- 1Department of Ophthalmology, Xinjiang 474 Hospital, China RongTong Medical Healthcare Group CO. LTD, Urumqi, Xinjiang Uygur Autonomous Region, China
- 2Faculty of Arts and Sciences, Harvard University, Cambridge, MA, United States
- 3Department of Neurosurgery, The First Affiliated Hospital, Sun Yat-sen University, Guangzhou, Guangdong, China
- 4Department of Ophthalmology, TongRen Municipal People’s Hospital, Tongren, Guizhou, China
- 5Department of Neurology and Clinical Research Center of Neurological Disease, The Second Affiliated Hospital of Soochow University, Soochow University, Suzhou, Jiangsu, China
Visual impairment, stemming from genetic, degenerative, and traumatic causes, affects millions globally. Recent advancements in ophthalmology present novel strategies for managing and potentially reversing these conditions. Here, we explore 10 emerging avenues—including gene therapy, stem cell therapy, advanced imaging, novel therapeutics, nanotechnology, artificial intelligence (AI) and machine learning, teleophthalmology, optogenetics, bionics, and neuro-ophthalmology—all making strides to improve diagnosis, treatment, and vision restoration. Among these, gene therapy and stem cell therapy are revolutionizing the treatment of retinal degenerative diseases, while advanced imaging technologies enable early detection and personalized care. Therapeutic advancements like anti-vascular endothelial growth factor therapies and neuroprotective agents, along with nanotechnology, have improved clinical outcomes for multiple ocular conditions. AI, especially machine learning, is enhancing diagnostic accuracy, facilitating early detection, and personalized treatment strategies, particularly when integrated with advanced imaging technologies. Teleophthalmology, further strengthened by AI, is expanding access to care, particularly in underserved regions, whereas emerging technologies like optogenetics, bionics, and neuro-ophthalmology offer new hope for patients with severe vision impairment. In light of ongoing research, we summarize the current clinical landscape and the potential advantages of these innovations to revolutionize the management of visual impairments. Additionally, we address the challenges and limitations associated with these emerging avenues in ophthalmology, providing insights into their future trajectories in clinical practice. Continued advancements in these fields promise to reshape the landscape of ophthalmic care, ultimately improving the quality of life for individuals with visual impairments.
1 Introduction
Visual impairment remains a significant global health challenge, affecting over 1 billion people worldwide. It encompasses a wide range of etiologies, from congenital genetic disorders to acquired conditions such as age-related macular degeneration (AMD) and diabetic retinopathy, resulting in partial vision loss to total blindness (1, 2). Traditional treatments have predominantly focused on symptom management, often leaving the underlying causes of vision loss unaddressed (3). However, the field of ophthalmology is undergoing a profound transformation, marked by groundbreaking therapeutic innovations, advanced diagnostic technologies, and the integration of cutting-edge fields such as gene therapy, stem cell therapy, nanotechnology, and artificial intelligence (AI), as well as emerging interdisciplinary approaches that promise to redefine visual restoration and care (4–7). These innovations have the potential not only to halt the progression of visual impairment but also to restore vision in conditions previously considered untreatable (3, 4). This review provides a comprehensive overview of 10 key areas of advancement in ophthalmology: gene therapy, stem cell therapy, advanced imaging, novel therapeutics, nanotechnology, AI and machine learning, teleophthalmology, optogenetics, bionics, and neuro-ophthalmology (Figure 1). Each of these areas represents a significant leap forward in our ability to diagnose, treat, and potentially cure various forms of visual impairment and loss.
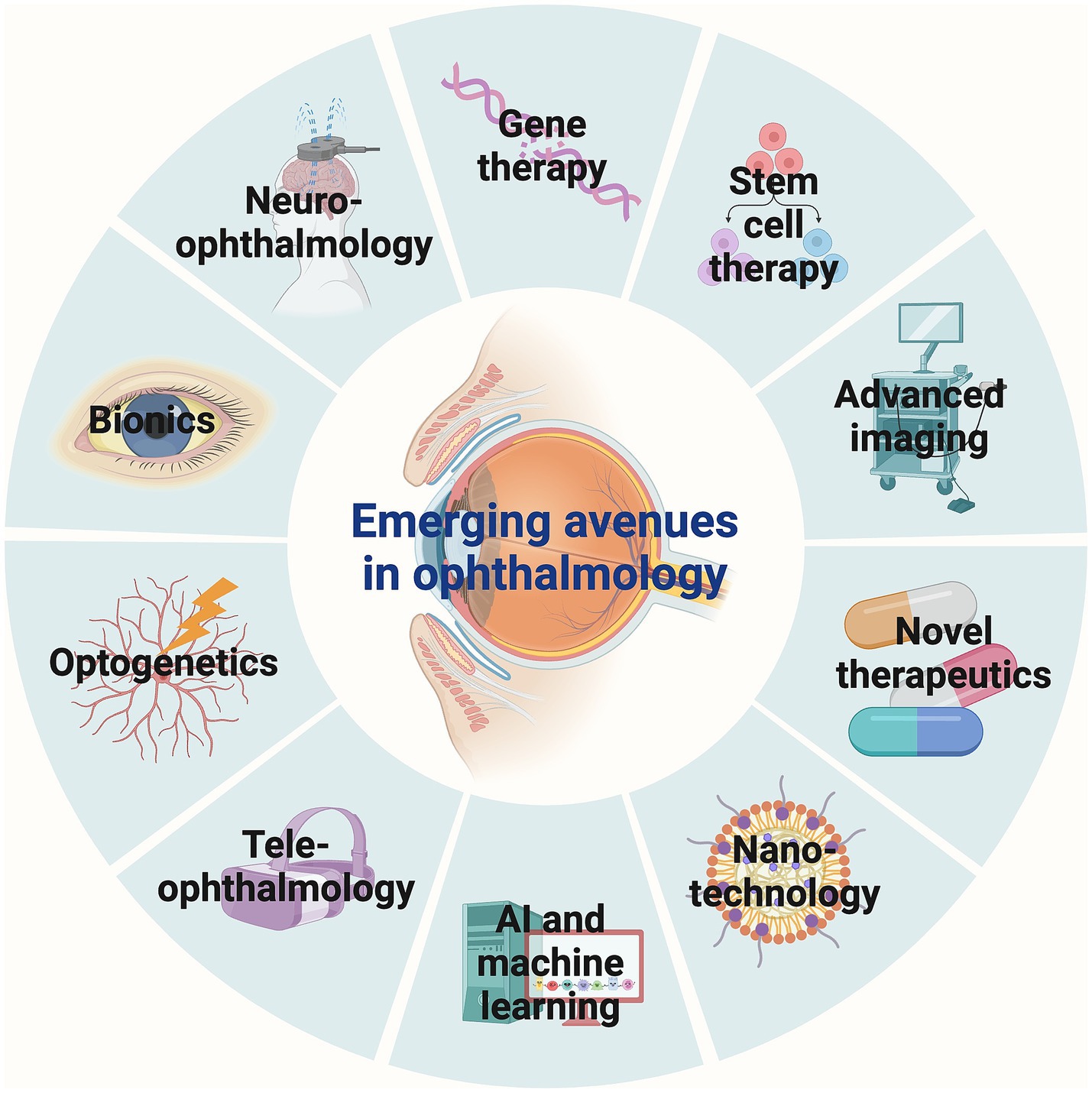
Figure 1. Emerging avenues in ophthalmology. Gene therapy, stem cell therapy, advanced imaging, novel therapeutics, nanotechnology, AI and machine learning, teleophthalmology, optogenetics, bionics, and neuro-ophthalmology represent 10 key areas of advancements in ophthalmology. AI, Artificial intelligence.
2 Gene therapy
Gene therapy has emerged as a groundbreaking approach for treating inherited retinal diseases caused by mutations in specific genes. These disorders, including retinitis pigmentosa, Leber congenital amaurosis (LCA), and achromatopsia, often lead to progressive vision loss and, in many cases, blindness (8). The underlying principle of gene therapy is to introduce functional copies of defective genes into affected cells, thereby correcting the genetic defect and restoring normal cellular function (9). One of the most significant developments in gene therapy for ophthalmology is the use of adeno-associated viruses (AAVs) as vectors to deliver therapeutic genes into retinal cells. AAVs are preferred due to their ability to effectively transduce retinal cells and their relatively low immunogenicity (10). The success of gene therapy in ophthalmology was exemplified by the Food and Drug Administration (FDA) approval of Luxturna® (voretigene neparvovec-rzyl) in 2017, the first gene therapy approved for an inherited disease (Figure 2) (11, 12). Luxturna® delivers a functional copy of the retinal pigment epithelium-specific 65 (RPE65) gene to the retinal pigment epithelium (RPE) cells of patients with LCA, leading to significant improvements in vision (13). Beyond RPE65, gene therapy is being explored for various other genetic eye conditions. For example, ongoing clinical trials are targeting the cyclic nucleotide-gated cation channel alpha-3 (CNGA3) and beta-3 (CNGB3) genes responsible for achromatopsia, with early results indicating safety and potential efficacy (14). Similarly, therapies targeting the vascular endothelial growth factor (VEGF) gene for wet AMD aim to reduce the frequency of anti-VEGF injections, which are currently the standard treatment. These trials utilize AAV vectors to deliver genes that produce anti-VEGF proteins within the eye, potentially providing a long-lasting therapeutic effect (15). For instance, Phase I/IIa of RGX-314 has been completed, with ongoing long-term follow-up. The study has shown that subretinal delivery of RGX-314 via a transvitreal approach is generally well-tolerated, without unusual immune responses or ocular inflammation (15, 16). Another area of active research is gene therapy for X-linked retinitis pigmentosa, a condition caused by mutations in the retinitis pigmentosa GTPase regulator (RPGR) gene. Initial trials using AAV vectors to deliver a functional RPGR gene have shown promising results, with some patients experiencing stabilization of their vision (17). A comprehensive list of clinical studies assessing different gene therapies in ophthalmology is provided in Table 1.
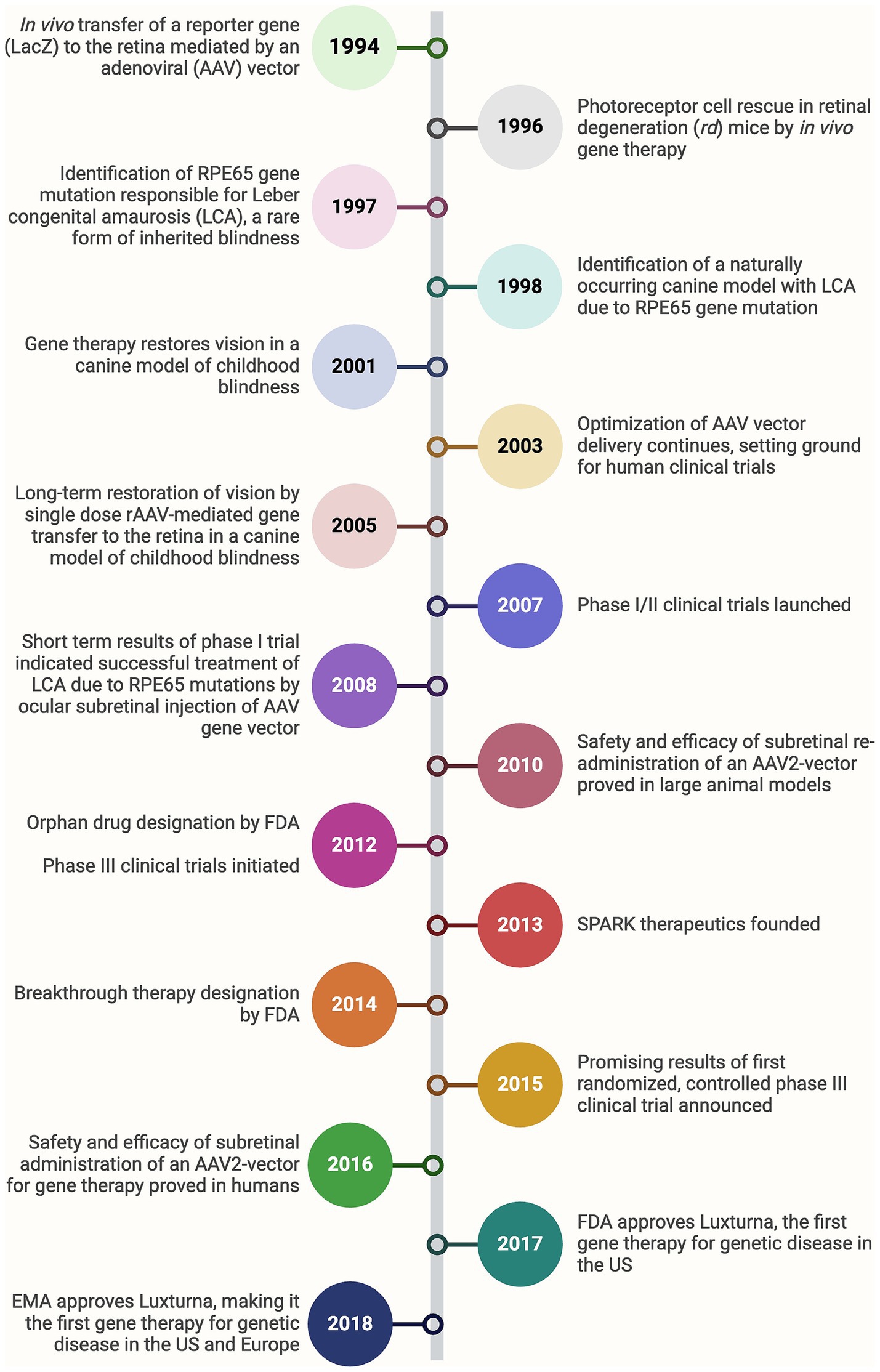
Figure 2. Bench-to-bedside timeline of the development of Luxturna, the first-ever gene therapy approved for a genetic disease. FDA, Food and drug administration; EMA, European medicine agency.
While gene therapy offers significant promise, several challenges must be addressed. The immune response to viral vectors poses a risk of inflammation and could reduce the effectiveness of the therapy (18, 19). Additionally, the long-term efficacy of these therapies remains under investigation, as retinal degeneration is a complex process that may require combination therapies to achieve optimal outcomes (20). The high upfront cost of gene therapies, such as Luxturna (~$850,000 for both eyes), raises accessibility concerns, yet their potential to provide a one-time treatment could yield long-term pharmacoeconomic benefits by reducing ongoing care costs and vision loss-related expenses (e.g., caregiving, lost productivity) (21). A 2025 analysis from Precision Medicine Online suggests that newer therapies like Nanoscope’s retinal gene therapy may be more cost-effective, with estimated costs of $67,400–$101,300, as supported by the Institute for Clinical and Economic Review (ICER) (22). However, comprehensive pharmacoeconomic studies remain limited, and challenges like manufacturing scalability and long-term efficacy require further investigation to fully assess their economic viability. Researchers are also exploring ways to enhance the efficiency and safety of gene delivery, such as using non-viral methods like nanoparticles, which may reduce immunogenicity and improve targeting precision (23). Advances in gene editing technologies, including CRISPR-Cas9, offer the potential for more precise and durable genetic corrections (24). A key example is the CRISPR-gene editing system called EDIT-101. In a small Phase I/II study, 14 individuals with CEP290-associated LCA received an EDIT-101 injection in one eye and were followed for 3 years. Results indicated improved daytime and central vision for six participants, enhanced vision with corrective lenses for four, and an overall increase in vision-related quality of life for six, with no serious adverse events (25). The future holds the potential for significant advancements in gene therapy through the integration of cutting-edge technologies and personalized medicine, aimed at enhancing the precision, efficacy, and accessibility of these treatments.
3 Stem cell therapy
Stem cells are undifferentiated cells with the ability to develop into various specialized cell types, including RPE cells and photoreceptors. This is why stem cell therapy offers exciting potential for addressing degenerative ocular diseases, particularly for restoring vision by replacing damaged or degenerated retinal cells (Table 2) (26, 27). One example of this is the use of limbal stem cells (LSCs) in treating limbal stem cell deficiency (LSCD), a condition characterized by impaired or insufficient LSCs, leading to symptoms such as dryness, reduced vision, and photophobia (28). Since Kenyon and Tseng first developed limbal tissue transplantation in 1989 (29), a range of techniques has evolved. One such technique, cultivated limbal epithelium transplantation (CLET), uses autologous limbal cells and has shown significant long-term success and safety. For instance, autologous CLET has been reported to have better long-term survival and fewer complications than allogenic CLET, such as chronic inflammation and scarring (30). Other approaches include simple limbal epithelial transplantation (SLET), which simplifies the process by using a small biopsy of healthy limbal tissue for transplantation. Sangwan et al. demonstrated that SLET allows for the in vivo expansion of LSCs, reducing the need for donor tissue and making the procedure more cost-effective (31). Additionally, an ongoing clinical trial is comparing the efficacy of CLET and SLET in patients with LSCD caused by ocular burns, further evaluating which technique offers superior outcomes. SLET’s cost-effectiveness and reduced need for donor tissue make it a valuable alternative for treating LSCD in resource-limited settings (32). Moreover, advancements such as using contact lenses as a scaffold for limbal stem cell cultures have shown promise in delivering stem cells to damaged corneas efficiently, while also being more accessible and cost-effective (33). These developments represent significant progress in stem cell-based treatments for LSCD, offering hope for patients with severe ocular surface disorders.
In retinal degenerative diseases, such as AMD and retinitis pigmentosa, stem cell-based therapies are advancing. One promising application is the replacement of RPE cells (34). RPE cells are essential for maintaining photoreceptors, and their degeneration leads to vision loss (35). Several studies have explored the transplantation of human embryonic stem cell (hESC)-derived RPE cells into the subretinal space. In a Phase I clinical study, patients with dry AMD showed improved visual acuity following the subretinal transplantation of hESC-derived RPE cells, with no signs of tumorigenicity or immune rejection over 4 months (36). In a separate study, a mismatched donor RPE monolayer implanted into a severely degenerated retina survived and functioned for 2 years, demonstrating limited immunogenicity of the allogeneic hESC-RPE cells (37). These early results suggest that RPE replacement can restore retinal function in AMD patients. Stargardt disease type 1 (STGD1), a hereditary form of macular degeneration, has also been a target for stem cell therapy. In a clinical trial involving the subretinal injection of hESC-derived RPE cell suspension, patients with early-stage STGD1 experienced no adverse reactions, although changes in visual function were variable (38). In another study using adipose-derived MSCs (ADMSCs) for suprachoroidal implantation, patients with STGD1 showed improvements in visual field and acuity. However, larger patient populations are needed to confirm the efficacy of this approach (39). In retinitis pigmentosa, characterized by the degeneration of rod and cone photoreceptors, early trials have focused on the safety and efficacy of transplanting retinal progenitor cells (RPCs). Patients with retinitis pigmentosa who received intravitreal injections of RPCs have shown improvements in visual acuity, although these gains were not sustained beyond 6 months (40). Alternatively, visual acuity score improvements for 50% of retinitis pigmentosa patients treated with neural RPC layers and RPE transplantation have been observed in another clinical trial (41). Additionally, neural precursor cell-derived astrocytes and RPE are being investigated for the treatment of retinitis pigmentosa (42), though extended research is needed to develop strategies that ensure long-term survival and integration of transplanted cells.
Glaucoma is another condition where stem cell therapy is being explored. The trabecular meshwork plays a crucial role in regulating intraocular pressure (IOP), and damage to trabecular meshwork cells leads to elevated IOP and optic nerve damage in glaucoma (43). Stem cell therapies, such as the injection of trabecular meshwork stem cells (TMSCs) into the anterior chamber, have shown potential in repopulating the trabecular meshwork and restoring its function. In studies using induced pluripotent stem cell-derived trabecular meshwork cells, both ex vivo and in vivo models demonstrated reduced IOP and restored TM function (44, 45). Notably, injecting mesenchymal stem cells (MSCs) directly into the ocular anterior chamber provides neuroprotection comparable to TMSC therapy. Based on a Phase I clinical study investigating trabecular meshwork regeneration (46), ADMSCs have been recommended for clinical trials due to their reduced risk of immune rejection and tumorigenesis (47). In a pilot study, intravenous injections of autologous bone marrow mesenchymal stem cells (ABMSCs) were administered to patients with diabetic retinopathy, a common complication of diabetes. Over 6 months, these patients showed reductions in macular thickness and improvements in visual acuity, suggesting that ABMSCs could offer a safe and effective treatment for diabetic retinopathy (48). Dry eye disease (DED), a multifactorial condition of the tear and ocular surface, is often associated with discomfort and vision impairment (49). Allogeneic ADMSCs injected into the lacrimal gland have been shown to reduce inflammation and improve symptoms in severe cases of DED. These stem cells can enhance tissue repair and modulate immune responses, providing relief to patients suffering from DED associated with conditions such as chronic graft-versus-host disease (cGVHD) (50). MSC-derived exosomes have also demonstrated efficacy in treating DED by alleviating inflammation and improving tear production when administered as eye drops. In addition, MSC-derived exosomes significantly reduced DED symptoms in patients with cGVHD (51), presenting a new approach for managing severe dry eyes.
Stem cell therapies, although promising, face challenges such as immune rejection, potential tumorigenicity, and the difficulty of ensuring long-term integration and function of transplanted cells (52, 53). However, ongoing developments in immune evasion strategies and the use of autologous stem cells may help mitigate some of these issues, enhancing the safety and efficacy of these therapies in the future (54). Pharmacoeconomic implications of stem cell therapies are also underexplored in current research. High initial costs for cell cultivation and delivery procedures may be offset by long-term savings if they halt disease progression and reduce the need for repeated interventions, potentially improving quality of life for patients with conditions like AMD (55). Studies suggest autologous approaches could lower costs, though scalability and regulatory hurdles pose economic challenges (36). Further pharmacoeconomic analyses are needed to quantify these benefits and guide clinical adoption.
4 Advanced imaging
Advanced imaging techniques have become indispensable in modern ophthalmology, offering critical insights into the structure and function of the retina and other ocular tissues (Figure 3 and Table 3) (56). Fluorescein angiography (FA), developed in the 1960s, was among the earliest techniques for visualizing retinal and choroidal blood flow. FA uses sodium fluorescein dye, which absorbs excitation light of wavelengths between 465 and 490 nm (blue) and emits light at wavelengths between 520 and 530 nm (yellow). A specialized camera captures detailed images of retinal blood vessels, enabling the detection of vascular abnormalities such as leakage and neovascularization. FA remains a valuable tool for diagnosing conditions like diabetic retinopathy and macular degeneration, providing essential insights into retinal vascular health (57, 58). Fundus photography, also emerging in the 1960s–1970s, captures detailed photographs of the retina, optic disc, and blood vessels. This technique is widely used for documenting and monitoring the progression of retinal and optic nerve diseases. Its ability to provide high-quality, wide-field images has made it a staple in ophthalmic diagnostics, facilitating long-term monitoring of conditions such as glaucoma and diabetic retinopathy (59). Indocyanine green angiography (ICG), introduced in the 1970s, provides an alternative to FA for visualizing the deeper choroidal circulation. ICG uses indocyanine green dye, which penetrates the RPE more effectively, making it particularly useful for diagnosing choroidal neovascularization and polypoidal choroidal vasculopathy. This technique offers a deeper view of the choroidal vasculature, providing insights into diseases affecting the deeper layers of the eye (60).
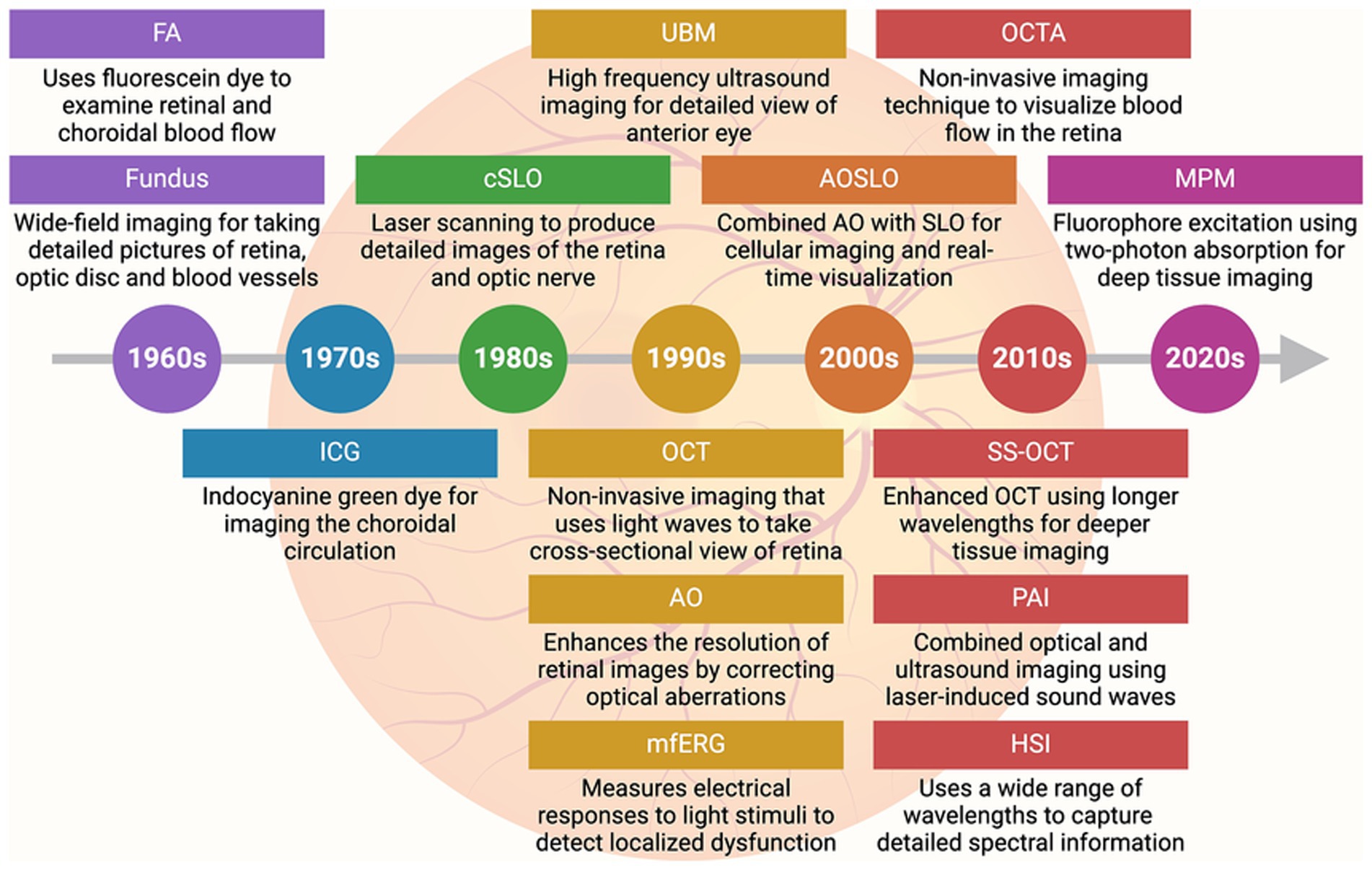
Figure 3. Advances in imaging technology in ophthalmology. Timeline (with brief description) of key imaging advancements made in the field of ophthalmology. FA, Fluorescein angiography; ICG, Indocyanine green angiography; cSLO, Confocal scanning laser ophthalmoscopy; UBM, Ultrasound biomicroscopy; OCT, Optical coherence tomography; AO, Adaptive optics; MfERG, Multifocal electroretinography; AOSLO, Adaptive optics scanning laser ophthalmoscopy; OCTA, Optical coherence tomography angiography; SS-OCT, Swept-source optical coherence tomography; PAI, Photoacoustic imaging; HSI, Hyperspectral imaging; MPM, Multiphoton microscopy.
Confocal scanning laser ophthalmoscopy (cSLO), developed in the 1980s, uses laser scanning to produce high-contrast images of the retina and optic nerve. It is highly effective for diagnosing and managing glaucoma, macular degeneration, and diabetic retinopathy (61). Recent methods have enabled real-time visualization of retinal ganglion cells (RGCs) using cSLO, allowing for longitudinal studies to monitor RGC survival and disease progression or remission following treatment (62). Ultrasound biomicroscopy (UBM), introduced in the 1990s, provides high-frequency ultrasound imaging of the anterior segment of the eye, including the iris, ciliary body, and anterior chamber angle. UBM has proven useful in diagnosing and managing glaucoma and anterior segment tumors (63). Advancements toward three-dimensional (3D) UBM have enabled detailed visualization and measurement of anterior eye tissues in a 3D context, aiding in treatment planning such as lens placement and microcatheter cannulation (64). Optical coherence tomography (OCT), a major breakthrough of the 1990s, revolutionized ophthalmic imaging by using light waves to capture high-resolution, cross-sectional images of the retina. OCT allows clinicians to visualize retinal layers in great detail, making it a cornerstone in diagnosing and managing AMD, diabetic retinopathy, and glaucoma. By detecting subtle changes in retinal thickness and other pathological features, OCT facilitates early diagnosis and intervention, crucial for preserving vision (65). Multifocal electroretinography (mfERG), also developed in the 1990s, measures the electrical responses of different areas of the retina to light stimuli, providing a functional assessment of localized retinal regions. Unlike traditional ERG, mfERG can detect localized dysfunction, making it especially useful for diagnosing and monitoring early diabetic retinopathy and retinitis pigmentosa (66).
Adaptive optics (AO), introduced in the 1990s, has been instrumental in enhancing retinal imaging by correcting optical aberrations caused by the eye’s optics. AO enables detailed imaging of photoreceptors and other fine retinal structures, providing new insights into the pathophysiology of retinal diseases (67). The combination of AO with scanning laser ophthalmoscopy (AOSLO), developed in the 2000s, further enhanced the resolution and contrast of retinal images. AOSLO allows real-time visualization of cellular structures in the retina, which is particularly valuable for monitoring inherited retinal diseases, such as retinitis pigmentosa (68). This technique is also used to assess the efficacy of gene and stem cell therapies at the cellular level (69). Optical coherence tomography angiography (OCTA), developed in the 2010s, expanded OCT capabilities by allowing visualization of retinal and choroidal blood flow without dye injection. OCTA provides detailed maps of the retinal vasculature, making it useful for detecting ischemia, neovascularization, and vascular abnormalities in conditions like AMD and diabetic retinopathy. It also enables non-invasive monitoring of treatment efficacy and disease progression (70). Swept-source optical coherence tomography (SS-OCT), introduced in the 2010s, is an advanced form of OCT that uses longer wavelengths for deeper tissue imaging. SS-OCT allows faster image acquisition and better visualization of the choroid and deeper retinal structures, making it highly valuable for diagnosing diseases affecting these areas (71). Photoacoustic imaging, also developed in the 2010s, combines optical and ultrasound imaging using laser-induced sound waves. This technique provides insights into oxygen saturation and blood vessel networks in the retina, aiding in the early detection of tumors and vascular abnormalities (72, 73). Hyperspectral imaging, emerging in the 2010s and continuing into the 2020s, uses a wide range of wavelengths to capture detailed spectral information about ocular tissues. This technique has been particularly useful in detecting early changes in diabetic retinopathy and AMD, as it provides metabolic and functional data alongside structural imaging (74). Finally, multiphoton microscopy, gaining traction in the 2020s, is an advanced microscopy technique that excites fluorophores using two-photon absorption. It allows high-resolution, deep tissue imaging, which is useful for tracking cellular activity in vivo (75, 76). This technique has opened new possibilities for understanding the cellular mechanisms underlying retinal diseases and their response to treatments (77). As these technologies continue to evolve, they hold the potential to enhance early diagnosis, guide personalized treatments, and improve long-term outcomes for patients with ocular diseases.
5 Novel therapeutics
Therapeutic advancements are making significant strides in improving the treatment of various ocular diseases. Among these, anti-angiogenesis, anti-fibrosis, and neuroprotection stand out as major areas of focus (Table 4) (78, 79). Anti-VEGF drugs have revolutionized the treatment of neovascular AMD and diabetic macular edema (DME) (79). These conditions, characterized by abnormal blood vessel growth and fluid leakage in the retina, can lead to severe vision loss if left untreated. Anti-VEGF therapies work by inhibiting VEGF, a protein that promotes the growth of these abnormal vessels. By blocking VEGF, these drugs help reduce fluid accumulation, stabilize the retina, and prevent further vision deterioration (80, 81). The most widely used anti-VEGF drugs include ranibizumab (Lucentis) and aflibercept (Eylea), both of which have demonstrated efficacy in numerous clinical trials (82–84). However, high per-dose costs (e.g., $1,000–$2,000 per injection) and the frequent dosing schedules required for these treatments—often involving monthly injections—pose a significant burden for patients and healthcare systems (82–84). This has driven the development of newer formulations and delivery methods, such as extended-release options, to reduce the frequency of injections while maintaining or improving treatment efficacy in AMD (85, 86). Brolucizumab (Beovu) is a newer anti-VEGF drug that has garnered attention for its longer duration of action compared to earlier treatments. Approved by the FDA in 2019 for the treatment of neovascular AMD, brolucizumab offers the advantage of less frequent dosing, potentially reducing the treatment burden for patients (87). Clinical trials, including the HAWK and HARRIER studies, have demonstrated that brolucizumab is effective in reducing retinal fluid and improving vision in patients with neovascular AMD, with some patients able to extend their treatment intervals to 12 weeks or more (88). These findings suggest that brolucizumab could offer a more convenient treatment option for patients, particularly those who struggle with the logistics and discomfort of frequent injections. Overall, these therapies may justify their expense by delaying vision loss, yet specific cost-effectiveness data is lacking (89). Future research should focus on quantifying long-term economic benefits to optimize their clinical use.
Targeting transforming growth factor-beta (TGF-β) for ocular conditions has also gained attention in recent years due to its involvement in fibrosis and abnormal vascularization in the retina (90). In this context, ISTH0036, an antisense oligonucleotide targeting TGF-β2, is progressing in trials for treating retinal diseases. In a Phase I trial involving glaucoma patients undergoing trabeculectomy, intravitreal ISTH0036 was well-tolerated with no related adverse events and effectively maintained IOP below 10 mmHg (91). In an ongoing Phase II study, ISTH0036 has shown potential in reducing fibrosis and fluid volumes in treatment-naïve and anti-VEGF-pretreated patients with wet AMD and DME. The treatment led to improved best-corrected visual acuity and reduced central retinal thickness, with stable IOP and minimal cataract worsening (92). These results highlight ISTH0036’s promise as a novel anti-fibrotic therapy for retinal conditions.
Neuroprotective agents represent a promising approach for treating neurodegenerative eye diseases, such as glaucoma and optic neuropathies (93). Glaucoma is characterized by the progressive degeneration of RGCs and their axons, which form the optic nerve. Current treatments for glaucoma primarily focus on lowering IOP, the main modifiable risk factor for the disease (94). However, lowering IOP alone does not prevent disease progression in all patients, particularly those with normal-tension glaucoma or advanced disease. Consequently, there is growing interest in developing therapies that can protect RGCs from degeneration, offering additional benefits beyond conventional IOP-lowering treatments (95). One of the most studied neuroprotective agents in glaucoma is brimonidine, an alpha-2 adrenergic agonist traditionally used as an IOP-lowering agent (96). Brimonidine has been shown to have neuroprotective effects in preclinical models, where it promotes the survival of RGCs and reduces axonal damage in the optic nerve (97). Its mechanism of action is believed to involve the upregulation of anti-apoptotic pathways, inhibition of excitotoxicity, and reduction of oxidative stress within the retina (98–100). Early clinical studies in glaucoma patients suggest that brimonidine may help preserve RGCs and slow the progression of the disease, making it a promising candidate for neuroprotection in glaucoma (101). As research in this area continues, identifying additional neuroprotective targets and optimizing drug delivery to the retina will be crucial for translating these promising agents into effective clinical therapies. The ultimate goal of these advancements is to provide more effective, convenient, and long-lasting treatments that can improve patient outcomes and quality of life.
6 Nanotechnology
Nanotechnology is rapidly emerging as a transformative field in ophthalmology, offering innovative solutions for drug delivery, imaging, and tissue engineering (6). Among its most promising applications is drug delivery. Traditional methods often face challenges such as poor bioavailability, rapid degradation, and limited penetration into ocular tissues (102). Nanoparticles can overcome these issues by providing controlled and sustained release of therapeutic agents, improving drug stability, and enhancing tissue penetration (103). For example, cyclodextrin-based nanoparticles have been developed to deliver dexamethasone for treating DME. These nanoparticles enhance the solubility and bioavailability of dexamethasone, enabling effective delivery to the retina. Clinical trials have demonstrated that nanoparticle-based dexamethasone formulations significantly reduce macular thickness and improve visual acuity in patients with DME (104–106), presenting a potential alternative to traditional steroid injections. In glaucoma, nanoparticles are being developed to enhance drug delivery for reducing IOP. For example, liposomal nanoparticles carrying latanoprost have been studied for their ability to provide sustained drug release, reducing administration frequency and improving patient compliance (107). Early clinical trials suggest these nanoparticles effectively lower IOP, potentially offering an improvement over traditional eye drops (108). Nanotechnology also holds promise for non-invasive treatments in ophthalmology. Topical nanoparticle formulations are being investigated for conditions such as cataracts and dry eye syndrome (109). For cataract treatment, polymeric nanoparticles carrying urea have shown potential in dissolving cataractous lens opacities (110). Although still in early research stages, this approach could offer a non-surgical alternative to cataract removal. For dry eye syndrome, liposomal nanoparticles have been developed to deliver artificial tears and anti-inflammatory agents, enhancing the retention time of therapeutic agents on the ocular surface and providing longer-lasting relief from dry eye symptoms (111). A comprehensive list of clinical studies evaluating different nano-formulated drugs in ophthalmology is provided in Table 5.
In addition to nano-medicines undergoing clinical evaluation, several nanoformulations for ocular therapies have been developed and commercialized. Drug-free nanoemulsions, such as Restasis®—the first nanoemulsion-based product containing cyclosporin A for chronic dry eye treatment—have been approved (112). Durezol®, another nanoemulsion containing difluprednate, is approved for eye inflammation treatment. Additionally, Visudyne®, a liposomal formulation of verteporfin marketed by Novartis Pharma AG, received FDA approval in 2000 for intravenous treatment of choroidal neovascularization associated with conditions like AMD, pathological myopia, and ocular histoplasmosis syndrome (113, 114). Macugen®, a PEGylated anti-VEGF aptamer, was approved by the FDA in 2004 for treating wet AMD via intravitreal injection (115). As of 2024, SYSTANE®, a propylene glycol-based nanoemulsion for dry eye treatment, has completed Phase IV clinical trials and is commercially available (116, 117). The increasing number of nano-based ocular therapies in clinical trials and on the market highlights the promise of nanotechnology in ophthalmology. However, further research is needed to optimize nanostructure delivery to the eye, address safety and biocompatibility concerns, and understand the long-term effects of nanoparticle accumulation in ocular tissues (118, 119). Nanotechnology’s potential to enhance drug delivery offers pharmacoeconomic promise by improving bioavailability and reducing treatment frequency, though specific cost data is absent from current studies. Initial development costs may be high, but sustained-release nanoparticles could lower long-term expenses by minimizing drug waste and injections, as seen in trials for DME (104–106). Nano-based systems may be cost-effective for chronic conditions, yet manufacturing scalability and safety concerns require further economic evaluation (6, 120). More studies are needed to validate these benefits. Additionally, challenges related to the manufacturing and scalability of nanoparticle-based therapies need to be addressed for widespread clinical adoption (121).
7 Artificial intelligence
Since its inception in 1956, AI has made significant strides in medical science, optimizing efficiency and driving technological innovations. AI’s transformative impact extends across various medical specialties, including ophthalmology, where it has facilitated improvements in patient care through enhanced data analysis for diagnosis and disease stratification, improved imaging and visualization, automated genetic analysis, and aid in surgical procedures (Figure 4 and Table 6) (122, 123). One of the most impactful applications of AI in ophthalmology is the screening and diagnosis of diabetic retinopathy, a leading cause of vision loss worldwide. The use of convolutional neural networks (CNNs) has been pivotal in this regard. Initial studies in 2016 demonstrated that CNNs could accurately detect diabetic retinopathy, achieving high areas under the curve (AUC) values of 0.980 and 0.991 (124, 125). Subsequent research, utilizing deep learning systems, further validated these findings in larger datasets, achieving an AUC of 0.93 with 90.5% sensitivity and 91.6% specificity for referable diabetic retinopathy, and an AUC of 0.958 with 100% sensitivity and 91.1% specificity for vision-threatening diabetic retinopathy (126). Today, AI algorithms can analyze retinal images to detect early signs of diabetic retinopathy, such as microaneurysms, hemorrhages, and exudates, with accuracy comparable to that of expert ophthalmologists. For instance, the EyeArt v2.1 system detects referable diabetic retinopathy with 95.7% accuracy (127). Beyond standard fundus photography, AI applications have extended to OCT and ultra-widefield imaging, which enhance the detection of diabetic-related peripheral diseases and macular edema. For example, a CNN model developed on OCT images detected macular edema with a cross-validation Dice coefficient of 0.911 (128), while a CNN developed on ultra-widefield images identified proliferative diabetic retinopathy with 94.7% sensitivity and 97.2% specificity (129).
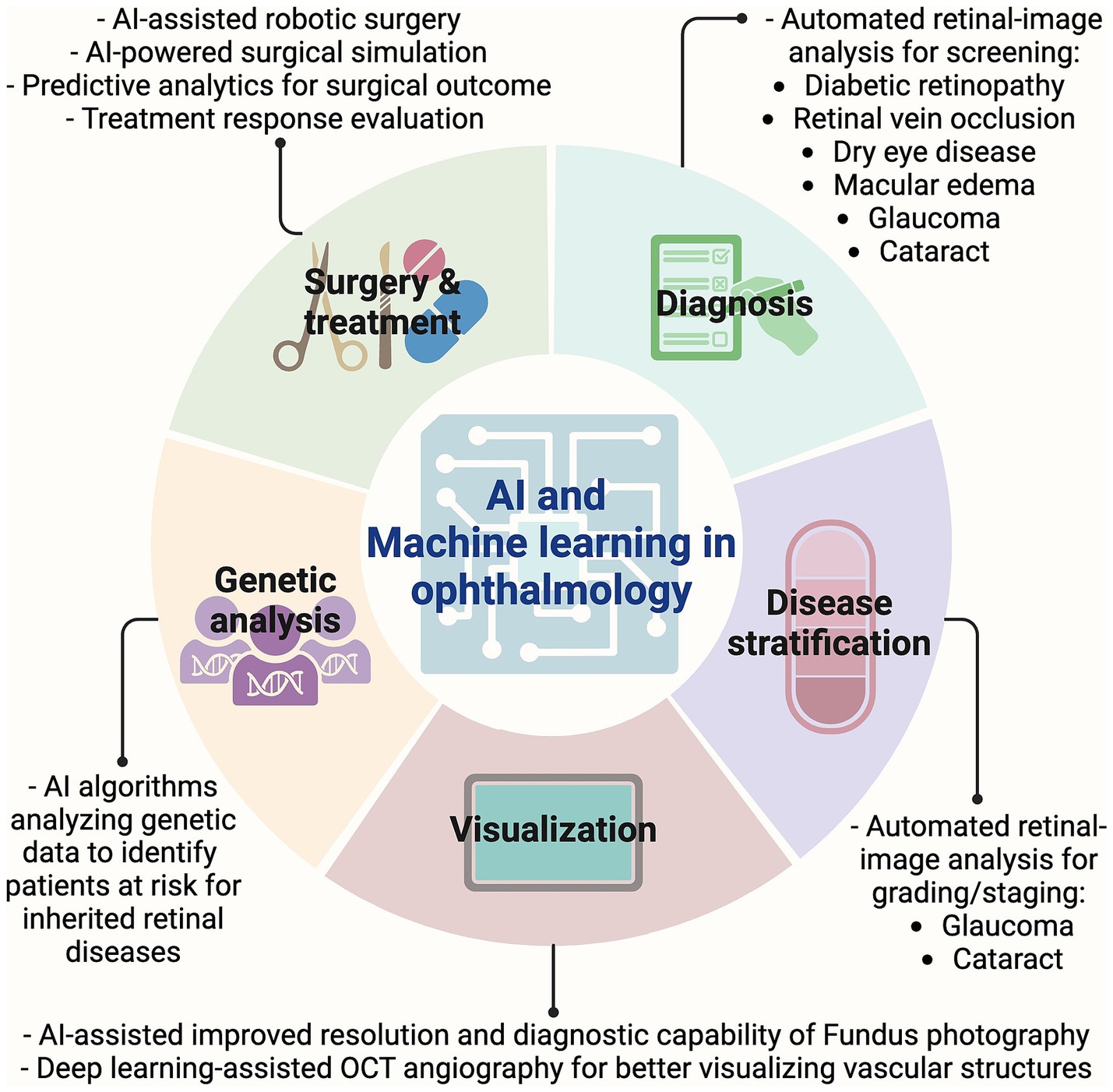
Figure 4. AI and machine learning in ophthalmology. Graphic showing timeline of key imaging advancements made in the field of ophthalmology. AI, Artificial intelligence; OCT, Optical coherence tomography.
AMD treatment also benefits from AI’s ability to analyze large datasets of OCT and retinal photographs. A deep learning algorithm developed from a database of over 130,000 images from 4,613 patients achieved 92% accuracy in detecting moderate to advanced AMD (130). An independent study demonstrated that combining deep learning modalities, such as fundus photographs, OCT, and OCT angiography scans, could increase detection accuracy for AMD to 96% (131). AI also plays a role in quantifying AMD features such as intraretinal fluid and subretinal hyperreflective material, with recent advancements allowing for precise monitoring of treatment responses (132, 133). AI’s potential extends beyond diagnosing specific conditions to encompass broader retinal health assessments. An OCT-based deep learning system achieved a 99.21% AUC in identifying various retinal diseases and demonstrated proficiency in detecting conditions such as neovascular AMD and macular edema, showing comparable performance to that of retinal specialists (134). Glaucoma, the second leading cause of irreversible blindness, also benefits from AI innovations. Machine learning models can analyze optic nerve images and visual field tests to detect glaucoma at an early stage, when treatment is most effective. These models can also predict disease progression and identify patients at high risk of rapid vision loss, allowing for more personalized treatment strategies (135). For instance, deep learning systems have accurately differentiated glaucomatous damage from healthy eyes, with AUCs of 0.944 and 0.940 (136). These findings have been replicated through independent studies worldwide (137–139). Additionally, a deep learning-based CNN system trained on OCT images has efficiently differentiated progressing from non-progressing glaucoma (140). Notably, deep learning networks have been developed to predict the development of glaucomatous visual field changes up to 5 years into the future with high accuracy (141).
AI is also aiding in cataract detection, grading, and surgical assistance. The DeepLensNet, a deep-learning model designed to detect and quantify cataracts from slit-lamp and retroillumination images, has been introduced. This model accurately grades nuclear sclerosis, cortical lens opacity, and posterior subcapsular cataracts, showcasing its potential for automating cataract evaluation and improving global accessibility (142). Additionally, ResNet50 and XGBoost classifiers screen for visually significant cataracts from fundus images, achieving AUCs of 0.916–0.965. This approach streamlines the screening process using a single imaging modality, simplifying cataract detection and allowing integration with existing AI systems for posterior-segment diseases (143). Furthermore, an AI-driven platform has been developed to enhance phacoemulsification cataract surgery, providing real-time guidance to surgeons and achieving high accuracy in phase recognition and pupil segmentation. Surgeons have found this system useful, suggesting that AI could play a transformative role in both cataract screening and surgery (144). Meanwhile, AI also has made a significant breakthrough in areas of refractive surgery, cornea, and optic nerve diseases.
Despite the tremendous potential of AI in ophthalmology, several challenges must be addressed. Ensuring the accuracy and reliability of AI algorithms requires large, diverse datasets and rigorous validation (122, 145). Additionally, integrating AI into clinical practice requires careful consideration of ethical and legal issues, including patient privacy and data security. The reliance on vast amounts of patient data to train AI models underscores the critical need for robust data privacy measures, informed consent processes, and cybersecurity protocols to protect sensitive information. In this context, a smartphone-based offline AI system with high sensitivity for detecting diabetic retinopathy has been developed (146). The risk of algorithmic bias is also a pressing concern, as models trained on non-representative datasets may inadvertently perpetuate healthcare disparities, making it essential to develop transparent and explainable AI systems that can be scrutinized for fairness and accuracy (147, 148). Accountability in AI-driven clinical decision-making further complicates the landscape, as the diffusion of responsibility among developers, healthcare providers, and institutions calls for clearly defined ethical guidelines and regulatory frameworks to delineate liability in cases of diagnostic or treatment errors (149). Societally, while AI holds the promise of extending high-quality ophthalmic care, particularly in underserved regions, its integration also necessitates a transformation in workforce dynamics, with healthcare professionals requiring specialized training to effectively interpret and manage AI outputs (122, 150). Cost-effectiveness analysis of integration of AI into clinical practice is also necessary, though has not fully explored. By automating screening and reducing specialist reliance, AI tools like IDx-DR could lower costs, particularly in underserved areas, while early intervention may prevent expensive vision loss outcomes (151). AI-driven screening is also cost-effective, with savings from reduced referrals, though initial development costs are significant (152, 153). Ongoing research should further delineate these economic advantages to support broader implementation. Finally, the evolving regulatory environment, marked by efforts from bodies such as the FDA and the European Commission, is pivotal in setting rigorous safety and efficacy standards for AI applications in healthcare, thus ensuring that innovation is balanced with patient protection and ethical responsibility.
8 Teleophthalmology
Teleophthalmology, the application of telemedicine in ophthalmic care, has experienced rapid growth in recent years, particularly during the COVID-19 pandemic. This approach utilizes digital technology to provide remote eye care services, expanding access to diagnosis, monitoring, and treatment for patients who may not have easy access to specialized care (154). One of the major breakthroughs has been the use of teleophthalmology for screening retinal diseases. Diabetic retinopathy screening has been one of the most successful implementations of telemedicine, especially in rural and underserved communities where access to ophthalmologists is limited. Teleophthalmology programs, such as those developed in India, have established efficient workflows for capturing retinal images using portable devices and transferring them for remote diagnosis by specialists. This model has been shown to reduce unnecessary referrals and facilitate timely treatment, which is crucial for preventing vision loss (155). Similarly, tele-glaucoma monitoring has made significant strides with devices like the iCare HOME tonometer. Studies have demonstrated that this device, combined with AI algorithms, facilitates continuous monitoring of IOP and optic nerve health, allowing for better management of glaucoma by providing timely data to guide treatment decisions (156). Smart contact lenses with embedded sensors, anticipated in 2024, will enable continuous monitoring of IOP and facilitate timely interventions for glaucoma patients (157). A breakthrough phase 3 randomized controlled trial, involving 105 children aged 4–7 years with amblyopia, has evaluated dichoptic digital therapeutic against full-time glasses wear alone. Participants in the treatment group used the therapeutic at home for 1 h per day, 6 days a week, while the control group continued with glasses only. At 12 weeks, the amblyopic eye visual acuity improved by 1.8 lines in the treatment group compared to 0.8 lines in the control group, a difference that reached statistical significance and led to early study termination for success. Importantly, no serious adverse events were reported, supporting the therapeutic’s safety and clinical efficacy as an alternative treatment for amblyopia (158). However, a recent systematic review evaluating home-based screening tools for amblyopia in children, analyzed data from 28 studies involving various platforms such as smartphone/tablet applications, internet-based tools, digital cameras, and visual acuity charts. The review found significant variability in diagnostic accuracy, and noted methodological limitations, including selection biases and inconsistent recruitment methods. These issues highlight the need for standardized protocols and higher-quality studies to validate the efficacy of these home-based screening tools for amblyopia (159). Remote postoperative care is another important application of teleophthalmology, allowing patients recovering from eye surgeries to receive follow-up care through telemedicine platforms (160). This approach improves patient outcomes and convenience by reducing the need for frequent in-person visits and enabling closer monitoring of recovery progress.
The role of AI in teleophthalmology represents another key advancement, particularly in the development of deep learning systems for automated diagnosis (161). A notable development is the use of AI-powered platforms for diabetic retinopathy screening. The National Health Service in the UK has implemented a widely recognized AI integration program for diabetic retinopathy screening. The program uses algorithms to analyze retinal images and has demonstrated efficacy in improving early detection and management of diabetic retinopathy, which is crucial for reducing vision loss (162). AI models trained on retinal images can detect conditions like diabetic retinopathy, glaucoma, and AMD with high accuracy. For instance, the Singapore-based deep learning algorithm SELENA+ has been validated for its ability to detect multiple pathologies, achieving high sensitivity and specificity across several conditions (126, 163). Additionally, home-based monitoring technologies, such as smartphone apps like Alleye (164) and Home Vision Monitor (165), and portable OCT devices like Vision Home OCT (166, 167) have emerged, allowing patients with conditions like AMD to self-monitor their vision and transmit data to their healthcare providers. These innovations enhance both the quality and accessibility of eye care. Key teleophthalmology advancements are chronologically listed in Table 7.
Despite these advances, teleophthalmology also brings forth multifaceted ethical, societal, and regulatory challenges that demand careful integration alongside its promising technological innovations. Ethically, ensuring robust informed consent and data security in remote environments is critical, particularly given the integration of AI algorithms that may inadvertently perpetuate biases and undermine the patient–provider relationship (168). One primary barrier is the digital divide, which can limit access to telehealth services, particularly among older adults, those with lower digital literacy, and economically disadvantaged groups (169). The COVID-19 pandemic highlighted these inequalities, as many patients struggled with the transition to remote consultations. There are also concerns regarding the depersonalization of the patient–doctor relationship, with some patients and providers reporting difficulties in building rapport through virtual platforms (170). Furthermore, technological issues, such as poor image quality due to media opacities or inadequate infrastructure, can affect the accuracy and reliability of remote diagnoses (171). Concurrently, the regulatory landscape has struggled to keep pace with these innovations, necessitating the development of updated guidelines for cross-border data transfers, licensure, cybersecurity, and reimbursement (172, 173). Addressing these interconnected issues is vital to fostering trust, ensuring equitable access, and maintaining high clinical standards, ultimately enabling teleophthalmology to fulfill its potential as an effective and ethical extension of traditional ophthalmic services. Looking to the future, several developments hold promise for overcoming these limitations. The continued refinement of AI and deep learning algorithms could automate more aspects of eye care, reducing the need for specialist input in initial screenings, which would be particularly beneficial in regions with a shortage of ophthalmologists (174). The deployment of 5G networks and advancements in data transfer technologies will also improve the speed and efficiency of teleophthalmology services, enabling real-time collaboration between healthcare providers across vast distances (175). However, the successful integration of teleophthalmology into mainstream healthcare will require robust infrastructure, significant investment in digital literacy programs, and ongoing efforts to ensure patient privacy and data security (176). In this context, virtual reality is being used to train healthcare providers in teleophthalmology techniques and patient management, enhancing training outcomes and ensuring providers are well-prepared to deliver high-quality care in a telemedicine setting (177). Meanwhile, blockchain technology is being explored for securing patient data in teleophthalmology to enhance patient trust in remote eye care services (178). Finally, economic analyses are needed to validate the cost-effectiveness of these technologies and ensure their widespread adoption (179), though initial equipment and training costs are notable barriers, but long-term savings from early detection and reduced blindness-related expenditures highlight its cost-effectiveness, particularly in remote regions (180).
9 Optogenetics
Optogenetics is a technique that uses light-sensitive proteins, known as opsins, to control the activity of specific cells in the retina. By introducing these opsins into retinal cells via viral vectors, researchers can make the cells responsive to light, effectively creating new photoreceptor functions in the diseased retina (181, 182). This approach is particularly promising for patients with retinitis pigmentosa, where photoreceptors are lost but inner retinal cells remain intact (Table 8) (183). Clinical trials of optogenetic therapies are currently underway, with some showing early signs of success. For instance, a Phase I/IIa trial evaluating GS030 for advanced non-syndromic retinitis pigmentosa combines gene therapy with a visual stimulation device. GS030-DP uses an AAV2.7 m8 capsid to deliver the ChrimsonR-tdTomato opsin to RGCs. ChrimsonR is notable for its red-shifted peak wavelength of 590 nm, providing enhanced safety and reduced phototoxicity compared to traditional blue light-based opsins (184, 185). The companion device, GS030-MD, emits 595 nm light pulses to activate ChrimsonR-expressing RGCs. Preliminary results suggest promising safety and partial vision recovery (186). Notably, in 2017, the FDA granted Orphan Drug Designation to GS030-MD for treating retinitis pigmentosa. In contrast, RST-001, developed by Allergan, utilizes an AAV-2 vector to deliver ChR2 to RGCs. ChR2, a Type 1 opsin, requires high-intensity blue light, which can be phototoxic (187). Despite no serious adverse events reported in a Phase I sequential dose-escalating study, the therapy’s effectiveness in improving visual function remains unproven. Additionally, it is being evaluated for dry AMD, with a Phase II study currently ongoing (182). Bionic Sight’s BS01 integrates ChronosFP gene therapy with a neuroprosthetic system. This system uses an encoder to translate visual input into brain-readable signals and a transducer to project these signals onto the retina (188). The approach aims to enhance the interaction between gene therapy and visual prosthetics, with early results indicating initial success in light and motion detection. Nanoscope Therapeutics’ vMCO-010 is an ambient light-sensitive opsin delivered via an AAV2 vector to bipolar cells. This therapy is notable for its broad activation spectrum, spanning from blue to red wavelengths, and its potential to restore vision without requiring artificial light (189, 190). Preliminary Phase I/IIa results showed significant improvements in visual acuity, and the Phase IIb trial is ongoing to further assess efficacy and safety. RST-001, GS030-MD, and vMCO-010 have all been granted Orphan Drug Designation by the FDA for the treatment of retinitis pigmentosa in 2014, 2017, and 2020, respectively (182).
Given its one-time treatment potential, preliminary data suggest potential efficacy of optogenetics, but no specific cost-effectiveness studies are available, posing a critical challenge in clinical translation of this technique (191). In the future, optimizing gene delivery methods to avoid inflammation and retinal detachment must be a key focus in optogenetics. In this context, subretinal delivery of transgenes using an AAV2 vector has proven safe and effective in treating LCA, offering a favorable biodistribution profile compared to intravitreal injections, which, while less technically challenging, may require higher viral doses and increase inflammation risks (192, 193). New delivery methods, such as suprachoroidal and sub-internal limiting membrane injections, are being investigated to potentially reduce complications and improve treatment outcomes (194).
10 Bionics
Bionic eyes, also known as retinal prostheses, represent an innovative approach to restoring vision in patients with severe retinal degeneration. These devices convert visual information into electrical signals that can be transmitted to the brain via surviving retinal cells or the optic nerve (195, 196). The Argus II retinal prosthesis, developed by Second Sight, was approved for use in patients with retinitis pigmentosa. The device featured a small camera mounted on glasses, which captures visual information and transmits it to an array of electrodes implanted on the retina. These electrodes stimulate the remaining retinal cells, allowing the patient to perceive light and shapes (197). However, despite its transformative potential, the Argus II was eventually discontinued. This decision was driven by several factors, including the device’s limited market size, significant financial challenges faced by Second Sight, and concerns over long-term reliability and safety. Consequently, the cessation of active technical support has raised concerns about device maintenance and the potential for adverse events in existing patients. While bionics offer limited visual resolution, they have been life-changing for some patients, enabling them to navigate their environment more independently. However, the visual experience provided by bionic eyes is still far from normal vision, and ongoing research aims to improve the resolution and functionality of these devices. For instance, the PRIMA bionic eye, developed by Pixium Vision, uses a subretinal implant with a higher density of electrodes, potentially offering improved visual resolution. Early clinical trials have shown that the PRIMA device can restore some degree of central vision in patients with geographic atrophy, a late-stage form of AMD (198). A list of bionics currently being clinically tested for various ocular conditions is provided in Table 9.
Key goals for advancing bionic eyes include improving resolution, color contrast, and expanding the field of view (199, 200). Additionally, integrating visual information with the brain’s processing mechanisms presents a significant challenge, as the brain must adapt to decode the artificial signals generated by these devices. Therefore, a deeper understanding of retina-to-brain signal processing is essential for developing more effective bionic eyes (201). Despite these challenges, bionic eyes represent an exciting frontier in the quest to restore vision. As these technologies continue to evolve, they hold the potential to significantly improve the quality of life for patients with severe retinal degenerative diseases, offering new hope for those who have lost their vision.
11 Neuro-ophthalmology
Neuro-ophthalmology, a subspecialty that bridges neurology and ophthalmology, has seen significant advancements in recent years, particularly in developing innovative treatments for optic nerve and visual pathway disorders. These innovations aim to restore vision by bypassing damaged ocular structures and directly stimulating the visual pathways (Table 10) (202). One of the most promising developments is the Intracortical Visual Prosthesis (ICVP), which aims to restore vision by interfacing directly with the visual cortex. This technology involves implanting intracortical electrode arrays that stimulate the visual cortex in response to external visual inputs (203). Early experimental studies have focused on the safety and biocompatibility of these implants, with some showing potential for restoring basic visual functions such as light perception and shape recognition in patients with severe visual impairments (204, 205). Another innovative project in neuro-ophthalmology is Cortivis, which seeks to develop a cortical visual prosthesis similar to the ICVP. Cortivis involves implanting electrodes in the visual cortex to bypass damaged retinal or optic nerve pathways (206). It utilizes the Utah Electrode Array, which consists of 100 electrodes with lengths ranging from 1.0 to 1.5 mm (207). Currently in the preclinical stage, Cortivis plans to initiate human trials soon. If successful, it could provide a new treatment option for patients with conditions like retinitis pigmentosa or optic nerve atrophy, where traditional treatments are ineffective (208). The Orion project, developed by Second Sight, is one of the most advanced cortical visual prostheses. Orion bypasses the eye and optic nerve entirely, directly stimulating the visual cortex to restore some degree of vision in blind individuals (209, 210). Clinical trials have demonstrated that Orion can provide basic visual cues, such as the perception of light and simple shapes, in patients who have lost their vision due to retinal or optic nerve damage (211). In a study involving 5 blind and 15 sighted patients, researchers tested Orion electrodes implanted in the visual cortex to stimulate vision. They compared two fixation methods: unimanual fixation (using one finger) and bimanual fixation (overlaying one finger on the other). The study found that bimanual fixation combined with relative mapping provided the most accurate estimates of phosphene organization (212). These promising results have led to FDA-approved clinical trials in the United States, marking a significant milestone in the development of cortical visual prostheses (211). The Artificial Vision by Direct Optic Nerve Electrode (AV-DONE) system is another notable development. It involves an electrode device implanted into the optic disc. During trials, patients underwent electrical stimulation sessions 9 and 23 months after implantation. Over 50% of the tests successfully produced phosphene perceptions, with thresholds identified by the stimulation current. Ophthalmologic exams conducted before implantation and every 6 months during a 25-month follow-up revealed no severe complications. The device was found to reduce surgical time, minimize damage to optic nerve fibers, and allow for more electrodes to be fixed compared to earlier devices, indicating its safety and potential benefits for future patients (213).
Despite these advancements, several challenges remain. The complexity of visual pathways and the brain’s processing of visual information pose significant hurdles to developing effective cortical prostheses (196, 214). The Genneris project addresses this by integrating brain-computer interface (BCI) technology with personalized visual prosthetics (215). The Genneris system includes a headband with a camera, a visual processing device with software, a wireless transmitter, and brain tiles measuring 9 mm × 9 mm. The camera captures video, which is processed to extract essential information, and this data is wirelessly transmitted to the implanted brain tiles. The tiles generate electrical pulses that stimulate the brain through a microelectrode array, creating visual patterns with up to 473 light dots (phosphenes). This system provides crucial information about environments and the presence of people and objects. It can transmit data to up to 10 implants, each stimulating 43 areas of the visual cortex (215, 216). By tailoring visual prosthetics to individual needs, Genneris aims to enhance the efficacy of neuroprosthetic devices. While still in development, Genneris has the potential to improve artificial vision quality in patients with severe visual impairments (215). Overall, as these neuro-ophthalmology-based technologies advance, they hold the potential to transform the treatment of optic nerve and visual pathway disorders, offering new hope to patients with previously untreatable conditions.
12 Conclusion
The landscape of ophthalmology is undergoing rapid transformation, driven by cutting-edge technological advancements. Gene and stem cell therapies are leading this revolution, with gene therapy correcting genetic defects and stem cell therapy offering potential for retinal regeneration. These approaches have already demonstrated significant promise, particularly for inherited retinal diseases such as retinitis pigmentosa and LCA. Therapeutic advancements, including anti-VEGF therapies, continue to improve the management of conditions like AMD and DME, with newer treatments providing longer-lasting effects and reducing the treatment burden. Nanotechnology is playing a crucial role in enhancing drug delivery systems, ensuring more targeted and effective treatments for ocular diseases. Advanced imaging techniques, supported by AI, are enhancing early diagnosis, disease monitoring, and personalized treatment planning. AI’s integration into teleophthalmology is expanding access to care, particularly in remote and/or underserved regions, offering new opportunities for timely and efficient eye care. Emerging technologies such as optogenetics and bionic eyes hold exciting potential for restoring vision in patients with severe retinal degeneration, while innovations in neuro-ophthalmology, such as cortical visual prostheses, could restore vision in patients with optic nerve damage. However, significant challenges remain, including issues related to cost, safety, and long-term efficacy. As these technologies mature, their potential to address previously untreatable conditions grows, offering new hope to millions affected by visual impairment. Continued research, collaboration, and refinement of these therapies will be essential in realizing the full potential of these breakthroughs, ultimately bringing us closer to a future where vision loss is no longer inevitable.
Author contributions
FL: Conceptualization, Writing – original draft, Writing – review & editing. YS: Writing – original draft, Writing – review & editing. CZ: Writing – original draft, Writing – review & editing. FA: Visualization, Writing – review & editing. SY: Visualization, Writing – review & editing. SH: Visualization, Writing – review & editing. XS: Conceptualization, Writing – review & editing. YY: Conceptualization, Writing – review & editing.
Funding
The author(s) declare that financial support was received for the research and/or publication of this article. This work was supported by the Guangdong Province Key Technologies R&D Program for “Brain Science and Brain-like Intelligence Research” (2023B0303020002), Guangdong Basic and Applied Basic Research Foundation (2023A1515011644 and 2024A1515011697) and National Natural Science Foundation of China (82302385).
Acknowledgments
Figures were created with BioRender.
Conflict of interest
FL, YS, and CZ were employed by China RongTong Medical Healthcare Group CO. LTD.
The remaining authors declare that the research was conducted in the absence of any commercial or financial relationships that could be construed as a potential conflict of interest.
Generative AI statement
The authors declare that no Gen AI was used in the creation of this manuscript.
Publisher’s note
All claims expressed in this article are solely those of the authors and do not necessarily represent those of their affiliated organizations, or those of the publisher, the editors and the reviewers. Any product that may be evaluated in this article, or claim that may be made by its manufacturer, is not guaranteed or endorsed by the publisher.
References
1. Little, J-A, Congdon, NG, Resnikoff, S, Braithwaite, T, Leasher, J, Naidoo, K, et al. Global estimates on the number of people blind or visually impaired by uncorrected refractive error: a meta-analysis from 2000 to 2020. Eye. (2024) 38:2083–101. doi: 10.1038/s41433-024-03106-0
2. Hastings, MH, Reddy, AB, and Maywood, ES. A clockwork web: circadian timing in brain and periphery, in health and disease. Nat Rev Neurosci. (2003) 4:649–61. doi: 10.1038/nrn1177
3. Burton, MJ, Ramke, J, Marques, AP, Bourne, RRA, Congdon, N, Jones, I, et al. The lancet global health commission on global eye health: vision beyond 2020. Lancet Glob Health. (2021) 9:e489–551. doi: 10.1016/s2214-109x(20)30488-5
4. Redd, TK, and Al-Khaled, T. Technology and innovation in global ophthalmology: the past, the potential, and a path forward. Int Ophthalmol Clin. (2023) 63:25–32. doi: 10.1097/iio.0000000000000450
5. Gandhewar, R. Why is ophthalmology so brilliant? Eye. (2023) 37:3528–9. doi: 10.1038/s41433-023-02560-6
6. Afarid, M, Mahmoodi, S, and Baghban, R. Recent achievements in nano-based technologies for ocular disease diagnosis and treatment, review and update. J Nanobiotechnol. (2022) 20:361. doi: 10.1186/s12951-022-01567-7
7. Miotti, G, Parodi, PC, and Zeppieri, M. Stem cell therapy in ocular pathologies in the past 20 years. World J Stem Cells. (2021) 13:366–85. doi: 10.4252/wjsc.v13.i5.366
8. Brar, AS, Parameswarappa, DC, Takkar, B, Narayanan, R, Jalali, S, Mandal, S, et al. Gene therapy for inherited retinal diseases: from laboratory bench to patient bedside and beyond. Ophthalmol Therapy. (2024) 13:21–50. doi: 10.1007/s40123-023-00862-2
9. Kohn, DB, Chen, YY, and Spencer, MJ. Successes and challenges in clinical gene therapy. Gene Ther. (2023) 30:738–46. doi: 10.1038/s41434-023-00390-5
10. Wang, J-H, Gessler, DJ, Zhan, W, Gallagher, TL, and Gao, G. Adeno-associated virus as a delivery vector for gene therapy of human diseases. Sig Transduct Target Ther. (2024) 9:78. doi: 10.1038/s41392-024-01780-w
11. Russell, S, Bennett, J, Wellman, JA, Chung, DC, Yu, ZF, Tillman, A, et al. Efficacy and safety of Voretigene Neparvovec (Aav2-Hrpe65v2) in patients with Rpe65-mediated inherited retinal dystrophy: a randomised, controlled, open-label, phase 3 trial. Lancet. (2017) 390:849–60. doi: 10.1016/S0140-6736(17)31868-8
12. Fda approves hereditary blindness gene therapy. Nat Biotechnol. (2018) 36:6. doi: 10.1038/nbt0118-6a
13. Smalley, E. First Aav gene therapy poised for landmark approval. Nat Biotechnol. (2017) 35:998–9. doi: 10.1038/nbt1117-998
14. Michalakis, S, Gerhardt, M, Rudolph, G, Priglinger, S, and Priglinger, C. Achromatopsia: genetics and gene therapy. Mol Diagn Ther. (2022) 26:51–9. doi: 10.1007/s40291-021-00565-z
15. Blasiak, J, and Pawlowska, E. A new generation of gene therapies as the future of wet Amd treatment. Int J Mol Sci. (2024) 25:2386. doi: 10.3390/ijms25042386
16. Sisk, R. Subretinal delivery of Rgx-314: a gene therapy for neovascular age-related macular degeneration (Namd). Invest Ophthalmol Vis Sci. (2023) 64:5061.
17. Cehajic-Kapetanovic, J, Xue, K, Martinez-Fernandez de la Camara, C, Nanda, A, Davies, A, Wood, LJ, et al. Initial results from a first-in-human gene therapy trial on X-linked retinitis Pigmentosa caused by mutations in Rpgr. Nat Med. (2020) 26:354–9. doi: 10.1038/s41591-020-0763-1
18. Ren, D, Fisson, S, Dalkara, D, and Ail, D. Immune responses to gene editing by viral and non-viral delivery vectors used in retinal gene therapy. Pharmaceutics. (2022) 14:1973. doi: 10.3390/pharmaceutics14091973
19. Hamilton, BA, and Wright, JF. Challenges posed by immune responses to Aav vectors: addressing root causes. Front Immunol. (2021) 12:675897. doi: 10.3389/fimmu.2021.675897
20. Shamshad, A, Kang, C, Jenny, LA, Persad-Paisley, EM, and Tsang, SH. Translatability barriers between preclinical and clinical trials of Aav gene therapy in inherited retinal diseases. Vis Res. (2023) 210:108258. doi: 10.1016/j.visres.2023.108258
21. Johnson, S, Buessing, M, O’Connell, T, Pitluck, S, and Ciulla, TA. Cost-effectiveness of voretigene neparvovec-rzyl vs standard care for Rpe65-mediated inherited retinal disease. JAMA Ophthalmol. (2019) 137:1115–23. doi: 10.1001/jamaophthalmol.2019.2512
22. Precision Medicine Online. Icer finds nanoscope retinal gene therapy cost effective in $67,400 to $101,300 Price range. Available at: https://www.precisionmedicineonline.com/inherited-disease/icer-finds-nanoscope-retinal-gene-therapy-cost-effective-67400-101300-price-range (Accessed March 28, 2025).
23. Ren, W, Duan, S, Dai, C, Xie, C, Jiang, L, and Shi, Y. Nanotechnology lighting the way for gene therapy in ophthalmopathy: from opportunities toward applications. Molecules. (2023) 28:3500. doi: 10.3390/molecules28083500
24. Choi, EH, Suh, S, Sears, AE, Hołubowicz, R, Kedhar, SR, Browne, AW, et al. Genome editing in the treatment of ocular diseases. Exp Mol Med. (2023) 55:1678–90. doi: 10.1038/s12276-023-01057-2
25. Pierce, EA, Aleman, TS, Jayasundera, KT, Ashimatey, BS, and Kim, K. Gene editing for Cep290-associated retinal degeneration. N Engl J Med. (2024) 390:1972–84. doi: 10.1056/NEJMoa2309915
26. Hoang, DM, Pham, PT, Bach, TQ, Ngo, ATL, Nguyen, QT, Phan, TTK, et al. Stem cell-based therapy for human diseases. Signal Transduct Target Ther. (2022) 7:272. doi: 10.1038/s41392-022-01134-4
27. Radu, M, Brănișteanu, DC, Pirvulescu, RA, Dumitrescu, OM, Ionescu, MA, and Zemba, M. Exploring stem-cell-based therapies for retinal regeneration. Life. (2024) 14:668. doi: 10.3390/life14060668
28. Moshirfar, M, Masud, M, and Harvey, DH. The multifold etiologies of limbal stem cell deficiency: a comprehensive review on the etiologies and additional treatment options for limbal stem cell deficiency. J Clin Med. (2023) 12:4418. doi: 10.3390/jcm12134418
29. Kenyon, KR, and Tseng, SC. Limbal autograft transplantation for ocular surface disorders. Ophthalmology. (1989) 96:709–22; discussion 22–3. doi: 10.1016/s0161-6420(89)32833-8
30. Borderie, VM, Ghoubay, D, Georgeon, C, Borderie, M, de Sousa, C, Legendre, A, et al. Long-term results of cultured limbal stem cell versus limbal tissue transplantation in stage III limbal deficiency. Stem Cells Transl Med. (2019) 8:1230–41. doi: 10.1002/sctm.19-0021
31. Sangwan, VS, Basu, S, MacNeil, S, and Balasubramanian, D. Simple limbal epithelial transplantation (Slet): a novel surgical technique for the treatment of unilateral limbal stem cell deficiency. Br J Ophthalmol. (2012) 96:931–4. doi: 10.1136/bjophthalmol-2011-301164
32. Jackson, CJ, Myklebust Ernø, IT, Ringstad, H, Tønseth, KA, Dartt, DA, and Utheim, TP. Simple Limbal epithelial transplantation: current status and future perspectives. Stem Cells Transl Med. (2020) 9:316–27. doi: 10.1002/sctm.19-0203
33. Bobba, S, Chow, S, Watson, S, and Di Girolamo, N. Clinical outcomes of Xeno-free expansion and transplantation of autologous ocular surface epithelial stem cells via contact lens delivery: a prospective case series. Stem Cell Res Ther. (2015) 6:23. doi: 10.1186/s13287-015-0009-1
34. Uyama, H, Mandai, M, and Takahashi, M. Stem-cell-based therapies for retinal degenerative diseases: current challenges in the establishment of new treatment strategies. Develop Growth Differ. (2021) 63:59–71. doi: 10.1111/dgd.12704
35. Yang, S, Zhou, J, and Li, D. Functions and diseases of the retinal pigment epithelium. Front Pharmacol. (2021) 12:727870. doi: 10.3389/fphar.2021.727870
36. Schwartz, SD, Hubschman, JP, Heilwell, G, Franco-Cardenas, V, Pan, CK, Ostrick, RM, et al. Embryonic stem cell trials for macular degeneration: a preliminary report. Lancet (London, England). (2012) 379:713–20. doi: 10.1016/s0140-6736(12)60028-2
37. Kashani, AH, Lebkowski, JS, Hinton, DR, Zhu, D, Faynus, MA, Chen, S, et al. Survival of an Hla-mismatched, bioengineered Rpe implant in dry age-related macular degeneration. Stem Cell Rep. (2022) 17:448–58. doi: 10.1016/j.stemcr.2022.01.001
38. Mehat, MS, Sundaram, V, Ripamonti, C, Robson, AG, Smith, AJ, Borooah, S, et al. Transplantation of human embryonic stem cell-derived retinal pigment epithelial cells in macular degeneration. Ophthalmology. (2018) 125:1765–75. doi: 10.1016/j.ophtha.2018.04.037
39. Oner, A, Gonen, ZB, Sevim, DG, Smim Kahraman, N, and Unlu, M. Suprachoroidal adipose tissue-derived mesenchymal stem cell implantation in patients with dry-type age-related macular degeneration and Stargardt’s macular dystrophy: 6-month follow-up results of a phase 2 study. Cell Reprog. (2018) 20:329–36. doi: 10.1089/cell.2018.0045
40. Liu, Y, Chen, SJ, Li, SY, Qu, LH, Meng, XH, Wang, Y, et al. Long-term safety of human retinal progenitor cell transplantation in retinitis Pigmentosa patients. Stem Cell Res Ther. (2017) 8:209. doi: 10.1186/s13287-017-0661-8
41. Radtke, ND, Aramant, RB, Petry, HM, Green, PT, Pidwell, DJ, and Seiler, MJ. Vision improvement in retinal degeneration patients by implantation of retina together with retinal pigment epithelium. Am J Ophthalmol. (2008) 146:172–182.e1. doi: 10.1016/j.ajo.2008.04.009
42. Ahmed, I, Johnston, RJ Jr, and Singh, MS. Pluripotent stem cell therapy for retinal diseases. Ann Transl Med. (2021) 9:1279. doi: 10.21037/atm-20-4747
43. Sun, H, Zhu, Q, Guo, P, Zhang, Y, Tighe, S, and Zhu, Y. Trabecular meshwork cells are a valuable resource for cellular therapy of glaucoma. J Cell Mol Med. (2019) 23:1678–86. doi: 10.1111/jcmm.14158
44. Zhu, W, Gramlich, OW, Laboissonniere, L, Jain, A, Sheffield, VC, Trimarchi, JM, et al. Transplantation of Ipsc-derived tm cells rescues glaucoma phenotypes in vivo. Proc Natl Acad Sci USA. (2016) 113:E3492–500. doi: 10.1073/pnas.1604153113
45. Zhu, W, Jain, A, Gramlich, OW, Tucker, BA, Sheffield, VC, and Kuehn, MH. Restoration of aqueous humor outflow following transplantation of Ipsc-derived trabecular meshwork cells in a transgenic mouse model of glaucoma. Invest Ophthalmol Vis Sci. (2017) 58:2054–62. doi: 10.1167/iovs.16-20672
46. Coulon, SJ, Schuman, JS, Du, Y, Bahrani Fard, MR, Ethier, CR, and Stamer, WD. A novel glaucoma approach: stem cell regeneration of the trabecular meshwork. Prog Retin Eye Res. (2022) 90:101063. doi: 10.1016/j.preteyeres.2022.101063
47. Musiał-Wysocka, A, Kot, M, and Majka, M. The pros and cons of mesenchymal stem cell-based therapies. Cell Transplant. (2019) 28:801–12. doi: 10.1177/0963689719837897
48. Gu, X, Yu, X, Zhao, C, Duan, P, Zhao, T, Liu, Y, et al. Efficacy and safety of autologous bone marrow mesenchymal stem cell transplantation in patients with diabetic retinopathy. Cell Physiol Biochem. (2018) 49:40–52. doi: 10.1159/000492838
49. Shimazaki, J. Definition and diagnostic criteria of dry eye disease: historical overview and future directions. Invest Ophthalmol Vis Sci. (2018) 59:DES7–DES12. doi: 10.1167/iovs.17-23475
50. Weng, J, He, C, Lai, P, Luo, C, Guo, R, Wu, S, et al. Mesenchymal stromal cells treatment attenuates dry eye in patients with chronic graft-versus-host disease. Mol Ther. (2012) 20:2347–54. doi: 10.1038/mt.2012.208
51. Zhou, T, and He, C. Mir-204-containing exosomes ameliorate Gvhd-associated dry eye disease. Sci Adv. (2022) 8:eabj9617. doi: 10.1126/sciadv.abj9617
52. Fortress, AM, Miyagishima, KJ, Reed, AA, Temple, S, Clegg, DO, Tucker, BA, et al. Stem cell sources and characterization in the development of cell-based products for treating retinal disease: an Nei town hall report. Stem Cell Res Ther. (2023) 14:53. doi: 10.1186/s13287-023-03282-y
53. Reboussin, É, and Buffault, J. Evaluation of neuroprotective and immunomodulatory properties of mesenchymal stem cells in an ex vivo retinal explant model. J Neuroinflammation. (2022) 19:63. doi: 10.1186/s12974-022-02418-w
54. Chua, CYX, Jiang, AY, Eufrásio-da-Silva, T, Dolatshahi-Pirouz, A, Langer, R, Orive, G, et al. Emerging immunomodulatory strategies for cell therapeutics. Trends Biotechnol. (2023) 41:358–73. doi: 10.1016/j.tibtech.2022.11.008
55. Niu, Y, Ji, J, Yao, K, and Fu, Q. Regenerative treatment of ophthalmic diseases with stem cells: principles, progress, and challenges. Adv Ophthalmol Pract Res. (2024) 4:52–64. doi: 10.1016/j.aopr.2024.02.001
56. Li, Y, Xia, X, and Paulus, YM. Advances in retinal optical imaging. Photo-Dermatology. (2018) 5:2. doi: 10.3390/photonics5020009
57. Weiss, SJ, and Papakostas, TD. Fluorescein angiography In: D Albert, J Miller, D Azar, and LH Young, editors. Albert and Jakobiec’s principles and practice of ophthalmology. Cham: Springer International Publishing (2020). 1–24.
58. Baddam, DO, Ragi, SD, Tsang, SH, and Ngo, WK. Ophthalmic fluorescein angiography. Methods Mol Biol (Clifton, NJ). (2023) 2560:153–60. doi: 10.1007/978-1-0716-2651-1_15
59. Panwar, N, Huang, P, Lee, J, Keane, PA, Chuan, TS, Richhariya, A, et al. Fundus photography in the 21st century--a review of recent technological advances and their implications for worldwide healthcare. Telemed J e-health. (2016) 22:198–208. doi: 10.1089/tmj.2015.0068
60. Delgado-Tirado, S, Gonzalez-Buendia, L, and Kim, LA. Indocyanine Green angiography In: D Albert, J Miller, D Azar, and LH Young, editors. Albert and Jakobiec’s principles and practice of ophthalmology. Cham: Springer International Publishing (2020). 1–29.
61. Mohankumar, A, and Gurnani, B. Scanning laser ophthalmoscope In: Statpearls. Treasure Island (FL): StatPearls Publishing (2024)
62. Hao, L, Liu, Y, Liu, X, and Lee, RK. Confocal scanning laser ophthalmoscopy to image retinal ganglion cells in real-time. Methods Mol Biol (Clifton, NJ). (2023) 2708:115–21. doi: 10.1007/978-1-0716-3409-7_12
63. Ishikawa, H, and Schuman, JS. Anterior segment imaging: ultrasound biomicroscopy. Ophthalmol Clin N Am. (2004) 17:7–20. doi: 10.1016/j.ohc.2003.12.001
64. Helms, RW, Minhaz, AT, Wilson, DL, and Örge, FH. Clinical 3d imaging of the anterior segment with ultrasound biomicroscopy. Transl Vis Sci Technol. (2021) 10:11. doi: 10.1167/tvst.10.3.11
65. Zeppieri, M, and Marsili, S. Optical coherence tomography (Oct): a brief look at the uses and technological evolution of ophthalmology. Medicina (Kaunas). (2023) 59:2114. doi: 10.3390/medicina59122114
66. Asanad, S, and Karanjia, R. Multifocal electroretinogram In: Statpearls. Treasure Island (FL): StatPearls Publishing (2024)
67. Akyol, E, and Hagag, AM. Adaptive optics: principles and applications in ophthalmology. Eye (Lond). (2021) 35:244–64. doi: 10.1038/s41433-020-01286-z
68. Godara, P, Dubis, AM, Roorda, A, Duncan, JL, and Carroll, J. Adaptive optics retinal imaging: emerging clinical applications. Optom Vis Sci. (2010) 87:930–41. doi: 10.1097/OPX.0b013e3181ff9a8b
69. Morgan, JIW, Jiang, YY, Vergilio, GK, Serrano, LW, Pearson, DJ, Bennett, J, et al. Short-term assessment of subfoveal injection of adeno-associated virus-mediated Hchm gene augmentation in choroideremia using adaptive optics ophthalmoscopy. JAMA Ophthalmol. (2022) 140:411–20. doi: 10.1001/jamaophthalmol.2022.0158
70. Foulsham, W, Chien, J, Lenis, TL, and Papakostas, TD. Optical coherence tomography angiography: clinical utility and future directions. J Vitreoretin Dis. (2022) 6:229–42. doi: 10.1177/24741264221080376
71. Zheng, F, Deng, X, Zhang, Q, He, J, Ye, P, Liu, S, et al. Advances in swept-source optical coherence tomography and optical coherence tomography angiography. Adv Ophthalmol Pract Res. (2023) 3:67–79. doi: 10.1016/j.aopr.2022.10.005
72. Yanxiu, L, and Yannis, MP. Photoacoustic imaging of the eye In: RG Reda, editor. Photoacoustic imaging. Rijeka: IntechOpen (2019). 6.
73. Liu, W, and Zhang, HF. Photoacoustic imaging of the eye: a mini review. Photo-Dermatology. (2016) 4:112–23. doi: 10.1016/j.pacs.2016.05.001
74. Lemmens, S, Van Eijgen, J, Van Keer, K, Jacob, J, Moylett, S, De Groef, L, et al. Hyperspectral imaging and the retina: worth the wave? Transl Vis Sci Technol. (2020) 9:9. doi: 10.1167/tvst.9.9.9
75. Jamali, A, Seyed-Razavi, Y, Chao, C, Ortiz, G, Kenyon, B, Blanco, T, et al. Intravital multiphoton microscopy of the ocular surface: alterations in conventional dendritic cell morphology and kinetics in dry eye disease. Front Immunol. (2020) 11:742. doi: 10.3389/fimmu.2020.00742
76. Ortiz, G, Chao, C, Jamali, A, Seyed-Razavi, Y, Kenyon, B, Harris, DL, et al. Effect of dry eye disease on the kinetics of lacrimal gland dendritic cells as visualized by Intravital multi-photon microscopy. Front Immunol. (2020) 11:1713. doi: 10.3389/fimmu.2020.01713
77. Hong, M, Chong, SZ, Goh, YY, and Tong, L. Two-photon and multiphoton microscopy in anterior segment diseases of the eye. Int J Mol Sci. (2024) 25:1670. doi: 10.3390/ijms25031670
78. Ou, K, Li, Y, Liu, L, Li, H, Cox, K, Wu, J, et al. Recent developments of neuroprotective agents for degenerative retinal disorders. Neural Regen Res. (2022) 17:1919–28. doi: 10.4103/1673-5374.335140
79. Yanai, R, Okunuki, Y, Park, DH, and Zunaina, E. Editorial: next therapeutic targets in ocular diseases. Front Med. (2022) 9:953377. doi: 10.3389/fmed.2022.953377
80. Tah, V, Orlans, HO, Hyer, J, Casswell, E, Din, N, Sri Shanmuganathan, V, et al. Anti-Vegf therapy and the retina: an update. J Ophthalmol. (2015) 2015:627674. doi: 10.1155/2015/627674
81. Hang, A, Feldman, S, Amin, AP, Ochoa, JAR, and Park, SS. Intravitreal anti-vascular endothelial growth factor therapies for retinal disorders. Pharmaceuticals (Basel, Switzerland). (2023) 16:1140. doi: 10.3390/ph16081140
82. Rosenfeld, PJ, Brown, DM, Heier, JS, Boyer, DS, Kaiser, PK, Chung, CY, et al. Ranibizumab for neovascular age-related macular degeneration. NEJM. (2006) 355:1419–31. doi: 10.1056/NEJMoa054481
83. Lanzetta, P, Korobelnik, J-F, Heier, JS, Leal, S, Holz, FG, Clark, WL, et al. Intravitreal Aflibercept 8 mg in neovascular age-related macular degeneration (Pulsar): 48-week results from a randomised, double-masked, non-inferiority, phase 3 trial. Lancet. (2024) 403:1141–52. doi: 10.1016/S0140-6736(24)00063-1
84. Wykoff, CC, Brown, DM, Reed, K, Berliner, AJ, Gerstenblith, AT, Breazna, A, et al. Effect of high-dose intravitreal Aflibercept, 8 mg, in patients with neovascular age-related macular degeneration: the phase 2 Candela randomized clinical trial. JAMA Ophthalmol. (2023) 141:834–42. doi: 10.1001/jamaophthalmol.2023.2421
85. Mantel, I, Gillies, MC, and Souied, EH. Switching between ranibizumab and aflibercept for the treatment of neovascular age-related macular degeneration. Surv Ophthalmol. (2018) 63:638–45. doi: 10.1016/j.survophthal.2018.02.004
86. Gillies, MC, Hunyor, AP, Arnold, JJ, Guymer, RH, Wolf, S, Ng, P, et al. Effect of ranibizumab and aflibercept on best-corrected visual acuity in treat-and-extend for neovascular age-related macular degeneration: a randomized clinical trial. JAMA Ophthalmol. (2019) 137:372–9. doi: 10.1001/jamaophthalmol.2018.6776
87. Montesel, A, Bucolo, C, Sallo, FB, and Eandi, CM. Short-term efficacy and safety outcomes of brolucizumab in the real-life clinical practice. Front Pharmacol. (2021) 12:720345. doi: 10.3389/fphar.2021.720345
88. Dugel, PU, Koh, A, Ogura, Y, Jaffe, GJ, Schmidt-Erfurth, U, Brown, DM, et al. Hawk and Harrier: phase 3, multicenter, randomized, double-masked trials of brolucizumab for neovascular age-related macular degeneration. Ophthalmology. (2020) 127:72–84. doi: 10.1016/j.ophtha.2019.04.017
89. Dakin, HA, Wordsworth, S, Rogers, CA, Abangma, G, Raftery, J, Harding, SP, et al. Cost-effectiveness of ranibizumab and bevacizumab for age-related macular degeneration: 2-year findings from the Ivan randomised trial. BMJ Open. (2014) 4:e005094. doi: 10.1136/bmjopen-2014-005094
90. Hachana, S, and Larrivée, B. Tgf-Β superfamily signaling in the eye: implications for ocular pathologies. Cells. (2022) 11:2336. doi: 10.3390/cells11152336
91. Pfeiffer, N, Voykov, B, Renieri, G, Bell, K, Richter, P, Weigel, M, et al. First-in-human phase I study of Isth0036, an antisense oligonucleotide selectively targeting transforming growth factor beta 2 (Tgf-Β2), in subjects with open-angle glaucoma undergoing glaucoma filtration surgery. PLoS One. (2017) 12:e0188899. doi: 10.1371/journal.pone.0188899
92. Munk, MR, Gupta, V, Bolz, M, Strauss, R, Wedrich, A, Brar, M, et al. First results of the phase 2 better trial using intravitreal Isth0036, a selective Tgf-Β2 blocking antisense in Amd and Dme. Investig Ophthalmol Vis Sci. (2024) 65:3309.
93. Sena, DF, and Lindsley, K. Neuroprotection for treatment of glaucoma in adults. Cochrane Database Syst Rev. (2017) 1:CD006539. doi: 10.1002/14651858.CD006539.pub4
94. Davis, BM, Crawley, L, Pahlitzsch, M, Javaid, F, and Cordeiro, MF. Glaucoma: the retina and beyond. Acta Neuropathol. (2016) 132:807–26. doi: 10.1007/s00401-016-1609-2
95. Tribble, JR, Hui, F, Quintero, H, El Hajji, S, Bell, K, Di Polo, A, et al. Neuroprotection in glaucoma: mechanisms beyond intraocular pressure lowering. Mol Asp Med. (2023) 92:101193. doi: 10.1016/j.mam.2023.101193
96. Cantor, LB. Brimonidine in the treatment of glaucoma and ocular hypertension. Ther Clin Risk Manag. (2006) 2:337–46. doi: 10.2147/tcrm.2006.2.4.337
97. Fujita, Y, Sato, A, and Yamashita, T. Brimonidine promotes axon growth after optic nerve injury through Erk phosphorylation. Cell Death Dis. (2013) 4:e763. doi: 10.1038/cddis.2013.298
98. Jung, KI, Kim, JH, Han, JS, and Park, CK. Exploring neuroprotective effects of topical brimonidine in experimental diabetic retinopathy. In vivo (Athens, Greece). (2024) 38:1609–20. doi: 10.21873/invivo.13611
99. Lee, KY, Nakayama, M, Aihara, M, Chen, YN, and Araie, M. Brimonidine is neuroprotective against glutamate-induced neurotoxicity, oxidative stress, and hypoxia in purified rat retinal ganglion cells. Mol Vis. (2010) 16:246–51.
100. Cai, J, Li, J, Liu, W, Han, Y, and Wang, H. Alpha2-adrenergic receptors in spiral ganglion neurons may mediate protective effects of brimonidine and yohimbine against glutamate and hydrogen peroxide toxicity. Neuroscience. (2013) 228:23–35. doi: 10.1016/j.neuroscience.2012.10.004
101. Krupin, T, Liebmann, JM, Greenfield, DS, Ritch, R, and Gardiner, S. A randomized trial of brimonidine versus timolol in preserving visual function: results from the low-pressure glaucoma treatment study. Am J Ophthalmol. (2011) 151:671–81. doi: 10.1016/j.ajo.2010.09.026
102. Akhter, MH, and Ahmad, I. Drug delivery challenges and current progress in nanocarrier-based ocular therapeutic system. Gels (Basel, Switzerland). (2022) 8:82. doi: 10.3390/gels8020082
103. Oucif Khaled, MT, Zaater, A, Ben Amor, I, Zeghoud, S, Ben Amor, A, Hemmami, H, et al. Drug delivery methods based on nanotechnology for the treatment of eye diseases. Ann Med Surg. (2023) 85:6029–40. doi: 10.1097/ms9.0000000000001399
104. Tanito, M, Hara, K, Takai, Y, Matsuoka, Y, Nishimura, N, Jansook, P, et al. Topical dexamethasone-cyclodextrin microparticle eye drops for diabetic macular edema. Invest Ophthalmol Vis Sci. (2011) 52:7944–8. doi: 10.1167/iovs.11-8178
105. Ohira, A, Hara, K, Jóhannesson, G, Tanito, M, Ásgrímsdóttir, GM, Lund, SH, et al. Topical dexamethasone Γ-cyclodextrin nanoparticle eye drops increase visual acuity and decrease macular thickness in diabetic macular oedema. Acta Ophthalmol. (2015) 93:610–5. doi: 10.1111/aos.12803
106. Stefansson, E, Loftsson, T, Larsen, M, Papp, A, Kaarniranta, K, Munk, MR, et al. DX‐211 study group topical treatment of diabetic macular edema using dexamethasone ophthalmic suspension: a randomized, double-masked, vehicle-controlled study. Acta Ophthalmol. (2023) 101:22–33. doi: 10.1111/aos.15215
107. Natarajan, JV, Ang, M, Darwitan, A, Chattopadhyay, S, Wong, TT, and Venkatraman, SS. Nanomedicine for glaucoma: liposomes provide sustained release of latanoprost in the eye. Int J Nanomedicine. (2012) 7:123–31. doi: 10.2147/ijn.s25468
108. Goldberg, DF, and Williams, R. A phase 2 study evaluating safety and efficacy of the latanoprost punctal plug delivery system (L-Ppds) in subjects with ocular hypertension (oh) or open-angle glaucoma (Oag). Invest Ophthalmol Vis Sci. (2012) 53:5095.
109. Vaneev, A, and Tikhomirova, V. Nanotechnology for topical drug delivery to the anterior segment of the eye. Int J Mol Sci. (2021) 22:12368. doi: 10.3390/ijms222212368
110. Eldien, H. A randomized controlled trial comparing urea loaded nanoparticles to placebo: a new concept for cataract management. ClinicalTrial gov. (2016).
111. Coco, G, Buffon, G, Taloni, A, and Giannaccare, G. Recent advances in nanotechnology for the treatment of dry eye disease. Nanomaterials (Basel, Switzerland). (2024) 14:669. doi: 10.3390/nano14080669
112. Ames, P, and Galor, A. Cyclosporine ophthalmic emulsions for the treatment of dry eye: a review of the clinical evidence. Clin Invest. (2015) 5:267–85. doi: 10.4155/cli.14.135
113. Verteporfin In Photodynamic Therapy Study Group. Verteporfin therapy of subfoveal choroidal neovascularization in age-related macular degeneration: two-year results of a randomized clinical trial including lesions with occult with no classic choroidal neovascularization--verteporfin in photodynamic therapy report 2. Am J Ophthalmol. (2001) 131:541–60. doi: 10.1016/s0002-9394(01)00967-9
114. Keam, SJ, Scott, LJ, and Curran, MP. Verteporfin: a review of its use in the management of subfoveal choroidal neovascularisation. Drugs. (2003) 63:2521–54. doi: 10.2165/00003495-200363220-00016
115. Tobin, KA. Macugen treatment for wet age-related macular degeneration. Insight (American Society of Ophthalmic Registered Nurses). (2006) 31:11–4.
116. Yeu, E, Silverstein, S, Guillon, M, Schulze, M-M, Galarreta, D, Srinivasan, S, et al. Efficacy and safety of phospholipid nanoemulsion-based ocular lubricant for the management of various subtypes of dry eye disease: a phase IV, multicenter trial. Clin Ophthalmol (Auckland, NZ). (2020) 14:2561–70. doi: 10.2147/OPTH.S261318
117. Bickle, K, Miller, JR, Tauber, J, and Awisi-Gyau, D. Multi-symptom relief with propylene glycol-hydroxypropyl-guar nanoemulsion lubricant eye drops in subjects with dry eye disease: a post-marketing prospective study. Ophthalmol Therapy. (2024) 13:481–94. doi: 10.1007/s40123-023-00853-3
118. Liu, LC, Chen, YH, and Lu, DW. Overview of recent advances in nano-based ocular drug delivery. Int J Mol Sci. (2023) 24:15352. doi: 10.3390/ijms242015352
119. Zhu, S, Gong, L, Li, Y, Xu, H, and Gu, Z. Safety assessment of nanomaterials to eyes: an important but neglected issue. Adv Sci (Weinheim, Baden-Wurttemberg, Germany). (2019) 6:1802289. doi: 10.1002/advs.201802289
120. Li, S, Chen, L, and Fu, Y. Nanotechnology-based ocular drug delivery systems: recent advances and future prospects. J Nanobiotechnol. (2023) 21:232. doi: 10.1186/s12951-023-01992-2
121. Đorđević, S, Gonzalez, MM, Conejos-Sánchez, I, Carreira, B, Pozzi, S, Acúrcio, RC, et al. Current hurdles to the translation of nanomedicines from bench to the clinic. Drug Deliv Transl Res. (2022) 12:500–25. doi: 10.1007/s13346-021-01024-2
122. Li, Z, Wang, L, Wu, X, Jiang, J, Qiang, W, Xie, H, et al. Artificial intelligence in ophthalmology: the path to the real-world clinic. Cell Rep Med. (2023) 4:101095. doi: 10.1016/j.xcrm.2023.101095
123. Srivastava, O, Tennant, M, Grewal, P, Rubin, U, and Seamone, M. Artificial intelligence and machine learning in ophthalmology: a review. Indian J Ophthalmol. (2023) 71:11–7. doi: 10.4103/ijo.IJO_1569_22
124. Abràmoff, MD, Lou, Y, Erginay, A, Clarida, W, Amelon, R, Folk, JC, et al. Improved automated detection of diabetic retinopathy on a publicly available dataset through integration of deep learning. Invest Ophthalmol Vis Sci. (2016) 57:5200–6. doi: 10.1167/iovs.16-19964
125. Gulshan, V, Peng, L, Coram, M, Stumpe, MC, Wu, D, Narayanaswamy, A, et al. Development and validation of a deep learning algorithm for detection of diabetic retinopathy in retinal fundus photographs. JAMA. (2016) 316:2402–10. doi: 10.1001/jama.2016.17216
126. Ting, DSW, Cheung, CY, Lim, G, Tan, GSW, Quang, ND, Gan, A, et al. Development and validation of a deep learning system for diabetic retinopathy and related eye diseases using retinal images from multiethnic populations with diabetes. JAMA. (2017) 318:2211–23. doi: 10.1001/jama.2017.18152
127. Heydon, P, Egan, C, Bolter, L, Chambers, R, Anderson, J, Aldington, S, et al. Prospective evaluation of an artificial intelligence-enabled algorithm for automated diabetic retinopathy screening of 30 000 patients. Br J Ophthalmol. (2021) 105:723–8. doi: 10.1136/bjophthalmol-2020-316594
128. Lee, CS, Baughman, DM, and Lee, AY. Deep learning is effective for the classification of Oct images of normal versus age-related macular degeneration. Ophthalmol Retina. (2017) 1:322–7. doi: 10.1016/j.oret.2016.12.009
129. Nagasawa, T, Tabuchi, H, Masumoto, H, Enno, H, Niki, M, Ohara, Z, et al. Accuracy of ultrawide-Field fundus ophthalmoscopy-assisted deep learning for detecting treatment-Naïve proliferative diabetic retinopathy. Int Ophthalmol. (2019) 39:2153–9. doi: 10.1007/s10792-019-01074-z
130. Burlina, PM, Joshi, N, Pekala, M, Pacheco, KD, Freund, DE, and Bressler, NM. Automated grading of age-related macular degeneration from color fundus images using deep convolutional neural networks. JAMA Ophthalmol. (2017) 135:1170–6. doi: 10.1001/jamaophthalmol.2017.3782
131. Vaghefi, E, Hill, S, Kersten, HM, and Squirrell, D. Multimodal retinal image analysis via deep learning for the diagnosis of intermediate dry age-related macular degeneration: a feasibility study. J Ophthalmol. (2020) 2020:7493419. doi: 10.1155/2020/7493419
132. Liefers, B, Colijn, JM, González-Gonzalo, C, Verzijden, T, Wang, JJ, Joachim, N, et al. A deep learning model for segmentation of geographic atrophy to study its long-term natural history. Ophthalmology. (2020) 127:1086–96. doi: 10.1016/j.ophtha.2020.02.009
133. Fu, DJ, Faes, L, Wagner, SK, Moraes, G, Chopra, R, Patel, PJ, et al. Predicting incremental and future visual change in neovascular age-related macular degeneration using deep learning. Ophthalmol Retina. (2021) 5:1074–84. doi: 10.1016/j.oret.2021.01.009
134. De Fauw, J, Ledsam, JR, Romera-Paredes, B, Nikolov, S, Tomasev, N, Blackwell, S, et al. Clinically applicable deep learning for diagnosis and referral in retinal disease. Nat Med. (2018) 24:1342–50. doi: 10.1038/s41591-018-0107-6
135. Zhu, Y, Salowe, R, Chow, C, Li, S, Bastani, O, and O’Brien, JM. Advancing glaucoma care: integrating artificial intelligence in diagnosis, management, and progression detection. Bioengineering (Basel, Switzerland). (2024) 11:122. doi: 10.3390/bioengineering11020122
136. Medeiros, FA, Jammal, AA, and Thompson, AC. From machine to machine: an Oct-trained deep learning algorithm for objective quantification of glaucomatous damage in fundus photographs. Ophthalmology. (2019) 126:513–21. doi: 10.1016/j.ophtha.2018.12.033
137. Yang, HK, Kim, YJ, Sung, JY, Kim, DH, Kim, KG, and Hwang, JM. Efficacy for differentiating nonglaucomatous versus glaucomatous optic neuropathy using deep learning systems. Am J Ophthalmol. (2020) 216:140–6. doi: 10.1016/j.ajo.2020.03.035
138. Orlando, JI, Fu, H, Barbosa Breda, J, van Keer, K, Bathula, DR, Diaz-Pinto, A, et al. Refuge challenge: a unified framework for evaluating automated methods for glaucoma assessment from fundus photographs. Med Image Anal. (2020) 59:101570. doi: 10.1016/j.media.2019.101570
139. Thompson, AC, Jammal, AA, and Medeiros, FA. A deep learning algorithm to quantify neuroretinal rim loss from optic disc photographs. Am J Ophthalmol. (2019) 201:9–18. doi: 10.1016/j.ajo.2019.01.011
140. Medeiros, FA, Jammal, AA, and Mariottoni, EB. Detection of progressive glaucomatous optic nerve damage on fundus photographs with deep learning. Ophthalmology. (2021) 128:383–92. doi: 10.1016/j.ophtha.2020.07.045
141. Wen, JC, Lee, CS, Keane, PA, Xiao, S, Rokem, AS, Chen, PP, et al. Forecasting future Humphrey visual fields using deep learning. PLoS One. (2019) 14:e0214875. doi: 10.1371/journal.pone.0214875
142. Keenan, TD, Chen, Q, Agrón, E, Tham, Y-C, Goh, JHL, Lei, X, et al. Deeplensnet: deep learning automated diagnosis and quantitative classification of cataract type and severity. Ophthalmology. (2022) 129:571–84. doi: 10.1016/j.ophtha.2021.12.017
143. Tham, Y-C, Goh, JHL, Anees, A, Lei, X, Rim, TH, Chee, M-L, et al. Detecting visually significant cataract using retinal photograph-based deep learning. Nature aging. (2022) 2:264–71. doi: 10.1038/s43587-022-00171-6
144. Nespolo, RG, Yi, D, Cole, E, Valikodath, N, Luciano, C, and Leiderman, YI. Evaluation of artificial intelligence–based intraoperative guidance tools for phacoemulsification cataract surgery. JAMA Ophthalmol. (2022) 140:170–7. doi: 10.1001/jamaophthalmol.2021.5742
145. González-Gonzalo, C, Thee, EF, Klaver, CCW, Lee, AY, Schlingemann, RO, Tufail, A, et al. Trustworthy AI: closing the gap between development and integration of AI systems in ophthalmic practice. Prog Retin Eye Res. (2022) 90:101034. doi: 10.1016/j.preteyeres.2021.101034
146. Natarajan, S, Jain, A, Krishnan, R, Rogye, A, and Sivaprasad, S. Diagnostic accuracy of community-based diabetic retinopathy screening with an offline artificial intelligence system on a smartphone. JAMA Ophthalmol. (2019) 137:1182–8. doi: 10.1001/jamaophthalmol.2019.2923
147. Ting, DSW, Pasquale, LR, Peng, L, Campbell, JP, Lee, AY, Raman, R, et al. Artificial intelligence and deep learning in ophthalmology. Br J Ophthalmol. (2019) 103:167–75. doi: 10.1136/bjophthalmol-2018-313173
148. Mehrabi, N, Morstatter, F, Saxena, N, Lerman, K, and Galstyan, A. A survey on bias and fairness in machine learning. ACM Comput Surv. (2021) 54:1–35. doi: 10.1145/3457607
149. Morley, J, Machado, CCV, Burr, C, Cowls, J, Joshi, I, Taddeo, M, et al. The ethics of AI in health care: a mapping review. Soc Sci Med. (2020) 260:113172. doi: 10.1016/j.socscimed.2020.113172
150. Ahmed, MI, Spooner, B, Isherwood, J, Lane, M, Orrock, E, and Dennison, A. A systematic review of the barriers to the implementation of artificial intelligence in healthcare. Cureus. (2023) 15:e46454. doi: 10.7759/cureus.46454
151. Alsadoun, L, Ali, H, Mushtaq, MM, Mushtaq, M, Burhanuddin, M, Anwar, R, et al. Artificial intelligence (AI)-enhanced detection of diabetic retinopathy from fundus images: the current landscape and future directions. Cureus. (2024) 16:e67844. doi: 10.7759/cureus.67844
152. Wu, H, Jin, K, Yip, CC, Koh, V, and Ye, J. A systematic review of economic evaluation of artificial intelligence-based screening for eye diseases: from possibility to reality. Surv Ophthalmol. (2024) 69:499–507. doi: 10.1016/j.survophthal.2024.03.008
153. Ipp, E, Liljenquist, D, Bode, B, Shah, VN, Silverstein, S, Regillo, CD, et al. Pivotal evaluation of an artificial intelligence system for autonomous detection of referrable and vision-threatening diabetic retinopathy. JAMA Netw Open. (2021) 4:e2134254. doi: 10.1001/jamanetworkopen.2021.34254
154. Ricur, G, and Reyes, J. Surfing the COVID-19 tsunami with teleophthalmology: the advent of new models of eye care. Curr Ophthalmol Rep. (2023) 11:1, 1–12. doi: 10.1007/s40135-023-00308-9
155. Fonda, SJ, Bursell, SE, Lewis, DG, Clary, D, Shahon, D, and Horton, MB. The Indian health service primary care-based teleophthalmology program for diabetic eye disease surveillance and management. Telemed J e-health. (2020) 26:1466–74. doi: 10.1089/tmj.2019.0281
156. Hu, GY, Prasad, J, Chen, DK, Alcantara-Castillo, JC, Patel, VN, and Al-Aswad, LA. Home monitoring of glaucoma using a home tonometer and a novel virtual reality visual field device: acceptability and feasibility. Ophthalmology Glaucoma. (2023) 6:121–8. doi: 10.1016/j.ogla.2022.05.001
157. Kazanskiy, NL, and Khonina, SN. Smart contact lenses-a step towards non-invasive continuous eye health monitoring. Biosensors. (2023) 13:933. doi: 10.3390/bios13100933
158. Xiao, S, Angjeli, E, Wu, HC, Gaier, ED, Gomez, S, Travers, DA, et al. Randomized controlled trial of a dichoptic digital therapeutic for amblyopia. Ophthalmology. (2022) 129:77–85. doi: 10.1016/j.ophtha.2021.09.001
159. Sii, SSZ, Chean, CS, Kuht, H, and Bunce, C. Home-based screening tools for amblyopia: a systematic review. Eye (Lond). (2023) 37:2649–58. doi: 10.1038/s41433-023-02412-3
160. Dole, K, Pakhale, S, Gandhi, A, Deshpande, M, Deshpande, R, and Kulkarni, S. A comparative clinical study of postoperative care by teleophthalmology and in-person consultation at hospital outpatient department after an uncomplicated cataract surgery. Oman J Ophthalmol. (2023) 16:446–51. doi: 10.4103/ojo.ojo_82_22
161. Nikolaidou, A, and Tsaousis, KT. Teleophthalmology and artificial intelligence as game changers in ophthalmic care after the COVID-19 pandemic. Cureus. (2021) 13:e16392. doi: 10.7759/cureus.16392
162. Sim, DA, Mitry, D, Alexander, P, Mapani, A, Goverdhan, S, Aslam, T, et al. The evolution of teleophthalmology programs in the United Kingdom: beyond diabetic retinopathy screening. J Diabetes Sci Technol. (2016) 10:308–17. doi: 10.1177/1932296816629983
163. Ta, AWA, Goh, HL, Ang, C, Koh, LY, Poon, K, and Miller, SM. Two Singapore public healthcare AI applications for national screening programs and other examples. Health Care Sci. (2022) 1:41–57. doi: 10.1002/hcs2.10
164. Schmid, MK, Thiel, MA, Lienhard, K, Schlingemann, RO, Faes, L, and Bachmann, LM. Reliability and diagnostic performance of a novel mobile app for hyperacuity self-monitoring in patients with age-related macular degeneration. Eye (Lond). (2019) 33:1584–9. doi: 10.1038/s41433-019-0455-6
165. Kaiser, PK, Wang, YZ, He, YG, Weisberger, A, Wolf, S, and Smith, CH. Feasibility of a novel remote daily monitoring system for age-related macular degeneration using mobile handheld devices: results of a pilot study. Retina (Philadelphia, Pa). (2013) 33:1863–70. doi: 10.1097/IAE.0b013e3182899258
166. Liu, Y, Holekamp, NM, and Heier, JS. Prospective, longitudinal study: daily self-imaging with home Oct for neovascular age-related macular degeneration. Ophthalmol Retina. (2022) 6:575–85. doi: 10.1016/j.oret.2022.02.011
167. Keenan, TDL, Goldstein, M, Goldenberg, D, Zur, D, Shulman, S, and Loewenstein, A. Prospective, longitudinal pilot study: daily self-imaging with patient-operated home Oct in neovascular age-related macular degeneration. Ophthalmol Sci. (2021) 1:100034. doi: 10.1016/j.xops.2021.100034
168. Solimini, R, Busardò, FP, and Gibelli, F. Ethical and legal challenges of telemedicine in the era of the COVID-19 pandemic. Medicina (Kaunas). (2021) 57:1314. doi: 10.3390/medicina57121314
169. Dolar-Szczasny, J, and Barańska, A. Evaluating the efficacy of teleophthalmology in delivering ophthalmic care to underserved populations: a literature review. J Clin Med. (2023) 12:3161. doi: 10.3390/jcm12093161
170. Andreadis, K, Muellers, K, Ancker, JS, Horowitz, C, Kaushal, R, and Lin, JJ. Telemedicine impact on the patient-provider relationship in primary care during the COVID-19 pandemic. Med Care. (2023) 61:S83–8. doi: 10.1097/mlr.0000000000001808
171. Than, J, Sim, PY, Muttuvelu, D, Ferraz, D, Koh, V, Kang, S, et al. Teleophthalmology and retina: a review of current tools, pathways and services. Int J Retina Vitreous. (2023) 9:76. doi: 10.1186/s40942-023-00502-8
172. Palaniappan, K, Lin, EYT, and Vogel, S. Global regulatory frameworks for the use of artificial intelligence (AI) in the healthcare services sector. Healthcare (Basel, Switzerland). (2024) 12:562. doi: 10.3390/healthcare12050562
173. Mazzuca, D, Borselli, M, Gratteri, S, Zampogna, G, and Feola, A. Applications and current medico-legal challenges of telemedicine in ophthalmology. Int J Environ Res Public Health. (2022) 19:5614. doi: 10.3390/ijerph19095614
174. Gunasekeran, DV, Zheng, F, Lim, GYS, Chong, CCY, Zhang, S, Ng, WY, et al. Acceptance and perception of artificial intelligence usability in eye care (appraise) for ophthalmologists: a multinational perspective. Front Med. (2022) 9:875242. doi: 10.3389/fmed.2022.875242
175. Ahad, A, Ali, Z, Mateen, A, Tahir, M, Hannan, A, Garcia, NM, et al. A comprehensive review on 5g-based smart healthcare network security: taxonomy, issues, solutions and future research directions. Array. (2023) 18:100290. doi: 10.1016/j.array.2023.100290
176. Fitzpatrick, PJ. Improving health literacy using the power of digital communications to achieve better health outcomes for patients and practitioners. Front Digit Health. (2023) 5:1264780. doi: 10.3389/fdgth.2023.1264780
177. Tan, TF, Li, Y, Lim, JS, Gunasekeran, DV, Teo, ZL, Ng, WY, et al. Metaverse and virtual health care in ophthalmology: opportunities and challenges. Asia-Pacific J Ophthalmol (Philadelphia, Pa). (2022) 11:237–46. doi: 10.1097/apo.0000000000000537
178. Kunal, S, Gandhi, P, Rathod, D, Amin, R, and Sharma, S. Securing patient data in the healthcare industry: a blockchain-driven protocol with advanced encryption. J Educ Health Promot. (2024) 13:94. doi: 10.4103/jehp.jehp_984_23
179. Sharafeldin, N, Kawaguchi, A, Sundaram, A, Campbell, S, Rudnisky, C, Weis, E, et al. Review of economic evaluations of teleophthalmology as a screening strategy for chronic eye disease in adults. Br J Ophthalmol. (2018) 102:1485–91. doi: 10.1136/bjophthalmol-2017-311452
180. Anawade, PA, Sharma, D, and Gahane, S. A comprehensive review on exploring the impact of telemedicine on healthcare accessibility. Cureus. (2024) 16:e55996. doi: 10.7759/cureus.55996
181. Emiliani, V, and Entcheva, E. Optogenetics for light control of biological systems. Nat Rev Methods Primers. (2022) 2:2. doi: 10.1038/s43586-022-00136-4
182. Prosseda, PP, and Tran, M. Advances in ophthalmic optogenetics: approaches and applications. Biomol Ther. (2022) 12:269. doi: 10.3390/biom12020269
183. Parnami, K, and Bhattacharyya, A. Current approaches to vision restoration using optogenetic therapy. Front Cell Neurosci. (2023) 17:1236826. doi: 10.3389/fncel.2023.1236826
184. Sahel, JA, Boulanger-Scemama, E, and Pagot, C. Partial recovery of visual function in a blind patient after optogenetic therapy. Nat Med. (2021) 27:1223–9. doi: 10.1038/s41591-021-01351-4
185. Klapoetke, NC, Murata, Y, Kim, SS, Pulver, SR, Birdsey-Benson, A, Cho, YK, et al. Independent optical excitation of distinct neural populations. Nat Methods. (2014) 11:338–46. doi: 10.1038/nmeth.2836
186. Posch, C, Matolin, D, and Wohlgenannt, R. A Qvga 143 Db dynamic range frame-free Pwm image sensor with lossless pixel-level video compression and time-domain Cds. IEEE J Solid State Circuits. (2010) 46:259–75. doi: 10.1109/JSSC.2010.2085952
187. Bi, A, Cui, J, Ma, YP, Olshevskaya, E, Pu, M, Dizhoor, AM, et al. Ectopic expression of a microbial-type rhodopsin restores visual responses in mice with photoreceptor degeneration. Neuron. (2006) 50:23–33. doi: 10.1016/j.neuron.2006.02.026
188. Nirenberg, S, and Pandarinath, C. Retinal prosthetic strategy with the capacity to restore normal vision. Proc Natl Acad Sci USA. (2012) 109:15012–7. doi: 10.1073/pnas.1207035109
189. Batabyal, S, Gajjeraman, S, Pradhan, S, Bhattacharya, S, Wright, W, and Mohanty, S. Sensitization of on-bipolar cells with ambient light activatable multi-characteristic opsin rescues vision in mice. Gene Ther. (2021) 28:162–76. doi: 10.1038/s41434-020-00200-2
190. Wright, W, Gajjeraman, S, Batabyal, S, Pradhan, S, Bhattacharya, S, Mahapatra, V, et al. Restoring vision in mice with retinal degeneration using multicharacteristic opsin. Neurophotonics. (2017) 4:041505. doi: 10.1117/1.NPh.4.4.041505
191. Lindner, M, Gilhooley, MJ, Hughes, S, and Hankins, MW. Optogenetics for visual restoration: from proof of principle to translational challenges. Prog Retin Eye Res. (2022) 91:101089. doi: 10.1016/j.preteyeres.2022.101089
192. Maguire, AM, Simonelli, F, Pierce, EA, Pugh, EN Jr, Mingozzi, F, Bennicelli, J, et al. Safety and efficacy of gene transfer for Leber’s congenital amaurosis. N Engl J Med. (2008) 358:2240–8. doi: 10.1056/NEJMoa0802315
193. Seitz, IP, Michalakis, S, Wilhelm, B, Reichel, FF, Ochakovski, GA, Zrenner, E, et al. Superior retinal gene transfer and biodistribution profile of subretinal versus intravitreal delivery of Aav8 in nonhuman primates. Invest Ophthalmol Vis Sci. (2017) 58:5792–801. doi: 10.1167/iovs.17-22473
194. Yiu, G, Chung, SH, Mollhoff, IN, Nguyen, UT, Thomasy, SM, Yoo, J, et al. Suprachoroidal and subretinal injections of Aav using Transscleral microneedles for retinal gene delivery in nonhuman Primates. Mol Ther Methods Clin Dev. (2020) 16:179–91. doi: 10.1016/j.omtm.2020.01.002
195. Merabet, LB. Building the bionic eye: an emerging reality and opportunity. Prog Brain Res. (2011) 192:3–15. doi: 10.1016/b978-0-444-53355-5.00001-4
196. Wu, KY, Mina, M, Sahyoun, J-Y, Kalevar, A, and Tran, SD. Retinal prostheses: engineering and clinical perspectives for vision restoration. Sensors. (2023) 23:5782. doi: 10.3390/s23135782
197. Finn, AP, Grewal, DS, and Vajzovic, L. Argus ii retinal prosthesis system: a review of patient selection criteria, surgical considerations, and post-operative outcomes. Clin Ophthalmol (Auckland, NZ). (2018) 12:1089–97. doi: 10.2147/opth.s137525
198. Muqit, MMK, Le Mer, Y, Olmos de Koo, L, Holz, FG, Sahel, JA, and Palanker, D. Prosthetic visual acuity with the Prima subretinal microchip in patients with atrophic age-related macular degeneration at 4 years follow-up. Ophthalmol Sci. (2024) 4:100510. doi: 10.1016/j.xops.2024.100510
199. Huang, W, editor. Enhancing the bionic eye: a real-time image optimization framework to encode color and spatial information into retinal prostheses. 2021 IEEE MIT undergraduate research technology conference (URTC). (2021).
200. Zhang, B, Zhang, R, Zhao, J, Yang, J, and Xu, S. The mechanism of human color vision and potential implanted devices for artificial color vision. Front Neurosci. (2024) 18:1408087. doi: 10.3389/fnins.2024.1408087
201. Ruda, K, Zylberberg, J, and Field, GD. Ignoring correlated activity causes a failure of retinal population codes. Nat Commun. (2020) 11:4605. doi: 10.1038/s41467-020-18436-2
202. Lessell, S. What can we expect in neuro-ophthalmology in the next century? Arch Ophthalmol. (2000) 118:553–4. doi: 10.1001/archopht.118.4.553
203. Troyk, PR. The Intracortical visual prosthesis project In: VP Gabel, editor. Artificial vision: a clinical guide. Cham: Springer International Publishing (2017). 203–14.
204. Frederick, RA, and Troyk, PR. Wireless microelectrode arrays for selective and chronically stable peripheral nerve stimulation for Hindlimb movement. J Neural Eng. (2021) 18:056058. doi: 10.1088/1741-2552/ac2bb8
205. Sanie-Jahromi, F, and Azizi, A. Effect of electrical stimulation on ocular cells: a means for improving ocular tissue engineering and treatments of eye diseases. Biomed Res Int. (2021) 2021:6548554. doi: 10.1155/2021/6548554
206. Allen, PJ, and Ayton, LN. Development and experimental basis for the future of prosthetic vision In: A Chang, WF Mieler, and M Ohji, editors. Macular surgery: current practice and trends. Singapore: Springer (2020). 449–62.
207. Normann, RA, and Fernandez, E. Clinical applications of penetrating neural interfaces and Utah electrode array technologies. J Neural Eng. (2016) 13:061003. doi: 10.1088/1741-2560/13/6/061003
208. Fernández, E, Alfaro, A, Soto-Sánchez, C, Gonzalez-Lopez, P, Lozano, AM, Peña, S, et al. Visual percepts evoked with an Intracortical 96-channel microelectrode array inserted in human occipital cortex. J Clin Invest. (2021) 131:e151331. doi: 10.1172/jci151331
209. Barry, MP, Salas, MA, Patel, U, Wuyyuru, V, Niketeghad, S, Bosking, WH, et al. Video-mode percepts are smaller than sums of single-electrode phosphenes with the Orion® visual cortical prosthesis. Invest Ophthalmol Vis Sci. (2020) 61:927.
210. Kosta, P, Paknahad, J, Rodríguez, ESG, Loizos, K, Roy, A, Talbot, N, et al. Electromagnetic safety assessment of a cortical implant for vision restoration. IEEE J Electromagnet. (2018) 2:56–63. doi: 10.1109/JERM.2018.2812302
211. Wang, V, and Kuriyan, AE. Optoelectronic devices for vision restoration. Curr Ophthalmol Rep. (2020) 8:69–77. doi: 10.1007/s40135-020-00232-2
212. Oswalt, D, Bosking, W, Sun, P, Sheth, SA, Niketeghad, S, Salas, MA, et al. Multi-electrode stimulation evokes consistent spatial patterns of phosphenes and improves phosphene mapping in blind subjects. Brain Stimul. (2021) 14:1356–72. doi: 10.1016/j.brs.2021.08.024
213. Nishida, K, Sakaguchi, H, Kamei, M, Cecilia-Gonzalez, C, Terasawa, Y, Velez-Montoya, R, et al. Visual sensation by electrical stimulation using a new direct optic nerve electrode device. Brain Stimul. (2015) 8:678–81. doi: 10.1016/j.brs.2015.03.001
214. Liu, X, Chen, P, Ding, X, Liu, A, Li, P, Sun, C, et al. A narrative review of cortical visual prosthesis systems: the latest progress and significance of nanotechnology for the future. Ann Transl Med. (2022) 10:716. doi: 10.21037/atm-22-2858
215. Rosenfeld, JV, Wong, YT, Yan, E, Szlawski, J, Mohan, A, Clark, JC, et al. Tissue response to a chronically implantable wireless intracortical visual prosthesis (Gennaris Array). J Neural Eng. (2020) 17:046001. doi: 10.1088/1741-2552/ab9e1c
216. Lowery, AJ, Rosenfeld, JV, Lewis, PM, Browne, D, Mohan, A, Brunton, E, et al. Restoration of vision using wireless cortical implants: the Monash vision group project. Annual international conference of the IEEE engineering in medicine and biology society IEEE engineering in medicine and biology society annual international conference (2015), 1041–1044.
Glossary
3D - Three-dimensional
AAVs - Adeno-associated viruses
ABMSCs - Autologous bone marrow mesenchymal stem cells
ADMSCs - Adipose-derived MSCs
AI - Artificial intelligence
AMD - Age-related macular degeneration
AO - Adaptive optics
AOSLO - AO with scanning laser ophthalmoscopy
AUC - Areas under the curve
AV-DONE - Artificial Vision by Direct Optic Nerve Electrode
BCI - Brain-computer interface
cGVHD - Chronic graft-versus-host disease
CLET - Cultivated limbal epithelium transplantation
CNGA3 - Cyclic nucleotide-gated cation channel alpha-3
CNGB3 - Cyclic nucleotide-gated cation channel beta-3
CNNs - Convolutional neural networks
cSLO - Confocal scanning laser ophthalmoscopy
DED - Dry eye disease
DME - Diabetic macular edema
FA - Fluorescein angiography
FDA - Food and Drug Administration
hESC - Human embryonic stem cell
ICG - Indocyanine green angiography
ICVP - Intracortical Visual Prosthesis
IOP - Intraocular pressure
LCA - Leber congenital amaurosis
LSCD - Limbal stem cell deficiency
LSCs - Limbal stem cells
mfERG - Multifocal electroretinography
MSCs - Mesenchymal stem cells
OCT - Optical coherence tomography
OCTA - Optical coherence tomography angiography
RGCs - Retinal ganglion cells
RPCs - Retinal progenitor cells
RPE - Retinal pigment epithelium
RPGR - Retinitis pigmentosa GTPase regulator
SLET - Simple limbal epithelial transplantation
SS-OCT - Swept-source optical coherence tomography
STGD1 - Stargardt disease type 1
TGF-β - Transforming growth factor-beta
TMSCs - Trabecular meshwork stem cells
UBM - Ultrasound biomicroscopy
VEGF - Vascular endothelial growth factor
Keywords: gene therapy, stem cell therapy, nanotechnology, artificial intelligence, teleophthalmology, optogenetics, bionics, neuro-ophthalmology
Citation: Lin F, Su Y, Zhao C, Akter F, Yao S, Huang S, Shao X and Yao Y (2025) Tackling visual impairment: emerging avenues in ophthalmology. Front. Med. 12:1567159. doi: 10.3389/fmed.2025.1567159
Edited by:
Kanwal Ken Nischal, University of Pittsburgh Medical Center, United StatesReviewed by:
Ante Orbanić, Clinical Hospital Dubrava, CroatiaKim Merrill, University of Minnesota Health Sciences, University of Minnesota Medical Center, United States
Copyright © 2025 Lin, Su, Zhao, Akter, Yao, Huang, Shao and Yao. This is an open-access article distributed under the terms of the Creative Commons Attribution License (CC BY). The use, distribution or reproduction in other forums is permitted, provided the original author(s) and the copyright owner(s) are credited and that the original publication in this journal is cited, in accordance with accepted academic practice. No use, distribution or reproduction is permitted which does not comply with these terms.
*Correspondence: Fang Lin, MTQzNjg5ODk1MEBxcS5jb20=; Xiaodong Shao, c2hhb3hkZG9jdG9yQGhvdG1haWwuY29t; Yizheng Yao, eXp5YW8yOEBzdWRhLmVkdS5jbg==
†These authors have contributed equally to this work