- 1Department of Communications and Networking, Aalto University, Espoo, Finland
- 2Telefónica Research, Madrid, Spain
1. Introduction
We introduce a large-scale dataset of mouse cursor movements that can be used to predict user attention, infer demographics information, and analyze fine-grained movements. Attention is a finite resource, so people spend their time on things they find valuable, especially when browsing online. Objective measurements of attentional processes are increasingly sought after by researchers, advertisers, and other key stakeholders from both academia and industry. With every click, digital footprints are created and logged, providing a detailed record of a person's online activity. However, click data provide an incomplete picture of user interaction, as they inform mainly about a users' end choice. A user click is often preceded by several valuable interactions, such as scrolling, hovers, aimed movements, etc. and thus having access to this kind of data can lead to an overall better understanding of the user's cognitive processes. For example, previous work has evidenced that when the mouse cursor is motionless, the user is processing information (Hauger et al., 2011; Huang et al., 2011; Diriye et al., 2012; Boi et al., 2016), i.e., essentially “users first focus and then execute actions” (Martín-Albo et al., 2016). We have collected mouse cursor tracking logs from near 3K subjects performing a transactional search task that together account for roughly 2 h worth of interaction data. Our dataset has associated attention labels and five demographics attributes that may help researchers to conduct several analysis, like the ones we discuss later in this section.
Research in mouse cursor tracking has a long track record. Chen et al. (2001) were among the first ones to note a relationship between gaze position and cursor position during web browsing. Mueller and Lockerd (2001) investigated the use of mouse tracking to create compelling visualizations and model the users' interests. It has been argued that mouse movements can reveal subtle patterns like reading (Hauger et al., 2011) or hesitation (Martín-Albo et al., 2016), and can help the user regain context after an interruption (Leiva, 2011a). Others have also noted the utility of mouse cursor analysis as a low-cost and scalable proxy of eye tracking (Huang et al., 2012; Navalpakkam et al., 2013). Several works have investigated closely the utility of mouse cursor data in web search (Arapakis et al., 2015; Lagun and Agichtein, 2015; Liu et al., 2015; Arapakis and Leiva, 2016; Chen et al., 2017) and web page usability evaluation (Arroyo et al., 2006; Atterer et al., 2006; Leiva, 2011b), two of the most prominent use cases of this technology. Mouse biometrics is another active research area that has shown promise in controlled settings (Lu et al., 2017; Krátky and Chudá, 2018). Researchers have started to analyze mouse movements on websites for the detection of neurodegenerative disorders (White et al., 2018; Gajos et al., 2020). In practice, commercial web search engines often use mouse cursor tracking to improve search results (Huang et al., 2011, 2012), optimize page design (Leiva, 2012; Diaz et al., 2013), and offer better recommendations to their users (Speicher et al., 2013). In what follows, we provide a brief survey of what others have accomplished by analyzing mouse cursor movements in web search tasks. These analyses highlight potential use cases of our dataset, thereby allowing researchers to investigate similar environments and behaviors.
1.1. Inferring Interest
For a long time, commercial search engines have been interested in how users interact with Search Engine Result Pages (SERPs), to anticipate better placement and allocation of ads in sponsored search or to optimize the content layout. Early work considered simple, coarse-grained features derived from mouse cursor data to be surrogate measurements of user interest (Goecks and Shavlik, 2000; Claypool et al., 2001; Shapira et al., 2006). Follow-up research transitioned to more fine-grained mouse cursor features (Guo and Agichtein, 2008, 2010) that were shown to be more effective. These approaches have been directed at predicting open-ended tasks like search success (Guo et al., 2012) or search satisfaction (Liu et al., 2015). Mouse cursor position is mostly aligned to eye gaze, especially on SERPs (Guo and Agichtein, 2012; Lagun et al., 2014a), and that can be used as a good proxy for predicting good and bad abandonment (Diriye et al., 2012; Brückner et al., 2020).
1.2. Inferring Visual Attention
Mouse cursor tracking has been used to survey the visual focus of the user, thus revealing valuable information regarding the distribution of user attention over the various SERP components. Despite the technical challenges that may arise from this analysis, previous work has shown the utility of mouse movement patterns to measure within-content engagement (Arapakis et al., 2014a; Carlton et al., 2019) and predict reading experiences (Hauger et al., 2011; Arapakis et al., 2014b). Lagun et al. (2014a) introduced the concept of motifs, or frequent cursor subsequences, in the estimation of search result relevance. Similarly, Liu et al. (2015) applied the motifs concept to SERPs and predicted search result utility, searcher effort, and satisfaction at the search task level. Boi et al. (2016) proposed a method for predicting whether the user is actually looking at the content pointed by the cursor, exploiting the mouse cursor data and a segmentation of the web page contents. Lastly, Arapakis and Leiva (2016) investigated user engagement with direct displays on SERPs and provided further evidence that supports the utility of mouse cursor data for measuring user attention at a display-level granularity (Arapakis and Leiva, 2020; Arapakis et al., 2020).
1.3. Inferring Emotion
The connection between mouse cursor movements and the underlying psychological states has been a topic of research since the early 90s (Card et al., 1987; Accot and Zhai, 1997). Some studies have investigated the utility of mouse cursor data for predicting the user's emotional state. For example, Zimmermann et al. (2003) investigated the effect of induced affective states on the motor-behavior of online shoppers and found that the total duration of mouse cursor movements and the number of velocity changes were associated to the experienced arousal. Kaklauskas et al. (2009) created a system that extracts physiological and motor-control parameters from mouse cursor interactions and then triangulated those with psychological data taken from self-reports, to correlate the users' emotional state and productivity. In a similar line, Azcarraga and Suarez (2012) combined electroencephalography signals and mouse cursor interactions to predict self-reported emotions like frustration, interest, confidence and excitement. Yamauchi (2013) studied the relationship between mouse cursor trajectories and generalized anxiety in human subjects. Lastly, Kapoor et al. (2007) predicted whether a user experiences frustration, using an array of affective-aware sensors.
1.4. Inferring Demographics
Prior work has linked age with motor control and pointing performance in tasks that involve the use of a computer mouse (Walker et al., 1997; Bohan and Chaparro, 1998; Hsu et al., 1999; Smith et al., 1999; Jastrzembski et al., 2003; Lindberg et al., 2006). Overall, aging is marked by a decline in motor control abilities, therefore it is expected to affect the users' pointing performance and, by extension, how they move the computer mouse. For example, Smith et al. (1999) observed that older people incurred in longer mouse movement times, more sub-movements, and more pointing errors than the young. These findings underline potential age effects on the way a mouse device is used in an online search task. Prior research has also noted sensory-motor differences due to gender (Landauer, 1981; Chen and Chen, 2008; Yamauchi et al., 2015), such as significant variation in the cursor movement distance, pointing time, and cursor patterns. The cause of these variations has been attributed to gender-based differences in how users move a mouse cursor or to different cognitive mechanisms (perceptual and spatial processes) involved in motor control.
Others have also examined the extent to which mouse cursor movements can help identify gender and age (Yamauchi and Bowman, 2014; Kratky and Chuda, 2016; Pentel, 2017), however the experimental settings have limited generalizability, either because the tasks are not well-connected to typical activities that users perform online, such as web search, because the data include multiple samples per participant, thereby increasing the risks of information leakage, or because researchers could not verify their ground-truth data. In our dataset, we limit the training samples to exactly one mouse cursor trajectory per participant, who are verified, high-quality crowdworkers.
2. Method
We ran an online crowdsourcing study that reproduced the conditions of a transactional search task. Participants were presented with a simulated information need that explained that they were interested in purchasing some product for them or a friend. Overall, the study consisted of three parts, to be described later: (1) pre-task guidelines, (2) the web search task, and (3) a post-task questionnaire.
2.1. Participants
We recruited participants from the FIGURE EIGHT crowdsourcing platform1. They were of mixed nationality (e.g., American, Belgian, British, German) and had diverse educational backgrounds (see Table 1). All participants were proficient in English and were experienced (Level 3) contributors, i.e., they had a proven track record of successfully completed tasks and of a different variety, thus being considered very reliable contributors.
2.2. Materials
Starting from Google Trends2, we selected a subset of the Top Categories and Shopping Categories that were suitable representatives of transactional tasks. Then, we extracted the top search queries issued in the US during the last 12 months. Next, we narrowed down our search query collection to 150 representative popular queries. The final collection of transactional queries was repeated as many times needed to produce the desired number of search sessions for the final dataset.
Using this final selection of search queries, we produced the static version of the corresponding Google SERPs and injected custom JavaScript code that allowed us to capture all client-side user interactions. For this, we used EVTRACK3, an open source JavaScript event tracking library derived from the smt2ϵ mouse tracking system (Leiva and Vivó, 2013). EVTRACK can capture browser events either via event listeners (the event is captured as soon as it is fired) or via event polling (the event is captured at fixed-time intervals). We captured mousemove events via event polling, every 150 ms to avoid unnecessary data overhead (Leiva and Huang, 2015), and all the other browser events (e.g., load, click, scroll) via event listeners. Whenever an event was recorded, we logged the following information: mouse cursor position (x and y coordinates), timestamp, event name, XPath of the DOM element that relates to the event, and the DOM element attributes (if any).
All queries triggered some form of advertisements on the SERPs, according to three different formats: “native” (organic ads) or “bundled” (direct display ads). All SERPs included one or more native ads together with one bundled ad. The native advertisements could appear either at the top or bottom position of the SERP, whereas the bundled ads could appear either at the top-left or top-right position. We ensured that only one ad was visible per condition and participant at a time. This was possible by instrumenting each downloaded SERP with custom JavaScript code that removed all ads excepting one that would be selected for a given participant. In any case, native bottom-most ads were not shown to the participants.
2.3. Pre-task Guidelines
Participants were instructed to read carefully the terms and conditions of the study which, among other things, informed them that they should perform the task from a desktop or laptop computer using a computer mouse (and refrain from using a touchpad, tablet, or mobile device) and that their browsing activity would be logged. Moreover, participants consented to share their browsing data and their (anonymized) responses for later analysis.
Participants were asked to act naturally and choose anything that would best answer a given search query, since all “clickable” elements (e.g., result links, images, etc.) on the SERP were considered valid answers. The instructions were followed by a brief search task description using this template: “You want to buy <noun> (for you or someone else as a gift) and you have submitted the search query <noun> to Google Search. Please browse the search results page and click on the element that you would normally select under this scenario.” The template was populated with the corresponding <noun> entities, based on the assigned query.
Participants were allowed as much time as they needed to examine the SERP and proceed with the search task, which would conclude whenever they clicked on any SERP element. The payment for the participation was $0.20. Participants could also opt out at any moment, in which case they were not compensated. Each participant could take the study only once.
2.4. Task Procedure
Each participant was presented with a search task description, then provided with a predefined search query (selected at random from our pool of queries) and the corresponding SERP, and they were asked to click on any element of the page that best solved the task. This way, we ensured that participants interacted with the same pool of web search queries and avoided any unaccounted systematic bias due to query quality variation. All possible combinations of query and ad style (i.e., format and position) were pre-computed so that whenever a new user accessed the study, they were assigned one of these combinations at random.
Participants accessed the instrumented SERPs through a dedicated web server that did not alter the look and feel of the original SERPs. This allowed us to capture fine-grained user interactions while ensuring that the content of the SERPs remained consistent with the original version. Each participant was allowed to perform the search task only once to avoid introducing possible carry over effects and, thus, altering their browsing behavior in subsequent search tasks. In sum, each participant was exposed only to a single condition; i.e., a unique combination of query and ad style. Finally, at the end of the study participants had to copy a unique code and paste it on FIGURE EIGHT in order to have their job validated.
2.5. Post-task Questionnaire
Upon concluding the search task, participants were asked to answer a series of questions. The questions were forced-choice type and allowed multi-point response options.
The first question asked the degree to which the user noticed the advertisements shown on the SERP: While performing the search task, to what extent did you pay attention to the advertisement? We used a 5-point Likert-type scale to collect the labels: 1 (“Not at all”), 2 (“Not much”), 3 (“I can't decide”), 4 (“Somewhat”), and 5 (“Very much”). In practice, these scores should be collapsed to binary labels (true/false), but we felt it was necessary to use a 5-point Likert-type scale for several reasons. First, using 2-point scales often results in highly skewed data (Johnson et al., 1982). Second, it is important to leave room for neutral responses, because some users may not want to say one way or another, otherwise this can produce response biases. But 3-point scales can lead more users to stay neutral, because the remaining options can be seen as “too extreme.” Therefore, we opted for a 5-point scale, which leaves more room for “soft responses” and in addition is easy to understand. With this scoring scheme, therefore, we are confident that eventual binary labels would actually reflect positive and negative user votes.
The questionnaire also comprised the following demographics-related questions:
1. What is your gender? [Male, Female, Prefer not to say]
2. What is your age group? [18–23, 24–29,…, 60–65, +66, Prefer not to say]
3. What is your native language? [Pull-down list, Prefer not to say]
4. What is your education level? [High school, College,…, Doctorate, Prefer not to say]
5. What is your current income? [25K, 35K,…, 250K, Prefer not to say]
3. Validation and Filtering
Crowdsourcing studies offer several advantages over in-situ methods of experimentation (Mason and Suri, 2012), such as access at a larger and more diverse pool of participants with stable availability, collection of real usage data at a relatively large scale, and a low-cost alternative to the more expensive laboratory-based experiments. On the downside, experimenters have to account for potential threats to ecological validity, distractions in the physical environment of the participant, and privacy issues, to name a few. Still, crowdsourcing allows for exploring a wider range of parameters in a more controlled manner as compared to in-the-wild large-scale studies.
We collected self-reported ground-truth labels in a similar vein to previous work (Feild et al., 2010; Lagun et al., 2014b; Liu et al., 2015; Arapakis and Leiva, 2016) which also administered post-task questionnaires. To mitigate and discount low-quality responses, several preventive measures were put into practice, such as introducing test (gold-standard) questions to our tasks, selecting experienced contributors with high accuracy rates, and monitoring their task completion time, thus ensuring the internal validity of our experiment.
Starting from a set of 3,223 participants who initially accessed the study, we filtered automatically those who did not finish it (138 cases) as well as participants who did not move their mouse at all (176 cases). We concluded to a dataset with 2,909 observations comprising at least one mouse movement, together with their associated browser's and user's metadata. See Table 1 for a summary of the available demographics information.
There are 92 unique combinations of query and ad style, each of which assessed by 32 users on average (SD = 17 users). There are 1,942 observations from the attended condition (self-reported Likert-type score ≥4), 776 observations from the non-attended condition (score ≤ 2), and 191 observations from the neutral condition (score of 3). The average mouse cursor trajectory has 15.78 coordinates (SD = 16.5, min = 1, max = 222), which is around the same order of magnitude as reported in similar studies (Huang et al., 2011; Leiva and Huang, 2015; Arapakis and Leiva, 2016).
Excepting the automatic filtering procedure explained above, our data is in raw form and therefore some columns require further processing. For example, most columns pertaining demographics information are stored as integers, therefore researchers should consult Table 1 to retrieve the corresponding categorical labels. We also recommend researchers to apply other filtering methods, depending on the nature of their experiments, such as collapsing the ground-truth attention labels from the original 1–5 scale to a binary scale (Arapakis and Leiva, 2020; Arapakis et al., 2020) or ignoring cursor trajectories having <5 coordinates, which in most cases would correspond to 1 s of interaction data.
3.1. Data Format
The Attentive Cursor dataset includes the following resources:
1. A folder with mouse tracking log files, as recorded by the EVTRACK software:
a. Browser events: space-delimited files (CSV) with information about each event type (8 columns).
b. Browser metadata: XML files with information about the user's browser (e.g., viewport size).
2. A TSV file with ground-truth labels (4 columns).
3. A tab-delimited file (TSV) with user's demographics and stimulus condition (12 columns).
4. A folder with all SERPs in HTML format.
5. A README file with a detailed explanation of each resource.
Figure 1 provides some examples of the kind of data that researchers can find in our dataset. We provide the URL to the repository in the “Data Availability Statement” section below.
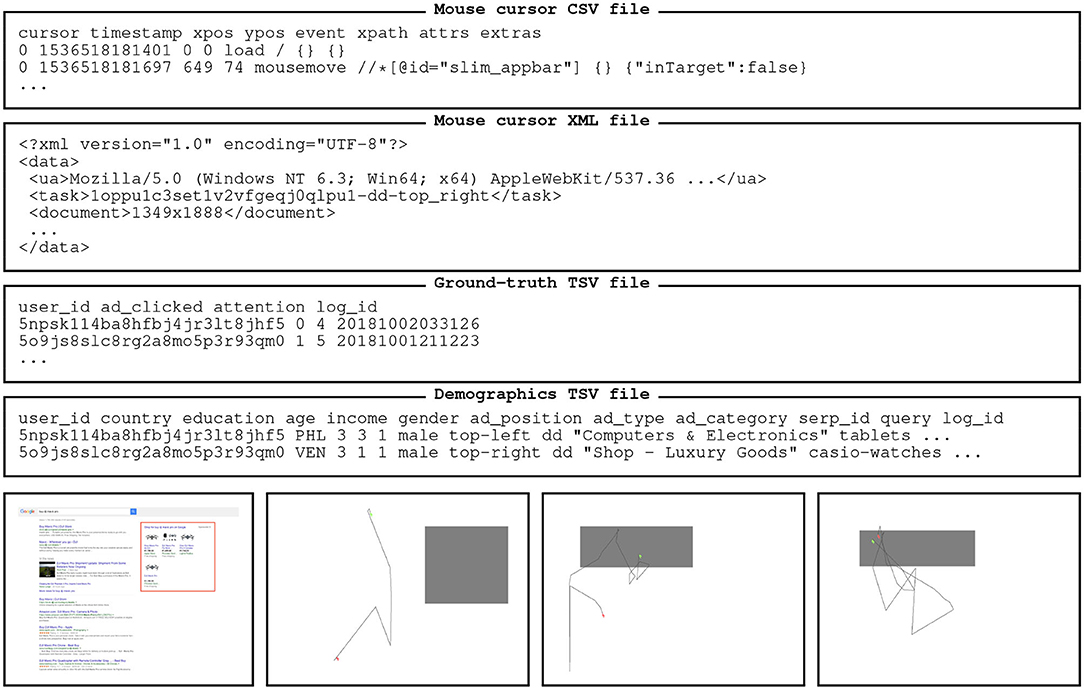
Figure 1. File content samples (top) and SERP snapshots with mouse cursor trajectories (bottom). An ellipsis (…) denotes an intentional omission of some data, for brevity's sake. The gray-colored rectangles in the bottommost figures denote the different ad types, from left to right: right-aligned bundled ad, left-aligned bundled ad, and native ad.
4. Conclusion
We have presented a large-scale, in-the-wild dataset of mouse cursor movements in web search, with associated ground-truth labels about user's attention and demographics attributes. The dataset represents real-world behavior of individuals completing a transactional web search task. What makes this dataset both unique and challenging is the fact that there is only one observation per user. It is not possible to leak information from any data splits; e.g., training, validation, and testing splits typically used in machine learning studies. It is our hope that the dataset will foster research in several scientific domains, Including, e.g., information retrieval, movement science, and psychology.
Data Availability Statement
The raw data supporting the conclusions of this article will be made available by the authors, without undue reservation.
Ethics Statement
Ethical review and approval was not required for the study on human participants in accordance with the local legislation and institutional requirements. The patients/participants provided their written informed consent to participate in this study.
Author Contributions
All authors listed have made a substantial, direct and intellectual contribution to the work, and approved it for publication.
Conflict of Interest
IA was employed by the company Telefonica Research, though no payment or services from the institution has been received or requested for any aspect of the submitted work.
The remaining author declares that the research was conducted in the absence of any commercial or financial relationships that could be construed as a potential conflict of interest.
Acknowledgments
Two manuscripts using a post-processed version of this dataset have been recently published by the authors (Arapakis and Leiva, 2020; Arapakis et al., 2020). LAL acknowledged the support from the Finnish Center for Artificial Intelligence (FCAI).
Footnotes
References
Accot, J., and Zhai, S. (1997). “Beyond fitts' law: Models for trajectory-based HCI tasks,” in Proceedings of CHI (Atlanta, GA), 295–302. doi: 10.1145/258549.258760
Arapakis, I., Lalmas, M., Cambazoglu, B. B., Marcos, M. C., and Jose, J. M. (2014a). User engagement in online news: Under the scope of sentiment, interest, affect, and gaze. J. Assoc. Inf. Sci. Technol. 65, 1988–2005. doi: 10.1002/asi.23096
Arapakis, I., Lalmas, M, and Valkanas, G. (2014b). “Understanding within-content engagement through pattern analysis of mouse gestures,” in Proceedings of CIKM, 1439–1448. doi: 10.1145/2661829.2661909
Arapakis, I., and Leiva, L. A. (2016). “Predicting user engagement with direct displays using mouse cursor information,” in Proceedings of SIGIR (Pisa), 599–608. doi: 10.1145/2911451.2911505
Arapakis, I., and Leiva, L. A. (2020). “Learning efficient representations of mouse movements to predict user attention,” in Proceedings of SIGIR. doi: 10.1145/3397271.3401031
Arapakis, I., Leiva, L. A., and Cambazoglu, B. B. (2015). “Know your onions: understanding the user experience with the knowledge module in web search,” in Proceedings of CIKM (Melbourne, VIC), 1695–1698. doi: 10.1145/2806416.2806591
Arapakis, I., Penta, A., Joho, H., and Leiva, L. A. (2020). A price-per-attention auction scheme using mouse cursor information. ACM Trans. Inf. Syst. 38:13. doi: 10.1145/3374210
Arroyo, E., Selker, T., and Wei, W. (2006). “Usability tool for analysis of web designs using mouse tracks,” in Proceedings of CHIEA (Montréal, QC), 357–362. doi: 10.1145/1125451.1125557
Atterer, R., Wnuk, M., and Schmidt, A. (2006). “Knowing the user's every move: user activity tracking for website usability evaluation and implicit interaction,” in Proceedings of WWW (Edinburgh), 203–212. doi: 10.1145/1135777.1135811
Azcarraga, J., and Suarez, M. T. (2012). “Predicting academic emotions based on brainwaves, mouse behaviour and personality profile,” in Proceedings of PRICAI (Berlin; Heidelberg: Springer), 728–733. doi: 10.1007/978-3-642-32695-0_64
Bohan, M., and Chaparro, A. (1998). Age-related differences in performance using a mouse and trackball. Hum. Factors 42, 152–155. doi: 10.1177/154193129804200202
Boi, P., Fenu, G., Spano, L. D., and Vargiu, V. (2016). Reconstructing user's attention on the web through mouse movements and perception-based content identification. ACM Trans. Appl. Percept. 13, 15:1–15:21. doi: 10.1145/2912124
Brückner, L., Arapakis, I., and Leiva, L. A. (2020). “Query abandonment prediction with deep learning models of mouse cursor movements,” in Proceedings of CIKM. doi: 10.1145/3340531.3412126
Card, S. K., English, W. K., and Burr, B. J. (1987). “Evaluation of mouse, rate controlled isometric joystick, step keys, and text keys, for text selection on a CRT,” in Human-Computer Interaction, eds R. M. Baecker and W. A. S. Buxton (Taylor & Francis), 386–392.
Carlton, J., Brown, A., Jay, C., and Keane, J. (2019). “Inferring user engagement from interaction data,” in Proceedings of CHI EA, 1212, 1–6. doi: 10.1145/3290607.3313009
Chen, M. C., Anderson, J. R., and Sohn, M. H. (2001). “What can a mouse cursor tell us more? Correlation of eye/mouse movements on web browsing,” in Proceedings of CHI EA, 281–282. doi: 10.1145/634067.634234
Chen, R. C. C., and Chen, T.-K. (2008). The effect of gender-related difference on human-centred performance using a mass assessment method. IJCAT 32, 322–333. doi: 10.1504/IJCAT.2008.021387
Chen, Y., Liu, Y., Zhang, M., and Ma, S. (2017). User satisfaction prediction with mouse movement information in heterogeneous search environment. IEEE Trans. Knowl. Data. Eng. 29, 2470–2483. doi: 10.1109/TKDE.2017.2739151
Claypool, M., Le, P., Wased, M., and Brown, D. (2001). “Implicit interest indicators,” in Proceedings of IUI, 33–40. doi: 10.1145/359784.359836
Diaz, F., White, R., Buscher, G., and Liebling, D. (2013). “Robust models of mouse movement on dynamic web search results pages,” in Proceedings of CIKM (San Francisco, CA), 1451–1460. doi: 10.1145/2505515.2505717
Diriye, A., White, R., Buscher, G., and Dumais, S. (2012). “Leaving so soon? Understanding and predicting web search abandonment rationales,” in Proceedings of CIKM, 1025–1034. doi: 10.1145/2396761.2398399
Feild, H. A., Allan, J., and Jones, R. (2010). “Predicting searcher frustration,” in Proceedings of SIGIR, 34–41. doi: 10.1145/1835449.1835458
Gajos, K. Z., Reinecke, K., Donovan, M., Stephen, C. D., Hung, A. Y., Schmahmann, J. D., et al. (2020). Computer mouse use captures ataxia and parkinsonism, enabling accurate measurement and detection. Mov. Disord. 35, 354–358. doi: 10.1002/mds.27915
Goecks, J., and Shavlik, J. (2000). “Learning users' interests by unobtrusively observing their normal behavior,” in Proceedings of IUI, 129–132. doi: 10.1145/325737.325806
Guo, Q., and Agichtein, E. (2008). “Exploring mouse movements for inferring query intent,” in Proceedings of SIGIR (Singapore), 707–708. doi: 10.1145/1390334.1390462
Guo, Q., and Agichtein, E. (2010). “Ready to buy or just browsing? Detecting web searcher goals from interaction data,” in Proceedings of SIGIR, 130–137. doi: 10.1145/1835449.1835473
Guo, Q., and Agichtein, E. (2012). “Beyond dwell time: estimating document relevance from cursor movements and other post-click searcher behavior,” in Proceedings ofWWW, 569–578. doi: 10.1145/2187836.2187914
Guo, Q., Lagun, D., and Agichtein, E. (2012). “Predicting web search success with fine-grained interaction data,” in Proceedings of CIKM, 2050–2054. doi: 10.1145/2396761.2398570
Hauger, D., Paramythis, A., and Weibelzahl, S. (2011). “Using browser interaction data to determine page reading behavior,” in Proceedings of UMAP (Berlin; Heidelberg: Springer), 147–158. doi: 10.1007/978-3-642-22362-4_13
Hsu, S. H., Huang, C. C., Tsuang, Y. H., and Sun, J. S. (1999). Effects of age and gender on remote pointing performance and their design implications. Int. J. Ind. Ergon. 23, 461–471. doi: 10.1016/S0169-8141(98)00013-4
Huang, J., White, R., and Buscher, G. (2012). “User see, user point: gaze and cursor alignment in web search,” in Proceedings of CHI, 1341–1350. doi: 10.1145/2207676.2208591
Huang, J., White, R. W., and Dumais, S. (2011). “No clicks, no problem: using cursor movements to understand and improve search,” in Proceedings of CHI, 1225–1234. doi: 10.1145/1978942.1979125
Jastrzembski, T., Charness, N., Holley, P., and Feddon, J. (2003). Input devices for web browsing: age and hand effects. Universal Access Inf. 4, 39–45. doi: 10.1007/s10209-003-0083-5
Johnson, S., Smith, P., and Tucker, S. (1982). Response format of the job descriptive index: assessment of reliability and validity by the multitrait-multimethod matrix. J. Appl. Psychol. 67, 500–505. doi: 10.1037/0021-9010.67.4.500
Kaklauskas, A., Krutinis, M., and Seniut, M. (2009). “Biometric mouse intelligent system for student's emotional and examination process analysis,” in Proceedings of ICALT (Riga), 189–193. doi: 10.1109/ICALT.2009.130
Kapoor, A., Burleson, W., and Picard, R. W. (2007). Automatic prediction of frustration. Int. J. Hum. Comput. Stud. 65, 724–736. doi: 10.1016/j.ijhcs.2007.02.003
Kratky, P., and Chuda, D. (2016). “Estimating gender and age of web page visitors from the way they use their mouse,” in Proceedings of WWW Companion, 61-62. doi: 10.1145/2872518.2889384
Krátky, P., and Chudá, D. (2018). Recognition of web users with the aid of biometric user model. J. Intell. Inf. Syst. 51, 621–646. doi: 10.1007/s10844-018-0500-0
Lagun, D., Ageev, M., Guo, Q., and Agichtein, E. (2014a). “Discovering common motifs in cursor movement data for improving web search,” in Proceedings of WSDM, 183–192. doi: 10.1145/2556195.2556265
Lagun, D., and Agichtein, E. (2015). “Inferring searcher attention by jointly modeling user interactions and content salience,” in Proceedings of SIGIR, 483–492. doi: 10.1145/2766462.2767745
Lagun, D., Hsieh, C.-H., Webster, D., and Navalpakkam, V. (2014b). “Towards better measurement of attention and satisfaction in mobile search,” in Proceedings of SIGIR, 113–122. doi: 10.1145/2600428.2609631
Landauer, A. A. (1981). Sex differences in decision and movement time. Percept. Mot. Skills 52, 90–90. doi: 10.2466/pms.1981.52.1.90
Leiva, L. A. (2011a). “Mousehints: easing task switching in parallel browsing,” in Proceedings of CHI EA, 1957–1962. doi: 10.1145/1979742.1979861
Leiva, L. A. (2011b). “Restyling website design via touch-based interactions,” in Proceedings of Mobile HCI (Stockholm), 599–604. doi: 10.1145/2037373.2037467
Leiva, L. A. (2012). “Automatic web design refinements based on collective user behavior,” in Proceedings of CHI EA, 1607–1612. doi: 10.1145/2212776.2223680
Leiva, L. A., and Huang, J. (2015). Building a better mousetrap: compressing mouse cursor activity for web analytics. Inf. Process. Manag. 51, 114–129. doi: 10.1016/j.ipm.2014.10.005
Leiva, L. A., and Vivó, R. (2013). Web browsing behavior analysis and interactive hypervideo. ACM Trans. Web 7, 20:1–20:28. doi: 10.1145/2529995.2529996
Lindberg, T., Näsänen, R., and Müller, K. (2006). How age affects the speed of perception of computer icons. Displays 27, 170–177. doi: 10.1016/j.displa.2006.06.002
Liu, Y., Chen, Y., Tang, J., Sun, J., Zhang, M., Ma, S., et al. (2015). “Different users, different opinions: predicting search satisfaction with mouse movement information,” in Proceedings of SIGIR (Santiago), 493–502. doi: 10.1145/2766462.2767721
Lu, H., Rose, J., Liu, Y., Awad, A., and Hou, L. (2017). “Combining mouse and eye movement biometrics for user authentication,” in Information Security Practices, eds I. Traoré, A. Awad, and I. Woungang (Cham: Springer), 55–71. doi: 10.1007/978-3-319-48947-6_5
Martín-Albo, D., Leiva, L. A., Huang, J., and Plamondon, R. (2016). Strokes of insight: user intent detection and kinematic compression of mouse cursor trails. Inf. Process. Manag. 52, 989–1003. doi: 10.1016/j.ipm.2016.04.005
Mason, W., and Suri, S. (2012). Conducting behavioral research on Amazon's Mechanical Turk. Behav. Res. Methods 44, 1–23. doi: 10.3758/s13428-011-0124-6
Mueller, F., and Lockerd, A. (2001). “Cheese: tracking mouse movement activity on websites, a tool for user modeling,” in Proceedings of CHI EA, 279–280. doi: 10.1145/634067.634233
Navalpakkam, V., Jentzsch, L., Sayres, R., Ravi, S., Ahmed, A., and Smola, A. (2013). “Measurement and modeling of eye-mouse behavior in the presence of nonlinear page layouts,” in Proceedings of WWWW, 953–964. doi: 10.1145/2488388.2488471
Pentel, A. (2017). “Predicting age and gender by keystroke dynamics and mouse patterns,” in Adjunct Proceedings of UPMAP (Bratislava), 381–385. doi: 10.1145/3099023.3099105
Shapira, B., Taieb-Maimon, M, and Moskowitz, A. (2006). “Study of the usefulness of known and new implicit indicators and their optimal combination for accurate inference of users interests,” in Proceedings of SAC (Dijon), 1118–1119. doi: 10.1145/1141277.1141542
Smith, M. W., Sharit, J., and Czaja, S. J. (1999). Aging, motor control, and the performance of computer mouse tasks. Hum. Factors 41, 389–396. doi: 10.1518/001872099779611102
Speicher, M., Both, A., and Gaedke, M. (2013). “TellMyRelevance! Predicting the relevance of web search results from cursor interactions,” in Proceedings of CIKM (Burlingame, CA), 1281–1290. doi: 10.1145/2505515.2505703
Walker, N., Philbin, D. A., and Fisk, A. D. (1997). Age-related differences in movement control: adjusting submovement structure to optimize performance. J. Gerontol. A Biol. Sci. Med. Sci. 52, 389–396. doi: 10.1093/geronb/52B.1.P40
White, R., Doraiswamy, P., and Horvitz, E. (2018). Detecting neurodegenerative disorders from web search signals. NPJ Digit. Med. 1:8. doi: 10.1038/s41746-018-0016-6
Yamauchi, T. (2013). “Mouse trajectories and state anxiety: feature selection with random forest,” in Proceedings of ACII (Geneva), 399–404. doi: 10.1109/ACII.2013.72
Yamauchi, T., and Bowman, C. (2014). “Mining cursor motions to find the gender, experience, and feelings of computer users,” in Proceedings of ICDMW (Shenzhen), 221–230. doi: 10.1109/ICDMW.2014.131
Yamauchi, T., Seo, J. H., Jett, N., Parks, G., and Bowman, C. (2015). Gender differences in mouse and cursor movements. Int. J. Hum. Comput. Interact. 31, 911–921. doi: 10.1080/10447318.2015.1072787
Keywords: aimed movements, attention, demographics, web search, mouse cursor
Citation: Leiva LA and Arapakis I (2020) The Attentive Cursor Dataset. Front. Hum. Neurosci. 14:565664. doi: 10.3389/fnhum.2020.565664
Received: 25 May 2020; Accepted: 19 October 2020;
Published: 16 November 2020.
Edited by:
Claudio De Stefano, University of Cassino, ItalyReviewed by:
Hugo Gamboa, New University of Lisbon, PortugalNicole Cilia, University of Cassino, Italy
Copyright © 2020 Leiva and Arapakis. This is an open-access article distributed under the terms of the Creative Commons Attribution License (CC BY). The use, distribution or reproduction in other forums is permitted, provided the original author(s) and the copyright owner(s) are credited and that the original publication in this journal is cited, in accordance with accepted academic practice. No use, distribution or reproduction is permitted which does not comply with these terms.
*Correspondence: Luis A. Leiva, Zmlyc3RuYW1lLmxhc3RuYW1lQGFhbHRvLmZp