- 1Institute of Pharmaceutical Sciences of Western Switzerland, University of Geneva, Centre Médical Universitaire, Geneva, Switzerland
- 2School of Pharmaceutical Sciences, University of Geneva, Centre Médical Universitaire, Geneva, Switzerland
- 3Department of Biochemistry, Faculty of Sciences, University of Geneva, Geneva, Switzerland
- 4Department of Biology, University of Fribourg, Fribourg, Switzerland
- 5Green Mission Department, Herbal Products Laboratory, Pierre Fabre Research Institute, Toulouse, France
Antibiotics resistance is a clear threat to the future of current tuberculosis treatments like rifampicin, prompting the need for new treatment options in this field. While plants can offer a plethora of chemical diversity in their constitutive natural products to tackle this issue, finding potentially bioactive compounds in them has not always proven to be that simple. Classical bioactivity-guided fractionation approaches are still trendy, but they bear significant shortfalls, like their time-consuming nature as well as the ever-increasing risk of isolating known bioactive compounds. In this regard, we have developed an alternative method to the latter approach that allows for natural derivatives of a known bioactive scaffold to be efficiently targeted and isolated within a large library of plant extracts. Hence our approach allows for the anticipation of bioactive structure independently of preliminary bioassays. By relying on the chemical diversity of a set of 1,600 plant extracts analyzed by HRMS/MS, we were able to isolate and characterize several minor derivatives of a previously reported bioactive aza-anthraquinone compound from Cananga brandisiana, selected within the plant set. Assessment of bioactivity on these derivatives (especially onychine, with an IC50 value of 39 µM in infection) confirmed their expected activity on Mycobacterium marinum in our anti-infective assay. This proof-of-concept study has established an original path towards bioactive compounds isolation, with the advantage of potentially highlighting minor bioactive compounds, whose activity may not even be detectable at the extract level.
1 Introduction
The reputation of natural products (NPs) as a rich source of drug lead compounds has been solid throughout the past few decades. Of all drugs approved between 1981 and 2010, it is estimated that around 50% were of natural origin (NPs, derivatives and analogues) (Newman and Cragg, 2007; Schmitt et al., 2011; Newman and Cragg, 2012; Newman and Cragg, 2020). Yet it is also estimated that more than 95% of the world’s biodiversity has not been evaluated for any biological activity, highlighting that current challenges remain in the access and valorization of this natural chemical diversity (David et al., 2015). Major disease areas like cancers or bacterial infections among others, could benefit from such an untapped source of unique bioactive compounds.
Tuberculosis (TB) is one such disease with unmet clinical needs, it is the 13th leading cause of death worldwide, claiming about 1.6 million lives in 2021. It is deemed a public health crisis by the WHO as of 2022, prompting the need for further research in the field to uncover new treatments (World Health Organisation, 2022). While drugs like rifampicin are a good example of NP (isolated from a bacteria, Amycolatopsis rifamycinica) that has reached the market for its antibiotic activity (FDA approval in 1971), Multidrug-resistant TB strains (MDR-TB) pose a clear threat to the future of this kind of TB treatments (McHugh, 2013). Yet many promising bioactive NPs scaffolds have been and continue to be discovered, with many of them also reported as active against resistant isolates of mycobacteria (Cazzaniga et al., 2021).
In this context, plants and their constitutive NPs represent a vast source of anti-mycobacterial compounds with variable mechanisms of action. Historically, new bioactive NPs were often identified through bioactivity-guided fractionations, known to be a particularly tedious process, with high chances of resulting in the isolation of already known compounds. This is one of the main reasons why NPs research started falling out of fashion with pharmaceutical programs after the 1970s (Han et al., 2022).
Recent developments in metabolomics opened new avenues for more effective searches of bioactive compounds from complex biological natural matrices. A broad range of computational tools have been developed to generate a wealth of putative structural information about constituents of those natural extracts through annotation based on mass spectrometric (MS) measurements recorded on instruments of ever-increasing capabilities (Wolfender et al., 2019). In parallel, other additional tools relevant to medicinal chemistry, like NP-likeness scores (Ertl et al., 2008) or chemical similarity search filters offered by software such as DataWarrior (DW) (DataWarrior User Manual, 2015; Sander et al., 2015) open the possibility to query the metabolome information from a new perspective. In this context, effective queries can only be performed if bioactive hits were previously characterized and if valuable bioactivity data on reference chemical libraries is available.
Analysis of bioassay results on standard compound libraries can therefore pave the way for the search of chemically related compounds in the metabolome data of natural extracts and enable their isolation and characterization as new hits of interest. In this context and in recent years, several phenotypic screens were performed in the context of infected host models to identify anti-mycobacterial compounds (Brodin et al., 2010; Kalsum et al., 2022; Theriault et al., 2022). Striving for a system that could recapitulate the intracellular infection course of Mycobacterium tuberculosis, the etiological agent of TB, we developed the Dictyostelium discoideum (D. discoideum) and Mycobacterium marinum (M. marinum) host-pathogen system (Tobin and Ramakrishnan, 2008; Habjan et al., 2021). D. discoideum is a social amoeba and a professional phagocyte with conserved innate immune response pathways, such as phagocytosis, ROS production and autophagy (Dunn et al., 2018). Working on Mycobacterium tuberculosis (Mtb) as a model in laboratories entails high operational costs and constitutes a clear obstacle to the development of high-throughput testing methods (World Health Organisation, 2012), as it presents high risks of contaminations for humans and therefore requires biosafety level 3 laboratories. M. marinum is a genetically close relative of Mtb (Sapriel and Brosch, 2019) and a promising alternative for the search of new anti-mycobacterial compounds (Tobin and Ramakrishnan, 2008; Habjan et al., 2021). We have established the D. discoideum-M. marinum model system as an alternative screening system to identify anti-infective activity of a set of anti-TB compounds from GlaxoSmithKline (GSK) (Hanna et al., 2020). Our data demonstrated that compounds with anti-infective activity were similarly active in the D. discoideum-M. marinum system and the more commonly used M. marinum-macrophage system. Using a fluorescent D. discoideum and a bioluminescent M. marinum allows to monitor both the impact of NP samples on the intracellular growth of M. marinum, as well as potential inhibitory effects on the host or cytotoxic activity. Consequently, we can easily evaluate the anti-infective properties and selective activity of NP samples in one combined assay. Additionally, samples can also be tested on M. marinum growing in broth to evaluate their antibiotic potential. Taken together, testing samples both “in infection” and “in broth” can allow us to confidently identify samples which are active on mycobacteria residing inside a host cell.
Herein, we leveraged our previously performed screen of drug-like compounds on the D. discoideum-M. marinum infection model system (Tobin and Ramakrishnan, 2008). A Highly Diverse Pathway-Based Library (HD-PBL) consisting of small ligand-based chemicals was established. It comprised 1,255 different compounds chosen from the ZINC lead-like database (Sterling and Irwin, 2015), with an incentive to maximize the chemical diversity of the library and the drug likeness of candidates chosen. This was achieved by querying the ZINC database for 18 host-pathogen interaction relevant biological pathways and collecting chemo diverse hit pharmacophores, determined by the LINGO method (Vidal et al., 2005). The derived set of compounds was screened for anti-infective activity in a combination of three different assays including the D. discoideum-M. marinum infection model. From this study, 37 compounds stood out as hit structures, with 29 specifically inhibiting the intracellular growth of M. marinum by more than 20% (Hanna et al., 2020).
In the present study, we took advantage of the screening of drug-related compounds on the above-mentioned D. discoideum-M. marinum infection model system by specifically searching for potential hits through their predicted structures in natural extracts (metabolomics). Our aim was to evaluate if metabolome information generated at large scale by MS-based metabolomics on a library of plant extracts could be effectively used to guide us through the identification of bioactive NP analogs of reference hit compounds. This proof-of-concept study, being based on known information, also aims to avoid resource-intensive isolation of already reported bioactive metabolites which can be obtained with the classical bioactivity-guided untargeted approach. Subsequently, the targeted and isolated natural products were characterized and compared to a reference hit, regarding their anti-bacterial and anti-infective activities on M. marinum in broth and in the D. discoideum-M. marinum infection system. To do this, we have taken advantage of the MS based metabolomics results that we have previously obtained on a biodiverse collection of over 1,600 plant extracts (Allard et al., 2023).
2 Materials and methods
2.1 General experimental procedures
HPLC analyses were conductedon an HP 1260 system equipped with a diode-array detection unit (Agilent Technologies, Santa Clara, CA, United States) using an InterChim® Puriflash HQ C18 column (250 × 4.6 mm i.d., 15 μm, Montluçon, France). UHPLC-HRMS/MS analysis was performed on a Waters Acquity UHPLC system interfaced to a Q-Exactive™ Focus Orbitrap (Thermo Scientific, Bremen, Germany) supplemented with heated electrospray Ionization source (HESI-II) using an Acquity BEH C18 column (50 × 2.1 mm i.d., 1.7 µm, Waters, Milford, MA, United States). Flash chromatography was performed on a Büchi Flash chromatography system (Büchi Pump Module C-605, UV Photometer C-640, Control Unit C-620, Fraction Collector C-660) using an InterChim® Puriflash HQ C18 column (120 g, 210 × 30 mm i.d., 15 μm, Montluçon, France). Semi-preparative HPLC-UV was conducted on a Shimadzu system equipped with an LC-20 A module pumps, an SPD-20 A UV/VIS, a 7725I Rheodyne® valve, and an FRC-40 fraction collector (Shimadzu, Kyoto, Japan) using an XBridge BEH C18 OBD Prep column (250 × 19 mm i.d., 5 μm, Waters®). NMR data were recorded on a Bruker Avance Neo 600 MHz NMR spectrometer equipped with a QCI 5 mm cryoprobe and a SampleJet automated sample changer (Bruker BioSpin, Rheinstetten, Germany). Technical grade hexane (Hex), ethyl acetate (EtOAc) and methanol (MeOH) were purchased from Thommen-Furler AG, Rüti b. Büren, Switzerland. HPLC grade methanol and ethyl acetate were purchased from Fisher Chemicals, Reinach, Switzerland. LC-MS grade water, acetonitrile (ACN), and formic acid were purchased from Fisher Chemicals, Reinach, Switzerland. Dimethyl Sulfoxide (DMSO) molecular biology grade was purchased from Sigma-Aldrich, St Louis, United States.
2.2 Plant material
The plant containing the compounds of interest was Cananga brandisiana (Pierre) Saff. (Annonaceae). This plant belongs to the Pierre Fabre Laboratories (PFL) collection with over 17,000 unique samples collected worldwide. The PFL collection was registered at the European Commission under the accession number 03-FR-2020. This registration certifies that the collection meets the criteria set out in the EU ABS Regulation which implements at EU level the requirements of the Nagoya Protocol regarding access to genetic resources and the fair and equitable sharing of benefits arising from their utilization (Sharing nature, 2022). PFL supplied all the vegetal material (ground dry material). The collected samples have photographs, herbarium vouchers, and leaf extracts preserved in dry silica gel. Precise localization of the initial collection, unique ID and barcode, and GPS data are stored in the dedicated data management system. The plant material was dried for 3 days at 55°C in an oven; then the material was ground and stored in plastic pots at controlled temperature and humidity in PFL facilities. Two plant parts of C. brandisiana were used in this study, with their following unique ID within the PFL collection: V114260 (roots) and V114261 (trunk bark).
2.3 Plant extraction
The extraction of the plant was carried out through maceration of the dried and ground plant material in hexane followed by ethyl acetate and methanol respectively (all technical grade). Maceration was carried out three times for each solvent, the mixture was filtered in-between each maceration and the filtrates were collected in round bottom flasks, combined and evaporated to dryness on a rotary evaporator (Büchi Rotavapor R114™ Labortechnik AG, Switzerland) for each solvent to constitute the final extracts.
The preparation of the extracts of C. brandisiana (Annonaceae) was performed on very limited amounts of dried plant material. For the extraction of roots of C. brandisiana (Annonaceae), 14.15 g of dried ground plant material were used, yielding after solvent evaporation on a rotary evaporator 85.8 mg of hexane extract, 72.8 mg of ethyl acetate extract and 778.6 mg of methanol extract. Equally, for the extraction of the trunk bark of C. brandisiana (Annonaceae), 48.97 g of dried ground plant material were used, yielding 232.9 mg of hexane extract, 90.2 mg of ethyl acetate extract and 3.5816 g of methanol extract.
2.4 Solid phase extraction prior to UHPLC analyses
Prior to UHPLC-HRMS/MS analysis, the latter extracts were subjected to Solid Phase Extraction (SPE) on C18 cartridges (1 g, Finisterre by Teknokroma), with the hexane extracts excluded because of the lipophilicity of their content. The cartridges were pre-conditioned with 3 mL of MeOH:H2O (1:1), followed by 3 mL MeOH. The sample was loaded on the cartridge as 1 mL of 10 mg.mL−1 extract in MeOH and the filtrate was collected, along with 1 mL of MeOH added to wash the cartridge. For the ethyl acetate extracts, masses of 8.4 mg (roots) and 7.8 mg (trunk bark) were recovered from the initial load and for the methanol extract, masses of 9.4 mg (roots) and 8.8 mg (trunk bark) were recovered. These masses were re-dissolved in methanol to reach a final concentration of 5 mg.mL−1. These samples were then subjected to UHPLC-HRMS/MS analysis as described hereafter.
2.5 UHPLC-PDA-HRMS/MS analysis
Untargeted data-dependent UHPLC-HRMS/MS analyses were performed on all freshly obtained extracts of C. brandisiana and for metabolite profiling of all fractions of interest in order to localize hit compounds. These conditions were similar to those used to analyze the metabolome of the entire extract collection (Allard et al., 2023).
UHPLC-HRMS/MS analysis was performed on a Waters Acquity UHPLC system interfaced to a Q-Exactive™ Focus Orbitrap (Thermo Scientific, Bremen, Germany) supplemented with heated electrospray Ionization source (HESI-II). The system was controlled by Xcalibur v. 4.5 (Thermo Scientific). The HESI-II parameters were as follows: source voltage, 3.5 kV (+); sheath gas flow rate (N2), 42 units; auxiliary gas flow rate, 10 units; spare gas flow rate, 2; capillary temperature, 270°C, S-Lens RF Level, 45. The PDA wavelength absorbance was set between 210 and 400 nm. The crude extract (conc. 5 mg/mL, vol. 2 μL) was injected into an Acquity BEH C18 column (50 × 2.1 mm i.d., 1.7 µm, Waters, Milford, MA, United States) for 9 min. The mobile phase was H2O (A) and MeCN (B), both containing 0.1% FA. Gradient mode was as follows: 5%–100% of B in 7 min followed by 1 min of 100% B, then 100%–5% in 0.10 min and 0.90 min at 5%. The column was heated at 40°C, the samples kept at 8°C and the flow rate was 0.6 mL/min.
2.6 HRMS data analysis and generation of a molecular networking
Proteowizard’s msConvert software (v.3.0) was used to convert raw MS files into mzXML formats (Kessner et al., 2008; Chambers et al., 2012). The converted files were treated by MZMine software v. 2.53. In positive mode, MS1 and MS2 for each scan were detected at threshold of 1E6 and 0, respectively. The ADAP module was used to build chromatograms by connecting data points from mass lists. The chromatograms were deconvoluted into individual peaks by application of the “wavelet” algorithm. In the presence of isotopic patterns, isotopes were grouped to the lowest m/z ion. The extracted ions were aligned in a table and represented as features that demonstrate m/z, RT and peak area. The parameters for each mentioned treatment were adjusted according to Rutz et al. (2022). MNs were built online through the GNPS platform (GNPS, 2016) and visualized by Cytoscape, 2001 (Cytoscape: an open-source platform for complex network analysis and visualization, 2001). The GNPS parameters were adjusted according to Houriet et al. (2020).
For the annotation of the features generated, each of their recorded MS/MS spectra was compared against an In-Silico MS/MS fragmentation database (ISDB) (Allard et al., 2016) corresponding to structures reported in the Dictionary of Natural Products (DNP) (Chapman, 2020) using taxonomical informed scoring (Rutz et al., 2019).
2.7 DataWarrior structure similarity search
DataWarrior is open and interactive software for data analysis and visualization that integrates well-established and novel chemoinformatics algorithms in a single environment and notably offers the possibility of exploring chemical space (López-López et al., 2019). In DW, the FragFp descriptor (value ranging from 0 to 1) was used to establish chemical similarity to a reference compound as a filter. A threshold of 0.80 on the latter metric was chosen as a minimum value for the descriptor to display chemically similar structures (www.openmolecules.org, 2015).
2.8 HPLC-PDA
Analyses were conductedon an HP 1260 system equipped with a diode-array detection unit (Agilent Technologies, Santa Clara, CA, United States) using an InterChim® Puriflash HQ C18 column (250 × 4.6 mm i.d., 15 μm, Moluçon, France). The detection was performed by PDA. The PDA parameters were set as follows: UV wavelength at 220, 254, 270, 310 and 366 nm, UV spectra between 190–500 nm were recorded with a threshold of 10 mAU and setting increments of 2 nm. The HPLC conditions were as follows: mobile phase H2O (A) and MeOH (B) both containing 0.1% FA. The flow rate was 1 mL/min, injection volume was 10 μL (at 10 mg/mL concentration), separation temperature was 25°C and sample concentration was 10 mg/mL dissolved in MeOH. The gradient of the mobile phases was set as follows: an initial hold of 1 min at 5% B, gradient flow of 5%–54% of B in 60 min, then another gradient step of 54%–100% in 20 min, followed by 5 min washing by 100% B.
2.9 Flash chromatography with UV detection
The methanol extract of C. brandisiana (roots) was purified with a Büchi Flash chromatography system (Büchi Pump Module C-605, UV Photometer C-640, Control Unit C-620, Fraction Collector C-660), using an InterChim® Puriflash HQ C18 column (120 g, 210 × 30 mm i.d., 15 μm, Moluçon, France). 676.6 mg of extract were mixed in the stationary phase (C18 Zeoprep® 40–63 μm) and sand (50–70 mesh particle size) in a proportion of 1:1:1 in a dry load cell. The detection was performed by a UV Photometer. The Photometer parameters were set as follows: UV wavelengths at 220, 254, 270, 310 nm. The mobile phase was composed of MilliQ water (A) and technical grade methanol (B), both containing 0.1% F.A. The gradient slope was set as follows: an initial hold of 1 min at 5% B, gradient flow of 5%–54% of B in 123 min, then another gradient flow of 54%–100% in 43 min, followed by 10 min washing by 100% B. The separation yielded 71 Fractions of 50 mL each (F01-F71) that were dried using a multi-units evaporator (Multivapor™, Büchi Labortechnik AG, Switzerland). Fraction F19 was identified as containing 16.9 mg of reticuline (10) and its structure was confirmed after HRMS and NMR analyses.
2.10 Semi-preparative HPLC-UV
Fractions from the previous Flash chromatography purification step with MS signals potentially corresponding to alkaloids of interest (fractions number F49, F51 + F52, F56, F57, F59), were subjected to further purification using a Shimadzu system equipped with an LC-20 A module pumps, an SPD-20 A UV/VIS, a 7725I Rheodyne® valve, and an FRC-40 fraction collector (Shimadzu, Kyoto, Japan). The system was controlled by the LabSolutions software, also from Shimadzu. Each fraction was dissolved in 200 µL of MeOH and was mixed with the stationary phase (C18 Zeoprep® 40–63 μm, one spatula), to form uniform slurries. MeOH was evaporated to obtain fine powders which were introduced in a dry-load cell according to our previously published method (Queiroz et al., 2019). The separation was performed with an XBridge BEH C18 OBD Prep column (250 × 19 mm i.d., 5 μm, Waters®). The mobile phase was composed of MilliQ-grade water with 2 mM NEt3 (A) and HPLC-grade acetonitrile with 2 mM NEt3 (B). Fractions were collected using 220 and 254 nm UV signals. The fractions were evaporated to dryness using a Büchi rotary-evaporator system (Büchi Rotavapor R114™ Labortechnik AG, Switzerland).
Fraction F49, obtained by flash chromatography (Section 2.8), was purified by semi-preparative HPLC-UV under the following conditions: The mass of the fraction injected was 5.0 mg, the gradient slope was set as follows: from 5% of B gradually increased to 50% over 60 min, followed by 1 min to go from 50% to 100% of B, with 9 min at 100% of B to finish the run. Compound 2 (marcanine A, 79 µg) was collected at 22 min into the run and compound 5 liriodenine, 0.2 mg) was collected at 44 min.
Fraction F51 and F52, obtained by flash chromatography (Section 2.8), was purified by semi-preparative HPLC-UV under the following conditions: The mass of the fraction injected was 7.9 mg, the gradient slope was set as follows: from 5% of B gradually increased to 50% over 60 min, followed by 1 min to go from 50% to 100% of B, with 9 min at 100% of B to finish the run. Compound 9 (eupolauridine, 64 µg) was collected at 39 min and compound 1 (cleistopholine, 77 µg) was collected at 40.5 min.
Fraction F56, obtained by flash chromatography (Section 2.8), was purified by semi-preparative HPLC-UV under the following conditions: The mass of the fraction injected was 10.6 mg, the gradient slope was set as follows: from 5% of B gradually increased to 50% over 60 min, followed by 1 min to go from 50% to 100% of B, with 9 min at 100% of B to finish the run. Compound 3 (onychine, combined with what was isolated in F57, total mass: 64 µg) was collected at 48 min.
Fraction F57, obtained by flash chromatography (Section 2.8), was purified by semi-preparative HPLC-UV under the following conditions: The mass of the fraction injected was 6.5 mg, the gradient slope was set as follows: from 5% of B gradually increased to 50% over 60 min, followed by 1 min to go from 50% to 100% of B, with 9 min at 100% of B to finish the run. Compound 3 (onychine, combined with what was isolated in F56, total mass: 64 µg) was collected at 48 min and compound 8 (atherospermidine, 16 µg) was collected at 50 min.
Fraction F59, obtained by flash chromatography (Section 2.8), was purified by semi-preparative HPLC-UV under the following conditions: The mass of the fraction injected was 5.4 mg, the gradient slope was set as follows: from 5% of B gradually increased to 50% over 60 min, followed by 1 min to go from 50% to 100% of B, with 9 min at 100% of B to finish the run. Compound 7 (geovanine, 26 µg in total) was collected at 48.5 min into the run and compound 6 (kalasinamide, 28 µg) was collected at 50 min.
2.11 Nuclear magnetic resonance (NMR) measurements
Chemical shifts were reported in parts per million (δ) using the residual CD3OD (δH 3.31; δC 49.0) as internal standards for 1H and 13C NMR, with coupling constants (J) reported in Hz. Additional 2D experiments (HSQC, HMBC, COSY and ROESY) as well as comparisons with literature were used when performing complete structural assignments. Besides, mass measurements for compounds with masses below 0.5 mg were measured using the ERETIC technique (Akoka et al., 1999; Tyburn and Coutant, 2016) on the same NMR 600 MHz spectrometer that was used for structural elucidation, this allowed quantification of all isolated compounds at the µg level for bioactivity measurements. The spectral data of all described compounds can be found in the Supplementary Section S2.
2.12 Bioassays
D. discoideum Ax2(ka) expressing mCherry at the act5 locus (Paschke et al., 2019) was cultured in 10 cm culture dishes in Hl5c medium (Formedium). M. marinum M strain expressing the bacterial lux operon (luxCDABE) was cultured at 32°C under shaking conditions in 7H9 broth (Becton Dickinson, Difco Middlebrook 7H9) containing 0.2% glycerol (Sigma Aldrich), 10% OADC (Becton Dickinson) and 0.05% tyloxapol (Sigma Aldrich). Infection was performed at MOI 25 by spinoculation in Hl5c, as described (Mottet et al., 2021). Then, the cell suspension of infected amoeba was detached from the culture dish and resuspended in Hl5c with 5 U/mL penicillin and 5 μg/mL of streptomycin (Gibco) to inhibit extracellular growth of bacteria (Supplementary Figure S1A). The density of infected cells was quantified using a Countess (Thermo Fisher Scientific), and 1*104 cells were seeded into each well of 384-well plates (Interchim FP-BA8240). Extracts or test compounds resuspended in 30% DMSO in PBS were added to a final vehicle control concentration of 0.3% DMSO and 1% PBS. The plates were sealed (Carl Roth) and fluorescence and luminescence were monitored using a BioTek H1 plate reader in a temperature-controlled environment (set to 24°C). A detailed account of the D. discoideum-M. marinum host pathogen system as it is applied currently will be published in the future.
To determine the anti-bacterial activity of the tested extracts or compounds, M. marinum culture density was assessed by optical density measurement at 600 nm and adjusted to 3.75*105 bacteria per mL in culture medium. Analogously, 7.5*103 bacteria were seeded into each well of 384-well plates (Interchim FP-BA8240), extracts or compounds were added, the plates were sealed and luminescence monitored using a BioTek H1 plate reader in a temperature-controlled environment set to 27°C (Supplementary Figure S1B).
For both assays, growth curves were obtained by measuring the luminescence and fluorescence as a proxy for bacterial growth and host growth, respectively, for 72 h with time-points taken every hour. The normalized residual growth was computed by calculating the area under the curve (AUC, trapezoid method) and normalization to the vehicle control (0.3% DMSO in PBS, set to 1) and a baseline curve (set to 0). The baseline curve was calculated by taking the median of the first measurement of all wells in a plate and assuming this value over the full time-course (Supplementary Figure S1C).
Normalized values were averaged over technical and biological replicates (all experiments at least N = 3) before estimating the IC50. For IC50 estimation, a PL4 regression was used, constraining top and bottom value to 1 and 0, respectively. This was performed using GraphPad Prism (Version 8.0.1).
3 Results and discussion
Our aim in this study is to evaluate the possibility of efficiently finding bioactive hit analogs in a collection of natural extracts. This collection has been subjected to systematic detailed metabolite profiling by LC-HRMS/MS to generate, through extensive MS feature annotation, what we define as a “virtual chemical space.” This proof-of-concept study should demonstrate that targeted isolation of potential bioactive compounds is possible without the need for either time-consuming preliminary bioactivity-guided fractionation procedures, nor bioactivity screening at the extract level. To carry out this work, the following strategy has been developed and is summarized in Figure 1, each of the key steps is presented and described below:
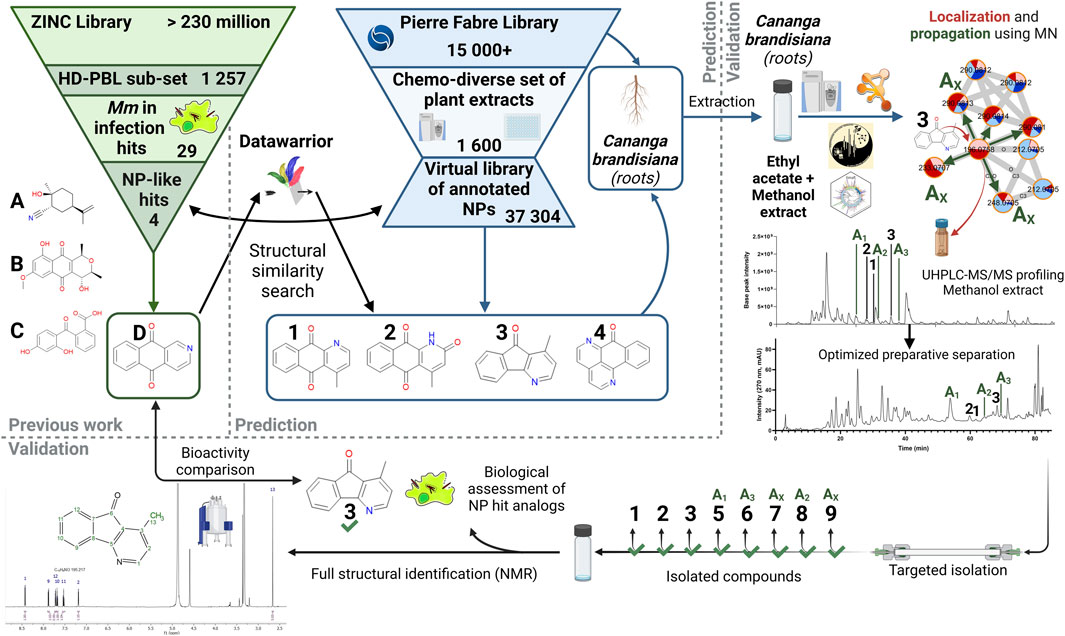
FIGURE 1. General workflow for the targeted isolation of NP hit analogs in a selected plant. Starting from the ZINC Library and the HD-PBL subset (Hanna et al., 2020), 29 Hits stood out, of which only 4 have positive NP-likeness scores (A–D). These structures were searched in the virtual library based on a chemo-diverse subset (Allard et al., 2023) of the PFL using DataWarrior. Compounds 1-4 were detected in this virtual library as constituents of Cananga brandisiana and analogs of compound (D). Annotations were then validated in a newly generated extract and propagated to other analogs (Ax) that were previously undetected. Their LC-peaks were then localized in the extract UHPLC-HRMS metabolite profile and their targeted isolation was performed. Full de novo structural identification by NMR and biological assessment of newly isolated structures (1-3 and 5–9) were carried out.
Reference hits structures were retrieved from our previous study on the anti-mycobacterial activites of a subset of 1,255 commercially available drug-like compounds that target 18 different host/pathogen pathways (ZINC library) (Hanna et al., 2020). Among hits obtained on our intracellular M. marinum infection model, a first step of determination of their possible structural similarity with Natural Products was carried out. This was performed by calculation of a “Natural-Product likeness” score (cf. 3.1) (Ertl et al., 2008) virtual chemical space. The re-ranked list of structures according to this score was used for a systematic search of analogs in the virtual chemical space consisting of all structural annotations obtained on the massive metabolome dataset (1,600 extracts) of our Natural Extracts (NE) library (Allard et al., 2023). To efficiently search for hits in the virtual chemical library of all extracts, a software enabling molecular similarity measures (Datawarrior) was used. This procedure allowed the ranking of the annotated structures that were the most similar to each of the identified hits of the ZINC library. All structures annotated in the NE library are linked to the extract of the plant part from which they originated. This enabled the identification of the plant extracts in which analogs should be found (cf. 3.2). In order to isolate hit analogs, extracts were newly prepared from the original plant material at a larger scale (cf. 3.3). Based on the profiling of these extracts, hit analogs were localized and their annotations could be propagated to find additional analogs that were previously undetected. From there, targeted isolation of hit analogs was carried out. These pure NPs were fully characterized by NMR for full de novo structural identification (cf. 3.4) and their biological activities were assessed (cf. 3.5).
3.1 Determination of the NP-likeness-score for hits of the ZINC library
From our previous study (Hanna et al., 2020), the 37 hit compounds active on M. marinum were considered. We excluded hits from anti-virulence assays on M. marinum to focus exclusively on hits that reduced its growth in infection, resulting in 29 hit compounds (Supplementary Data table for DW). A first round of filtering was applied to the 29 hit compounds from the ZINC Library, using a score of “Natural Product likeness” described by Ertl et al. (2008) for each of those hits. This score translates into numerical values that vary between −5 (least NP-like) and +5 (most NP-like).
Application of this NP-likeness score gives values ranging from −2.667 to 1.718 for these 29 hit compounds. Based on the distribution of the NP-likeness scores for a collection of synthetic molecules (mostly negative scores) compared to that of Natural Products libraries (mostly positive scores), it was decided that a cut-off at 0 would be suitable for the choice of prioritized NP-like hits. Compounds having positive NP-likeness scores were therefore considered. In our case, only compounds A, B, C and D (Figure 1) fell into that category and obtained respective NP-likeness scores of 1.718, 1.578, 0.121 and 0.033. This filtering confirmed that the HD-PBL compounds were mostly of synthetic origin, with only 4 out of 29 hit structures passing the positive threshold for NP-likeness.
3.2 Search for analogs of the NP-like hits in a virtual library of annotated compounds
In order to search for these four compounds (A, B, C, D) and/or analogs in NEs, we exploited a set of structural annotations obtained from a library of 1,600 chemo-diverse plant extracts previously profiled by LC-HRMS/MS analysis (Allard et al., 2023). For this we compared spectra of recorded MS/MS spectra against an In-Silico MS/MS fragmentation database (ISDB) (Allard et al., 2016) corresponding to structures reported in the Dictionary of Natural Products (DNP) (®CRC Press, 2020) using taxonomical informed scoring (Rutz et al., 2019). This yielded a set of 36,128 annotations (which can be found in Supplementary Tables S1, S2). In this library of putative annotations, all compounds annotated are linked to the extract they originated from and this can be efficiently visualized at the level of each detected feature in MNs. This collection of structural candidates thereby constitutes a “virtual chemical library” which can be used for structural similarity search against the HD-PBL set. The description of the extract set can be found in Allard et al. (2023).
All structures of the virtual library (in SMILES format) and their associated metadata (extract and taxonomical information) were imported in Datawarrior (DW) (DataWarrior User Manual, 2015; Sander et al., 2015). Each of the NP-like hits (A, B, C and D) from the HD-PBL were searched against all annotated compounds using the structural similarity filter of DW. The number of annotations displayed was limited to no more than 10 molecules most relevant structurally to the hit, by varying the similarity score using the FragFp descriptor (usually by placing it at a minimum score of 0.80). This descriptor relies on a dictionary of 512 predefined structure fragments and contains one bit for each of these fragments. Each bit can be set to 1 if the corresponding fragment is present in the molecule at least one time. Most heteroatoms have also been replaced by wild cards, so that single atom replacements do not affect the similarity score too much, to reflect a chemist’s natural similarity perception (www.openmolecules.org, 2015).
Using this approach for each hit compound, we always observed less than 10 plants usually showing the presence of one predicted analog or the hit structure itself. One plant (C. brandisiana) however clearly stood out, as it contained several analogs at a time of the aza-anthraquinone hit compound D. These analogs were annotated as cleistopholine 1, marcanine A 2, onychine 3, sampangine 4. This particular plant was therefore selected for further phytochemical investigations since it had the potential to yield an interesting subset of compounds structurally related to the hit.
3.3 Localization of hit analogs in extracts using molecular networks (MN)
To prepare for the isolation of targeted metabolites ethyl acetate and methanol extracts from both roots and trunk barks of C. brandisiana were newly prepared from limited dry material available from the plant part collection (14 g for roots and 49 g for trunk barks). They were submitted to metabolite profiling and a new Feature Based Molecular Network (FBMN) was constructed. Three out of the four targeted compounds (cleistopholine 1, marcanine A 2, onychine 3) were found again within that MN. They were located within a larger cluster of MS features of compounds classified as “Aporphines” (Figure 2A). A “cluster” in this case refers to the aggregates of several features (or nodes) that can be observed in Figure 2A. Nodes are linked together to form clusters whenever their MS/MS spectral similarity passes a certain set threshold. This threshold of similarity is usually described on a range of “cosine scores,” ranging from 0 (complete dissimilarity) to 1 (same spectrum) and is typically set at a minimum of 0.7 required for an “edge” to be formed between two nodes. Detailed annotation of the MN indicated that other “Aporphines” clusters were also present, which allowed us to propagate the annotations from the nodes we targeted. This way we could identify additional analogs that were previously undetected (compounds 5, 6, 7, 8 and 9, Figure 1).
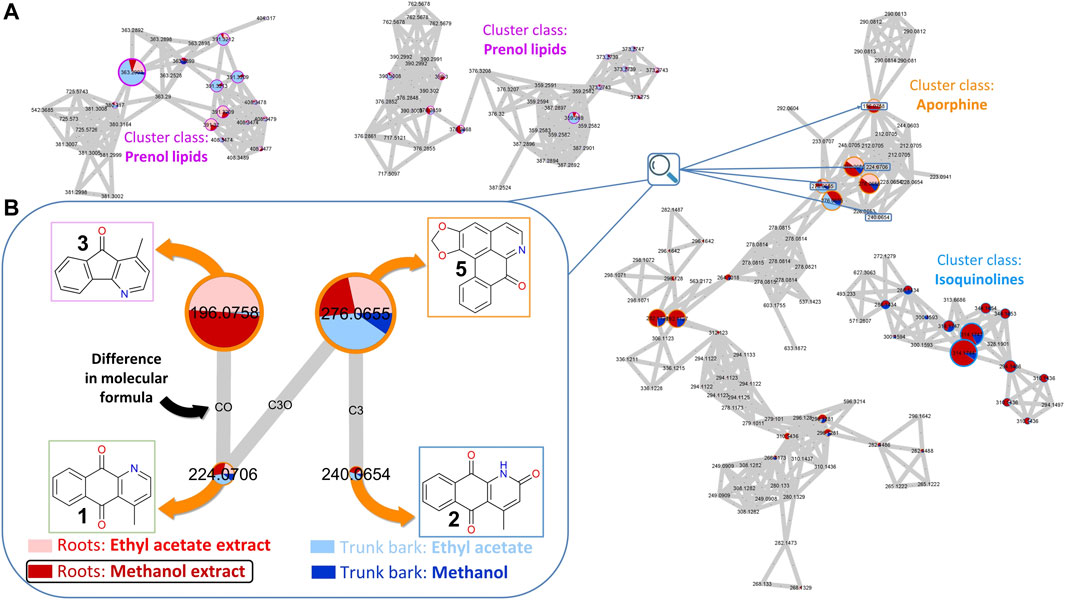
FIGURE 2. Feature-Based Molecular Network (FBMN) of C. brandisiana (roots and trunk bark). (A) Snapshot of the FBMN highlighting the aporphine cluster containing the targeted compounds, with some surrounding clusters—(B) Nodes of interest of 4 analogs of the initial hit structure and their connections highlighted. The relative size of the nodes reflects the intensity of the corresponding ion in the methanol extract of C. brandisiana roots. The coloured pie chart on each node corresponds to the relative intensities of a given ion in the various extracts. The outside circles on each node are coloured according to each cluster class. Edges between each nodes were drawn if the cosine factor between two nodes exceeded 0.7 and were labelled according to the difference in their atomic composition.
In the MN presented in Figure 2, the node size corresponds to the intensity of the corresponding MS feature. Each node has an associated retention time which enables precise localization of all compounds of interest in the UHPLC-HRMS metabolite profile of the extract. Careful mining of the latter cluster highlighted an additional analog compound that also appeared to strike out around the nodes of interest: liriodenine (5) (Figure 2B). Most of the other features detected were of low intensity in the corresponding MS trace.
On the other hand, the pie charts within the nodes corresponds to the relative intensities of a feature in different extracts (different colors). This highlighted that compound 3 was only found in the roots while the three other compounds (1, 2, 5) appeared in both plant parts. As all extracts were analyzed at a fixed concentration and injection volume (5 mg.mL−1 and 2 µL, respectively), comparing ion intensities between extracts proved relevant, despite not being an absolute metric for compound quantities measurements. Since the methanol roots extract (778.6 mg) was obtained in much larger quantities than the ethyl acetate roots extract (72.8 mg), the targeted isolation was performed on that extract.
3.4 Targeted isolation of hit analogs in a selected extract for chemical and biological characterization
Based on the retention times and m/z values of the feature of interest, their corresponding LC-peaks could be efficiently located in the MS profile of the entire extract and allowed us to establish a zone of interest within which to optimize the gradient of separation (Figure 3A). It was decided that a gradient transfer (Guillarme et al., 2008) from analytical HPLC to Flash chromatography would be ideal to work with 700 mg of extract, while retaining acceptable quality for the separation (Figure 3B).
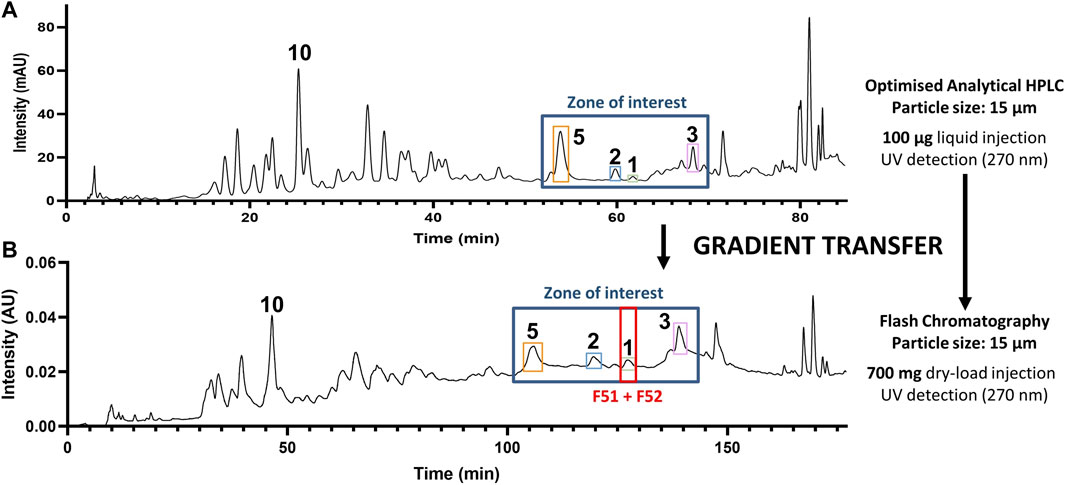
FIGURE 3. Gradient transfer from analytical HPLC to flash chromatography. (A) HPLC-PDA analytical-scale separation and (B) Flash chromatography separation of the methanol extract of C. brandisiana (roots) with a highlight of the zone containing compounds of interest. Gradient transfer calculations between both separations were carried according to the method described (Guillarme et al., 2008).
Post-chromatographic analysis by LC-MS of all fractions in the region of interest revealed that all targeted features were present, however they still co-eluted with other compounds. This first separation allowed a major alkaloid (10) to be isolated at this stage. Most targeted compounds in the zone of interest would thus require a further round of purification by High-resolution semi-preparative HPLC combined with dry-load injection (Queiroz et al., 2019), as they were often flagged within fractions that remained complex in composition (Figure 4A). To achieve a different chromatographic selectivity and according to the expected structures of the compounds targeted (alkaloids), we chose to switch the solvent buffers from standard 0.1% Formic acid (FA) to 2 mM triethylamine (NEt3), to play with the pKa of basic amines contained in most molecules. In standard FA-buffered conditions, compounds like cleistopholine (1) would be protonated on their nitrogen atom as the separation medium is slightly acidic. When switching to a more basic medium with 2 mM NEt3 (with an observed pH of around 9), compound 1 was more likely to be deprotonated, therefore less hydrophilic, which explained why it was more retained on a hydrophobic C18 column (Figure 4B).
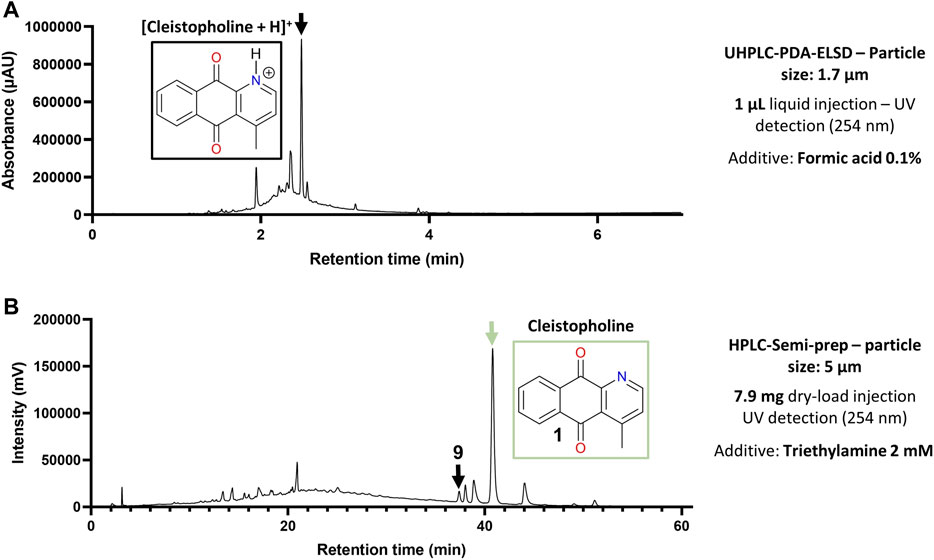
FIGURE 4. Optimization of the chromatographic conditions for alkaloids isolation. (A) Chromatographic behavior of cleistopholine (3) on the chromatogram of flash fraction F51 with 0.1% Formic acid in solvents. (B) Retention time shift of 3 in flash fractions F51 and F52 with 2 mM NEt3 in solvents.
The very low masses obtained in the end for most compounds of interest (µg-scale) suggested that the amounts present in the mixture were overestimated due to the high ionization potential of such compounds, as well as their extensive UV-absorbing π-systems. Yet those quantities proved to be sufficient for both structural characterization and preliminary assessment of biological activity. At this scale however, we had to resort to NMR-based quantification techniques (ERETIC) (Akoka et al., 1999; Tyburn and Coutant, 2016) to have an accurate measurement of the mass of compounds obtained (Figure 5), which was crucial for any biological assessment to take place. Among the 12 isolated compounds, some were previously reported to occur in C. brandisiana (1), others at the genus Cananga level (4), others only at the Family-level (Annonaceae) (3) and the remaining were only reported in unrelated taxa (4). All compounds isolated in this work along with their corresponding taxonomical information were shared for further use by the community in the LOTUS database (Rutz et al., 2022).
3.5 Biological activities of the plant extracts, the NP-like hit and NP hit analogs
Based on our search for NP-like hit analogs, several analogs of the bioactive hit were predicted in C. brandisiana extracts (see Section 3.2). In order to verify that bioactivity could be observed already at the level of the plant extract, the anti-bacterial and the anti-infective properties of these extracts were examined prior to testing of isolated NP hit analogs.
Methanol extracts of C. brandisiana trunk bark and roots were tested in the D. discoideum-M. marinum system and on M. marinum in broth at 25 μg/mL and growth curves were monitored for 72 h to determine their activity (Figure 6). Both plant parts were highly active on M. marinum in infection, showing an inhibition of M. marinum growth reaching 90% compared to the vehicle control (Figures 6A, D, normalized residual growth of 0.1 ± 0.08 and −0.22 ± 0.05 for roots and trunk bark extracts, respectively). When these extracts were tested on bacteria in broth however, the growth inhibition was less striking compared to M. marinum in infection (Figures 6C, D, normalized residual growth of 0.73 ± 0.04 and 0.36 ± 0.08 for roots and trunk bark extracts, respectively). On the host side, the trunk bark methanolic extract also strongly restricted D. discoideum growth hinting towards a potential cytotoxic activity, whereas the root extract showed only a slight restriction of D. discoideum growth (Figures 6B, D, normalized residual growth of 0.93 ± 0.1 and −0.06 ± 0.1 for roots and trunk bark extracts, respectively). Interestingly and as expected from its chemical composition, the methanol extract of C. brandisiana exhibited striking and selective anti-infective activity on intracellular M. marinum during infection compared to M. marinum in broth.
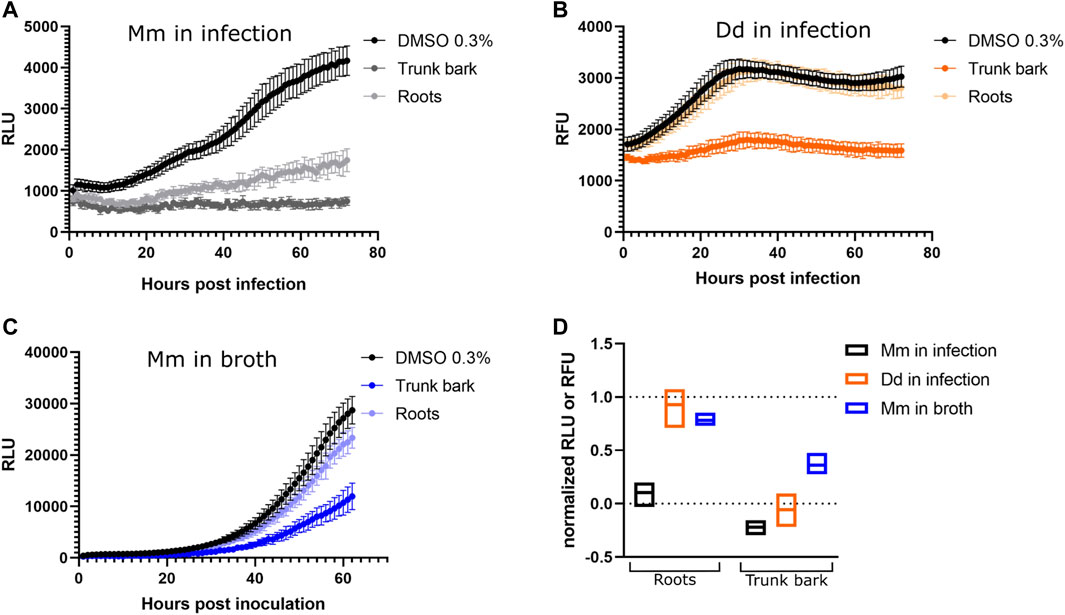
FIGURE 6. Activity data of extracts. (A–C) Growth curves of M. marinum in infection, D. discoideum in infection and M. marinum in broth, respectively. The x-axes represent time, either in hours post infection (A,B) or hours post inoculation (C). The infection was followed for 72 h (A,B), the growth in broth for 62 h (C). The y-axes represent Random Luminescence Units (RLU) (A,C) or Random Fluorescence Units (RFU) (B). Growth curves of the methanol extracts of C. brandisiana, trunk bark and roots, are represented in different shades of colors, whereas the vehicle control (0.3% DMSO) is depicted in black. The graphs show the averages over biological and technical replicates and the corresponding standard deviations. (D): Summarized activity data of C. brandisiana methanol extracts in infection (black and orange) and in broth (blue). Both plant parts of C. brandisiana are highly active on M. marinum in infection (normalized residual growth of 0.1 ± 0.08 and −0.22 ± 0.05, respectively), and to lesser extent in broth (normalized residual growth of 0.73 ± 0.04 and 0.36 ± 0.08, respectively), while growth of the amoeba is not restricted with roots parts but is markedly restricted with the trunk bark (normalized residual growth of 0.93 ± 0.1 and −0.06 ± 0.1, respectively). Extracts were tested at 25 μg/mL.
Following the targeted isolations (see Section 3.4), the anti-infective activity of the isolated structures was assessed in the D. discoideum-M. marinum system. Compounds (1–3, 5–10) were resuspended in 30% DMSO in PBS and tested at 25 μg/mL. Preliminary fluorescence and luminescence data indicated strong activity on M. marinum in infection, but also a growth inhibition of D. discoideum, hinting at a detrimental effect of these compounds on the host as well (data not shown). This non-specific effect was observed in a preliminary experiment for most of the isolated compounds from C. brandisiana (1, 2, 6, 7, 9). The notable exception to this however was onychine (3) which seemed most selective towards the pathogen over the host.
As we reported in Hanna et al. (2020), the reference hit compound D (ZINC00120276) was tested at 30 µM and inhibited growth of M. marinum in D. discoideum by 28% compared to the vehicle control. To validate the compound’s activity and to disentangle a possible host inhibition effect, we used our dual readout of the D. discoideum-M. marinum system to determine the IC50 of this compound on M. marinum in broth and in infection. The compound was tested in a serial dilution ranging from 12.5 to 0.05 µM with a dilution factor of 2. The obtained data confirmed our initial observation showing that the compound D (ZINC00120276) exerted an anti-infective activity. In addition, we calculated the IC50 using a PL4 regression on M. marinum in infection and in broth which was 1.8 and 1.9 µM, respectively. On the other hand, we could not calculate the IC50 on D. discoideum as the tested concentrations did not show any drastic inhibition of the host growth. This data clearly indicates that the reference hit compound D has a selective anti-mycobacterial activity with an IC50 in the low micromolar range and a low activity on D. discoideum (Figure 7A).
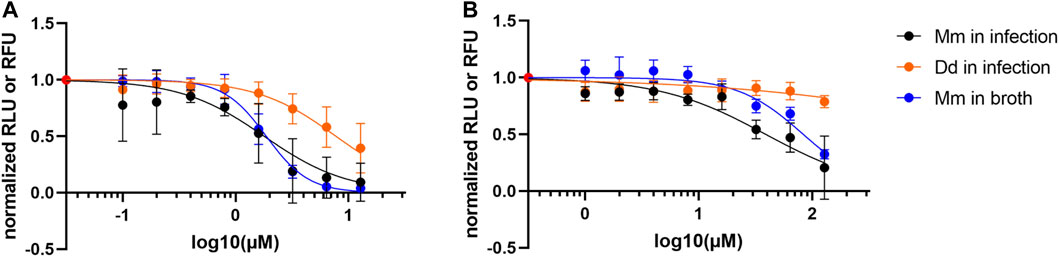
FIGURE 7. Activity data of compounds. (A) Dose response curve of ZINC00120276. The compound is active on M. marinum in infection and in broth (IC50 estimates: 1.8 and 1.9 µM respectively), while affecting amoeba growth to a lesser extent. The compound was tested in a serial dilution of 8 dosages, with 12.8 µM being the top dose and a dilution factor of 2. (B) Dose response curve of Onychine (3). The compound is active on M. marinum in infection and in broth (IC50 estimates: 39 and 83 µM, respectively), while affecting amoeba growth to a lesser extent. The compound was tested in a serial dilution of eight dosages, with 128 µM being the top dose and a dilution factor of 2.
After preliminary biological assays performed on isolated compounds, onychine (3), a 4-azafluorenone alkaloid, retained our interest due to its selective activity on M. marinum. Onychine (3) was isolated at the µg scale with precise quantification by NMR, allowing us to characterize the NP-like hit analog more precisely by obtaining a dose-response curve. The subsequently observed anti-infective properties of onychine exhibited activity both on M. marinum in infection and in broth (Figure 7B, IC50 of 39 and 83 µM, respectively), while restricting D. discoideum growth to a very low extent. Although onychine was approximately 20 times less potent than ZINC00120276 on M. marinum in infection, they share a similar activity profile. Both compounds share selective activity on M. marinum in infection over D. discoideum and both compounds act in infection and in broth.
4 Conclusion
Taken together, we illustrated how the combination of molecular networks and bioassays could leverage a large natural extracts library. The transformation of the NE library through extensive annotation processes to a virtual chemical library enabled the search for active compounds via chemical similarity. This proof-of-concept study demonstrates how different natural analogs of an a priori hit scaffold originating from a lead-like library can be targeted in a NE. It led to the effective identification of NP-like structural analogs of reference hits that were then characterized as active natural analogs in biological assays. Out of the four structures annotated in the extract of C. brandisiana, we targeted the isolation of three of them. We could then propagate the annotations with a MN generated through the GNPS platform which was used to isolate a total of eight structural hit analogs. The set of targeted structures showed preliminary activity as well as the extract itself, with striking selectivity. Similar bioactivity profiles were obtained for the reference hit structure and for one of the natural analogs (onychine, with an IC50 of 39 µM in infection), thus validating the approach. We highlight that this combination of computational, analytical and biological workflows translates the navigation of the virtual chemical space of annotated metabolites into the effective isolation of hit analogs.
Such an approach will accelerate the discovery of new lead compounds in the fight against antibiotic resistance in TB, but also for other pathogens. It could therefore be generalized for finding analogs of bioactive hit compounds and rapidly generate subsets of structurally related compounds for any type of biological activity assessment. This would serve as a first set of structure-activity information that could help target the synthesis of structural analogs, before envisioning full Structure-Activity Relationship (SAR) studies on a chosen scaffold.
Data availability statement
The datasets presented in this study can be found in online repositories. The names of the repository/repositories and accession number(s) can be found in the article/Supplementary Material. The raw data for the NMR analyses is available at doi: https://doi.org/10.26037/yareta:4kk4gbotzzhqhppyp6hisefr7m The Molecular Network used in this study can be accessed through its GNPS Job ID = e038d15b1a9e49b7aec23e47f9562e32.
Author contributions
OK: Conceptualization, Data curation, Investigation, Methodology, Validation, Visualization, Writing–original draft, Writing–review and editing. JN: Conceptualization, Data curation, Investigation, Methodology, Validation, Visualization, Writing–original draft, Writing–review and editing. P-MA: Conceptualization, Data curation, Methodology, Software, Writing–review and editing. LM: Data curation, Investigation, Writing–review and editing. BD: Resources, Writing–review and editing. AG: Resources, Writing–review and editing. NH: Conceptualization, Writing–review and editing, Data curation, Investigation, Methodology, Supervision. EQ: Investigation, Methodology, Supervision, Writing–review and editing. TS: Conceptualization, Funding acquisition, Project administration, Supervision, Writing–review and editing. J-LW: Conceptualization, Funding acquisition, Project administration, Supervision, Writing–original draft, Writing–review and editing.
Funding
The authors declare financial support was received for the research, authorship, and/or publication of this article. The authors are thankful to the Swiss National Science Foundation (SNSF) for the financial support of this project (no. CRSII5_189921/1). J-LW is also thankful to the SNSF for the support in the acquisition of the NMR 600 MHz (SNF Research Equipment grant 316030_164095).
Acknowledgments
The authors are grateful to Green Mission Pierre Fabre and the Pierre Fabre Research Institute, Toulouse, France for establishing and sharing their unique libraries of plants and extracts. BioRender was used for the conception of most figures with a publication license provided by author TS. Graphs were made using GraphPad Prism (version 9.2.0) and chemical structures were drawn with ChemDraw (version 20.1.0.110), both licensed to OK by the University of Geneva. The Dictionary of Natural Products (DNP) was used under the license acquired by the Wolfender Lab.
Conflict of interest
The authors declare that the research was conducted in the absence of any commercial or financial relationships that could be construed as a potential conflict of interest.
The authors PM-A, EQ, and TS declared that they were editorial board members of Frontiers, at the time of submission. This had no impact on the peer review process and the final decision.
Publisher’s note
All claims expressed in this article are solely those of the authors and do not necessarily represent those of their affiliated organizations, or those of the publisher, the editors and the reviewers. Any product that may be evaluated in this article, or claim that may be made by its manufacturer, is not guaranteed or endorsed by the publisher.
Supplementary material
The Supplementary Material for this article can be found online at: https://www.frontiersin.org/articles/10.3389/fntpr.2023.1279761/full#supplementary-material
References
Akoka, S., Barantin, L., and Trierweiler, M. (1999). Concentration measurement by proton NMR using the ERETIC method. Anal. Chem. 71, 2554–2557. doi:10.1021/ac981422i
Allard, P.-M., Gaudry, A., Quirós-Guerrero, L.-M., Rutz, A., Dounoue-Kubo, M., Walker, T. W. N., et al. (2023). Open and reusable annotated mass spectrometry dataset of a chemodiverse collection of 1,600 plant extracts. GigaScience 12, giac124. doi:10.1093/gigascience/giac124
Allard, P.-M., Péresse, T., Bisson, J., Gindro, K., Marcourt, L., Pham, V. C., et al. (2016). Integration of molecular networking and in-silico MS/MS fragmentation for natural products dereplication. Anal. Chem. 88, 3317–3323. doi:10.1021/acs.analchem.5b04804
Brodin, P., Poquet, Y., Levillain, F., Peguillet, I., Larrouy-Maumus, G., Gilleron, M., et al. (2010). High content phenotypic cell-based visual screen identifies Mycobacterium tuberculosis acyltrehalose-containing glycolipids involved in phagosome remodeling. Public Libr. Sci. Pathog. 6, e1001100. doi:10.1371/journal.ppat.1001100
Cazzaniga, G., Mori, M., Chiarelli, L. R., Gelain, A., Meneghetti, F., and Villa, S. (2021). Natural products against key Mycobacterium tuberculosis enzymatic targets: emerging opportunities for drug discovery. Eur. J. Med. Chem. 224, 113732. doi:10.1016/j.ejmech.2021.113732
Chambers, M. C., Maclean, B., Burke, R., Amodei, D., Ruderman, D. L., Neumann, S., et al. (2012). A cross-platform toolkit for mass spectrometry and proteomics. Nat. Biotechnol. 30, 918–920. doi:10.1038/nbt.2377
Chapman, H. (2020). Dictionary of natural products on DVD (23: 1). CRC Press, Taylor & Francis Group. Available at: http://dnp.chemnetbase.com/"
Cytoscape (2001). Cytoscape: an open-source platform for complex network analysis and visualization. Available at: https://cytoscape.org/ (Accessed June 5, 2023).
DataWarrior User Manual (2015). openmolecules.org. Available at: https://openmolecules.org/help/similarity.html (Accessed December 20, 2022).
David, B., Wolfender, J.-L., and Dias, D. A. (2015). The pharmaceutical industry and natural products: historical status and new trends. Phytochem. Rev. 14, 299–315. doi:10.1007/s11101-014-9367-z
Dunn, J. D., Bosmani, C., Barisch, C., Raykov, L., Lefrançois, L. H., Cardenal-Muñoz, E., et al. (2018). Eat prey, live: dictyostelium discoideum as a model for cell-autonomous defenses. Front. Immunol. 8, 1906. doi:10.3389/fimmu.2017.01906
Ertl, P., Roggo, S., and Schuffenhauer, A. (2008). Natural product-likeness score and its application for prioritization of compound libraries. J. Chem. Inf. Model. 48, 68–74. doi:10.1021/ci700286x
GNPS (2016). Gnps - analyze, connect, and network with your mass spectrometry data. Available at: https://gnps.ucsd.edu/ProteoSAFe/static/gnps-splash.jsp (Accessed June 5, 2023).
Guillarme, D., Nguyen, D. T. T., Rudaz, S., and Veuthey, J.-L. (2008). Method transfer for fast liquid chromatography in pharmaceutical analysis: application to short columns packed with small particle. Part II: gradient experiments. Eur. J. Pharm. Biopharm. 68, 430–440. doi:10.1016/j.ejpb.2007.06.018
Habjan, E., Ho, V. Q. T., Gallant, J., van Stempvoort, G., Jim, K. K., Kuijl, C., et al. (2021). An anti-tuberculosis compound screen using a zebrafish infection model identifies an aspartyl-tRNA synthetase inhibitor. Dis. Model. Mech. 14, dmm049145. doi:10.1242/dmm.049145
Han, J., Liu, X., Zhang, L., Quinn, R. J., and Feng, Y. (2022). Anti-mycobacterial natural products and mechanisms of action. Nat. Prod. Rep. 39, 77–89. doi:10.1039/D1NP00011J
Hanna, N., Kicka, S., Chiriano, G., Harrison, C., Sakouhi, H. O., Trofimov, V., et al. (2020). Identification of anti-mycobacterium and anti-legionella compounds with potential distinctive structural scaffolds from an HD-PBL using phenotypic screens in amoebae host models. Front. Microbiol. 11, 266. doi:10.3389/fmicb.2020.00266
Houriet, J., Allard, P.-M., Queiroz, E. F., Marcourt, L., Gaudry, A., Vallin, L., et al. (2020). A mass spectrometry-based metabolite profiling workflow for selecting abundant specific markers and their structurally related multi-component signatures in traditional Chinese medicine multi-herb formulae. Front. Pharmacol. 11, 578346. doi:10.3389/fphar.2020.578346
Kalsum, S., Otrocka, M., Andersson, B., Welin, A., Schön, T., Jenmalm-Jensen, A., et al. (2022). A high content screening assay for discovery of antimycobacterial compounds based on primary human macrophages infected with virulent Mycobacterium tuberculosis. Tuberculosis 135, 102222. doi:10.1016/j.tube.2022.102222
Kessner, D., Chambers, M., Burke, R., Agus, D., and Mallick, P. (2008). ProteoWizard: open source software for rapid proteomics tools development. Bioinformatics 24, 2534–2536. doi:10.1093/bioinformatics/btn323
López-López, E., Naveja, J. J., and Medina-Franco, J. L. (2019). DataWarrior: an evaluation of the open-source drug discovery tool. Expert Opin. Drug Discov. 14, 335–341. doi:10.1080/17460441.2019.1581170
Mottet, M., Bosmani, C., Hanna, N., Nitschke, J., Lefrançois, L. H., and Soldati, T. (2021). “Novel single-cell and high-throughput microscopy techniques to monitor Dictyostelium discoideum–Mycobacterium marinum (M. marinum) infection dynamics,” in Mycobacteria protocols methods in molecular biology. Editors T. Parish,, and A. Kumar (New York, NY: Springer US), 183–203. doi:10.1007/978-1-0716-1460-0_7
Newman, D. J., and Cragg, G. M. (2012). Natural products as sources of new drugs over the 30 years from 1981 to 2010. J. Nat. Prod. 75, 311–335. doi:10.1021/np200906s
Newman, D. J., and Cragg, G. M. (2007). Natural products as sources of new drugs over the last 25 years. J. Nat. Prod. 70, 461–477. doi:10.1021/np068054v
Newman, D. J., and Cragg, G. M. (2020). Natural products as sources of new drugs over the nearly four decades from 01/1981 to 09/2019. J. Nat. Prod. 83, 770–803. doi:10.1021/acs.jnatprod.9b01285
openmolecules 2015, www.openmolecules.org. Available at: https://openmolecules.org/help/similarity.html [Accessed June 5, 2023].
Paschke, P., Knecht, D. A., Williams, T. D., Thomason, P. A., Insall, R. H., Chubb, J. R., et al. (2019). Genetic engineering of Dictyostelium discoideum cells based on selection and growth on bacteria. J. Vis. Exp., e58981. doi:10.3791/58981
Queiroz, E. F., Alfattani, A., Afzan, A., Marcourt, L., Guillarme, D., and Wolfender, J.-L. (2019). Utility of dry load injection for an efficient natural products isolation at the semi-preparative chromatographic scale. J. Chromatogr. A 1598, 85–91. doi:10.1016/j.chroma.2019.03.042
Rutz, A., Dounoue-Kubo, M., Ollivier, S., Bisson, J., Bagheri, M., Saesong, T., et al. (2019). Taxonomically informed scoring enhances confidence in natural products annotation. Front. Plant Sci. 10, 1329. doi:10.3389/fpls.2019.01329
Rutz, A., Sorokina, M., Galgonek, J., Mietchen, D., Willighagen, E., Gaudry, A., et al. (2022). The LOTUS initiative for open knowledge management in natural products research. eLife 11, e70780. doi:10.7554/eLife.70780
Sander, T., Freyss, J., von Korff, M., and Rufener, C. (2015). DataWarrior: an open-source program for chemistry aware data visualization and analysis. J. Chem. Inf. Model. 55, 460–473. doi:10.1021/ci500588j
Sapriel, G., and Brosch, R. (2019). Shared pathogenomic patterns characterize a new phylotype, revealing transition toward host-adaptation long before speciation of Mycobacterium tuberculosis. Genome Biol. Evol. 11, 2420–2438. doi:10.1093/gbe/evz162
Schmitt, E. K., Moore, C. M., Krastel, P., and Petersen, F. (2011). Natural products as catalysts for innovation: A pharmaceutical industry perspective. Curr. Opin. Chem. Biol. 15, 497–504. doi:10.1016/j.cbpa.2011.05.018
Sharing nature (2022). Sharing nature’s genetic resources. Available at: https://environment.ec.europa.eu/topics/nature-and-biodiversity/sharing-natures-genetic-resources_en (Accessed June 5, 2023).
Sterling, T., and Irwin, J. J. (2015). Zinc 15 – ligand discovery for everyone. J. Chem. Inf. Model. 55, 2324–2337. doi:10.1021/acs.jcim.5b00559
Theriault, M. E., Pisu, D., Wilburn, K. M., Lê-Bury, G., MacNamara, C. W., Michael Petrassi, H., et al. (2022). Iron limitation in M. tuberculosis has broad impact on central carbon metabolism. Commun. Biol. 5, 685. doi:10.1038/s42003-022-03650-z
Tobin, D. M., and Ramakrishnan, L. (2008). Comparative pathogenesis of Mycobacterium marinum and Mycobacterium tuberculosis. Cell. Microbiol. 10, 1027–1039. doi:10.1111/j.1462-5822.2008.01133.x
Tyburn, J.-M., and Coutant, J. (2016). TopSpin ERETIC 2 electronic to access in-vivo concentration user manual version 001.
Vidal, D., Thormann, M., and Pons, M. (2005). LINGO, an efficient holographic text-based method to calculate biophysical properties and intermolecular similarities. J. Chem. Inf. Model. 45, 386–393. doi:10.1021/ci0496797
Wolfender, J.-L., Litaudon, M., Touboul, D., and Ferreira Queiroz, E. (2019). Innovative omics-based approaches for prioritisation and targeted isolation of natural products – new strategies for drug discovery. Nat. Prod. Rep. 36, 855–868. doi:10.1039/C9NP00004F
World Health Organisation (2022). Tuberculosis. World Health Organ. Available at: https://www.who.int/news-room/fact-sheets/detail/tuberculosis (Accessed June 5, 2023]. doi:10.1016/S2666-5247(22)00359-7
World Health Organisation (2012). Tuberculosis laboratory biosafety manual. Available at: https://www.who.int/publications/i/item/9789241504638 (Accessed June 5, 2023).
Keywords: molecular network, bioactive compounds anticipation, anti-mycobacterial, targeted isolation, structural similarity search, large collection of plants, natural products
Citation: Kirchhoffer OA, Nitschke J, Allard P-M, Marcourt L, David B, Grondin A, Hanna N, Queiroz EF, Soldati T and Wolfender J-L (2023) Targeted isolation of natural analogs of anti-mycobacterial hit compounds based on the metabolite profiling of a large collection of plant extracts. Front. Nat. Produc. 2:1279761. doi: 10.3389/fntpr.2023.1279761
Received: 18 August 2023; Accepted: 21 September 2023;
Published: 12 October 2023.
Edited by:
Zhiyong Li, Shanghai Jiao Tong University, ChinaReviewed by:
Smith B. Babiaka, University of Buea, CameroonDaniel Pecoraro Demarque, Universidade de São Paulo, Brazil
Copyright © 2023 Kirchhoffer, Nitschke, Allard, Marcourt, David, Grondin, Hanna, Queiroz, Soldati and Wolfender. This is an open-access article distributed under the terms of the Creative Commons Attribution License (CC BY). The use, distribution or reproduction in other forums is permitted, provided the original author(s) and the copyright owner(s) are credited and that the original publication in this journal is cited, in accordance with accepted academic practice. No use, distribution or reproduction is permitted which does not comply with these terms.
*Correspondence: Jean-Luc Wolfender, amVhbi1sdWMud29sZmVuZGVyQHVuaWdlLmNo