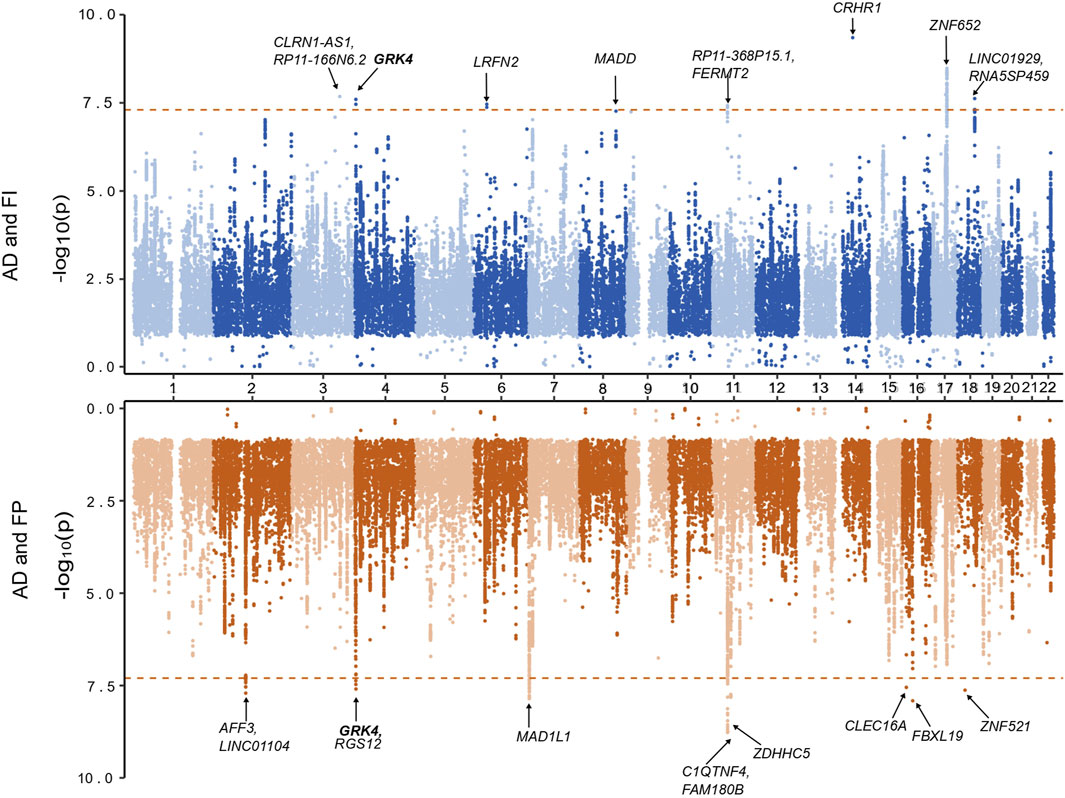
Figure 2. Miami plot based on the cross-trait meta-analyses of AD and frailty. The top plot is based on the meta-analysis of AD and the frailty index. The bottom plot is based on the meta-analysis of AD and frailty phenotype. The gene highlighted in bold is identified in both of the meta-analyses.
Additionally, we identified 115 independent SNPs at 83 loci at the genome-wide suggestive association (pmeta-analysis < 1 × 10−5). Five of the 8 genome-wide significant loci (excluding loci: 3q25.1, 6p21.2, 14q22.1) were replicated at genome-wide suggestive association level for AD and FI (Supplementary Table S4). Also, we found SNPs and loci that were already known to be associated (pGWAS < 5 × 10−8) with AD (AD lead SNPs) and, upon meta-analyses, were related to FI and vice versa (Supplementary Table S5).
Regarding the FP assessment, a total of 6,665,979 overlapping SNPs in the AD and FP GWAS were meta-analyzed in the combined 551,175 individuals. Meta-analysis of AD and FP identified 54 SNPs reaching a genome-wide significant association (Supplementary Table S3), and we identified 9 independent (r2 < 0.1) genomic loci. Of these 9 loci, 8 were novel for both AD and FP, and one locus was novel for FP only (Table 1; Figure 2), which was also identified in a pleiotropy study between AD and gastroesophageal reflux disease (GERD). Eleven genes (AFF3, C1QTNF4, CLEC16A, FAM180B, FBXL19, GRK4, LINC01104, MAD1L1, RGS12, ZDHHC5, and ZNF521) were mapped to the 9 lead SNPs and their regions are either intronic (66.3%), intergenic (16.7%) or downstream (16.7%). All the genes are novel to FP, 8 of the genes are novel to both AD and FP (MAD1L1 being the exception), and 3 genes (AFF3, LINC01104, MAD1L1) have been previously associated with the pleiotropy analysis of AD with educational attainment (EA) (Kulminski et al., 2022). Based on the posterior probability (m-value), the effect exists for both AD and FP in all the SNPs, and SNPS within the locus 11p11.2 (chr11:47611152—47232038) had an ambiguous effect on FP (Supplementary Table S3). The loci 4p16.3 and 11p11.2 and gene GRK4 were shared with both pairs of AD and frailty.
We identified 120 independent SNPs at 79 loci at the genome-wide suggestive association (pmeta-analysis < 1 × 10−5). Seven of the 8 significant genome-wide loci (excluding locus 18q11.2) were replicated at genome-wide suggestive association levels for AD and FP (Supplementary Table S5). Additionally, we found SNPs and loci that were already known to be associated (PGWAS < 5 × 10−8) with AD (AD lead SNPs) and, upon our meta-analyses, were related to FP too, and vice versa (see details in Supplementary Table S6).
Colocalization analysis: shared loci across genomic regions
We assessed the genomic regions shared between AD and frailty using GWAS-PW (Supplementary Tables S7, S8). The findings of this research imply that AD and frailty share all the loci found in the meta-analyses with varying posterior probability (PPA4 > 0.2) (Table 2). The posterior probability result (PPA3 < 0.2) of causal variants suggests that those variants in the locus may be in strong LD, which restricts the GWAS-PW analysis ability to differentiate between model 3, where the locus is shared between both traits, from model 4, where the locus is shared between both traits, but through other causal variant (Pickrell et al., 2016). Additional shared genomic regions with PPA4 > 0.9 were identified in chromosomes 6, 10, and 11 for AD and FI, and PPA4 > 0.8 were identified in chromosomes 1, 3, 5, 6, 7, 11, 14, and 19 (Supplementary Table S7). For AD and FP, genomic regions in chromosomes 7, 10, 11, 14, and 19 had PPA4 > 0.9 and PPA4 > 0.8 in chromosomes 2, 5, 6, 10, 11, 15, 16, and 17 (Supplementary Table S8).
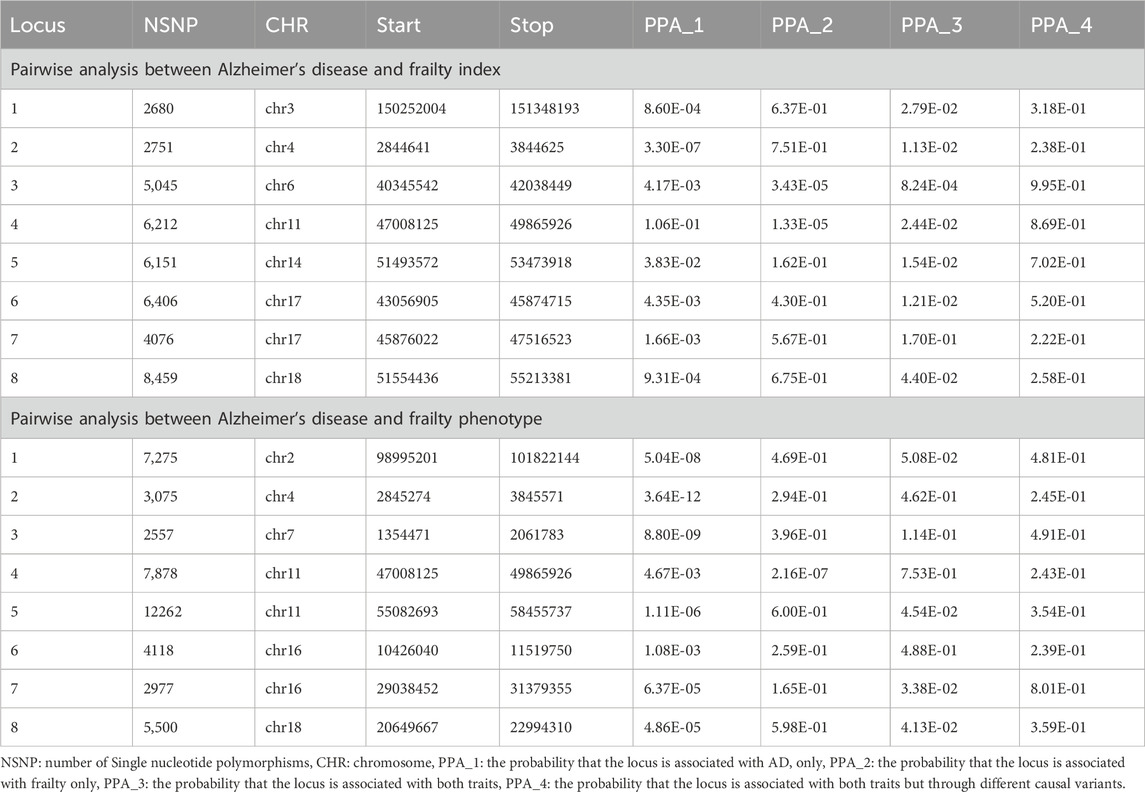
Table 2. Colocalization analysis on the meta-analysis significant loci between Alzheimer’s disease and frailty.
Causal association analysis by MR
Since both AD and frailty are diagnosed in the later stage of aging population, it is cumbersome to determine the temporality between these disorders. Therefore, to evaluate the probable causal relationship between AD and frailty, we used the two-sample MR method. Regardless of the direction of the investigation, we employed the 5 MR methods [MR Egger, weighted median, inverse variance weighted (IVW), simple mode, and weighted mode] implemented in the 2SMR R package. Although, no statistically significant evidence was detected for a causal association between AD and frailty (AD as outcome and frailty as exposure, and vice versa) within all the tests (Table 3), the effect of the variants is significant on these combined traits.
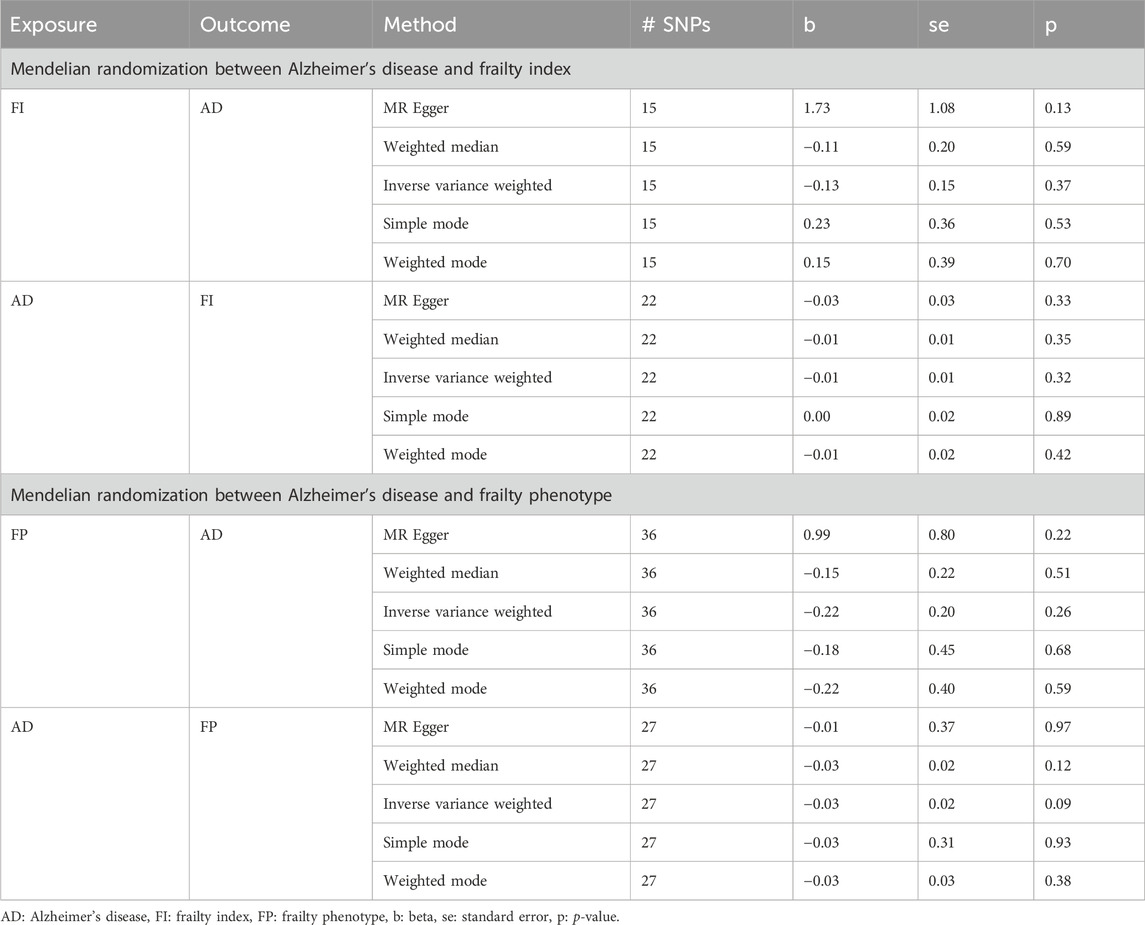
Table 3. Summary of Mendelian randomization analysis results between Alzheimer’s disease and frailty.
Gene association analysis identified AD-related genes
Using the overlapping SNPs between AD and FI, we performed gene-based analysis using MAGMA. We found a total of 17,919 protein-coding genes shared between AD and FI. By applying Bonferroni correction p-value cutoff (0.05/17919, 2.79 × 10−6), we identified 37 protein coding genome-wide significant genes for AD (Supplementary Table S9) and 43 for FI (Supplementary Table S10). A total of 17 genes (pgene < 2.79 × 10−6) shared between AD and FI were found by using the FCP technique (Supplementary Table S13), 16 of which were significant (pgene < 2.79 × 10−6). All these genes are novel for FI, and 3 of those genes are novel for both AD and FI (Supplementary Table S13). Eleven genes (ANCA7, ARHGAP45, C17orf107, CD33, CLU, CRHR1, KANSL1, LAMB2, MAPT, PICALM, SPPL2c) have been previously related to AD in the EBI GWAS Catalog. We found 2 overlapping loci (4p16.3 and 17q21.31) between gene analysis and SNP meta-analysis but no overlapping gene.
We found 17,683 protein-coding genes shared between AD and FP. After applying the Bonferroni correction (0.05/17683, p-value = 2.83 × 10−6), we identified 40 genome-wide significant genes for AD (Supplementary Table S11) and 86 for FP (Supplementary Table S12). A total of 41 shared genes were found using the FCP technique (Supplementary Table S14). All these genes were significant at the pgene < 2.83 × 10−6 level. All these genes were novel for FP, and 22 were novel for both AD and FP (Supplementary Table S14). Eighteen genes (ADAM10, ATXN2L, BCL1A, CD33, CELF1, CRHR1, EPHA1, FNBP4, KANSL1, LAMB2, MAD1L1, MAPT, MS4A3, NUP160, PICALM, PSMC3, SPI1, and SPPL2C) were previously related to AD in the EBI GWAS Catalog. There were 3 overlapping loci (4p16.3, 7p22.3, and 11p11.2) and genes (C1QTNF4, MAD1L1, and RGS12) between the gene analysis and SNP meta-analysis. CD33, CRHR1, KANSL1, LAMB2, and MAPT were the overlapping genes between FI and FP, with AD based on the SNP and gene-level analysis.
Pathway-based analysis
We used the g: Profiler, a web-based platform to perform pathway-based enrichment analysis to functionally interpret the genes that overlap between AD and frailty. We examined the genes associated with AD and frailty (pgene < 0.1, FCP <0.05) (Supplementary Table S15, S16). We found multiple enriched biological pathways, suggesting a shared role in the biological mechanisms between AD and FI. This analysis identified 50 significantly enriched biological pathways or processes, mostly related to receptor activity, amyloid fibril formation, lipoprotein particle, and leukocyte activation. These include serine/threonine kinase activity (padjusted = 1.18 × 10−4), protein homodimerization activity (padjusted = 6.12 × 10−4), plasma lipoprotein particle organization (padjusted = 8.81 × 10−5), Cdc42 protein signal transduction (padjusted = 5.46 × 10−4), amyloid fibril formation (padjusted = 2.86 × 10−8), among others (Supplementary Table S17). Cytoscape identified six major clusters: ‘homodimerization activity dimerization,’ ‘receptor serine activin,’ ‘lipoprotein particle plasma,’ ‘leukocyte activation cell,’ ‘cdc42 signal transduction’, and ‘amyloid fibril formation’ (Figure 3).
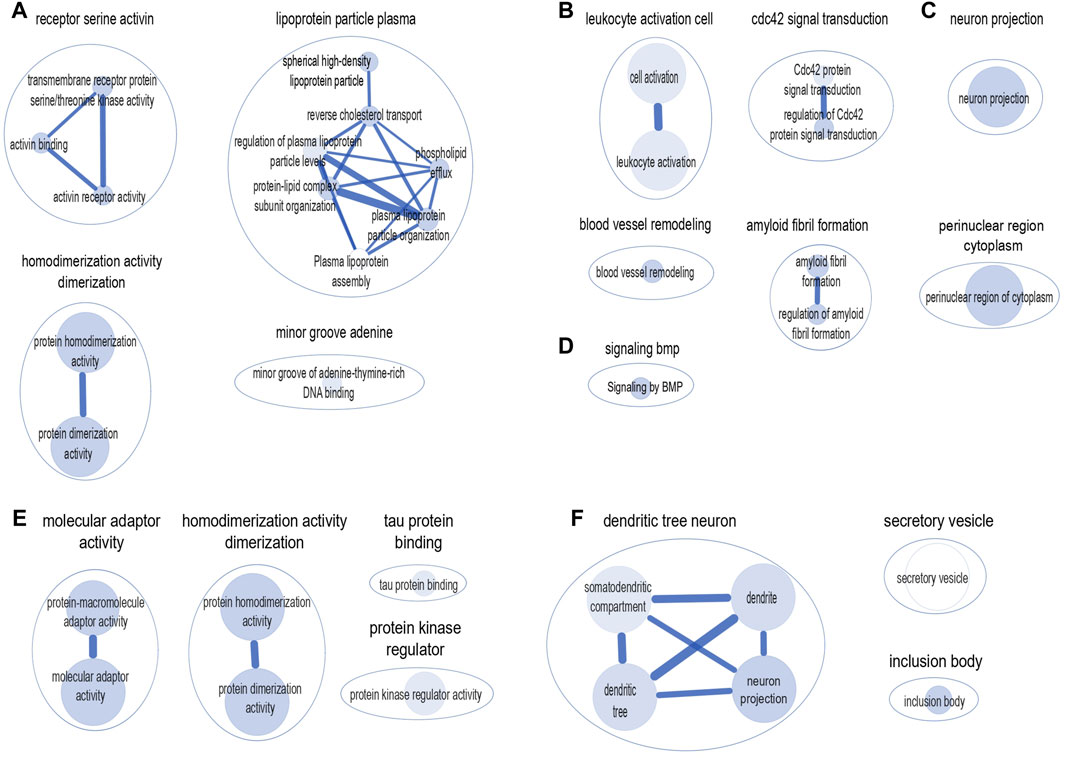
Figure 3. Clusters of biological pathways enriched with the genes shared between AD and frailty. Pathways enriched with the genes shared between AD and frailty index: (A) Gene Ontology: Molecular Function. (B) Gene Ontology: Biological Pathway. (C) Gene Ontology: Cellular Components. (D) Reactome: Biological Pathway. Pathways enriched with the genes shared between AD and frailty phenotype: (E) Gene Ontology: Molecular Function. (F) Gene Ontology: Cellular Components. The size of the inner circle represents the number of genes within the pathway. The bigger the circle, the higher the number of genes associated with that pathway. The color darkness of the inner circle denotes the strength of the p-value. The higher the p-value, the lighter the color of the circle.
Similarly, between AD and FP, 18 biological pathways were identified, which include ‘protein-macromolecule adaptor activity’ (padjusted = 1.25 × 10−4), ‘protein homodimerization activity’ (padjusted = 2.11 × 10−4), and ‘tau protein binding’ (padjusted = 4.51 × 10−4) (Supplementary Table S18). Cytoscape shows four major clusters: ‘molecular adaptor activity’, ‘homodimerization activity dimerization’, ‘protein kinase regulator’, and ‘tau protein binding’ (Figure 3). Of note, the ‘homodimerization pathway’ was shared between the two frailty assessments with AD.
Discussion
Epidemiological studies have reported comorbidity of aging disorders like AD with frailty, but no previous research has focused on the shared genetic architecture between AD and frailty. Similarly, in a cross-sectional study in mice models, increased frailty has been seen in AD (Kapphan et al., 2023). In addition, observational studies in humans have suggested a shared relationship between AD biomarkers and frailty (Wallace et al., 2018). In this study, we comprehensively assessed the genetic overlap, causal relationship, shared genes, and biological pathway between AD and frailty using GWAS summary statistics. Similar to the previous observational studies, our findings suggested a shared genetic architecture between AD and frailty.
In our analyses, LDSC did not provide a significant genetic correlation between AD and frailty, which may be due to the mixed effect of the influence of SNPs on each phenotype. The genetic correlation between FI and FP was 0.75. We did not find studies measuring the phenotypic correlation between FI and FP in the UK biobank population. To the best of our knowledge, longitudinal studies based on the European ancestry population showed a modest kappa agreement ranging from 0.38 to 0.45 (Thompson et al., 2018; Kim et al., 2022). The kappa score >0.8 suggests a strong agreement. The genetic correlation method, like LDSC, failed to capture the mixture of effect directions across the shared variants, i.e., nullifying the negative effect of the variant on one trait and the positive effect of the variant on another trait (Frei et al., 2019). Since the LDSC approach cannot reveal the completely shared variants associated with AD and frailty, we followed a multi-statistical approach to identify shared variants and genes at the SNP and gene levels and performed the pathway analysis to explore the shared biological mechanism.
The cross-trait meta-analyses identified 8 loci shared between AD and FI; among them, 3 (FERMT2, LRFN2, ZNF652) were previously associated with AD. The loci 11p11.2 (MADD) and 17q21.31 (CRHR1) were previously reported in the pleiotropy relationship between AD and EA (Kulminski et al., 2022). The loci 17q21.33 (ZNF652) was previously reported in the pleiotropic relationship between AD and GERD (Adewuyi et al., 2022). The cross-trait analysis of AD and FP identified 9 loci, 8 of which were novel for both AD and FP, and 1 locus was novel for FP only. All the genes were novel for FP, and 3 genes (AFF3, LINC01104, and MAD1L1) were in a pleiotropy relationship between AD and EA. The lead SNP rs1635298 (mapped to 17q21.31, CRHR1) has a RegulomeDB score of 1f, which suggests regulatory functionality. SNP rs1785498 (11q12.1, ZDHHC5) has a CADD score of 20, suggesting its deleteriousness. The colocalization analysis showed a moderate to high (20%-99%) probability of association signals at the loci identified from the meta-analyses and high association signals in the regions of chromosomes 1, 2, 3, 5, 6, 7, 11, 14, 15, 16, and 19 between AD and frailty. However, these association signals were not sufficiently strong to provide any bidirectional causal relationship between AD and frailty. The gene analyses by Fisher’s test identified several loci (4p16.3, 7p22.3, 11p11.2, and 17q21.31) and genes (RGS12, MAD1L1, and C1QTNF4) that were shared with the meta-analyses results.
Of note, there were several genes (AFF3, CRHR1, FERMT2, GRK4, LINC01104, LRFN2, MADD, MAD1L1, and ZNF652) identified in the meta-analyses were associated with AD or had a pleiotropic relationship with AD and its risk factors such as obesity-related traits (Comuzzie et al., 2012), cognitive performance (Lee et al., 2018), EA (Okbay et al., 2022), hypertension (Hoffmann et al., 2017), immune diseases (Johansson et al., 2019), neuropsychiatric disorders (Yao et al., 2021), and BMI (Tachmazidou et al., 2017). We discussed some of them below.
AFF3 is a putative transcription activator involved in oncogenesis and lymphoid development. It has been associated with immune diseases like rheumatoid arthritis and type 1 diabetes, plays role in controlling immune responses and protects against infections (Tsukumo et al., 2022). CRHR1, which showed regulatory functionality in our analyses, encodes a Gprotein coupled receptor for CRH (corticotropin-releasing factor) and UCN (urocortin). It is related to GPCR downstream signaling and activation of cAMP-dependent Protein kinase A (PKA) pathways. Activation of CRHR1 by CRH has been shown to increase the release of APP in rat cerebellar neurons, in human neuroblastoma IMR32 cell line, and in mouse hippocampal HT22 cells (Lezoualc’h et al., 2000). The reduction in CRH levels is associated with morphological abnormalities in brain areas affected by AD (Bissette et al., 1985; De Souza et al., 1986). Downregulation of the cAMP-response element-binding protein (CREB) in AD brains has been linked to cognitive and memory impairments (Liang et al., 2007). FERMT2 (encoding fermitin family member 2) is a scaffolding protein mediated by TLN1 and/or TLN2 that enhances integrin activation and plays a role in the TGFB1 and integrin signaling pathways. FERMT2, implicated in tau metabolism (Shulman et al., 2014), has also been associated with AD risk factors such as systolic and diastolic blood pressures (Hoffmann et al., 2017). The 4p16.3 (GRK4) locus was shared between AD and frailty. GRK4 (G Protein-Coupled Receptor Kinase 4), a paralog for GRK5, has been associated with AD pathology (Guimaraes et al., 2021) and is a potential drug target (Zhang et al., 2022). LINC01104, an RNA gene, has a pleiotropic relationship between AD and EA (Kulminski et al., 2022). LRFN2, encoding Leucine Rich Repeat and Fibronectin Type III Domain Containing 2, is a protein-coding gene that promotes neurite outgrowth in hippocampal neurons and processes the frequency of synaptic transmission from a neuron to a target cell across a synapse. A proteomic study of synaptic markers has shown a strong association of LRFN2 with cognitive decline in an AD population (Bereczki et al., 2018). LRFN2 is also associated with obesity-related traits (Comuzzie et al., 2012), cognitive performance (Lee et al., 2018), EA (Okbay et al., 2022), psychiatric conditions such as insomnia (Watanabe et al., 2022) and schizophrenia (Trubetskoy et al., 2022), and multiple cancers (lung cancer, gastric cancer, and squamous cell carcinoma) (Jin et al., 2012). MADD (MAP Kinase Activating Death Domain protein) belongs to the DENN protein family. It regulates the Rab family of small GTPases. MADD acts as a guanine nucleotide exchange factor (GEF) for Rab3, which is present on synaptic vesicles and regulates neurotransmitter release. MADD has been associated with several diseases, including Deeah syndrome, which affects developmental, intellectual growth, and neurodevelopmental disorders with dysmorphic facies, impaired speech, and hypotonia. MADD is associated with glycemic traits (blood insulin and glucose measurement) (Masotti et al., 2019), which impacts insulin resistance in depressed people, a common risk factor for AD and frailty (Fernandes et al., 2022). It is also involved in several pathways, including the TNF signaling and TNFR1 pathway. The TNFR1 and MADD proteins interact and mediate downstream protein signaling pathways that result in neuronal cell death and AD, possibly being drug targets for AD (Hassan et al., 2021). MAD1L1 is a part of the spindle-assembly checkpoint that delays the beginning of anaphase until all chromosomes are correctly positioned at the metaphase plate (Jin et al., 1999; Nakano et al., 2010; Ji et al., 2018). Neurodevelopmental processes in mice and human organoids have shown a role for MAD1L1 in the impairment of neuronal migration and neurite outgrowth (Goo et al., 2023). Gene ZNF652 is predicted to enable DNA-binding transcription factor activity, RNA polymerase II-specific, and RNA polymerase II cis-regulatory region sequence-specific DNA binding activity. It has been identified as a risk gene for hypertension (Hoffmann et al., 2017), one of the known AD risk factors. ZNF652 is associated with allergic diseases such as asthma, eczema, and allergic rhinitis (Johansson et al., 2019), and it was reported to be associated in a pleiotropic relationship between AD and asthma (Enduru et al., 2024).
The gene analyses cumulatively identified 77 genes for AD and 129 genes for frailty (includes both FI and FP). Overall, 58 genes were identified between AD and frailty at FCP cutoff and they help in the understanding of their shared biological mechanisms. Since frailty is classified based on multi-comorbidities, using the genes associated with AD and frailty can help to identify novel drug targets for multiple diseases and offer more treatment options for these comorbidities.
Our pathway enrichment analysis of the genes shared between AD and frailty revealed several pathways, including ‘Activin binding,’ ‘amyloid fibril formation,’ and ‘lipoprotein and dendrite tree neurons.’ Activins are members of the transforming growth factor β (TGFβ) family and play a pivotal role in signal transduction across the central nervous system (CNS). They serve as multifunctional regulatory proteins in many tissues and organs (Link et al., 2016). Activins can also activate pathways like ‘mitogen-activated protein kinase (MAPK) signaling’ (Moustakas and Heldin, 2005), which are involved in the pathophysiology and pathogenesis of AD (Zhu et al., 2002). Activin type II receptor, part of the TGFβ family has been related to loss of muscle in aging population and heart failure severity, the risk factors associated to frailty (Roh et al., 2019). ‘Amyloid fibrils’ are self-assembled fibrous protein structures of β-rich forms (Aβ1-40), linked to many currently incurable disorders, including AD and Parkinson’s disease (Ow and Dunstan, 2014). Studies have shown that neuronal disruption with the Aβ fibril formation results in symptoms similar to AD (Paola et al., 2000). Aβ has been a prominent protein in the study of amyloid fibril formation (AFF). Many therapeutics have focused on developing inhibitors capable of preventing AFF (Hardy and Selkoe, 2002; Liu et al., 2022) by using antibodies against AFF (Hardy and Selkoe, 2002; Mangialasche et al., 2010) and decreasing the production of Aβ, all of which could possibly halt the progression of AD (Hardy and Selkoe, 2002; Lichtenthaler, 2011). Varying plasma lipoprotein cholesterol levels of ApoA and ApoC have been suggested to alter regional brain volumes related to AD. Higher cholesterol/ApoA ratios were linked to lower cortical grey matter volume and higher ventricular volume. In contrast, higher ApoA and ApoJ/ApoA ratios were linked to higher cortical grey matter volume (and for ApoA-II, higher hippocampal volume) and lower ventricular volume (Pedrini et al., 2022). The shared ‘dendrite tree neuron pathway’ between AD and FI provides evidence for the association of excessive neuron loss and regression in dendrites in both AD and frailty (Coleman and Flood, 1987; Cochran et al., 2014).
In our study, some pathophysiology mechanisms have been identified and several biological pathways, both in each condition in isolation and shared between AD and frailty are reported, such as the inflammation pathway, which reported kynurenine pathway in association with AD (Fernandes et al., 2023) and frailty (Kim et al., 2020). Several observational studies reported the relationship between brain pathologies and frailty. Previous study showed rapid progression of frailty in the presence of pathologies related to macroinfarcts, AD, Lewy body and nigral neuronal loss (Buchman et al., 2013). Other similar studies have shown the accumulation of Aβ from cerebrospinal fluid to be associated to worsening frailty (Yoon et al., 2018; Maltais et al., 2019). In this line, our analysis provided evidence of genetic overlap between AD and frailty, similarly to observational studies that have suggested a consistent comorbid association between AD and frailty. Studies suggested that those with higher amount of frailty were more likely to have more AD pathology, which later are expressed as dementia (Wallace et al., 2019; Ward et al., 2022).
A key aspect of this study is the use of various complementary statistical genetic techniques, which allowed for a deep analysis of the genetic relationships between AD and frailty. We used only clinically diagnosed cases of AD to reduce the possibility of false-positive and false-negative findings. In addition, we used well-powered GWAS, which is considerably less affected by the small sample size frequently observed in traditional observational studies. Our results highlight the shared genomic loci between AD and frailty with minimal genetic correlation and no causality. Our study comes with some limitations. First, diagnosing frailty is challenging. The FI is based on health deficits accumulated during the life course, and the specific health deficits may vary from person to person. A minimum number of accumulated health deficits must be considered to adequately calculate the FI. The FP is based on the presence of three out of five physical components (weakness, slow walking speed, inadequate physical activity, exhaustion, and unexpected weight loss), and there may be misdiagnosis based on the participant’s responses to health questionnaires. Our analyses were restricted to only individuals of European ancestry; thus, our results may not be representative of other populations. Further genetic studies are needed to validate and refine our findings. For example, additional GWAS and genome sequencing data from cohorts ascertaining these phenotypes and the related large datasets from general cohorts such as UK Biobank and All of Us could provide further evidence to support of our findings. In addition, other types of omics data such as epigenetic data can provide additional biological evidence regarding genetic relationships. Finally, animal models and cell lines can provide additional tools to validate the potential function and phenotypic outcomes by functional genetic studies, including mutation knock in or out in mice.
To conclude, our study explores the shared genetic relationship between the two aging-related conditions, AD and frailty. Our study utilized the 54 frailty items which focused on various mental health conditions, cardiovascular diseases, immune-mediated diseases, pain, and physical frailty to mention a few (5 FP, 49 FI items) in the aging population. It provides some novel insights into the shared genetic architecture from SNP and gene-level to biological pathways that are associated with AD and frailty. Our findings based on SNP analyses show minimal genetic correlation but reveal significant shared loci and genes between AD and frailty, from gene-based analyses. These shared loci present a significant shared genetic association between AD and frailty with a varying posterior probability, with several loci (4p16.3, 7p22.3, 11p11.2, and 17q21.31) and genes (RGS12, MAD1L1, and C1QTNF4) being shared in the SNP meta-analyses and gene analyses. Finally, we identified several biological pathways common to AD and frailty. This is the first genetic study exploring the genetic and biological relationship between AD and frailty using extensive yet complementary statistical approaches. Overall, our findings provide evidence of genetic relationship between the commonly co-occurring conditions of AD and frailty.
Web resources
The software’s used in this study are freely available here: Genetic correlation analysis: https://github.com/bulik/ldsc.
Cross-trait analysis: http://genetics.cs.ucla.edu/meta colocalization analysis: https://github.com/joepickrell/gwas-pw mendelian randomization: https://mrcieu.github.io/TwoSampleMR/
MAGMA: https://ctg.cncr.nl/software/magma gprofiler: https://biit.cs.ut.ee/gprofiler/gost.
Cytoscape: https://cytoscape.org/
Data availability statement
Publicly available datasets were analyzed in this study. This data can be found here: The GWAS summary statistics for Alzheimer’s disease and frailty phenotype are available through the GWAS catalog (https://www.ebi.ac.uk/gwas/) under accession no. GCST013196 and GCST90020053. The GWAS summary statistics for frailty index is freely available in [https://figshare.com/articles/dataset/Genome-Wide_Association_Study_of_the_Frailty_Index_-_Atkins_et_al_2019/9204998].
Author contributions
NE: Conceptualization, Formal Analysis, Software, Visualization, Writing–original draft, Writing–review and editing, Data curation, Methodology. BF: Conceptualization, Supervision, Writing–original draft, Writing–review and editing, Methodology, Project administration. ZZ: Conceptualization, Funding acquisition, Investigation, Methodology, Resources, Supervision, Writing–original draft, Writing–review and editing.
Funding
The author(s) declare that financial support was received for the research, authorship, and/or publication of this article. This research was partially supported by National Institutes of Health grants awarded to ZZ (U01AG079847, R01LM012806, R01LM012806-07S1, and R03AG077191). We thanked the technical support from the Cancer Prevention and Research Institute of Texas (CPRIT RP180734). The funders had no role in the study design, data collection and analysis, decision to publish, or preparation of the manuscript.
Acknowledgments
We thank those investigators who generated and shared the genetic datasets used in the present study. We also thank the members of the Bioinformatics and Systems Medicine Laboratory for the valuable discussion and help.
Conflict of interest
The authors declare that the research was conducted in the absence of any commercial or financial relationships that could be construed as a potential conflict of interest.
The author(s) declared that they were an editorial board member of Frontiers, at the time of submission. This had no impact on the peer review process and the final decision.
Publisher’s note
All claims expressed in this article are solely those of the authors and do not necessarily represent those of their affiliated organizations, or those of the publisher, the editors and the reviewers. Any product that may be evaluated in this article, or claim that may be made by its manufacturer, is not guaranteed or endorsed by the publisher.
Supplementary material
The Supplementary Material for this article can be found online at: https://www.frontiersin.org/articles/10.3389/fgene.2024.1376050/full#supplementary-material
References
Adewuyi, E. O., O’Brien, E. K., Nyholt, D. R., Porter, T., and Laws, S. M. (2022). A large-scale genome-wide cross-trait analysis reveals shared genetic architecture between Alzheimer’s disease and gastrointestinal tract disorders. Commun. Biol. 5 (1), 691. doi:10.1038/s42003-022-03607-2
Alvarado, J. C., Fuentes-Santamaria, V., and Juiz, J. M. (2021). Frailty syndrome and oxidative stress as possible links between age-related hearing loss and Alzheimer’s disease. Front. Neurosci. 15, 816300. doi:10.3389/fnins.2021.816300
Alzheimers Dement (2023). 2023 Alzheimer’s disease facts and figures. Alzheimers Dement. 19 (4), 1598–1695. doi:10.1002/alz.13016
Atkins, J. L., Jylhava, J., Pedersen, N. L., Magnusson, P. K., Lu, Y., Wang, Y., et al. (2021). A genome-wide association study of the frailty index highlights brain pathways in ageing. Aging Cell 20 (9), e13459. doi:10.1111/acel.13459
Bereczki, E., Branca, R. M., Francis, P. T., Pereira, J. B., Baek, J. H., Hortobagyi, T., et al. (2018). Synaptic markers of cognitive decline in neurodegenerative diseases: a proteomic approach. Brain 141 (2), 582–595. doi:10.1093/brain/awx352
Bissette, G., Reynolds, G. P., Kilts, C. D., Widerlov, E., and Nemeroff, C. B. (1985). Corticotropin-releasing factor-like immunoreactivity in senile dementia of the Alzheimer type. Reduced cortical and striatal concentrations. JAMA 254 (21), 3067–3069. doi:10.1001/jama.254.21.3067
Boyle, A. P., Hong, E. L., Hariharan, M., Cheng, Y., Schaub, M. A., Kasowski, M., et al. (2012). Annotation of functional variation in personal genomes using RegulomeDB. Genome Res. 22 (9), 1790–1797. doi:10.1101/gr.137323.112
Buchman, A. S., Boyle, P. A., Wilson, R. S., Tang, Y., and Bennett, D. A. (2007). Frailty is associated with incident Alzheimer’s disease and cognitive decline in the elderly. Psychosom. Med. 69 (5), 483–489. doi:10.1097/psy.0b013e318068de1d
Buchman, A. S., Yu, L., Wilson, R. S., Schneider, J. A., and Bennett, D. A. (2013). Association of brain pathology with the progression of frailty in older adults. Neurology 80 (22), 2055–2061. doi:10.1212/WNL.0b013e318294b462
Bulik-Sullivan, B. K., Loh, P. R., Finucane, H. K., Ripke, S., and Yang, J. (2015). LD Score regression distinguishes confounding from polygenicity in genome-wide association studies. Nat. Genet. 47 (3), 291–295. doi:10.1038/ng.3211
Butler, A., Gallagher, D., Gillespie, P., Crosby, L., Ryan, D., Lacey, L., et al. (2016). Frailty: a costly phenomenon in caring for elders with cognitive impairment. Int. J. Geriatr. Psychiatry 31 (2), 161–168. doi:10.1002/gps.4306
Canevelli, M., Arisi, I., Bacigalupo, I., Arighi, A., Galimberti, D., Vanacore, N., et al. (2021). Biomarkers and phenotypic expression in Alzheimer’s disease: exploring the contribution of frailty in the Alzheimer’s Disease Neuroimaging Initiative. Geroscience 43 (2), 1039–1051. doi:10.1007/s11357-020-00293-y
Chen, X., Mao, G., and Leng, S. X. (2014). Frailty syndrome: an overview. Clin. Interv. Aging 9, 433–441. doi:10.2147/CIA.S45300
Clarke, L., Fairley, S., Zheng-Bradley, X., Streeter, I., Perry, E., Lowy, E., et al. (2017). The international Genome sample resource (IGSR): a worldwide collection of genome variation incorporating the 1000 Genomes Project data. Nucleic Acids Res. 45 (D1), D854–D859. doi:10.1093/nar/gkw829
Cochran, J. N., Hall, A. M., and Roberson, E. D. (2014). The dendritic hypothesis for Alzheimer’s disease pathophysiology. Brain Res. Bull. 103, 18–28. doi:10.1016/j.brainresbull.2013.12.004
Coleman, P. D., and Flood, D. G. (1987). Neuron numbers and dendritic extent in normal aging and Alzheimer’s disease. Neurobiol. Aging 8 (6), 521–545. doi:10.1016/0197-4580(87)90127-8
Comuzzie, A. G., Cole, S. A., Laston, S. L., Voruganti, V. S., Haack, K., Gibbs, R. A., et al. (2012). Novel genetic loci identified for the pathophysiology of childhood obesity in the Hispanic population. PLoS One 7 (12), e51954. doi:10.1371/journal.pone.0051954
Davies, N. M., Holmes, M. V., and Davey Smith, G. (2018). Reading Mendelian randomisation studies: a guide, glossary, and checklist for clinicians. BMJ 362, k601. doi:10.1136/bmj.k601
de Bakker, P. I., and Raychaudhuri, S. (2012). Interrogating the major histocompatibility complex with high-throughput genomics. Hum. Mol. Genet. 21 (R1), R29–R36. doi:10.1093/hmg/dds384
de Leeuw, C. A., Mooij, J. M., Heskes, T., and Posthuma, D. (2015). MAGMA: generalized gene-set analysis of GWAS data. PLoS Comput. Biol. 11 (4), e1004219. doi:10.1371/journal.pcbi.1004219
De Souza, E. B., Whitehouse, P. J., Kuhar, M. J., Price, D. L., and Vale, W. W. (1986). Reciprocal changes in corticotropin-releasing factor (CRF)-like immunoreactivity and CRF receptors in cerebral cortex of Alzheimer’s disease. Nature 319 (6054), 593–595. doi:10.1038/319593a0
Di Bona, D., Scapagnini, G., Candore, G., Castiglia, L., Colonna-Romano, G., Duro, G., et al. (2010). Immune-inflammatory responses and oxidative stress in Alzheimer’s disease: therapeutic implications. Curr. Pharm. Des. 16 (6), 684–691. doi:10.2174/138161210790883769
Enduru, N., Fernandes, B. S., Bahrami, S., Dai, Y., Andreassen, O. A., and Zhao, Z. (2024). Genetic overlap between Alzheimer’s disease and immune-mediated diseases: an atlas of shared genetic determinants and biological convergence. Mol. Psychiatry. doi:10.1038/s41380-024-02510-y
Fernandes, B. S., Inam, M. E., Enduru, N., Quevedo, J., and Zhao, Z. (2023). The kynurenine pathway in Alzheimer’s disease: a meta-analysis of central and peripheral levels. Braz J. Psychiatry 45 (3), 286–297. doi:10.47626/1516-4446-2022-2962
Fernandes, B. S., Salagre, E., Enduru, N., Grande, I., Vieta, E., and Zhao, Z. (2022). Insulin resistance in depression: a large meta-analysis of metabolic parameters and variation. Neurosci. Biobehav Rev. 139, 104758. doi:10.1016/j.neubiorev.2022.104758
Frei, O., Holland, D., Smeland, O. B., Shadrin, A. A., Fan, C. C., Maeland, S., et al. (2019). Bivariate causal mixture model quantifies polygenic overlap between complex traits beyond genetic correlation. Nat. Commun. 10 (1), 2417. doi:10.1038/s41467-019-10310-0
Fried, L. P., Ferrucci, L., Darer, J., Williamson, J. D., and Anderson, G. (2004). Untangling the concepts of disability, frailty, and comorbidity: implications for improved targeting and care. J. Gerontol. A Biol. Sci. Med. Sci. 59 (3), 255–263. doi:10.1093/gerona/59.3.m255
Fried, L. P., Tangen, C. M., Walston, J., Newman, A. B., Hirsch, C., Gottdiener, J., et al. (2001). Frailty in older adults: evidence for a phenotype. J. Gerontol. A Biol. Sci. Med. Sci. 56 (3), M146–M156. doi:10.1093/gerona/56.3.m146
Gomez-Gomez, M. E., and Zapico, S. C. (2019). Frailty, cognitive decline, neurodegenerative diseases and nutrition interventions. Int. J. Mol. Sci. 20 (11), 2842. doi:10.3390/ijms20112842
Goo, B. S., Mun, D. J., Kim, S., Nhung, T. T. M., Lee, S. B., Woo, Y., et al. (2023). Schizophrenia-associated Mitotic Arrest Deficient-1 (MAD1) regulates the polarity of migrating neurons in the developing neocortex. Mol. Psychiatry 28 (2), 856–870. doi:10.1038/s41380-022-01856-5
Guimaraes, T. R., Swanson, E., Kofler, J., and Thathiah, A. (2021). G protein-coupled receptor kinases are associated with Alzheimer’s disease pathology. Neuropathol. Appl. Neurobiol. 47 (7), 942–957. doi:10.1111/nan.12742
Han, B., and Eskin, E. (2011). Random-effects model aimed at discovering associations in meta-analysis of genome-wide association studies. Am. J. Hum. Genet. 88 (5), 586–598. doi:10.1016/j.ajhg.2011.04.014
Hardy, J., and Selkoe, D. J. (2002). The amyloid hypothesis of Alzheimer’s disease: progress and problems on the road to therapeutics. Science 297 (5580), 353–356. doi:10.1126/science.1072994
Hassan, M., Zahid, S., Alashwal, H., Kloczkowski, A., and Moustafa, A. A. (2021). Mechanistic insights into TNFR1/MADD death domains in Alzheimer’s disease through conformational molecular dynamic analysis. Sci. Rep. 11 (1), 12256. doi:10.1038/s41598-021-91606-4
Hemani, G., Zheng, J., Elsworth, B., Wade, K. H., Haberland, V., Baird, D., et al. (2018). The MR-Base platform supports systematic causal inference across the human phenome. Elife 7, e34408. doi:10.7554/eLife.34408
Hoffmann, T. J., Ehret, G. B., Nandakumar, P., Ranatunga, D., Schaefer, C., Kwok, P. Y., et al. (2017). Genome-wide association analyses using electronic health records identify new loci influencing blood pressure variation. Nat. Genet. 49 (1), 54–64. doi:10.1038/ng.3715
Ji, W., Luo, Y., Ahmad, E., and Liu, S. T. (2018). Direct interactions of mitotic arrest deficient 1 (MAD1) domains with each other and MAD2 conformers are required for mitotic checkpoint signaling. J. Biol. Chem. 293 (2), 484–496. doi:10.1074/jbc.RA117.000555
Jin, D. Y., Kozak, C. A., Pangilinan, F., Spencer, F., Green, E. D., and Jeang, K. T. (1999). Mitotic checkpoint locus MAD1L1 maps to human chromosome 7p22 and mouse chromosome 5. Genomics 55 (3), 363–364. doi:10.1006/geno.1998.5654
Jin, G., Ma, H., Wu, C., Dai, J., Zhang, R., Shi, Y., et al. (2012). Genetic variants at 6p21.1 and 7p15.3 are associated with risk of multiple cancers in Han Chinese. Am. J. Hum. Genet. 91 (5), 928–934. doi:10.1016/j.ajhg.2012.09.009
Johansson, A., Rask-Andersen, M., Karlsson, T., and Ek, W. E. (2019). Genome-wide association analysis of 350 000 Caucasians from the UK Biobank identifies novel loci for asthma, hay fever and eczema. Hum. Mol. Genet. 28 (23), 4022–4041. doi:10.1093/hmg/ddz175
Kapphan, L. M., Nguyen, V. T. T., Heinrich, I., Tuscher, O., Passauer, P., Schwiertz, A., et al. (2023). Comparison of frailty and chronological age as determinants of the murine gut microbiota in an Alzheimer’s disease mouse model. Microorganisms 11 (12), 2856. doi:10.3390/microorganisms11122856
Kim, B. J., Lee, S. H., and Koh, J. M. (2020). Clinical insights into the kynurenine pathway in age-related diseases. Exp. Gerontol. 130, 110793. doi:10.1016/j.exger.2019.110793
Kim, D. J., Massa, M. S., Clarke, R., Scarlett, S., O’Halloran, A. M., Kenny, R. A., et al. (2022). Variability and agreement of frailty measures and risk of falls, hospital admissions and mortality in TILDA. Sci. Rep. 12 (1), 4878. doi:10.1038/s41598-022-08959-7
Koch, G., Belli, L., Giudice, T. L., Lorenzo, F. D., Sancesario, G. M., Sorge, R., et al. (2013). Frailty among Alzheimer’s disease patients. CNS Neurol. Disord. Drug Targets 12 (4), 507–511. doi:10.2174/1871527311312040010
Kojima, G., Liljas, A., Iliffe, S., and Walters, K. (2017). Prevalence of frailty in mild to moderate Alzheimer’s disease: a systematic review and meta-analysis. Curr. Alzheimer Res. 14 (12), 1256–1263. doi:10.2174/1567205014666170417104236
Kulminski, A. M., Loiko, E., Loika, Y., and Culminskaya, I. (2022). Pleiotropic predisposition to Alzheimer’s disease and educational attainment: insights from the summary statistics analysis. Geroscience 44 (1), 265–280. doi:10.1007/s11357-021-00484-1
Lambert, J. C., Grenier-Boley, B., Chouraki, V., Heath, S., Zelenika, D., Fievet, N., et al. (2010). Implication of the immune system in Alzheimer’s disease: evidence from genome-wide pathway analysis. J. Alzheimers Dis. 20 (4), 1107–1118. doi:10.3233/JAD-2010-100018
Lambert, J. C., Ibrahim-Verbaas, C. A., Harold, D., Naj, A. C., Sims, R., Bellenguez, C., et al. (2013). Meta-analysis of 74,046 individuals identifies 11 new susceptibility loci for Alzheimer’s disease. Nat. Genet. 45 (12), 1452–1458. doi:10.1038/ng.2802
Lee, J. J., Wedow, R., Okbay, A., Kong, E., Maghzian, O., Zacher, M., et al. (2018). Gene discovery and polygenic prediction from a genome-wide association study of educational attainment in 1.1 million individuals. Nat. Genet. 50 (8), 1112–1121. doi:10.1038/s41588-018-0147-3
Lezoualc’h, F., Engert, S., Berning, B., and Behl, C. (2000). Corticotropin-releasing hormone-mediated neuroprotection against oxidative stress is associated with the increased release of non-amyloidogenic amyloid beta precursor protein and with the suppression of nuclear factor-kappaB. Mol. Endocrinol. 14 (1), 147–159. doi:10.1210/mend.14.1.0403
Liang, Z., Liu, F., Grundke-Iqbal, I., Iqbal, K., and Gong, C. X. (2007). Down-regulation of cAMP-dependent protein kinase by over-activated calpain in Alzheimer disease brain. J. Neurochem. 103 (6), 2462–2470. doi:10.1111/j.1471-4159.2007.04942.x
Lichtenthaler, S. F. (2011). α-secretase in Alzheimer’s disease: molecular identity, regulation and therapeutic potential. J. Neurochem. 116 (1), 10–21. doi:10.1111/j.1471-4159.2010.07081.x
Link, A. S., Zheng, F., and Alzheimer, C. (2016). Activin signaling in the pathogenesis and therapy of neuropsychiatric diseases. Front. Mol. Neurosci. 9, 32. doi:10.3389/fnmol.2016.00032
Liu, A., Manuel, A. M., Dai, Y., Fernandes, B. S., Enduru, N., Jia, P., et al. (2022). Identifying candidate genes and drug targets for Alzheimer’s disease by an integrative network approach using genetic and brain region-specific proteomic data. Hum. Mol. Genet. 31 (19), 3341–3354. doi:10.1093/hmg/ddac124
Maltais, M., De Souto Barreto, P., Hooper, C., Payoux, P., Rolland, Y., Vellas, B., et al. (2019). Association between brain β-amyloid and frailty in older adults. J. Gerontol. A Biol. Sci. Med. Sci. 74 (11), 1747–1752. doi:10.1093/gerona/glz009
Mangialasche, F., Solomon, A., Winblad, B., Mecocci, P., and Kivipelto, M. (2010). Alzheimer’s disease: clinical trials and drug development. Lancet Neurol. 9 (7), 702–716. doi:10.1016/S1474-4422(10)70119-8
Marioni, R. E., Harris, S. E., Zhang, Q., McRae, A. F., Hagenaars, S. P., Hill, W. D., et al. (2018). GWAS on family history of Alzheimer’s disease. Transl. Psychiatry 8 (1), 99. doi:10.1038/s41398-018-0150-6
Masotti, M., Guo, B., and Wu, B. (2019). Pleiotropy informed adaptive association test of multiple traits using genome-wide association study summary data. Biometrics 75 (4), 1076–1085. doi:10.1111/biom.13076
Mhaolain, A. M., Gallagher, D., Crosby, L., Ryan, D., Lacey, L., Coen, R. F., et al. (2012). Frailty and quality of life for people with Alzheimer’s dementia and mild cognitive impairment. Am. J. Alzheimers Dis. Other Demen 27 (1), 48–54. doi:10.1177/1533317511435661
Mitnitski, A. B., Mogilner, A. J., and Rockwood, K. (2001). Accumulation of deficits as a proxy measure of aging. ScientificWorldJournal 1, 323–336. doi:10.1100/tsw.2001.58
Moustakas, A., and Heldin, C. H. (2005). Non-Smad TGF-beta signals. J. Cell Sci. 118 (Pt 16), 3573–3584. doi:10.1242/jcs.02554
Nakano, H., Funasaka, T., Hashizume, C., and Wong, R. W. (2010). Nucleoporin translocated promoter region (Tpr) associates with dynein complex, preventing chromosome lagging formation during mitosis. J. Biol. Chem. 285 (14), 10841–10849. doi:10.1074/jbc.M110.105890
Okbay, A., Wu, Y., Wang, N., Jayashankar, H., Bennett, M., Nehzati, S. M., et al. (2022). Polygenic prediction of educational attainment within and between families from genome-wide association analyses in 3 million individuals. Nat. Genet. 54 (4), 437–449. doi:10.1038/s41588-022-01016-z
Ow, S. Y., and Dunstan, D. E. (2014). A brief overview of amyloids and Alzheimer’s disease. Protein Sci. 23 (10), 1315–1331. doi:10.1002/pro.2524
Paola, D., Domenicotti, C., Nitti, M., Vitali, A., Borghi, R., Cottalasso, D., et al. (2000). Oxidative stress induces increase in intracellular amyloid beta-protein production and selective activation of betaI and betaII PKCs in NT2 cells. Biochem. Biophys. Res. Commun. 268 (2), 642–646. doi:10.1006/bbrc.2000.2164
Pedrini, S., Doecke, J. D., Hone, E., Wang, P., Thota, R., Bush, A. I., et al. (2022). Plasma high-density lipoprotein cargo is altered in Alzheimer’s disease and is associated with regional brain volume. J. Neurochem. 163 (1), 53–67. doi:10.1111/jnc.15681
Pickrell, J. K., Berisa, T., Liu, J. Z., Segurel, L., Tung, J. Y., and Hinds, D. A. (2016). Detection and interpretation of shared genetic influences on 42 human traits. Nat. Genet. 48 (7), 709–717. doi:10.1038/ng.3570
Rajan, K. B., Weuve, J., Barnes, L. L., McAninch, E. A., Wilson, R. S., and Evans, D. A. (2021). Population estimate of people with clinical Alzheimer’s disease and mild cognitive impairment in the United States (2020-2060). Alzheimers Dement. 17 (12), 1966–1975. doi:10.1002/alz.12362
Raudvere, U., Kolberg, L., Kuzmin, I., Arak, T., Adler, P., Peterson, H., et al. (2019). g:Profiler: a web server for functional enrichment analysis and conversions of gene lists (2019 update). Nucleic Acids Res. 47 (W1), W191–W198. doi:10.1093/nar/gkz369
Reimand, J., Isserlin, R., Voisin, V., Kucera, M., Tannus-Lopes, C., Rostamianfar, A., et al. (2019). Pathway enrichment analysis and visualization of omics data using g:Profiler, GSEA, Cytoscape and EnrichmentMap. Nat. Protoc. 14 (2), 482–517. doi:10.1038/s41596-018-0103-9
Rentzsch, P., Witten, D., Cooper, G. M., Shendure, J., and Kircher, M. (2019). CADD: predicting the deleteriousness of variants throughout the human genome. Nucleic Acids Res. 47 (D1), D886–D894. doi:10.1093/nar/gky1016
Roh, J. D., Hobson, R., Chaudhari, V., Quintero, P., Yeri, A., Benson, M., et al. (2019). Activin type II receptor signaling in cardiac aging and heart failure. Sci. Transl. Med. 11 (482), eaau8680. doi:10.1126/scitranslmed.aau8680
Sabbatinelli, J., Ramini, D., Giuliani, A., Recchioni, R., Spazzafumo, L., and Olivieri, F. (2021). Connecting vascular aging and frailty in Alzheimer’s disease. Mech. Ageing Dev. 195, 111444. doi:10.1016/j.mad.2021.111444
Sargent, L., Nalls, M., Starkweather, A., Hobgood, S., Thompson, H., Amella, E. J., et al. (2018). Shared biological pathways for frailty and cognitive impairment: a systematic review. Ageing Res. Rev. 47, 149–158. doi:10.1016/j.arr.2018.08.001
Scheltens, P., Blennow, K., Breteler, M. M., de Strooper, B., Frisoni, G. B., Salloway, S., et al. (2016). Alzheimer’s disease. Lancet 388 (10043), 505–517. doi:10.1016/S0140-6736(15)01124-1
Schwartzentruber, J., Cooper, S., Liu, J. Z., Barrio-Hernandez, I., Bello, E., Kumasaka, N., et al. (2021). Genome-wide meta-analysis, fine-mapping and integrative prioritization implicate new Alzheimer’s disease risk genes. Nat. Genet. 53 (3), 392–402. doi:10.1038/s41588-020-00776-w
Shannon, P., Markiel, A., Ozier, O., Baliga, N. S., Wang, J. T., Ramage, D., et al. (2003). Cytoscape: a software environment for integrated models of biomolecular interaction networks. Genome Res. 13 (11), 2498–2504. doi:10.1101/gr.1239303
Shulman, J. M., Imboywa, S., Giagtzoglou, N., Powers, M. P., Hu, Y., Devenport, D., et al. (2014). Functional screening in Drosophila identifies Alzheimer’s disease susceptibility genes and implicates Tau-mediated mechanisms. Hum. Mol. Genet. 23 (4), 870–877. doi:10.1093/hmg/ddt478
Siriwardhana, D. D., Hardoon, S., Rait, G., Weerasinghe, M. C., and Walters, K. R. (2018). Prevalence of frailty and prefrailty among community-dwelling older adults in low-income and middle-income countries: a systematic review and meta-analysis. BMJ Open 8 (3), e018195. doi:10.1136/bmjopen-2017-018195
Sollis, E., Mosaku, A., Abid, A., Buniello, A., Cerezo, M., Gil, L., et al. (2023). The NHGRI-EBI GWAS Catalog: knowledgebase and deposition resource. Nucleic Acids Res. 51 (D1), D977–D985. doi:10.1093/nar/gkac1010
Tachmazidou, I., Suveges, D., Min, J. L., Ritchie, G. R. S., Steinberg, J., Walter, K., et al. (2017). Whole-genome sequencing coupled to imputation discovers genetic signals for anthropometric traits. Am. J. Hum. Genet. 100 (6), 865–884. doi:10.1016/j.ajhg.2017.04.014
Thompson, M. Q., Theou, O., Yu, S., Adams, R. J., Tucker, G. R., and Visvanathan, R. (2018). Frailty prevalence and factors associated with the frailty phenotype and frailty index: findings from the north west adelaide health study. Australas. J. Ageing 37 (2), 120–126. doi:10.1111/ajag.12487
Trubetskoy, V., Pardinas, A. F., Qi, T., Panagiotaropoulou, G., Awasthi, S., Bigdeli, T. B., et al. (2022). Mapping genomic loci implicates genes and synaptic biology in schizophrenia. Nature 604 (7906), 502–508. doi:10.1038/s41586-022-04434-5
Tsukumo, S. I., Subramani, P. G., Seija, N., Tabata, M., Maekawa, Y., Mori, Y., et al. (2022). AFF3, a susceptibility factor for autoimmune diseases, is a molecular facilitator of immunoglobulin class switch recombination. Sci. Adv. 8 (34), eabq0008. doi:10.1126/sciadv.abq0008
Wallace, L., Theou, O., Rockwood, K., and Andrew, M. K. (2018). Relationship between frailty and Alzheimer’s disease biomarkers: a scoping review. Alzheimers Dement. (Amst) 10, 394–401. doi:10.1016/j.dadm.2018.05.002
Wallace, L. M. K., Theou, O., Godin, J., Andrew, M. K., Bennett, D. A., and Rockwood, K. (2019). Investigation of frailty as a moderator of the relationship between neuropathology and dementia in Alzheimer’s disease: a cross-sectional analysis of data from the Rush Memory and Aging Project. Lancet Neurol. 18 (2), 177–184. doi:10.1016/S1474-4422(18)30371-5
Wang, K., Li, M., and Hakonarson, H. (2010). ANNOVAR: functional annotation of genetic variants from high-throughput sequencing data. Nucleic Acids Res. 38 (16), e164. doi:10.1093/nar/gkq603
Ward, D. D., Ranson, J. M., Wallace, L. M. K., Llewellyn, D. J., and Rockwood, K. (2022). Cumulative health deficits, APOE genotype, and risk for later-life mild cognitive impairment and dementia. J. Neurol. Neurosurg. Psychiatry 93 (4), 136–142. doi:10.1136/jnnp-2020-324081
Watanabe, K., Jansen, P. R., Savage, J. E., Nandakumar, P., Wang, X., andMe Research, T., et al. (2022). Genome-wide meta-analysis of insomnia prioritizes genes associated with metabolic and psychiatric pathways. Nat. Genet. 54 (8), 1125–1132. doi:10.1038/s41588-022-01124-w
Watanabe, K., Taskesen, E., van Bochoven, A., and Posthuma, D. (2017). Functional mapping and annotation of genetic associations with FUMA. Nat. Commun. 8 (1), 1826. doi:10.1038/s41467-017-01261-5
Wightman, D. P., Jansen, I. E., Savage, J. E., Shadrin, A. A., Bahrami, S., Holland, D., et al. (2021). A genome-wide association study with 1,126,563 individuals identifies new risk loci for Alzheimer’s disease. Nat. Genet. 53 (9), 1276–1282. doi:10.1038/s41588-021-00921-z
Wightman, H., Quinn, T. J., Mair, F. S., Lewsey, J., McAllister, D. A., and Hanlon, P. (2023). Frailty in randomised controlled trials for dementia or mild cognitive impairment measured via the frailty index: prevalence and prediction of serious adverse events and attrition. Alzheimers Res. Ther. 15 (1), 110. doi:10.1186/s13195-023-01260-3
Yao, X., Glessner, J. T., Li, J., Qi, X., Hou, X., Zhu, C., et al. (2021). Integrative analysis of genome-wide association studies identifies novel loci associated with neuropsychiatric disorders. Transl. Psychiatry 11 (1), 69. doi:10.1038/s41398-020-01195-5
Ye, Y., Noche, R. B., Szejko, N., Both, C. P., Acosta, J. N., Leasure, A. C., et al. (2023). A genome-wide association study of frailty identifies significant genetic correlation with neuropsychiatric, cardiovascular, and inflammation pathways. Geroscience 45 (4), 2511–2523. doi:10.1007/s11357-023-00771-z
Yoon, D. H., Lee, J. Y., Shin, S. A., Kim, Y. K., and Song, W. (2018). Physical frailty and amyloid-β deposits in the brains of older adults with cognitive frailty. J. Clin. Med. 7 (7), 169. doi:10.3390/jcm7070169
Zhang, Y., Zhang, J., Wang, J., Chen, H., Ouyang, L., and Wang, Y. (2022). Targeting GRK2 and GRK5 for treating chronic degenerative diseases: advances and future perspectives. Eur. J. Med. Chem. 243, 114668. doi:10.1016/j.ejmech.2022.114668
Keywords: frailty index, frailty phenotype, GWAS, colocalization, Mendelian randomization, genetic correlation, gene association, pathway analysis
Citation: Enduru N, Fernandes BS and Zhao Z (2024) Dissecting the shared genetic architecture between Alzheimer’s disease and frailty: a cross-trait meta-analyses of genome-wide association studies. Front. Genet. 15:1376050. doi: 10.3389/fgene.2024.1376050
Received: 24 January 2024; Accepted: 04 April 2024;
Published: 19 April 2024.
Edited by:
Phillip E. Melton, University of Tasmania, AustraliaReviewed by:
Sanila Amber, National University of Sciences and Technology (NUST), PakistanYeunjoo E. Song, Case Western Reserve University, United States
Vincent P. Diego, Department of Human Genetics, School of Medicine, The University of Texas Rio Grande Valley, United States
Copyright © 2024 Enduru, Fernandes and Zhao. This is an open-access article distributed under the terms of the Creative Commons Attribution License (CC BY). The use, distribution or reproduction in other forums is permitted, provided the original author(s) and the copyright owner(s) are credited and that the original publication in this journal is cited, in accordance with accepted academic practice. No use, distribution or reproduction is permitted which does not comply with these terms.
*Correspondence: Zhongming Zhao, emhvbmdtaW5nLnpoYW9AdXRoLnRtYy5lZHU=