- 1Department of Psychiatry, Yale University School of Medicine, New Haven, CT, United States
- 2Institute for International Internet Interventions for Health (i4Health), Palo Alto University, Palo Alto, CA, United States
- 3Department of Psychiatry and Behavioral Sciences, University of California, San Francisco, San Francisco, CA, United States
- 4School of Mathematical and Statistical Sciences, University of Texas Rio Grande Valley, Edinburg, TX, United States
Introduction: Adults from low-income backgrounds who smoke face significant health disparities related to tobacco use, often at disproportionately high rates. These individuals are more likely to endure multiple mental and physical (MP) health conditions, which can negatively influence their self-rated health (SRH). The quality and effectiveness of patient-clinician communication (PCC) can influence how patients perceive their own health. Understanding how PCC influences SRH among low-income adults who smoke and suffer from multiple MP conditions is essential for clinical care as multimorbidity is on the rise. This study examines how PCC may influence the health perceptions of low-income adults who smoke and have varying MP conditions.
Methods: Low-income adults who smoke (N = 58) were recruited from the San Francisco Health Network (SFHN) and were assessed for number of MP conditions, PCC, and SRH. A moderation analysis was performed to examine whether PCC moderated relations between MP conditions and SRH. Follow-up analyses were conducted to examine differences and relationships among variables. In planned exploratory analysis, all possible choices for moderator-independent-dependent-variable selections to explore the best model fit were conducted.
Results: The results revealed that PCC moderated the association between MP conditions (p < 0.05) and SRH. In follow-up analyses, number of MP conditions predicted poorer SRH for low-income smokers who experienced low (p < 0.001) and average (p < 0.01) levels of PPC but not high levels of PCC. In planned exploratory analysis, based on the Akaike Information Criterion, a quantitative basis for considering SRH as the dependent variable was established.
Conclusion: The intersection of tobacco-related disparities among low-income adults who smoke and manage multiple MP conditions is complex. Among this vulnerable population, poor and average PCC adversely influences how patients perceive their own health. Results highlight the importance of quality and effective communication between patients and providers. A culturally informed patient-centered approach to care may improve PCC as it encourages collaborative, individually tailored treatment that empowers patients to actively participate in their own health care.
1 Introduction
Smoking remains a modifiable health risk that affects every organ in the body and is the leading cause of global preventable death and preventable disease (1, 2). Health disparities are directly linked to tobacco disparities (3, 4) and low-income people who smoke suffer from multiple social, medical, and psychological needs at disproportionate rates compared to those from higher income strata (5, 6). Like tobacco disparities, multimorbidity—the coexistence of two or more chronic conditions which can include a physical non-communicable disease of long duration, a mental health condition of long duration, and/or an infectious disease of long duration (7, 8)—is also linked to health disparities (8, 9). Research has demonstrated that individuals belonging to the lowest level of income are four times more likely to experience multimorbidity compared with the highest level of income (10) and smoking has been found to be one of the primary predictors of multimorbidity (11–13).
Self-rated health (SRH) is a brief, single proxy measure of overall health and is the most widely used measure of health in medical, social, and behavioral science research that utilizes survey data (14, 15). It has been widely validated and is a critical indicator and strong independent predictor of morbidity and mortality even after adjusting for covariates including demographic, mental health, physiological, and behavioral risk factors (16, 17). As such, individuals with poor SRH tend to be in mental, physical, and/or social distress (18). Individuals who indicate poorer self-rated health tend to suffer disproportionately from health disparities (19) and the cycle of poor health within low-income populations has been extensively documented (20, 21). Behavioral risk factors, such as smoking, have been identified as key components that impact SRH (22, 23). Prior research demonstrates that frequent smoking is associated with over twice the odds of reporting poor SRH (22). Likewise, SRH is influenced by multimorbidity which may be due to the physiological consequences and psychological distress of managing multiple conditions (8, 24). Managing multiple chronic conditions in turn affects daily functioning and consequently individuals with multimorbidity tend to experience a decline in their quality of life (25). Thus, multimorbidity has been found to be negatively associated with SRH (26, 27).
Patient-clinician communication (PCC) plays an integral role in the management of mental and physical conditions (28). While there is no general consensus regarding the operational definition of PCC (29), in the current study PCC is defined as the extent to which a patient prepares for appointments, seeks clarification through active questioning, and engages in personal disclosure to enhance understanding and treatment outcomes. This definition highlights both the patient’s responsibility to actively engage in their care while also recognizing the clinician’s responsibility to foster trust (e.g., creating space for patients to share relevant personal concerns that may impact their health and/or treatment) and open communication. Quality PCC has been associated with greater satisfaction of care, medication adherence, and better health outcomes (28, 30–32). PCC is particularly important with low-income and underrepresented populations who tend to suffer disproportionately from health disparities (33, 34). Some factors that may deleteriously impact PCC in low-income and underrepresented populations include unmet social needs (e.g., food and housing insecurity), lower education levels, acculturation level, discrimination, immigrant status, language barriers (e.g., limited English proficiency), and low health literacy (28, 35). These patient-level factors impact PCC on various levels. For example, patients with limited English proficiency may experience challenges in understanding and adhering to medical advice from providers which could result in further delays in receiving appropriate medical care and medical errors (36). Similarly, patients with low health literacy have difficulty disclosing health related information due to limited health knowledge and have difficulty following treatment instructions prescribed to them by their providers leading to poorer health outcomes (37–39).
In addition to the aforementioned factors, low-income people who smoke who are at a higher risk for multimorbidity also have a greater risk for experiencing adverse health outcomes, receiving conflicting treatment recommendations due to having multiple clinicians and conditions, and experience greater self-management burdens (8, 40, 41). Thus, these patients have a greater need for clear and effective PCC. Barriers to effective PCC such as limited English proficiency and low health literacy have been linked to poorer SRH even after adjusting for covariates (42). SRH is linked to communication inequalities—differences in socioeconomic status (SES), race, gender that impact access and ability to take advantage of the information given by providers—that are impacted by the social determinants of health with health information avoidance mediating this relationship (43). Conversely, quality PCC is associated with better SRH and better patient-clinician relationships (31). Quality PCC is associated with higher treatment satisfaction, medication adherence, better self-management, reducing stigma, and higher subjective decision quality, all of which may positively impact SRH (30, 32, 44–46).
Low-income people who smoke experience health disparities and are more likely to suffer from multimorbidity, both of which adversely impact patient SRH (12, 13, 33, 34). Similarly, depending on its quality and effectiveness, PCC can impact SRH adversely or positively (28, 31, 32, 45). Although existing literature has examined various elements of PCC across different healthcare settings, there is a gap in the literature regarding how PCC influences SRH among low-income people who smoke and who suffer from multiple chronic conditions. Understanding this interaction is essential as multimorbidity has proven to be an escalating global issue with considerable clinical implications, especially for those who are socioeconomically deprived and smoke (8). Therefore, the primary aim of the current study examines how the number of mental and physical (MP) conditions and PCC interact and relate to SRH. We hypothesized that PCC would moderate the relationship between number of MP and SRH, where greater PCC would result in better SRH. In planned exploratory analysis, we examined all possible choices for moderator-independent-dependent-variable selections to explore the best model fit.
2 Methods
2.1 Participants and procedures
The current study is based on secondary analyses from an outcome study that was initially developed to explore differences between low-income people who smoke with and without chronic illnesses in terms of nicotine dependence, depression, anxiety, and smoking abstinence self-efficacy. Participants were recruited from primary care clinics within the San Francisco Health Network (SFHN) and other sites in the San Francisco Bay Area, that primarily serve low-income individuals. Recruitment flyers were posted in waiting rooms and other locations with the approval of each of these sites. Staff at various recruitment sites were also provided with flyers to inform prospective participants about the study. Eligible participants were English-speaking, low-income, as defined by the poverty threshold for San Francisco Bay Area residents (47), adults (aged ≥18 years) who smoke and have thought about or intended to quit smoking within 30 days. All procedures were approved by the University of California, San Francisco and Palo Alto University.
2.2 Measures
2.2.1 Demographic questionnaire
Participants reported their age, race, ethnicity, gender, marital status, employment status, total household income, and years of education.
2.2.2 Number of mental and physical (MP) conditions
Four different measures were used to assess for mental and physical conditions. To assess for mental health conditions, participants completed the Generalized Anxiety Disorder – 7 (GAD-7) (48) and the Patient Health Questionnaire (PHQ-9) (49). Cut off scores for the GAD-7 and PHQ-9 were ≥8 and ≥10, respectively, to categorize participants as screening positive for a diagnosable generalized anxiety disorder and/or major depressive episode (50). Nicotine dependence was conceptualized as a mental health condition for this study and to assess for nicotine dependence, participants completed the Fagerström Test of Nicotine Dependence (FTND). A cut-off score of 6 was utilized to determine whether a participant was dependent on nicotine (51).
To assess for chronic physical conditions, a self-report questionnaire asking for the presence or absence of 22 chronic illnesses over the past year was administered, modeled after the physical health self-report module described by Atwoli et al. (52). The list of chronic physiological conditions included: high blood pressure, high cholesterol, heart attack, stroke, heart disease, asthma, seasonal allergies, other lung disease, diabetes, thyroid condition, osteoporosis, acid reflux, ulcer, obesity, arthritis, chronic pain, frequent headaches, neurological disease, epilepsy, cancer, kidney disease, and other, to be specified by the participant.
2.2.3 Self-rated health
Self-rated health was measured by a well-established single item developed by the Stanford Patient Education Research Center, which has been widely used in diverse samples to assess for perceived general health status (42, 53, 54). The item asked, “In general, would you say your health is…” There were five response options: 1 (“Excellent”), 2 (“Very good”), 3 (“Good”), 4 (“Fair”) and 5 (“Poor”). Higher scores on this scale signify the patient perceives their health as worse.
2.2.4 Patient-clinician communication
Patient-clinician communication was measured by a three-item questionnaire developed by the Stanford Patient Education Research Center (54). The three items begin with, “When you visit your doctor, how often do you do the following” and include the following: (1) “Prepare a list of questions for your doctor…,” (2) “Ask questions about the things you want to know and things you do not understand about your treatment…,” and (3) “Discuss any personal problems that may be related to your illness…” Responses are coded on a 6-point Likert scale ranging from 0 “Never” to 5 “Always.” The total score is the mean of the three items. Higher scores indicate better communication.
2.3 Statistical analyses
2.3.1 Power estimates
An a priori power analysis was performed for sample size estimation using G*Power (55) version 3.1.9.2. This study was designed for adequate power for a moderated regression that examined the interaction between two predictors (number of MP and PCC). Power calculations were conducted based on a 0.05 alpha level and 80% power (1-β). These calculations suggested that for a moderated regression, total sample size of 25 to 55 is required to detect a medium to large effect size via Cohen’s ƒ2 (0.15 ≤ effect size ≥ 0.35). Given these power approximations, we expected our study sample of 58 to be appropriately powered to detect the hypothesized effects.
2.3.2 Data processing
All records for which a patient had missing data, be that a missing yes/no for a health condition or a missed question on an instrument such as PCC were removed; a total of 6 participants were removed.
2.3.3 Descriptive and statistical model analyses
Analyses for the primary aim were performed using IBM SPSS Statistics for Macintosh Version 29 (IBM Corp., Armonk, N.Y., USA). Prior to analyses, bivariate correlations were examined among predictor variables to test for multicollinearity and dependent variable normality was confirmed. To examine the interplay between number of MP conditions, PCC, and SRH, a moderation analysis was performed using Hayes PROCESS Model 1 (56). The model included SRH as the criterion, with number of MP conditions, PCC, and number of MP conditions-by-PCC interaction term as fixed effects. We included age (24–70 years old), gender, race, and years of education as covariates in the model. To clarify the direction of a significant interaction, we performed follow-up tests that examined associations between number of chronic conditions and SRH at low, average, and high levels of PCC, where low and high represent 1 standard deviation below and above the group mean, respectively.
2.3.4 Moderation model comparisons for exploratory analyses
Under moderation (57), the dependent variable Y, the independent variable X, and the moderator M are related via:
The models for planned exploratory analyses were run in Python 3.9.12. Parameters were computed via least squares using the Numpy library (59). Normality was tested for with the Shapiro-Wilks Test from the SciPy library (60) at a p-value of 0.05.
3 Results
3.1 Participant characteristics
The total sample consisted of 58 low-income primary care patients who smoke. The age ranged between 24 and 70 years (44.97 ± 10.69). The mean years of education attainment was 12.05 ± 2.82 years of schooling. Most participants identified as cisgender males (n = 37; 63.8%) and about one third identified as cisgender females (n = 20; 34.5%), with one participant identifying as transgender. Most participants identified as not Latinx/Hispanic (n = 35; 60.3%), Non-Hispanic White (n = 20; 34.5%), and African American/Black (n = 18; 31.0%). The remainder of the participants identified as Asian (n = 1; 1.7%), Mestizo (n = 2; 3.4%), Native American/Alaskan Native (n = 3; 5.2%), Native Hawaiian/Pacific Islander (n = 2; 3.4%), Biracial (n = 11; 19.0%), or an unknown or unreported racial identity (n = 1; 1.7%). Most participants reported that they were single/never married (n = 35; 60.3%), followed by married or in a domestic partnership (n = 10; 17.2%), separated (n = 3; 5.2%), divorced (n = 7; 12.1%), and widowed (n = 3; 5.2%). Most participants reported being unemployed (n = 42; 72.4%) and reported a total household income of less than $20,000 per year (n = 47; 81.0%). Many participants reported having three or more clinical conditions within the past year (n = 26; 44.8%). Characteristics of the sample are summarized in Table 1.
3.2 Moderation analysis
3.2.1 Interaction between number of MP conditions and PCC on SRH
Results indicate that the overall moderation model was significant (p < 0.01) and a significant number of MP conditions-by-PCC interaction for SRH was observed [β = −0.09, (95% CI, −0.17 to −0.02), p < 0.05]. Further interrogation of this interaction revealed that number of MP conditions predicted SRH at low and average levels of PCC [Low: β = 0.26, (95% CI, 0.10–0.43), p < 0.001]; [Average: β = 0.13, (95% CI, 0.03–0.23), p < 0.01] but not high levels of PCC [High: β = −0.01, (95% CI, −0.13 – 0.12), p = 0.93]. Estimated marginal means of linear trends for this model can be found in Table 2 and a graphical representation is located in Figure 1; for more in-depth perspective of the moderation, that includes the moderation predictions and the raw data, see Figure 2. Results also indicated that covariates had no significant influence on the IV.
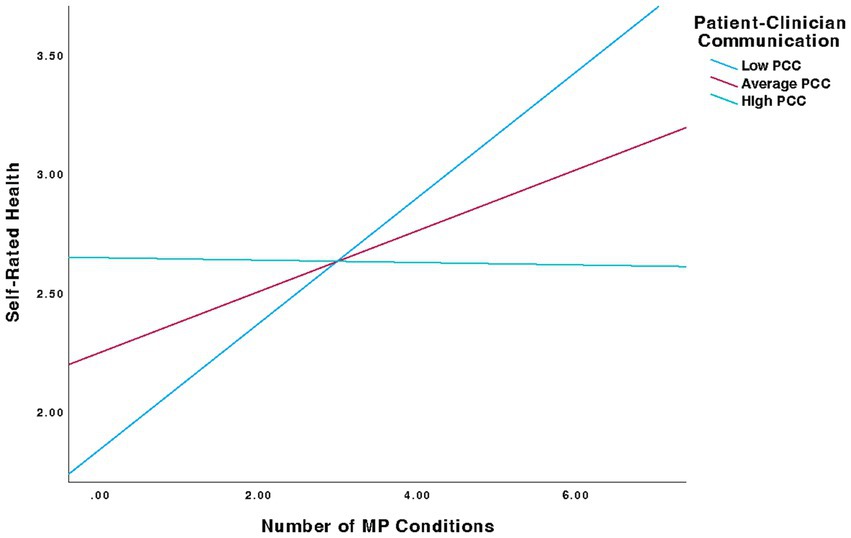
Figure 1. Moderation analysis illustrating the interaction between number of MP conditions (X) and patient-clinician communication (M) in predicting self-rated health (Y); results indicate a significant interaction effect (p <.05).
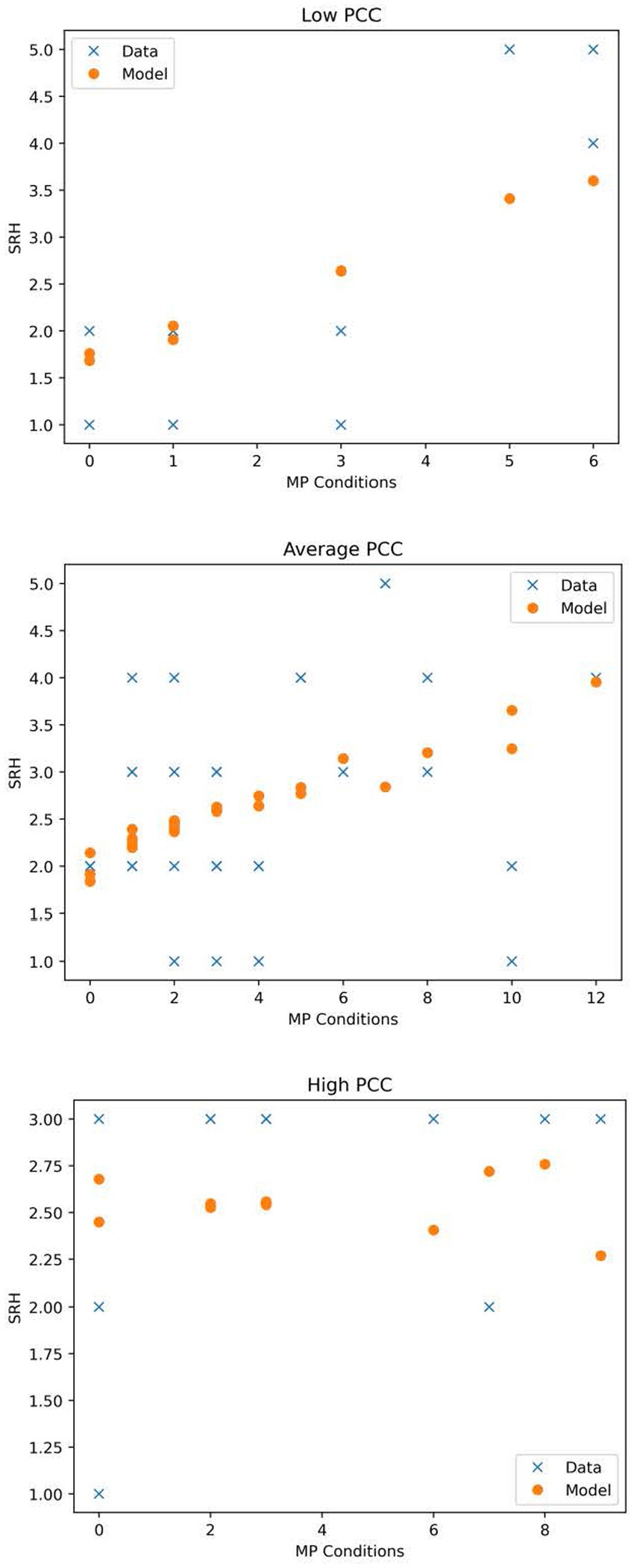
Figure 2. Expanded visualization of the interaction between number of MP conditions and patient-clinician communication in predicting self-rated health. This figure depicts both the moderation model (see equation) and raw data for patients with low (1 or more standard deviations below the mean), average (within one standard deviation of the mean), and high (1 or more standard deviations above the mean) levels of patient-clinician communication.
3.3 Exploratory analyses
A summary of the different moderation models can be found in Table 3. The best models due to the lowest AIC-value have SRH as the dependent variable with PCC and number of MP conditions both being equally good moderators/independent variables. This is to be expected because, based on the form of the model, X and M can be switched and the model is the same. The AICs were not reported with number of MP conditions being a dependent variable because the errors were not normally distributed. The data thereby provide a quantitative basis for making SRH a dependent variable, with either PCC or number of MP conditions being the moderator.
4 Discussion
The results of this study magnify the significance of culturally tailoring interventions that aim to improve PCC for health disparity and minority populations. The management of multiple conditions, each with their own complex set of treatment regimens prescribed by different providers, is challenging (8). Clear and effective PCC is especially important for low-income people who smoke as they are significantly impacted by pervasive social inequities in health and are more likely to suffer from multimorbidity and its associated adverse health outcomes (10, 11, 13, 28, 30). Our primary findings indicate that PCC plays a role in how low-income people who smoke and have multiple health conditions perceive their overall health. In a moderation model, we observed an interaction between number of MP conditions and PCC on SRH, where the association between number of MP conditions and SRH differed according to low, average, or high levels of PCC. Specifically, for low-income primary care patients who indicated low (i.e., 1 standard deviation below the mean) or average PCC, number of MP conditions was associated with poorer SRH, whereas number of MP conditions and SRH were unrelated among patients who indicated high PCC. Additionally, findings from exploratory analyses indicated that the direction of the conceptual model represented in the study holds strong quantitatively as well. The exploratory findings suggest that an interaction between the number of MP conditions and the quality of PCC does indeed impact SRH.
These findings suggest that poor and average PCC may adversely influence how low-income people who smoke and have multiple conditions may perceive their own health. This is consistent with a study that found a strong association between the patient-provider relationship and SRH among vulnerable patients; additionally, the study found that good patient-provider relationships were also associated with quality of care such as patient satisfaction and health outcomes (31). Previous studies have drawn similar conclusions and have found that PCC directly impacts health outcomes (61). For example, in a recent study, patients with uncontrolled blood pressure were more likely to improve their hypertensive outcomes when being treated by physicians who underwent a communication skills training program (62). Likewise, in another study, Black patients who report high levels of communication with their physicians were more likely to adhere to their medication regimen (30). Higher levels of PCC may make a significant difference in a patient’s quality of life and impact future morbidity and mortality given its direct influence over health outcomes. Interestingly, the findings also indicated that MP conditions and SRH were unrelated among patients who indicated high PCC. While current research indicates that effective PCC is often associated with positive health outcomes, studies have also demonstrated that PCC may not always have a significant impact on SRH (63). Multiple factors such as organizational and heath care system culture, being proactive as a patient, and health literacy may significantly contribute to SRH among those with multiple chronic illnesses despite effective PCC (31, 64).
Quality PCC is particularly important as it may serve as a function to reduce or maintain health disparities among vulnerable populations such as low-income people who smoke. PCC is influenced by SES in that providers tend to give fewer positive socio-emotional responses, engage in a directive consultation style, and provide less information and direction to lower SES patients while higher SES patients tend to experience a more egalitarian model of patient-provider communication (65). Among low-income patients, having a more egalitarian doctor-patient relationship (e.g., patient centered care) has been found to have better ratings of SRH whereas more traditional paternalistic doctor-patient relationship has been associated with worse SRH (66). It is important to note that socioeconomic status (SES) is merely one aspect of a patient’s multiple intersecting identities that may influence a provider’s communication style; ineffective communication that fails to account for the multiple cultural nuances of one’s identity may prove detrimental during a significant clinical encounter.
A patient centered approach to care is one such method that may improve PCC among low-income people who smoke and lead to more culturally informed and context-based interventions during clinical encounters. Health care providers who engage in patient-centered care are encouraged to take a collaborative approach in which patients and providers design personalized care together; this provides patients with high-quality, individually tailored care that may improve the efficiency and effectiveness of health care systems (67). Patient centered care has been found to improve provider behaviors—e.g., interpersonal skills, history taking, counseling—during clinical encounters with minoritized patients (68) and empowers patients to engage in shared decision making in their own health care (69).
Effective and productive communication during clinical encounters is also influenced by a patients’ level of active participation (70). While there is a vast literature focused on improving provider communication in clinical encounters, research that centers on training patients to interact more productively with their providers is largely underdeveloped (70). Patients who are active communicators with their providers tend to state their treatment preferences more frequently, experience improved psychological wellbeing, and are more likely to check for understanding to confirm the factors and issues being discussed during clinical encounters (71–73). With support and resources, encouraging low-income patients to improve their communication skills can be both achievable and impactful. Teaching and empowering underserved populations to become effective communicators with their health care providers has been found to improve health outcomes (70), which may also play a role in alleviating health disparities. Likewise, educating patients on how to engage more effectively with their providers may improve health literacy, build confidence in navigating the healthcare system, and empower them to demonstrate more respectful and assertive communication (70, 72, 74, 75). By providing patients with skills to prepare for appointments, seek clarification through active questioning, and engage in open communication about their concerns, we can foster stronger, more collaborative relationships, ultimately leading to better health outcomes.
This study has several limitations. First, the cross-sectional nature of the research design limits our ability to make causal interpretations of the results. Although we were able to gather meaningful insights on when PCC may impact SRH among low-income people who smoke, we did not measure changes through time. Second, although adequately powered for the analyses that were run, the study had a relatively small sample size which limited our ability to run more robust analyses. Third, given that the sample consists of low-income people who smoke who were recruited from an urban area of the United States, we are unable to generalize to other low-income populations who reside in rural areas or areas outside of the United States. In addition, given the voluntary nature of the study, the results presented cannot be generalized to individuals who chose not to participate. Fourth, this study relied on self-report data; the mental and physical conditions were not objectively measured by providers who could assess and draw conclusions for formal diagnoses. Fifth, the three item PCC measure utilized in this study focuses exclusively on the patient perspective of PCC, which may result in an oversimplified view of a complex relationship between patient and provider; however, it is important to note that the provider bears the responsibility to prioritize patient values, needs, and preferences throughout the provision of care (64). Sixth, the authors recognize that there are other unmeasured factors that may influence the moderation model reported in this study such as different aspects of the social determinants of health (e.g., discrimination, access to care, health literacy) and medical mistrust. Despite these limitations however, the study team was able to draw meaningful conclusions on the perceptions of low-income people who smoke, particularly in the context of their varying levels of communication quality with healthcare providers. Furthermore, this study provides additional insights into a population that is greatly impacted by the social determinants of health.
Future research in this area is warranted. Future intervention research focused on provider-patient communication may consider a motivational interviewing (MI) approach which takes a collaborative, acceptance based, compassionate, and empowering approach to care (76). Additionally, it may be important for providers to receive training on how to conceptualize a patient’s readiness for change while prioritizing patient well-being. Providing training to healthcare providers on person-centered, collaborative treatment approaches in low-income clinical settings may improve SRH. Future studies may consider a longitudinal approach to better understand how SRH may change over time in low-income people who smoke based on changes in PCC quality. Finally, future investigations focused on teaching patients how to interact more productively—e.g., interpersonal effectiveness skills training—with their providers may improve PCC.
Data availability statement
The datasets presented in this article are not readily available because of confidentiality regarding the involvement of a vulnerable population. Requests to access the datasets should be directed to bW9uaXF1ZS5jYW5vQHlhbGUuZWR1.
Ethics statement
The studies involving humans were approved by the University of California, San Francisco Committee on Human Research (15-17597) and the Palo Alto University Institutional Review Board (15-042-H). The studies were conducted in accordance with the local legislation and institutional requirements. The participants provided their written informed consent to participate in this study.
Author contributions
MC: Conceptualization, Formal analysis, Funding acquisition, Investigation, Methodology, Software, Writing – original draft. ML: Formal analysis, Writing – review & editing. RM: Funding acquisition, Investigation, Project administration, Resources, Supervision, Validation, Visualization, Writing – review & editing.
Funding
The author(s) declare that financial support was received for the research and/or publication of this article. This research was supported by the Tobacco-Related Disease Research Program (24RT-0027 [MTC and RFM]). Manuscripts writing was supported by the National Institute on Drug Abuse (5K12DA000167–33 [MTC]).
Conflict of interest
The authors declare that the research was conducted in the absence of any commercial or financial relationships that could be construed as a potential conflict of interest.
Generative AI statement
The authors declare that no Gen AI was used in the creation of this manuscript.
Publisher’s note
All claims expressed in this article are solely those of the authors and do not necessarily represent those of their affiliated organizations, or those of the publisher, the editors and the reviewers. Any product that may be evaluated in this article, or claim that may be made by its manufacturer, is not guaranteed or endorsed by the publisher.
References
1. National Center for Chronic Disease Prevention and Health Promotion (US) Office on Smoking and Health. The health consequences of smoking—50 years of Progress: a report of the surgeon general [internet]. Atlanta (GA): Centers for Disease Control and Prevention (US); (2014). Available online at: http://www.ncbi.nlm.nih.gov/books/NBK179276/ (Accessed December 20, 2022).
2. World Health Organization. WHO report on the global tobacco epidemic, 2017: Monitoring tobacco use and prevention policies [internet]. Geneva: World Health Organization; (2017). 135. Available online at: https://apps.who.int/iris/handle/10665/255874 (Accessed December 20, 2022).
3. Kingsbury, JH, D’Silva, J, O’Gara, E, Parks, MJ, and Boyle, RG. How much Progress have we made? Trends in disparities in tobacco use. Prev Chronic Dis. (2020) 17:E107. doi: 10.5888/pcd17.200090
4. Sheffer, CE, Williams, JM, Erwin, DO, Smith, PH, Carl, E, and Ostroff, JS. Tobacco-related disparities viewed through the Lens of intersectionality. Nicotine Tob Res. (2021) 24:285–8. doi: 10.1093/ntr/ntab193
5. Cano, MT, Pennington, DL, Reyes, S, Pineda, BS, Llamas, JA, Periyakoil, VS, et al. Factors associated with smoking in low-income persons with and without chronic illness. Tob Induc Dis. (2021) 19:1–11. doi: 10.18332/tid/138241
6. Jamal, A, Phillips, E, Gentzke, AS, Homa, DM, Babb, SD, King, BA, et al. Current cigarette smoking among adults — United States, 2016. MMWR Morb Mortal Wkly Rep. (2018) 67:53–9. doi: 10.15585/mmwr.mm6702a1
7. The Lancet. Making more of multimorbidity: an emerging priority. Lancet. (2018) 391:1637. doi: 10.1016/S0140-6736(18)30941-3
8. Skou, ST, Mair, FS, Fortin, M, Guthrie, B, Nunes, BP, Miranda, JJ, et al. Multimorbidity. Nat Rev Dis Primers. (2022) 8:48. doi: 10.1038/s41572-022-00376-4
9. Dugravot, A, Fayosse, A, Dumurgier, J, Bouillon, K, Rayana, TB, Schnitzler, A, et al. Social inequalities in multimorbidity, frailty, disability, and transitions to mortality: a 24-year follow-up of the Whitehall II cohort study. Lancet Public Health. (2020) 5:e42–50. doi: 10.1016/S2468-2667(19)30226-9
10. Ingram, E, Ledden, S, Beardon, S, Gomes, M, Hogarth, S, McDonald, H, et al. Household and area-level social determinants of multimorbidity: a systematic review. J Epidemiol Community Health. (2021) 75:232–41. doi: 10.1136/jech-2020-214691
11. Freisling, H, Viallon, V, Lennon, H, Bagnardi, V, Ricci, C, Butterworth, AS, et al. Lifestyle factors and risk of multimorbidity of cancer and cardiometabolic diseases: a multinational cohort study. BMC Med. (2020) 18:1–11. doi: 10.1186/s12916-019-1474-7
12. Katikireddi, SV, Skivington, K, Leyland, AH, Hunt, K, and Mercer, SW. The contribution of risk factors to socioeconomic inequalities in multimorbidity across the lifecourse: a longitudinal analysis of the Twenty-07 cohort. BMC Med. (2017) 15:152. doi: 10.1186/s12916-017-0913-6
13. Shang, X, Peng, W, Wu, J, He, M, and Zhang, L. Leading determinants for multimorbidity in middle-aged Australian men and women: a nine-year follow-up cohort study. Prev Med. (2020) 141:106260. doi: 10.1016/j.ypmed.2020.106260
14. Garbarski, D. Research in and prospects for the measurement of health using self-rated health. Public Opin Q. (2016) 80:977–97. doi: 10.1093/poq/nfw033
15. Layes, A, Asada, Y, and Kephart, G. Whiners and deniers – what does self-rated health measure? Soc Sci Med. (2012) 75:1–9. doi: 10.1016/j.socscimed.2011.10.030
16. DeSalvo, KB, Fan, VS, McDonell, MB, and Fihn, SD. Predicting mortality and healthcare utilization with a single question. Health Serv Res. (2005) 40:1234–46. doi: 10.1111/j.1475-6773.2005.00404.x
17. Idler, EL, and Benyamini, Y. Self-rated health and mortality: a review of twenty-seven community studies. J Health Soc Behav. (1997) 38:21–37. doi: 10.2307/2955359
18. Byun, M, Kim, E, and Ahn, H. Factors contributing to poor self-rated health in older adults with lower income. Healthcare (Basel). (2021) 9:1515. doi: 10.3390/healthcare9111515
19. Moor, I, Spallek, J, and Richter, M. Explaining socioeconomic inequalities in self-rated health: a systematic review of the relative contribution of material, psychosocial and behavioural factors. J Epidemiol Community Health. (2017) 71:565–75. doi: 10.1136/jech-2016-207589
20. Kondo, N, Sembajwe, G, Kawachi, I, van Dam, RM, Subramanian, SV, and Yamagata, Z. Income inequality, mortality, and self rated health: meta-analysis of multilevel studies. BMJ. (2009) 339:b4471. doi: 10.1136/bmj.b4471
21. Marmot, M. Social determinants of health inequalities. Lancet. (2005) 365:1099–104. doi: 10.1016/S0140-6736(05)71146-6
22. Mauro, PM, Canham, SL, Martins, SS, and Spira, AP. Substance-use coping and self-rated health among US middle-aged and older adults. Addict Behav. (2015) 42:96–100. doi: 10.1016/j.addbeh.2014.10.031
23. Ortiz, C, López-Cuadrado, T, Rodríguez-Blázquez, C, Pastor-Barriuso, R, and Galán, I. Clustering of unhealthy lifestyle behaviors, self-rated health and disability. Prev Med. (2022) 155:106911. doi: 10.1016/j.ypmed.2021.106911
24. Marques, A, Peralta, M, Gouveia, ÉR, Chávez, FG, and Valeiro, MG. Physical activity buffers the negative relationship between multimorbidity, self-rated health and life satisfaction. J Public Health. (2018) 40:e328–35. doi: 10.1093/pubmed/fdy012
25. Makovski, TT, Schmitz, S, Zeegers, MP, Stranges, S, and van den Akker, M. Multimorbidity and quality of life: systematic literature review and meta-analysis. Ageing Res Rev. (2019) 53:100903. doi: 10.1016/j.arr.2019.04.005
26. Ge, L, Ong, R, Yap, CW, and Heng, BH. Effects of chronic diseases on health-related quality of life and self-rated health among three adult age groups. Nurs Health Sci. (2019) 21:214–22. doi: 10.1111/nhs.12585
27. Umeh, K. Self-rated health and multimorbidity in patients with type 2 diabetes. J Health Psychol. (2022) 27:1659–78. doi: 10.1177/13591053211001419
28. Pérez-Stable, EJ, and El-Toukhy, S. Communicating with diverse patients: how patient and clinician factors affect disparities. Patient Educ Couns. (2018) 101:2186–94. doi: 10.1016/j.pec.2018.08.021
29. Ishikawa, H, Hashimoto, H, and Kiuchi, T. The evolving concept of “patient-centeredness” in patient-physician communication research. Soc Sci Med. (2013) 96:147–53. doi: 10.1016/j.socscimed.2013.07.026
30. Chang, TJ, Bridges, JFP, Bynum, M, Jackson, JW, Joseph, JJ, Fischer, MA, et al. Association between patient-clinician relationships and adherence to antihypertensive medications among black adults: an observational study design. J Am Heart Assoc. (2021) 10:e019943. doi: 10.1161/JAHA.120.019943
31. Kamimura, A, Higham, R, Rathi, N, Panahi, S, Lee, E, and Ashby, J. Patient-provider relationships among vulnerable patients: the association with health literacy, continuity of care, and self-rated health. J Patient Exp. (2020) 7:1450–7. doi: 10.1177/2374373519895680
32. White, RO, Eden, S, Wallston, KA, Kripalani, S, Barto, S, Shintani, A, et al. Health communication, self-care, and treatment satisfaction among low-income diabetes patients in a public health setting. Patient Educ Couns. (2015) 98:144–9. doi: 10.1016/j.pec.2014.10.019
33. Aaron, DG, and Stanford, FC. Medicine, structural racism, and systems. Soc Sci Med. (2022) 298:114856. doi: 10.1016/j.socscimed.2022.114856
34. Homan, P, Brown, TH, and King, B. Structural intersectionality as a new direction for health disparities research. J Health Soc Behav. (2021) 62:350–70. doi: 10.1177/00221465211032947
35. Kaphingst, KA, Goodman, M, Pandya, C, Garg, P, Stafford, J, and Lachance, C. Factors affecting frequency of communication about family health history with family members and doctors in a medically underserved population. Patient Educ Couns. (2012) 88:291–7. doi: 10.1016/j.pec.2011.11.013
36. Twersky, SE, Jefferson, R, Garcia-Ortiz, L, Williams, E, and Pina, C. The impact of limited English proficiency on healthcare access and outcomes in the U.S.: a scoping review. Healthcare (Basel). (2024) 12:364. doi: 10.3390/healthcare12030364
37. Murugesu, L, Heijmans, M, Rademakers, J, and Fransen, MP. Challenges and solutions in communication with patients with low health literacy: perspectives of healthcare providers. PLoS One. (2022) 17:e0267782. doi: 10.1371/journal.pone.0267782
38. Mor-Anavy, S, Lev-Ari, S, and Levin-Zamir, D. Health literacy, primary care health care providers, and communication. Health Lit Res Pract. (2021) 5:e194–200. doi: 10.3928/24748307-20210529-01
39. Sim, D, Yuan, SE, and Yun, JH. Health literacy and physician-patient communication: a review of the literature. Int J Commun Health. (2016) 10:101–14.
40. Eton, DT, Ridgeway, JL, Linzer, M, Boehm, DH, Rogers, EA, Yost, KJ, et al. Healthcare provider relational quality is associated with better self-management and less treatment burden in people with multiple chronic conditions. Patient Prefer Adherence. (2017) 11:1635–46. doi: 10.2147/PPA.S145942
41. Schuttner, L, Hockett Sherlock, S, Simons, CE, Johnson, NL, Wirtz, E, Ralston, JD, et al. My goals are not their goals: barriers and facilitators to delivery of patient-centered Care for Patients with multimorbidity. J Gen Intern Med. (2022) 37:4189–96. doi: 10.1007/s11606-022-07533-1
42. Tsoh, JY, Sentell, T, Gildengorin, G, Le, GM, Chan, E, Fung, LC, et al. Healthcare communication barriers and self-rated health in older Chinese American immigrants. J Community Health. (2016) 41:741–52. doi: 10.1007/s10900-015-0148-4
43. Jung, M, Ramanadhan, S, and Viswanath, K. Effect of information seeking and avoidance behavior on self-rated health status among cancer survivors. Patient Educ Couns. (2013) 92:100–6. doi: 10.1016/j.pec.2013.02.008
44. Chao, MT, Handley, MA, Quan, J, Sarkar, U, Ratanawongsa, N, and Schillinger, D. Disclosure of complementary health approaches among low income and racially diverse safety net patients with diabetes. Patient Educ Couns. (2015) 98:1360–6. doi: 10.1016/j.pec.2015.06.011
45. Martinez, KA, Resnicow, K, Williams, GC, Silva, M, Abrahamse, P, Shumway, DA, et al. Does physician communication style impact patient report of decision quality for breast cancer treatment? Patient Educ Couns. (2016) 99:1947–54. doi: 10.1016/j.pec.2016.06.025
46. Shen, MJ, Hamann, HA, Thomas, AJ, and Ostroff, JS. Association between patient-provider communication and lung Cancer stigma. Support Care Cancer. (2016) 24:2093–9. doi: 10.1007/s00520-015-3014-0
47. Fukumori, Ryan, and Robbennolt, Simone. Who is low income and very low income in the Bay Area? (an updated look). San Francisco Bay Area: Bay Area Equity Atlas; (2023). Available online at: https://bayareaequityatlas.org/distribution-of-incomes (Accessed January 27, 2025).
48. Spitzer, RL, Kroenke, K, Williams, JBW, and Löwe, B. A brief measure for assessing generalized anxiety disorder: the GAD-7. Arch Intern Med. (2006) 166:1092–7. doi: 10.1001/archinte.166.10.1092
49. Kroenke, K, Spitzer, RL, and Williams, JBW. The PHQ-9. J Gen Intern Med. (2001) 16:606–13. doi: 10.1046/j.1525-1497.2001.016009606.x
50. Delgadillo, J, Dawson, A, Gilbody, S, and Böhnke, JR. Impact of long-term medical conditions on the outcomes of psychological therapy for depression and anxiety. Br J Psychiatry. (2017) 210:47–53. doi: 10.1192/bjp.bp.116.189027
51. Heatherton, TF, Kozlowski, LT, Frecker, RC, and Fagerstrom, KO. The Fagerstrom test for nicotine dependence: a revision of the Fagerstrom tolerance questionnaire. Addiction. (1991) 86:1119–27. doi: 10.1111/j.1360-0443.1991.tb01879.x
52. Atwoli, L, Platt, JM, Basu, A, Williams, DR, Stein, DJ, and Koenen, KC. Associations between lifetime potentially traumatic events and chronic physical conditions in the south African stress and health survey: a cross-sectional study. BMC Psychiatry. (2016) 16:214. doi: 10.1186/s12888-016-0929-z
53. Kim, G, Worley, CB, Allen, RS, Vinson, L, Crowther, MR, Parmelee, P, et al. Vulnerability of older Latino and Asian immigrants with limited English proficiency. J Am Geriatr Soc. (2011) 59:1246–52. doi: 10.1111/j.1532-5415.2011.03483.x
54. Lorig, K, Stewart, A, Ritter, P, González, V, Laurent>, D, and Lynch, J. Outcome measures for health education and other health care interventions. Thousand Oaks, CA, US: Sage Publications, Inc. (1996).
55. Faul, F, Erdfelder, E, Buchner, A, and Lang, AG. Statistical power analyses using G*power 3.1: tests for correlation and regression analyses. Behav Res Methods. (2009) 41:1149–60. doi: 10.3758/BRM.41.4.1149
56. Hayes, AF. Introduction to mediation, moderation, and conditional process analysis: A regression-based approach. New York, NY: Guilford Publications (2017).
57. Igartua, JJ, and Hayes, AF. Mediation, moderation, and conditional process analysis: concepts, computations, and some common confusions. Span J Psychol. (2021) 24:e49. doi: 10.1017/SJP.2021.46
58. Portet, S. A primer on model selection using the Akaike information criterion. Infect Dis Model. (2020) 5:111–28. doi: 10.1016/j.idm.2019.12.010
59. Harris, CR, Millman, KJ, van der Walt, SJ, Gommers, R, Virtanen, P, Cournapeau, D, et al. Array programming with NumPy. Nature. (2020) 585:357–62. doi: 10.1038/s41586-020-2649-2
60. Virtanen, P, Gommers, R, Oliphant, TE, Haberland, M, Reddy, T, Cournapeau, D, et al. SciPy 1.0: fundamental algorithms for scientific computing in Python. Nat Methods. (2020) 17:261–72. doi: 10.1038/s41592-019-0686-2
61. Kelley, JM, Kraft-Todd, G, Schapira, L, Kossowsky, J, and Riess, H. The influence of the patient-clinician relationship on healthcare outcomes: a systematic review and Meta-analysis of randomized controlled trials. PLoS One. (2014) 9:e94207. doi: 10.1371/journal.pone.0094207
62. Tavakoly Sany, SB, Behzhad, F, Ferns, G, and Peyman, N. Communication skills training for physicians improves health literacy and medical outcomes among patients with hypertension: a randomized controlled trial. BMC Health Serv Res. (2020) 20:60. doi: 10.1186/s12913-020-4901-8
63. Slatore, CG, Cecere, LM, Reinke, LF, Ganzini, L, Udris, EM, Moss, BR, et al. Patient-clinician communication. Chest. (2010) 138:628–34. doi: 10.1378/chest.09-2328
64. Iroegbu, C, Tuot, DS, Lewis, L, and Matura, LA. The influence of patient–provider communication on self-management among patients with chronic illness: a systematic mixed studies review. J Adv Nurs. (2025) 81:1678–99. doi: 10.1111/jan.16492
65. Willems, S, De Maesschalck, S, Deveugele, M, Derese, A, and De Maeseneer, J. Socio-economic status of the patient and doctor-patient communication: does it make a difference? Patient Educ Couns. (2005) 56:139–46. doi: 10.1016/j.pec.2004.02.011
66. Präg, P, Wittek, R, and Mills, MC. The educational gradient in self-rated health in Europe: does the doctor–patient relationship make a difference? Acta Sociol. (2017) 60:325–41. doi: 10.1177/0001699316670715
67. Santana, MJ, Manalili, K, Jolley, RJ, Zelinsky, S, Quan, H, and Lu, M. How to practice person-centred care: a conceptual framework. Health Expect. (2018) 21:429–40. doi: 10.1111/hex.12640
68. Beach, MC, Rosner, M, Cooper, LA, Duggan, PS, and Shatzer, J. Can patient-centered attitudes reduce racial and ethnic disparities in care? Acad Med. (2007) 82:193–8. doi: 10.1097/ACM.0b013e31802d94b2
69. Rohrer, JE, Wilshusen, L, Adamson, SC, and Merry, S. Patient-centredness, self-rated health, and patient empowerment: should providers spend more time communicating with their patients? J Eval Clin Pract. (2008) 14:548–51. doi: 10.1111/j.1365-2753.2007.00914.x
70. D’Agostino, TA, Atkinson, TM, Latella, LE, Rogers, M, Morrissey, D, DeRosa, AP, et al. Promoting patient participation in healthcare interactions through communication skills training: a systematic review. Patient Educ Couns. (2017) 100:1247–57. doi: 10.1016/j.pec.2017.02.016
71. Robinson, JD, Hoover, DR, Venetis, MK, Kearney, TJ, and Street, RL. Consultations between patients with breast cancer and surgeons: a pathway from patient-centered communication to reduced hopelessness. J Clin Oncol. (2013) 31:351–8. doi: 10.1200/JCO.2012.44.2699
72. Steinwachs, DM, Roter, DL, Skinner, EA, Lehman, AF, Fahey, M, Cullen, B, et al. A web-based program to empower patients who have schizophrenia to discuss quality of care with mental health providers. Psychiatr Serv. (2011) 62:1296–302. doi: 10.1176/ps.62.11.pss6211_1296
73. Venetis, MK, Robinson, JD, and Kearney, T. Breast-cancer patients’ participation behavior and coping during presurgical consultations: a pilot study. Health Commun. (2015) 30:19–25. doi: 10.1080/10410236.2014.943633
74. Schaffler, J, Leung, K, Tremblay, S, Merdsoy, L, Belzile, E, Lambrou, A, et al. The effectiveness of self-management interventions for individuals with low health literacy and/or low income: a descriptive systematic review. J Gen Intern Med. (2018) 33:510–23. doi: 10.1007/s11606-017-4265-x
75. Farley, H. Promoting self-efficacy in patients with chronic disease beyond traditional education: a literature review. Nurs Open. (2020) 7:30–41. doi: 10.1002/nop2.382
76. Miller, WR, and Rollnick, S. Motivational interviewing: helping people change and grow [internet]. Guilford Publications; (2023). Available online at: https://books.google.com/books?hl=en&lr=&id=QPLMEAAAQBAJ&oi=fnd&pg=PP1&dq=motivational+interviewing+helping+people+change+and+grow&ots=0Y0jYDTQ-H&sig=_fe4ldob0Byv294glZj_VYne1uA (Accessed March 7, 2024).
Keywords: patient-clinician communication, tobacco use, low-income adults, multimorbidity, self-rated health, culturally informed care, patient-centered care, health disparities
Citation: Cano MT, Lindstrom MR and Muñoz RF (2025) The dialogue dilemma: the role of patient-clinician communication for low-income people who smoke and manage multiple conditions. Front. Med. 12:1567725. doi: 10.3389/fmed.2025.1567725
Edited by:
Kelly Smith, University of Toronto, CanadaReviewed by:
Jannie Hugo, University of Pretoria, South AfricaKishan Kariippanon, The University of Sydney, Australia
Monalisa Chandra, University of Texas MD Anderson Cancer Center, United States
Copyright © 2025 Cano, Lindstrom and Muñoz. This is an open-access article distributed under the terms of the Creative Commons Attribution License (CC BY). The use, distribution or reproduction in other forums is permitted, provided the original author(s) and the copyright owner(s) are credited and that the original publication in this journal is cited, in accordance with accepted academic practice. No use, distribution or reproduction is permitted which does not comply with these terms.
*Correspondence: Monique T. Cano, bW9uaXF1ZS5jYW5vQHlhbGUuZWR1