- 1School of Population Medicine and Public Health, Chinese Academy of Medical Sciences and Peking Union Medical College, Beijing, China
- 2Faculty of Health and Medicine, Division of Health Research, Lancaster University, Lancaster, United Kingdom
- 3School of Public Health, Dalian Medical University, Dalian, China
- 4The First Affiliated Hospital, Dalian Medical University, Dalian, China
- 5Global Health Research Center, Dalian Medical University, Dalian, China
Background: Artificial intelligence (AI) needs to be accepted and understood by physicians and medical students, but few have systematically assessed their attitudes. We investigated clinical AI acceptance among physicians and medical students around the world to provide implementation guidance.
Materials and methods: We conducted a two-stage study, involving a foundational systematic review of physician and medical student acceptance of clinical AI. This enabled us to design a suitable web-based questionnaire which was then distributed among practitioners and trainees around the world.
Results: Sixty studies were included in this systematic review, and 758 respondents from 39 countries completed the online questionnaire. Five (62.50%) of eight studies reported 65% or higher awareness regarding the application of clinical AI. Although, only 10–30% had actually used AI and 26 (74.28%) of 35 studies suggested there was a lack of AI knowledge. Our questionnaire uncovered 38% awareness rate and 20% utility rate of clinical AI, although 53% lacked basic knowledge of clinical AI. Forty-five studies mentioned attitudes toward clinical AI, and over 60% from 38 (84.44%) studies were positive about AI, although they were also concerned about the potential for unpredictable, incorrect results. Seventy-seven percent were optimistic about the prospect of clinical AI. The support rate for the statement that AI could replace physicians ranged from 6 to 78% across 40 studies which mentioned this topic. Five studies recommended that efforts should be made to increase collaboration. Our questionnaire showed 68% disagreed that AI would become a surrogate physician, but believed it should assist in clinical decision-making. Participants with different identities, experience and from different countries hold similar but subtly different attitudes.
Conclusion: Most physicians and medical students appear aware of the increasing application of clinical AI, but lack practical experience and related knowledge. Overall, participants have positive but reserved attitudes about AI. In spite of the mixed opinions around clinical AI becoming a surrogate physician, there was a consensus that collaborations between the two should be strengthened. Further education should be conducted to alleviate anxieties associated with change and adopting new technologies.
Background
Artificial intelligence (AI) refers to machine-based systems which simulate problem-solving and decision-making processes involved in human thought. The success of Google’s AlphaGo program in 2016 propelled Deep Learning (DL) led AI into a new era, and stimulated interest in the development and implementation of AI systems in many fields, including healthcare. Between 1997 and 2015, fewer than 30 AI-enabled medical devices were approved by the U.S. Food and Drug Administration (FDA), however this number rose to more than 350 by mid-2021 (1). Also, there is an increasing number of studies which have found that DL algorithms are at least equivalent to clinicians in terms of diagnostic performance (2–4). This means that DL-enabled AI has the potential to provide a number of advantages in clinical care. For example, DL-enabled AI could be used to address current dilemmas such as the workforce shortage and could ensure there is consistency by reducing variability in medical practice and by standardizing the quality of care (5). Some have suggested that the increasing use of AI will fundamentally change the nature of healthcare provision and clinical practice (6–8). However, this gradual transition could also cause concerns within the medical profession because adopting new technologies requires changes to medical practice.
At present, the relatively limited use of clinical AI partly reflects a reluctance to change as well as potential misperceptions and negative attitudes held by physicians (9, 10). Of course, physicians are likely to be the “earliest” adopters and inevitably become direct AI operators. Therefore, physicians play a pivotal role in the acceptance and implementation of clinical AI, and so their views need to be explored and understood. AI-driven changes will also inevitably affect medical students, the future generations of doctors. Therefore, research should be designed to understand their sentiments in order to develop effective education and health policies. There is a growing evidence-base around the attitudes of physicians and medical students toward AI. However, there are distinctions between countries and cultures and the majority of this research has been conducted in developed, western countries (11, 12). While there has also been a couple of systematic reviews on this topic (9, 13), we can still say that this provides only a narrow understanding. There is a need to understand the views of medical students and physicians in developing countries in Asia and Africa. Therefore, we conducted a two-stage study, involving a foundational systematic review which enabled us to design a suitable questionnaire that was then distributed among physicians and medical students around worldwide. This approach was implemented to obtain more comprehensive data and to discuss contrasting ideas, in order to gain insights to improve the uptake and use of clinical AI.
Materials and methods
We initially conducted a systematic review to understand what is already known about physicians’ and medical students’ perspectives on clinical AI. The initial systematic review followed rigorous procedures set out in the Preferred Reporting Items for PRISMA (Preferred reporting items for systematic reviews and meta-analysis) statement (14). The main themes, identified through the systematic review, were used to develop a questionnaire, which was then distributed through a network of associates.
STROBE checklist was provided for this cross-sectional study (15). Participation in the questionnaire was voluntary and informed consent was obtained before completing the questionnaire. The research ethics committee of Chinese Academy of Medical Sciences and Peking Union Medical College approved this study (IEC-2022-022).
Systematic review
Clinical AI, during the systematic review stage, was defined as “AI designed to automate intelligent behaviors in clinical settings for the purpose of supporting physician-mediated care-related tasks”. These clinical AI technologies excluded consumer utilized products such as wearable devices. PUBMED, EMBASE, IEEE Xplore and Web of Science were systematically searched for published research. Any original study appraising physician or medical student acceptance of clinical AI, published in English from January 1st 2017 to March 6th 2022, was initially included. Conference abstracts and comments presenting conclusions without numerical data were excluded. Search strategies are listed in the Supplementary Material 1.
Bibliographic data obtained were loaded into Endnote (version 20) and duplicates were removed. Authors BZ and ZC independently reviewed titles and abstracts to identify pertinent research which met the established inclusion criteria. Full-text assessment was conducted for inclusion. BZ and ZC independently extracted data from each eligible study using a pre-designed template. Inconsistencies were resolved through discussion with MC.
Questionnaire survey
A web-based questionnaire was generated based on the findings of the systematic review under the guidance of two experts in clinical AI. The draft questionnaire was then pre-tested across a sample of 110 students, and two participants were interviewed about their understanding of each question and about any difficulties met while completing the survey. The questionnaire was adjusted according to feedback from the pilot study (Supplementary Material 2).
The questionnaire was constructed around three main elements. The first section focused on respondent characteristics and practical experiences of clinical AI. The second included 13 statements to assess respondent’s views of clinical AI. These included aspects such as awareness and knowledge, acceptability, as well as AI as surrogate physicians. Respondents were asked to indicate their level of agreement with statements using a five-point Likert scale. In this instance, one was understood as strong disagreement while five was considered to be strong agreement with the statement. In the third section, respondents were asked to suggest factors which they feel are associated with intentionality, as well as around the perceived relationship between physicians and clinical AI. Section three was also designed to gain insights into the perceived challenges involved in the development and implementation of clinical AI. The online questionnaire was distributed among physicians and medical students through our professional network in March 2022.
Statistical analysis
Continuous variables are presented as means with corresponding standard deviations. Categorical variables are described using frequencies and percentages. Differences between physicians and medical students in clinical AI practice was compared using a standard Chi-square test. Comparisons of the response distribution on 13 statements across subgroups were performed by Mann–Whitney U test.
For descriptive statistics categories “strongly disagree” and “disagree” were summarized as disagreement while “agree” and “strongly agree” were summarized as agreement. Correlations between demographics and a willingness to adopt clinical AI were assessed using multivariable logistic regression, in physicians and medical students separately. Under statistical analysis, the “willingness to use clinical AI” was dichotomized according to having responded “strongly agree or agree” as opposed to “neutral or disagree or strongly disagree” for statement “I am willing to use clinical AI if needed”. All statistical analyses were performed using R (version 4.1.0). A p value <0.05 was established as the threshold for statistical significance.
Results
Description of included studies and respondent characteristics
Figure 1 provides the Systematic reviews and Meta-Analyses (PRISMA) flow diagram of this systematic review. Characteristics and main findings of the included studies have been summarized in Table 1 and Supplementary Table 1. Of the 60 included studies, there were 47 (78%) quantitative studies, 7 (12%) qualitative studies, and 6 (10%) mixed methods studies. All studies were published between 2019 and 2022. In the study population, 41 (68%) studies recruited physicians, 13 (22%) studies surveyed medical students, and 6 (10%) studies included both physicians and medical students. Regarding the type of AI being studied, 20 (33%) studies assessed AI in radiology, 13 (22%) assessed AI that was broadly defined, 9 (15%) assessed AI-based decision support system in clinic, 5 (8%) for AI in dermatology, 3 (5%) for AI in gastroenterology, 2 (3%) for AI in ophthalmology, and 2 (3%) for AI in psychiatry, etc. 35 (58%) studies were conducted in high-income countries, 6 (10%) were conducted in upper-middle income countries, 4 (7%) in lower-middle income countries, and 13 (21%) were conducted worldwide or regionally. The geographical distribution of included studies is presented in Figure 2.
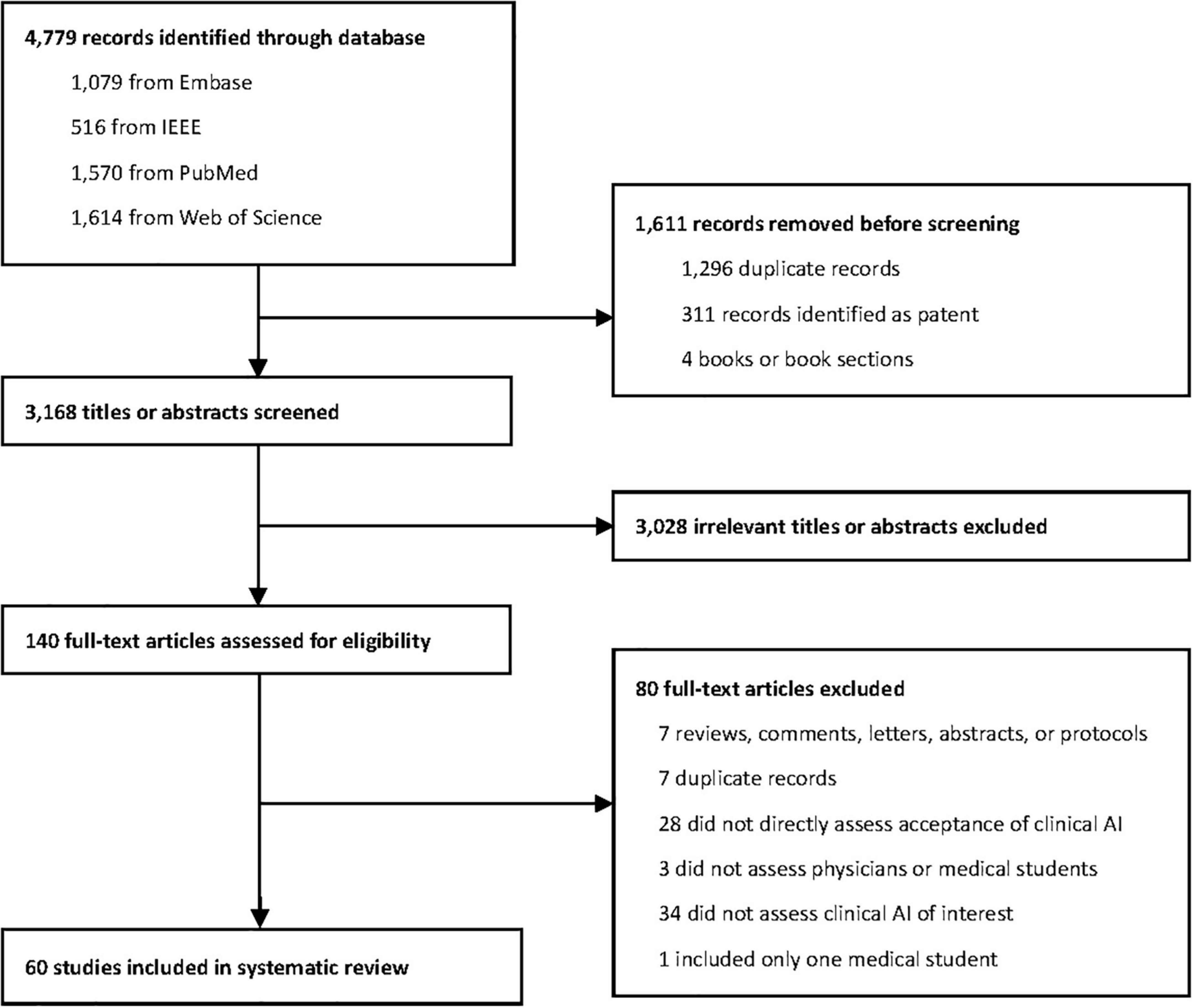
Figure 1. PRISMA (Preferred reporting items for systematic reviews and meta-analysis) systematic review flow diagram. Displayed is the PRISMA flow of each article selection process.
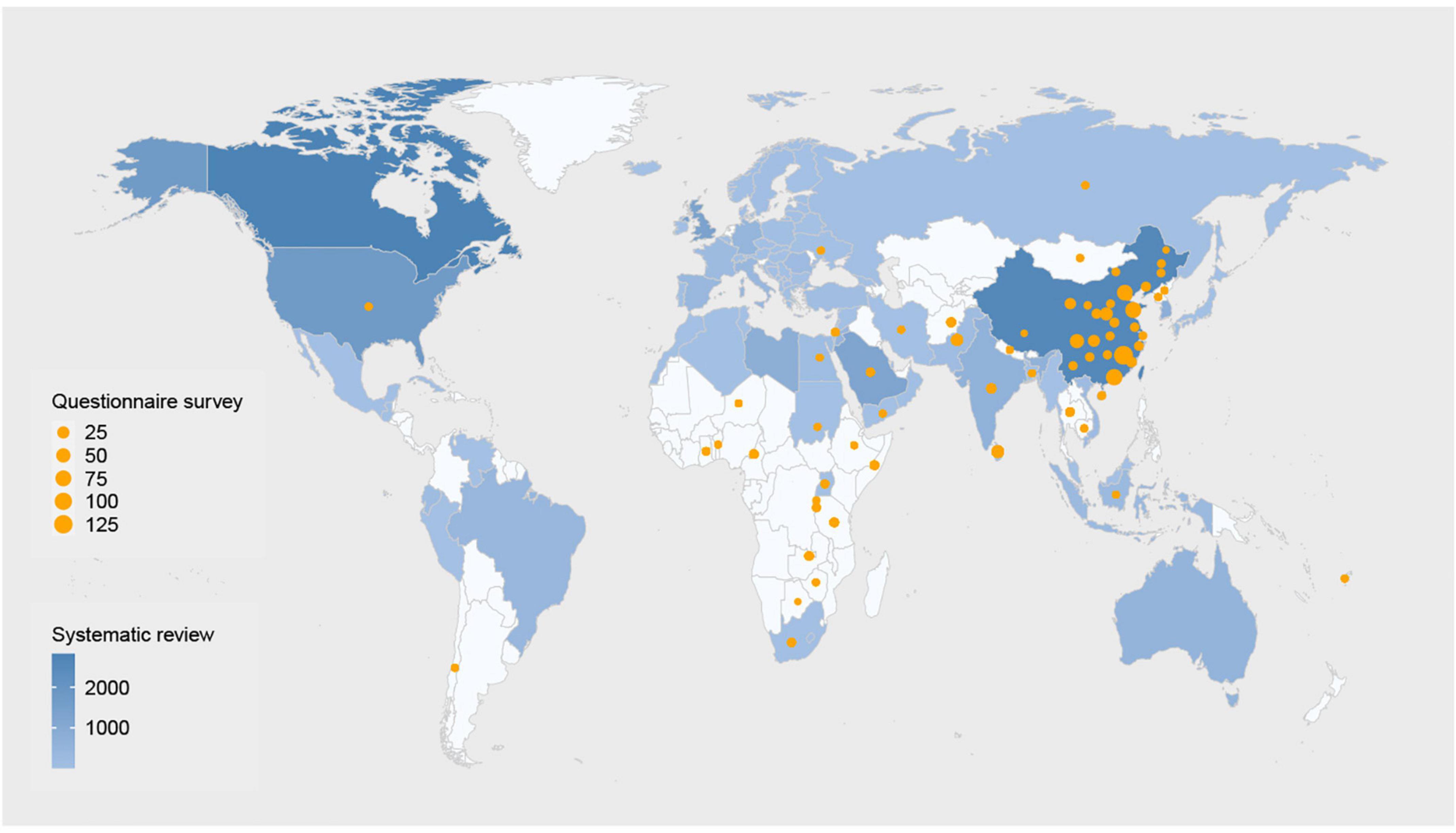
Figure 2. Geographic distribution of participants in the systematic review and the survey. The blue indicates the number of participants of studies included in the systematic review. The darker the color, the more participants. The orange dots indicate the number of participants in our questionnaire survey. The larger the dots, the more participants. Studies without providing specific locations are not shown in the figure. Please see Table 1 for detailed number and locations of participants.
Of the 818 individuals who clicked on the link to our questionnaire, 13 did not give their consent to participate in the survey. Additionally, 47 responders were removed from further analysis because they did not meet the requirements of our target population or because they provided an inappropriate answer to the quality control question. Finally, 758 individuals from 39 countries completed the survey, of whom 96 (12.66%) were from low- and lower-middle-income countries. Geographic distribution of responders has also been provided in Figure 2. Table 2 provides details around the characteristics of our responder sample. The average age of respondents was 30.63 years. 532 (70.18%) respondents were women. 344 (45.38%) were practising physicians and the remaining 414 (54.62%) were medical students.
Understanding and experience of clinical artificial intelligence
According to the systematic review, 5 (62.50%) out of eight included studies reported 65% or higher awareness of the wide application of clinical AI among physicians and medical students (16–20). Between 10–30% of all respondents had actually used clinical AI systems in their practice (18, 19, 21–27). This finding was consistent with the findings of our survey, with that only 148 (19.53%) participants having direct experience of clinical AI. We found that physicians were more likely to have used clinical AI than medical students (27.62% versus 12.80%, p < 0.001). Of those who had used AI systems, 103 (69.59%) indicated that they had encountered errors made by AI. 69 (46.62%) reported patient supportive attitude to clinical AI, but 30 (20.27%) were unclear about patient views. Detailed information is provided in Table 3.
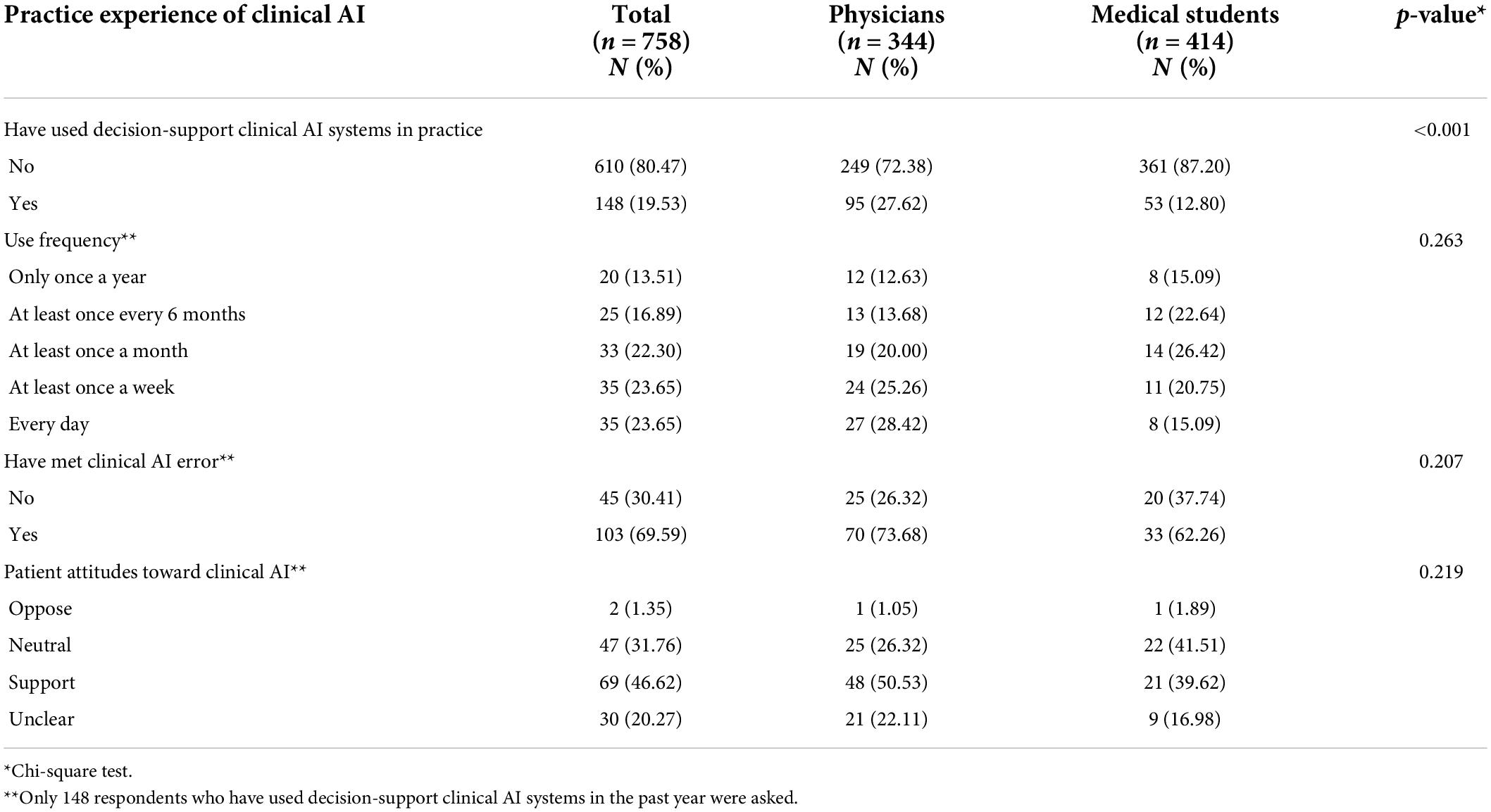
Table 3. Respondent practical experience of clinical artificial intelligence (AI) over the past year.
Thirty-five included studies mentioned the knowledge level of physicians or medical students on clinical AI, of which 26 (74.29%) showed that participants lacked basic knowledge (16–19, 23, 25, 26, 28–46). Many physicians felt that the current training and educational tools, provided by their departments, were inadequate (47, 48). Medical students also felt that they mainly heard about AI from media and colleagues, but received minimal training from their schools (18, 30). Accordingly, 15 studies suggested an urgent need to integrate AI into residency programs or school curricula (17–19, 21, 29–33, 38, 41, 45, 49–51). Our questionnaire appears to confirm this situation with few respondents having good knowledge of AI (13% agreement). Our respondents also expressed a high willingness to learn (77% agreement) as well as a demand for relevant training to be provided by hospitals or schools (78% agreement). Please see Figure 3 for further details.
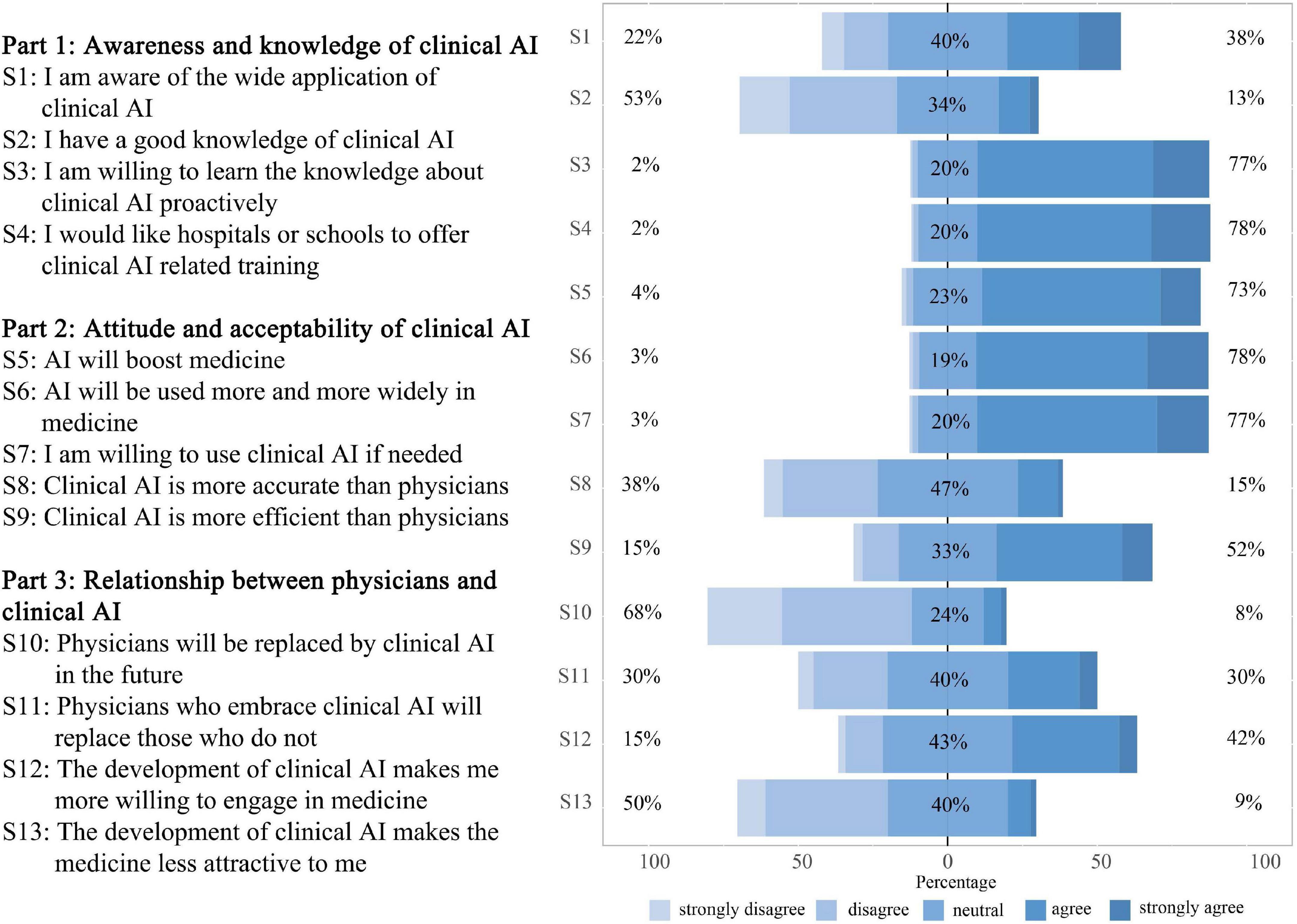
Figure 3. Respondent perspectives toward clinical artificial intelligence (AI). 13 statements were set to assess respondent perspectives toward clinical AI from three dimensions. Statement 1 to 4 assessed respondent awareness and knowledge of clinical AI. Statement 5 to 9 assessed attitude and acceptability of clinical AI. Statement 10 to 13 assessed respondent perception of the relationship between physicians and clinical AI.
Attitude and acceptability of clinical artificial intelligence
Forty-five included studies mentioned the views of physicians and medical students on clinical AI, and more than 60% of the respondents in 38 (84.44%) studies had an optimistic outlook regarding it (11, 12, 17–20, 22–26, 29, 30, 32, 33, 35, 36, 38–41, 45–61). For example, 75% of 487 pathologists from 59 countries were enthusiastic about the progress of AI (11); 77% of 1271 dermatologists from 92 countries agreed that AI would improve dermatologic practice (19). Similar positive opinions also existed among radiologists (22, 23, 25, 29, 33, 39, 47, 48, 53, 55, 61), gastroenterologists (24, 35), general practitioners (28, 62), psychiatrists (59, 63), ophthalmologists (23, 50). Additionally, in 14 studies reporting use intentionality, more than 60% respondents in 10 (71.43%) studies were willing to incorporate AI into their clinical practice (17, 21, 26, 34, 36, 44, 49, 55, 56, 61). The perceived benefits of AI included promoting workflow efficiency, quality assurance, improving standardization in the interpretation of results, as well as liberating doctors from mundane tasks and providing more time to expand their medical knowledge and focus on interacting with patients (11, 22, 35, 50, 64). Participants in our survey were also optimistic about the prospect of clinical AI and showed a high intention of use, with 78% in agreement that “AI will be used more and more widely in medicine” and 77% agreed that they are “willing to use clinical AI if needed” (Figure 3).
Although participants in several studies, included in the systematic review, believed that AI diagnostic performance was comparable and even superior to human doctors (3, 37, 46, 52), many respondents expressed a lack of trust in clinical AI and preferred results checked by human clinicians, and voiced concerns about the unpredictability of results and errors related to clinical AI (11, 33, 45, 48). Other concerns mentioned included operator dependence and increased procedural time caused by clinical AI, poor performance of AI in unexpected situations, and its lack of empathy or communication (20, 46, 62). In our questionnaire, few agreed that AI is more accurate than physicians (15% agreement), but these objectors seemed to be more confident in AI’s efficiency with 52% agreeing that “clinical AI is more efficient than physicians” (Figure 3).
Four studies used structural equation modeling to identify determinants of adoption intention for clinical AI among healthcare providers and medical students (38, 65–67). Perceived usefulness, the experience of using mHealth, subjective norms, and social influence had a positive effect on adoption intention, while perceived risk had the opposite effect. In our questionnaire, accuracy, ease of use, and efficiency were the top three perceived factors affecting respondent willingness to use clinical AI, with more than 70% considering these elements. Cost-effectiveness and interpretability followed, with more than 60% voicing their concerns (Figure 4A).
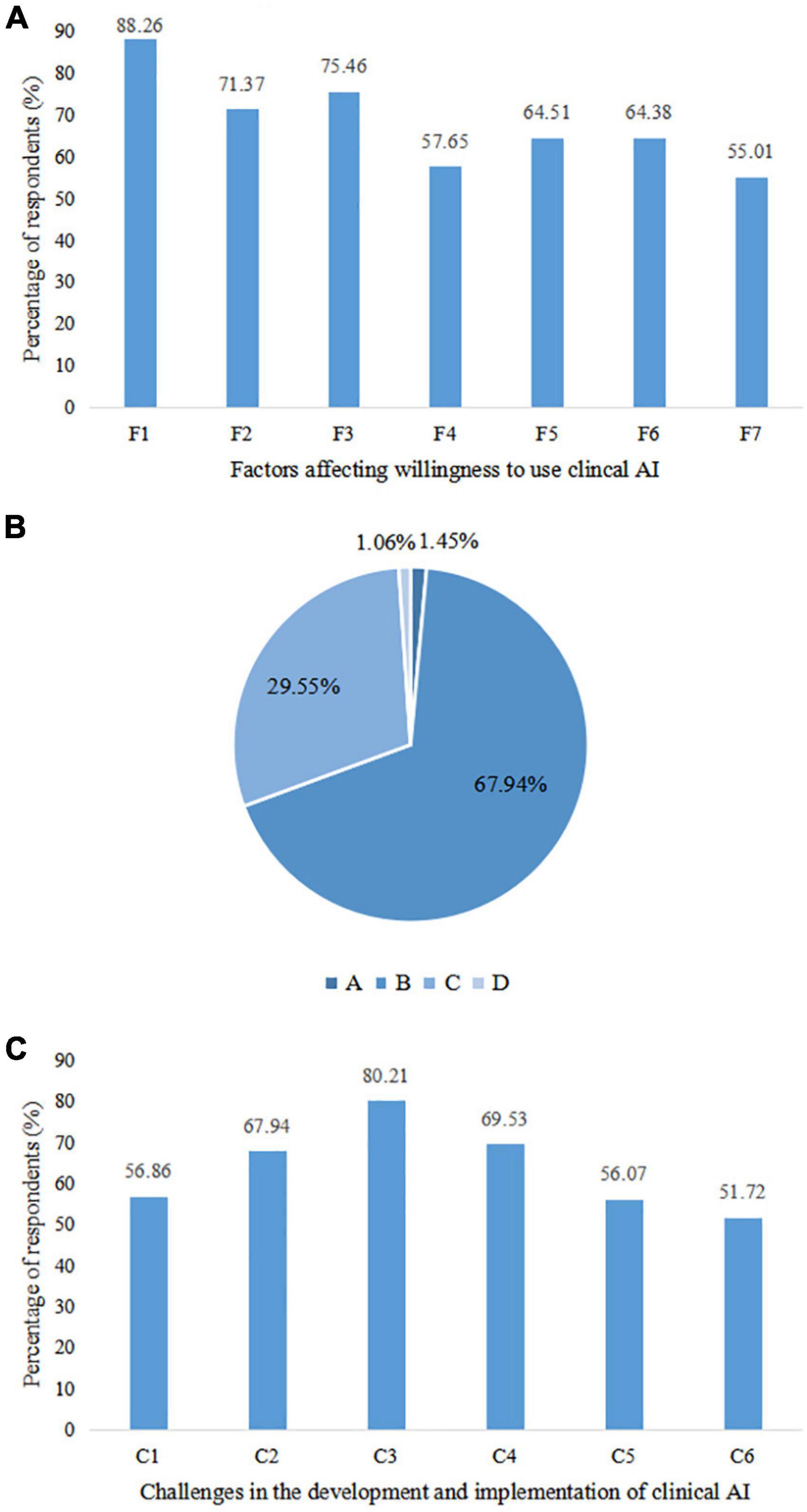
Figure 4. Factors related to use willingness, perceived relationship between physicians and artificial intelligence (AI), and challenges faced by clinical artificial intelligence (AI). (A) Factors associated with willingness to use clinical AI. F1: Accuracy; F2: Efficiency; F3: Ease of use; F4: Widely adopted; F5: Cost-effectiveness; F6: Interpretability; F7: Privacy protection capability. (B) Perceived relationship between physicians and clinical AI. A: Physicians don’t need to use clinical AI; B: Physicians lead the diagnosis and treatment process while clinical AI only plays an auxiliary role; C: Clinical AI completes the diagnosis and treatment process independently under the supervision and optimization of physicians; D: Clinical AI completely replaces physicians for diagnosis and treatment. (C) Challenges to be overcome in the development and implementation of clinical AI. C1: Inadequate algorithms and computational power of clinical AI; C2: Lack of high-quality data for clinical AI training; C3: Lack of inter-disciplinary talents with both medical and AI knowledge; C4: Lack of regulatory standards; C5: Difficulties in integrating clinical AI with existing medical process; C6: Insufficient understanding and acceptance of clinical AI among physicians and medical students.
Relationship between physicians and clinical artificial intelligence
Forty included studies mentioned potentially replacing physicians and changes in employment market caused by clinical AI. The support rate for the statement that AI could replace human physicians ranged from 6 to 78% (19, 37, 58), of which 31 (77.50%) studies showed that the support rate was less than half (11, 16–19, 21, 22, 24, 25, 30, 33–37, 39–42, 44–50, 55, 56, 59, 60, 63). Radiologists did not view AI as a threat to their professional roles or their autonomy, however, radiographers showed greater concern about AI undermining their job security (68). In our questionnaire, most disagreed that physicians will be replaced by AI in the future (68% disagreed). Although the number of those in agreement and with disagreement was balanced around whether physicians who embrace AI will replace those who do not (30% agreement vs. 30% disagreement; Figure 3).
In spite of the controversial opinions, there was consensus that AI should become a partner of physicians rather than a competitor (17). Respondents from several studies predicted that humans and machines would increasingly collaborate on healthcare (11, 17, 56, 59, 69). However, diagnostic decision-making should remain a predominantly human task or one shared equally with AI (11), which was consistent with our findings, that 68% agreed that AI should assist physicians (Figure 4B). While AI can assist in daily healthcare activities and contribute to workflow optimization (33, 56), physicians were not comfortable acting on reports independently issued by AI, and double checking by physicians would be preferred (39, 69). All investigated members of the European Society of Radiology believed that radiologists should be involved in clinical AI development and validation. 434 (64%) thought that acting as supervisors in AI projects would be most welcomed by radiologists, followed by 5359 (3%) who considered task definition and 197 (29%) in image labeling (22). Respondents from 18 medical societies and committees also pointed out that involving physicians in system design, procurement and updating could help realize the benefits of clinical decision support systems (57).
Clinical AI was considered as an influencer behind career choices, and radiologists seemed to be the most affected specialty with almost half of all medical students feeling less enthusiastic about their specialty as a result of AI (27, 34, 39, 41–44, 61). Yurdaisik et al. reported 55% of their sample of respondents thought that new physicians should choose professional fields in which AI would not dominate (37). However, developments in AI also positively affected career preferences for many physicians and medical students, making them optimistic about the future in their chosen specialty (25, 36, 37). Our survey found that 42% believed that the development of clinical AI made them more willing to engage in medicine, although 9% reported that it actually made medicine a less attractive option (Figure 3).
Challenges to clinical artificial intelligence development and implementation
Multiple challenges were emphasized in the development and implementation of clinical AI, including an absence of ethically defensible laws and policies (11, 33, 49, 55, 57, 59), ambiguous medico-legal responsibility for errors made by AI (11, 22–24, 37, 48, 57), data security and the risk of privacy disclosure (35, 40, 54, 69), “black box” nature of AI algorithms (57, 70), low availability of high-quality datasets for training and validation (57), and shortage of interdisciplinary talents (11). Among the respondents in our survey, the lack of interdisciplinary talents was the primary concern, followed by an absence of regulatory standards and a scarcity in high-quality data for AI training (Figure 4C).
Statistically significant associations
A comparison of response distributions across subgroups has been provided in Figure 5 and Supplementary Table 2. Moreover, Figure 5A illustrates that respondents who have used clinical AI in the past year expressed stronger feelings about the wide application of AI and reported having a better understanding of AI-related knowledge than those who had not. They were also more positive when considering the accuracy of clinical AI technologies. As can be seen in Figure 5B, in general, where there was a statistically significant difference between identities, physicians carried a more optimistic outlook regarding the performance and prospect of clinical AI, and expressed stronger willingness to use and learn clinical AI. Physicians also agreed more than medical students, that physicians would be replaced by clinical AI and conservative physicians will be replaced by those who embrace AI. Facing the rapid development of clinical AI, physicians showed greater enthusiasm than medical students.
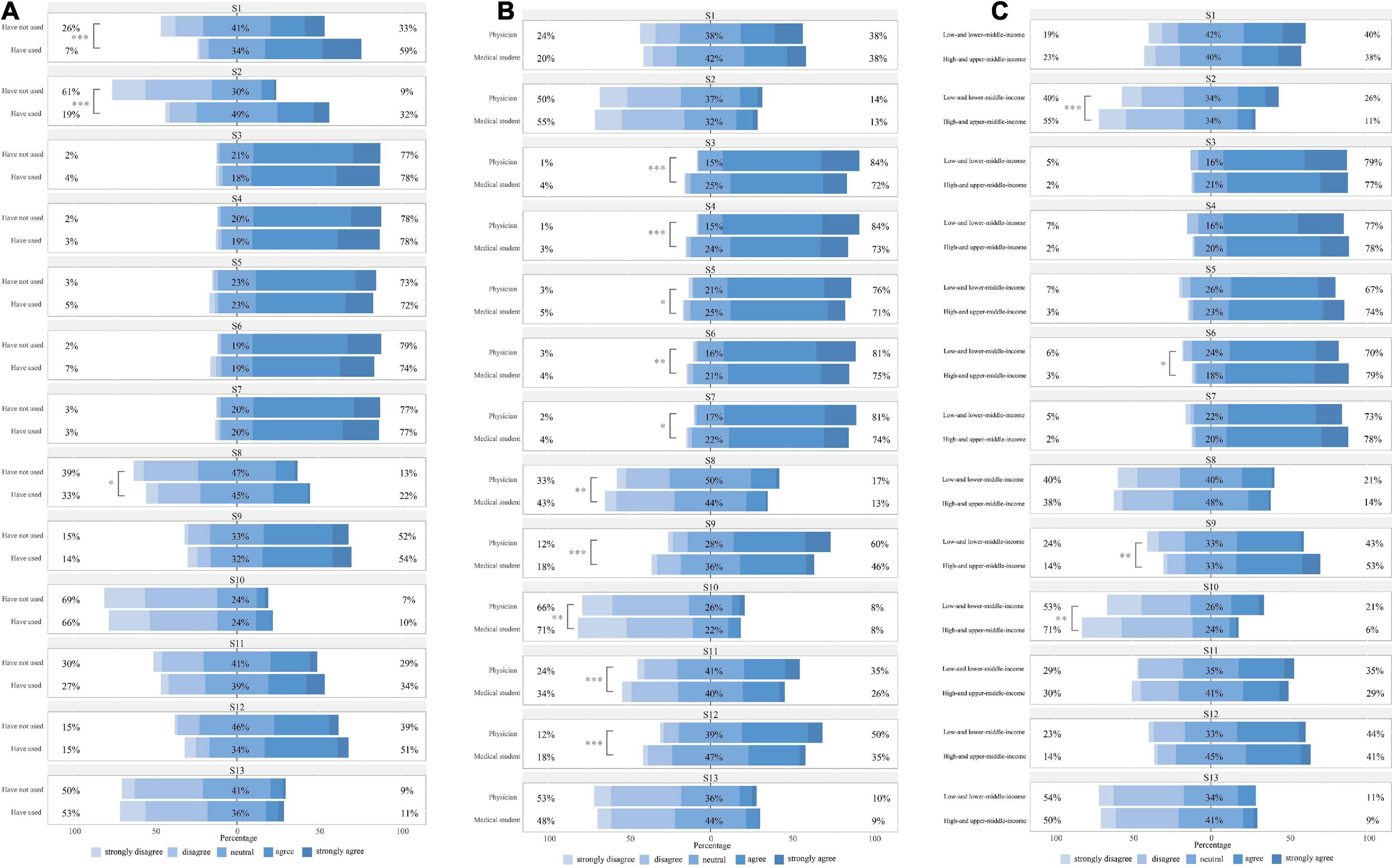
Figure 5. Subgroup analysis of responses to 13 statements. (A) By clinical artificial intelligence (AI) use experience; (B) By identity; (C) By country specific income levels. Mann–Whitney U test, *p < 0.05, **p < 0.01, ***p < 0.001.
Figure 5C compares respondent views on clinical AI in countries with different income levels. Compared with respondents from high- and upper-middle-income countries, those from low- and lower-middle-income countries reported subjectively more knowledge around AI, but tended to be less confident about the efficiency and wide application of clinical AI, with more agreeing that AI would replace physicians. Multivariable logistic regression revealed that physicians who worked in tertiary hospitals were more willing to use clinical AI [aOR 2.16 (1.11–4.25)]. Older physicians were also more positive about using clinical AI [aOR 1.08 (1.02–1.16)]. There were no statistically significant differences between medical students from various backgrounds. Detailed information has been provided in the Supplementary Tables 3, 4.
Discussion
Through this systematic review and evidence-based survey, we found that most physicians and medical students were aware of the increasing application of AI in medicine. However, few had actually experienced clinical AI first-hand and there appears to be a lack basic knowledge about these technologies. Overall, participants appeared optimistic about clinical AI but also had reservations. These reservations were not entirely dependent upon AI performance, but also appear related to responder characteristics. Even though the notion that AI could replace human physicians was contended, most believed that the collaboration between the two should be strengthened while maintaining physician’s autonomy. Additionally, a number of challenges emerged regarding clinical AI development pathways and around implementing novel AI technologies.
There is an optimistic yet reserved attitude about clinical AI, which suggests that AI is widely considered a complex socio-technical system with both positive and negative aspects. Rather than the physician spending a lot of time analyzing a patient’s condition in real-time, AI can process a huge amount clinical data using complex algorithms, which can provide diagnosis and treatment recommendations more quickly and more accurately (46, 58, 62). Although, it is also held that AI can generate unpredictable errors in uncommon or complex situations, especially where there is no specific algorithmic training (11). Actually, since the data sets used to train AI models always appear to exclude elderly people, rural communities, ethnic minorities, and other disadvantage groups, AI’s outputs might be inaccurate when applied to under-represented populations (6). Another issue in establishing trust in AI is the poor interpretability of AI algorithms. To be fair, algorithms with good explainability and high accuracy cannot be developed overnight. Therefore, it is particularly important to clearly explain the validation process of AI systems. Physicians need more information, such as data used for AI training, model construction process, and variables underlying AI models, to help them judge whether the AI results are reliable. However, unclear methodological interpretation, lacking a standardized nomenclature and heterogeneity in outcome measures for current clinical research limits the downstream evaluation of these technologies and their potential real-word benefits. Considering issues raised by AI-driven modalities, many well-known reporting guidelines have been extended to AI versions to improve reproducibility and transparency of clinical studies (71, 72). However, it takes time to establish norms and then to generate high-quality research outputs.
Although the current discourse around physician acceptance and utility of clinical AI has shifted from direct replacement to implementation and incorporation, the adoption of AI still has the possibility of transferring decision-making from human to machines, which may undermine human authority. In order to maintain autonomy in practice, physicians need to learn how to operate AI tools, judge the reliability of AI results outputs, as well as redesign current workflows. It appears that the most adaptable physicians, those who embrace AI will progress, while those who are unable or unwilling to adopt novel AI technologies may be left behind. Furthermore, physicians should not only become primary AI users, but also should be involved in the construction of AI technologies. The development of AI requires interdisciplinary collaboration, not just the task of computer scientists. Physicians have particular insight into clinical practice which can inspire AI developers to design AI tools that truly meet clinical needs. Physicians can also participate in the validation of AI systems to promote quality control.
Compared with the more positive views of direct clinical AI users, respondents without having had direct experience appeared to perceive clinical AI in more abstract manner and were more guarded in their opinions. Similarly, medical students appear to hold more conservative attitudes than physicians although this is at least partly due to limited experience. Physicians working in high-level hospitals are more likely to accept clinical AI than those from relatively low-level hospitals. This may be because there are differences in hospital resources which has influenced thinking about advancements in both superior and relatively inferior hospitals. High-level hospitals certainly have greater financial support with well-developed management mechanisms. Therefore, it might be wise to establish pilot AI programs in these hospitals. This will enable us to explore evolving practices and the challenges related to change, such as formulating new regulatory standards, defining responsibilities and determining accountability. Ensuring “early experiences” are captured and appraised will bring broader benefits to the community.
Our online questionnaire investigated some participants from low- and lower-middle-income countries who were not covered in previous studies. It was found that they were less optimistic about the prospect of clinical AI and more believed that AI would replace physicians than those from high- and upper-middle-income countries. Bisdas et al. also found that compared with medical students from developed countries, those from developing countries agreed less that AI will revolutionize medicine and more agreed that physicians would be replaced by AI (17). This discrepancy may be due to the gap in health infrastructures and in health workforces between countries with different income levels. For example, computed tomography (CT) scanner density in low-income countries is 1 in 65 of those in high-income countries (73). Having a Picture Archiving and Communication System (PACS) is also not so commonplace in low-income countries. However, many AI systems are embedded within hardware like CT scanners and are deployed using delivery platforms such as PACS. Therefore, inadequate infrastructures have seriously hampered the delivery and maintenance of AI. As for health workforce, skilled physicians in developed counties have the capability to judge AI outputs based on knowledge and clinical scenarios, but such expertise and labor are lacking in poorly resourced countries. Physicians in low-income countries may be less confident in their medical skills and may rely too much on AI, giving reason for the common belief that physicians will be replaced by AI. What we can say, is that the introduction of AI into resource-poor countries will proceed differently to high-income countries. Low-income countries need a site-specific tailored approach for integrating digital infrastructures and for clinical education, to maximize the benefits of clinical AI.
Before providing recommendations, we must acknowledge the limitations of this study. First, we did not assess risk of bias of each included study in the systematic review. We also note that our questionnaire and many of the studies included in the systematic review were Internet-based, which may have introduced non-response bias. The possibility that respondents are more likely to hold stronger views on this issue than non-respondents should be considered. Second, the relatively small sample size and uneven population distribution of our cross-sectional study means that our findings are less generalizable. Although we conducted subgroup analysis to evaluate differences in perspective among our respondents, these differences are likely to be fluid and to change as technologies evolve. However, the two-stage approach made our insights and comparisons more reliable. While beyond the remit of this study, we can see the general demand for AI-related education to overcome some of the anxieties associated with adopting new clinical AI technologies. Clearly, there is a need to incorporate health informatics, computer science and statistics into medical school and residency programs. This will increase awareness which can alleviate some of the stress involved in change, as well as facilitate safe and efficient implementation of clinical AI.
Conclusion
This novel study combined a systematic review with a cross-sectional survey to comprehensively understand physician and medical student acceptance of clinical AI. We found that a majority of physicians and medical students were aware of the increasing application of AI in medicine, but most had not actually used clinical AI and lacked basic knowledge. In general, participants were optimistic about clinical AI but had reservations. In spite of the contentious opinions around clinical AI becoming a surrogate physician, there was unanimity regarding strengthening collaborations between AI and human physicians. Relevant education is needed to overcome potential anxieties associated with adopting new technologies and to facilitate the successful implementation of clinical AI.
Data availability statement
The raw data supporting the conclusions of this article will be made available by the authors, without undue reservation.
Ethics statement
The research ethics committee of the Chinese Academy of Medical Sciences and Peking Union Medical College approved this study (CAMS&PUMC-IEC-2022-022). Participation in the questionnaire was voluntary and informed consent was obtained before completing the questionnaire.
Author contributions
MC, BZ, and PX conceptualized the study. BZ, ZC, and MC designed the systematic review, extracted data, and synthesis results. MC, BZ, MJG, NMA, and RR designed the questionnaire and conducted the analysis. MC and SS wrote the manuscript. YQ, PX, and YJ revised the manuscript. MC and BZ contributed equally to this article. All authors approved the final version of the manuscript and take accountability for all aspects of the work.
Funding
This study was supported by CAMS Innovation Fund for Medical Sciences (Grant #: CAMS 2021-I2M-1-004).
Conflict of interest
The authors declare that the research was conducted in the absence of any commercial or financial relationships that could be construed as a potential conflict of interest.
Publisher’s note
All claims expressed in this article are solely those of the authors and do not necessarily represent those of their affiliated organizations, or those of the publisher, the editors and the reviewers. Any product that may be evaluated in this article, or claim that may be made by its manufacturer, is not guaranteed or endorsed by the publisher.
Supplementary material
The Supplementary Material for this article can be found online at: https://www.frontiersin.org/articles/10.3389/fmed.2022.990604/full#supplementary-material
References
1. FDA. Artificial Intelligence and Machine Learning (Ai/Ml)-Enabled Medical Devices. (2021). Available online at: https://www.fda.gov/medical-devices/software-medical-device-samd/artificial-intelligence-and-machine-learning-aiml-enabled-medical-devices?utm_source=FDALinkedin#resources (accessed May 13, 2022).
2. Liu X, Faes L, Kale AU, Wagner SK, Fu DJ, Bruynseels A, et al. A comparison of deep learning performance against health-care professionals in detecting diseases from medical imaging: a systematic review and meta-analysis. Lancet Digit Health. (2019) 1:e271–97. doi: 10.1016/s2589-7500(19)30123-2
3. Xue P, Wang J, Qin D, Yan H, Qu Y, Seery S, et al. Deep learning in image-based breast and cervical cancer detection: a systematic review and meta-analysis. NPJ Digit Med. (2022) 5:19. doi: 10.1038/s41746-022-00559-z
4. Xue P, Tang C, Li Q, Li Y, Shen Y, Zhao Y, et al. Development and validation of an artificial intelligence system for grading colposcopic impressions and guiding biopsies. BMC Med. (2020) 18:406. doi: 10.1186/s12916-020-01860-y
5. Huynh E, Hosny A, Guthier C, Bitterman DS, Petit SF, Haas-Kogan DA, et al. Artificial intelligence in radiation oncology. Nat Rev Clin Oncol. (2020) 17:771–81. doi: 10.1038/s41571-020-0417-8
6. WHO. Ethics and Governance of Artificial Intelligence for Health: WHO Guidance Executive Summary. (2021). Available online at: https://www.who.int/publications/i/item/9789240037403 (accessed May 13, 2022).
7. Su X, You Z, Wang L, Hu L, Wong L, Ji B, et al. SANE: a sequence combined attentive network embedding model for COVID-19 drug repositioning. Appl Soft Comput. (2021) 111:107831. doi: 10.1016/j.asoc.2021.107831
8. Su X, Hu L, You Z, Hu P, Wang L, Zhao B. A deep learning method for repurposing antiviral drugs against new viruses via multi-view nonnegative matrix factorization and its application to SARS-CoV-2. Brief Bioinform. (2022) 23:bbab526. doi: 10.1093/bib/bbab526
9. Scott IA, Carter SM, Coiera E. Exploring stakeholder attitudes towards AI in clinical practice. BMJ Health Care Inform. (2021) 28:e100450. doi: 10.1136/bmjhci-2021-100450
10. He J, Baxter SL, Xu J, Xu J, Zhou X, Zhang K. The practical implementation of artificial intelligence technologies in medicine. Nat Med. (2019) 25:30–6. doi: 10.1038/s41591-018-0307-0
11. Sarwar S, Dent A, Faust K, Richer M, Djuric U, Van Ommeren R, et al. Physician perspectives on integration of artificial intelligence into diagnostic pathology. NPJ Digit Med. (2019) 2:28. doi: 10.1038/s41746-019-0106-0
12. Park CJ, Yi PH, Siegel EL. Medical student perspectives on the impact of artificial intelligence on the practice of medicine. Curr Probl Diagn Radiol. (2021) 50:614–9. doi: 10.1067/j.cpradiol.2020.06.011
13. Santomartino SM, Yi PH. Systematic review of radiologist and medical student attitudes on the role and impact of AI in radiology. Acad Radiol. (2022). 29:S1076-6332(21)00624-3. doi: 10.1016/j.acra.2021.12.032 [Epub ahead of print].
14. Page MJ, McKenzie JE, Bossuyt PM, Boutron I, Hoffmann TC, Mulrow CD, et al. The PRISMA 2020 statement: an updated guideline for reporting systematic reviews. BMJ. (2021) 372:n71. doi: 10.1136/bmj.n71
15. Vandenbroucke JP, von Elm E, Altman DG, Gøtzsche PC, Mulrow CD, Pocock SJ, et al. Strengthening the reporting of observational studies in epidemiology (STROBE): explanation and elaboration. PLoS Med. (2007) 4:e297. doi: 10.1371/journal.pmed.0040297
16. Abuzaid MM, Elshami W, Tekin H, Issa B. Assessment of the willingness of radiologists and radiographers to accept the integration of artificial intelligence into radiology practice. Acad Radiol. (2022) 29:87–94. doi: 10.1016/j.acra.2020.09.014
17. Bisdas S, Topriceanu CC, Zakrzewska Z, Irimia AV, Shakallis L, Subhash J, et al. Artificial intelligence in medicine: a multinational multi-center survey on the medical and dental students’Perception. Front Public Health. (2021) 9:795284. doi: 10.3389/fpubh.2021.795284
18. Polesie S, McKee PH, Gardner JM, Gillstedt M, Siarov J, Neittaanmäki N, et al. Attitudes toward artificial intelligence within dermatopathology: an international online survey. Fron Med. (2020) 7:591952. doi: 10.3389/fmed.2020.591952
19. Polesie S, Gillstedt M, Kittler H, Lallas A, Tschandl P, Zalaudek I, et al. Attitudes towards artificial intelligence within dermatology: an international online survey. Br J Dermatol. (2020) 183:159–61. doi: 10.1111/bjd.18875
20. Wadhwa V, Alagappan M, Gonzalez A, Gupta K, Brown JRG, Cohen J, et al. Physician sentiment toward artificial intelligence (AI) in colonoscopic practice: a survey of US gastroenterologists. Endosc Int Open. (2020) 8:E1379–84. doi: 10.1055/a-1223-1926
21. Qurashi AA, Alanazi RK, Alhazmi YM, Almohammadi AS, Alsharif WM, Alshamrani KM. Saudi radiology personnel’s perceptions of artificial intelligence implementation: a cross-sectional study. J Multidiscip Healthc. (2021) 14:3225–31. doi: 10.2147/JMDH.S340786
22. European Society of Radiology [ESR]. Impactof artificial intelligence on radiology: a euroaim survey among members of the european society of radiology. Insights Imaging. (2019) 10:105. doi: 10.1186/s13244-019-0798-3
23. Scheetz J, Rothschild P, McGuinness M, Hadoux X, Soyer HP, Janda M, et al. A survey of clinicians on the use of artificial intelligence in ophthalmology, dermatology, radiology and radiation oncology. Sci Rep. (2021) 11:5193. doi: 10.1038/s41598-021-84698-5
24. Leenhardt R, Sainz IFU, Rondonotti E, Toth E, Van de Bruaene C, Baltes P, et al. Peace: perception and expectations toward artificial intelligence in capsule endoscopy. J Clin Med. (2021) 10:5708. doi: 10.3390/jcm10235708
25. Waymel Q, Badr S, Demondion X, Cotten A, Jacques T. Impact of the rise of artificial intelligence in radiology: what do radiologists think? Diagn Interv Imaging. (2019) 100:327–36. doi: 10.1016/j.diii.2019.03.015
26. Zheng B, Wu MN, Zhu SJ, Zhou HX, Hao XL, Fei FQ, et al. Attitudes of medical workers in china toward artificial intelligence in ophthalmology: a comparative survey. BMC Health Serv Res. (2021) 21:1067. doi: 10.1186/s12913-021-07044-5
27. Reeder K, Lee H. Impact of artificial intelligence on US medical students’ choice of radiology. Clin Imaging. (2022) 81:67–71. doi: 10.1016/j.clinimag.2021.09.018
28. Buck C, Doctor E, Hennrich J, Jöhnk J, Eymann T. General practitioners’ attitudes toward artificial intelligence–enabled systems: interview study. J Med Internet Res. (2022) 24:e28916. doi: 10.2196/28916
29. Khafaji MA, Safhi MA, Albadawi RH, Al-Amoudi SO, Shehata SS, Toonsi F. Artificial intelligence in radiology: are saudi residents ready prepared, and knowledgeable? Saudi Med J. (2022) 43:53–60. doi: 10.15537/smj.2022.43.1.20210337
30. Kansal R, Bawa A, Bansal A, Trehan S, Goyal K, Goyal N, et al. Differences in knowledge and perspectives on the usage of artificial intelligence among doctors and medical students of a developing country: a cross-sectional study. Cureus. (2022) 14:e21434. doi: 10.7759/cureus.21434
31. Eiroa D, Antolín A, Fernández Del Castillo Ascanio M, Pantoja Ortiz V, Escobar M, Roson N. The current state of knowledge on imaging informatics: a survey among spanish radiologists. Insights Imaging. (2022) 13:34. doi: 10.1186/s13244-022-01164-0
32. Teng M, Singla R, Yau O, Lamoureux D, Gupta A, Hu Z, et al. Health care students’ perspectives on artificial intelligence: countrywide survey in Canada. JMIR Med Educ. (2022) 8:e33390. doi: 10.2196/33390
33. Huisman M, Ranschaert E, Parker W, Mastrodicasa D, Koci M, Pinto de Santos D, et al. An international survey on AI in radiology in 1041 radiologists and radiology residents part 2: expectations, hurdles to implementation, and education. Eur Radiol. (2021) 31:8797–806. doi: 10.1007/s00330-021-07782-4
34. Huisman M, Ranschaert E, Parker W, Mastrodicasa D, Koci M, Pinto de Santos D, et al. An international survey on AI in radiology in 1,041 radiologists and radiology residents part 1: fear of replacement, knowledge, and attitude. Eur Radiol. (2021) 31:7058–66. doi: 10.1007/s00330-021-07781-5
35. Kochhar GS, Carleton NM, Thakkar S. Assessing perspectives on artificial intelligence applications to gastroenterology. Gastrointest Endosc. (2021) 93:971–5.e2. doi: 10.1016/j.gie.2020.10.029
36. Cho SI, Han B, Hur K, Mun JH. Perceptions and attitudes of medical students regarding artificial intelligence in dermatology. J Eur Acad Dermatol and Venereol. (2021) 35:e72–3. doi: 10.1111/jdv.16812
37. Yurdaisik I, Aksoy SH. Evaluation of knowledge and attitudes of radiology department workers about artificial intelligence. Ann Clin Anal Med. (2021) 12:186–90. doi: 10.4328/ACAM.20453
38. Tran AQ, Nguyen LH, Nguyen HSA, Nguyen CT, Vu LG, Zhang M, et al. Determinants of intention to use artificial intelligence-based diagnosis support system among prospective physicians. Front Public Health. (2021) 9:755644. doi: 10.3389/fpubh.2021.755644
39. Eltorai AEM, Bratt AK, Guo HH. Thoracic radiologists’ versus computer scientists’ perspectives on the future of artificial intelligence in radiology. J Thorac Imaging. (2020) 35:255–9. doi: 10.1097/RTI.0000000000000453
40. Castagno S, Khalifa M. Perceptions of artificial intelligence among healthcare staff: a qualitative survey study. Front Artif Intell. (2020) 3:578983. doi: 10.3389/frai.2020.578983
41. Sit C, Srinivasan R, Amlani A, Muthuswamy K, Azam A, Monzon L, et al. Attitudes and perceptions of UK medical students towards artificial intelligence and radiology: a multicentre survey. Insights Imaging. (2020) 11:14. doi: 10.1186/s13244-019-0830-7
42. Bin Dahmash A, Alabdulkareem M, Alfutais A, Kamel AM, Alkholaiwi F, Alshehri S, et al. Artificial intelligence in radiology: does it impact medical students preference for radiology as their future career? BJR Open. (2020) 2:20200037. doi: 10.1259/bjro.20200037
43. Brandes GIG, D’Ippolito G, Azzolini AG, Meirelles G. Impact of artificial intelligence on the choice of radiology as a specialty by medical students from the city of São Paulo. Radiol Bras. (2020) 53:167–70. doi: 10.1590/0100-3984.2019.0101
44. Gong B, Nugent JP, Guest W, Parker W, Chang PJ, Khosa F, et al. Influence of artificial intelligence on canadian medical students’ preference for radiology specialty: a national survey study. Acad Radiol. (2019) 26:566–77. doi: 10.1016/j.acra.2018.10.007
45. Pinto dos Santos D, Giese D, Brodehl S, Chon SH, Staab W, Kleinert R, et al. Medical students’ attitude towards artificial intelligence: a multicentre survey. Eur Radiol. (2019) 29:1640–6. doi: 10.1007/s00330-018-5601-1
46. Oh S, Kim JH, Choi SW, Lee HJ, Hong J, Kwon SH. Physician confidence in artificial intelligence: an online mobile survey. J Med Internet Res. (2019) 21:e12422. doi: 10.2196/12422
47. Batumalai V, Jameson MG, King O, Walker R, Slater C, Dundas K, et al. Cautiously optimistic: a survey of radiation oncology professionals’ perceptions of automation in radiotherapy planning. Tech Innov Patient Support Radiat Oncol. (2020) 16:58–64. doi: 10.1016/j.tipsro.2020.10.003
48. Shelmerdine SC, Rosendahl K, Arthurs OJ. Artificial intelligence in paediatric radiology: international survey of health care professionals’Opinions. Pediatr Radiol. (2022) 52:30–41. doi: 10.1007/s00247-021-05195-5
49. Pangti R, Gupta S, Gupta P, Dixit A, Sati HC, Gupta S. Acceptability of artificial intelligence among indian dermatologists. Indian J Dermatol Venereol Leprol. (2021) 88:232–4. doi: 10.25259/IJDVL_210_2021
50. Valikodath NG, Al-Khaled T, Cole E, Ting DSW, Tu EY, Campbell JP, et al. Evaluation of pediatric ophthalmologists’ perspectives of artificial intelligence in ophthalmology. J AAPOS. (2021) 25:e1–5. doi: 10.1016/j.jaapos.2021.01.011
51. Wood EA, Ange BL, Miller DD. Are we ready to integrate artificial intelligence literacy into medical school curriculum: students and faculty survey. J Med Educ Curric Dev. (2021) 8:1–5. doi: 10.1177/23821205211024078
52. Hah H, Goldin DS. How clinicians perceive artificial intelligence-assisted technologies in diagnostic decision making: mixed methods approach. J Med Internet Res. (2021) 23:e33540. doi: 10.2196/33540
53. Wong K, Gallant F, Szumacher E. Perceptions of Canadian radiation oncologists, radiation physicists, radiation therapists and radiation trainees about the impact of artificial intelligence in radiation oncology – national survey. J Med Imaging Radiat Sci. (2021) 52:44–8. doi: 10.1016/j.jmir.2020.11.013
54. Layard Horsfall H, Palmisciano P, Khan DZ, Muirhead W, Koh CH, Stoyanov D, et al. Attitudes of the surgical team toward artificial intelligence in neurosurgery: international 2-stage cross-sectional survey. World Neurosurg. (2021) 146:e724–30. doi: 10.1016/j.wneu.2020.10.171
55. Coppola F, Faggioni L, Regge D, Giovagnoni A, Golfieri R, Bibbolino C, et al. Artificial intelligence: radiologists’ expectations and opinions gleaned from a nationwide online survey. Radiol Med. (2021) 126:63–71. doi: 10.1007/s11547-020-01205-y
56. Shen C, Li C, Xu F, Wang Z, Shen X, Gao J, et al. Web-Based study on chinese dermatologists’ attitudes towards artificial intelligence. Ann Transl Med. (2020) 8:698. doi: 10.21037/atm.2019.12.102
57. Petkus H, Hoogewerf J, Wyatt JC. What do senior physicians think about AI and clinical decision support systems: quantitative and qualitative analysis of data from specialty societies. Clin Med. (2020) 20:324–8. doi: 10.7861/clinmed.2019-0317
58. Abdullah R, Fakieh B. Health care employees’ perceptions of the use of artificial intelligence applications: survey study. J Med Internet Res. (2020) 22:e17620. doi: 10.2196/17620
59. Blease C, Locher C, Leon-Carlyle M, Doraiswamy M. Artificial intelligence and the future of psychiatry: qualitative findings from a global physician survey. Digit Health. (2020) 6:1–18. doi: 10.1177/2055207620968355
60. Kasetti P, Botchu R. The impact of artificial intelligence in radiology: as perceived by medical students. Russ Electron J Radiol. (2020) 10:179–85. doi: 10.21569/2222-7415-2020-10-4-179-185
61. van Hoek J, Huber A, Leichtle A, Härmä K, Hilt D, von Tengg-Kobligk H, et al. A survey on the future of radiology among radiologists, medical students and surgeons: students and surgeons tend to be more skeptical about artificial intelligence and radiologists may fear that other disciplines take over. Eur J Radiol. (2019) 121:108742. doi: 10.1016/j.ejrad.2019.108742
62. Blease C, Kaptchuk TJ, Bernstein MH, Mandl KD, Halamka JD, DesRoches CM. Artificial intelligence and the future of primary care: exploratory qualitative study of UK general practitioners’views. J Med Internet Res. (2019) 21:e12802. doi: 10.2196/12802
63. Doraiswamy PM, Blease C, Bodner K. Artificial intelligence and the future of psychiatry: insights from a global physician survey. Artifi Intell Med. (2020) 102:101753. doi: 10.1016/j.artmed.2019.101753
64. Nelson CA, Pachauri S, Balk R, Miller J, Theunis R, Ko JM, et al. Dermatologists perspectives on artificial intelligence and augmented intelligence - a cross-sectional survey. JAMA Dermatol. (2021) 157:871–4. doi: 10.1001/jamadermatol.2021.1685
65. Pan J, Ding S, Wu D, Yang S, Yang J. Exploring behavioural intentions toward smart healthcare services among medical practitioners: a technology transfer perspective. Int J Prod Res. (2019) 57:5801–20. doi: 10.1080/00207543.2018.1550272
66. Zhai H, Yang X, Xue J, Lavender C, Ye T, Li JB, et al. Radiation oncologists’ perceptions of adopting an artificial intelligence-assisted contouring technology: model development and questionnaire study. J Med Internet Res. (2021) 23:e27122. doi: 10.2196/27122
67. Prakash AV, Das S. Medical practitioner’s adoption of intelligent clinical diagnostic decision support systems: a mixed-methods study. Inf Manag. (2021) 58:103524. doi: 10.1016/j.im.2021.103524
68. Chen Y, Stavropoulou C, Narasinkan R, Baker A, Scarbrough H. Professionals’ responses to the introduction of AI innovations in radiology and their implications for future adoption: a qualitative study. BMC Health Serv Res. (2021) 21:813. doi: 10.1186/s12913-021-06861-y
69. Lim SS, Phan TD, Law M, Goh GS, Moriarty HK, Lukies MW, et al. Non-radiologist perception of the use of artificial intelligence (AI) in diagnostic medical imaging reports. J Med Imaging Radiat Oncol. (2022). doi: 10.1111/1754-9485.13388 [Epub ahead of print].
70. Martinho A, Kroesen M, Chorus CA. Healthy debate: exploring the views of medical doctors on the ethics of artificial intelligence. Artifi Intell Med. (2021) 121:102190. doi: 10.1016/j.artmed.2021.102190
71. Sounderajah V, Ashrafian H, Aggarwal R, De Fauw J, Denniston AK, Greaves F, et al. Developing specific reporting guidelines for diagnostic accuracy studies assessing AI interventions: the stard-AI steering group. Nat Med. (2020) 26:807–8. doi: 10.1038/s41591-020-0941-1
72. Shelmerdine SC, Arthurs OJ, Denniston A, Sebire NJ. Review of study reporting guidelines for clinical studies using artificial intelligence in healthcare. BMJ Health Care Inform. (2021) 28:e100385. doi: 10.1136/bmjhci-2021-100385
73. Mollura DJ, Culp MP, Pollack E, Battino G, Scheel JR, Mango VL, et al. Artificial intelligence in low- and middle-income countries: innovating global health radiology. Radiology. (2020) 297:513–20. doi: 10.1148/radiol.2020201434
74. Pumplun L, Fecho M, Wahl N, Peters F, Buxmann P. Adoption of machine learning systems for medical diagnostics in clinics: qualitative interview study. J Med Internet Res. (2021) 23:e29301. doi: 10.2196/29301
75. Staartjes VE, Stumpo V, Kernbach JM, Klukowska AM, Gadjradj PS, Schröder ML, et al. Machine learning in neurosurgery: a global survey. Acta Neurochir. (2020) 162:3081–91. doi: 10.1007/s00701-020-04532-1
Keywords: artificial intelligence (AI), acceptance, physicians, medical students, attitude
Citation: Chen M, Zhang B, Cai Z, Seery S, Gonzalez MJ, Ali NM, Ren R, Qiao Y, Xue P and Jiang Y (2022) Acceptance of clinical artificial intelligence among physicians and medical students: A systematic review with cross-sectional survey. Front. Med. 9:990604. doi: 10.3389/fmed.2022.990604
Received: 10 July 2022; Accepted: 01 August 2022;
Published: 31 August 2022.
Edited by:
Lun Hu, Xinjiang Technical Institute of Physics and Chemistry (CAS), ChinaReviewed by:
Shaokai Zhang, Henan Provincial Cancer Hospital, ChinaBo-Wei Zhao, Xinjiang Technical Institute of Physics and Chemistry (CAS), China
Copyright © 2022 Chen, Zhang, Cai, Seery, Gonzalez, Ali, Ren, Qiao, Xue and Jiang. This is an open-access article distributed under the terms of the Creative Commons Attribution License (CC BY). The use, distribution or reproduction in other forums is permitted, provided the original author(s) and the copyright owner(s) are credited and that the original publication in this journal is cited, in accordance with accepted academic practice. No use, distribution or reproduction is permitted which does not comply with these terms.
*Correspondence: Youlin Qiao, cWlhb3lAY2ljYW1zLmFjLmNu; Peng Xue, eHVlcGVuZ19wdW1jQGZveG1haWwuY29t; Yu Jiang, amlhbmd5dUBwdW1jLmVkdS5jbg==
†These authors have contributed equally to this work and share first authorship
‡ORCID: Samuel Seery, https://orcid.org/0000-0001-8277-1076; Youlin Qiao, https://orcid.org/0000-0001-6380-0871; Peng Xue, https://orcid.org/0000-0003-3002-8146