- 1Department of Medicine, Aga Khan University, Karachi, Pakistan
- 2Center of Excellence in Women and Child Health, Aga Khan University, Karachi, Pakistan
- 3Head and Neck Oncology, The Ohio State University, Columbus, OH, United States
- 4Institute for Global Health and Development, Aga Khan University, Karachi, Pakistan
- 5Department of Pediatrics and Child Health, Aga Khan University, Karachi, Pakistan
Background: Artificial Intelligence (AI) holds considerable promise for diagnostics in the field of gastroenterology. This systematic review and meta-analysis aims to assess the diagnostic accuracy of AI models compared with the gold standard of experts and histopathology for the diagnosis of various gastrointestinal (GI) luminal pathologies including polyps, neoplasms, and inflammatory bowel disease.
Methods: We searched PubMed, CINAHL, Wiley Cochrane Library, and Web of Science electronic databases to identify studies assessing the diagnostic performance of AI models for GI luminal pathologies. We extracted binary diagnostic accuracy data and constructed contingency tables to derive the outcomes of interest: sensitivity and specificity. We performed a meta-analysis and hierarchical summary receiver operating characteristic curves (HSROC). The risk of bias was assessed using Quality Assessment for Diagnostic Accuracy Studies-2 (QUADAS-2) tool. Subgroup analyses were conducted based on the type of GI luminal disease, AI model, reference standard, and type of data used for analysis. This study is registered with PROSPERO (CRD42021288360).
Findings: We included 73 studies, of which 31 were externally validated and provided sufficient information for inclusion in the meta-analysis. The overall sensitivity of AI for detecting GI luminal pathologies was 91.9% (95% CI: 89.0–94.1) and specificity was 91.7% (95% CI: 87.4–94.7). Deep learning models (sensitivity: 89.8%, specificity: 91.9%) and ensemble methods (sensitivity: 95.4%, specificity: 90.9%) were the most commonly used models in the included studies. Majority of studies (n = 56, 76.7%) had a high risk of selection bias while 74% (n = 54) studies were low risk on reference standard and 67% (n = 49) were low risk for flow and timing bias.
Interpretation: The review suggests high sensitivity and specificity of AI models for the detection of GI luminal pathologies. There is a need for large, multi-center trials in both high income countries and low- and middle- income countries to assess the performance of these AI models in real clinical settings and its impact on diagnosis and prognosis.
Systematic review registration: [https://www.crd.york.ac.uk/prospero/display_record.php?RecordID=288360], identifier [CRD42021288360].
Introduction
Gastrointestinal (GI) pathologies contribute to a significant burden of disease worldwide. With 89 million global disability-adjusted life years (DALYs), GI pathologies contributed 3.5% (2,280 million cases) to the total global DALYs in 2019, with a greater prevalence in low and middle-income countries (LMICs) (1). In 2018, there were more than 36⋅8 million ambulatory visits in the United States for GI symptoms and 43⋅4 million had a primary GI diagnosis. Annually, a total of 22.2 million GI endoscopies were performed, with 284,844 new GI cancers diagnosed and 255,407 deaths (2). Other parts of the world, including LMICs also have an increasing burden of GI pathologies, as 80% of the esophageal cancer burden of the world is from LMICs, with poor survival (3). The morbidity and mortality due to GI causes is higher than other common pathologies and hence underscores a significant burden that GI adds to the overall health care system.
Despite this high burden of disease, there are multiple challenges that hinder the provision of optimal GI care. In LMICs, it is often the lack of resources such as endoscopy equipment and availability of skills and experts for timely diagnosis and intervention (4). In high income regions, these challenges include high costs along with discrepancies in facilities and training (5). Overcoming these challenges would require significant amount of resources and time. Although progress has been made globally to enhance these skills, this capacity is still lagging. However, innovations in technology have proven to be a beacon to overcome these challenges adeptly and efficiently.
The introduction of artificial intelligence (AI) in health care has led to innovations in diagnosis, management and prognosis of many conditions at a fast pace. AI algorithms in gastroenterology have been studied over many years to automate the interpretation of diagnostic procedures in gastroenterology albeit with varying levels of success. Since 2010, AI has explored multiple procedures and pathologies in gastroenterology (6). The AI models have been applied to the interpretation of endoscopy, pill video endoscopy, ultrasound manometry, and microcytoscopy (7–17). Traditionally, these procedures yield large amounts of data which require an expert’s time and attention to draw clinical conclusions. However, AI models in these studies have shown to recognize polyps, areas of inflammation, and degrees of inflammation accurately. A recent randomized controlled trial reported much lower miss rates for a deep learning model for polyp detection compared to the standard (20.1% vs. 31.2%) (18). The massive influx and availability of data, along with promising performance of AI in lesion detection, makes incorporation of AI in healthcare promising. However, there is a need to synthesize the existing literature to quantify the accuracy of AI algorithm in detection of GI disease.
The primary objective of this systematic review and meta-analysis was to assess the diagnostic accuracy of AI models compared with the gold standard of experts and histopathology for the diagnosis of various gastrointestinal luminal pathologies, including polyps, neoplasms, inflammatory bowel disease (IBD), celiac disease, and Barrett’s esophagus. The secondary objective was to describe the diagnostic accuracy of different types of AI models for the diagnosis of each GI luminal pathology.
Methods
The protocol for this review was prospectively registered at PROSPERO (CRD42021288360). We followed the Preferred Reporting Items for Systematic reviews and Meta-Analyses (PRISMA) guidelines for diagnostic test accuracy for analysis reporting in this publication (19).
Eligibility criteria and search strategy
We included all observational studies that reported the diagnostic results of an AI algorithm for the detection of GI luminal pathologies when compared to a reference standard (expert opinion or consensus, histopathology, or laboratory testing such as urea breath test for H. pylori etc.). No restrictions were applied based on the age at diagnosis or type of AI algorithm used in the study. Studies with an unclear description of reference standard or type of GI luminal pathologies, published in a language other than English, and those that graded the severity of an already diagnosed disease were excluded. We excluded letters, opinions, preprints, scientific reports, and narrative reviews. Studies based on animals or non-human samples or that presented duplicate data were excluded.
We searched PubMed, CINAHL, Wiley Cochrane Library, and Web of Science electronic databases to identify relevant articles published until January 27, 2021. The keywords used for the search included, “Algorithms,” “Artificial Intelligence,” “Machine Learning,” “Deep Learning,” “Supervised Machine Learning,” “Unsupervised Machine Learning,” “Gastroenterology,” “Celiac Disease,” “Inflammatory Bowel Disease*,” “Irritable Bowel Syndrome,” “Polyp*,” “Crohn Disease,” “Gastro*,” “Endoscopy,” “Scopy,” “Capsule Endoscopy,” “Endomicroscopy,” “Colonoscopy,” “Ultrasound Manometry,” “Diagnosis,” “Diagnos*,” “Accuracy,” “Sensitivity and Specificity,” “Area Under Curve,” “Sensitivity,” and “Specificity.” A full search strategy for each database is available in Supplementary material. All records were imported to Covidence, and duplicates were removed.
Screening and data extraction
Two authors (AS and FR) independently screened titles and abstracts to assess for potential eligibility. Full texts of all screened studies were also reviewed by two authors for final selection. We manually searched bibliographies and citations of included studies and relevant systematic reviews to identify any additional relevant articles that might have been missed in the initial search. Eligibility assessment was done by two reviewers at all stages independently, and disagreements were resolved by involving a third reviewer (JKD, OP).
Two authors (AS and UJ) independently extracted information to a pre-formed data extraction sheet on Excel. Data obtained included information about the study (first author, year of publication, journal, study title, country, income region of the country according to the World Bank, aim of the study, study design, study setting, sample size (including size of training and test set), method of population selection, patient characteristics (age range, type of GI luminal pathologies), the AI algorithm used, the reference standard, the type of data used for analysis (per-image, per-lesion, or per-patient analysis), reported performance metrics (sensitivity, specificity, and area under the curve), validation of the model (internal or external) and sub-group data if present.
Risk of bias assessment
The risk of bias was assessed by two authors (AS, UJ) independently using quality assessment for diagnostic accuracy studies-2 (QUADAS-2) tool (20). Domains for risk of bias included patient selection, index test, reference standard, and flow and timing, with the first three domains also considered in terms of applicability concerns. If one of the questions within the domain was scored at high risk of bias, the domain was scored as high risk. Disagreements in data extraction and quality assessment were resolved by discussion with a third reviewer (ZH or JKD).
Data analysis
Where possible, we extracted 2 × 2 contingency tables or data to construct such tables. Contingency tables consisted of true-positive, false-positive, true-negative, and false-negative results, and were used to calculate sensitivity, specificity, and accuracy. External validation is important to establish the quality and generalizability of machine learning models (21), while internally validation alone has a potential to overestimate the accuracy of the model (22), therefore to estimate the accuracy of AI algorithms, we conducted a meta-analysis of studies that provided data for contingency tables separate for externally validated data (test data) and internally validated data (training data). If a study tested more than one AI model or more than one dataset, all contingency tables were included in the meta-analysis.
For all included studies, we entered the data provided into Review Manager (RevMan 5.4.1) software (23) where the sensitivity, specificity and their 95% confidence intervals (CIs) were presented in the form of forest plots and receiver operating characteristic (ROC) curves. This analysis utilized the sensitivity and specificity results from each included study using the metandi command for bivariate model in STATA version 17 (24) to generate hierarchical Summary ROC (HSROC) curves.
Subgroup analyses were conducted based on the type of GI luminal pathologies, AI model, reference standard, and data used for analysis (image, lesions, or patients). Subgroup analyses were performed if at least four studies in each sub-group could be analyzed together. Subgroup analysis was conducted on the various GI luminal pathologies listed, types of AI models used, reference standards and types of input data (per patient, image, or lesion). We grouped AI models together according to their class (Figure 1). We performed separate analysis for externally and internally validated studies. We also conducted an exploratory analysis on internally validated studies to evaluate the diagnostic accuracy of AI models on internally validated data.
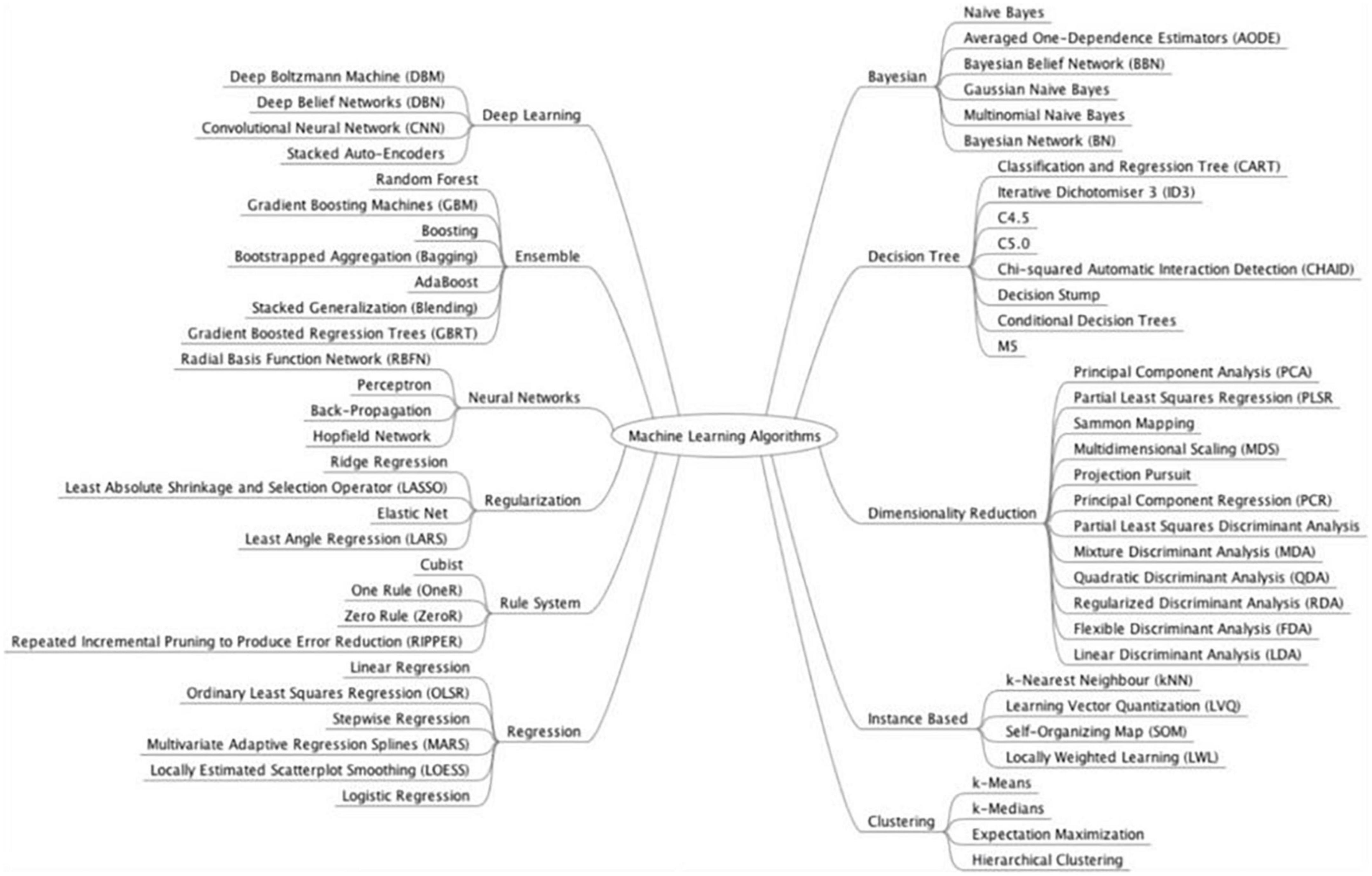
Figure 1. Model classification. Source: Brownlee (104).
Results
The search strategy identified 5,586 articles for title/abstract screening, of which 219 full texts were screened for eligibility. Altogether, 73 studies were included in the review. Of these studies, 68 studies were externally validated and five were internally validated. Among the externally validated studies 31 (42.5%) studies were included in the meta-analysis (10, 17, 25–53) while 37 (50.7%) studies were narratively synthesized due to insufficient information to calculate the contingency tables (11–14, 54–86). The five (6.8%) internally validated studies provided sufficient information to calculate contingency tables and were included in the exploratory analysis (87–91). The details of the study flow diagram have been shown in Figure 2.
Among the 73 included studies, 53 (72.6%) were case-control, 17 (23.3%) were cohort, one (1.4%) was a mixed-method study, and two (2.7%) studies failed to define the study design (Tables 1,2). Majority of the included studies were conducted in high income countries (HICs) (n = 54, 73.9%) and upper–middle income countries (UMICs) (n = 14, 19.2%), while four (5.5%) were conducted in both HICs and UMICs, and one (1.4%) in both HIC and LMIC. Diagnostic performance of AI was most tested on colorectal polyps (n = 29, 39.7%), followed by ulcers (n = 7, 9.6%), celiac disease (n = 6, 8.2%), IBD (n = 5, 6.8%), Barrett’s esophagus (n = 3, 4.1%), and gastric polyps (n = 3, 4.1%). Sixteen studies used AI to differentiate between benign and precancerous or cancerous polyps, while 10 studies used AI to diagnose more than one type of disease.
Only 23 (31.5%) studies reported the age of the participants. Nineteen studies (82.6%) reported on adult population, three (13%) on pediatric population and one (4.3%) study reported on both. At least one dataset used in 16 (21.9%) studies, was a publicly available database (34, 37–39, 42, 43, 47, 50, 54, 58, 60, 67, 68, 71, 76, 81). Sixty three (86.3%) studies used their own data (10–14, 17, 25–36, 38–41, 44–46, 48, 49, 51–53, 55–59, 61–66, 69, 70, 72–91), while 18 (28.6%) collected data prospectively (11, 13, 26, 27, 32, 35, 38, 39, 45, 55, 56, 59, 65, 70, 73, 74, 80, 87).
Methodological quality of the included studies
Details of risk of bias and applicability concerns are presented in Figure 3. Fifty-six studies (76.7%) had a high risk of bias in patient selection (11–13, 17, 25–27, 29–31, 34, 36–39, 41–44, 46, 47, 50–54, 57, 58, 60–72, 74–79, 81, 83–86, 88–91), mostly due to the case-control study design (n = 54, 74%) (11–13, 17, 25–27, 29–31, 34, 36–39, 41–44, 46, 47, 50–54, 57, 58, 60–64, 66–72, 75–79, 81, 83–86, 88–91). Two studies had low risk of bias for patient selection because of random participant sampling, and due to appropriate exclusion of participants (55, 87), while 15 studies (20.5%) did not provide sufficient information for risk classification (10, 14, 28, 32, 33, 35, 40, 45, 48, 49, 56, 59, 73, 80, 82). Majority of the studies had unclear (n = 63, 86.3%) (10, 11, 13, 14, 17, 25–33, 35, 36, 38, 39, 41–45, 48–55, 57–59, 61–88, 91) or high (n = 9, 12.3%) (12, 34, 37, 46, 47, 56, 60, 89, 90) risk of bias for index test, which was most often due to insufficient information on blinding of the index test (n = 67, 91.8%) (11–14, 17, 25–33, 36–39, 41–64, 66–72, 74–91) and pre-specification of diagnostic threshold (n = 61, 83.6%) (10, 11, 13, 14, 17, 25, 26, 29–33, 35, 36, 38, 39, 41–45, 48–55, 57–59, 61–88, 91). Fifty-four (74.0%) studies were classified as low risk for reference standard due to correct classification of the condition and interpretation of diagnostic test without the knowledge of the index test (10–13, 17, 26–36, 38, 39, 41, 44, 45, 47–49, 51–53, 56, 58–63, 69–72, 74–79, 81–90), while 49 (67.1%) were categorized as low risk for flow and timing biases due to inclusion of all the participants, appropriate interval between index test and reference standard and due to provision of same reference standard to the all the ails of risk of bias and applicab participants (10, 11, 13, 17, 26–33, 35–37, 41, 45, 48, 51–53, 55–64, 69, 70, 72, 74–79, 81–85, 87–89, 91). No study had concerns about applicability in all three domains.
Detection of any gastrointestinal luminal pathology by externally validated artificial intelligence models
Thirty-six studies provided sufficient information to calculate contingency table values (10, 17, 25–53, 87–91). Of these, 31 studies (86.1%) conducted external validation and were included in the meta-analysis (10, 17, 25–53). Contingency tables of per-image analyses were used for the meta-analysis; if the study did not report per-image analyses, then per-lesion results were included. If neither per-image nor per-lesion analyses were reported, per-patient results were used. The hierarchical summary ROC curve of these studies is shown in Figure 4. The overall sensitivity from these studies was 91.9% (95% CI: 89.0–94.1) while the specificity was 91.7% (95% CI: 87.4–94.7).
Subgroup analyses based on different gastrointestinal luminal pathologies
Polyps (n = 13, 36.1%) (29, 34, 38, 42–48, 50, 52, 91) and neoplasms (n = 14, 38.9%) (25–27, 32, 33, 36, 37, 39, 40, 45, 48, 87, 89, 90) were the most commonly reported GI luminal pathologies. Two studies (5.5%) modeled AI for the detection of celiac disease (17, 49), while ulcers (51), IBD (10), and GERD (88) were reported in one study (2.8%) each. The sensitivity of AI models for detecting polyps was 94.0% (95% CI: 88.7–97.0) and the specificity was 95.4% (89.9–98.0) (Figure 5A). AI models demonstrated a sensitivity of 86.3% (95% CI: 82.0–89.7) and specificity of 82.6% (95% CI: 75.6–87.9) for diagnosing GI luminal neoplasms (Figure 5B). No subgroup analyses were performed for the other pathologies due to insufficient number of studies.
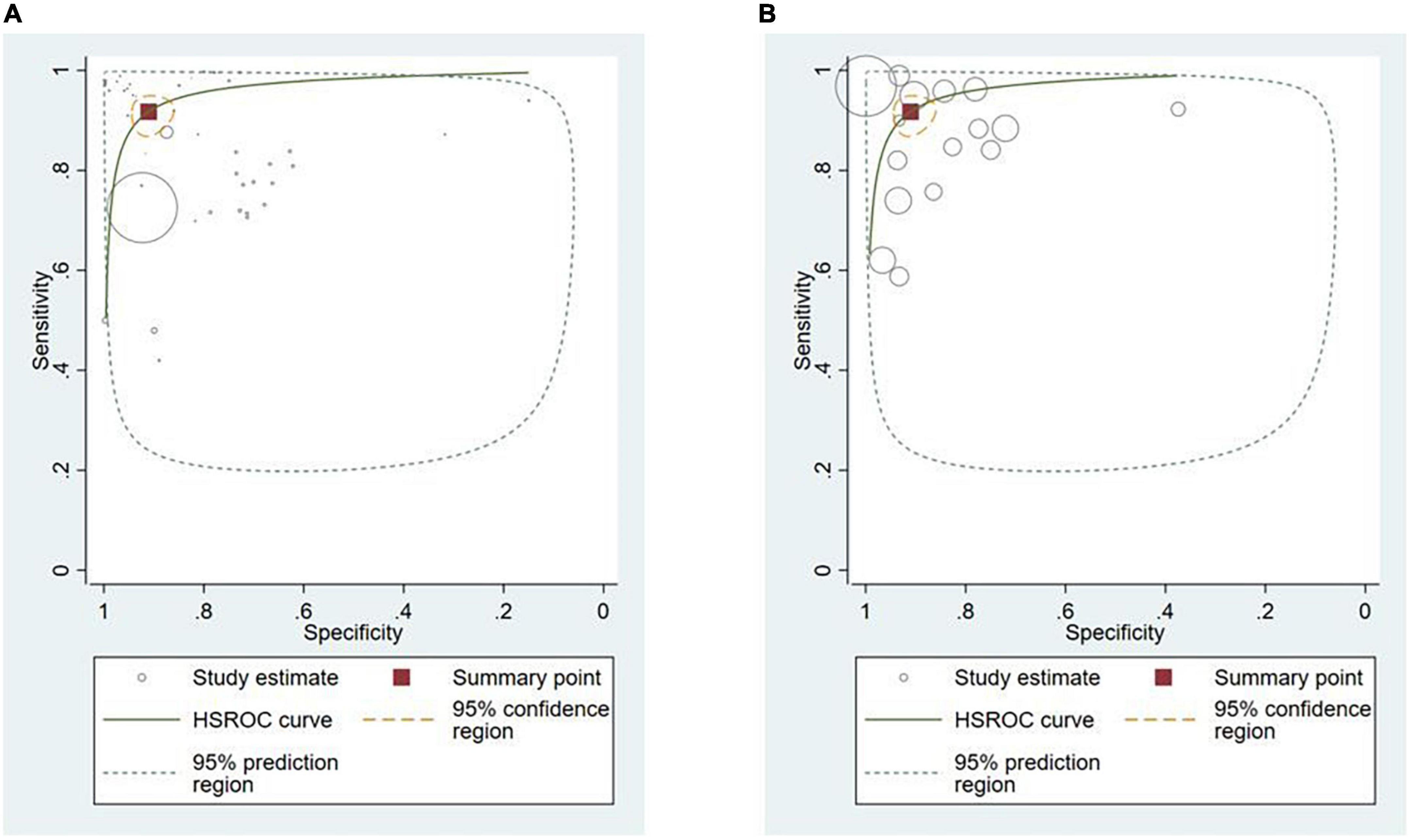
Figure 5. Hierarchical summary ROC curve for detecting GI luminal diseases. (A) GI polyps and (B) GI neoplasms.
Subgroup analyses based on artificial intelligence model
Twenty-two studies used deep learning (61.1%) (17, 25, 26, 28, 30–32, 34, 36–42, 44–46, 51–53, 89) and 15 studies used ensemble methods (41.7%) (10, 27, 29, 33, 42–44, 47–50, 87, 88, 90, 91) for the detection of GI luminal pathologies, while the other AI models reported by the studies included artificial neural networks (ANN) (n = 1, 2.8%) (35), k-nearest neighbors (kNN) (n = 1, 2.8%) (50) learning vector quantization (LVQ) (n = 1, 2.8%) (44) and decision trees (n = 1, 2.8%) (50). The sensitivity of deep learning models to detect any GI luminal pathology was 89.8% (95% CI: 85.9–92.7) while that of ensemble methods was 95.4% (95% CI: 91.3–97.6). The specificity of deep learning and ensemble methods was 91.9% (95% CI: 85.7–95.6) and 90.9% (95% CI: 86.2–94.1), respectively (Supplementary Figures). ANN, k-NN, LVQ, and decision trees weren’t included in the subgroup analyses due to the lack of sufficient number of studies.
Subgroup analyses based on different reference standards
Twenty (55.5%) studies used expert diagnosis (17, 27, 28, 34, 36–38, 41–47, 50–53, 88, 91) and 14 (38.9%) studies used histopathology as the reference standard (10, 25, 26, 32, 33, 38–40, 45, 48, 49, 87, 89, 90). The sensitivity and specificity of AI models when compared to expert opinion was 90.5% (95% CI: 86.5–93.4) and 93.3% (95% CI: 88.1–96.4). Studies that used histopathology as the reference standard reported 79.8% (95% CI: 38.2–96.2) sensitivity and 97.6% specificity (95% CI: 94.0–99.1) (Supplementary Figures).
Detection of any gastrointestinal luminal pathology by internally validated artificial intelligence models
The performance of AI models on internally validated data (n = 5, 13.8%) (87–91) was similar to its performance on externally validated data (sensitivity: 91.9%; 95% CI: 89.0–94.1, specificity: 91.7%; 95% CI: 87.4–94.7). The exploratory analysis of internally validated studies showed a sensitivity of 92.9% (95% CI: 89.3–95.4) and specificity of 90.1% (83.8–94.1%).
Discussion
This meta-analysis aimed to synthesize the existing evidence regarding diagnostic accuracy of AI models in detecting common GI luminal pathologies as compared to the reference standard. AI models such as deep learning techniques and ensemble methods were the most commonly deployed models to detect GI luminal pathologies with reported high sensitivity and specificity (> 90%). The most common GI luminal pathologies investigated were the occurrence of polyps and GI neoplasm, both of which had high accuracy.
AI is rapidly gaining momentum across various industries including healthcare. AI has been used in diagnostics, management and improving administrative efficiency of healthcare systems (92). Benefits of AI have been reported in several fields of medicine including cardiology (93), radiology (94) and pediatrics (95). Gastroenterologists are a subset of clinicians who handle large volumes of clinical as well as imaging data obtained through various procedures such as endoscopy and colonoscopy (96). AI algorithms have proven to show high accuracy in diagnosing various gastroenterological pathologies such as polyps, Barrett’s esophagus, celiac disease and Inflammatory Bowel disease (96). These algorithms range from neural networks to ensemble methods such as support vector machines and deep learning techniques (96). In the current review, deep learning and ensemble methods were the most commonly used AI models in the included studies. Our study reported a sensitivity of 86.3% and specificity of 82.6% for diagnosing GI luminal neoplasms. This is similar to the meta-analysis done by Zhang et al. which reported a sensitivity of 94% and specificity of 82% for AI models on esophageal neoplasms only (97). Similarly, a meta-analysis of the accuracy of AI was superior to experts in the detection of conditions such as Barrett’s esophagus and helicobacter Pylori infection (98).
From the 73 studies included in this review, only one study was from a LMIC (Pakistan) while the remaining were from UMIC or HICs. As demonstrated by the Global Burden of Disease data, the burden of benign and neoplastic GI pathologies in LMICs is significantly higher than UMIC and HICs (99). The disproportionately high prevalence of disease alongside poor access to healthcare facilities, lack of equipment and trained professionals in these regions contributes to poor health outcomes (100). It is in these regions that AI may play a disruptive role in healthcare. The areas of impact of AI in LMICs have been well documented by the United States Agency for International Development (USAID) where physician decision support systems may be one of the domains where deployment of these algorithms could help increase access and high-quality care for medical conditions such as GI pathologies which are highly prevalent in these regions (101). Despite this potential implication and similar to other complex conditions prevalent in LMICs (102), published literature on use of AI in healthcare in these regions is lacking.
As seen in the current review as well, majority of literature on AI in medicine is retrospective. The number of prospective studies where AI is implemented in a clinical setting is limited. There is lack of methodological rigor in the design and conduct of the studies published with the risk of bias in most of the domains stated as unclear or high. It is for this reason that clinicians specifically and health systems in general, lack the confidence in such algorithms thus precluding implementation and large-scale benefits of AI in real world settings (103). Future work needs to include methodological rigor in prospectively designed collaborative studies to demonstrate the use of AI in detection of GI pathologies in HICs as well as LMICs. With higher accuracy of detecting these conditions as compared to a human interpreter, the implications of AI in terms of efficient use of resources and better patient outcomes can be substantial.
To the best of our knowledge, this is the first meta-analysis on the diagnostic accuracy of AI algorithms in both upper and lower GI pathologies. Compared to other reviews, we also explored various subgroups in terms of best performing AI models and across world regions where these studies had been conducted. However, there are some limitations of our work. Our review included studies published in the English Language only. This review focused on certain GI luminal pathologies and is no means an exhaustive exercise to include other GI conditions such as liver, pancreatic and biliary pathology. We also did not include studies where AI models utilized in gastroenterological radiology were compared to expert radiologists.
AI models have the potential to accurately diagnose GI lesions based on endoscopic findings as compared to experts. These results could have significant implications for patient related outcomes in resource constrained settings where trained personnel to interpret these images are limited. However, to fully reap the benefits of AI models, prospectively designed large, multi-center studies are required to demonstrate the effectiveness of the results and for implementation of AI into routine clinical practice across HIC and LMICs.
Data availability statement
The original contributions presented in this study are included in the article/Supplementary material, further inquiries can be directed to the corresponding author.
Author contributions
AS, UJ, and FR were involved in the literature search, data collection, analysis, and writing. AR were involved in data analysis, interpretation, and writing. OP, ZH, ZP, and JD were involved in study design, data analysis, interpretation, and writing of the manuscript. All authors contributed to the article and approved the submitted version.
Conflict of interest
The authors declare that the research was conducted in the absence of any commercial or financial relationships that could be construed as a potential conflict of interest.
Publisher’s note
All claims expressed in this article are solely those of the authors and do not necessarily represent those of their affiliated organizations, or those of the publisher, the editors and the reviewers. Any product that may be evaluated in this article, or claim that may be made by its manufacturer, is not guaranteed or endorsed by the publisher.
Supplementary material
The Supplementary Material for this article can be found online at: https://www.frontiersin.org/articles/10.3389/fmed.2022.1018937/full#supplementary-material
References
1. IHME,. Digestive Diseases — Level 2 Cause. (2019). Available online at: https://www.healthdata.org/results/gbd_summaries/2019/digestive-diseases-level-2-cause (accessed June 30, 2022).
2. Peery AF, Crockett SD, Murphy CC, Jensen ET, Kim HP, Egberg MD, et al. Burden and cost of gastrointestinal, liver, and pancreatic diseases in the United States: update 2021. Gastroenterology. (2022) 162:621–44. doi: 10.1053/j.gastro.2021.10.017
3. Pourhoseingholi MA, Vahedi M, Baghestani AR. Burden of gastrointestinal cancer in Asia; an overview. Gastroenterol Hepatol Bed Bench. (2015) 8:19–27.
4. Chuks NS. Challenges of Gastrointestinal Endoscopy in Resource-Poor Countries. In: Pascu O editor. Gastrointestinal Endoscopy. Rijeka: IntechOpen (2011).
5. Maida M, Alrubaiy L, Bokun T, Bruns T, Castro V, China L, et al. Current challenges and future needs of clinical and endoscopic training in gastroenterology: a European survey. Endosc Int Open. (2020) 8:E525–33. doi: 10.1055/a-1093-0877
6. Le Berre C, Sandborn WJ, Aridhi S, Devignes M-D, Fournier L, Smaïl-Tabbone M, et al. Application of artificial intelligence to gastroenterology and hepatology. Gastroenterology. (2020) 158:76–94.e2. doi: 10.1053/j.gastro.2019.08.058
7. Ciaccio EJ, Tennyson CA, Bhagat G, Lewis SK, Green PH. Implementation of a polling protocol for predicting celiac disease in videocapsule analysis. World J Gastrointest Endosc. (2013) 5:313–22. doi: 10.4253/wjge.v5.i7.313
8. Girgis HZ, Mitchell B, Dassopouios T, Mullin G, Haga G. An intelligent system to detect Crohn’s disease inflammation in Wireless Capsule Endoscopy videos. 2010 IEEE International Symposium on Biomedical Imaging: From Nano to Macro. Rotterdam: IEEE (2010). p. 1373–6.
9. Kumar R, Zhao Q, Seshamani S, Mullin G, Hager G, Dassopoulos T. Assessment of crohn’s disease lesions in wireless capsule endoscopy images. IEEE Trans Bio-Med Eng. (2012) 59:355–62. doi: 10.1109/TBME.2011.2172438
10. Maeda Y, Kudo S-E, Mori Y, Misawa M, Ogata N, Sasanuma S, et al. Fully automated diagnostic system with artificial intelligence using endocytoscopy to identify the presence of histologic inflammation associated with ulcerative colitis (with video). Gastrointest Endosc. (2019) 89:408–15. doi: 10.1016/j.gie.2018.09.024
11. Mossotto E, Ashton JJ, Coelho T, Beattie RM, MacArthur BD, Ennis S. Classification of paediatric inflammatory bowel disease using machine learning. Sci Rep. (2017) 7:2427. doi: 10.1038/s41598-017-02606-2
12. Ozawa T, Ishihara S, Fujishiro M, Saito H, Kumagai Y, Shichijo S, et al. Novel computer-assisted diagnosis system for endoscopic disease activity in patients with ulcerative colitis. Gastrointest Endosc. (2019) 89:416–21.e1. doi: 10.1016/j.gie.2018.10.020
13. Syed S, Al-Boni M, Khan MN, Sadiq K, Iqbal NT, Moskaluk CA, et al. Assessment of machine learning detection of environmental enteropathy and celiac disease in children. JAMA Netw Open. (2019) 2:e195822. doi: 10.1001/jamanetworkopen.2019.5822
14. Urban G, Tripathi P, Alkayali T, Mittal M, Jalali F, Karnes W, et al. Deep learning localizes and identifies polyps in real time with 96% accuracy in screening colonoscopy. Gastroenterology. (2018) 155:1069–78.e8. doi: 10.1053/j.gastro.2018.06.037
15. Vécsei A, Amann G, Hegenbart S, Liedlgruber M, Uhl A. Automated Marsh-like classification of celiac disease in children using local texture operators. Comput Biol Med. (2011) 41:313–25. doi: 10.1016/j.compbiomed.2011.03.009
16. Wimmer G, Vécsei A, Uhl A editors. CNN transfer learning for the automated diagnosis of celiac disease. 2016 Sixth International Conference on Image Processing Theory, Tools and Applications (IPTA). Oulu: IEEE (2016). doi: 10.1038/s41598-022-07199-z
17. Zhou T, Han G, Li BN, Lin Z, Ciaccio EJ, Green PH, et al. Quantitative analysis of patients with celiac disease by video capsule endoscopy: a deep learning method. Comput Biol Med. (2017) 85:1–6. doi: 10.1016/j.compbiomed.2017.03.031
18. Glissen Brown JR, Mansour NM, Wang P, Chuchuca MA, Minchenberg SB, Chandnani M, et al. Deep learning computer-aided polyp detection reduces adenoma miss rate: a united states multi-center randomized tandem colonoscopy study (CADeT-CS Trial). Clin Gastroenterol Hepatol. (2021) 20:1499–507.e4. doi: 10.1016/j.cgh.2021.09.009
19. Page MJ, McKenzie JE, Bossuyt PM, Boutron I, Hoffmann TC, Mulrow CD, et al. The PRISMA 2020 statement: an updated guideline for reporting systematic reviews. BMJ. (2021) 372:n71. doi: 10.1136/bmj.n71
20. Whiting PF, Rutjes AWS, Westwood ME, Mallett S, Deeks JJ, Reitsma JB, et al. QUADAS-2: a revised tool for the quality assessment of diagnostic accuracy studies. Ann Int Med. (2011) 155:529–36. doi: 10.7326/0003-4819-155-8-201110180-00009
21. Ho SY, Phua K, Wong L, Goh WWB. Extensions of the external validation for checking learned model interpretability and generalizability. Patterns. (2020) 1:100129. doi: 10.1016/j.patter.2020.100129
22. Barish M, Bolourani S, Lau LF, Shah S, Zanos TP. External validation demonstrates limited clinical utility of the interpretable mortality prediction model for patients with COVID-19. Nat Mach Intell. (2021) 3:25–7.
25. Byrne MF, Chapados N, Soudan F, Oertel C, Pérez ML, Kelly R, et al. Real-time differentiation of adenomatous and hyperplastic diminutive colorectal polyps during analysis of unaltered videos of standard colonoscopy using a deep learning model. Gut. (2019) 68:94–100. doi: 10.1136/gutjnl-2017-314547
26. Chen PJ, Lin MC, Lai MJ, Lin JC, Lu HHS, Tseng VS. Accurate classification of diminutive colorectal polyps using computer-aided analysis. Gastroenterology. (2018) 154:568–75. doi: 10.1053/j.gastro.2017.10.010
27. de Groof AJ, Struyvenberg MR, Fockens KN, van der Putten J, van der Sommen F, Boers TG, et al. Deep learning algorithm detection of Barrett’s neoplasia with high accuracy during live endoscopic procedures: a pilot study (with video). Gastrointest Endosc. (2020) 91:1242–50. doi: 10.1016/J.GIE.2019.12.048
28. Ding Z, Shi H, Zhang H, Meng L, Fan M, Han C, et al. Gastroenterologist-level identification of small-bowel diseases and normal variants by capsule endoscopy using a deep-learning model. Gastroenterology. (2019) 157:1044–54.e5. doi: 10.1053/J.GASTRO.2019.06.025
29. Figueiredo P, Figueiredo I, Pinto L, Kumar S, Tsai Y-H, Mamonov A. Polyp detection with computer-aided diagnosis in white light colonoscopy: comparison of three different methods. Endosc Int Open. (2019) 7:E209–15. doi: 10.1055/a-0808-4456
30. Guo L, Gong H, Wang Q, Zhang Q, Tong H, Li J, et al. Detection of multiple lesions of gastrointestinal tract for endoscopy using artificial intelligence model: a pilot study. Surg Endosc. (2020) 35:6532–8. doi: 10.1007/s00464-020-08150-x
31. Hwang Y, Lee HH, Park C, Tama BA, Kim JS, Cheung DY, et al. Improved classification and localization approach to small bowel capsule endoscopy using convolutional neural network. Dig Endosc. (2020) 33:598–607. doi: 10.1111/den.13787
32. Jin EH, Lee D, Bae JH, Kang HY, Kwak MS, Seo JY, et al. Improved accuracy in optical diagnosis of colorectal polyps using convolutional neural networks with visual explanations. Gastroenterology. (2020) 158:2169–79.e8. doi: 10.1053/j.gastro.2020.02.036
33. Kudo S, Misawa M, Mori Y, Hotta K, Ohtsuka K, Ikematsu H, et al. Artificial intelligence-assisted system improves endoscopic identification of colorectal neoplasms. Clin Gastroenterol Hepatol. (2020) 18:1874–81.e2. doi: 10.1016/J.CGH.2019.09.009
34. Lee JY, Jeong J, Song EM, Ha C, Lee HJ, Koo JE, et al. Real-time detection of colon polyps during colonoscopy using deep learning: systematic validation with four independent datasets. Sci Rep. (2020) 10:8379. doi: 10.1038/s41598-020-65387-1
35. Maslekar S, Gardiner AB, Monson JRT, Duthie GS. Artificial neural networks to predict presence of significant pathology in patients presenting to routine colorectal clinics. Colorectal Dis. (2010) 12:1254–9. doi: 10.1111/j.1463-1318.2009.02005.x
36. Namikawa K, Hirasawa T, Nakano K, Ikenoyama Y, Ishioka M, Shiroma S, et al. Artificial intelligence-based diagnostic system classifying gastric cancers and ulcers: comparison between the original and newly developed systems. Endoscopy. (2020) 52:1077–83. doi: 10.1055/a-1194-8771
37. Patel K, Li K, Tao K, Wang Q, Bansal A, Rastogi A, et al. A comparative study on polyp classification using convolutional neural networks. PLoS One. (2020) 15:e0236452. doi: 10.1371/journal.pone.0236452
38. Poon CCY, Jiang Y, Zhang R, Lo WWY, Cheung MSH, Yu R, et al. AI-doscopist: a real-time deep-learning-based algorithm for localising polyps in colonoscopy videos with edge computing devices. NPJ Digit Med. (2020) 3:73. doi: 10.1038/s41746-020-0281-z
39. Renner J, Phlipsen H, Haller B, Navarro-Avila F, Saint-Hill-Febles Y, Mateus D, et al. Optical classification of neoplastic colorectal polyps – a computer-assisted approach (the COACH study). Scand J Gastroenterol. (2018) 53:1100–6. doi: 10.1080/00365521.2018.1501092
40. Rodriguez-Diaz E, Baffy G, Lo WK, Mashimo H, Vidyarthi G, Mohapatra SS, et al. Real-time artificial intelligence–based histologic classification of colorectal polyps with augmented visualization. Gastrointest Endosc. (2021) 93:662–70. doi: 10.1016/J.GIE.2020.09.018
41. Saito H, Aoki T, Aoyama K, Kato Y, Tsuboi A, Yamada A, et al. Automatic detection and classification of protruding lesions in wireless capsule endoscopy images based on a deep convolutional neural network. Gastrointest Endosc. (2020) 92:144–51.e1. doi: 10.1016/J.GIE.2020.01.054
42. Shin Y, Balasingham I editors. Comparison of hand-craft feature based SVM and CNN based deep learning framework for automatic polyp classification. 2017 39th Annual International Conference of the IEEE Engineering in Medicine and Biology Society (EMBC). Jeju: IEEE (2017). doi: 10.1109/EMBC.2017.8037556
43. Shin Y, Balasingham I. Automatic polyp frame screening using patch based combined feature and dictionary learning. Comput Med Imaging Graph. (2018) 69:33–42. doi: 10.1016/J.COMPMEDIMAG.2018.08.001
44. Silva J, Histace A, Romain O, Dray X, Granado B, Pinna A editors. Towards real-time in situ polyp detection in WCE images using a boosting-based approach. 2013 35th Annual International Conference of the IEEE Engineering in Medicine and Biology Society (EMBC). Osaka: IEEE (2013). doi: 10.1109/EMBC.2013.6610847
45. Song EM, Park B, Ha CA, Hwang SW, Park SH, Yang DH, et al. Endoscopic diagnosis and treatment planning for colorectal polyps using a deep-learning model. Sci Rep. (2020) 10:30. doi: 10.1038/s41598-019-56697-0
46. Tajbakhsh N, Gurudu SR, Liang J editors. A comprehensive computer-aided polyp detection system for colonoscopy videos. Inform Proc Med Imaging. (2015) 24:327–38.
47. Tajbakhsh N, Gurudu SR, Liang J. Automated polyp detection in colonoscopy videos using shape and context information. IEEE Trans Med Imaging. (2016) 35:630–44. doi: 10.1109/TMI.2015.2487997
48. Taunk P, Atkinson CD, Lichtenstein D, Rodriguez-Diaz E, Singh SK. Computer-assisted assessment of colonic polyp histopathology using probe-based confocal laser endomicroscopy. Int J Colorectal Dis. (2019) 34:2043–51. doi: 10.1007/s00384-019-03406-y
49. Tenório JM, Hummel AD, Cohrs FM, Sdepanian VL, Pisa IT, De Fátima Marin H. Artificial intelligence techniques applied to the development of a decision–support system for diagnosing celiac disease. Int J Med Inform. (2011) 80:793–802. doi: 10.1016/J.IJMEDINF.2011.08.001
50. Viscaino M, Cheein FA editors. Machine learning for computer-aided polyp detection using wavelets and content-based image. 2019 41st Annual International Conference of the IEEE Engineering in Medicine and Biology Society (EMBC). Berlin: IEEE (2019). doi: 10.1109/EMBC.2019.8857831
51. Wang S, Xing Y, Zhang L, Gao H, Zhang H. A systematic evaluation and optimization of automatic detection of ulcers in wireless capsule endoscopy on a large dataset using deep convolutional neural networks. Phys Med Biol. (2019) 64:235014. doi: 10.1088/1361-6560/ab5086
52. Wang W, Tian J, Zhang C, Luo Y, Wang X, Li J. An improved deep learning approach and its applications on colonic polyp images detection. BMC Med Imaging. (2020) 20:83. doi: 10.1186/s12880-020-00482-3
53. Xia J, Xia T, Pan J, Gao F, Wang S, Qian Y-Y, et al. Use of artificial intelligence for detection of gastric lesions by magnetically controlled capsule endoscopy. Gastrointest Endosc. (2021) 93:133–9.e4. doi: 10.1016/j.gie.2020.05.027
54. Bagheri M, Mohrekesh M, Tehrani M, Najarian K, Karimi N, Samavi S, et al. Deep Neural Network based Polyp Segmentation in Colonoscopy Images using a Combination of Color Spaces. Annual International Conference of the IEEE Engineering in Medicine and Biology Society. Berlin: IEEE (2019). doi: 10.1109/EMBC.2019.8856793
55. Becq A, Chandnani M, Bharadwaj S, Baran B, Ernest-Suarez K, Gabr M, et al. Effectiveness of a deep-learning polyp detection system in prospectively collected colonoscopy videos with variable bowel preparation quality. J Clin Gastroenterol. (2020) 54:554–7. doi: 10.1097/MCG.0000000000001272
56. Blanes-Vidal V, Baatrup G, Nadimi ES. Addressing priority challenges in the detection and assessment of colorectal polyps from capsule endoscopy and colonoscopy in colorectal cancer screening using machine learning. Acta Oncol. (2019) 58:S29–36. doi: 10.1080/0284186X.2019.1584404
57. Charisis V, Hadjileontiadis LJ, Liatsos CN, Mavrogiannis CC, Sergiadis GD. Abnormal pattern detection in Wireless Capsule Endoscopy images using nonlinear analysis in RGB color space. Annual International Conference of the IEEE Engineering in Medicine and Biology. Buenos Aires: IEEE (2010). p. 3674–7. doi: 10.1109/IEMBS.2010.5627648
58. Charisis VS, Hadjileontiadis LJ. Potential of hybrid adaptive filtering in inflammatory lesion detection from capsule endoscopy images. World J Gastroenterol. (2016) 22:8641–57. doi: 10.3748/wjg.v22.i39.8641
59. Cho M, Kim JH, Kong HJ, Hong KS, Kim S. A novel summary report of colonoscopy: timeline visualization providing meaningful colonoscopy video information. Int J Colorectal Dis. (2018) 33:549–59. doi: 10.1007/s00384-018-2980-3
60. Fernández-Esparrach G, Bernal J, López-Cerón M, Córdova H, Sánchez-Montes C, Rodríguez de Miguel C, et al. Exploring the clinical potential of an automatic colonic polyp detection method based on the creation of energy maps. Endoscopy. (2016) 48:837–42. doi: 10.1055/s-0042-108434
61. Ganz M, Yang X, Slabaugh G. Automatic segmentation of polyps in colonoscopic narrow-band imaging data. IEEE Trans Biomed Eng. (2012) 59:2144–51. doi: 10.1109/TBME.2012.2195314
62. Itoh H, Roth H, Oda M, Misawa M, Mori Y, Kudo S-E, et al. Stable polyp-scene classification via subsampling and residual learning from an imbalanced large dataset. Healthcare Technol Lett. (2019) 6:237–42. doi: 10.1049/htl.2019.0079
63. Klang E, Barash Y, Yehuda Margalit R, Soffer S, Shimon O, Albshesh A, et al. Deep learning algorithms for automated detection of Crohn’s disease ulcers by video capsule endoscopy. Gastrointest Endosc. (2020) 91:606–13.e2.
64. Li BN, Wang X, Wang R, Zhou T, Gao R, Ciaccio EJ, et al. Celiac disease detection from videocapsule endoscopy images using strip principal component analysis. IEEE/ACM Trans Comput Biol Bioinformatics. (2019) 18:1396–404. doi: 10.1109/TCBB.2019.2953701
65. Mori Y, Kudo S-E, Misawa M, Saito Y, Ikematsu H, Hotta K, et al. Real-time use of artificial intelligence in identification of diminutive polyps during colonoscopy. Ann Intern Med. (2018) 169:357–66.
66. Otani K, Nakada A, Kurose Y, Niikura R, Yamada A, Aoki T, et al. Automatic detection of different types of small-bowel lesions on capsule endoscopy images using a newly developed deep convolutional neural network. Endoscopy. (2020) 52:786–91. doi: 10.1055/a-1167-8157
67. Owais M, Arsalan M, Choi J, Mahmood T, Park KR. Artificial intelligence-based classification of multiple gastrointestinal diseases using endoscopy videos for clinical diagnosis. J Clin Med. (2019) 8:986. doi: 10.3390/jcm8070986
68. Owais M, Arsalan M, Mahmood T, Kang Jin K, Park Kang R. Automated diagnosis of various gastrointestinal lesions using a deep learning–based classification and retrieval framework with a large endoscopic database: model development and validation. J Med Internet Res. (2020) 22:e18563. doi: 10.2196/18563
69. Ozawa T, Ishihara S, Fujishiro M, Kumagai Y, Shichijo S, Tada T. Automated endoscopic detection and classification of colorectal polyps using convolutional neural networks. Therap Adv Gastroenterol. (2020) 13:1756284820910659. doi: 10.1177/1756284820910659
70. Pu LZCT, Maicas G, Tian Y, Yamamura T, Nakamura M, Suzuki H, et al. Computer-aided diagnosis for characterization of colorectal lesions: comprehensive software that includes differentiation of serrated lesions. Gastrointest Endosc. (2020) 92:891–9. doi: 10.1016/J.GIE.2020.02.042
71. Qadir HA, Balasingham I, Solhusvik J, Bergsland J, Aabakken L, Shin Y. Improving automatic polyp detection using CNN by exploiting temporal dependency in colonoscopy video. IEEE J Biomed Health Inform. (2020) 24:180–93. doi: 10.1109/JBHI.2019.2907434
72. Ševo I, Avramoviæ A, Balasingham I, Elle OJ, Bergsland J, Aabakken L. Edge density based automatic detection of inflammation in colonoscopy videos. Comput Biol Med. (2016) 72:138–50. doi: 10.1016/J.COMPBIOMED.2016.03.017
73. Shi C, Xue Y, Jiang C, Tian H, Liu B. Gastroscopic panoramic view: application to automatic polyps detection under gastroscopy. Comput Math Methods Med. (2019) 2019:4393124. doi: 10.1155/2019/4393124
74. Tischendorf JJW, Gross S, Winograd R, Hecker H, Auer R, Behrens A, et al. Computer-aided classification of colorectal polyps based on vascular patterns: a pilot study. Endoscopy. (2010) 42:203–7. doi: 10.1055/s-0029-1243861
75. Vécsei A, Fuhrmann T, Liedlgruber M, Brunauer L, Payer H, Uhl A. Automated classification of duodenal imagery in celiac disease using evolved Fourier feature vectors. Comput Methods Prog Biomed. (2009) 95:S68–78. doi: 10.1016/j.cmpb.2009.02.017
76. Wang P, Xiao X, Glissen Brown JR, Berzin TM, Tu M, Xiong F, et al. Development and validation of a deep-learning algorithm for the detection of polyps during colonoscopy. Nat Biomed Eng. (2018) 2:741–8. doi: 10.1038/s41551-018-0301-3
77. Wang S, Xing Y, Zhang L, Gao H, Zhang H. Deep convolutional neural network for ulcer recognition in wireless capsule endoscopy: experimental feasibility and optimization. Comput Math Methods Med. (2019) 2019:7546215. doi: 10.1155/2019/7546215
78. Wang X, Qian H, Ciaccio EJ, Lewis SK, Bhagat G, Green PH, et al. Celiac disease diagnosis from videocapsule endoscopy images with residual learning and deep feature extraction. Comput Methods Prog Biomed. (2020) 187:105236. doi: 10.1016/J.CMPB.2019.105236
79. Yuan Y, Meng MQH. Deep learning for polyp recognition in wireless capsule endoscopy images. Med Phys. (2017) 44:1379–89. doi: 10.1002/mp.12147
80. Zachariah R, Samarasena J, Luba D, Duh E, Dao T, Requa J, et al. Prediction of polyp pathology using convolutional neural networks achieves “Resect and discard” thresholds. Off J Am Coll Gastroenterol. (2020) 115:138–44. doi: 10.14309/ajg.0000000000000429
81. Zhang R, Zheng Y, Mak TWC, Yu R, Wong SH, Lau JYW, et al. Automatic detection and classification of colorectal polyps by transferring low-level CNN features from nonmedical domain. IEEE J Biomed Health Inform. (2017) 21:41–7. doi: 10.1109/JBHI.2016.2635662
82. Zhang X, Chen F, Yu T, An J, Huang Z, Liu J, et al. Real-time gastric polyp detection using convolutional neural networks. PLoS One. (2019) 14:e0214133. doi: 10.1371/journal.pone.0214133
83. Zhao Q, Dassopoulos T, Mullin G, Hager G, Meng MQH, Kumar R editors. Towards integrating temporal information in capsule endoscopy image analysis. 2011 Annual International Conference of the IEEE Engineering in Medicine and Biology Society. Boston, MA: IEEE (2011). doi: 10.1109/IEMBS.2011.6091634
84. Zhao Q, Mullin GE, Meng MQH, Dassopoulos T, Kumar R. A general framework for wireless capsule endoscopy study synopsis. Comput Med Imaging Graph. (2015) 41:108–16. doi: 10.1016/J.COMPMEDIMAG.2014.05.011
85. Zheng W, Zhang X, Kim JJ, Zhu X, Ye G, Ye B, et al. High accuracy of convolutional neural network for evaluation of helicobacter pylori infection based on endoscopic images: preliminary experience. Clin Transl Gastroenterol. (2019) 10:e00109. doi: 10.14309/ctg.0000000000000109
86. Fu JJC, Yu YW, Lin HM, Chai JW, Chen CCC. Feature extraction and pattern classification of colorectal polyps in colonoscopic imaging. Comput Med Imaging Graph. (2014) 38:267–75. doi: 10.1016/J.COMPMEDIMAG.2013.12.009
87. Gross S, Trautwein C, Behrens A, Winograd R, Palm S, Lutz HH, et al. Computer-based classification of small colorectal polyps by using narrow-band imaging with optical magnification. Gastrointest Endosc. (2011) 74:1354–9. doi: 10.1016/J.GIE.2011.08.001
88. Huang C-R, Chen Y-T, Chen W-Y, Cheng H-C, Sheu B-S. Gastroesophageal reflux disease diagnosis using hierarchical heterogeneous descriptor fusion support vector machine. IEEE Trans Biomed Eng. (2016) 63:588–99. doi: 10.1109/TBME.2015.2466460
89. Struyvenberg MR, de Groof AJ, van der Putten J, van der Sommen F, Baldaque-Silva F, Omae M, et al. A computer-assisted algorithm for narrow-band imaging-based tissue characterization in Barrett’s esophagus. Gastrointest Endosc. (2021) 93:89–98. doi: 10.1016/J.GIE.2020.05.050
90. Swager AF, van der Sommen F, Klomp SR, Zinger S, Meijer SL, Schoon EJ, et al. Computer-aided detection of early Barrett’s neoplasia using volumetric laser endomicroscopy. Gastrointest Endosc. (2017) 86:839–46. doi: 10.1016/J.GIE.2017.03.011
91. Yang J, Chang L, Li S, He X, Zhu T. WCE polyp detection based on novel feature descriptor with normalized variance locality-constrained linear coding. Int J Comput Assist Radiol Surg. (2020) 15:1291–302. doi: 10.1007/s11548-020-02190-3
92. Amisha, Malik P, Pathania M, Rathaur VK. Overview of artificial intelligence in medicine. J Family Med Prim Care. (2019) 8:2328–31. doi: 10.4103/jfmpc.jfmpc_440_19
93. Johnson KW, Torres Soto J, Glicksberg BS, Shameer K, Miotto R, Ali M, et al. Artificial intelligence in cardiology. J Am Coll Cardiol. (2018) 71:2668–79. doi: 10.1016/j.jacc.2018.03.521
94. Pesapane F, Codari M, Sardanelli F. Artificial intelligence in medical imaging: threat or opportunity? radiologists again at the forefront of innovation in medicine. Eur Radiol Exp. (2018) 2:35. doi: 10.1186/s41747-018-0061-6
95. Hoodbhoy Z, Masroor Jeelani S, Aziz A, Habib MI, Iqbal B, Akmal W, et al. Machine learning for child and adolescent health: a systematic review. Pediatrics. (2021) 147:e2020011833. doi: 10.1542/peds.2020-011833
96. Yang YJ, Bang CS. Application of artificial intelligence in gastroenterology. World J Gastroenterol. (2019) 25:1666–83. doi: 10.3748/wjg.v25.i14.1666
97. Zhang SM, Wang YJ, Zhang ST. Accuracy of artificial intelligence-assisted detection of esophageal cancer and neoplasms on endoscopic images: a systematic review and meta-analysis. J Dig Dis. (2021) 22:318–28. doi: 10.1111/1751-2980.12992
98. Lui TK, Tsui VW, Leung WK. Accuracy of artificial intelligence–assisted detection of upper GI lesions: a systematic review and meta-analysis. Gastrointest Endosc. (2020) 92:821–30.e9. doi: 10.1016/j.gie.2020.06.034
99. Xie X, Ren K, Zhou Z, Dang C, Zhang H. The global, regional and national burden of peptic ulcer disease from 1990 to 2019: a population-based study. BMC Gastroenterol. (2022) 22:58. doi: 10.1186/s12876-022-02130-2
100. Schwalbe N, Wahl B. Artificial intelligence and the future of global health. Lancet. (2020) 395:1579–86. doi: 10.1016/s0140-6736(20)30226-9
101. USAID. Artificial Intelligence in Global Health. (2019). Available online at: https://www.usaid.gov/cii/ai-in-global-health. (accessed July 7, 2022).
102. Hoodbhoy Z, Jiwani U, Sattar S, Salam R, Hasan B, Das JK. Diagnostic accuracy of machine learning models to identify congenital heart disease: a meta-analysis. Front Artif Intell. (2021) 4:708365. doi: 10.3389/frai.2021.708365
103. Ben-Israel D, Jacobs WB, Casha S, Lang S, Ryu WHA, de Lotbiniere-Bassett M, et al. The impact of machine learning on patient care: a systematic review. Artif Intell Med. (2020) 103:101785. doi: 10.1016/j.artmed.2019.101785
104. Brownlee, J. Supervised and Unsupervised Machine Learning Algorithms. Machine Learning Mastery. (2016) 16. Available Online at: https://machinelearningmastery.com/supervised-and-unsupervised-machine-learning-algorithms/
Keywords: artificial intelligence, systematic review, gastroenterology, diagnostic accuracy, pathologies
Citation: Parkash O, Siddiqui ATS, Jiwani U, Rind F, Padhani ZA, Rizvi A, Hoodbhoy Z and Das JK (2022) Diagnostic accuracy of artificial intelligence for detecting gastrointestinal luminal pathologies: A systematic review and meta-analysis. Front. Med. 9:1018937. doi: 10.3389/fmed.2022.1018937
Received: 15 August 2022; Accepted: 03 October 2022;
Published: 04 November 2022.
Edited by:
Adina Turcu-Stiolica, University of Medicine and Pharmacy of Craiova, RomaniaReviewed by:
Mihaela-Simona Subtirelu, University of Medicine and Pharmacy of Craiova, RomaniaNicole Rübsamen, Faculty of Medicine, University of Münster, Germany
Copyright © 2022 Parkash, Siddiqui, Jiwani, Rind, Padhani, Rizvi, Hoodbhoy and Das. This is an open-access article distributed under the terms of the Creative Commons Attribution License (CC BY). The use, distribution or reproduction in other forums is permitted, provided the original author(s) and the copyright owner(s) are credited and that the original publication in this journal is cited, in accordance with accepted academic practice. No use, distribution or reproduction is permitted which does not comply with these terms.
*Correspondence: Jai K. Das, amFpLmRhc0Bha3UuZWR1