- 1School of Electrical and Computer Engineering, Georgia Institute of Technology, Atlanta, GA, United States
- 2Wallace H. Coulter Department of Biomedical Engineering, Georgia Institute of Technology, Atlanta, GA, United States
- 3Department of Neurology, Emory University School of Medicine, Atlanta, GA, United States
Levodopa is the most common therapy to reduce motor symptoms of parkinsonism. However, levodopa has potential to exacerbate cardiovascular autonomic (CVA) dysfunction that may co-occur in patients. Heart rate variability (HRV) is the most common method for assessing CVA function, but broader monitoring of CVA function and levodopa effects is typically limited to clinical settings and symptom reporting, which fail to capture its holistic nature. In this study, we evaluated the feasibility of a multimodal wearable chest patch for monitoring changes in CVA function during clinical and 24-h ambulatory (at home) conditions in 14 patients: 11 with Parkinson’s disease (PD) and 3 with multiple system atrophy (MSA). In-clinic data were analyzed to examine the effects of orally administered levodopa on CVA function using a pre (OFF) and 60-min (ON) post-exposure protocol. Wearable-derived physiological markers related to the electrical and mechanical activity of the heart alongside vascular function were extracted. Pre-ejection period (PEP) and ratio of PEP to left ventricular ejection time index (LVETi) increased significantly (p
1 Introduction
Parkinson’s disease (PD) and parkinsonian multiple system atrophy (MSA) are alpha-synucleinopathies (
The reported cardiovascular effects of levodopa vary widely as studies often differ in route of administration, dosage, type and timing of physiological measurements, and participant characteristics. When administered in large doses, levodopa can produce a sustained positive inotropic effect in individuals with PD and heart failure (Whitsett and Goldberg, 1972; Rajfer et al., 1984); however, in studies of parkinsonian individuals, typical therapeutic amounts for motor parkinsonism observed a negative inotropic effect (Wolf et al., 2006; Noack et al., 2014), with reductions in stroke volume and blood pressure (BP) (Bouhaddi et al., 2004; Liu et al., 2023; Cani et al., 2024; Earl et al., 2024). Notably, these cardiovascular effects have primarily been assessed with traditional bench-top sensors, confining this form of physiological monitoring of levodopa responses to controlled clinical settings. In addition to its hemodynamic effects, some studies have reported that levodopa induces alterations in short-term HRV measures (Sriranjini et al., 2011; Ruonala et al., 2015). Given the complexity of levodopa’s influence on both hemodynamic and autonomic function, wearable sensing technologies capable of continuously extracting markers of CVA function beyond HRV alone could enhance the characterization of levodopa’s effects and facilitate measurements in a broader range of environments.
Outside of monitoring motor symptoms in parkinsonisms (Patel et al., 2009; Ossig et al., 2016; Heijmans et al., 2019; Kamo et al., 2024), the use of wearable technology in
To address these gaps, we propose the use of a multimodal chest patch that enables the monitoring of markers closely related to CVA function, such as HRV, systolic timing intervals, and vascular reactivity. The wearable patch measures raw physiological waveforms including electrocardiogram (ECG), seismocardiogram (SCG) and photoplethysmogram (PPG) signals (Chan et al., 2021) from which several indices of CVA can be extracted on a beat-by-beat basis. The pre-ejection period (PEP) and left ventricular ejection time (LVET), both of which can be derived from the combination of ECG and SCG signals, are strongly associated with changes in beta-adrenergic sympathetic activity, cardiac contractility, and, consequently, stroke volume (Lewis et al., 1977; Kelsey, 2012). Notably, SCG has not been used, to our knowledge, to characterize CVA responses to levodopa in individuals with parkinsonism, despite its demonstrated efficacy in other contexts (Inan et al., 2015). We hypothesize that sensing both cardiomechanical and vascular activity, measured via PPG signals, will provide a more comprehensive understanding of levodopa’s hypotensive effects and provide early indications suggesting their underlying mechanisms.
The aim of this study is to assess the feasibility of using wearable multimodal sensing to monitor levodopa-induced changes in CVA function and to investigate the potential of wearable-derived physiological markers for capturing these effects. The conceptual design of this work is illustrated in Figure 1. We collected data with a wearable chest patch from n = 14 participants with PD (n = 11) and MSA (n = 3) during a clinical protocol, where the participants received their typical dosage of dopaminergic medication, and during a 24-h period at home. We examined the patch’s markers of CVA function before (OFF) and after levodopa administration (ON), for the first time, in both clinic and at-home settings. We additionally explored how the magnitude of the CVA responses captured by the patch were related to the administered dosage, and how they differed between participants with OH from those without OH. As the use of wearables for CVA monitoring in parkinsonian populations has been scarce, this work advances the state of wearable research and provides complementary insight into the acute CVA effects of levodopa, providing a framework to further explore these findings.
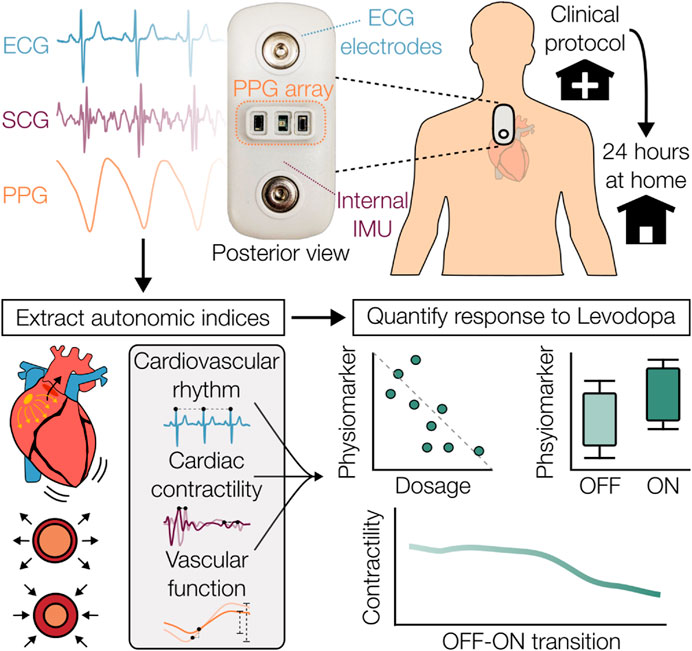
Figure 1. Overview of wearable sensing system and aims of this study. A wearable chest patch that measures electrocardiogram (ECG), seismocardiogram (SCG), and photoplethysmogram (PPG) signals was used in a population of Parkinson’s disease and multiple system atrophy during a clinical protocol and 24-h ambulatory study at home. Physiological markers related to cardiovascular autonomic function were extracted from the patch’s signals and were used to characterize the response to levodopa.
2 Materials and methods
2.1 Study protocol
The experimental protocol, summarized in Figure 2, was conducted under approval by the institutional review boards of both the Georgia Institute of Technology (#H21492) and Emory University School of Medicine (#00003055). Participants with mild to moderate idiopathic PD (Hoehn and Yahr, stages 1–3 (Hoehn and Yahr, 1967)) or the parkinsonian variant of MSA were enrolled. Participants with a medical history of cardiac diseases, arrhythmias, or other neurologic diseases beyond PD or MSA were excluded.
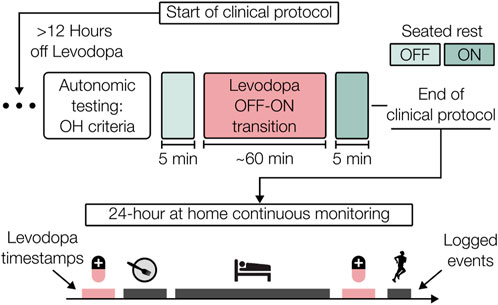
Figure 2. Clinical and at-home protocol overview. After a 12-h washout period, participants underwent autonomic testing followed by administration of their typical oral levodopa medication. The OFF-ON response was measured and then a 24-h period of continuous at-home monitoring was carried out. Participant diaries were used to provide event timestamps, including administration of medications.
The protocol began early in the morning after a minimum of 12 h abstinence from dopaminergic medications, vasoactive medications, caffeine, and other neurodepressants and neurostimulants, including medications to combat OH, to mitigate the potential cardiovascular influences of these substances. OFF-state motor symptom severity was examined via Part 3 motor sub-scale of the Movement Disorders Society Unified Parkinson’s Disease Rating Scale (MDS-UPDRS III) (Goetz et al., 2008) by a movement disorders trained neurologist. After the wearable patch and additional sensors were attached, data were acquired from periods of quiet rest and during autonomic function testing. An active standing challenge was performed to identify OH according to the census criteria, which specifies a sustained drop of at least 20 mmHg systolic BP (SBP) or 10 mmHg diastolic BP (DBP) within 3 min of upright posture (Freeman et al., 2011). Reference BP values were acquired continuously by either a ccNexfin system (Edwards Lifesciences, Irvine, CA, USA) or a CNAP monitor (CNSystems, Austria), with a VS 9 brachial cuffed BP monitor (Mindray, Shenzhen, China) to confirm the presence of OH.
Following the completion of these maneuvers in the OFF-medication state, the participant’s typical levodopa equivalent dosage (LED) was administered orally. Sensors other than the wearable patch were disconnected and a battery of symptom related questionnaires and assessments were completed. After approximately 60 min had passed, a time imposed to allow for the dopaminergic medication to take full effect (Noack et al., 2014), motor symptoms were reassessed (ON-state).
Participants were then given the wearable patch to wear for the next 24 h with an autonomic diary to log events relevant to autonomic function such as meals, administration of medications, sleep, and symptoms suggestive of OH (e.g., dizziness, light-headedness). Participants were instructed to carry out their normal activities of daily living and partake of their normal medications while wearing the patch. The patch was removed for brief periods to replace the ECG electrodes, if needed, or while bathing. Upon return of the wearable patch by the participant, waveform data were extracted from the onboard secure digital (SD) card and the logged autonomic diary events were converted to digital timestamps to contextualize the data.
2.2 Wearable multimodal sensor
A multimodal wearable patch, shown in Figure 1, was attached to the mid-sternum, roughly 2 cm below the suprasternal notch, to capture physiological waveforms pertinent to CVA activity. The patch was adhered to the chest with a pair of standard 3M-2670 Ag/AgCl gel electrodes (3M, St. Paul, MN, USA). This device acquires single-lead ECG, two sets of multiwavelength—infrared (IR), red, and green—PPGs, and triaxial acceleration from which the SCG is derived. The ECG, PPG, and SCG signals are sampled at 500, 67, and 1,000 Hz, respectively. The wearable patch’s hardware remains unchanged from prior studies (Chan et al., 2021; Berkebile et al., 2023). To briefly summarize: the ECG signal is measured from the ADS1292 (Texas Instruments, Dallas, TX, USA) analog front-end (AFE), the ADXL355 (Analog Devices, Norwood, MA, United States) digital low-noise accelerometer captures the triaxial SCG signal, and a combination of the Maxim 86,170 (Maxim Integrated, San Jose, California, United States) AFE, SFH 7016 (OSRAM, Munich, Germany) light emitting diodes, and VEMD 8080 (Vishay Semiconductors, Heilbronn, Baden Württemberg, Germany) photodiodes enable the extraction of reflectance-based PPG. Similar versions of this hardware have been deployed and validated in studies investigating heart failure (Inan et al., 2018), stroke volume monitoring (Ganti et al., 2022), and stress in participants with myocardial infarction (Nawar et al., 2023).
2.3 Segmenting OFF and ON periods
In accordance with prior work (Sriranjini et al., 2011; Noack et al., 2014; Ruonala et al., 2015), we analyzed periods prior to levodopa administration (OFF) and an hour after taking levodopa (ON) to assess its effects on CVA function. Raw waveforms measured by the wearable patch during 5-min periods of seated rest were extracted from both OFF and ON states during the clinical protocol. The separation between these two periods was as close to 60 min as possible, but was dependent on participant behavior, e.g., bathroom break or delayed completion of assessments. We also analyzed at-home levodopa administrations to gain insight into CVA responses outside of controlled clinical environments. Levodopa administration times were logged in the participant diaries during the 24-h at-home portion of the study. These times were referenced alongside the wearable patch’s accelerometer to identify periods of inactivity similar to those employed in the clinical portion (Godfrey et al., 2011). 5-min periods were selected as close as possible to the administration time and 60 min following administration. To ensure consistency, these periods were further constrained to ensure that posture was uniform across the OFF and ON periods, with minimal activity during both the 5-min interval and immediately preceding the period.
2.4 Signal processing and heartbeat segmentation
The raw wearable patch signals acquired during the OFF and ON states were preprocessed in Python (3.10.14) unless otherwise noted. The signal processing and physiological marker (physiomarker) extraction pipeline is shown in Figure 3 and largely follows that of our prior work (Berkebile et al., 2025). Only the dorsoventral (DV) component of the SCG and the IR wavelength PPG were employed in this work to capture cardiomechanical and vascular activity, respectively. Firstly, the ECG, SCG, and PPG waveforms were uniformly resampled to 500 Hz. The SCG and PPG signals were bandpass filtered to 1–40 Hz and 1–8 Hz, respectively. The ECG was passed through multiple filter banks as three R-peak detectors were used from the Neurokit2 (NK) toolbox: the NK method, Kalidas (Kal) method, and Martinez (Mar) method (Makowski et al., 2021; Kalidas and Tamil, 2017; Martinez et al., 2004). The final set of R-peaks was obtained by fusing the three individual R-peak sets, with the NK method serving as the reference. Only the R-peaks that multiple detectors successfully located within a lenient tolerance of 150 ms were reserved for further use. This fusion improved the reliability of R-peak detection for participants where individual detectors were less effective on the single-lead ECG waveform. The fused set of R-peaks formed R-R intervals which were filtered based on physiological implausibility for the given conditions (outside of 40–180 bpm) and flagged for outliers. Outliers were designated using sliding windows (30-beat, 90% overlap), flagging values
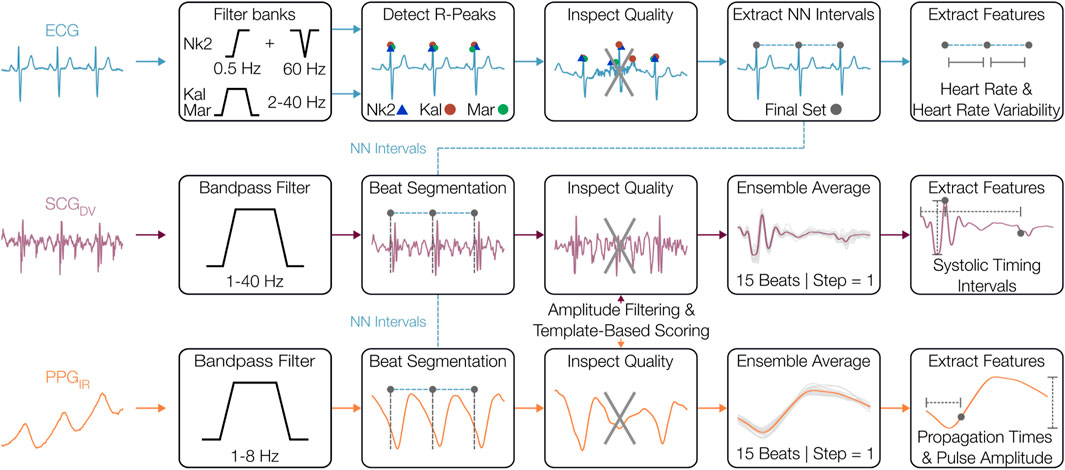
Figure 3. Signal preprocessing and physiomarker extraction. The electrocardiogram (ECG), seismocardiogram (SCG), and photoplethysmogram (PPG) signals were initially filtered to their respective frequency bands where cardiovascular information is most prominent. From the ECG, R-peaks were located and used to extract normal-normal (NN) intervals, from which the other signals were segmented on a beat-by-beat basis. The quality of these beats was assessed using template distance metrics and amplitude outlier removal. The SCG and PPG beats were then ensemble-averaged before features were extracted from each heartbeat.
The filtered SCG and PPG waveforms were segmented into heartbeats using the R-peaks with a fixed duration of 600 ms, which captures the salient systolic cardiac events and simplifies the signal processing (Shandhi et al., 2021). These SCG and PPG beats underwent signal quality assessment including amplitude-based outlier removal and local morphological similarity scoring. First, outlier SCG and PPG beats were removed using both global and sliding windows (30 beats, step = 1) or beat amplitudes, with beats flagged if the amplitude was
2.5 Physiomarker extraction
After the preprocessing stage, physiological markers (physiomarkers) related to CVA function were extracted from known landmarks found in ECG, SCG, and PPG beats. For ECG-related features, an HR time-series was computed from NN intervals and was further used in the computation of several time and frequency indices of HRV using the NK library. HRV parameters were computed from 5-min windows. After replacing missing values in the NN interval time-series with piecewise cubic Hermite interpolating polynomial (pchip) interpolation (Morelli et al., 2019; Benchekroun et al., 2023), time-domain parameters including root mean square of successive differences (RMSSD) and standard deviation of NN intervals (SDNN) were computed. The NN intervals were first resampled to 8 Hz before computing frequency metrics (Clifford and Tarassenko, 2005; Singh et al., 2004). Using NK’s implementation of the Welch method, the power in the LF band [0.04–0.15 Hz] and HF band [0.15–0.4 Hz] were derived, as well as the LF/HF ratio. The length of the analysis window was computed following the NK default implementation, which ensures at least two cycles of the lowest detectable frequency (0.013 Hz) are captured in each analysis window. An overlap of 50% was used to improve the robustness of spectral estimates. These HRV parameters were selected as some of the most commonly used to quantify levodopa responses in relevant work (Sriranjini et al., 2011).
Physiomarkers related to cardiac contractility and vasomotor reactivity were obtained from the SCG and PPG signals. PEP and HR-corrected LVET (LVETi) values, as well as their ratio PEP/LVETi, were derived from the aortic valve opening (AO) and the aortic valve closing (AC) fiducial points identified on the SCG (Lewis et al., 1977; Zia et al., 2019). Due to intersubject variability and longitudinal differences in SCG morphologies, multiple prominent peaks and valleys of the SCG waveform that strongly covary were tracked as candidate fiducial points. The median of these candidates was taken to mitigate errors in the PEP and LVET estimates that could occur in single candidate tracking. Note that these PEP and LVET estimates may be slightly offset from the true PEP and LVET, due to the complexity of SCG morphology, but reliably track longitudinal changes in these timings (Lin et al., 2021). The maximum and minimum points in the AO complex were used to compute the SCG amplitude (
2.6 Statistical analysis of levodopa responses
The wearable-based features extracted during the 5-min seated periods in both OFF and ON states were averaged for each subject and then grouped by medication state for analysis. The features were subsequently compared to identify any levodopa-induced change in resting physiology, akin to comparisons made in (Noack et al., 2014). While acknowledging the pathophysiological differences between PD and MSA regarding the origins of autonomic failure (Iodice et al., 2011), patients with PD and MSA were analyzed together given that levodopa-induced worsening of orthostatic hypotension is a commonly observed effect in both conditions (Coon and Ahlskog, 2021). The comparisons included all 13 wearable patch features. Prior to comparisons between groups, the groups were tested for normality with the Shapiro-Wilk test and equality of variance via Levene’s test. If either normality or equal variance was rejected, a two-sided Wilcoxon signed-rank test was performed; otherwise, a two-sided paired t-test was performed. Cohen’s effect size (d) for paired comparisons was computed. Separately, the physiomarkers acquired in the OFF-ON levodopa transitions detected at home required linear mixed effect models to account for repeated measurements, as most participants took levodopa multiple times over the 24-h monitoring period. The effect of state (OFF vs. ON) was examined on each physiomarker, with random intercepts fit for each participant.
Due to participants’ typical LED being taken, we investigated the relationship of the magnitude of physiomarker changes from OFF to ON with the LED that was administered. These correlations used Spearman’s
The potential hypotensive effects of levodopa are of primary interest in people with
In alignment with the aims of this study, which is focused on whether levodopa affects the patch’s multimodal physiomarkers in addition to the commonly employed HRV markers, Benjamini–Hochberg corrections were applied to control the false discovery rate across related physiological parameters (Benjamini and Hochberg, 1995). Specifically, HR and HRV parameters were grouped together, representing the most studied markers of autonomic regulation. Meanwhile, the cardiomechanical and vasomotor-related physiomarkers from SCG and PPG signals were analyzed as a separate group. P values are accordingly corrected for each set of analyses. Given the exploratory nature of this study, we report both adjusted (
3 Results
3.1 Dataset characteristics
Wearable sensor data were collected from n = 14 participants, 11 with PD and 3 with MSA, for the clinical portion. OH was confirmed through autonomic testing in n = 7 participants, 2 with MSA and 5 with PD. The clinicodemographics, motor symptom assessments, and levodopa dosages are detailed further in Table 1. Average supine, seated, and standing heart rates and BPs, measured during the autonomic testing are reported in Supplementary Table I. Motor testing in the ON-state revealed significant reductions in the MDS-UPDRS III score (p
3.2 Levodopa-induced changes in cardiovascular autonomic physiomarkers
Several seated physiomarkers differed significantly between the OFF and ON states measured in clinic, as depicted by the boxplots in Figure 4A. Specifically, significant increases in SCG-derived PEP (
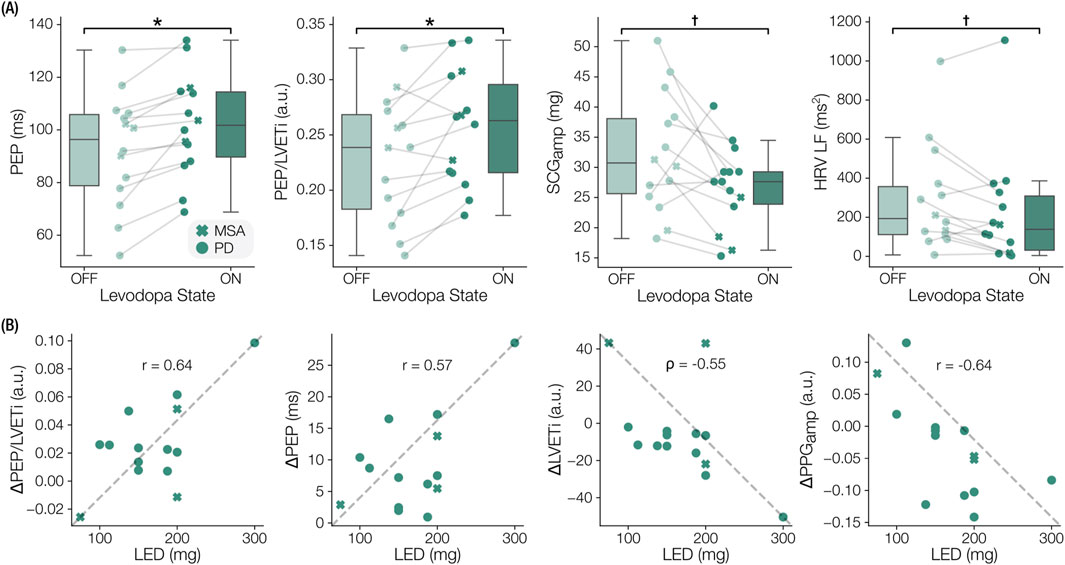
Figure 4. Notable levodopa-induced physiomarker changes and dose-response correlations. (A) Boxplots indicating significant physiomarkers changes from prior to levodopa (OFF) to an hour after (ON). Pre-ejection period (PEP), the ratio of PEP to left ventricular ejection time index (PEP/LVETi), seismocardiogram amplitude (
3.3 Relationship of physiomarker changes to levodopa dosage
We observed that the magnitudes of some physiomarker responses were associated with the LED administered as part of the clinical protocol. SCG-based features such as the change in PEP (p = 0.031, r = 0.573), LVETi (p = 0.042,
3.4 Levodopa response differences between OH and no-OH groups
In participants with OH, the in-clinic RMSSD of NN intervals was significantly reduced (
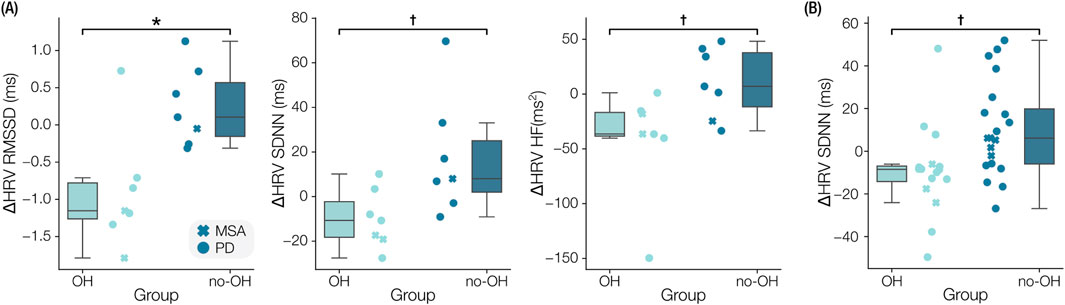
Figure 5. Comparison of levodopa responses between participants with orthostatic hypotension (OH) and without OH (no-OH). (A) Boxplots of the clinical levodopa responses are shown for the root-mean-square-of-successive-differences (RMSSD), standard deviation of normal-normal intervals (SDNN), and high frequency of heart rate variability (HRV-HF). (B) Boxplot of the difference in SDNN measured in the at-home responses. *
3.5 Case study: physiomarker time-series during adverse levodopa response
A participant with PD and OH experienced an adverse reaction to levodopa prior to completion of the clinical protocol. Symptoms of nausea precipitated a marked fall in BP (SBP/DBP: 73/44 mmHg) roughly 80 min after levodopa administration. The participant recovered by lying supine until BP increased to 168/74 mmHg and the feelings of nausea were reduced. Time-series of exemplary physiomarkers from onset of nausea, about 40 min following administration of 300 mg LED, to the resolution of symptoms are shown in Figure 6. The physiomarkers depict the underlying hemodynamic changes throughout the symptomatic period and subsequent recovery, thus illustrating the sensitivity of the measured waveforms to CVA dysfunction in an individual participant.
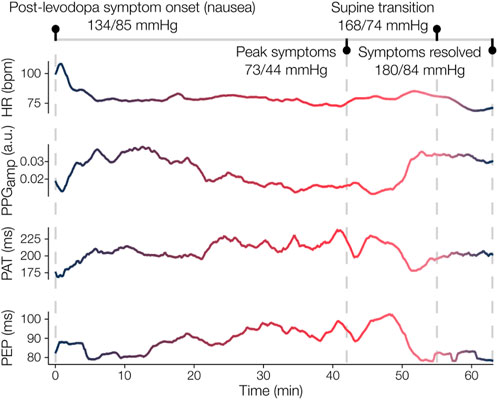
Figure 6. Case study of adverse levodopa reaction in participant with PD and OH. The participant initially experienced nausea about 40 min after taking levodopa, which worsened over the next 40 min. During this time, a drop in blood pressure (BP) from 134/85 mmHg (systolic/diastolic) to 73/44 mmHg was recorded. Concurrently, the wearable patch measured an increase in pulse arrival time (PAT) and pre-ejection period (PEP), which similarly indicates decreased arterial stiffness and decreased cardiac contractility given the stable heart rate (HR). After lying supine, the participant’s BP eventually surged (180/84 mmHg) and their symptoms dissipated. The marked responses in PAT and PEP, as well as the increase in photoplethysmogram amplitude (
4 Discussion
4.1 Principal findings
In this work, we demonstrate a novel wearable-based approach for evaluating the CVA dynamics associated with levodopa in patients with parkinsonism. Notably, this study is the first to employ a small form-factor chest patch that captures multiple physiological paradigms—namely the electrical and cardiomechanical activity of the heart alongside vascular reactivity—to extract meaningful measures of CVA state related to the effects of levodopa. We found that markers of cardiac contractility obtained from SCG waveforms were sensitive to the administration of levodopa and depicted a potential negative inotropic effect. We further explored the OFF-ON transitions and observed relationships between physiomarker changes and levodopa dosages as well as differences in HRV responses between participants with and without OH. Finally, we illustrated the utility of continuous monitoring via the wearable patch by capturing levodopa responses in an uncontrolled, at-home environment where the CVA effects of levodopa appeared subtler. The presented results demonstrate the feasibility of wearable sensing for improved monitoring of CVA function as well as grant further insight into the influence of levodopa on CVA health in patients with parkinsonism.
The multimodal sensing approach employed in this study enabled a more comprehensive assessment of CVA function than was captured in several prior studies examining the effects of levodopa, which often measure HRV alone (Sriranjini et al., 2011; Ruonala et al., 2015). The extracted physiomarkers themselves proved to be sensitive to the levodopa-induced changes in CVA state, primarily in the clinical data, during both periods of rest (Figures 4, 5) and the exemplary symptomatic period (Figure 6). In addition to a richer set of physiomarkers, the form factor of the wearable patch and acquisition of ECG, SCG, and PPG signals also facilitated remote continuous monitoring of ambulatory hemodynamic responses to levodopa, which have not been previously reported. The physiomarkers relying on cross-modal information, specifically the systolic timing interval measures, were key to characterizing CVA changes. Further work on coupling the presented physiomarkers with the accelerometer’s measures, which provide activity and postural context (Godfrey et al., 2011) and crude indices of motor symptoms in parkinsonism (Thorp et al., 2018), could lead to a more holistic solution for monitoring aspects of both non-motor and motor effects of levodopa.
Our results provide further support for the previously reported negative inotropic effect associated with levodopa in patients with
Whereas the levodopa evoked notable changes in physiomarkers measured in the clinic, there were no similar findings in the at-home data outside of the reduced RMSSD in the OH group compared to the no-OH group. Several factors could be influencing this finding. Given the at least 12-h washout period preceding the clinical protocol, the CVA effects of levodopa measured in clinic may have been exaggerated compared to typical administrations. Potential habituation to levodopa may have contributed to diminished autonomic responses measured at home, where levodopa was taken more regularly by participants. Inaccuracies in the patient-reported administration times could have introduced differences in the timing of the OFF-ON periods as defined for the in-clinic portion. Though the analyzed OFF-ON periods at home were selected similarly to the clinical periods, i.e., same posture with minimal activity, the lack of control in the at-home environment likely introduced more noise to the physiomarker extraction pipeline, thereby reducing the comparability between the clinical and home data. Finally, the effects of levodopa could have been obscured due to participants with OH taking other pressor agents concurrent with levodopa in order to reduce its potential hypotensive side effects. As this is the first study, to the best of our knowledge, to characterize at-home CVA responses to levodopa in this manner, future studies are warranted to validate these findings and address potential confounding factors, such as concurrent medication use, variable dosing schedules, and the influence of environmental and activity-related noise on the measurement of wearable physiomarkers.
Many studies examining the CVA effects of levodopa administer a fixed LED for all participants (Sriranjini et al., 2011; Noack et al., 2014). As the participants in this study took their typical LED, we were able to explore several significant, before correction, correlations between the magnitude of the physiomarkers changes and the participant’s LED. These correlations suggest that greater doses elicit larger changes in SCG-based systolic timing intervals, trending towards the reduced contractile state as discussed earlier. The relationship to
In analyzing how levodopa responses vary between people with and without CVA dysfunction, specifically OH, we found that HRV parameters responded distinctly between groups. A significant reduction in RMSSD was accompanied by significant, before correction, reductions in SDNN and HRV HF in the OH group relative to the no-OH group following levodopa administration in the clinic. The at-home data exhibited similar magnitudes of HRV changes in the OH and no-OH groups compared to the clinical data, with reduced HRV in the OH group relative to the no-OH group. The HRV reduction following levodopa in the OH group may indicate an exacerbation of baroreflex dysfunction (Earl et al., 2024), which results in impaired heart rate and peripheral vasoconstriction responses (Kaufmann and Palma, 2017). These results, though exploratory, suggest that levodopa may further impair autonomic regulation mostly in patients with known or latent CVA dysfunction (Cani et al., 2024), highlighting the need for improved monitoring of CVA function when initiating or escalating an individual’s dose of LED.
4.2 Limitations
This study has several limitations that should be considered when interpreting the results. Firstly, the number of participants and inclusion of multiple pathologies (PD and MSA) constrains the inferences made in this work. Specifically, CVA effects of levodopa are more commonly examined in PD and not MSA (Sénard et al., 1995; Sriranjini et al., 2011; Noack et al., 2014). Indeed, peripheral cardiac sympathetic denervation is near universal in PD (Goldstein, 2003), as opposed to severe central autonomic neurodegeneration in MSA, which could impact cardiac contractility findings here and in prior work (Noack et al., 2014). Yet, comparisons of PEP, PEP/LVET, and LVET were significant participants with PD alone Additionally, the complexity of the statistical modeling was limited by the number of subjects. This limitation may have masked subtler effects of levodopa on CVA function particularly for ambulatory analyses. Furthermore, there was no direct comparison with gold-standard measurements of cardiomechanical activity; however, the observed levodopa-induced changes in CVA function align with prior findings using controlled protocols and bench-top equipment (Noack et al., 2014; Ruonala et al., 2015; Wolf et al., 2006). Additionally, the ambulatory portion of this study was completely uncontrolled, which introduces variability to the analyses of levodopa responses. While the participant diaries provided some insight, a participant’s autonomic state may have been influenced by other activities, such as eating, or mental state (e.g., stress). The repeatability of at-home responses could be better assessed in future work with a semi-controlled measure at home whereby a participant performs a pre-and-post levodopa supine-to-stand or sit-to-stand test.
Future work should aim to better understand the clinical implications and mechanisms of the observed effects of levodopa, both in the lab and at home. In larger populations, exploring additional factors and interactions might unveil, or refute, further relationships between levodopa, CVA dysfunction, concomitant pressor agents, and parkinsonian state. Specifically, assessing the interaction between levodopa state (OFF/ON) and orthostatic responses with the wearable patch could provide insight into levodopa’s influences on cardiomechanical activity during postural changes. The integration of wearable sensing and clinical decision-making is promising but remains unproven in individuals with
Data availability statement
The raw data supporting the conclusions of this article will be made available by the authors, without undue reservation.
Ethics statement
The studies involving humans were approved by Emory University Institutional Review Board. The studies were conducted in accordance with the local legislation and institutional requirements. The participants provided their written informed consent to participate in this study.
Author contributions
JB: Conceptualization, Data curation, Formal Analysis, Investigation, Methodology, Resources, Software, Validation, Visualization, Writing – original draft, Writing – review and editing. OI: Conceptualization, Funding acquisition, Methodology, Project administration, Resources, Supervision, Validation, Writing – review and editing. PB: Conceptualization, Data curation, Funding acquisition, Investigation, Methodology, Project administration, Resources, Supervision, Validation, Writing – review and editing.
Funding
The author(s) declare that financial support was received for the research and/or publication of this article. This work was supported by McCamish Parkinson’s Disease Innovation Program at Georgia Tech and the American Parkinson Disease Association (APDA) Center for Advanced Research in Parkinson’s Disease at Emory University.
Acknowledgments
The authors thank J. Seppa and B. Kern of the Emory Brain Health Center for their role in recruitment.
Conflict of interest
OI is a co-founder and has significant financial interest in Cardiosense, Inc., a start-up that developed the wearable patch sensor used in this study; OI is also Chief Scientific Officer of Biozen, LLC, a start-up developing cuffless blood pressure monitoring technologies.
The remaining authors declare that the research was conducted in the absence of any commercial or financial relationships that could be construed as a potential conflict of interest.
Generative AI statement
The author(s) declare that no Generative AI was used in the creation of this manuscript.
Publisher’s note
All claims expressed in this article are solely those of the authors and do not necessarily represent those of their affiliated organizations, or those of the publisher, the editors and the reviewers. Any product that may be evaluated in this article, or claim that may be made by its manufacturer, is not guaranteed or endorsed by the publisher.
Supplementary material
The Supplementary Material for this article can be found online at: https://www.frontiersin.org/articles/10.3389/fnetp.2025.1543838/full#supplementary-material
References
Ahmed, S. S., Levinson, G. E., Schwartz, C. J., and Ettinger, P. O. (1972). Systolic time intervals as measures of the contractile state of the left ventricular myocardium in man. Circulation 46, 559–571. doi:10.1161/01.CIR.46.3.559
Arasteh, E., Mirian, M. S., Verchere, W. D., Surathi, P., Nene, D., Allahdadian, S., et al. (2023). An individualized multi-modal approach for detection of medication “off” episodes in Parkinson’s disease via wearable sensors. J. Personalized Med. 13, 265. doi:10.3390/jpm13020265
Barrachina-Fernández, M., Valenzuela-López, L., Moreno-Verdú, M., Sánchez-Cuesta, F. J., González-Zamorano, Y., Romero, J. P., et al. (2023). Advanced analysis of electrodermal activity measures to detect the onset of on state in Parkinson’s disease. Mathematics 11, 4822. doi:10.3390/math11234822
Benchekroun, M., Chevallier, B., Zalc, V., Istrate, D., Lenne, D., and Vera, N. (2023). The impact of missing data on heart rate variability features: a comparative study of interpolation methods for ambulatory health monitoring. IRBM 44, 100776. doi:10.1016/j.irbm.2023.100776
Benjamini, Y., and Hochberg, Y. (1995). Controlling the false discovery rate: a practical and powerful approach to multiple testing. J. R. Stat. Soc. Ser. B Methodol. 57, 289–300. doi:10.1111/j.2517-6161.1995.tb02031.x
Berkebile, J. A., Gazi, A. H., Chan, M., Albarran, T. D., Rozell, C. J., Inan, O. T., et al. (2025). Remote monitoring of cardiovascular autonomic dysfunction in synucleinopathies with a wearable chest patch. IEEE Sensors J. 25, 7250–7262. doi:10.1109/JSEN.2024.3523849
Berkebile, J. A., Inan, O. T., and Beach, P. A. (2023). “Evaluating orthostatic responses with wearable chest-based photoplethysmography in patients with Parkinson’s disease,” in 2023 ieee sensors, 1–4. doi:10.1109/SENSORS56945.2023.10324998
Bouhaddi, M., Vuillier, F., Fortrat, J. O., Cappelle, S., Henriet, M. T., Rumbach, L., et al. (2004). Impaired cardiovascular autonomic control in newly and long-term-treated patients with Parkinson’s disease: involvement of l-dopa therapy. Aut. Neurosci. 116, 30–38. doi:10.1016/j.autneu.2004.06.009
Calne, D. B., Brennan, J., Spiers, A. S. D., and Stern, G. M. (1970). Hypotension caused by L-dopa. Br. Med. J. 1, 474–475. doi:10.1136/bmj.1.5694.474
Cani, I., Guaraldi, P., Giannini, G., Sambati, L., Barletta, G., Cortelli, P., et al. (2024). Levodopa-induced orthostatic hypotension in parkinsonism: a red flag of autonomic failure. Eur. J. Neurology 31, e16061. doi:10.1111/ene.16061
Chan, M., Ganti, V. G., Heller, J. A., Abdallah, C. A., Etemadi, M., and Inan, O. T. (2021). Enabling continuous wearable reflectance pulse oximetry at the sternum. Biosensors 11, 521. doi:10.3390/BIOS11120521
Clifford, G., and Tarassenko, L. (2005). Quantifying errors in spectral estimates of HRV due to beat replacement and resampling. IEEE Trans. Biomed. Eng. 52, 630–638. doi:10.1109/TBME.2005.844028
Coon, E. A., and Ahlskog, J. E. (2021). My treatment approach to multiple system atrophy. Mayo Clin. Proc. 96, 708–719. doi:10.1016/j.mayocp.2020.10.005
Devos, D., Kroumova, M., Bordet, R., Vodougnon, H., Guieu, J. D., Libersa, C., et al. (2003). Heart rate variability and Parkinson’s disease severity. J. Neural Transm. 110, 997–1011. doi:10.1007/s00702-003-0016-8
Earl, T., Jridi, A., Thulin, P. C., Zorn, M., McKee, K. E., Mitrovich, K., et al. (2024). Effect of levodopa on postural blood pressure changes in Parkinson disease: a randomized crossover study. Clin. Auton. Res. 34, 117–124. doi:10.1007/s10286-024-01024-5
Freeman, R. (2008). Clinical practice. Neurogenic orthostatic hypotension. N. Engl. J. Med. 358, 615–624. doi:10.1056/nejmcp074189
Freeman, R., Wieling, W., Axelrod, F. B., Benditt, D. G., Benarroch, E., Biaggioni, I., et al. (2011). Consensus statement on the definition of orthostatic hypotension, neurally mediated syncope and the postural tachycardia syndrome. Clin. Auton. Res. 21, 69–72. doi:10.1007/s10286-011-0119-5
Ganti, V. G., Gazi, A. H., An, S., Srivatsa, A. V., Nevius, B. N., Nichols, C. J., et al. (2022). Wearable seismocardiography-based assessment of stroke volume in congenital heart disease. J. Am. Heart Assoc. 11, e026067. doi:10.1161/JAHA.122.026067
Gazi, A. H., Sundararaj, S., Harrison, A. B., Gurel, N. Z., Wittbrodt, M. T., Alkhalaf, M., et al. (2021). “Transcutaneous cervical vagus nerve stimulation inhibits the reciprocal of the pulse transit time’s responses to traumatic stress in posttraumatic stress disorder,” in 2021 43rd annual international conference of the IEEE engineering in medicine and biology society (EMBC), 1444–1447. doi:10.1109/EMBC46164.2021.9630415
Giorgino, T. (2009). Computing and visualizing dynamic time warping alignments in R: the dtw package. J. Stat. Softw. 31, 1–24. doi:10.18637/JSS.V031.I07
Godfrey, A., Bourke, A. K., Ólaighin, G. M., van de Ven, P., and Nelson, J. (2011). Activity classification using a single chest mounted tri-axial accelerometer. Med. Eng. and Phys. 33, 1127–1135. doi:10.1016/j.medengphy.2011.05.002
Goetz, C. G., Tilley, B. C., Shaftman, S. R., Stebbins, G. T., Fahn, S., Martinez-Martin, P., et al. (2008). Movement disorder society-sponsored revision of the unified Parkinson’s disease rating scale (MDS-UPDRS): scale presentation and clinimetric testing results. Mov. Disord. 23, 2129–2170. doi:10.1002/mds.22340
Goldberg, L. I., and Whitsett, T. L. (1971). Cardiovascular effects of levodopa. Clin. Pharmacol. and Ther. 12, 376–382. doi:10.1002/cpt1971122part2376
Goldstein, D. S. (2003). Dysautonomia in Parkinson’s disease: neurocardiological abnormalities. Lancet. Neurol. 2, 669–676. doi:10.1016/s1474-4422(03)00555-6
Goldstein, D. S., Eldadah, B. A., Holmes, C., Pechnik, S., Moak, J., Saleem, A., et al. (2005). Neurocirculatory abnormalities in Parkinson disease with orthostatic hypotension: independence from levodopa treatment. Hypertension 46, 1333–1339. doi:10.1161/01.HYP.0000188052.69549.e4
Heijmans, M., Habets, J. G., Herff, C., Aarts, J., Stevens, A., Kuijf, M. L., et al. (2019). Monitoring Parkinson’s disease symptoms during daily life: a feasibility study. npj Parkinson’s Dis. 5, 21–26. doi:10.1038/s41531-019-0093-5
Hemon, M. C., and Phillips, J. P. (2016). Comparison of foot finding methods for deriving instantaneous pulse rates from photoplethysmographic signals. J. Clin. Monit. Comput. 30, 157–168. doi:10.1007/s10877-015-9695-6
Hoehn, M. M., and Yahr, M. D. (1967). Parkinsonism: onset, progression, and mortality. Neurology 17, 427–442. doi:10.1212/wnl.17.5.427
Inan, O. T., Baran Pouyan, M., Javaid, A. Q., Dowling, S., Etemadi, M., Dorier, A., et al. (2018). Novel wearable seismocardiography and machine learning algorithms can assess clinical status of heart failure patients. Circ. Heart Fail. 11, e004313. doi:10.1161/CIRCHEARTFAILURE.117.004313
Inan, O. T., Migeotte, P. F., Park, K. S., Etemadi, M., Tavakolian, K., Casanella, R., et al. (2015). Ballistocardiography and seismocardiography: a review of recent advances. IEEE J. Biomed. Health Inf. 19, 1414–1427. doi:10.1109/JBHI.2014.2361732
Iodice, V., Low, D. A., Vichayanrat, E., and Mathias, C. J. (2011). Cardiovascular autonomic dysfunction in MSA and Parkinson’s disease: similarities and differences. J. Neurological Sci. 310, 133–138. doi:10.1016/j.jns.2011.07.014
Jain, S., and Goldstein, D. S. (2012). Cardiovascular dysautonomia in Parkinson disease: from pathophysiology to pathogenesis. Neurobiol. Dis. 46, 572–580. doi:10.1016/j.nbd.2011.10.025
Kalidas, V., and Tamil, L. (2017). “Real-time QRS detector using stationary wavelet transform for automated ECG analysis,” in 2017 IEEE 17th international conference on bioinformatics and bioengineering (BIBE), 457–461. doi:10.1109/BIBE.2017.00-12
Kallio, M., Haapaniemi, T., Turkka, J., Suominen, K., Tolonen, U., Sotaniemi, K., et al. (2000). Heart rate variability in patients with untreated Parkinson’s disease. Eur. J. Neurology 7, 667–672. doi:10.1046/j.1468-1331.2000.00127.x
Kamo, H., Oyama, G., Yamasaki, Y., Nagayama, T., Nawashiro, R., and Hattori, N. (2024). A proof of concept: digital diary using 24-hour monitoring using wearable device for patients with Parkinson’s disease in nursing homes. Front. Neurol. 15, 1356042. doi:10.3389/fneur.2024.1356042
Kaufmann, H., and Palma, J.-A. (2017). Neurogenic orthostatic hypotension: the very basics. Clin. Auton. Res. 27, 39–43. doi:10.1007/s10286-017-0437-3
Kelsey, R. M. (2012). “Beta-adrenergic cardiovascular reactivity and adaptation to stress: the cardiac pre-ejection period as an index of effort,” in How motivation affects cardiovascular response: mechanisms and applications (Washington, DC, US: American Psychological Association), 43–60. doi:10.1037/13090-002
Kujawa, K., Leurgans, S., Raman, R., Blasucci, L., and Goetz, C. C. G. C. C. G. (2000). Acute orthostatic hypotension when starting dopamine agonists in Parkinson’s disease. Archives Neurology 57, 1461–1463. doi:10.1001/archneur.57.10.1461
Lewis, R. P., Rittgers, S. E., Forester, W. F., and Boudoulas, H. (1977). A critical review of the systolic time intervals. Circulation 56, 146–158. doi:10.1161/01.CIR.56.2.146
Li, Q., and Clifford, G. D. (2012). Dynamic time warping and machine learning for signal quality assessment of pulsatile signals. Physiol. Meas. 33, 1491–1501. doi:10.1088/0967-3334/33/9/1491
Lin, D. J., Kimball, J. P., Zia, J. S., Ganti, V. G., and Inan, O. T. (2021). Reducing the impact of external vibrations on fiducial point detection in seismocardiogram signals. IEEE Trans. Biomed. Eng. 69, 176–185. doi:10.1109/TBME.2021.3090376
Liu, Z., Su, D., Zhou, J., Wang, X., Wang, Z., Yang, Y., et al. (2023). Acute effect of levodopa on orthostatic hypotension and its association with motor responsiveness in Parkinson’s disease: results of acute levodopa challenge test. Park. and Relat. Disord. 115, 105860. doi:10.1016/j.parkreldis.2023.105860
Ludwig, J., Remien, P., Guballa, C., Binder, A., Binder, S., Schattschneider, J., et al. (2007). Effects of subthalamic nucleus stimulation and levodopa on the autonomic nervous system in Parkinson’s disease. J. Neurology, Neurosurg. and Psychiatry 78, 742–745. doi:10.1136/jnnp.2006.103739
Makowski, D., Pham, T., Lau, Z. J., Brammer, J. C., Lespinasse, F., Pham, H., et al. (2021). NeuroKit2: a Python toolbox for neurophysiological signal processing. Behav. Res. 53, 1689–1696. doi:10.3758/s13428-020-01516-y
Martinez, J., Almeida, R., Olmos, S., Rocha, A., and Laguna, P. (2004). A wavelet-based ECG delineator: evaluation on standard databases. IEEE Trans. Biomed. Eng. 51, 570–581. doi:10.1109/TBME.2003.821031
Mesec, A., Šega, S., and Kiauta, T. (1993). The influence of the type, duration, severity and levodopa treatment of Parkinson’s disease on cardiovascular autonomic responses. Clin. Aut. Res. 3, 339–344. doi:10.1007/BF01827336
Morelli, D., Rossi, A., Cairo, M., and Clifton, D. A. (2019). Analysis of the impact of interpolation methods of missing RR-intervals caused by motion artifacts on HRV features estimations. Sensors 19, 3163. doi:10.3390/s19143163
Mukkamala, R., Hahn, J. O., Inan, O. T., Mestha, L. K., Kim, C. S., Toreyin, H., et al. (2015). Toward ubiquitous blood pressure monitoring via pulse transit time: theory and practice. IEEE Trans. Biomed. Eng. 62, 1879–1901. doi:10.1109/TBME.2015.2441951
Nawar, A., Gazi, A. H., Chan, M., Sanchez-Perez, J. A., Rahman, F. N., Ziegler, C., et al. (2023). “Towards quantifying stress in patients with a history of myocardial infarction: validating ECG-derived patch features,” in 2023 45th annual international conference of the IEEE engineering in medicine and biology society (EMBC), 1–4. doi:10.1109/EMBC40787.2023.10340614
Noack, C., Schroeder, C., Heusser, K., and Lipp, A. (2014). Cardiovascular effects of levodopa in Parkinson’s disease. Park. and Relat. Disord. 20, 815–818. doi:10.1016/j.parkreldis.2014.04.007
Olanow, C. W., Agid, Y., Mizuno, Y., Albanese, A., Bonucelli, U., Damier, P., et al. (2004). Levodopa in the treatment of Parkinson’s disease: current controversies. Mov. Disord. 19, 997–1005. doi:10.1002/mds.20243
Ossig, C., Antonini, A., Buhmann, C., Classen, J., Csoti, I., Falkenburger, B., et al. (2016). Wearable sensor-based objective assessment of motor symptoms in Parkinson’s disease. J. Neural Transm. 123, 57–64. doi:10.1007/s00702-015-1439-8
Patel, S., Lorincz, K., Hughes, R., Huggins, N., Growdon, J., Standaert, D., et al. (2009). Monitoring motor fluctuations in patients with Parkinson’s disease using wearable sensors. IEEE Trans. Inf. Technol. Biomed. 13, 864–873. doi:10.1109/TITB.2009.2033471
Posada-Quintero, H. F., Florian, J. P., Orjuela-Cañón, Á. D., and Chon, K. H. (2016). Highly sensitive index of sympathetic activity based on time-frequency spectral analysis of electrodermal activity. Am. J. Physiol. Regul. Integr. Comp. Physiol. 311, R582–R591. doi:10.1152/ajpregu.00180.2016
Rajfer, S. I., Anton, A. H., Rossen, J. D., and Goldberg, L. I. (1984). Beneficial hemodynamic effects of oral levodopa in heart failure. Relation to the generation of dopamine. N. Engl. J. Med. 310, 1357–1362. doi:10.1056/NEJM198405243102105
Reisner, A., Shaltis, P. A., McCombie, D., and Asada, H. H. (2008). Utility of the photoplethysmogram in circulatory monitoring. Anesthesiology 108, 950–958. doi:10.1097/ALN.0b013e31816c89e1
Ruonala, V., Tarvainen, M. P., Karjalainen, P. A., Pekkonen, E., and Rissanen, S. M. (2015). “Autonomic nervous system response to L-dopa in patients with advanced Parkinson’s disease,” in 2015 37th annual international conference of the IEEE engineering in medicine and biology society (EMBC), 6162–6165. doi:10.1109/EMBC.2015.7319799
Senard, J.-M., Chamontin, B., Rascol, A., and Montastruc, J.-L. (1992). Ambulatory blood pressure in patients with Parkinson’s disease without and with orthostatic hypotension. Clin. Aut. Res. 2, 99–104. doi:10.1007/BF01819664
Sénard, J.-M., Verwaerde, P., Rascol, O., and Montastruc, J.-L. (1995). Effects of acute levodopa administration on blood pressure and heart variability in never treated parkinsonians. Hypertens. Res. 18, S175–S177. doi:10.1291/hypres.18.SupplementI_S175
Shandhi, M. M. H., Bartlett, W. H., Heller, J. A., Etemadi, M., Young, A., Plotz, T., et al. (2021). Estimation of instantaneous oxygen uptake during exercise and daily activities using a wearable cardio-electromechanical and environmental sensor. IEEE J. Biomed. Health Inf. 25, 634–646. doi:10.1109/JBHI.2020.3009903
Singh, D., Vinod, K., and Saxena, S. C. (2004). Sampling frequency of the RR interval time series for spectral analysis of heart rate variability. J. Med. Eng. and Technol. 28, 263–272. doi:10.1080/03091900410001662350
Sprenger, F., and Poewe, W. (2013). Management of motor and non-motor symptoms in Parkinson’s disease. CNS Drugs 27, 259–272. doi:10.1007/s40263-013-0053-2
Sriranjini, S. J., Ganesan, M., Datta, K., Pal, P. K., and Sathyaprabha, T. N. (2011). Effect of a single dose of standard levodopa on cardiac autonomic function in Parkinson’s disease. Neurol. India 59, 659–663. doi:10.4103/0028-3886.86536
Thorp, J. E., Adamczyk, P. G., Ploeg, H.-L., and Pickett, K. A. (2018). Monitoring motor symptoms during activities of daily living in individuals with Parkinson’s disease. Front. Neurol. 9, 1036. doi:10.3389/fneur.2018.01036
Whitsett, T. L., and Goldberg, L. I. (1972). Effects of levodopa on systolic preejection period, blood pressure, and heart rate during acute and chronic treatment of Parkinson’s disease. Circulation 45, 97–106. doi:10.1161/01.CIR.45.1.97
Wolf, J.-P., Bouhaddi, M., Louisy, F., Mikehiev, A., Mourot, L., Cappelle, S., et al. (2006). Side-effects of L-dopa on venous tone in Parkinson’s disease: a leg-weighing assessment. Clin. Sci. 110, 369–377. doi:10.1042/CS20050247
Yahr, M. D., Duvoisin, R. C., Schear, M. J., Barrett, R. E., and Hoehn, M. M. (1969). Treatment of parkinsonism with levodopa. Archives Neurology 21, 343–354. doi:10.1001/archneur.1969.00480160015001
Keywords: wearable sensing, Parkinson’s disease, autonomic dysfunction, cardiovascular monitoring, multiple system atrophy, parkinsonism, levodopa, ambulatory monitoring
Citation: Berkebile JA, Inan OT and Beach PA (2025) Wearable multimodal sensing for quantifying the cardiovascular autonomic effects of levodopa in parkinsonism. Front. Netw. Physiol. 5:1543838. doi: 10.3389/fnetp.2025.1543838
Received: 11 December 2024; Accepted: 10 April 2025;
Published: 24 April 2025.
Edited by:
Peter A. Tass, Stanford University, United StatesReviewed by:
Anna M. Bianchi, Polytechnic University of Milan, ItalyGuadalupe Dorantes-Méndez, Autonomous University of San Luis Potosí, Mexico
Copyright © 2025 Berkebile, Inan and Beach. This is an open-access article distributed under the terms of the Creative Commons Attribution License (CC BY). The use, distribution or reproduction in other forums is permitted, provided the original author(s) and the copyright owner(s) are credited and that the original publication in this journal is cited, in accordance with accepted academic practice. No use, distribution or reproduction is permitted which does not comply with these terms.
*Correspondence: John A. Berkebile, amFiZXJrZWJpbGVAZ2F0ZWNoLmVkdQ==