- 1Department of Medical Laboratory Sciences, College of Applied Medical Sciences, University of Bisha, Bisha, Saudi Arabia
- 2Department of Pathology, College of Medicine, University of Bisha, Bisha, Saudi Arabia
- 3Department of Microbiology and Clinical Parasitology, College of Medicine, University of Bisha, Bisha, Saudi Arabia
Introduction: Thrombosis is a serious vascular disorder influenced by genetic factors, including nonsynonymous single nucleotide polymorphisms (nsSNPs) in the PROCR gene, which encodes the endothelial protein C receptor (EPCR). These mutations may disrupt EPCR stability and impair its anticoagulant function, thereby increasing the risk of thrombosis.
Methods: We employed a multi-layered computational approach to analyze 217 nsSNPs in the PROCR gene. Functional impacts were predicted using Sorting Intolerant From Tolerant (SIFT), Polymorphism Phenotyping v2 (PolyPhen-2), Screening for Non-Acceptable Polymorphisms 2 (SNAP2), and Protein Analysis Through Evolutionary Relationships (PANTHER). Disease associations were assessed using Single Nucleotide Polymorphisms and Gene Ontology (SNP&GO) and Predictor of Human Deleterious Single Nucleotide Polymorphisms (PhD-SNP). Protein stability was evaluated using I-Mutant and MUpro, while structural implications were analyzed with Mutation Prediction (MutPred), ConSurf, and Have Our Protein Explained (HOPE). Active binding sites were identified using PyMOL. Finally, 100-nanosecond molecular dynamics (MD) simulations were conducted using GROningen MAchine for Chemical Simulations (GROMACS) to compare structural deviations, flexibility, and solvent interactions between wild-type EPCR and key mutant proteins.
Results: Our integrated analysis identified three high-risk nsSNPs—T174I, N136I, and L168P—that detrimentally affect EPCR function. These variants disrupt critical glycosylation sites, α-helix integrity, and catalytic residues, leading to increased root mean square deviation (RMSD) and root mean square fluctuation (RMSF), reduced hydrogen bonding, and higher solvent-accessible surface area (SASA) in mutants compared to the wild-type. Disease association tools further linked these mutations to an elevated thrombotic risk.
Discussion: These findings suggest that the identified nsSNPs destabilize EPCR by altering its structural dynamics and reducing its capacity to activate protein C. This provides mechanistic insight into how PROCR variation may contribute to thrombotic disorders and highlights the utility of in silico approaches for prioritizing potentially pathogenic variants.
Conclusion: Our study demonstrates that deleterious nsSNPs in the PROCR gene can significantly impair EPCR stability and function, thereby increasing susceptibility to thrombosis. These findings provide a foundation for future experimental validation and may inform the development of personalized therapeutic strategies for managing thrombotic disorders.
1 Introduction
Thrombosis is a severe health disorder characterized by the formation of blood clots that restrict or block normal blood flow through the circulatory system (Koupenova et al., 2017). This can result in serious conditions such as stroke, myocardial infarction (Mackman, 2012), or venous thromboembolism. The endothelial protein C receptor (EPCR), encoded by the PROCR gene, is a key component of the anticoagulation pathway. It facilitates the activation of protein C, which plays a critical role in degrading the procoagulant factors Va and VIIIa and directly inhibiting the formation of pathological clots (Dahlbäck and Villoutreix, 2005). Genetic variants, particularly nonsynonymous single nucleotide polymorphisms (nsSNPs), in the PROCR gene can significantly alter the structure and function of the endothelial protein C receptor (EPCR). EPCR plays a crucial anticoagulant role by binding to activated protein C (APC) and enhancing its proteolytic inactivation of coagulation factors Va and VIIIa, thereby reducing thrombin generation. Mutations that destabilize EPCR or disrupt its APC-binding region can impair this anticoagulant function. Some nsSNPs lead to reduced cell-surface expression of EPCR or modify its glycosylation pattern, affecting receptor trafficking and recycling. Others enhance the shedding of soluble EPCR (sEPCR), which competes with membrane-bound EPCR for APC binding and promotes a prothrombotic state. For instance, the Ser219Gly variant has been associated with elevated sEPCR levels and an increased risk of venous thromboembolism and myocardial infarction. While nsSNPs may also influence gene expression, splicing, or protein trafficking, this study focuses specifically on their effects on EPCR’s structural stability and conformation (Reiner et al., 2008; Kallel et al., 2012). This emphasis is grounded in the fact that EPCR function depends critically on the integrity of its three-dimensional structure, particularly the ligand-binding groove and membrane-anchoring domains. Alterations in folding or flexibility can disrupt APC interaction, increase susceptibility to proteolytic cleavage, or reduce cell-surface localization—mechanisms that directly elevate thrombotic risk. Furthermore, many deleterious nsSNPs exert their effects at the post-translational level rather than at the transcriptional level. Thus, structural investigation through molecular dynamics simulations offers a mechanistic framework for understanding how specific amino acid substitutions may destabilize EPCR and compromise its anticoagulant role (Saposnik et al., 2004; Qu et al., 2007; Medina et al., 2005). Previous studies have emphasized the gene’s importance in maintaining vascular integrity and proper blood coagulation (Esmon, 2003; Van de Wouwer et al., 2004; Feistritzer and Riewald, 2005; Taylor et al., 2001). Specifically, nsSNPs that induce amino acid substitutions are known to affect both the structural and functional integrity of the EPCR protein (Van de Wouwer et al., 2004; Feistritzer and Riewald, 2005; Fukudome and Esmon, 1994). These genetic variants may lead to either gain or loss of function, both of which are associated with an increased risk of thrombosis (Taylor et al., 2001). To date, no comprehensive mechanistic studies have elucidated how these nsSNPs affect EPCR stability and function. Molecular dynamics simulations have increasingly been employed to explore the impact of genetic variation on protein structure and function (Hollingsworth and Dror, 2018; Lemkul, 2018; Shaw et al., 2010; Karplus and McCammon, 2002). These simulations allow researchers to track protein behavior in a near-native environment over time, offering insights into the stability of both wild-type and mutant proteins.
This gene has been reported to be crucial for maintaining vascular integrity and blood coagulation by former studies (Esmon, 2003; Van de Wouwer et al., 2004; Feistritzer and Riewald, 2005; Taylor et al., 2001). Mutations in the PROCR gene are known to affect both the structural and functional integrity of EPCR protein, especially nsSNPs that induce amino acid substitutions (Van de Wouwer et al., 2004; Feistritzer and Riewald, 2005; Fukudome and Esmon, 1994). These mutations can be gain or loss of function, and both result in an increased thrombotic risk (Taylor et al., 2001). No detailed mechanistic study on how these nsSNPs specifically affect the stability and function of EPCR protein could be identified. Molecular dynamics simulations have been increasingly used to explore the consequences of genetic variation disrupting protein structure and function (Hollingsworth and Dror, 2018; Lemkul, 2018; Shaw et al., 2010; Karplus and McCammon, 2002). Using these simulations, thereby following the behavior of proteins in their natural environment over time gives us access to not only wild-type protein stability dynamics but also dynamics associated with disease mutations. This study aims to identify and characterize the functional impact of deleterious nonsynonymous single nucleotide polymorphisms (nsSNPs) in the PROCR gene using an integrative computational approach. Specifically, we seek to determine how these mutations may affect protein structure, stability, and function, and whether they may contribute to thrombotic risk. We hypothesize that certain nsSNPs, particularly those occurring in conserved or functionally important domains of EPCR, induce conformational changes that impair ligand binding, alter structural stability, or increase solvent accessibility. To test this hypothesis, we employed a multi-step workflow involving variant prioritization, conservation analysis, structural modeling, and molecular dynamics simulations.
2 Materials and methods
2.1 Work plan
The work plan (Figure 1) involves analyzing missense SNPs in the PROCR gene using data from the SNP database (dbSNP) of the National Center for Biotechnology Information (NCBI) and Universal Protein Resource (UniProt). Functional impacts were predicted with SIFT, PolyPhen2, SNAP2, and PANTHER, while disease associations with thrombosis were assessed using PhD-SNP and SNPs&GO. Protein stability effects were quantified with I-Mutant and MUpro, and structural-functional implications were evaluated using MutPred. Conservation analysis was performed with ConSurf, biochemical impacts with HOPE, and active binding sites were mapped using PyMol. Molecular dynamics simulations in GROMACS provided structural insights analyzed with tools like g_rms and g_rmsf and visualized in XMGRACE.
2.2 Data collection
Missense SNP data for the PROCR gene were collected from the dbSNP database (NCBI) (https://www.ncbi.nlm.nih.gov) using gene ID 10544 and accession number NG_032899.2. In addition, the UniProt ID Q9UNN8 and PDB ID: 4V3D, were used to retrieve EPCR protein sequences and functional annotations from the UniProt database (https://www.uniprot.org) for further analysis.
2.3 SNPs-based functional impact scoring
The effect of the identified SNPs on EPCR protein function was evaluated using several predictive bioinformatics tools to provide solid and reliable predictions. SIFT, the application was used to determine whether an amino acid substitution affects the function of a protein by exploiting sequence homology and physical characteristics of the amino acids in the proteins (https://sift.bii.a-star.edu.sg/www/SIFT_seq_submit2.html) (Choi and Chan, 2015). PolyPhen2 was used to predict the possible impact of an amino acid substitution on the structure and function of a protein, considering sequence and structure-based features (http://genetics.bwh.harvard.edu/pph2/) (Adzhubei et al., 2013). SNAP2 is a method to predict the functional effect of SNPs by using neural networks trained on a data set of experimental mutation data (https://github.com/Rostlab/SNAP2) (Hecht et al., 2015). PANTHER version 14.0 was used to predict the impact of amino acid substitutions on protein function based on evolutionary relationships among proteins (https://www.pantherdb.org/tools/csnpScoreForm.jsp) (Mi et al., 2019).
2.4 Identification of disease associations of SNPs
The following predictive tools were utilized to forecast if a given SNP was likely associated with disease, especially thrombosis. PhD-SNP was used to predict disease-associated variants according to protein sequences (https://snps.biofold.org/phd-snp/phd-snp.html) (Capriotti and Fariselli, 2023). SNPS&GO, a genome-wide resource for functional-disease associations using region-based coding and non-coding variant-to-gene mappings (https://snps-and-go.biocomp.unibo.it/snps-and-go/) (Calabrese et al., 2009).
2.5 Quantifying the effect of SNPs on protein stability
The following predictive tools were used to assess the effect of SNPs on EPCR protein stability. I-Mutant predicted changes in protein stability caused by SNPs using both the sequence and structure of the protein (https://folding.biofold.org/i-mutant/i-mutant2.0.html) (Capriotti et al., 2005). MUpro, used support vector machines and neural networks to determine the effect of SNPs on protein stability, a source of extra predictive power (https://mupro.proteomics.ics.uci.edu) (Cheng et al., 2006).
2.6 Predicting structural and functional consequences
The MutPred Server was used to predict the potential impact of SNPs on the structure and function of EPCR protein. This system also incorporates several algorithms that predict structural and functional consequences of amino acid substitutions (http://mutpred.mutdb.org/#qform) (Pejaver et al., 2020).
2.7 Analysis of protein sequence conservation using the ConSurf server
Evolutionary conservation of amino acid residues within the EPCR protein was analyzed using ConSurf tool at an online server (http://consurf.tau.ac.il/). This evaluation provided information about which residues are likely functionally important based on conservation scores for homologous sequences (Ashkenazy et al., 2016).
2.8 Proteins: biochemical and structural analysis
Using the HOPE tool, SNPs in EPCR protein were analyzed for their biochemical properties and structural implications. We fed these mutant sequences for the predictive algorithm of HOPE and took insight into the vessel layer they may affect, including the effect on the function and 3D structure of the protein (https://www3.cmbi.umcn.nl/hope/input/) (Venselaar et al., 2010).
2.9 Identifying protein active sites
Structural visualization and mapping of putative active binding sites on EPCR protein were done using PyMol software (Version 2.5.4). This was indicated as an important survey for searching out that SNPs might affect protein binding with other molecules and give a clue for supporting functional disruption (Faure et al., 2019). In addition to PyMOL-based structural visualization, the CASTp server was also used. CASTp predicted a large pocket near residues N136, L168, and T174, which were subsequently prioritized for molecular dynamics simulations.
2.10 Molecular dynamics simulations (MDS)
Molecular dynamics simulations were performed using GROMACS 2021.3 (Lindahl et al., 2023) to investigate structural and dynamic differences between wild-type EPCR and three mutants; L168P, N136I, and T174I. The OPLS-AA force field was applied to parameterize the systems. Each protein was solvated in a cubic water box with a 1.0 nm buffer distance, and charge neutrality was achieved by adding ten sodium (Na+) ions using the genion tool. Energy minimization was conducted via the steepest descent algorithm (50,000 steps) to eliminate steric clashes. Equilibration followed under NVT (constant particle number, volume, temperature) and NPT (constant particle number, pressure, temperature) ensembles for 100 ns, with temperature maintained at 310 K using the Berendsen thermostat and pressure at 1 bar using the Parrinello-Rahman barostat. Production runs were extended to 100 ns for both wild-type and mutant systems, with trajectory data saved at 1 ns intervals to capture structural evolution (Abraham et al., 2015).
Structural metrics were computed using GROMACS modules RMSD, RMSF, Radius of gyration (Rg), Hydrogen bonds, and Solvent-accessible surface area SASA. Trajectories were visualized and plotted using XMGRACE to compare wild-type and mutant systems (Van Der Spoel et al., 2005).
To validate the reliability of our computational workflow, we retrospectively, included the well-characterized S219G (rs867186) mutation in the initial screening. This variant has been previously associated with increased soluble EPCR levels and elevated thrombotic risk in several GWAS and clinical studies. In our analysis, this mutation was predicted as deleterious by MuPro with prediction that it decreases the protein stability, PhD-SNP, and HOPE suggesting a possible effect on the contact with the lipid membrane. Its concordance with known clinical data supports the predictive accuracy of our computational pipeline. Although S219G was not selected for full MD simulation due to its distal location from the reactive surface, its inclusion in the screening phase provided internal validation. This reinforces the rationale for selecting other novel nsSNPs (e.g., N136I, L168P, T174I) that exhibit similar or greater predicted destabilizing potential.
3 Results
We collected data for 217 nsSNPs in the PROCR gene from the NCBI database to predict their effect on protein function.
3.1 Functional predictions and disease associations
Table 1 summarizes the functional predictions for a series of PROCR gene nsSNPs as determined by SIFT, PANTHER, PolyPhen-2, and SNAP2. All analyzed variants, including T174I, F36C, T233I, Y89H, and others, were consistently predicted to affect protein function. SIFT scores were uniformly low (ranging from 0.00 to 0.03), while both PANTHER and PolyPhen-2 predominantly classified these substitutions as possibly or probably damaging. SNAP2 further reinforced these predictions by indicating a detrimental effect. These convergent computational results strongly suggest that the identified nsSNPs compromise the normal function of the PROCR protein.
The disease association analysis performed using SNP&GO and PhD-SNP as shown in (Table 2), indicates that several of these nsSNPs are linked to disease. Variants such as T174I, F36C, I229S, and L165P, among others, are associated with disease at varying reliability indices. This robust prediction of disease involvement supports the clinical relevance of these mutations, potentially implicating them in increased thrombotic risk.
3.2 Impact on protein stability and function
The impact of the nsSNPs on protein stability as predicted by I-Mutant and MuPro shown in (Table 3). With the exception of the N136I variant, which shows a discrepancy between the two tools (I-Mutant predicts decreased stability whereas MuPro suggests a slight increase), the majority of mutations are predicted to decrease protein stability. These findings imply that the structural integrity of the PROCR protein is likely compromised by most of these variants, which may adversely affect its biological function (Table 4). details the predicted functional consequences using MutPred. For instance, the T174I variant (score 0.538) is predicted to cause the loss of N-linked glycosylation at N172, while F36C (0.754) is associated with the loss of sulfation at Y35. Other variants, including R206P, N136I, L168P, and G235R, are predicted to disrupt critical structural features or post-translational modifications, such as the loss of pyrrolidone carboxylic acid at Q203, further loss of N-linked glycosylation, and even the loss of a catalytic site, thereby potentially impairing the protein’s regulatory functions.
3.3 Conservation and structural impacts
Evolutionary conservation analysis presented in (Figure 2; Table 5), using ConSurf, reveals that several mutated residues (T174I, R206P, N136I, and G235R) are highly conserved and either exposed or functional, emphasizing their critical roles in protein activity. In contrast, the L168P substitution is located in a conserved, buried region, suggesting a structural role whose alteration could have significant repercussions on the protein’s overall stability.
The HOPE analysis of the structural impacts of these nsSNPs is shown in Table 6. The R206P mutation results in a decrease in residue size with an increase in hydrophobicity, leading to a loss of charge and potential disruption of key interactions. Similarly, the G235R substitution introduces an increase in size and charge with decreased hydrophobicity, likely causing steric hindrance and structural perturbations. The L168P variant is predicted to disrupt the α-helix due to its reduced size and increased hydrophobicity, while N136I may lead to destabilization of the local structure through the loss of hydrogen bonds. T174I is associated with increases in both size and hydrophobicity, which could disrupt ligand interactions and multimeric contacts. Although several mutations were predicted to have structural and functional impacts, only N136I (MAF = 0.000111/1), L168P (MAF = 0.0000038/1), and T174I (0.0000038/1) were selected to be used for molecular dynamics (MD) simulations because these mutations were located within the protein’s reactive surface area, as identified by structural visualization using PyMol. This surface is directly involved in the protein’s interaction with physiological partners, such as activated protein C. In contrast, R206P and G235R, while highly conserved and functionally important according to ConSurf and HOPE analyses, are positioned in regions distal to the active or ligand-binding interface. Their predicted effects, including steric hindrance or altered charge, are likely to affect structural integrity or folding stability rather than direct functional interaction. Therefore, we prioritized mutations that are both functionally relevant and structurally accessible to ligands for dynamic simulation.
To assess the conformational changes introduced by selected point mutations, Ramachandran plots were generated using STRIDE tool for the wild-type protein and the three mutant variants: N136I, L168P, and T174I. The distribution of backbone dihedral angles (ϕ, ψ) was compared to evaluate deviations in secondary structure preferences. In the wild-type structure, most residues were confined within the allowed regions corresponding to canonical α-helical and β-sheet conformations. Only a few outliers were observed, indicating a well-folded, stable backbone geometry. In the N136I mutant, the overall distribution remained comparable to the wild type; however, an increase in outliers was observed in disallowed regions, particularly near ψ ≈ 60°–90° and ϕ > 90°. This suggests a minor local disturbance in backbone flexibility, potentially due to the bulkier isoleucine side chain replacing asparagine. In the L168P mutant, a more pronounced shift in backbone angles was noted. Several residues appeared outside the favored regions, including in the disallowed upper-right quadrant. This pattern is consistent with the structural rigidity introduced by proline, which restricts ϕ angles and often disrupts α-helical segments. The data suggest that L168P induces local destabilization of secondary structure, likely disrupting helical continuity. The T174I mutant also exhibited an increased number of residues with ϕ/ψ angles in disallowed regions, though to a lesser extent than L168P. The substitution of threonine with the bulkier and more hydrophobic isoleucine may affect local folding but without significant global disruption (Figure 3).
3.4 Identifying protein active sites
To further refine our focus, we used PyMOL to filter out mutations located outside the protein-facing portion of the reactive surface, as these are less likely to affect functionally important regions. This analysis identified three mutations—N136I, L168P, and T174I—located within the reactive surface (Figure 4). These mutations are of particular interest due to their strategic locations, which may significantly impact protein activity and stability. Focusing on these variants enhances our understanding of how specific structural changes contribute to protein dysfunction and disease pathogenesis.
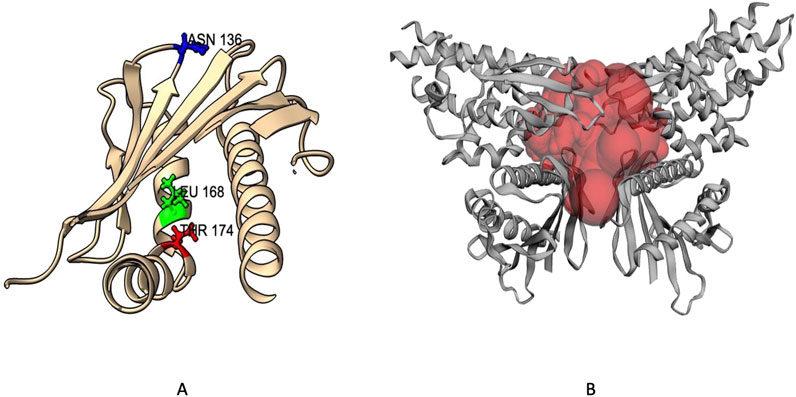
Figure 4. EPCR Protein Mutants within Reactive Surface: (A) Genetic variants highlighted in the protein 3D structure, (B) Multimeric protein structure showing the reactive site.
3.5 Root mean square deviation (RMSD)
We performed 100 ns molecular dynamics simulations to assess the effects of point mutations on protein structure, comparing the wild-type (WT) protein with three single mutants (L168P, N136I, and T174I). Analysis of the Cα RMSD (Figure 5) revealed an initial equilibration phase during the first 5–10 ns, followed by a plateau for all systems. The WT protein exhibited the highest average RMSD (∼1.7–1.8 nm), indicating greater conformational rearrangements. In contrast, L168P stabilized at a lower RMSD (∼1.5 nm), suggesting that the proline substitution confers increased rigidity and a more compact structure. Both N136I and T174I converged at intermediate RMSD values (∼1.6 nm), reflecting subtler structural changes compared to WT (Figure 5).
3.6 Root mean square fluctuation (RMSF)
To better visualize the impact of mutations, RMSF analysis was calculated at the residue level rather than at the atom level. This approach allows clearer interpretation of local flexibility changes, particularly around the mutation sites. For instance, residue-level RMSF plots show increased fluctuations in the vicinity of residues N136, and L168, but not T174 in the mutant structures compared to WT, suggesting local destabilization. Furthermore, the differences in RMSD and SASA trends between the wild-type and mutant systems were re-examined to clarify the dynamic deviation patterns, which further corroborate the destabilizing effect of these substitutions. Correspondingly, the RMSF plots and annotated structural regions are presented in Figure 6.
3.7 Radius of gyration
The radius of gyration (Figure 7) further supported these findings. The WT protein stabilized at an Rg of approximately 2.2 nm, indicating moderate compactness. L168P showed a lower Rg (∼2.1 nm), and N136I converged near 2.0 nm, both suggesting increased compactness relative to WT. T174I’s Rg remained close to that of WT, consistent with minimal perturbations in global dynamics.
3.8 Hydrogen bond
Hydrogen bond analysis (Figure 8) revealed stable intramolecular hydrogen-bond networks post-equilibration, with average counts ranging from 150 to 200. L168P exhibited a slightly higher number of hydrogen bonds, consistent with its compact structure, while N136I showed a moderate increase relative to WT. T174I maintained a hydrogen-bond count similar to WT, suggesting that its overall hydrogen-bonding network remains largely intact despite localized fluctuations.
3.9 Solvent accessible surface area (SASA)
SASA analysis (Figure 9) demonstrated that all systems stabilized after equilibration. WT showed a moderate plateau, while L168P consistently recorded the lowest SASA, reflecting its compact conformation. N136I showed a slightly reduced SASA compared to WT, and T174I’s profile closely mirrored that of the WT. These results suggest that L168P significantly stabilizes the protein by promoting a more compact and rigid structure, N136I induces moderate structural compaction, and T174I exerts a minimal effect on global architecture despite localized increases in flexibility.
4 Discussion
Several studies have reported associations between PROCR gene variants and thrombotic disorders through genome-wide association studies (GWAS) and clinical case analyses. Notably, the Ser219Gly (rs867186) polymorphism has been linked to increased levels of soluble EPCR (sEPCR) and a heightened risk of venous thromboembolism (VTE) and coronary artery disease. GWAS data have also implicated PROCR variants in myocardial infarction, deep vein thrombosis, and pregnancy-associated thrombosis, supporting the notion that genetic alterations in EPCR can modulate thrombotic risk. These findings highlight the importance of investigating the structural and functional consequences of nsSNPs in PROCR, particularly those that may destabilize EPCR or impair its anticoagulant function (Medina et al., 2008; Dennis et al., 2012; Klarin et al., 2019).
Our findings indicate that deleterious nsSNPs in the PROCR gene significantly compromise EPCR’s structural integrity and, by extension, its anticoagulant function. The present study demonstrates that mutations such as T174I, F36C, and L168P induce distinct structural perturbations, ranging from the disruption of glycosylation sites and impairment of α-helix integrity to altered catalytic residues, that collectively may elevate thrombotic risk.
The impact of different SNPs on the secondary structure was assessed by comparing Ramachandran plots of the wild-type and variant protein structures. This approach allowed us to examine shifts in the distribution of backbone dihedral angles and determine whether the mutations affected local flexibility or imposed conformational constraints on the backbone geometry. Structural Ramachandran analysis of the PROCR wild-type and mutants reveals distinct backbone perturbations. The wild-type receptor shows an excellent stereochemical profile, with the vast majority of φ/ψ angles in favored α-helical or β-sheet regions and very few outliers (Farajzadeh-Dehkordi et al., 2023). This indicates a well-ordered native fold. The N136I mutant deviates only slightly from this norm, exhibiting a modest increase in Ramachandran-disallowed residues. Such a minor shift suggests that N136I causes only local flexibility changes without global distortion–consistent with prior observations that conservative missense changes often preserve the backbone conformation. For example, a benign p53 variant (N235S) was found to maintain an intact core and near-native φ/ψ distribution (Tam et al., 2020). In contrast, the L168P mutant shows pronounced Ramachandran outliers, reflecting severe backbone disruption. Proline’s cyclic structure lacks a backbone H-bond donor and restricts φ, making it a known “helix breaker” that often induces kinks (Yuan et al., 1994). Accordingly, L168P places several residues in disallowed quadrants, in line with reports of proline substitutions causing abrupt helical distortions (Yuan et al., 1994). The T174I mutation has an intermediate effect: its bulkier isoleucine side chain likely imposes steric strain, pushing nearby φ/ψ angles slightly outside preferred regions. Similar moderate deviations in Ramachandran distributions have been noted in other coagulation proteins; for instance, a single-point mutant in Factor XII showed a drop in favored-region occupancy from ∼93% to 85%, signaling a measurable conformational perturbation (Pathak et al., 2015). These findings underscore how each PROCR variant uniquely alters structural geometry–from the almost native-like backbone in N136I to the pronounced helix unwinding in L168P–mirroring trends seen in MD studies of other receptors where pathogenic mutations induce clear Ramachandran shifts while milder substitutions do not (Datta et al., 2015).
Molecular dynamics simulations revealed increased RMSD and RMSF values in key functional regions, indicating destabilization of the native protein conformation. Interestingly, L168P exhibited a lower RMSD than the WT, reflecting reduced conformational flexibility due to disruption of the α-helical structure. This rigidity could hinder proper ligand binding and protein-protein interactions essential for protein C activation. The T174I variant, predicted to result in the loss of N-linked glycosylation at N172, may further exacerbate destabilization by eliminating post-translational modifications critical for EPCR folding and expression. These observations are consistent with prior studies showing that subtle alterations in secondary structure and glycosylation can impact protein function and lead to disease phenotypes (Islam et al., 2019; Behairy et al., 2022).
Our study also extends previous findings by providing detailed dynamic insights that go beyond static structural predictions. The integration of multiple computational tools with molecular dynamics simulations allowed us to capture both the global and local effects of nsSNPs on EPCR. The observed decrease in intramolecular hydrogen bonds and the increase in solvent-accessible surface area in the mutant proteins underscore the potential for these variants to weaken the overall stability of EPCR. These dynamic changes suggest a mechanistic link between the molecular alterations induced by these nsSNPs and the increased thrombotic susceptibility observed in patients, a connection that has been proposed but not fully delineated in earlier reports (Roy et al., 2024; Nimir et al., 2017).
Mechanistically, our results suggest that these mutations alter the energetic landscape of EPCR, disrupting its normal equilibrium between active and inactive conformations. One possible explanation is that the loss of glycosylation and the distortion of secondary structures reduce the protein’s ability to interact with its physiological ligands, thereby impairing its role in the protein C pathway. This interpretation is supported by our conservation analysis, which shows that several of the mutated residues are highly conserved and likely critical for the protein’s functional integrity (Klarin et al., 2019). In contrast, the T174I mutation, while affecting glycosylation, appears to induce only modest global structural changes, suggesting that its impact might be more nuanced and could potentially depend on the cellular context or interactions with other molecular partners.
Structural analysis of the mutant models revealed that certain substitutions may introduce unusual kinks or distortions in the EPCR backbone that could compromise receptor function. The L168P mutation introduces a proline residue within an α-helix, which is known to act as a helix breaker due to its rigid ring structure and inability to participate in standard hydrogen bonding (Kunz et al., 2002; Li et al., 1996). This likely causes a local kink, destabilizing the helix and altering the geometry of the ligand-binding region. Similarly, the T174I mutation replaces a polar residue with a bulkier, hydrophobic isoleucine, potentially disturbing the surface topology and protein-ligand interactions. These distortions may impair the presentation of EPCR on the cell surface, alter binding affinity to activated protein C (APC) (Liaw et al., 2001), or promote enhanced receptor shedding, which has been implicated in prothrombotic conditions. Mutations that introduce kinks or destabilize secondary structure elements may also affect receptor trafficking, glycosylation patterns, or interactions with membrane microdomains, ultimately contributing to disease phenotypes such as venous thromboembolism or inflammation-related vascular damage (Ireland et al., 2005).
While our study focuses on the structural and dynamic consequences of deleterious nsSNPs in EPCR, it is important to acknowledge that differences in protein expression levels between the wild-type and variants may also significantly influence biological function. Some mutations may not primarily affect protein structure or stability but instead impact transcriptional regulation, mRNA stability, or post-translational trafficking, leading to altered cell-surface expression of EPCR. These expression differences can act as confounding factors when interpreting the functional consequences of mutations solely from a structural perspective (Gandrille, 2008). For example, even structurally stable variants could be biologically inactive if their expression is reduced or mislocalized. Therefore, our structural predictions should be interpreted in the context of these limitations and considered as complementary to, but not a substitute for, experimental validation at the transcriptional and translational levels. Future in vitro studies assessing expression profiles, receptor localization, and APC-binding capacity are necessary to fully understand the phenotypic impact of the predicted mutations.
It is important to note that the majority of the nsSNPs analyzed in this study are rare variants with low allele frequencies in the general population, as reported in dbSNP database. In many cases, these variants are present at a frequency of less than 0.01% and are very rarely observed in homozygous individuals. This low prevalence may limit their direct impact at the population level, and in some cases, their phenotypic consequences may only become apparent in compound heterozygous states, under specific environmental conditions, or in individuals with additional risk factors. Nonetheless, even rare variants can have high penetrance and functional significance in specific individuals or subpopulations, especially if they occur in functionally critical regions such as the ligand binding interface of EPCR. Our findings provide a framework for prioritizing such variants for further functional assays and genotype-phenotype correlation studies in thrombotic disease cohorts.
Despite the valuable insights gained, our study has limitations. Computational predictions and MD simulations offer a robust platform for prioritizing variants, but they cannot fully replicate the complexity of cellular environments. Factors such as chaperone-mediated folding, cellular localization, and in vivo post-translational modifications are not entirely captured. Moreover, the 100 ns simulation timeframe provides only short-term insights; extended simulations and experimental validation are needed to assess long-term effects.
Future investigations should focus on experimental validation of these computational predictions. In vitro expression studies, protein stability assays, and functional tests of EPCR activity in cell models would be instrumental in corroborating our findings. Moreover, expanding the simulation timescale and exploring the effects of additional interacting partners could further elucidate the clinical significance of these nsSNPs. Ultimately, these efforts may pave the way for the development of personalized therapeutic interventions targeting thrombotic disorders, as our data provide a mechanistic framework linking genetic variation in PROCR to altered protein function and disease risk. In conclusion, our data provide compelling evidence that deleterious nsSNPs in the PROCR gene destabilize EPCR, thereby impairing its anticoagulant function and increasing thrombotic susceptibility. These insights not only enhance our understanding of the molecular mechanisms underlying thrombosis but also underscore the potential of integrated in silico approaches to inform risk assessment and personalized treatment strategies in vascular disorders (Behairy et al., 2022; Roy et al., 2024; Nimir et al., 2017).
5 Conclusion
Our integrative computational analysis and molecular dynamics simulations provide strong evidence that deleterious nsSNPs in the PROCR gene—particularly T174I, N136I, and L168P—significantly compromise EPCR’s structural integrity and stability. These mutations disrupt key structural elements, including glycosylation sites, α-helices, and catalytic residues, leading to altered protein dynamics, reduced hydrogen bonding, and increased solvent exposure. Collectively, these perturbations likely impair EPCR’s anticoagulant function, increasing thrombotic risk. While our findings highlight the value of in silico approaches for prioritizing pathogenic variants and elucidating disease mechanisms, experimental validation remains essential. These insights may ultimately support improved risk assessment and the development of personalized therapies for thrombotic disorders.
Data availability statement
The original contributions presented in the study are included in the article/Supplementary Material, further inquiries can be directed to the corresponding author.
Author contributions
HA: Conceptualization, Project administration, Writing – original draft, Writing – review and editing. KA: Data curation, Formal Analysis, Writing – original draft, Writing – review and editing. ME: Data curation, Formal Analysis, Writing – original draft, Writing – review and editing. EMA: Investigation, Methodology, Writing – original draft, Writing – review and editing. EWA: Investigation, Methodology, Writing – original draft, Writing – review and editing. AE: Resources, Validation, Writing – original draft, Writing – review and editing. AM: Resources, Validation, Writing – original draft, Writing – review and editing. EE: Resources, Validation, Writing – original draft, Writing – review and editing. TA: Methodology, Visualization, Writing – original draft, Writing – review and editing. BE: Methodology, Visualization, Writing – original draft, Writing – review and editing. HO: Methodology, Visualization, Writing – original draft, Writing – review and editing. EI: Methodology, Visualization, Writing – original draft, Writing – review and editing.
Funding
The author(s) declare that no financial support was received for the research and/or publication of this article.
Conflict of interest
The authors declare that the research was conducted in the absence of any commercial or financial relationships that could be construed as a potential conflict of interest.
Acknowledgments
The authors are thankful to the Deanship of Graduate Studies and Scientific Research at University of Bisha for supporting this work through the Fast-Track Research Support Program.
Generative AI statement
The author(s) declare that no Generative AI was used in the creation of this manuscript.
Publisher’s note
All claims expressed in this article are solely those of the authors and do not necessarily represent those of their affiliated organizations, or those of the publisher, the editors and the reviewers. Any product that may be evaluated in this article, or claim that may be made by its manufacturer, is not guaranteed or endorsed by the publisher.
Supplementary material
The Supplementary Material for this article can be found online at: https://www.frontiersin.org/articles/10.3389/fgene.2025.1580993/full#supplementary-material
References
Abraham, M., Murtola, T., Schulz, R., Páll, S., Smith, J., Hess, B., et al. (2015). GROMACS: high performance molecular simulations through multi-level parallelism from laptops to supercomputers. SoftwareX 1-2, 19–25. doi:10.1016/j.softx.2015.06.001
Adzhubei, I., Jordan, D. M., and Sunyaev, S. R. (2013). Predicting functional effect of human missense mutations using PolyPhen-2. Curr. Protoc. Hum. Genet. 7 (7), Unit7.20–20. doi:10.1002/0471142905.hg0720s76
Ashkenazy, H., Abadi, S., Martz, E., Chay, O., Mayrose, I., Pupko, T., et al. (2016). ConSurf 2016: an improved methodology to estimate and visualize evolutionary conservation in macromolecules. Nucleic Acids Res. 44, 344–350. doi:10.1093/nar/gkw408
Behairy, M. Y., Soltan, M. A., Adam, M. S., Refaat, A. M., Ezz, E. M., Albogami, S., et al. (2022). Computational analysis of deleterious SNPs in NRAS to assess their potential correlation with carcinogenesis. Front. Genet. 13, 872845. doi:10.3389/fgene.2022.872845
Calabrese, R., Capriotti, E., Fariselli, P., Martelli, P. L., and Casadio, R. (2009). Functional annotations improve the predictive score of human disease-related mutations in proteins. Hum. Mutat. 30, 1237–1244. doi:10.1002/humu.21047
Capriotti, E., and Fariselli, P. (2023). PhD-SNPg: updating a webserver and lightweight tool for scoring nucleotide variants. Nucleic Acids Res. 51, 451–458. doi:10.1093/nar/gkx369
Capriotti, E., Fariselli, P., and Casadio, R. (2005). I-Mutant2.0: predicting stability changes upon mutation from the protein sequence or structure. Nucleic Acids Res. 33, 306–310. doi:10.1093/nar/gki375
Cheng, J., Randall, A., and Baldi, P. (2006). Prediction of protein stability changes for single-site mutations using support vector machines. Proteins 62, 1125–1132. doi:10.1002/prot.20810
Choi, Y., and Chan, A. P. (2015). PROVEAN web server: a tool to predict the functional effect of amino acid substitutions and indels. Bioinformatics 31, 2745–2747. doi:10.1093/bioinformatics/btv195
Dahlbäck, B., and Villoutreix, B. O. (2005). Regulation of blood coagulation by the protein C anticoagulant pathway: novel insights into structure-function relationships and molecular recognition. Arterioscler. Thromb. Vasc. Biol. 25, 1311–1320. doi:10.1161/01.ATV.0000168421.13467.82
Datta, A., Mazumder, M. H., Chowdhury, A. S., and Hasan, M. A. (2015). Functional and structural consequences of damaging single nucleotide polymorphisms in human prostate cancer predisposition gene RNASEL. Biomed. Res. Int. 271458. doi:10.1155/2015/271458
Dennis, J., Johnson, C. Y., Adediran, A. S., De Andrade, M., Heit, J. A., Morange, P. E., et al. (2012). The endothelial protein C receptor (PROCR) Ser219Gly variant and risk of common thrombotic disorders: a HuGE review and meta-analysis of evidence from observational studies. Blood, J. Am. Soc. Hematol. 119 (10), 2392–2400. doi:10.1182/blood-2011-10-383448
Esmon, C. T. (2003). The protein C pathway. Chest 124, 26S-32S–32S. doi:10.1378/chest.124.3_suppl.26s
Farajzadeh-Dehkordi, M., Mafakher, L., Harifi, A., Samiee-Rad, F., and Rahmani, B. (2023). Computational analysis of the functional and structural impact of the most deleterious missense mutations in the human Protein C. Plos one 18 (11), e0294417. doi:10.1371/journal.pone.0294417
Faure, G., Joseph, A., Craveur, P., Narwani, T., Srinivasan, N., Gelly, J., et al. (2019). iPBAvizu: a PyMOL plugin for an efficient 3D protein structure superimposition approach. Source Code Biol. Med. 14, 5. doi:10.1186/s13029-019-0075-3
Feistritzer, C., and Riewald, M. (2005). Endothelial barrier protection by activated protein C through PAR1-dependent sphingosine 1-phosphate receptor-1 crossactivation. Blood 105, 3178–3184. doi:10.1182/blood-2004-10-3985
Fukudome, K., and Esmon, C. T. (1994). Identification, cloning, and regulation of a novel endothelial cell protein C/activated protein C receptor. J. Biol. Chem. 269, 26486–26491. doi:10.1016/s0021-9258(18)47220-1
Gandrille, S. (2008). Endothelial cell protein C receptor and the risk of venous thrombosis. Haematologica 93 (6), 812–816. doi:10.3324/haematol.13243
Hecht, M., Bromberg, Y., and Rost, B. (2015). Better prediction of functional effects for sequence variants. BMC Genomics 16, S1. doi:10.1186/1471-2164-16-S8-S1
Hollingsworth, S. A., and Dror, R. O. (2018). Molecular dynamics simulation for all. Neuron 99, 1129–1143. doi:10.1016/j.neuron.2018.08.011
Ireland, H., Konstantoulas, C. J., Cooper, J. A., Hawe, E., Humphries, S. E., Mather, H., et al. (2005). EPCR Ser219Gly: elevated sEPCR, prothrombin F1+ 2, risk for coronary heart disease, and increased sEPCR shedding in vitro. Atherosclerosis 183 (2), 283–292. doi:10.1016/j.atherosclerosis.2005.02.028
Islam, M. J., Khan, A. M., Parves, M. R., Hossain, M. N., and Halim, M. A. (2019). Prediction of deleterious non-synonymous SNPs of human STK11 gene by combining algorithms, molecular docking, and molecular dynamics simulation. Sci. Rep. 9, 16426. doi:10.1038/s41598-019-52308-0
Kallel, C., Cohen, W., Saut, N., Blankenberg, S., Schnabel, R., Rupprecht, H. J., et al. (2012). Association of soluble endothelial protein C receptor plasma levels and PROCR rs867186 with cardiovascular risk factors and cardiovascular events in coronary artery disease patients: the Athero Gene study. BMC Med. Genet. 13, 103–108. doi:10.1186/1471-2350-13-103
Karplus, M., and McCammon, J. A. (2002). Molecular dynamics simulations of biomolecules. Nat. Struct. Biol. 9, 646–652. doi:10.1038/nsb0902-646
Klarin, D., Busenkell, E., Judy, R., Lynch, J., Levin, M., Haessler, J., et al. (2019). Genome-wide association analysis of venous thromboembolism identifies new risk loci and genetic overlap with arterial vascular disease. Nat. Genet. 51 (11), 1574–1579. doi:10.1038/s41588-019-0519-3
Koupenova, M., Kehrel, B. E., Corkrey, H. A., and Freedman, J. E. (2017). Thrombosis and platelets: an update. Eur. Heart J. 38, 785–791. doi:10.1093/eurheartj/ehw550
Kunz, G., Ohlin, A. K., Adami, A., Zöller, B., Svensson, P., and Lane, D. A. (2002). Naturally occurring mutations in the thrombomodulin gene leading to impaired expression and function. Blood, J. Am. Soc. Hematol. 99 (10), 3646–3653. doi:10.1182/blood.v99.10.3646
Lemkul, J. A. (2018). From proteins to perturbed Hamiltonians: a suite of tutorials for the GROMACS-2018 molecular simulation package [article v1.0]. Living J. Comput. Mol. Sci. 1, 5068. doi:10.33011/livecoms.1.1.5068
Li, S. C., Goto, N. K., Williams, K. A., and Deber, C. M. (1996). Alpha-helical, but not beta-sheet, propensity of proline is determined by peptide environment. Proc. Natl. Acad. Sci. 93 (13), 6676–6681. doi:10.1073/pnas.93.13.6676
Liaw, C. P., Mather, T., Oganesyan, N., Ferrell, G. L., and Esmon, C. T. (2001). Identification of the protein C/activated protein C binding sites on the endothelial cell protein C receptor: implications for a novel mode of ligand recognition by a major histocompatibility complex class 1-type receptor. J. Biol. Chem. 276 (11), 8364–8370. doi:10.1074/jbc.M010572200
Lindahl, E., Abraham, M. J., Hess, B., and van der Spoel, D. (2023). GROMACS 2021.7 Manual. Geneva, Switzerland: Zenodo. doi:10.5281/zenodo.7586709
Mackman, N. (2012). New insights into the mechanisms of venous thrombosis. J. Clin. Invest 122, 2331–2336. doi:10.1172/JCI60229
Medina, P., Navarro, S., Corral, J., Zorio, E., Roldán, V., Estellés, A., et al. (2008). Endothelial protein C receptor polymorphisms and risk of myocardial infarction. haematologica 93 (9), 1358–1363. doi:10.3324/haematol.13066
Medina, P., Navarro, S., Estellés, A., Vayá, A., Bertina, R. M., and España, F. (2005). Influence of the 4600A/G and 4678G/C polymorphisms in the endothelial protein C receptor (EPCR) gene on the risk of venous thromboembolism in carriers of factor V Leiden. Thromb. Haemost. 94, 389–394. doi:10.1160/TH05-02-0089
Mi, H., Muruganujan, A., Ebert, D., Huang, X., and Thomas, P. D. (2019). PANTHER version 14: more genomes, a new PANTHER GO-slim and improvements in enrichment analysis tools. Nucleic Acids Res. 47, D419-D426–26. doi:10.1093/nar/gky1038
Nimir, M., Abdelrahim, M., Abdalla, M., Ahmed, W. E., Abdullah, M., Hamid, M. M. A., et al. (2017). In silico analysis of single nucleotide polymorphisms (SNPs) in human FOXC2 gene. F1000Res 6, 243. doi:10.12688/f1000research.10937.2
Pathak, M., Wilmann, P., Awford, J., Li, C., Hamad, B. K., Fischer, P. M., et al. (2015). Coagulation factor XII protease domain crystal structure. J. Thrombosis Haemostasis 13 (4), 580–591. doi:10.1111/jth.12849
Pejaver, V., Urresti, J., Lugo-Martinez, J., Pagel, K. A., Lin, G. N., Nam, H. J., et al. (2020). Inferring the molecular and phenotypic impact of amino acid variants with MutPred2. Nat. Commun. 11, 5918. doi:10.1038/s41467-020-19669-x
Qu, D., Wang, Y., Esmon, N. L., and Esmon, C. T. (2007). Regulated endothelial protein C receptor shedding is mediated by tumor necrosis factor-alpha converting enzyme/ADAM17. J. Thromb. Haemost. 5, 395–402. doi:10.1111/j.1538-7836.2007.02347.x
Reiner, A. P., Carty, C. L., Jenny, N. S., Nievergelt, C., Cushman, M., Stearns-Kurosawa, D. J., et al. (2008). PROC, PROCR andPROS1 polymorphisms, plasma anticoagulant phenotypes, and risk of cardiovascular disease and mortality in older adults: the Cardiovascular Health Study. J. Thrombosis Haemostasis 6 (10), 1625–1632. doi:10.1111/j.1538-7836.2008.03118.x
Roy, A., Feroz, T., Islam, M., Munim, M., Supti, D., Antora, N., et al. (2024). A computational approach for structural and functional analyses of disease-associated mutations in the human CYLD gene. Genom Inf. 22, 4. doi:10.1186/s44342-024-00007-2
Saposnik, B., Reny, J. L., Gaussem, P., Emmerich, J., Aiach, M., and Gandrille, S. (2004). A haplotype of the EPCR gene is associated with increased plasma levels of sEPCR and is a candidate risk factor for thrombosis. Blood 103, 1311–1318. doi:10.1182/blood-2003-07-2520
Shaw, D. E., Maragakis, P., Lindorff-Larsen, K., Piana, S., Dror, R. O., Eastwood, M. P., et al. (2010). Atomic-level characterization of the structural dynamics of proteins. Science 330, 341–346. doi:10.1126/science.1187409
Tam, B., Sinha, S., and Wang, S. M. (2020). Combining Ramachandran plot and molecular dynamics simulation for structural-based variant classification: using TP53 variants as model. Comput. Struct. Biotechnol. J. 18, 4033–4039. doi:10.1016/j.csbj.2020.11.041
Taylor, F. B. Jr, Peer, G. T., Lockhart, M. S., Ferrell, G., and Esmon, C. T. (2001). Endothelial cell protein C receptor plays an important role in protein C activation in vivo. Blood 97, 1685–1688. doi:10.1182/blood.v97.6.1685
Van Der Spoel, D., Lindahl, E., Hess, B., Groenhof, G., Mark, A. E., and Berendsen, H. J. (2005). GROMACS: fast, flexible, and free. J. Comput. Chem. 26, 1701–1718. doi:10.1002/jcc.20291
Van de Wouwer, M., Collen, D., and Conway, E. M. (2004). Thrombomodulin-protein C-EPCR system: integrated to regulate coagulation and inflammation. Arterioscler. Thromb. Vasc. Biol. 24, 1374–1383. doi:10.1161/01.ATV.0000134298.25489.92
Venselaar, H., te Beek, T., Kuipers, R., Hekkelman, M., and Vriend, G. (2010). Protein structure analysis of mutations causing inheritable diseases. An e-Science approach with life scientist friendly interfaces. BMC Bioinforma. 11, 548. doi:10.1186/1471-2105-11-548
Keywords: thrombosis, PROCR, nsSNPs, EPCR, protein stability, molecular dynamics
Citation: Abuagla HA, Adam KM, Elangeeb ME, Ahmed EM, Ali EW, Edris AM, MohamedAhmed AAE, Eltieb EI, Khalid TBA, Elamin BK, Osman HMA and Idris ES (2025) Impact of nonsynonymous single nucleotide polymorphisms in PROCR gene on protein stability and thrombotic risk: a molecular dynamic approach. Front. Genet. 16:1580993. doi: 10.3389/fgene.2025.1580993
Received: 21 February 2025; Accepted: 16 April 2025;
Published: 30 April 2025.
Edited by:
Federica Chiappori, National Research Council (CNR), ItalyReviewed by:
Silvia B Ramos, University of North Carolina at Chapel Hill, United StatesStalin Antony, University of Electronic Science and Technology of China, China
Davide Giana, University of Milan, Italy
Copyright © 2025 Abuagla, Adam, Elangeeb, Ahmed, Ali, Edris, MohamedAhmed, Eltieb, Khalid, Elamin, Osman and Idris. This is an open-access article distributed under the terms of the Creative Commons Attribution License (CC BY). The use, distribution or reproduction in other forums is permitted, provided the original author(s) and the copyright owner(s) are credited and that the original publication in this journal is cited, in accordance with accepted academic practice. No use, distribution or reproduction is permitted which does not comply with these terms.
*Correspondence: Hytham Ahmed Abuagla, YWFkbGFoQHViLmVkdS5zYQ==