- 1Department of Biology and Evolution of Marine Organisms, Stazione Zoologica Anton Dohrn, Napoli, Italy
- 2Department of Biology, University of Padova, Padova, Italy
- 3CIR-Myo Myology Center, University of Padova, Padova, Italy
Octopus vulgaris is a cephalopod mollusk and an active marine predator that has been at the center of a number of studies focused on the understanding of neural and biological plasticity. Studies on the machinery involved in e.g., learning and memory, regeneration, and neuromodulation are required to shed light on the conserved and/or unique mechanisms that these animals have evolved. Analysis of gene expression is one of the most essential means to expand our understanding of biological machinery, and the selection of an appropriate set of reference genes is the prerequisite for the quantitative real-time polymerase chain reaction (qRT-PCR). Here we selected 77 candidate reference genes (RGs) from a pool of stable and relatively high-expressed transcripts identified from the full-length transcriptome of O. vulgaris, and we evaluated their expression stabilities in different tissues through geNorm, NormFinder, Bestkeeper, Delta-CT method, and RefFinder. Although various algorithms provided different assemblages of the most stable reference genes for the different kinds of tissues tested here, a comprehensive ranking revealed RGs specific to the nervous system (Ov-RNF7 and Ov-RIOK2) and Ov-EIF2A and Ov-CUL1 across all considered tissues. Furthermore, we validated RGs by assessing the expression profiles of nine target genes (Ov-Naa15, Ov-Ltv1, Ov-CG9286, Ov-EIF3M, Ov-NOB1, Ov-CSDE1, Ov-Abi2, Ov-Homer2, and Ov-Snx20) in different areas of the octopus nervous system (gastric ganglion, as control). Our study allowed us to identify the most extensive set of stable reference genes currently available for the nervous system and appendages of adult O. vulgaris.
Introduction
Cephalopods and particularly the common octopus, Octopus vulgaris, are among the key invertebrate organisms recognized for their complex neural organization. The common octopus is an iconic species among cephalopods, at the center of a long tradition of research in diverse aspects of its biology and physiology (e.g., O’Brien et al., 2019).
The taxon belongs to Lophotrochozoa (i.e., a protostome animal), and thus it is very distant from vertebrates. Nevertheless, octopuses are known for possessing the largest nervous system among invertebrates in terms of the number of cells and body-to-brain size (Young, 1963; Packard and Albergoni, 1970; Giuditta et al., 1971; Packard, 1972), as well as their intricate neural network and manifold cellular complexity (Young, 1932; Shigeno and Ragsdale, 2015; Chung et al., 2022; Styfhals et al., 2022; Chung et al., 2023), with remarkable functional analogies to vertebrates (Shigeno et al., 2015, 2018). Octopus vulgaris has also served as an organism of study for the identification of the neural correlates of learning and memory and the search for a model of the brain (Young, 1964; Kandel, 1979; review in: Hochner et al., 2006; Borrelli and Fiorito, 2008; Marini et al., 2017). Nowadays, these mollusks continue to inspire the search for the biological and neural machinery underlying plasticity and cognition (Edelman and Seth, 2009; Albertin and Simakov, 2020; Ponte et al., 2022).
Over the last decade, a significant increase in the efforts of the scientific community has facilitated the release of a large set of genomic data (see Supplementary Info) for various cephalopod species, including the transcriptomes of O. vulgaris (Zhang et al., 2012; Petrosino, 2015; Petrosino et al., 2015; Liscovitch-Brauer et al., 2017; Petrosino et al., 2022; Prado-Álvarez et al., 2022; Styfhals et al., 2022) and reference genomes for more than 10 species (Supplementary Table 1). Although these resources still do not comprehensively represent the rich biological diversity of the approximately 800 living cephalopod species, their availability has greatly contributed to illuminating the biological and physiological complexity of these organisms and the ‘innovations’ they provided during their evolution (Albertin and Simakov, 2020; Albertin et al., 2022; Macchi et al., 2022; Schmidbaur et al., 2022).
These datasets, however, are not sufficient for the understanding of the molecular machinery implicated in neural plasticity (sensu: Cavallaro et al., 2002; Martinez et al., 2007; Asok et al., 2019). In addition, the current knowledge of the gene expression changes occurring in these animals during learning, memory, and behavioral plasticity is still poor. Only a few available studies are focused on some candidate molecules that are potentially involved in given functions. Thus, to the best of our knowledge, an investigation of the differential gene expression occurring in the brain of any cephalopod is still lacking. Here we contribute with a first step to fulfil this gap.
The accurate analysis of gene expression relies on the quantitative real-time polymerase chain reaction (qRT-PCR), one of the most utilized tools for assessing gene levels in different samples in experimental or biological conditions (Bustin, 2002; Huggett et al., 2005; Nolan et al., 2006). The technique offers numerous advantages (Bustin, 2002; Bustin et al., 2005). Nevertheless, its reliability and accuracy are based on the choice of reference genes (RGs) required for normalizing the expression levels of a given target gene. An ideal RG should have a moderate and stable expression level in different tissues, across biological phenomena, and under different experimental treatments (Huggett et al., 2005; Udvardi et al., 2008).
Most of the commonly used RGs for data normalization are the so-called housekeeping genes (e.g., elongation factor 1α, α-tubulin and β-tubulin, β-actin, and ubiquitin). Although widely employed in several species, in some instances they might lack the required stability when tested in different organisms and/or experimental contexts. In some circumstances, they may not match the requirements of an ideal candidate RG (Rubie et al., 2005; Hong et al., 2008; Eisenberg and Levanon, 2013).
In cephalopods, previous studies identified several candidate RGs (Supplementary Table 2) in a number of tissues (mainly brain masses), but to the best of our knowledge, they never encompassed testing of the peripheral ganglia or arms.
Our approach to build a list of potential stable reference genes in octopus was based on: (i) increasing the number of tissues to consider and (ii) exploring the available transcriptomes for O. vulgaris (Zhang et al., 2012; Petrosino, 2015; Petrosino et al., 2015, 2022). We selected genes that appeared stable and uniform in different tissues through in silico characterization of transcriptomes. Finally, we explored relative gene expression through qRT-PCR experiments by using a subset of target genes of the known expression in silico, thus validating our data and the use of the selected RGs in the brain and other ganglia. This approach allowed us to identify the most extensive set of stable reference genes currently available for adult O. vulgaris in the central and peripheral nervous system and in complex structures such as arms.
Materials and methods
In silico selection of candidate reference genes
Potential RGs were identified through in silico analysis of the RNA-seq available for O. vulgaris (for details, including assembly methods, see: Petrosino, 2015; Petrosino et al., 2015, 2022). The data included whole transcriptomes from nine tissues: the lobes of the adult octopus’ central nervous system (optic lobes, OL; supra-, SEM, and sub-oesophageal masses, SUB); the first anterior right arm (R1) with its distal extremity (Tip_R1), a proximal portion (ARM_R1), and muscle tissue (MUSC_R1; i.e., only muscle bundles, not the skin and arm nerve cord); the fourth posterior right arm (R4) with its proximal portion (ARM_R4); and two peripheral ganglia i.e. the left stellate and gastric ganglia (StG and GG, respectively; Figure 1).
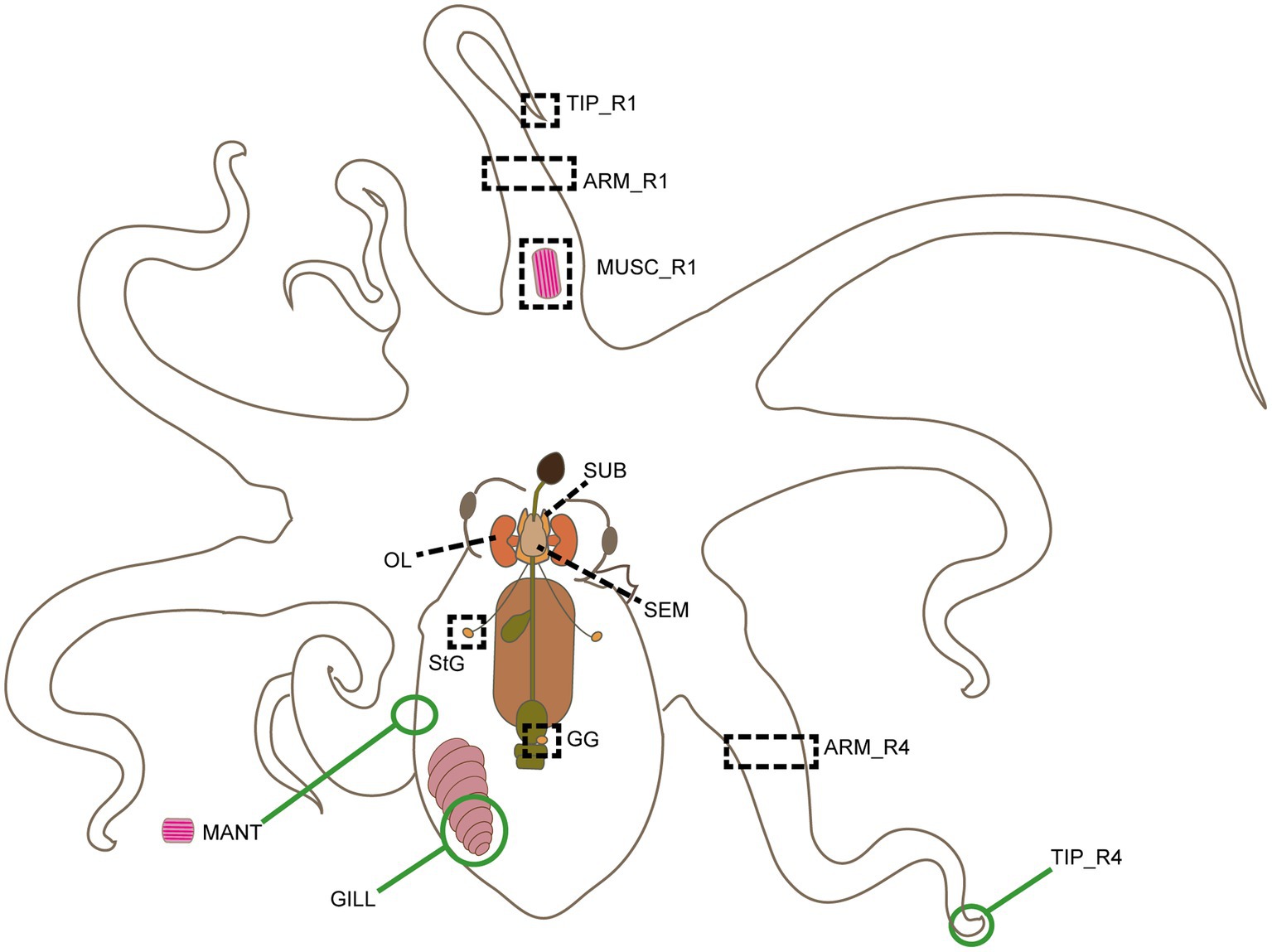
Figure 1. Schematic outline of Octopus vulgaris anatomy. Tissues sampled for transcriptomic analysis and biological validation are highlighted here. Black dotted rectangles and lines identify tissues included both in RNA-seq analysis and RT-qPCR experiments: supraoesophageal mass (SEM), suboesophageal mass (SUB) and left optic lobe (OL), gastric ganglion (GG), stellate ganglion (StG), R1 arm tip (Tip_R1), a piece of R1 and R4 arms (ARM_R1 and ARM_R4), and a piece of muscle from arm R1 (MUSC_R1). Green circles identify tissues only included in RT-qPCR experiments, i.e., a posterior portion of the left gill (GILL), a piece of muscles from the ventral side of the mantle without the skin (MANT), and R4 arm tip (Tip_R4).
As aforementioned, our rationale was to extend the biological diversity of the considered tissues. In addition, the anterior versus posterior arms were included on the basis of the scientific evidence of the potential variety of behavioral functions these may achieve (e.g., Mather, 1998; Huffard et al., 2005; Amodio et al., 2021).
Details on RNA isolation, quality and quantity assessment, and libraries construction are available in Petrosino (2015) and Petrosino et al. (2022) and not provided herein. Raw reads were analyzed by Trimmomatic (Bolger et al., 2014), which served for the filtering and trimming of low-quality bases. Normalization was performed, and the remaining reads were assembled in putative clustered transcripts to select unique sequences using Trinity (Grabherr et al., 2011). The raw reads were then mapped to the assembled transcriptome to measure the expression levels. Only annotated transcripts with a relative abundance greater than 1.5 counts per million (TPM) in all the biological replicates were considered. The annotation of these transcripts was finalized using the Annocript pipeline (Musacchia et al., 2015; Petrosino, 2015), thus counting 21,030 protein-coding sequences.
In order to identify the candidate RGs, we selected sequences based on their coefficient of variation (CV) of the relative abundance (TPM) of each transcript, i.e., the ratio of the standard deviation to the group mean of each transcript identified for four groups of tissues, as follows: (i) all the available tissues from adult individuals of O. vulgaris (Adult); (ii) the brain masses (Brain: SEM, SUB, and OL); (iii) The nervous tissues (Nervous: including tissues already listed in Brain group, plus StG and GG); (iv) tissues belonging to the arm (Arm: Tip_R1, ARM_R1, ARM R4, and MUSCLE_R1).
Genes were considered stable when their transcript’s CV was lower than 15%. For the Adult group, a cut-off of 20% CV was used to account for the higher tissue variability. Some genes were included in more than one group according to their CV values (Table 1 and Supplementary Figure 1).
Eight RGs from previous studies on cephalopods (Sirakov et al., 2009; García-Fernández et al., 2016; Baldascino et al., 2017; Imperadore, 2017; Xu and Zheng, 2018; Whang et al., 2020; see also Table 1 and Supplementary Table 2) were also included for subsequent validation analyses.
Sample collection and processing for RT-qPCR
To test the selected candidate RGs, tissues were harvested from five adult specimens of O. vulgaris (Supplementary Table 3) that did not show any signs of lesions, aberrant formations, or regenerating parts. From each octopus, 12 tissues were collected: SEM; SUB; OL; GG; StG; a portion of muscle from the ventral side of the mantle (MANT); arm tips from the anterior (Tip_R1) and posterior arms (Tip_R4); ARM_R1 and ARM_R4; MUSC_R1; and the left gill (GILL), considered here as a reference tissue for an internal organ differing from the muscles and nervous structures (Figure 1). The tissues were processed for RNA extraction; RNA integrity was tested using Agilent Bioanalyzer 2100 (see Supplementary Figure 2) and cDNA synthesis; cDNA samples were stored at −20°C until use (see Supplementary Info for specimens handling, sample harvesting, tissue processing, and RNA and cDNA processing and synthesis).
Primer design and amplification efficiency analysis for qRT-PCR
Primer3 Plus software (Untergasser et al., 2012) was used to design specific primers (Supplementary Table 4) to amplify the candidate genes. The following parameters were utilized: optimal melting temperature at 60°C, amplicon size 100–200 bp, and primer size between 18 and 27 bp (optimum set at 20 bp). Template RNA sequences were retrieved from previously mentioned RNA-seq studies. To obtain the most efficient primer couples, hairpin, homodimer, and heterodimer structures were evaluated for each primer couple using the Multiple Primer Analyzer1 (modified after Breslauer et al., 1986). In addition, 12 primer couples from eight genes were selected from the literature and slightly modified, when needed, to match with O. vulgaris sequences, or they were designed ex novo based on published ones (Supplementary Table 4).
RT-qPCR was performed on four-fold cDNA dilutions (from 10 ng/μL to 0.15625 ng/μL; see Supplementary Info) to calculate the primers’ efficiency, using the formula where m is the slope of the linear interpolation of dots representing Ct in the function of log10 [cDNA concentration].
To estimate the gene expression in each tissue, the primers were tested for RT-qPCR on individual samples in technical triplicates by using 2 μl cDNA [1.25 ng/μl] (see Supplementary Info for details).
Expression stability
The 12 tissues included in this study are highly diverse in structure, function, and gene expression profile. Thus, to account for this variability (with highest variability showed by the arm tips), we considered three groups for the expression stability analyses: Nervous (SEM, SUB, OL, GG, and StG); all tissues excluding the arm tips (Allex: Nervous, plus GILL, MANT, ARM_R1, ARM_R4, and MUSC_R1); and Adult (all the tissues including the arm tips; see also above and Figure 1).
The expression stability of each candidate gene across all samples within each tissue group was investigated using the mean Ct values and four different algorithms: geNorm (Vandesompele et al., 2002), NormFinder (Mestdagh et al., 2009), BestKeeper (Pfaffl et al., 2004), and the Delta-CT method (2−ΔΔCT) (Livak and Schmittgen, 2001). geNorm estimates the average pairwise variation in a specific gene with all the other potential reference genes. NormFinder computes the stability value for each gene according to their minimum variance. Both the geNorm and NormFinder values are lower for more stable genes (Amable et al., 2013). BestKeeper relies on the concept that the more stable the gene expression, the lower the Ct variation if the cDNA quantity is constant (Amable et al., 2013). Finally, the Delta Ct algorithm (Livak and Schmittgen, 2001) takes into account the expression of each gene in all samples and its standard deviation (SD); the gene with the lowest SD is considered the most appropriate reference gene (Silver et al., 2006).
The results from these approaches were integrated in RefFinder (Xie et al., 2012) to obtain an overall rank of expression stability for each of the three tissue groups. The method ranks each gene in each group and calculates the geometric mean of ranks for each gene. More stable genes show smaller geometric means, as they are ranked higher by all the methods.
Validation of reference genes
To validate the reliability of the data normalization, the combination of the two most stable candidate RGs, and of the most stable and unstable reference genes for the Nervous group were used to analyze the expression levels of the target genes. When two RGs were utilized for normalization, we relied on their geometric mean. The relative quantification of nine target genes was calculated for the Nervous group following Pfaffl's (2001) method, which takes into account the primer efficiencies of both targets and RGs.
Data analysis
Following Zar (1999), statistical significance was assessed after an ANOVA test, followed by Bonferroni multiple comparison tests. For all analyses, we used SPSS (rel. 18.0, SPSS Inc. - Chicago, 2009), with the exceptions mentioned above. All tests were two-tailed, and the alpha was set at 0.05.
Results
In silico identification of candidate reference genes
Candidate RGs were identified from the transcriptome of O. vulgaris (Petrosino, 2015; Petrosino et al., 2022). Genes with a relatively stable expression in silico in four tissue groups (Adult, Brain, Nervous, and Arm) were selected according to the relative abundance of each transcript (TPM counts) and their coefficient of variation (CV). Using a CV cut-off of 20%, 32 transcripts (out a total of 64,477 unique transcripts) were selected for Adult. The CV cut-off was decreased to 15% to identify the most stable transcripts in the Brain (1,540 transcripts), Nervous system (357), and Arm (125). A total of 2,145 transcripts was identified. Because the annotation results were not further curated in the original studies (Musacchia et al., 2015; Petrosino, 2015; Petrosino et al., 2015, 2022), we excluded non-annotated transcripts, thus identifying 88 potential RGs for the four tissue groups (Table 1). Seven of them resulted shared among more than one group (highlighted in Table 1).
We observed the highest variability in CVs among samples belonging to the Adult group (19 genes; mean CV = 13.6%; CV range: 8.8–16.2%; Table 1). In this set, 12 genes showed CV values lower than 15% (i.e., Ov-UBE2F, Ov-PRMT5, Ov-LTV1, Ov-CPIJ005834, Ov-EIF2A, Ov-rpf1, Ov-slc25a40, Ov-RIOK2, Ov-Dap3, Ov-Ppm1b, Ov-ATPAF2, and Ov-NOB1; Table 1). Lower CV values were observed when the Brain group was considered (20 genes; mean CV = 3.28%; CV range: 0.4–6.0%; Table 1), with seven genes exhibiting CV values below 3% (i.e., Ov-mts, Ov-AP5Z1, Ov-USP15, Ov-Gnaq, Ov-Fam160a2, Ov-MTX1, and Ov-WBP2; Table 1). Nineteen candidate RGs were identified for the Nervous group (mean CV = 6.5%; CV range: 3.9–9.9%; Table 1), with average CVs higher than those observed for the Brain group. In this case, 12 genes had CV values lower than 7% (i.e., Ov-Gsk3b, Ov-mts, Ov-timm, Ov-SUCLG2, Ov-CHCHD7, Ov-UBE2F, Ov-MTX1, Ov-gk5, Ov-Gnaq, Ov-Naa15, Ov-wdr44, and Ov-Klhdc). For the Arm group (19 genes; mean CV = 6.7%; CV range: 3.4–11.8%), we observed similar CVs to the Nervous group, with 9 genes (10 transcripts) having CVs lower than 7% (i.e., Ov-ESR16, Ov-C2CD2, Ov-nAChRalpha1, Ov-14-3-3zeta, Ov-Sdhd, Ov-Vbp1, Ov-PCK1, Ov-MRM2, and Ov-RNF7; Table 1).
A total of 69 candidate RGs were selected for biological validation. In addition, we considered eight RGs used in previous studies (Sirakov et al., 2009; Imperadore, 2017; Xu and Zheng, 2018), raising the final number of genes to be tested through qRT-PCR experiments to 77 (Table 1).
Candidate reference genes and their expression profiles
Eighty-one primer couples for the selected putative RGs were designed and tested for specificity and efficiency through standard PCR and qRT-PCR reactions (Supplementary Info), using the total mRNA extracted from 12 tissues (Figure 1) belonging to five O. vulgaris specimens. Three primer couples (i.e., Ov-wls, Ov-ESR16, and Ov-C2CD2 isoform X2) exhibited no or multiple amplification products when tested for standard PCR and were excluded from subsequent analyses. All other primer couples resulted in a single amplification product at the expected amplicon size (Supplementary Figure 3) and were therefore tested for RT-qPCR. The primer sequences, amplicon size, product Tm, and amplification efficiencies are shown in Supplementary Table 4.
A total of 59 primer pairs showed amplification efficiencies between 98 and 102%, while 19 did not fall within this range and were excluded from further analyses (Supplementary Table 4).
The expression levels of the final list of candidate RGs (n = 59) were estimated in each tissue sample (technical triplicates) through qRT-PCR. The reference genes displayed a wide range of transcription levels, with average Ct values ranging from 18.17 to 37.27 (Supplementary Table 5). Ov-Tuba1a showed the lowest mean Ct (21.63), i.e., the highest abundance in tissues. High expression levels were also noted for Ov-Rpl6, Ov-tollip, and Ov-RPS18 (mean Ct = 24.15, 24.40, and 24.79, respectively). In an opposite trend, Ov-NOB1 and Ov-TUBG1-FR presented a relatively low expression level (mean Ct = 31.31 in both cases; Supplementary Table 5).
Analysis of expression stability of the candidate reference genes
For the expression stability analyses, three tissue groups of increasing biological variability were considered (Nervous, ALLex, and Adult).
Our results suggested that the most suitable reference genes differed among the approaches used for the identification of RGs (see Methods), as well as among the groups considered, likely owing to their substantial tissue diversity (Table 2).
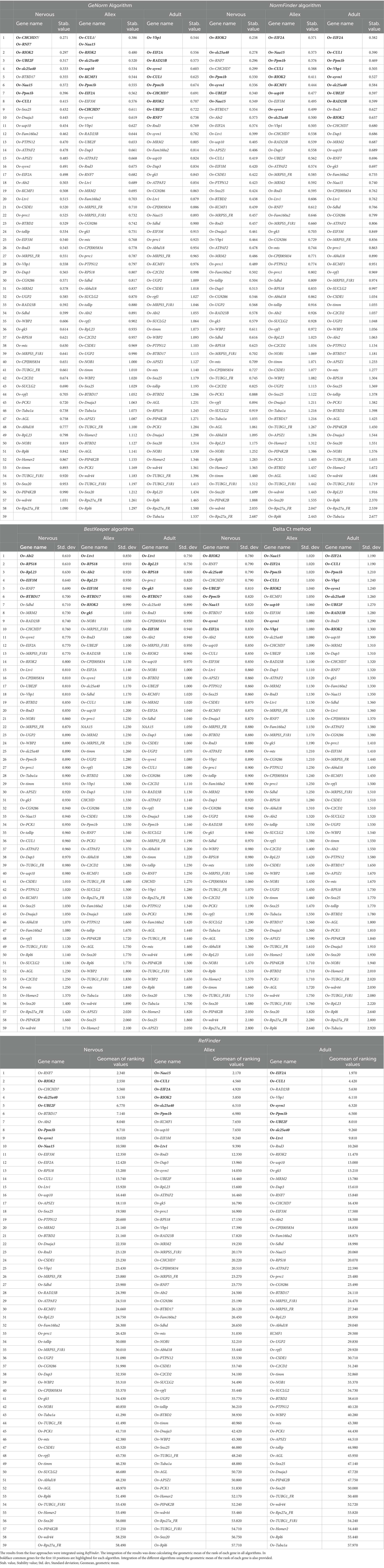
Table 2. Outcomes of the analysis for reference genes stability after application of the four algorithms (i.e., GeNorm, NormFinder, BestKeeper, and DeltaCt).
geNorm analysis. Ov-CHCHD7 and Ov-RNF7 were identified as the two most correlated genes and therefore scored as the most stable RGs for the Nervous tissues (Table 2), followed by Ov-RIOK2, Ov-UBE2F, Ov-slc25a40, Ov-BTBD17, Ov-Naa15, Ov-Ppm1b, Ov-CUL1, Ov-Snx25, and Ov-Dnaja3 (Table 2). Interestingly, the genes Ov-RpL23, Ov-Rpl6, Ov-TuBg1-F1R1, and Ov-Rps27a-FR, recently utilized as RGs in RT-qPCR experiments in cephalopods (Supplementary Table 2), were demonstrated to be among the most unstable genes (Table 2).
When additional tissues were considered (Allex), Ov-CUL1 and Ov-Naa15 were identified as the two most correlated genes, followed by Ov-RIOK2, Ov-slc25a40, Ov-usp10, Ov-KCMF1, Ov-Ppm1b, Ov-EIF2A, Ov-EIF3M, Ov-CHCHD7, and Ov-syvn1. The least stable genes included Ov-Rpl6, Ov-Rps27a-FR, Ov-TUBG1-F1R1, and Ov-TUBG1-FR (Table 2). In the analysis of all the tissues (Adult), Ov-Vbp1, Ov-EIF2A, Ov-RAD23B, Ov-syvn1, Ov-CUL1, Ov-Ppm1b, Ov-CHCHD7, Ov-RIOK2, Ov-UBE2F, and Ov-RNF7 emerged as the most stable genes. Similarly to Nervous and Allex, Ov-TUBG1-F1R1, Ov-TUBG1-FR, Ov-RpL23, Ov-Rpl6, Ov-Rps27a-FR, and Ov-Tuba1a were the least stable genes. Among the 10 most stable genes, only Ov-Ppm1b, Ov-CHCHD7, and Ov-CUL1 were shared by the three groups, while Ov-RIOK2, Ov-slc25a40, Ov-Naa15, Ov-RNF7, Ov-UBE2F, and Ov-EIF2A were shared between two groups (Table 2).
NormFinder analysis. We identified Ov-RIOK2, Ov-slc25a40, Ov-RNF7, Ov-CHCHD7, Ov-Ppm1b, Ov-syvn1, Ov-UBE2F, Ov-Naa15, Ov-BTBD17, and Ov-Abi2 as the most stable genes (Nervous, Table 2). For the Allex and Adult groups, some of the top genes in Nervous ranked at lower values (e.g., Ov-RIOK2 and Ov-slc25a40), while others were considered more stable (e.g., Ov-EIF2A and Ov-CUL1; Table 2). Ov-Naa15, Ov-EIF2A, Ov-CUL1, and Ov-UBE2F were identified as stable reference genes in both groups, while Ov-RIOK2, Ov-slc25a40, Ov-Ppm1b, and Ov-syvn1 were shared in all the considered tissue groups (Table 2).
BestKeeper analysis. Ov-Abi2, Ov-Ltv1, and Ov-gk5 were shown to be the most stable genes, which were shared between two groups, while Ov-RPS18, Ov-RpL23, Ov-EIF3M, and Ov-BTBD17 were shared between the three groups (see Table 2). Ov-RPS18 and Ov-RpL23 were identified as suitable reference genes by this algorithm.
Delta Ct method. Ov-RIOK2, Ov-RNF7, Ov-slc25a40, Ov-CHCHD7, Ov-UBE2F, Ov-Ppm1b, Ov-Naa15, Ov-BTBD17, Ov-syvn1, and Ov-EIF2A emerged as the most stable genes for the Nervous tissues (Table 2). However, several other genes showed a comparable standard deviation (Table 2). Ov-Naa15, Ov-EIF2A, Ov-Ppm1b, Ov-CUL1, Ov-RIOK2, Ov-KCMF1, Ov-usp10, Ov-EIF3M, Ov-syvn1, and Ov-Vbp1 were selected as references for the Allex group. When all tissues were considered (Adult), Ov-EIF2A, Ov-CUL1, Ov-Ppm1b, Ov-Vbp1, Ov-syvn1, Ov-slc25a40, Ov-UBE2F, Ov-RAD23B, Ov-Rnd3, and Ov-RIOK2 were identified as the most stable reference genes. Ov-slc25a40, Ov-Naa15, Ov-Vbp1, Ov-CUL1, and Ov-UBE2F were shared between two groups, while Ov-Ppm1b, Ov-syvn1, Ov-RIOK,2 and Ov-EIF2A were shared between the three groups (Table 2).
Comprehensive ranking of the reference genes
By comparing the 10 most stable genes identified by the four approaches in the same tissue group (Nervous), Ov-RIOK2, Ov-UBE2F, Ov-slc25a40, Ov-Naa15, and Ov-Ppm1b were identified as common in at least three algorithms, while Ov-CHCHD7, Ov-RNF7, and Ov-BTBD17 resulted from all the four methods (Table 2). When arms but not tips were included as tissues (Allex group), Ov-CUL1, Ov-Naa15, Ov-RIOK2, Ov-usp10, Ov-KCMF1, Ov-EIF2A, and Ov-Ppm1b (Table 2) emerged as the best reference genes using the three approaches, while Ov-EIF3M was shared among the four methods (Table 2). The analysis performed considering the Adult tissues led to the identification of Ov-RAD23B, Ov-EIF2A, Ov-Vbp1, Ov-syvn1, Ov-CUL1, Ov-Ppm1b, and Ov-UBE2F as reference genes by the three approaches, but none of them were shared in all the considered methods.
The results from the four approaches were integrated using RefFinder (Xie et al., 2012). Overall, the top 10 most stable genes in the Nervous tissues were Ov-RNF7, Ov-RIOK2, Ov-CHCHD7, Ov-slc25a40, Ov-UBE2F, Ov-BTBD17, Ov-Abi2, Ov-Ppm1b, Ov-syvn1, and Ov-Naa15 (Table 2). For Allex, the most stable genes were Ov-Naa15, Ov-CUL1, Ov-EIF2A, Ov-RIOK2, Ov-slc25a40, Ov-Ppm1b, Ov-KCMF1, Ov-usp10, Ov-EIF3M, and Ov-Ltv1 (Table 2). When all tissues were considered (Adults), Ov-EIF2A, Ov-CUL1, Ov-RAD23B, Ov-Vbp1, Ov-syvn1, Ov-Ppm1b, Ov-UBE2F, Ov-slc25a40, Ov-Ltv1, and Ov-Rnd3 were identified as reference genes, with Ov-EIF2A proving to be the best reference gene (see the geometric mean of the rank, Table 2). We also plotted the raw Ct for the best RGs identified (Supplementary Figure 4). The most stable genes shared by the combination of tissues were Ov-Naa15 and Ov-RIOK2 (Nervous and Allex); Ov-CUL1, Ov-EIF2A, and Ov-Ltv1 (Allex and Adult); and Ov-syvn1 and Ov-UBE2F (Nervous and Adult). Meanwhile, the Ov-slc25a40 and Ov-Ppm1b results were shared among the three groups (Table 2).
Reference genes validation
To investigate the reliability of the selected candidate RGs, the expression profiles of nine target genes (i.e., Ov-Naa15, Ov-Ltv1, Ov-CG9286, Ov-EIF3M, Ov-NOB1, Ov-CSDE1, Ov-Abi2, Ov-Homer2, and Ov-Snx20) were assessed in tissues belonging to the nervous system. The role of these genes (see Supplementary Info: Selected target genes for validation) is still unknown in cephalopods. The selection was based on their known functions in different organisms, particularly those related to their involvement in neuronal signaling, cytoskeleton functions, axon guidance, synaptogenesis, and behavioral plasticity.
This also allowed comparison of gene expression profiles among brain masses and peripheral ganglia (Nervous). The gastric ganglion (GG) was considered as the reference ‘tissue’. A combination of the two most stable (Ov-RNF7 and Ov-RIOK2), the most stable (Ov-RNF7), and the least stable (most unstable; Ov-Rps27a-FR) RGs was used to normalize the expression of the target genes.
When the best RGs combination and the most stable gene were used for normalization of the expression of target genes in the nervous system of O. vulgaris, similar expression profiles were obtained for Ov-CSDE1 and Ov-Homer2 in the SEM, SUB, and OL, but the StG showed a significantly lower expression (Figure 2). A similar trend was also highlighted for Ov-Snx20 (Figure 2) that showed a lower expression in the StG compared to the SEM and SUB. No significant differences resulted for the other target genes considered (Figure 2).
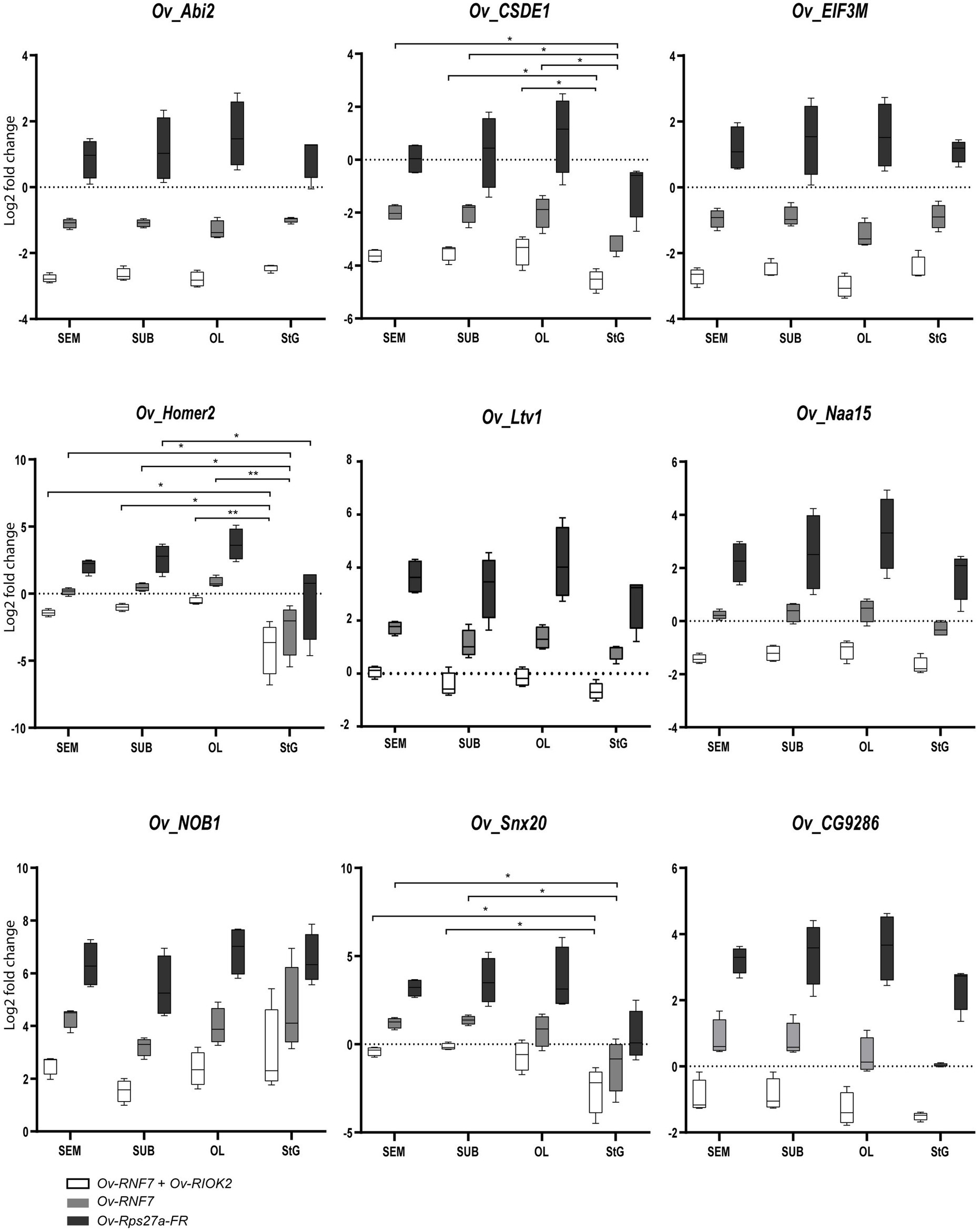
Figure 2. Relative expression levels of target genes: Ov-Naa15, Ov-Ltv1, Ov-CG9286, Ov-EIF3M, Ov-NOB1, Ov-CSDE1, Ov-Abi2, Ov-Homer2, and Ov-Snx20 across all considered tissues (optic Lobe: OL; supraesophageal mass: SEM; suboesophageal mass: SUB; stellate ganglion: StG) normalized by the most stable reference gene combination (white bars), the most stable gene (grey), and the most unstable gene (black). Significant differences were assessed after ANOVA (p < 0.05, Bonferroni post hoc). See main text for details.
When the least stable reference gene Ov-Rps27a-FR was used for normalization, none of the nine genes investigated showed any significant change in expression except for Ov-Homer2, which appeared to be less expressed in the StG compared to the OL (Figure 2).
Discussion
Exploring gene expression in the nervous system and other tissues helps to find molecular correlates of biological and neural plasticity, learning, and memory. In cephalopods, the study of the molecular machinery occurring in these processes is still limited. Few studies of cuttlefish (Agin et al., 2000, 2001, 2003; Focareta et al., 2014; Focareta and Cole, 2016; Bian et al., 2018), squid (Giuditta et al., 2002; Kimbell and McFall-Ngai, 2003; Burbach et al., 2019), and octopus (Zarrella, 2011; Zarrella et al., 2015; van Giesen et al., 2020; see also Prado-Álvarez et al., 2022) have been based on an exiguous number of specific candidate molecules involved in given functions or biological aspects of cephalopod plasticity.
Despite the availability of a few candidate RGs (review in Supplementary Table 2), the application of qRT-PCR in O. vulgaris also appears limited. Our approach was to expand the list of potential stable RGs in octopus through the use of the available transcriptomes (Petrosino, 2015; Petrosino et al., 2015, 2022), with the aim of facilitating a large-scale analysis of gene expression profiles under various conditions in different tissues.
We focused on genes that demonstrated stability and a uniform predicted expression within different tissues (peripheral and central nervous system and appendages). We explored their relative gene expression through qRT-PCR experiments using a subset of target genes. This approach allowed us to identify the most extensive set of stable reference genes currently available for the adult O. vulgaris.
Through in silico analysis of the octopus transcriptome, we found more than 2000 candidate RGs. However, we tested less than hundreds because of limitations in gene annotation. We identified a list of stable and uniformly expressed RGs across different body parts in adult individuals and in tissues including the nervous tissues (e.g., brain, gastric and stellate ganglia, and arm; Figure 1). The gene expression profiles of these potential RGs (n = 59) were assessed via qRT-PCR, and their stability was calculated and analyzed using different algorithms. The analysis of potential RGs in O. vulgaris revealed that there was no single reference gene that exhibited a constant expression level in all the samples, similarly to what has been reported in other organisms (e.g., Guo et al., 2014; Gao et al., 2017; Jin et al., 2019).
Via RefFinder, we identified RGs specific to the nervous system (Nervous, Ov-RNF7, and Ov-RIOK2), all tissues but the arm tips (Allex, Ov-Naa15, and Ov-CUL1), or those that are transcriptionally stable across all considered tissues (Adult, Ov-EIF2A, and Ov-CUL1, Table 2). In addition, Ov-slc25a40 and Ov-Ppm1b were identified as shared best reference genes in the Nervous, Allex, and Adult groups of tissues (Table 2). Notably, the arm tips showed the highest variation in gene expression among the analyzed anatomical structures, likely due to the biological peculiarities of the octopus’ arm that maintains the ability of regeneration and indeterminate growth throughout adult ontogeny (Fossati et al., 2013, 2015; Nödl et al., 2015; Zullo et al., 2017; Tarazona et al., 2019; e.g., Zullo et al., 2019; van Giesen et al., 2020; see also De Sio and Imperadore, 2023).
The identified RGs are related to ubiquitination, rRNA processing, translation, and post-translational protein modifications, which are housekeeping functions in line with the typical references. Interestingly, none of them has ever been used as references in cephalopods before. Our approach—i.e., a large number of candidate transcripts and several tissues belonging to putatively different cell types (Styfhals et al., 2022)—provided more than 70 candidate RGs for O. vulgaris.
We also validated RGs by assessing the expression profiles of nine target genes (Ov-Naa15, Ov-Ltv1, Ov-CG9286, Ov-EIF3M, Ov-NOB1, Ov-CSDE1, Ov-Abi2, Ov-Homer2, and Ov-Snx20) in different tissues of the octopus nervous system. The expression after normalization by Ov-RNF7 and Ov-RIOK2 (the most stable RGs) differed from that of Ov-Rps27a-FR (the least stable gene), which is commonly used as an RG for data normalization (Figure 2).
In conclusion, we utilized different algorithms to evaluate the expression profiles of tens of candidate RGs of O. vulgaris. We identified those that can be used in the normalization of the qRT-PCR data and suggested RGs that can be used cautiously with different tissue groups.
Our findings will aid future investigations of the transcriptional landscape of cephalopods and facilitate the study of the molecular basis of neural plasticity and other phenomena.
Data availability statement
The original contributions presented in the study are included in the article/Supplementary material, further inquiries can be directed to the corresponding author.
Ethics statement
Ethical review and approval was not required for the animal study because killing animals solely for tissue removal does not require authorization from the National Competent Authority under Directive 2010/63/EU (European Parliament & Council of the European Union, 2010) and its transposition into National legislation. Sampling of octopuses from artisanal fishermen included in this study was authorized by the local Animal Welfare Body (Ethical Clearance: case 5/2021/ec AWB-SZN).
Author contributions
PI and SC performed the experiments, data curation, and analysis and contributed to the study conceptualization. VA and CM contributed to the experiments and data curation. PI, SC, and GF contributed to writing the original draft. GF and GP contributed to the study conceptualization, investigation, writing, and funding acquisition. All authors contributed to the article and approved the submitted version.
Funding
This work was supported by the Stazione Zoologica Anton Dohrn intramural research fund granted to GF and GP and by a HSA-Ceph 1/2019 grant to GP. VA was supported by a short-term fellowship from the Stazione Zoologica Anton Dohrn.
Acknowledgments
We thank Elena Baldascino, Giuseppe Petrosino, and Remo Sanges for their advice and support.
Conflict of interest
The authors declare that the research was conducted in the absence of any commercial or financial relationships that could be identified as a potential conflict of interest.
Publisher’s note
All claims expressed in this article are solely those of the authors and do not necessarily represent those of their affiliated organizations, or those of the publisher, the editors and the reviewers. Any product that may be evaluated in this article, or claim that may be made by its manufacturer, is not guaranteed or endorsed by the publisher.
Supplementary material
The Supplementary material for this article can be found online at: https://www.frontiersin.org/articles/10.3389/fnmol.2023.1091305/full#supplementary-material
Footnotes
1. ^ https://www.thermofisher.com/it/en/home/brands/thermo-scientific/molecular-biology/molecular-biology-learning-center/molecular-biology-resource-library/thermo-scientific-web-tools/multiple-primer-analyzer.html
References
Agin, V., Chichery, R., and Chichery, M. P. (2000). Effects of learning and memory on regional brain variations of cytochrome oxidase activity in Sepia officinalis. Eur. J. Neurosci. 12:91.
Agin, V., Chichery, R., and Chichery, M. P. (2001). Effects of learning on cytochrome oxidase activity in cuttlefish brain. Neuroreport 12, 113–116. doi: 10.1097/00001756-200101220-00030
Agin, V., Chichery, R., Maubert, E., and Chichery, M. P. (2003). Time-dependent effects of cycloheximide on long-term memory in the cuttlefish. Pharmacol. Biochem. Behav. 75, 141–146. doi: 10.1016/S0091-3057(03)00041-8
Albertin, C. B., Medina-Ruiz, S., Mitros, T., Schmidbaur, H., Sanchez, G., Wang, Z. Y., et al. (2022). Genome and transcriptome mechanisms driving cephalopod evolution. Nat. Commun. 13:2427. doi: 10.1038/s41467-022-29748-w
Albertin, C. B., and Simakov, O. (2020). Cephalopod biology: at the intersection between genomic and organismal novelties. Ann. Rev. Anim. Biosci. 8, 71–90. doi: 10.1146/annurev-animal-021419-083609
Amable, P. R., Teixeira, M. V. T., Carias, R. B. V., Granjeiro, J. M., and Borojevic, R. (2013). Identification of appropriate reference genes for human mesenchymal cells during expansion and differentiation. PLoS One 8:e73792. doi: 10.1371/journal.pone.0073792
Amodio, P., Josef, N., Shashar, N., and Fiorito, G. (2021). Bipedal locomotion in Octopus vulgaris: a complementary observation and some preliminary considerations. Ecol. Evol. 11, 3679–3684. doi: 10.1002/ece3.7328
Asok, A., Kandel, E. R., and Rayman, J. B. (2019). The neurobiology of fear generalization. Front. Behav. Neurosci. 12:329. doi: 10.3389/fnbeh.2018.00329
Baldascino, E., Di Cristina, G., Tedesco, P., Hobbs, C., Shaw, T. J., Ponte, G., et al. (2017). The gastric ganglion of Octopus vulgaris: preliminary characterization of gene- and Putative neurochemical-complexity, and the effect of Aggregata octopiana digestive tract infection on gene expression. Front. Physiol. 8:1001. doi: 10.3389/fphys.2017.01001
Bian, L., Liu, C., Chen, S., Zhao, F., Ge, J., and Tan, J. (2018). Transcriptome analysis of gene expression patterns during embryonic development in golden cuttlefish (Sepia esculenta). Genes Genom. 40, 253–263. doi: 10.1007/s13258-017-0588-6
Bolger, A. M., Lohse, M., and Usadel, B. (2014). Trimmomatic: a flexible trimmer for Illumina sequence data. Bioinformatics 30, 2114–2120. doi: 10.1093/bioinformatics/btu170
Borrelli, L., and Fiorito, G. (2008). “Behavioral analysis of learning and memory in cephalopods” in Learning and memory: a comprehensive reference. ed. J. J. Byrne (Oxford: Academic Press), 605–627.
Breslauer, K. J., Frank, R., Blöcker, H., and Marky, L. A. (1986). Predicting DNA duplex stability from the base sequence. Proc. Natl. Acad. Sci. U. S. A. 83, 3746–3750. doi: 10.1073/pnas.83.11.3746
Burbach, J. P. H., Grant, P., Senft, S., Kripke, L., Hellemons, A. J. C. G. M., and Pant, H. C. (2019). FMRF gene expression in the nervous system of the squid Doryteuthis pealei hatchling. bioRxiv, 684001 [Preprint]. doi: 10.1101/684001
Bustin, S. (2002). Quantification of mRNA using real-time reverse transcription PCR (RT-PCR): trends and problems. J. Mol. Endocrinol. 29, 23–39. doi: 10.1677/jme.0.0290023
Bustin, S. A., Benes, V., Nolan, T., and Pfaffl, M. W. (2005). Quantitative real-time RT-PCR – a perspective. J. Mol. Endocrinol. 34, 597–601. doi: 10.1677/jme.1.01755
Cavallaro, S., D'Agata, V., Manickam, P., Dufour, F., and Alkon, D. L. (2002). Memory-specific temporal profiles of gene expression in the hippocampus. Proc. Natl. Acad. Sci. 99, 16279–16284. doi: 10.1073/pnas.242597199
Chung, W.-S., Kurniawan, N. D., and Marshall, N. J. (2022). Comparative brain structure and visual processing in octopus from different habitats. Curr. Biol. 32, 97–110.e114. doi: 10.1016/j.cub.2021.10.070
Chung, W.-S., López-Galán, A., Kurniawan, N. D., and Marshall, N. J. (2023). The brain structure and the neural network features of the diurnal cuttlefish Sepia plangon. iScience 26:105846. doi: 10.1016/j.isci.2022.105846
De Sio, F., and Imperadore, P. (2023). Deciphering regeneration through non-model animals: a century of experiments on cephalopod mollusks and an outlook at the future. Front. Cell Dev. Biol. 10:1072382. doi: 10.3389/fcell.2022.1072382
Edelman, D. B., and Seth, A. K. (2009). Animal consciousness: a synthetic approach. Trends Neurosci. 32, 476–484. doi: 10.1016/j.tins.2009.05.008
Eisenberg, E., and Levanon, E. Y. (2013). Human housekeeping genes, revisited. Trends Genet. 29, 569–574. doi: 10.1016/j.tig.2013.05.010
European Parliament & Council of the European Union (2010). Directive 2010/63/EU of the European Parliament and of the Council of 22 September 2010 on the protection of animals used for scientific purposes [Online]. Strasbourg: Concil of Europe. Available at: https://eur-lex.europa.eu/legal-content/EN/ALL/?uri=CELEX:32010L0063 (Accessed March, 2023).
Focareta, L., and Cole, A. G. (2016). Analyses of sox-B and sox-E family genes in the cephalopod Sepia officinalis: revealing the conserved and the unusual. PLoS One 11:e0157821. doi: 10.1371/journal.pone.0157821
Focareta, L., Sesso, S., and Cole, A. G. (2014). Characterization of homeobox genes reveals sophisticated regionalization of the central nervous system in the European cuttlefish Sepia officinalis. PLoS One 9:e109627. doi: 10.1371/journal.pone.0109627
Fossati, S. M., Candiani, S., Nodl, M. T., Maragliano, L., Pennuto, M., Domingues, P., et al. (2015). Identification and expression of acetylcholinesterase in Octopus vulgaris arm development and regeneration: a conserved role for ACHE? Mol. Neurobiol. 52, 45–56. doi: 10.1007/s12035-014-8842-2
Fossati, S. M., Carella, F., De Vico, G., Benfenati, F., and Zullo, L. (2013). Octopus arm regeneration: role of acetylcholinesterase during morphological modification. J. Exp. Mar. Biol. Ecol. 447, 93–99. doi: 10.1016/j.jembe.2013.02.015
Gao, X.-K., Zhang, S., Luo, J.-Y., Wang, C.-Y., Lü, L.-M., Zhang, L.-J., et al. (2017). Identification and validation of reference genes for gene expression analysis in Aphidius gifuensis (hymenoptera: Aphidiidae). PLoS One 12:e0188477. doi: 10.1371/journal.pone.0188477
García-Fernández, P., Castellanos-Martínez, S., Iglesias, J., Otero, J. J., and Gestal, C. (2016). Selection of reliable reference genes for RT-qPCR studies in Octopus vulgaris paralarvae during development and immune-stimulation. J. Invertebr. Pathol. 138, 57–62. doi: 10.1016/j.jip.2016.06.003
Giuditta, A., Eyman, M., and Kaplan, B. B. (2002). Gene expression in the squid giant axon: neurotransmitter modulation of RNA transfer from periaxonal glia to the axon. Biol. Bull. 203, 189–190. doi: 10.2307/1543389
Giuditta, A., Libonati, M., Packard, A., and Prozzo, N. (1971). Nuclear counts in the brain lobes of Octopus vulgaris as a function of body size. Brain Res. 25, 55–62. doi: 10.1016/0006-8993(71)90566-X
Grabherr, M. G., Haas, B. J., Yassour, M., Levin, J. Z., Thompson, D. A., Amit, I., et al. (2011). Full-length transcriptome assembly from RNA-Seq data without a reference genome. Nat. Biotechnol. 29, 644–652. doi: 10.1038/nbt.1883
Guo, J., Ling, H., Wu, Q., Xu, L., and Que, Y. (2014). The choice of reference genes for assessing gene expression in sugarcane under salinity and drought stresses. Sci. Rep. 4:7042. doi: 10.1038/srep07042
Hochner, B., Shomrat, T., and Fiorito, G. (2006). The octopus: a model for a comparative analysis of the evolution of learning and memory mechanisms. Biol. Bull. 210, 308–317. doi: 10.2307/4134567
Hong, S.-Y., Seo, P. J., Yang, M.-S., Xiang, F., and Park, C.-M. (2008). Exploring valid reference genes for gene expression studies in Brachypodium distachyon by real-time PCR. BMC Plant Biol. 8:112. doi: 10.1186/1471-2229-8-112
Huffard, C. L., Boneka, F., and Full, R. J. (2005). Underwater bipedal locomotion by octopuses in disguise. Science 307:1927. doi: 10.1126/science.1109616
Huggett, J., Dheda, K., Bustin, S., and Zumla, A. (2005). Real-time RT-PCR normalisation; strategies and considerations. Genes Immun. 6, 279–284. doi: 10.1038/sj.gene.6364190
Imperadore, P. (2017). Nerve regeneration in the cephalopod mollusc Octopus vulgaris: A journey into morphological, cellular and molecular changes including epigenetic modifications. PhD Thesis. Rende, Italy: Università della Calabria.
Jin, Y., Liu, F., Huang, W., Sun, Q., and Huang, X. (2019). Identification of reliable reference genes for qRT-PCR in the ephemeral plant Arabidopsis pumila based on full-length transcriptome data. Sci. Rep. 9:8408. doi: 10.1038/s41598-019-44849-1
Kandel, E.R. (1979). Behavioral biology of Aplysia. A contribution to the comparative study of opisthobranch molluscs. San Francisco, CA: W.H. Freeman and Company.
Kimbell, J. R., and McFall-Ngai, M. J. (2003). The squid-vibrio symbioses: from demes to Genes. Integr. Comp. Biol. 43, 254–260. doi: 10.1093/icb/43.2.254
Liscovitch-Brauer, N., Alon, S., Porath, H. T., Elstein, B., Unger, R., Ziv, T., et al. (2017). Trade-off between transcriptome plasticity and genome evolution in cephalopods. Cells 169, 191–202.e11. doi: 10.1016/j.cell.2017.03.025
Livak, K. J., and Schmittgen, T. D. (2001). Analysis of relative gene expression data using real-time quantitative PCR and the 2−ΔΔCT method. Methods 25, 402–408. doi: 10.1006/meth.2001.1262
Macchi, F., Edsinger, E., and Sadler, K. C. (2022). Epigenetic machinery is functionally conserved in cephalopods. BMC Biol. 20:202. doi: 10.1186/s12915-022-01404-1
Marini, G., De Sio, F., Ponte, G., and Fiorito, G. (2017). “Behavioral analysis of learning and memory in cephalopods” in Learning and memory: A comprehensive reference. ed. J. H. Byrne. 2nd ed (Amsterdam, The Netherlands: Academic Press, Elsevier), 441–462.
Martinez, J. L., Thompson, K. J., and Sikorski, A. M. (2007). “Chapter 4 - gene expression in learning and memory” in Neurobiology of learning and memory. eds. R. P. Kesner and J. L. Martinez. 2nd ed (Burlington, MA: Academic Press), 129–153.
Mather, J. A. (1998). How do octopuses use their arms? J. Comp. Psychol. 112, 306–316. doi: 10.1037/0735-7036.112.3.306
Mestdagh, P., Van Vlierberghe, P., De Weer, A., Muth, D., Westermann, F., Speleman, F., et al. (2009). A novel and universal method for microRNA RT-qPCR data normalization. Genome Biol. 10:R64. doi: 10.1186/gb-2009-10-6-r64
Musacchia, F., Basu, S., Petrosino, G., Salvemini, M., and Sanges, R. (2015). Annocript: a flexible pipeline for the annotation of transcriptomes able to identify putative long noncoding RNAs. Bioinformatics 31, 2199–2201. doi: 10.1093/bioinformatics/btv106
Nödl, M.-T., Fossati, S. M., Domingues, P., Sánchez, F. J., and Zullo, L. (2015). The making of an octopus arm. EvoDevo 6:19. doi: 10.1186/s13227-015-0012-8
Nolan, T., Hands, R. E., and Bustin, S. A. (2006). Quantification of mRNA using real-time RT-PCR. Nat. Protoc. 1, 1559–1582. doi: 10.1038/nprot.2006.236
O’Brien, C. E., Ponte, G., and Fiorito, G. (2019). “Octopus☆” in Encyclopedia of animal behavior. ed. J. C. Choe. Second ed (Oxford: Academic Press), 142–148. doi: 10.1016/B978-0-12-809633-8.90074-8
Packard, A. (1972). Cephalopods and fish: the limits of convergence. Biol. Rev. 47, 241–307. doi: 10.1111/j.1469-185X.1972.tb00975.x
Packard, A., and Albergoni, V. (1970). Relative growth, nucleic acid content and cell numbers of the brain in Octopus vulgaris (Lamarck). J. Exp. Biol. 52, 539–552. doi: 10.1242/jeb.52.3.539
Petrosino, G. (2015). The transcriptional landscape of the nervous system of Octopus vulgaris. PhD Thesis. Naples, Italy: Università degli Studi di Napoli Federico II.
Petrosino, G., Ponte, G., Volpe, M., Zarrella, I., Ansaloni, F., Langella, C., et al. (2022). Identification of LINE retrotransposons and long non-coding RNAs expressed in the octopus brain. BMC Biol. 20:116. doi: 10.1186/s12915-022-01303-5
Petrosino, G., Zarrella, I., Ponte, G., Basu, S., Calogero, R., Fiorito, G., et al. (2015). “De-novo assembly of the octopus transcriptome integrating custom and public sequencing data” in BIOINFO - BITS. Available at: https://bioinformatics.hsanmartino.it/bits_library/library/01652.pdf
Pfaffl's, M. W. (2001). A new mathematical model for relative quantification in real-time RT–PCR. Nucleic Acids Res. 29:e45. doi: 10.1093/nar/29.9.e45
Pfaffl, M. W., Tichopad, A., Prgomet, C., and Neuvians, T. P. (2004). Determination of stable housekeeping genes, differentially regulated target genes and sample integrity: BestKeeper – excel-based tool using pair-wise correlations. Biotechnol. Lett. 26, 509–515. doi: 10.1023/B:BILE.0000019559.84305.47
Ponte, G., Chiandetti, C., Edelman, D. B., Imperadore, P., Pieroni, E. M., and Fiorito, G. (2022). Cephalopod behavior: from neural plasticity to consciousness. Front. Syst. Neurosci. 15:787139. doi: 10.3389/fnsys.2021.787139
Prado-Álvarez, M., Dios, S., García-Fernández, P., Tur, R., Hachero-Cruzado, I., Domingues, P., et al. (2022). De novo transcriptome reconstruction in aquacultured early life stages of the cephalopod Octopus vulgaris. Sci. Data 9:609. doi: 10.1038/s41597-022-01735-2
Rubie, C., Kempf, K., Hans, J., Su, T., Tilton, B., Georg, T., et al. (2005). Housekeeping gene variability in normal and cancerous colorectal, pancreatic, esophageal, gastric and hepatic tissues. Mol. Cell. Probes 19, 101–109. doi: 10.1016/j.mcp.2004.10.001
Schmidbaur, H., Kawaguchi, A., Clarence, T., Fu, X., Hoang, O. P., Zimmermann, B., et al. (2022). Emergence of novel cephalopod gene regulation and expression through large-scale genome reorganization. Nat. Commun. 13:2172. doi: 10.1038/s41467-022-29694-7
Shigeno, S., Andrews, P. L. R., Ponte, G., and Fiorito, G. (2018). Cephalopod brains: an overview of current knowledge to facilitate comparison with vertebrates. Front. Physiol. 9:952. doi: 10.3389/fphys.2018.00952
Shigeno, S., Parnaik, R., Albertin, C. B., and Ragsdale, C. W. (2015). Evidence for a cordal, not ganglionic, pattern of cephalopod brain neurogenesis. Zool. Lett. 1:26. doi: 10.1186/s40851-015-0026-z
Shigeno, S., and Ragsdale, C. W. (2015). The gyri of the octopus vertical lobe have distinct neurochemical identities. J. Comp. Neurol. 523, 1297–1317. doi: 10.1002/cne.23755
Silver, N., Best, S., Jiang, J., and Thein, S. L. (2006). Selection of housekeeping genes for gene expression studies in human reticulocytes using real-time PCR. BMC Mol. Biol. 7:33. doi: 10.1186/1471-2199-7-33
Sirakov, M., Zarrella, I., Borra, M., Rizzo, F., Biffali, E., Arnone, M. I., et al. (2009). Selection and validation of a set of reliable reference genes for quantitative RT-PCR studies in the brain of the cephalopod mollusc Octopus vulgaris. BMC Mol. Biol. 10:70. doi: 10.1186/1471-2199-10-70
Styfhals, R., Zolotarov, G., Hulselmans, G., Spanier, K. I., Poovathingal, S., Elagoz, A. M., et al. (2022). Cell type diversity in a developing octopus brain. Nat. Commun. 13:7392. doi: 10.1038/s41467-022-35198-1
Tarazona, O. A., Lopez, D. H., Slota, L. A., and Cohn, M. J. (2019). Evolution of limb development in cephalopod mollusks. eLife 8:e43828. doi: 10.7554/eLife.43828
Udvardi, M. K., Czechowski, T., and Scheible, W.-R.d. (2008). Eleven Golden rules of quantitative RT-PCR. Plant Cell 20, 1736–1737. doi: 10.1105/tpc.108.061143
Untergasser, A., Cutcutache, I., Koressaar, T., Ye, J., Faircloth, B. C., Remm, M., et al. (2012). Primer3—new capabilities and interfaces. Nucleic Acids Res. 40:e115. doi: 10.1093/nar/gks596
van Giesen, L., Kilian, P. B., Allard, C. A. H., and Bellono, N. W. (2020). Molecular basis of chemotactile sensation in octopus. Cells 183, 594–604.e514. doi: 10.1016/j.cell.2020.09.008
Vandesompele, J., De Preter, K., Pattyn, F., Poppe, B., Van Roy, N., De Paepe, A., et al. (2002). Accurate normalization of real-time quantitative RT-PCR data by geometric averaging of multiple internal control genes. Genome Biol. 3 research0034.0031, 1–12. doi: 10.1186/gb-2002-3-7-research0034
Whang, I., Kang, H.-S., and Kim, Y. (2020). Validation of reference genes for quantitative gene expression studies in Octopus minor. Ocean Sci. J. 55, 183–191. doi: 10.1007/s12601-020-0007-9
Xie, F., Xiao, P., Chen, D., Xu, L., and Zhang, B. (2012). miRDeepFinder: a miRNA analysis tool for deep sequencing of plant small RNAs. Plant Mol. Biol. 80, 75–84. doi: 10.1007/s11103-012-9885-2
Xu, R., and Zheng, X. (2018). Selection of reference genes for quantitative real-time PCR in Octopus minor (Cephalopoda: Octopoda) under acute ammonia stress. Environ. Toxicol. Pharmacol. 60, 76–81. doi: 10.1016/j.etap.2018.04.010
Young, J. Z. (1932). Memoirs: on the cytology of the neurons of cephalopods. J. Cell Sci. s2-75, 1–47. doi: 10.1242/jcs.s2-75.297.1
Young, J. Z. (1963). The number and sizes of nerve cells in octopus. Proc. Zool. Soc. Lond 140, 229–254. doi: 10.1111/j.1469-7998.1963.tb01862.x
Zarrella, I. (2011). Testing changes in gene expression profiles for Octopus vulgaris (Mollusca, Cephalopoda). PhD Thesis, Naples, Italy: Stazione Zoologica Anton Dohrn (Italy) and Open University (UK).
Zarrella, I., Ponte, G., Baldascino, E., and Fiorito, G. (2015). Learning and memory in Octopus vulgaris: a case of biological plasticity. Curr. Opin. Neurobiol. 35, 74–79. doi: 10.1016/j.conb.2015.06.012
Zhang, X., Mao, Y., Huang, Z., Qu, M., Chen, J., Ding, S., et al. (2012). Transcriptome analysis of the Octopus vulgaris central nervous system. PLoS One 7:e40320. doi: 10.1371/journal.pone.0040320
Zullo, L., Eichenstein, H., Maiole, F., and Hochner, B. (2019). Motor control pathways in the nervous system of Octopus vulgaris arm. J. Comp. Physiol. A. 205, 271–279. doi: 10.1007/s00359-019-01332-6
Keywords: Octopus vulgaris , reference genes, qRT-PCR, cephalopods, nervous system, molecular fingerprint
Citation: Imperadore P, Cagnin S, Allegretti V, Millino C, Raffini F, Fiorito G and Ponte G (2023) Transcriptome-wide selection and validation of a solid set of reference genes for gene expression studies in the cephalopod mollusk Octopus vulgaris. Front. Mol. Neurosci. 16:1091305. doi: 10.3389/fnmol.2023.1091305
Edited by:
Margaret S. Saha, College of William & Mary, United StatesReviewed by:
Camino Gestal, Spanish National Research Council (CSIC), SpainMasa-aki Yoshida, Shimane University, Japan
Copyright © 2023 Imperadore, Cagnin, Allegretti, Millino, Raffini, Fiorito and Ponte. This is an open-access article distributed under the terms of the Creative Commons Attribution License (CC BY). The use, distribution or reproduction in other forums is permitted, provided the original author(s) and the copyright owner(s) are credited and that the original publication in this journal is cited, in accordance with accepted academic practice. No use, distribution or reproduction is permitted which does not comply with these terms.
*Correspondence: Pamela Imperadore, aW1wZXJhZG9yZS5wQGdtYWlsLmNvbQ==; cGFtZWxhLmltcGVyYWRvcmVAc3puLml0;
†These authors have contributed equally to this work