- 1Department of General Surgery (Gastrointestinal Surgery), The Affiliated Hospital of Southwest Medical University, Luzhou, China
- 2Department of Pediatrics, The Affiliated Hospital of Southwest Medical University, Luzhou, China
- 3Sichuan Clinical Research Center for Birth Defects, Luzhou, China
Objective: This study aims to elucidate the current status and trends in clinical decision support systems (CDSS). It will analyze the direction of research development in this field and provide valuable references for future research and the application of CDSS.
Methods: We conducted a search of the Web of Science Core Collection database from January 2014 to May 2024 to identify relevant literature on clinical decision support systems. CiteSpace (6.2. R4) software was utilized to visualize and analyze various aspects of the included literature, including publication volume, country of origin, authors, institutions, cited literature, keywords, and keyword clustering, and to generate corresponding graphs.
Results: A total of 2,668 articles were ultimately included in this study. The scholar with the highest number of publications is Professor Adam from the Department of Biomedical Information at Vanderbilt University in the United States. The top five countries contributing to this research are the United States, the United Kingdom, Germany, the Netherlands, and China. Based on an analysis of cited literature and keyword clustering, the research primarily focuses on predicting biochemical recurrence, cardiovascular disease, clinical guidelines, evidence-based computerized decision support systems, and intensive care units. The prominent topics in this field include artificial intelligence, natural language processing, venous thromboembolism, user-centered design, and emergency medicine.
Conclusion: Research on CDSS is demonstrating an upward trend and shows promising development prospects. Artificial intelligence, natural language processing, and user-centered design are the future trends.
1 Introduction
Clinical Decision Support Systems (CDSS) are computer-aided information systems that assist medical personnel in clinical diagnosis and treatment activities through information technology. By integrating medical knowledge with patient information, CDSS supports clinical decision-making (1). These systems offer intelligent services, including disease screening and diagnosis, disease warnings, medical order monitoring, adverse drug event alerts, and nursing support in healthcare (2–5). CDSS has been successfully implemented in various fields, such as venous thromboembolism, cardiovascular disease, and cancer, providing innovative solutions to diverse clinical challenges (6–8). Thus, there is an urgent need for a comprehensive, systematic, and clear understanding of the current international development status of CDSS.
Research on CDSS began in the late 1950s, culminating in the development of the world’s first CDSS at Stanford University in the mid-1970s (9). With the rapid advancement of electronic medical record (EMR) systems and artificial intelligence (AI), CDSS has increasingly become a significant application of AI in the medical field (10). The integration of AI technologies, including machine learning, neural network algorithms, and decision trees, with traditional CDSS allows for the rapid transformation of complex patient information into concise, organized data, facilitating support for clinical decision-making (11).
Quantitative research utilizing knowledge graphs can objectively illustrate the hotspots and emerging development trends within a specific research domain. To effectively assess the research status of CDSS, this article employs CiteSpace visualization software to analyze relevant literature from the past decade on an international scale. It aims to summarize the current research landscape, identify key hotspots, and outline future development directions in this field. This comprehensive understanding of CDSS is intended to serve as a reference for ongoing research and the sustainable advancement of CDSS in the future.
2 Data and methods
2.1 Source of information
This study utilized the Web of Science (WOS) core database as its primary literature source. The search period spanned from January 1, 2014, to May 1, 2024. A topic search was conducted using the formula TS = clinical decision support system OR clinical decision support systems, with filters applied for language (English) and literature type (article). The search results were subsequently exported in plain text format. Following a manual review to exclude irrelevant literature and deduplication, a total of 2,668 articles were obtained.
2.2 Research methods
In this study, the relevant literature was exported to the Web of Science (WOS) in plain text format and subsequently imported into the bibliometric software CiteSpace (version 6.2.R4). The analysis period (Time Slice) for CiteSpace is set from 2014 to 2024, with a single time partition (year per slice) configured to 1 year. The analysis aims to visualize and generate a graph based on various factors, including country, author, institution, cited literature, keywords, and keyword clustering.
3 Results
3.1 Analysis of publication volume
According to literature statistics from 2014 to 2024, the number of research articles on clinical decision support systems has demonstrated a consistent upward trend, rising from 157 in 2014 to 444 in 2023—more than doubling in this period. Especially in 2023, there will be a peak in publication volume. This indicates that research on clinical decision support systems is garnering increasing attention from the academic community. The distribution of research publications on clinical decision support systems is illustrated in Figure 1.
3.2 Analysis of national cooperation
Using CiteSpace software for analysis with countries as nodes, the results indicate that a total of 105 countries have published relevant literature. The top three countries in terms of publication frequency are the United States (1,108 articles), the United Kingdom (223 articles), and Germany (185 articles), which together account for 39.15% of the total publication volume. The top 10 countries in terms of publication volume collectively represent 63.95% of the total output (Table 1). The cooperation graph comprises 106 nodes and 330 connections, reflecting strong collaborative relationships among countries (Figure 2).
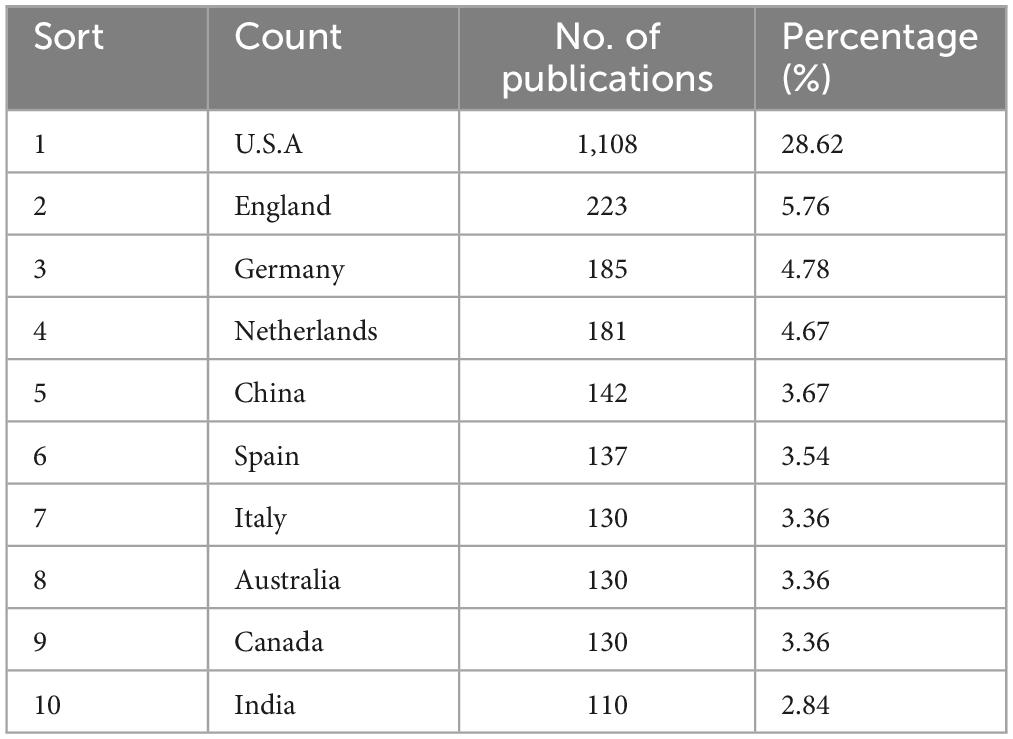
Table 1. Top 10 countries with the highest number of publications on decision support systems in the WOS database from 2014 to 2024.
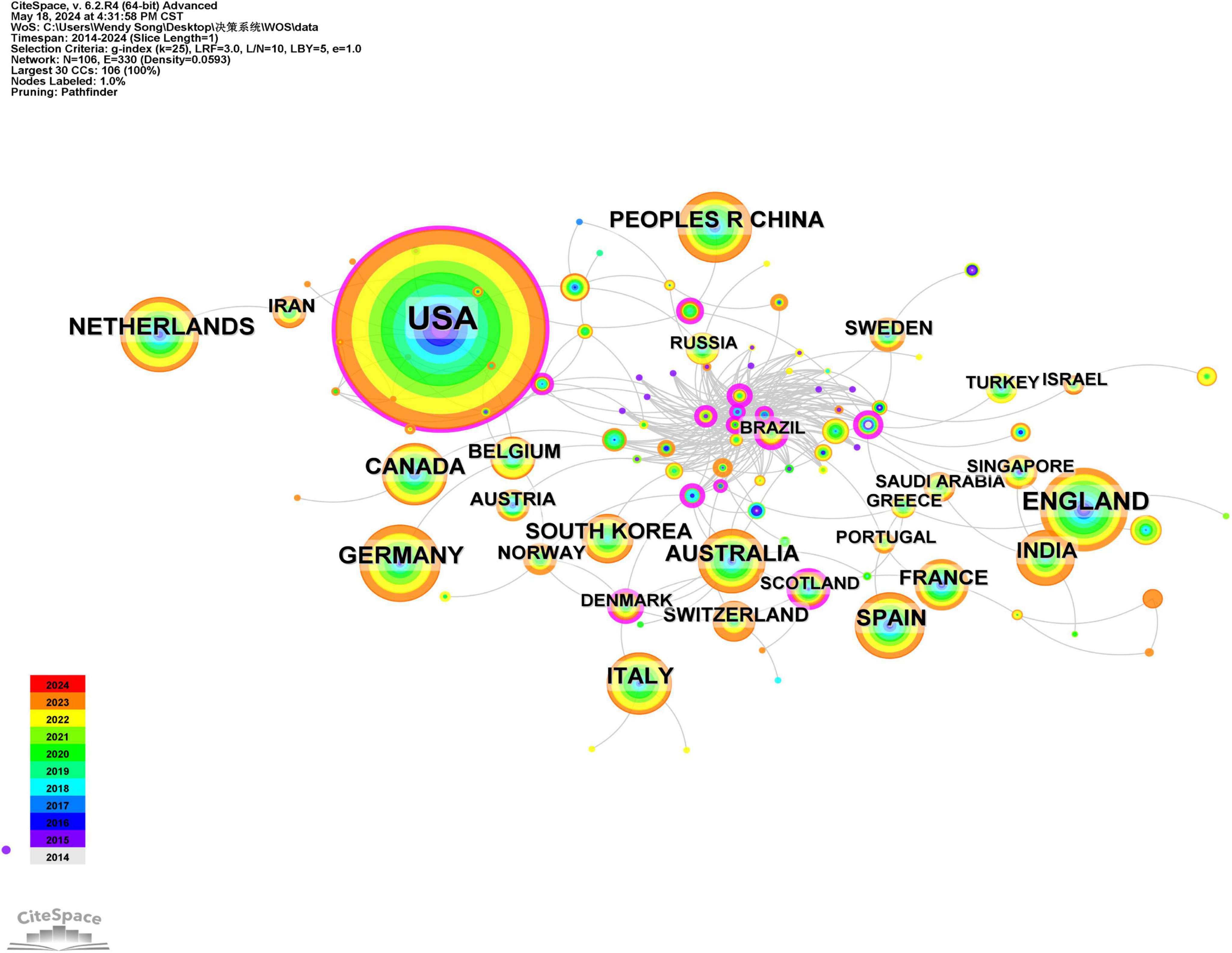
Figure 2. The national collaborative analysis atlas of clinical decision support systems in the WOS core set database from 2014 to 2024.
3.3 Author collaboration analysis
Using CiteSpace software, 491 authors have published literature related to clinical decision support systems, with the authors represented as nodes. The top three authors in terms of publication volume are Professor Wright and Adam from the Department of Biomedical Information at Vanderbilt University in the United States, Professor Bates and David W from Brigham and Women’s Hospital in the United States, and Professor O’Connor and Patrick J from the School of Education at the University of Georgia. Professor Wright and Adam have published 30 articles, ranking first. Their research primarily focuses on analyzing failures in clinical decision support systems and clinical medication safety warning reminders (12). Professor Bates, David W has published 29 articles, mainly concentrating on clinical decision support system fault analysis, electronic health records, and adverse drug event alerts (13). Professor O’Connor and Patrick J have published 19 articles, primarily focusing on the application of clinical decision support systems in managing chronic diseases such as hypertension, cardiovascular risk, diabetes, chronic kidney disease, and cancer prevention (14). The collaboration graph indicates a close working relationship between Professors Wright and Adam and Professors Bates and David W (15) (Figure 3).
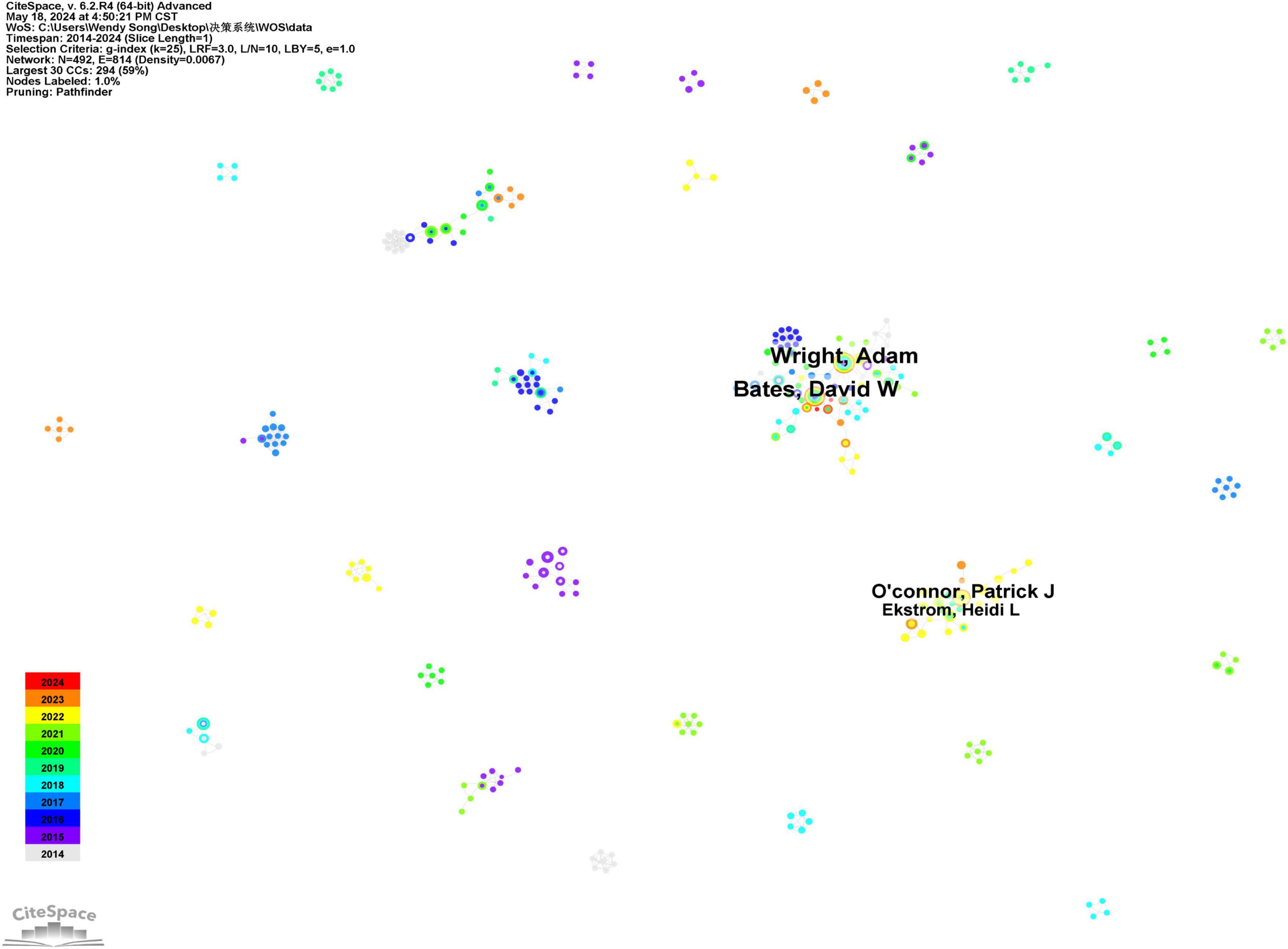
Figure 3. Author collaboration analysis graph of clinical decision support system in WOS core set database from 2014 to 2024.
3.4 Analysis of institutional cooperation
Using CiteSpace software, a total of 384 institutions published relevant literature, with these institutions represented as nodes. The top five institutions by publication volume are Harvard University, with 165 articles; Harvard Medical School, with 117 articles; the University of California System, with 110 articles; Brigham and Women’s Hospital, with 107 articles; and the Utah System of Higher Education, with 69 articles. These five institutions account for 12.79% of the total number of publications, while the top 10 institutions collectively account for 19.68% of the total publications, as illustrated in Table 2. Figure 4 depicts a collaboration graph that includes 384 nodes and 694 connections. The publishing institutions demonstrate close cooperation, particularly among the top five, which are all located in the same country, facilitating convenient collaboration.
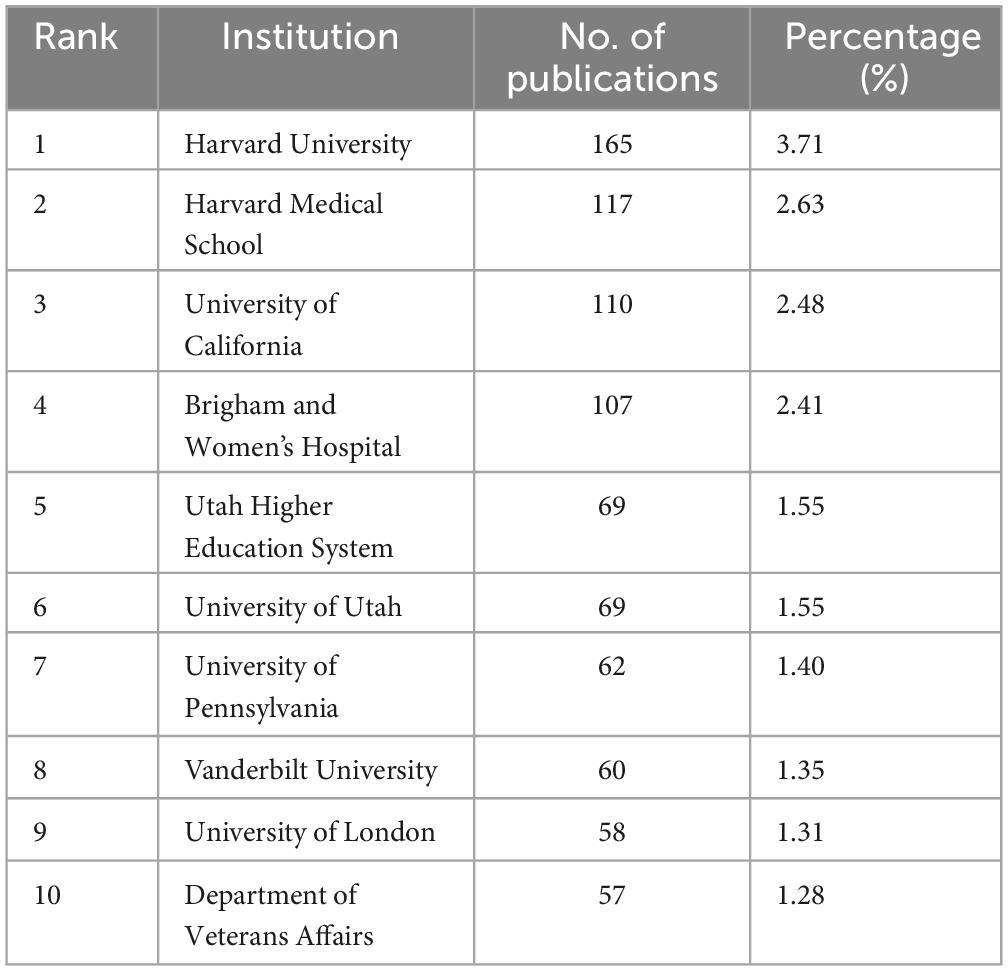
Table 2. Top 10 institutions with the highest number of publications on decision support systems in the WOS database from 2014 to 2024.
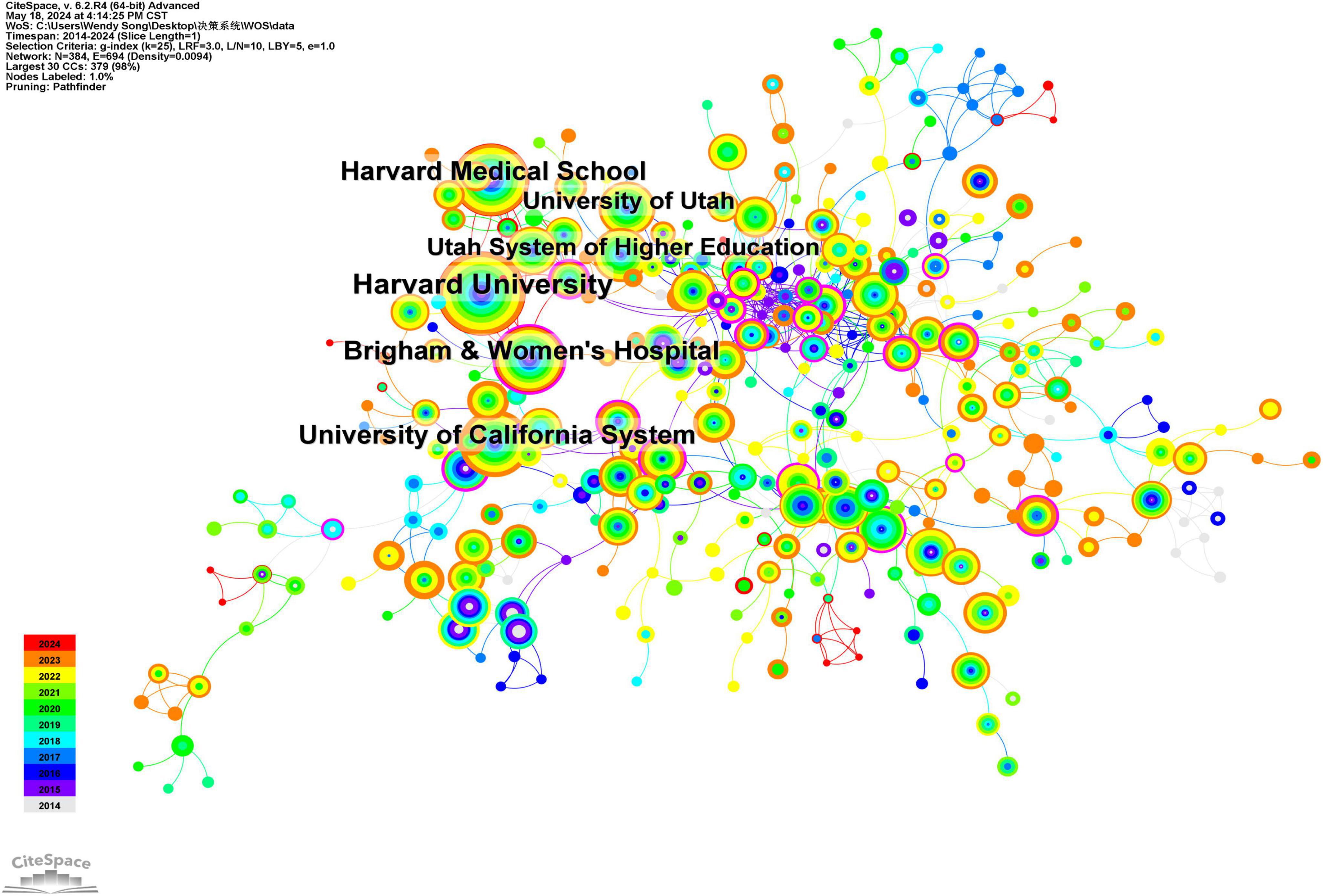
Figure 4. Clinical decision support system institutional collaboration analysis atlas in the WOS core set database from 2014 to 2024.
3.5 Citation analysis of cited literature
Reference citation refers to one reference is cited by at least two other references, allowing for the prediction of research hotspots and trends within a specific timeframe in a given research field. Utilizing CiteSpace software to analyze the cited literature as nodes, a total of 694 nodes and 1,068 connections were identified (Table 3; Figure 5). Between 2014 and 2024, 694 research papers in this domain were cited as core references. The top five cited references are ranked as follows: the first is a review of clinical decision support systems, which provides an overview of their application in medicine, encompassing various types, effective use cases, common pitfalls, and potential hazards. Evidence-based recommendations are proposed to mitigate risks in the design, implementation, evaluation, and maintenance of CDSS. The second most frequently cited article is a systematic review that discusses the role of CDSS, indicating that both commercially available and locally developed systems can effectively enhance various healthcare process measures. The third most frequently cited analysis primarily examines the reasons behind physicians’ reluctance to utilize CDSS, proposing two major models for CDSS design: user acceptance and system adaptive design models, along with input-output participation models. The fourth most frequently cited study is a retrospective cohort analysis investigating the impact of workload, work complexity, and repeated alerts on alert fatigue in CDSS, utilizing electronic health record data from 112 primary care physicians in outpatient settings. The findings reveal that clinicians are increasingly less likely to accept alerts due to the high volume of alerts received, particularly repeated ones. The fifth most frequently cited literature explores the relationship between computerized clinical decision support systems and absolute improvements in nursing, employing a meta-analysis.
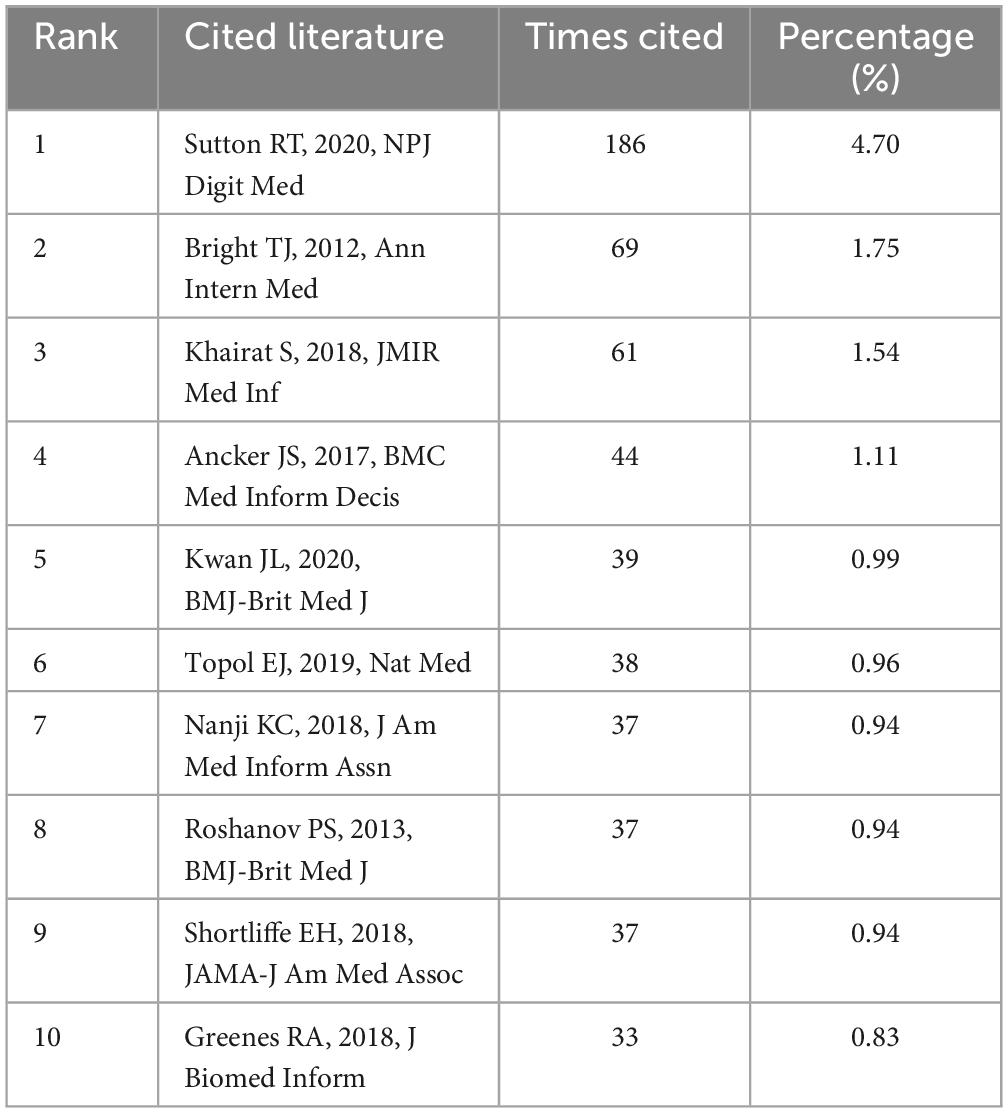
Table 3. The top 10 references with the highest citation frequency for decision support systems in the WOS database from 2014 to 2024.
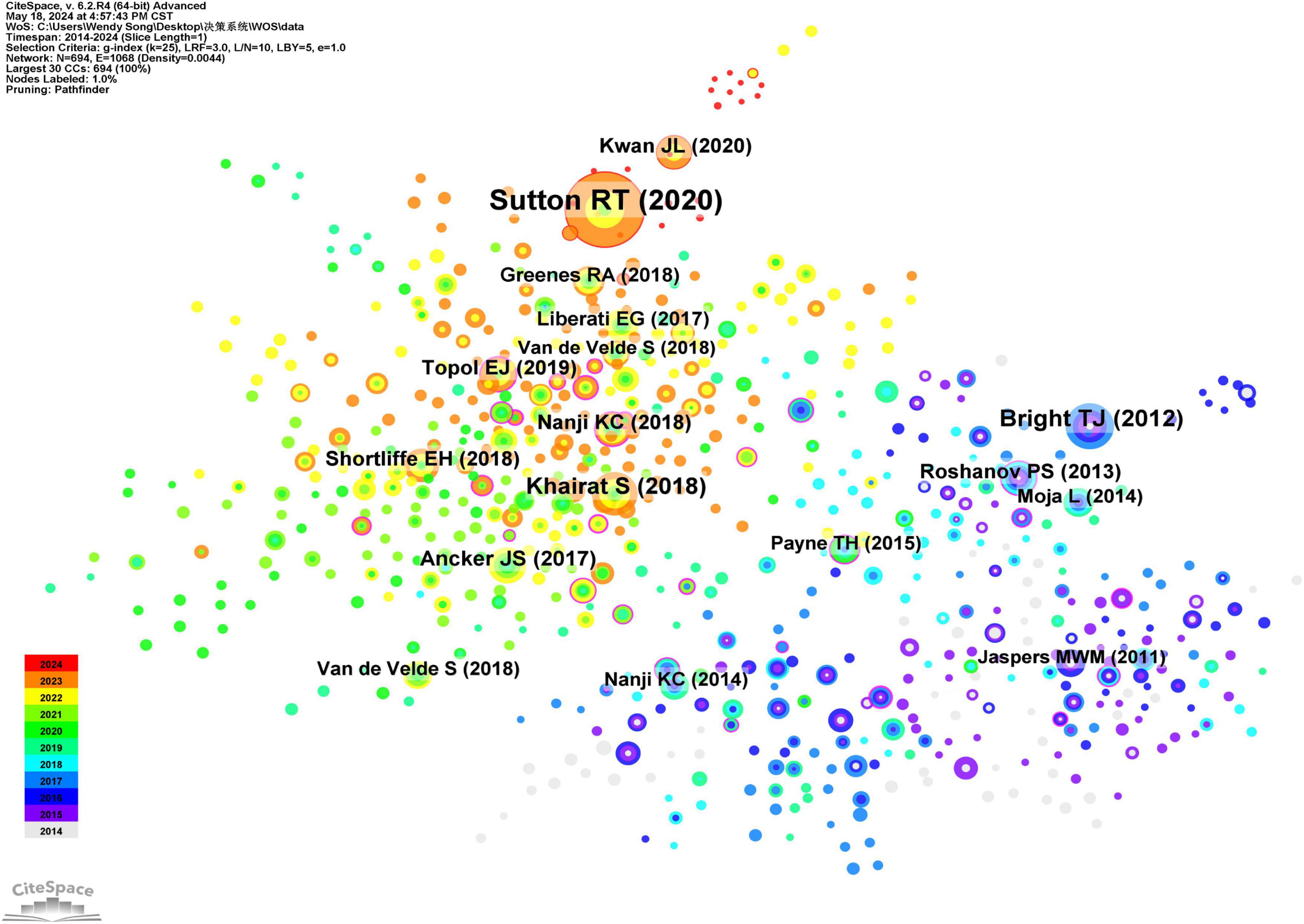
Figure 5. Collaborative analysis graph of highly cited coreliterature in the clinical decision support system of WOS core set database from 2014 to 2024.
3.6 Keyword colinear graph
Keywords are essential components of an article, reflecting the prevailing topics within the relevant field. Utilizing CiteSpace software for literature analysis, we identified 525 nodes and 795 connections (Figure 6). The top 20 keywords, based on the frequency of clinical decision support system-related literature published from 2014 to 2024, are summarized in Table 4. The keywords with high frequency of occurrence are categorized into three groups: ① Purpose of establishing CDSS—including clinical decision support, care, management, decision support, electronic health records, and primary healthcare; ② Functional Status and Design Optimization—encompassing systems, machine learning, decision support systems, and artificial intelligence; ③ Application situation—addressing impact, implementation, diagnosis, risk, and quality.
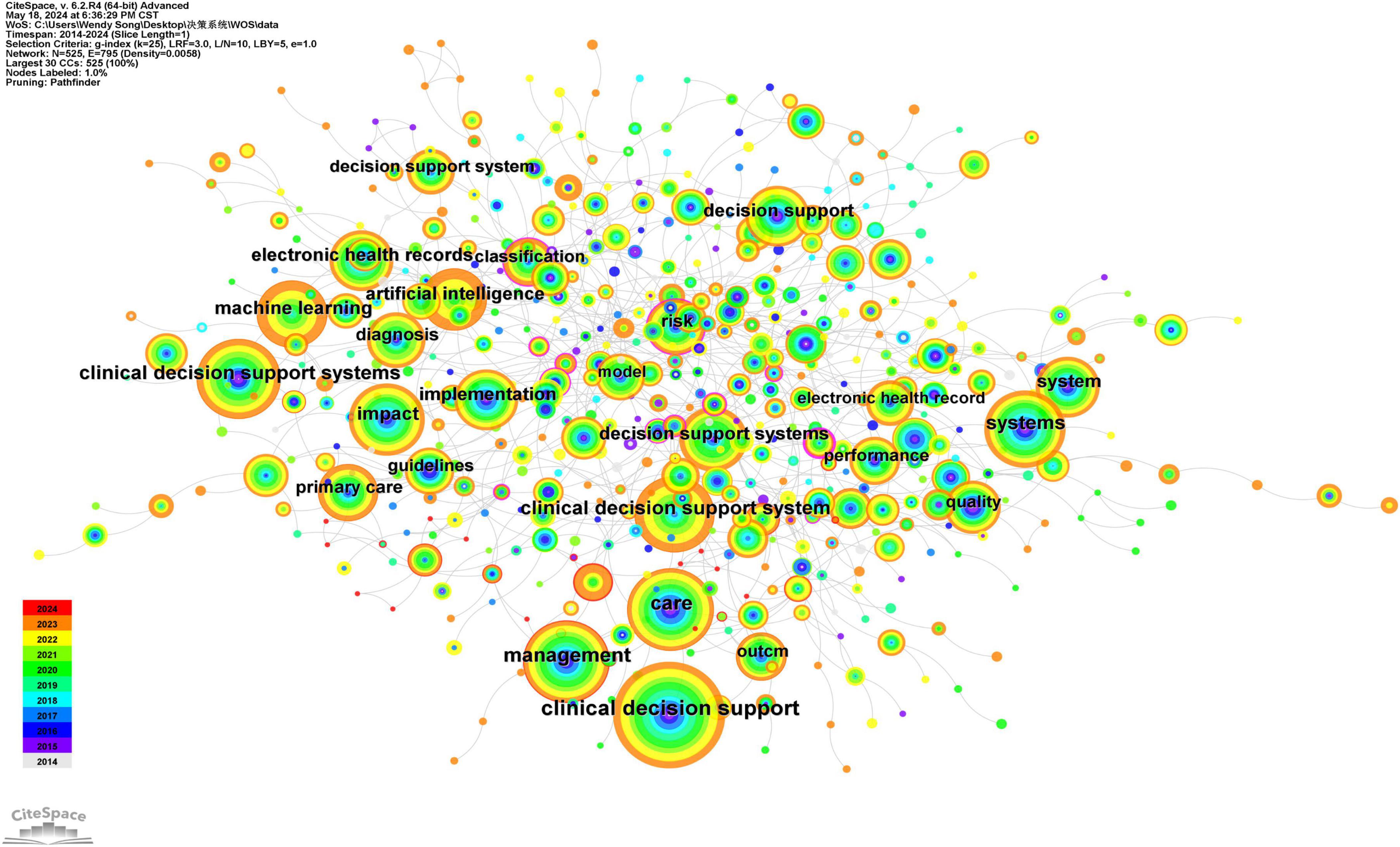
Figure 6. WOS core set database clinical decision support system keyword collinear network from 2014 to 2024.
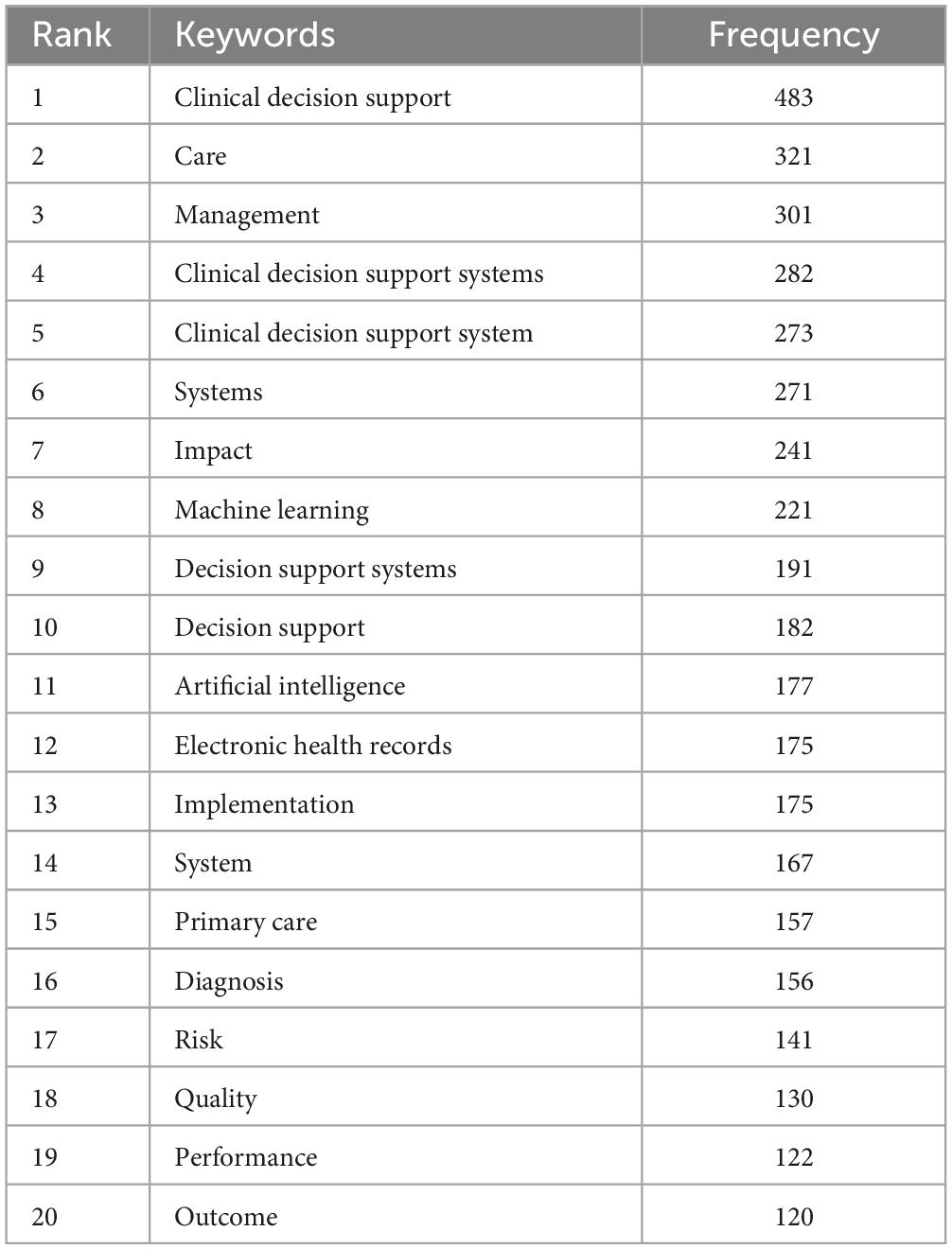
Table 4. The top 20 keywords in the frequency of decision support systems in the WOS database from 2014 to 2024.
3.7 Keyword clustering analysis
Based on the collinearity of keywords, a cluster analysis is conducted to group keywords into several clusters according to their thematic relevance. Each cluster represents a distinct focus within its respective research field, with larger clusters containing a greater number of keywords. The Q-value for keyword clustering in literature is 0.7531, and the S-value is 0.8891. This indicates that the clustering is reasonable. The top 10 keyword clusters (Figure 7) are as follows: #0 predicting biochemical recurrence, #1 cardiovascular diseases, #2 German university hospital, #3 clinical guidelines, #4 congestive heart failure, #5 evidence-based computerized decision support system, #6 intensive care unit, #7 emergency medicine physician, #8 hypertensive retinopathy, and #9 computer-based clinical decision support.
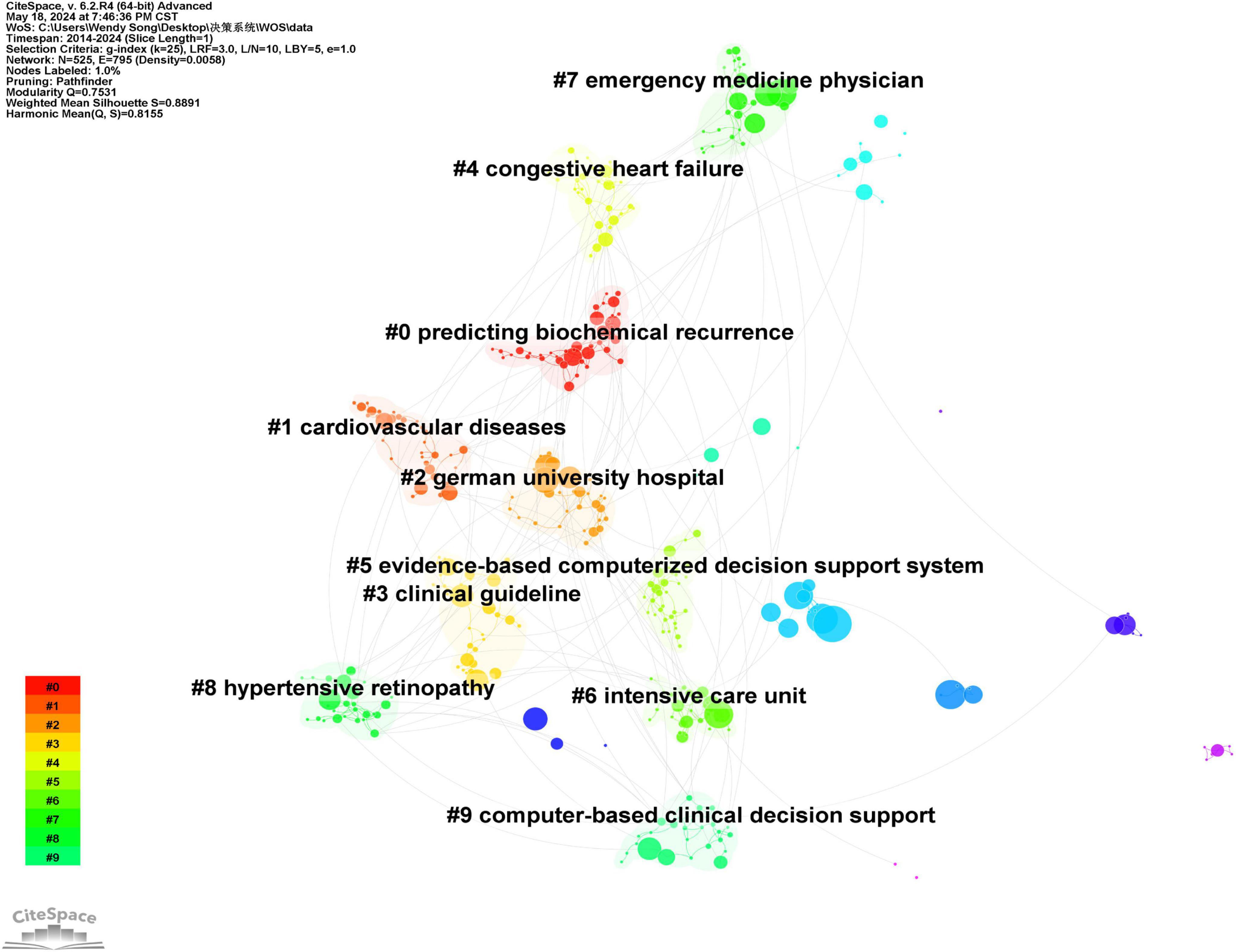
Figure 7. Keyword clustering map of clinical decision support system in WOS core set database from 2014 to 2024.
3.8 Timeline chart
The timeline for publishing papers related to clinical decision support systems from 2014 to 2024 indicates that keywords such as “diagnosis,” “management,” and “alerts” were prominent in 2014. In contrast, keywords like “digital health,” “natural language processing,” and “implementation science” emerged only in 2020 (Figure 8).
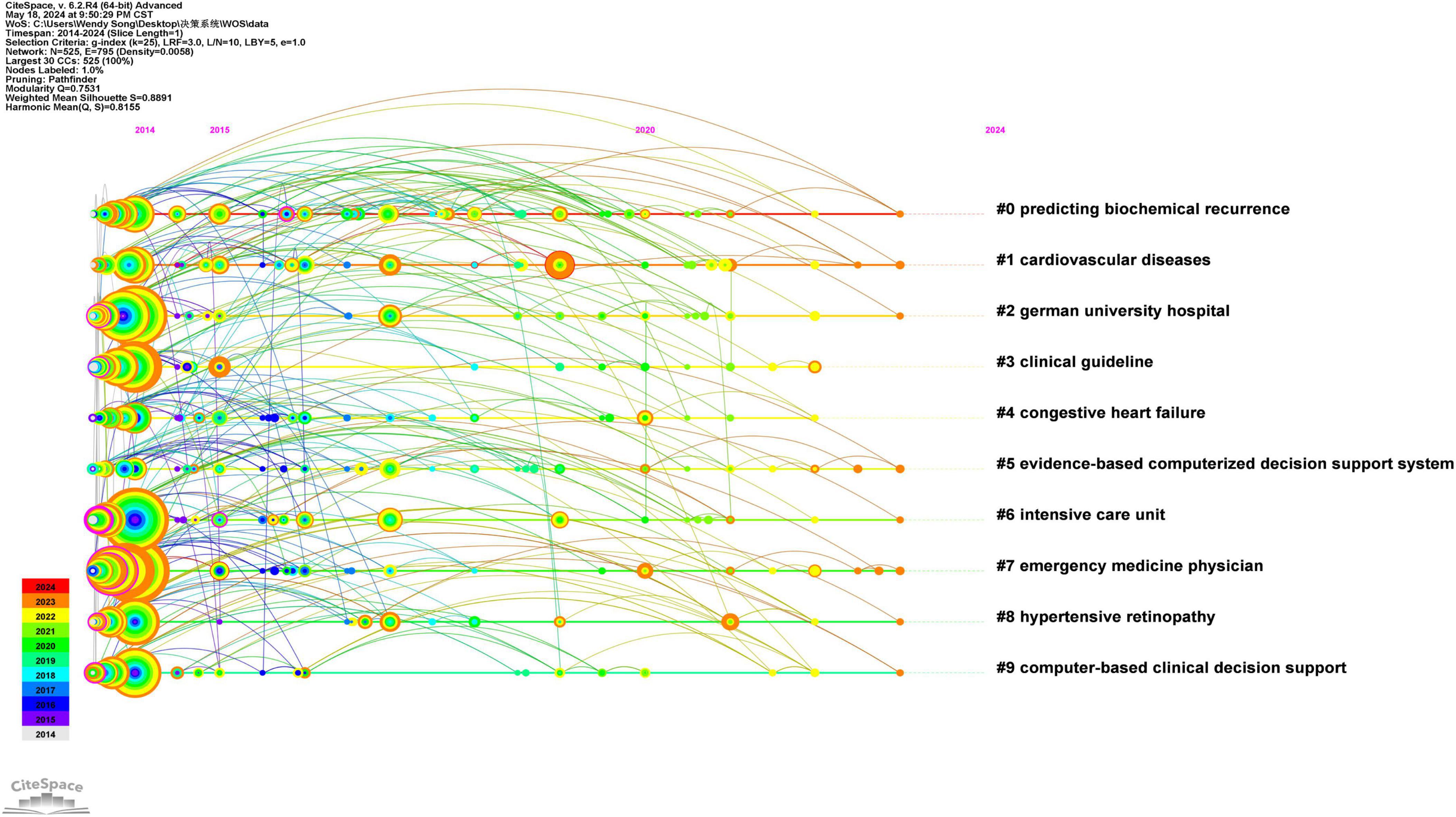
Figure 8. WOS core set database clinical decision support system keyword timeline from 2014 to 2024.
4 Discussion
According to the graph, the publication of research papers related to CDSS has demonstrated a consistent upward trend from 2014 to 2024. Prior to 2017, the growth rate was relatively slow; however, following a brief decline in 2018, the rate of increase has accelerated since 2019. Since 2014, the number of publications has consistently surpassed 150, reflecting a sustained rise in the international research community’s interest in CDSS. Regarding the countries of publication, there is a notable prevalence of contributions from European and American nations, particularly the United States, the United Kingdom, and Germany. This trend may be attributed to the early advancement of computer technology and the initial implementation of electronic medical record systems in these countries, which have fostered a conducive environment for the integration and evolution of CDSS (16, 17). In terms of authors and institutions, four CDSS institutions have each published over 100 articles. These institutions are located in the same country and maintain close collaborative ties; however, the collaboration among authors appears to be relatively fragmented. Globally, particularly in low- and middle-income countries, there remains a pressing need to further develop high-quality, shared, and open large-scale disease databases. Additionally, the organic integration of CDSS with other physical systems should be reinforced to enhance its adaptability. Furthermore, multi-disciplinary collaboration should be promoted to fully harness the potential and advantages of CDSS in disease management.
The concept of CDSS was first proposed in the 1960s (18, 19). Since 2014, the development of algorithm models for integrating CDSS with hospital electronic medical records has significantly increased (20–23). In May 2022, the DECIDE-AI expert group officially released the Guidelines for Early Clinical Evaluation Reports of AI-based Clinical Decision Support Systems (DECIDE-AI), which provide a comprehensive list of operational items for the early clinical evaluation of the actual performance and safety of CDSS (24, 25).
The results of the evolution analysis of cited literature, including keyword collinearity, clustering, mutation, and time dimension, indicate that CDSS research currently encompasses various fields such as hypertension, cardiovascular risk, diabetes, chronic kidney disease, cancer, rare diseases, and drug safety. This research predominantly manifests in the form of electronic alerts, clinician advice packages, and patient data reports, which serve as resources for clinicians. A substantial body of literature has validated the intervention effects of CDSS from diverse perspectives, including model performance, clinical behavior improvement, and patient prognosis (2–5).
CDSS can be categorized into two primary types based on their system structure. The first category is knowledge-based CDSS, which comprises three main components: a knowledge base, an inference engine, and a human-machine communication interface. Due to its closed nature and the absence of deep learning capabilities, this type of CDSS necessitates manual completion of data collection, compilation, organization, and rule establishment. Consequently, this results in high maintenance costs and limited timeliness for information updates (26). The second category consists of non-knowledge-based CDSS, often implemented in the form of artificial intelligence. These systems utilize artificial neural networks equipped with machine learning capabilities, allowing them to summarize and clarify knowledge through human-computer interaction and continuous training. They leverage knowledge data to offer suggestions to users. By delivering accurate decision recommendations through their efficient learning capabilities, non-knowledge-based CDSS represents a significant trend for future development (27).
According to the evaluation of module value and average contour value in keyword clustering analysis, a module value Q > 0.3 indicates a significant clustering structure, while an average contour value S > 0.7 suggests a strong clustering correlation and convincing results (28). The keyword clustering analysis conducted in this study yielded a Q-value of 0.753 and an S-value of 0.889, which underscores the reliability of the clustering outcomes. These results are consistent with the co-occurrence and mutation patterns observed in the keywords. Notably, clinical guidelines, evidence-based computerized decision support systems (CDSS), and computer-based clinical decision support have emerged prominently within the clustering results. Both domestic and international studies have demonstrated that guideline-based CDSS plays a crucial role in enhancing the quality of medical and nursing services, thereby improving patient clinical outcomes. CDSS is expected to leverage computer technologies such as artificial intelligence and machine learning to analyze and process extensive medical data—including clinical data, physiological parameters, genetic information, and imaging data—thus improving the accuracy and effectiveness of data analysis and decision support (29, 30). Moreover, greater emphasis should be placed on the user experience of CDSS, aiming to make system design more user-friendly and practical, which would facilitate easier interaction for clinical workers and enhance doctors’ recognition and acceptance of CDSS (31–33). In the future, artificial intelligence and machine learning will become important technologies for connecting massive medical data with precise treatment plans in the process of building CDSS (34). By deeply mining and analyzing massive medical database resources, integrating and utilizing heterogeneous data from multiple sources, a comprehensive disease knowledge network will be formed, and precise medical advice can be provided based on personalized patient characteristics. With the rapid growth of medical data and the rapid development of artificial intelligence technology, CDSS based on knowledge graph will more efficiently assist medical personnel in clinical decision-making.
5 Conclusion
CDSS-based disease diagnosis, system management, alerts, biochemical relapse prediction, clinical guidelines, and evidence-based computerized decision support systems are current research hotspots. Artificial intelligence, natural language processing, and user-centered design are the future trends.
6 Limitations
This study has limitations, due to its exclusive focus on literature from the WOS core database, which may introduce selection bias. Future research should continue to monitor the current status and development trends in CDSS research, while also exploring the refinement and analysis of relevant content across various subcategories of CDSS research.
Data availability statement
The original contributions presented in this study are included in this article/supplementary material, further inquiries can be directed to the corresponding author/s.
Ethics statement
Ethical review and approval was not required for the study on human participants in accordance with the local legislation and institutional requirements. Written informed consent for participation in this study was provided by the participants’ legal guardians/next of kin. Written informed consent was obtained from the participants’ legal guardians/next of kin for the publication of any potentially identifiable images or data included in this article.
Author contributions
MS: Conceptualization, Data curation, Investigation, Methodology, Software, Visualization, Writing – original draft. DL: Conceptualization, Data curation, Investigation, Project administration, Writing – review & editing.
Funding
The author(s) declare that financial support was received for the research and/or publication of this article. This study was supported by The Specialized Scientific Research Project on Wounds (Taige) of Sichuan Medical Association (Project No. 2024TG15).
Acknowledgments
We thank the reviewers for their helpful comments and for pointing out unclear and inaccurate statements contained in the manuscript.
Conflict of interest
The authors declare that the research was conducted in the absence of any commercial or financial relationships that could be construed as a potential conflict of interest.
Generative AI statement
The authors declare that no Generative AI was used in the creation of this manuscript.
Publisher’s note
All claims expressed in this article are solely those of the authors and do not necessarily represent those of their affiliated organizations, or those of the publisher, the editors and the reviewers. Any product that may be evaluated in this article, or claim that may be made by its manufacturer, is not guaranteed or endorsed by the publisher.
References
1. Tlili N, Robert L, Gerard E, Lemaitre M, Vambergue A, Beuscart J, et al. A systematic review of the value of clinical decision support systems in the prescription of antidiabetic drugs. Int J Med Inform. (2024) 191:105581. doi: 10.1016/j.ijmedinf.2024.105581
2. Muhiyaddin R, Abd-Alrazaq A, Househ M, Alam T, Shah Z. The impact of clinical decision support systems (CDSS) on physicians: A scoping review. Stud Health Technol Inform. (2020) 272:470–3. doi: 10.3233/SHTI200597
3. Del Fiol G, Rocha B, Nohama P. Design, implementation and evaluation of a clinical decision support system to prevent adverse drug events. Stud Health Technol Inform. (2000) 77:740–4. doi: 10.3233/978-1-60750-921-9-740
4. Dos Santos Diogo RC, Silva Butcher R, Peres H. Diagnostic concordance among nursing clinical decision support system users: A pilot study. J Am Med Inform Assoc. (2023) 30:1784–93. doi: 10.1093/jamia/ocad144
5. van Velzen M, de Graaf-Waar H, Ubert T, van der Willigen R, Muilwijk L, Schmitt M, et al. 21st century (clinical) decision support in nursing and allied healthcare. Developing a learning health system: A reasoned design of a theoretical framework. BMC Med Inform Decis Mak. (2023) 23:279. doi: 10.1186/s12911-023-02372-4
6. Ventura F, Sousa P, Dixe M, Ferreira P, Martinho R, Dias S, et al. A clinical decision support system for remote monitoring of cardiovascular disease patients: A clinical study protocol. Front Public Health. (2022) 10:859890. doi: 10.3389/fpubh.2022.859890
7. Jin Z, Zhang H, Tai M, Yang Y, Yao Y, Guo Y. Natural language processing in a clinical decision support system for the identification of venous thromboembolism: Algorithm development and validation. J Med Internet Res. (2023) 25:e43153. doi: 10.2196/43153
8. Gong E, Bang C, Lee J, Baik G, Lim H, Jeong J, et al. Deep learning-based clinical decision support system for gastric neoplasms in real-time endoscopy: Development and validation study. Endoscopy. (2023) 55:701–8. doi: 10.1055/a-2031-0691
9. Ledley R, Lusted L. Reasoning foundations of medical diagnosis; Symbolic logic, probability, and value theory aid our understanding of how physicians reason. Science. (1959) 130:9–21. doi: 10.1126/science.130.3366.9
10. Kwong J, Khondker A, Lajkosz K, McDermott M, Frigola X, McCradden M, et al. APPRAISE-AI tool for quantitative evaluation of AI studies for clinical decision support. JAMA Netw Open. (2023) 6:e2335377. doi: 10.1001/jamanetworkopen.2023.35377
11. Lang E, Neuschwander A, Favé G, Abback P, Esnault P, Geeraerts T, et al. Clinical decision support for severe trauma patients: Machine learning based definition of a bundle of care for hemorrhagic shock and traumatic brain injury. J Trauma Acute Care Surg. (2022) 92:135–43. doi: 10.1097/TA.0000000000003401
12. Rubins D, McCoy A, Dutta S, McEvoy D, Patterson L, Miller A, et al. Real-time user feedback to support clinical decision support system improvement. Appl Clin Inform. (2022) 13:1024–32. doi: 10.1055/s-0042-1757923
13. Cho I, Bates D. Behavioral economics interventions in clinical decision support systems. Yearb Med Inform. (2018) 27:114–21. doi: 10.1055/s-0038-1641221
14. Desai J, Saman D, Sperl-Hillen J, Pratt R, Dehmer S, Allen C, et al. Implementing a prediabetes clinical decision support system in a large primary care system: Design, methods, and pre-implementation results. Contemp Clin Trials. (2022) 114:106686. doi: 10.1016/j.cct.2022.106686
15. Samal L, Kilgallon J, Lipsitz S, Baer H, McCoy A, Gannon M, et al. Clinical decision support for hypertension management in chronic kidney disease: A randomized clinical trial. JAMA Intern Med. (2024) 184:484–92. doi: 10.1001/jamainternmed.2024.2589
16. Pearson S, Moxey A, Robertson J, Hains I, Williamson M, Reeve J, et al. Do computerised clinical decision support systems for prescribing change practice? A systematic review of the literature (1990-2007). BMC Health Serv Res. (2009) 9:154. doi: 10.1186/1472-6963-9-154
17. Wright A, Sittig D, Ash J, Sharma S, Pang J, Middleton B. Clinical decision support capabilities of commercially-available clinical information systems. J Am Med Inform Assoc. (2009) 16:637–44. doi: 10.1197/jamia.M3111
18. Evangelisti C, Goertzel G. The architecture of a decision support system. Int J Biomed Comput. (1985) 17:7–26. doi: 10.1016/0020-7101(85)90069-8
19. Goertzel G. Clinical decision support system. Ann N Y Acad Sci. (1969) 161:689–93. doi: 10.1111/j.1749-6632.1969.tb34100.x
20. Sutton R, Pincock D, Baumgart D, Sadowski D, Fedorak R, Kroeker K. An overview of clinical decision support systems: Benefits, risks, and strategies for success. NPJ Digit Med. (2020) 3:17. doi: 10.1038/s41746-020-0221-y
21. Ancker J, Edwards A, Nosal S, Hauser D, Mauer E, Kaushal R, et al. Effects of workload, work complexity, and repeated alerts on alert fatigue in a clinical decision support system. BMC Med Inform Decis Mak. (2017) 17:36. doi: 10.1186/s12911-019-0971-0
22. Khairat S, Marc D, Crosby W, Al Sanousi A. Reasons for physicians not adopting clinical decision support systems: Critical analysis. JMIR Med Inform. (2018) 6:e24. doi: 10.2196/medinform.8912
23. Kwan J, Lo L, Ferguson J, Goldberg H, Diaz-Martinez J, Tomlinson G, et al. Computerised clinical decision support systems and absolute improvements in care: Meta-analysis of controlled clinical trials. BMJ. (2020) 370:m3216. doi: 10.1136/bmj.m3216
24. Hamilton A. The future of artificial intelligence in surgery. Cureus. (2024) 16:e63699. doi: 10.7759/cureus.63699
25. Vasey B, Nagendran M, Campbell B, Clifton D, Collins G, Denaxas S, et al. Reporting guideline for the early-stage clinical evaluation of decision support systems driven by artificial intelligence: DECIDE-AI. Nat Med. (2022) 28:924–33. doi: 10.1038/s41591-022-01772-9
26. Seymour R, Wally M, Hsu J Primum Group. Impact of clinical decision support on controlled substance prescribing. BMC Med Inform Decis Mak. (2023) 23:234. doi: 10.1186/s12911-023-02314-0
27. Shaikh F, Dehmeshki J, Bisdas S, Roettger-Dupont D, Kubassova O, et al. Artificial intelligence-based clinical decision support systems using advanced medical imaging and radiomics. Curr Probl Diagn Radiol. (2021) 50:262–7. doi: 10.1067/j.cpradiol.2020.05.006
28. Liu Z, Yu Q, Liu H. Mesenchymal stem cells in heterotopic ossification in ankylosing spondylitis: A bibliometric study based on CiteSpace and VOSViewer. J Inflamm Res. (2023) 16:4389–98. doi: 10.2147/JIR.S421962
29. Wang L, Chen X, Zhang L, Li L, Huang Y, Sun Y, et al. Artificial intelligence in clinical decision support systems for oncology. Int J Med Sci. (2023) 20:79–86. doi: 10.7150/ijms.77205
30. Susanto A, Lyell D, Widyantoro B, Berkovsky S, Magrabi F. Effects of machine learning-based clinical decision support systems on decision-making, care delivery, and patient outcomes: A scoping review. J Am Med Inform Assoc. (2023) 30:2050–63. doi: 10.1093/jamia/ocad180
31. Jones N, Song S, Thomasian N, Samuels E, Ranney M. Behavioral health decision support systems and user interface design in the emergency department. Appl Clin Inform. (2023) 14:705–13. doi: 10.1055/s-0043-1771395
32. Gu Y, Andargoli A, Mackelprang J, Meyer D. Design and implementation of clinical decision support systems in mental health helpline Services: A systematic review. Int J Med Inform. (2024) 186:105416. doi: 10.1016/j.ijmedinf.2024.105416
33. Bright T, Wong A, Dhurjati R, Bristow E, Bastian L, Coeytaux R, et al. Effect of clinical decision-support systems: A systematic review. Ann Intern Med. (2012) 157:29–43. doi: 10.7326/0003-4819-157-1-201207030-00450
Keywords: clinical decision support system, hotspots, development trends, visual analysis, CiteSpace
Citation: Song M and Liu D (2025) International visualization analysis of research hotspots and development trends in the study of clinical decision support systems utilizing CiteSpace. Front. Med. 12:1546611. doi: 10.3389/fmed.2025.1546611
Received: 17 December 2024; Accepted: 11 April 2025;
Published: 28 April 2025.
Edited by:
Wei Wang, Sun Yat-sen University, ChinaReviewed by:
Miao Wu, Renmin Hospital of Wuhan University, ChinaJoyce W. Kuo, Stanford University, United States
Copyright © 2025 Song and Liu. This is an open-access article distributed under the terms of the Creative Commons Attribution License (CC BY). The use, distribution or reproduction in other forums is permitted, provided the original author(s) and the copyright owner(s) are credited and that the original publication in this journal is cited, in accordance with accepted academic practice. No use, distribution or reproduction is permitted which does not comply with these terms.
*Correspondence: Dong Liu, MTA0MDc5NjQzNUBxcS5jb20=