- 1Department of Public Health and Primary Care, Leiden University Medical Centre, Leiden, Netherlands
- 2Health Campus the Hague, Leiden University Medical Center, The Hague, Netherlands
Introduction: Cardiometabolic diseases (CMD) are the leading cause of death in high-income countries and are largely attributable to modifiable risk factors. Population health management (PHM) can effectively identify patient subgroups at high risk of CMD and address missed opportunities for preventive disease management. Guided by the Reach, Efficacy, Adoption, Implementation and Maintenance (RE-AIM) framework, this scoping review of PHM interventions targeting patients in primary care at increased risk of CMD aims to describe the reported aspects for successful implementation.
Methods: A comprehensive search was conducted across 14 databases to identify papers published between 2000 and 2023, using Arksey and O’Malley’s framework for conducting scoping reviews. The RE-AIM framework was used to assess the implementation, documentation, and the population health impact score of the PHM interventions.
Results: A total of 26 out of 1,100 studies were included, representing 21 unique PHM interventions. This review found insufficient reporting of most RE-AIM components. The RE-AIM evaluation showed that the included interventions could potentially reach a large audience and achieve their intended goals, but information on adoption and maintenance was often lacking. A population health impact score was calculated for six interventions ranging from 28 to 62%.
Discussion: This review showed the promise of PHM interventions that could reaching a substantial number of participants and reducing CMD risk factors. However, to better assess the generalizability and scalability of these interventions there is a need for an improved assessment of adoption, implementation processes, and sustainability.
1 Introduction
Cardiometabolic diseases (CMD), which include cardiovascular disease, diabetes mellitus, and chronic renal failure, are the leading cause of death in high-income countries and are increasing worldwide. If this situation continues unchecked it could potentially compromise the sustainability of healthcare systems (1–3). Cardiometabolic diseases can be prevented for a large part by addressing modifiable risk factors, such as elevated blood pressure, unhealthy dietary habits, and smoking (4–7). To accomplish this, the proactive identification of high-risk patients is essential for early detection of these modifiable risk factors (8).
Population health management (PHM) is a strategy that supports proactive care by identifying and addressing missed opportunities in chronic disease management (9). Population Health Management, in a clinical context, is also known as panel management and can be defined as ‘the proactive management of a total population at risk for adverse outcomes through various individual, organizational and cultural interventions based on a risk-stratified needs assessment of the population’ (9). Primary care occupies a central position in the implementation of PHM thanks to its inherent capacity for care coordination and integration, coupled with access to comprehensively coded routine care data. These unique characteristics of primary care also promote the effective identification of individuals at increased risk of CMD progression and the provision of appropriate care related to identified risk (10, 11).
While there is increasing interest in PHM in relation to CMD, a clear overview detailing how PHM interventions are best implemented in primary care is lacking. Although various implementation theories and frameworks are available, the RE-AIM framework provides a vital tool for evaluating and comprehending the effectiveness and sustainability of PHM interventions in primary care. The RE-AIM framework assesses the impact of population health intervention initiatives using five critical factors: Reach, Efficacy, Adoption, Implementation, and Maintenance (12). Additionally, this framework aids in determining the potential population health impact of these interventions.
This scoping review aimed to identify PHM interventions, which were targeted at patients with a high risk of CMD in the primary care setting. This was accomplished by obtaining information according to the dimensions outlined in the RE-AIM framework and estimating their potential population health impact. In doing so, the study has the objective of contributing to an understanding of the implementation of PHM interventions, their potential population health impact, and to better inform future research efforts.
2 Methods
2.1 Search strategy
This scoping review followed the recommendations of Preferred Reporting Items for Systematic Reviews and Meta-Analyses for Scoping Reviews (PRISMA-ScR) (13). The search was conducted in the electronic databases Pubmed, Embase, Web of Science, COCHRANE Library, Emcare, Academic Search Premier, IEEE Xplore, ACM Digital Library, MathSciNet, AAAi.org, arXiv, Epistemonikos, PsycINFO and Google Scholar. The search was formulated as a combination of terms that included PHM, primary care, and implementation. The terms were identified through searches of the National Library of Medicine MeSH Tree Structures and by the review team expert. The full search strategy can be found in Supplementary File 1.
2.2 Eligibility criteria
Peer-reviewed journal papers were included when they met the following eligibility criteria: (i) published between 2000–2023, (ii) written in English, (iii) published as original results, (iv) focused on risk-based identification of patient groups (panels) with a high risk of (progression of) CMD using a primary care data source or within a primary care setting, and (v) focused on original data about implementation as part of (pragmatic) randomized controlled trials, clinical trials, (retrospective) cohort, case–control, implementation studies, cost-effectiveness or (pilot) feasibility studies. Studies that focused on developing theoretical PHM interventions and those in which the strategy was implemented in a setting other than primary care were excluded.
2.3 Data extraction
Using the inclusion and exclusion criteria, two reviewers (M.M.R and S.P.C.P) independently screened all articles on title and abstract. Reading the full text, two team members (M.M.R and S.P.C.P) assessed the selected articles for eligibility. Disagreements were discussed by the core team (M.M.R, N.E.v.H, and S.P.C.P) until consensus was reached. Subsequently, the core team members (M.M.R., N.E.v.H, and S.P.C.P) independently completed full data extraction of study characteristics (publication year, purpose, target population, study design and steps within PHM), and the five dimensions of the RE-AIM framework (14). The three assessors addressed their differences until they came to a complete understanding.
For data extraction focused on RE-AIM dimensions, researchers employed a modified extraction technique created specifically designed for systematic reviews using a RE-AIM framework (see Supplementary File 2) (15, 16). Each of the five RE-AIM dimensions was broken down into a number of components, and the core team (M.M.R., N.E.v.H, and S.P.C.P) categorized each recorded article in relation to whether they reported on specific components. Components were based on inclusion criteria: process interventions to improve clinical health outcomes of a defined group of individuals through proactive care coordination and patient engagement. The components for Reach were: the description of the target population, method of identifying the population, recruitment strategies, inclusion/exclusion criteria, participation rate, and cost of the recruitment. For Effectiveness, quality of life measures, positive outcomes, unintended or negative consequences, and cost-effectiveness were reported. Adoption was extracted based on the site and staff participation rate, description of the intervention location, method of identifying setting and staff, level of expertise of providers, and inclusion or exclusion criteria for providers. Implementation was coded on intervention description, theory-based interventions, engagement, consistency of implementation, financial investment, and the number and timing of intervention contacts during implementation. Lastly, Maintenance was extracted based on follow-up time, program sustainability, and modifications to the original program. Subsequently, the RE-AIM average [((Participation rate + ESkey outcomes + adoption rate + implementation rate)/4) × 100] was computed by aggregating the scores across the RE-AIM dimensions. This RE-AIM average represented the potential population health impact of the interventions (12, 17).
3 Results
3.1 Intervention characteristics of the studies reviewed
Of the 1,110 studies initially identified, 78 remained after removing duplicates and screening titles and abstracts. Full-text screening led to the inclusion of 26 studies, representing 21 unique interventions (see Figure 1). Most PHM interventions were published in the last five years (13 of 21). Seven of the 21 included interventions were randomized controlled studies, and eight were prospective cohort studies. The characteristics of the reviewed interventions included in the analysis are summarized in Table 1.
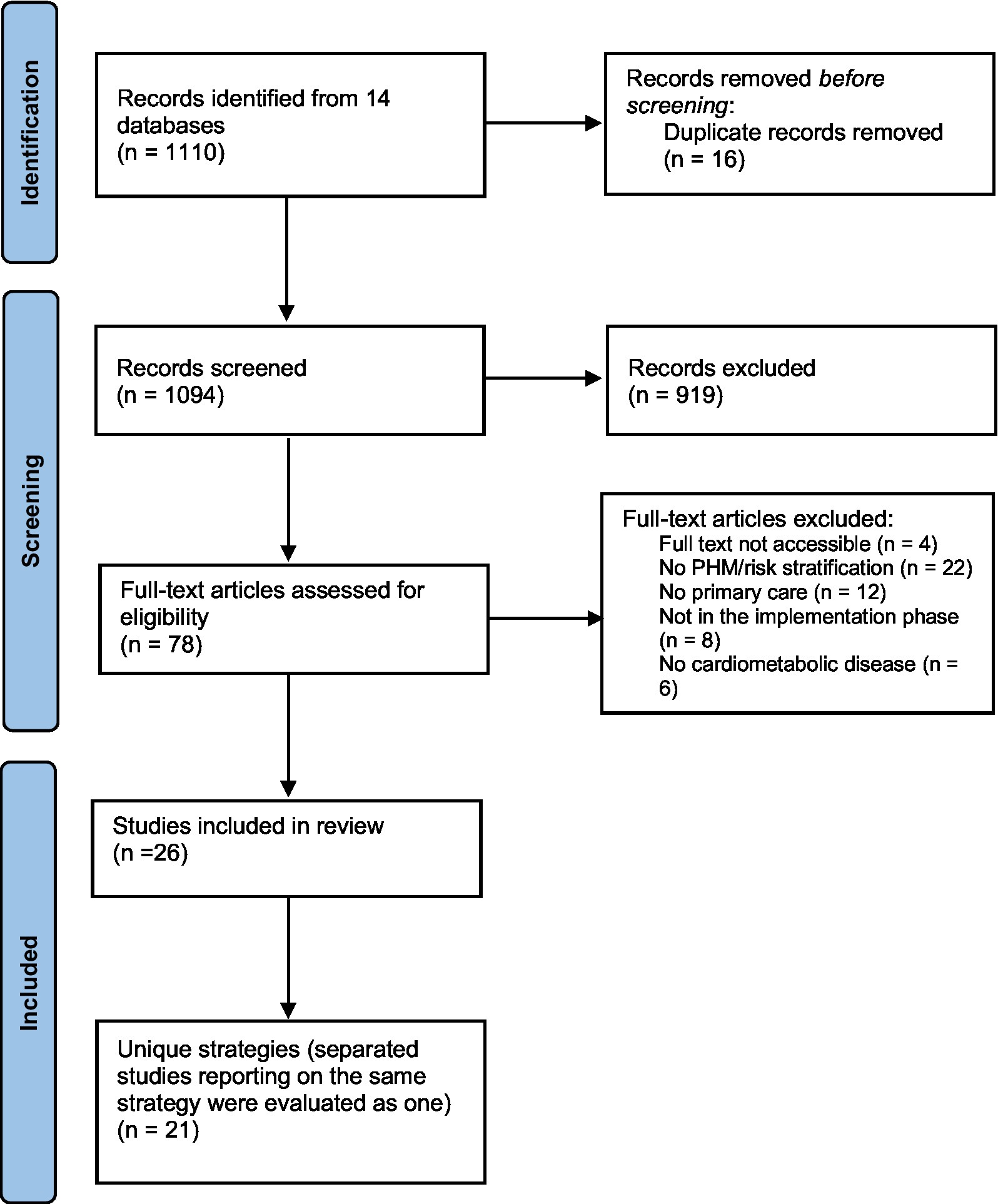
Figure 1. Preferred reporting items for systematic reviews and meta-analyses flow chart, which included searches of databases.
3.2 RE-AIM evaluation
A comprehensive overview of RE-AIM dimensions, including individual components, can be found in Table 2 (detailed data extraction is provided in Supplementary File 3). Three of the PHM interventions only reported data on (or at least one of the individual components of) three dimensions: reach, adoption and implementation. Another 12 interventions reported data on four dimensions: reach, effectiveness, adoption and implementation. Six interventions provided information on all five dimensions of the RE-AIM framework.
3.2.1 Reach
Among all the evaluated dimensions in the included interventions, reach was documented most extensively. In total, 15 interventions reported five out of seven reach components (19, 20, 22–25, 27–29, 34, 36–38, 44). All interventions provided information on the target population, and 20 described the method of identifying the target population. The sample size of the interventions ranged from 10 to 146,826 participants, with participation rates varying from 3 to 95%. Five interventions reported a participation rate below 10% (22, 24, 25, 33, 36); the participation rate of 3% was primarily due to non-compliance with inclusion criteria (25). In Mori’s study, the algorithm could not calculate risk for most patients, rendering them unidentifiable. The primary method of participant recruitment for most interventions (11 out of 21) was electronic health record data assessment using algorithms (20, 22, 23, 25, 26, 31, 33, 34, 36, 38, 44), the first step in the panel management approach. However, only a small proportion of interventions (3 out of 21) reported the cost of recruitment activities (22, 23, 28).
3.2.2 Effectiveness
Among the 21 interventions reviewed, nine interventions (43%) focused on patients at risk of (progression of) cardiovascular diseases (20, 23, 26, 27, 34–37, 44), six interventions (29%) on diabetes (18, 19, 22, 24, 28, 33), and six interventions (29%) on CMD (25, 30–33, 38). Of the 12 interventions that evaluated diabetes outcome measurements, only one (5%) reported no statistically significant impact (28), while six reported significantly positive outcomes on diabetes control (18, 19, 25, 30, 31, 38). Two interventions (10%) focused on feasibility and acceptability outcomes and found positive results in terms of better understanding and proficiency in managing individuals with complex diabetes in a primary care setting through PHM (24, 33). Only two interventions reported unintended consequences of the intervention (20, 23), and only one measured quality of life (18). A minority of interventions (24%) addressed the cost of the intervention (18, 23, 26, 29, 33), of which one included a formal cost-effectiveness analysis (18).
3.2.3 Adoption
All included interventions (n = 21) documented adoption, but none reported all eight adoption components. The proficiency level of staff was reported in 18 interventions (86%) (18–20, 23–25, 28–30, 32, 34–38, 44), and 19 interventions (91%) described the intervention location (1, 18–20, 24–32, 34–37, 44, 47). However, the staff participation rate and the method of staff identification were mentioned in only five (24%) and two (10%) interventions, respectively. Inclusion or exclusion criteria for staff were documented in three (14%) interventions (19, 20, 35). At the level of the clinical setting, 17 interventions (81%) reported the site participation rate, with an average of 50 to 12,000 individuals served per setting (19, 20, 22–26, 29–31, 33, 35–38, 44, 48). Only one study used qualitative methods to measure adoption, using surveys and observations (24).
3.2.4 Implementation
Descriptions of the intervention were provided for all 21 interventions. Only three interventions (14%) explained the theories or principles that guided the creation of the intervention (27, 34, 36). The frequency, duration, and timing of visits varied across interventions and were sometimes inadequately described. Patient engagement in intervention design was reported in only a single study (25), while five interventions (24%) involved healthcare professionals, experts, and local stakeholders in developing specific intervention components (19, 25, 26, 36, 44).
3.2.5 Maintenance
Maintenance was least often reported across all interventions. Five interventions (24%) reported on the continuation of the program after the study period (27, 29, 30, 33, 41), with just one providing details (27). Additionally, while three interventions (14%) reported modifications to the original program, these changes were implemented during the study period, not after completion (24, 25, 27).
3.2.6 Potential population health impact
Calculating the potential population health impact was possible for six out of 21 interventions (see Table 3), with scores ranging from 45 to 89%. The RE-AIM mean exhibited clear variation attributable to differences within each dimension, except for the implementation score, which remained consistent across all interventions.
4 Discussion
A total of 21 PHM interventions for patients at high risk of CMD in a primary care setting were identified. These interventions showed promise in engaging a substantial number of participants and reducing CMD risk factors. However, this study also revealed a widespread deficiency in reporting across most RE-AIM components. While the included interventions exhibited higher reporting accuracy concerning Reach, followed by Adoption and Implementation, the constructs Effectiveness and Maintenance were barely addressed. A similar trend was found regarding the population health impact score, as the score could only be calculated for six interventions.
Compared with previous systematic reviews (15, 49–52), Reach (especially the description and the method of identifying the target population) was well described, with most interventions using algorithms or risk stratification tools in electronic health records to identify potential participants. Population surveys or routine care checks were employed for those who did not use electronic health records. However, it is worth noting that the risk calculation primarily relied on clinical health outcomes and did not incorporate health behaviors or social determinants of health. Given their significance in determining the risk of a particular group (53, 54), integrating health behaviors and social determinants into the risk model may be crucial to ensure that all potentially suitable patients are included.
Secondly, most studies reported positive outcomes while neglecting to address negative consequences of the intervention adequately. Awareness of negative outcomes, such as attrition and adverse outcomes, is essential for developing effective implementation strategies and ensuring the sustainability of interventions (55). Moreover, most interventions lacked follow-up data and information on attrition, which raises concerns about their long-term effectiveness. However, the short observation period of many interventions may be attributed to the research funding structure, often relying on one-off grants with limited duration and insufficient structural support (56). Nonetheless, long-term results on maintenance and sustainability are crucial for reliable cost-effectiveness analysis, which policymakers and healthcare providers weigh when deciding whether or not to scale up and implement health interventions (16, 57).
Another issue was the lack of comprehensive information regarding Adoption, a multifaceted process involving two levels: setting and staff. Specifically, the descriptions of staff involvement were inadequate, potentially leading to a lack of clarity regarding the qualifications necessary to properly implement an intervention. Effective staff involvement is of paramount importance. Previous studies have highlighted the significance of implementation strategies such as education, training, and staff participation in decision-making in promoting successful implementation. Additionally, utilizing champions and opinion leaders to facilitate intervention implementation has been recommended in previous research (16, 58, 59). A lack of reporting on the components of Adoption and Maintenance makes it challenging to determine whether success can be attributed to the intervention itself, the elements of its implementation, or a combination of both. This consequently limits the prospects of disseminating results and thus extends the reach of an intervention (60).
Finally, an assessment of potential population health impact was conducted for six interventions, utilizing the RE-AIM average score as defined by Glasgow et al. (12). It is important to note that this score does not encompass all facets of the RE-AIM dimensions, necessitating caution in its interpretation. Two interventions resulted in the highest potential population impact scores, which may be linked to their higher participation rates in the Reach dimension (19, 20). This can in turn, be attributed to contacting eligible patients via email and telephone, as well as maintenance of extensive intervention contacts. These contacts, including navigator support, website and telephone services, were associated with significant reductions in risk factors for CMD. Moreover, these interventions consistently delivered all components as intended in their respective settings (19, 20).
4.1 Limitations of this review
Several limitations need to be taken into account when interpreting the results. Firstly, the search strategy was designed to capture information from English language publications only. Consequently, valuable publications utilizing other languages, housed in other databases, or employing alternative applicable MeSH terms may have been overlooked. Two widely used terms, “panel management” and “PHM,” were utilized to describe the proactive management of an entire population at risk of adverse outcomes. These terms are frequently used interchangeably in the literature, but their recent emergence suggests that a broader search might have yielded more publications. Secondly, current study focused exclusively on the RE-AIM framework and did not explore other frameworks such as the widely used Consolidated Framework for Implementation Research. This decision may have limited the exploration of potential barriers and facilitators to successful implementation. Nonetheless, as the objective was to better understand the potential of interventions for broader dissemination and adoption, the RE-AIM framework was intentionally selected because of its specific emphasis on assessing how interventions perform in real-world implementation settings. We acknowledge that the RE-AIM framework is not the only framework. Rather, it was used as an appropriate framework in which to present carefully systematized findings to enable readers to exercise their own discernment. Finally, another noteworthy limitation pertains to the scope of this review, which was centered on primary care. As the organization of primary care can vary considerably across different countries, it is prudent to exercise caution when applying the findings to countries with different healthcare systems. Nonetheless, it is worth emphasizing that the shared goal of providing accessible and appropriate care to all patients remains a common thread across these diverse settings.
4.2 Implications for research and practice
In line with the findings in this study, other health interventions tend to underreport aspects covered by RE-AIM dimensions, which may result in a poor understanding of the factors contributing to the success or failure of intervention implementation (15, 49–52). Providing clear, standardized documentation of the effectiveness of implementation would improve understanding of potential public health impacts and better inform future research efforts (16, 61). Moreover, a better understanding would help demonstrate practical impacts and potentially stimulate wider adoption of such interventions.
Decision-makers can use the population health impact score to assess the feasibility of implementing an intervention within their specific setting (55). However, caution is advised when interpreting an average score because it may not encompass all dimensions outlined in the RE-AIM framework. It may be more insightful to compare the scores for each dimension across different PHM interventions (62). This practical method allows for better visual communication with relevant stakeholders (63), providing a comprehensive view of intervention strengths and weaknesses regarding reach, effectiveness, adoption, implementation, and maintenance.
In conclusion, while many interventions did not fully report results across all RE-AIM dimensions, those that reported on Reach, Effectiveness, Adoption, Implementation, and Maintenance showed positive outcomes. Population Health Management interventions demonstrated their potential by reaching a significant number of participants and reducing CMD risk factors. Assessment of the RE-AIM average indicated that achieving the highest potential population health impact required reaching eligible participants through email or telephone, maintaining extensive intervention contacts via navigator support, website and telephone services, and consistently delivering all intended components within a specific setting. However, to further substantiate these results, reporting on adoption, implementation processes and the sustainability of these interventions must improve.
Data availability statement
The original contributions presented in the study are included in the article/Supplementary material, further inquiries can be directed to the corresponding author.
Author contributions
MR: Conceptualization, Formal analysis, Methodology, Project administration, Writing – original draft. NH: Conceptualization, Formal analysis, Writing – review & editing. SP: Formal analysis, Writing – review & editing. NC: Supervision, Writing – review & editing. HO: Funding acquisition, Supervision, Writing – review & editing. RV: Supervision, Writing – review & editing.
Funding
The author(s) declare financial support was received for the research, authorship, and/or publication of this article. The funding for this study was provided by Innovative Medical Devices Initiative (IMDI), LSHM21009. The funder had no role in the design and conduct of the study; collection, management, analysis, and interpretation of the data; preparation, review, or approval of the manuscript; and decision to submit the manuscript for publication.
Conflict of interest
The authors declare that the research was conducted in the absence of any commercial or financial relationships that could be construed as a potential conflict of interest.
The handling editor CL declared a past collaboration with the author NC.
Publisher’s note
All claims expressed in this article are solely those of the authors and do not necessarily represent those of their affiliated organizations, or those of the publisher, the editors and the reviewers. Any product that may be evaluated in this article, or claim that may be made by its manufacturer, is not guaranteed or endorsed by the publisher.
Supplementary material
The Supplementary material for this article can be found online at: https://www.frontiersin.org/articles/10.3389/fmed.2023.1275267/full#supplementary-material
References
1. Roth, GA, Mensah, GA, Johnson, CO, Addolorato, G, Ammirati, E, Baddour, LM, et al. Global burden of cardiovascular diseases and risk factors, 1990-2019: update from the GBD 2019 study. J Am Coll Cardiol. (2020) 76:2982–3021. doi: 10.1016/J.JACC.2020.11.010
2. Giedrimiene, D, and King, R. Burden of cardiovascular disease (CVD) on economic cost. Comparison of outcomes in US and Europe. Circ Cardiovasc Qual Outcomes. (2017) 10:10. doi: 10.1161/CIRCOUTCOMES.10.SUPPL_3.207
3. Van Dieren, S, Beulens, JWJ, Van Der Schouw, YT, Grobbee, E, and Neal, B. The global burden of diabetes and its complications: an emerging pandemic. Eur J Cardiovasc Prev Rehabil. (2010) 17:s3–8. doi: 10.1097/01.hjr.0000368191.86614.5a
4. Chatterjee, A, Harris, SB, Leiter, LA, Fitchett, DH, Teoh, H, and Bhattacharyya, OK. Cardiometabolic Risk Working Group (Canadian). Managing cardiometabolic risk in primary care: summary of the 2011 consensus statement. Can Fam Physician. (2012) 58:389–93, e196-201.
5. Kotseva, K, De Backer, G, De Bacquer, D, Rydén, L, Hoes, A, Grobbee, D, et al. Lifestyle and impact on cardiovascular risk factor control in coronary patients across 27 countries: results from the European Society of Cardiology ESC-EORP EUROASPIRE V registry. Eur J Prev Cardiol. (2019) 26:824–35. doi: 10.1177/2047487318825350
6. Wald, NJ, and Law, MR. A strategy to reduce cardiovascular disease by more than 80%. BMJ Br Med J. (2003) 326:1419–10. doi: 10.1136/BMJ.326.7404.1419
7. Rippe, JM. Lifestyle strategies for risk factor reduction, prevention, and treatment of cardiovascular disease. Am J Lifestyle Med. (2019) 13:204–12. doi: 10.1177/1559827618812395
8. Zwartkruis, VW, Groenewegen, A, Rutten, FH, Hollander, M, Hoes, AW, van der Ende, MY, et al. Proactive screening for symptoms: a simple method to improve early detection of unrecognized cardiovascular disease in primary care. Results from the lifelines cohort study. Prev Med Rep. (2020) 138:106143. doi: 10.1016/J.YPMED.2020.106143
9. Steenkamer, BM, Drewes, HW, Heijink, R, Baan, CA, and Struijs, JN. Defining population health management: a scoping review of the literature. Popul Health Manag. (2017) 20:74–85. doi: 10.1089/POP.2015.0149
10. Kuiper, JG, Bakker, M, Penning-Van Beest, FJA, and Herings, RMC. Existing data sources for clinical epidemiology: the PHARMO database network. Clin Epidemiol. (2020) 12:415–22. doi: 10.2147/CLEP.S247575
11. Smeets, HM, Kortekaas, MF, Rutten, FH, Bots, ML, Van Der Kraan, W, Daggelders, G, et al. Routine primary care data for scientific research, quality of care programs and educational purposes: the Julius general practitioners’ network (JGPN). BMC Health Serv Res. (2018) 18:1–9. doi: 10.1186/S12913-018-3528-5/TABLES/1
12. Glasgow, RE, Klesges, LM, Dzewaltowski, DA, Estabrooks, PA, and Vogt, TM. Evaluating the impact of health promotion programs: using the RE-AIM framework to form summary measures for decision making involving complex issues. Health Educ Res. (2006) 21:688–94. doi: 10.1093/HER/CYL081
13. Tricco, AC, Lillie, E, Zarin, W, O’Brien, KK, Colquhoun, H, Levac, D, et al. PRISMA extension for scoping reviews (PRISMA-ScR): checklist and explanation [internet]. Ann Intern Med American College of Physicians. (2018) 169:467–73. doi: 10.7326/M18-0850
14. Glasgow, RE, Harden, SM, Gaglio, B, Rabin, B, Smith, ML, Porter, GC, et al. RE-AIM planning and evaluation framework: adapting to new science and practice with a 20-year review. Front Public Heal. (2019) 7:64. doi: 10.3389/FPUBH.2019.00064/BIBTEX
15. Harden, SM, Gaglio, B, Shoup, JA, Kinney, KA, Johnson, SB, Brito, F, et al. Fidelity to and comparative results across behavioral interventions evaluated through the RE-AIM framework: a systematic review. Syst Rev. (2015) 4:1–13. doi: 10.1186/S13643-015-0141-0/TABLES/2
16. Gaglio, B, Shoup, JA, and Glasgow, RE. The RE-AIM framework: a systematic review of use over time. Am J Public Health. (2013) 103:e38–46. doi: 10.2105/AJPH.2013.301299
17. Glasgow, RE, Nelson, CC, Strycker, LA, and King, DK. Using RE-AIM metrics to evaluate diabetes self-management support interventions. Am J Prev Med. (2006) 30:67–73. doi: 10.1016/J.AMEPRE.2005.08.037
18. Singh, S, Price, H, Fayers, K, Leal, J, Donoghue, V, Hempenstall, J, et al. The WISDOM self-management intervention: a cost-effectiveness analysis to support the transformation of type 2 diabetes care in England. Diabet Med. (2022) 39:14928. doi: 10.1111/DME.14928
19. Ross, JAD, Barron, E, McGough, B, Valabhji, J, Daff, K, Irwin, J, et al. Uptake and impact of the English National Health Service digital diabetes prevention programme: observational study. BMJ Open Diabetes Res Care. (2022) 10:10. doi: 10.1136/BMJDRC-2021-002736
20. Plutzky, J, Benson, MD, Chaney, K, Bui, TV, Kraft, M, Matta, L, et al. Population health management of low-density lipoprotein cholesterol via a remote, algorithmic, navigator-executed program. Am Heart J. (2022) 243:15–27. doi: 10.1016/J.AHJ.2021.08.017
21. Sidebottom, AC, Benson, G, Vacquier, M, Pereira, R, Hayes, J, Boersma, P, et al. Population-level reach of cardiovascular disease prevention interventions in a rural community: findings from the heart of New Ulm project. Popul Health Manag. (2021) 24:86–100. doi: 10.1089/POP.2019.0196/ASSET/IMAGES/LARGE/POP.2019.0196_FIGURE2.JPEG
22. Wilson, KE, Michaud, TL, Almeida, FA, Schwab, RJ, Porter, GC, Aquilina, KH, et al. Using a population health management approach to enroll participants in a diabetes prevention trial: reach outcomes from the PREDICTS randomized clinical trial. Transl Behav Med. (2021) 11:1066–77. doi: 10.1093/TBM/IBAB010
23. Hickey, MD, Ayieko, J, Owaraganise, A, Sim, N, Balzer, LB, Kabami, J, et al. Effect of a patient-centered hypertension delivery strategy on all-cause mortality: secondary analysis of SEARCH, a community-randomized trial in rural Kenya and Uganda. PLoS Med. (2021) 18:e1003803. doi: 10.1371/JOURNAL.PMED.1003803
24. Kozlowska, O, Attwood, S, Lumb, A, Tan, GD, and Rea, R. Population health Management in Diabetes Care: combining clinical audit, risk stratification, and multidisciplinary virtual clinics in a community setting to improve diabetes Care in a Geographically Defined Population. An integrated diabetes care pilot in the north east locality, Oxfordshire, UK. Int J Integr Care. (2020) 20:21–11. doi: 10.5334/IJIC.5177
25. Baer, HJ, Rozenblum, R, De La Cruz, BA, Orav, EJ, Wien, M, Nolido, NV, et al. Effect of an online weight management program integrated with population health management on weight change: a randomized clinical trial. JAMA. (2020) 324:1737–46. doi: 10.1001/JAMA.2020.18977
26. Cykert, S, Keyserling, TC, Pignone, M, DeWalt, D, Weiner, BJ, Trogdon, JG, et al. A controlled trial of dissemination and implementation of a cardiovascular risk reduction strategy in small primary care practices. Health Serv Res. (2020) 55:944–53. doi: 10.1111/1475-6773.13571
27. Jazowski, SA, Bosworth, HB, Goldstein, KM, White-Clark, C, McCant, F, Gierisch, JM, et al. Implementing a population health management intervention to control cardiovascular disease risk factors. J Gen Intern Med. (2020) 35:1931–3. doi: 10.1007/S11606-020-05679-4
28. Jølle, A, Åsvold, BO, Holmen, J, Carlsen, SM, Tuomilehto, J, Bjørngaard, JH, et al. Basic lifestyle advice to individuals at high risk of type 2 diabetes: a 2-year population-based diabetes prevention study. The DE-PLAN intervention in the HUNT study, Norway. BMJ Open Diabetes Res Care. (2018) 6:000509. doi: 10.1136/BMJDRC-2018-000509
29. Van Houtven, CH, Greiner, MA, Heidenfelder, B, Spratt, SE, Granger, BB, Dunham, AA, et al. Medicare utilization, screening, and costs among participants in the southeastern diabetes initiative: a population-based evaluation. Prim Care Diabetes. (2018) 12:172–83. doi: 10.1016/J.PCD.2017.10.003
30. Wan, EYF, Fung, CSC, Jiao, FF, Yu, EYT, Chin, WY, Fong, DYT, et al. Five-year effectiveness of the multidisciplinary risk assessment and management Programme-diabetes mellitus (RAMP-DM) on diabetes-related complications and health service uses-a population-based and propensity-matched cohort study. Diabetes Care. (2018) 41:49–59. doi: 10.2337/DC17-0426
31. Ashburner, JM, Horn, DM, O’keefe, SM, Zai, AH, Chang, Y, Wagle, NW, et al. (2017). Chronic disease outcomes from primary care population health program implementation. Am J Manag Care. 23:728–735.
32. Price-Haywood, EG, Amering, S, Luo, Q, and Lefante, JJ. Clinical pharmacist team-based Care in a Safety net Medical Home: facilitators and barriers to chronic care management. Popul Health Manag. (2017) 20:123–31. doi: 10.1089/POP.2015.0177
33. Emerson, JF, Welch, M, Rossman, WE, Carek, S, Ludden, T, Templin, M, et al. A multidisciplinary intervention utilizing virtual communication tools to reduce health disparities: a pilot randomized controlled trial. Int J Environ Res Public Health. (2016) 13:31. doi: 10.3390/IJERPH13010031
34. Yu, EYT, Wan, EYF, Wong, CKH, Chan, AKC, Chan, KHY, Ho, SY, et al. Effects of risk assessment and management programme for hypertension on clinical outcomes and cardiovascular disease risks after 12 months: a population-based matched cohort study. J Hypertens. (2017) 35:627–36. doi: 10.1097/HJH.0000000000001177
35. Schwartz, MD, Jensen, A, Wang, B, Bennett, K, Dembitzer, A, Strauss, S, et al. Panel management to improve smoking and hypertension outcomes by VA primary care teams: a cluster-randomized controlled trial. J Gen Intern Med. (2015) 30:916–23. doi: 10.1007/S11606-015-3204-Y
36. Krantz, MJ, Coronel, SM, Whitley, EM, Dale, R, Yost, J, and Estacio, RO. Effectiveness of a community health worker cardiovascular risk reduction program in public health and health care settings. Am J Public Health. (2013) 103:e19–27. doi: 10.2105/AJPH.2012.301068
37. Evans, CD, Eurich, DT, Taylor, JG, and Blackburn, DF. The collaborative cardiovascular risk reduction in primary care (CCARP) study. Pharmacotherapy. (2010) 30:766–75. doi: 10.1592/PHCO.30.8.766
38. Clark, CM, Snyder, JW, Meek, RL, Stutz, LM, and Parkin, CG. A systematic approach to risk stratification and intervention within a managed care environment improves diabetes outcomes and patient satisfaction. Diabetes Care. (2001) 24:1079–86. doi: 10.2337/DIACARE.24.6.1079
39. Sidebottom, AC, Sillah, A, Miedema, MD, Vock, DM, Pereira, R, Benson, G, et al. Changes in cardiovascular risk factors after 5 years of implementation of a population-based program to reduce cardiovascular disease: the heart of New Ulm project. Am Heart J. (2016) 175:66–76. doi: 10.1016/J.AHJ.2016.02.006
40. Lewinski, AA, Bosworth, HB, Goldstein, KM, Gierisch, JM, Jazowski, S, McCant, F, et al. Improving cardiovascular outcomes by using team-supported, EHR-leveraged, active management: disseminating a successful quality improvement project. Contemp Clin trials Commun. (2021) 21:100705. doi: 10.1016/J.CONCTC.2021.100705
41. Jiao, FF, Fung, CSC, Wong, CKH, Wan, YF, Dai, D, Kwok, R, et al. Effects of the multidisciplinary risk assessment and management program for patients with diabetes mellitus (RAMP-DM) on biomedical outcomes, observed cardiovascular events and cardiovascular risks in primary care: a longitudinal comparative study. Cardiovasc Diabetol. (2014) 13:127. doi: 10.1186/S12933-014-0127-6
42. Strauss, SM, Jensen, AE, Bennett, K, Skursky, N, Sherman, SE, and Schwartz, MD. Clinicians’ panel management self-efficacy to support their patients’ smoking cessation and hypertension control needs. Transl Behav Med. (2015) 5:68–76. doi: 10.1007/S13142-014-0287-7
43. Smith, L, Atherly, A, Campbell, J, Flattery, N, Coronel, S, and Krantz, M. Cost-effectiveness of a statewide public health intervention to reduce cardiovascular disease risk. BMC Public Health. (2019) 19:1–8. doi: 10.1186/S12889-019-7573-8/TABLES/3
44. Sidebottom, AC, Sillah, A, Vock, DM, Miedema, MD, Pereira, R, Benson, G, et al. Assessing the impact of the heart of New Ulm project on cardiovascular disease risk factors: a population-based program to reduce cardiovascular disease. Prev Med. (2018) 112:216–21. doi: 10.1016/J.YPMED.2018.04.016
45. Wilson, D, Sheikh, A, Görgens, M, and Ward, K. Technology and universal health coverage: examining the role of digital health. J Glob Heal. (2021) 11:16006. doi: 10.7189/jogh.11.16006
46. Ross, J, Stevenson, F, Lau, R, and Murray, E. Exploring the challenges of implementing e-health: A protocol for an update of a systematic review of reviews. BMJ Open. 5:e006773. doi: 10.1136/bmjopen-2014-006773
47. Wilson, FA, Rampa, S, Trout, KE, and Stimpson, JP. Reimbursements for telehealth services are likely to be lower than non-telehealth services in the United States. J Telemed Telecare. (2016) 23:497–500. doi: 10.1177/1357633X16652288
48. Singh, K, Drouin, K, Newmark, LP, Jae, HL, Faxvaag, A, Rozenblum, R, et al. Many mobile health apps target high-need, high-cost populations, but gaps remain. Health Aff. (2016) 35:2310–8. doi: 10.1377/hlthaff.2016.0578
49. Racey, M, Markle-Reid, M, Fitzpatrick-Lewis, D, Ali, MU, Gagné, H, Hunter, S, et al. Applying the RE-AIM implementation framework to evaluate fall prevention interventions in community dwelling adults with cognitive impairment: a review and secondary analysis. BMC Geriatr. (2021) 21:1–15. doi: 10.1186/S12877-021-02376-7/TABLES/2
50. Compernolle, S, De Cocker, K, Lakerveld, J, Mackenbach, JD, Nijpels, G, Oppert, JM, et al. A RE-AIM evaluation of evidence-based multi-level interventions to improve obesity-related behaviours in adults: a systematic review (the SPOTLIGHT project). Int J Behav Nutr Phys Act. (2014) 11:1–13. doi: 10.1186/S12966-014-0147-3/FIGURES/2
51. Blackman, KCA, Zoellner, J, Berrey, LM, Alexander, R, Fanning, J, Hill, JL, et al. Assessing the internal and external validity of Mobile health physical activity promotion interventions: a systematic literature review using the RE-AIM framework. J Med Internet Res. (2013) 15:e224. doi: 10.2196/JMIR.2745
52. Bu, S, Smith, A, Janssen, A, Donnelly, C, Dadich, A, Mackenzie, LJ, et al. Optimising implementation of telehealth in oncology: a systematic review examining barriers and enablers using the RE-AIM planning and evaluation framework. Crit Rev Oncol Hematol. (2022) 180:103869. doi: 10.1016/J.CRITREVONC.2022.103869
53. Kist, JM, Smit, GWG, Mairuhu, ATA, Struijs, JN, Vos, RC, van Peet, PG, et al. Large health disparities in cardiovascular death in men and women, by ethnicity and socioeconomic status in an urban based population cohort. eClinicalMedicine. (2021) 40:101120. doi: 10.1016/j.eclinm.2021.101120
54. Powell-Wiley, TM, Baumer, Y, Baah, FO, Baez, AS, Farmer, N, Mahlobo, CT, et al. Social determinants of cardiovascular disease. Circ Res. (2022) 130:782–99. doi: 10.1161/CIRCRESAHA.121.319811
55. Glasgow, RE, Vogt, TM, and Boles, SM. Evaluating the public health impact of health promotion interventions: the RE-AIM framework. Am J Public Health. (1999) 89:1322–7. doi: 10.2105/AJPH.89.9.1322
56. Rakers, MM, van Os, HJA, Recourt, K, Mosis, G, Chavannes, NH, and Struijs, JN. Perceived barriers and facilitators of structural reimbursement for remote patient monitoring, an exploratory qualitative study. Heal Policy Technol. (2023) 12:100718. doi: 10.1016/J.HLPT.2022.100718
57. Milat, AJ, King, L, Newson, R, Wolfenden, L, Rissel, C, Bauman, A, et al. Increasing the scale and adoption of population health interventions: experiences and perspectives of policy makers, practitioners, and researchers. Heal Res policy Syst. (2014) 12:12. doi: 10.1186/1478-4505-12-18
58. Wells, S, Furness, S, Rafter, N, Horn, E, Whittaker, R, Stewart, A, et al. Integrated electronic decision support increases cardiovascular disease risk assessment four fold in routine primary care practice. Eur J Prev Cardiol. (2008) 15:173–8. doi: 10.1097/HJR.0b013e3282f13af4
59. Powell, BJ, Waltz, TJ, Chinman, MJ, Damschroder, LJ, Smith, JL, Matthieu, MM, et al. A refined compilation of implementation strategies: results from the expert recommendations for implementing change (ERIC) project. Implement Sci. (2015) 10:21. doi: 10.1186/S13012-015-0209-1
60. Tomoaia-Cotisel, A, Scammon, DL, Waitzman, NJ, Cronholm, PF, Halladay, JR, Driscoll, DL, et al. Context matters: the experience of 14 research teams in systematically reporting contextual factors important for practice change. Ann Fam Med. (2013) 11:S115–23. doi: 10.1370/AFM.1549
61. Glasgow, RE, and Estabrooks, PE. Pragmatic applications of RE-AIM for health care initiatives in community and clinical settings. Prev Chronic Dis. (2019) 15:E02. doi: 10.5888/PCD15.170271
62. Tunis, SR, Clarke, M, Gorst, SL, Gargon, E, Blazeby, JM, Altman, DG, et al. Improving the relevance and consistency of outcomes in comparative effectiveness research. J Comp Eff Res. (2016) 5:193–205. doi: 10.2217/CER-2015-0007
Keywords: population health management, cardiometabolic diseases, RE-AIM framework, population health impact score, modifiable risk factors
Citation: Rakers M, van Hattem N, Plag S, Chavannes N, van Os HJA and Vos RC (2024) Population health interventions for cardiometabolic diseases in primary care: a scoping review and RE-AIM evaluation of current practices. Front. Med. 10:1275267. doi: 10.3389/fmed.2023.1275267
Edited by:
Christos Lionis, University of Crete, GreeceReviewed by:
Luca Marino, Sapienza University of Rome, ItalyShooka Mohammadi, University of Malaya, Malaysia
Copyright © 2024 Rakers, van Hattem, Plag, Chavannes, van Os and Vos. This is an open-access article distributed under the terms of the Creative Commons Attribution License (CC BY). The use, distribution or reproduction in other forums is permitted, provided the original author(s) and the copyright owner(s) are credited and that the original publication in this journal is cited, in accordance with accepted academic practice. No use, distribution or reproduction is permitted which does not comply with these terms.
*Correspondence: Margot Rakers, bS5tLnJha2Vyc0BsdW1jLm5s