- 1Department of Natural Sciences and Environmental Health, University of South-Eastern Norway, Bø, Norway
- 2Department of Sports, Physical Education and Outdoor Studies, University of South-Eastern Norway, Bø, Norway
- 3Landslagslegen.no, Top Sports Medical Office, Tønsberg, Norway
- 4The Norwegian Biathlon Association, Oslo, Norway
- 5Department of Circulation and Medical Imaging, Norwegian University of Science and Technology, Trondheim, Norway
- 6Myworkout, Medical Rehabilitation Centre, Trondheim, Norway
The aim was to investigate the effect of training, sex, age and selected genes on physiological and performance variables and adaptations before, and during 6 months of training in well-trained cross-country skiers. National-level cross-country skiers were recruited for a 6 months observational study (pre – post 1 – post 2 test). All participants were tested in an outside double poling time trial (TTDP), maximal oxygen uptake in running (RUN-VO2max), peak oxygen uptake in double poling (DP-VO2peak), lactate threshold (LT) and oxygen cost of double poling (CDP), jump height and maximal strength (1RM) in half squat and pull-down. Blood samples were drawn to genetically screen the participants for the ACTN3 R577X, ACE I/D, PPARGC1A rs8192678, PPARG rs1801282, PPARA rs4253778, ACSL1 rs6552828, and IL6 rs1474347 polymorphisms. The skiers were instructed to train according to their own training programs and report all training in training diaries based on heart rate measures from May to October. 29 skiers completed all testing and registered their training sufficiently throughout the study period. At pre-test, significant sex and age differences were observed in TTDP (p < 0.01), DP-VO2peak (p < 0.01), CDP (p < 0.05), MAS (p < 0.01), LTv (p < 0.01), 1RM half squat (p < 0.01), and 1RM pull-down (p < 0.01). For sex, there was also a significant difference in RUN-VO2max (p < 0.01). No major differences were detected in physiological or performance variables based on genotypes. Total training volume ranged from 357.5 to 1056.8 min per week between participants, with a training intensity distribution of 90–5–5% in low-, moderate- and high-intensity training, respectively. Total training volume and ski-specific training increased significantly (p < 0.05) throughout the study period for the whole group, while the training intensity distribution was maintained. No physiological or performance variables improved during the 6 months of training for the whole group. No differences were observed in training progression or training adaptation between sexes or age-groups. In conclusion, sex and age affected physiological and performance variables, with only a minor impact from selected genes, at baseline. However, minor to no effect of sex, age, selected genes or the participants training were shown on training adaptations. Increased total training volume did not affect physiological and performance variables.
Introduction
Cross-country skiing is regarded as one of the most demanding aerobic endurance sports, where male and female athletes have displayed some of the highest maximal oxygen uptakes (VO2max) ever recorded (Sandbakk and Holmberg, 2017). VO2max, often measured in running (RUN-VO2max), is suggested as a main predictor for cross-country skiing and overall endurance performance (Pate and Kriska, 1984; Ingjer, 1991; di Prampero, 2003; Støren et al., 2013; Sandbakk and Holmberg, 2017; Sunde et al., 2019; Johansen et al., 2020). However, in cases where RUN-VO2max is relatively homogenous or held constant, differences in work economy (C) (Conley and Krahenbuhl, 1980; di Prampero, 2003) and/or maximal strength (Hoff et al., 2002; Støren et al., 2008; Sunde et al., 2010, 2019) are regarded as major contributors for differentiating performance in endurance athletes.
Although the main determining factors for cross-country skiing performance are relatively clear, the best way to develop these physiological factors over longer periods in every individual skier is still under investigation (Stöggl and Sperlich, 2015). Traditionally, endurance training makes up almost 90% of the total training for competitive cross-country skiers, while the rest is strength training and speed training (Losnegaard et al., 2013; Stöggl and Sperlich, 2015; Sandbakk et al., 2016). The endurance training during season preparation for both junior and senior cross-country skiers is characterized with high volumes of low-intensity training (LIT) and low to moderate volumes of moderate- (MIT) and high-intensity training (HIT). This has been regarded as an “optimal” intensity distribution for developing higher performance capacity in cross-country skiers (Ingjer, 1992; Seiler and Kjerland, 2006; Sandbakk et al., 2016; Solli et al., 2017). Stöggl and Sperlich (2014) suggests that a polarized training intensity distribution, with high LIT volumes (∼80%) and relatively high HIT volumes (∼20%) with low volumes of MIT, would be more beneficial for further improvements of well-trained endurance athletes, compared to training models with higher volumes of MIT. Additionally, higher volumes of HIT are considered as a more efficient way to elevate VO2max compared to LIT, both in well-trained to elite cross-country skiers and recreational skiers (Nilsson et al., 2004; Helgerud et al., 2007; Støren et al., 2012; Rønnestad et al., 2014, 2016; Stöggl and Sperlich, 2014; Johansen et al., 2020).
Ingjer (1992) observed that young cross-country skiers started to level-off in VO2max at age 19–20 following a training regime similar to that described above, at least in values relative to body mass. Following the same training pattern year after year has not proven to be an effective strategy to increase VO2max further in well-trained and elite adult cross-country skiers (Gaskill et al., 1999; Solli et al., 2017). In Gaskill et al. (1999) and Støren et al. (2012), major changes in the relative intensity distribution of the endurance training led to significant improvements in VO2max and performance in well-trained endurance athletes. However, a recent study showed substantial differences in training response to the same HIT protocol among well-trained cyclists (Bratland-Sanda et al., 2020). This points to the need for better individualization of training programs.
Earlier studies have mainly explored training characteristics in cross-country skiers retrospectively, with no opportunity to investigate the direct physiological effect of the athlete’s training. However, the study of Losnegaard et al. (2013) performed several tests through the preparation phase and the competitive season in elite male cross-country skiers competing at an international and national level. The study revealed improvements in skiing economy (V2 skating), O2-deficit and skating performance on a time trial on a roller-skiing treadmill. No improvements were observed in VO2max. These were the results of a traditional high volume LIT and low to moderate volume of MIT and HIT regime. However, mainly retrospective studies have been performed on sub-elite and junior cross-country skiers over longer time periods (>10 weeks). No studies have investigated training characteristics and the subsequent physiological effects in both sub-elite senior and junior cross-country skiers competing at a national and regional level over longer periods.
Sex differences in performance determining factors in cross-country skiing is generally reported to be between 10 and 30%, where greater sex differences are shown when the upper-body is used more extensively (Sandbakk et al., 2014; Hegge et al., 2016; Sunde et al., 2019). Sex differences have been examined in recent years among cross-country skiers, however, sex comparisons in training responses to a similar training regimen is not well examined in well-trained cross-country skiers. Previous investigations have revealed no difference in training responses between males and females following the same training program in both sedentary and well-trained individuals (Astorino et al., 2011; Støren et al., 2017; Varley-Campbell et al., 2018), suggesting that this may also be the case for well-trained cross-country skiers. Although both junior and senior skiers have been investigated separately (Ingjer, 1992; Sandbakk et al., 2010, 2016; Losnegaard et al., 2013), direct comparisons of training responses in these age-groups have not been executed previously in cross-country skiers. Investigations of both sex and age-related differences in training responses may be crucial to understand differences in training adaptations, and further improve the quality of the individualization of training programs.
The genetic component of sports performance and trainability has received increasing attention the last two decades. Sports performance is considered a complex trait, influenced by many genes. A number of single nucleotide polymorphisms (SNPs) have been associated with various aspects of athletic ability and sports performance. Two polymorphisms that have been intensely investigated are the ACTN3 R577X and ACE I/D (Jacques et al., 2019). The ACTN3 gene codes for α-actinin-3, a protein expressed in fast-twitch muscle fibers. The common R577X polymorphism leads to the deficiency of the protein in individuals with the XX genotype (North et al., 1999), which is the case for around 19% of Caucasians (Roth et al., 2008; Goleva-Fjellet et al., 2020). Lack of the α-actinin-3 has been associated with increased muscle endurance, and decreased maximal power generation (MacArthur et al., 2008). The ACE gene encodes the angiotensin I-converting enzyme, having a role in the regulation of blood pressure, fluid-electrolyte balance and affecting the muscle function (Puthucheary et al., 2011; Pescatello et al., 2019). ACE seems to play a role in exercise induced adaptations and the I allele has been regarded as the endurance allele (Ma et al., 2013; Pescatello et al., 2019). Few studies have investigated these polymorphisms in relation to cross-country skiing performance. Magi et al. (2016) found higher frequencies of the ACTN3 RR and ACE ID genotype in male skiers compared to controls. In addition, male skiers with XX genotype tended to exhibit greater increase in VO2peak over a 5-year period. The same finding applied to female skiers with the ID genotype. Orysiak et al. (2013), on the other hand, did not find any associations between the ACE I/D and VO2max in well trained winter sports athletes. No previous studies have compared the genotype distribution for selected genes between regional to national cross-country skiers and the normal population within the same region. Goleva-Fjellet et al. (2020) genotyped ACE and ACTN3 in a cohort representing the region of South East Norway, making it possible to compare this with an athletic cohort.
The PPARGC1A rs8192678 SNP has also gained attention in exercise genetics. The protein encoded by the gene, PGC1α (peroxisome proliferator-activated receptor gamma co-activator-1-alpha), induce the mitochondrial biogenesis and modulate the composition and functions of the mitochondria (Austin and St-Pierre, 2012). Recent reviews have concluded that the rs8192678 polymorphism is associated with aerobic trainability and sports performance (Petr et al., 2018, 2020; Tharabenjasin et al., 2019). Peroxisome proliferator-activated receptor genes, e.g., PPARG (rs1801282) and PPARA (rs4253778), have also been investigated in relation to trainability and athletic ability (Petr et al., 2018, 2020). According to Bouchard et al. (2011) the rs6552828 SNP of the acyl-CoA synthase long-chain member 1 gene (ACSL1) could explain around 6% of the training response of VO2max to standardized exercise training programs. A recent study by Harvey et al. (2020) reported that the rs1474347 polymorphisms in the interleukin-6 (IL6) gene was associated with training induced improvements in VO2max in both moderately and well trained participants.
To the best of our knowledge, no study have investigated effects of sex, age, training and selected genes on physiological and performance adaptations in the same study. Therefore, the primary aim of this study was to investigate training adaptations in physiological and performance variables in well-trained cross-country skiers after 6 months of training during season preparation (i.e., May to October). Secondly, we wanted to investigate possible differences between gender and age groups in baseline values and training adaptations during the study period. Thirdly, we wanted to investigate the effects of specific candidate genes on physiological and performance variables at baseline. We hypothesized that age and sex would influence on baseline values, but not training adaptations, and that differences in training would impact training adaptations. Further, we hypothesized that the distribution of the selected genetic variants would represent the distribution of the general population for this region and not impact physiological or performance values at baseline.
Materials and Methods
Experimental Approach
The main purpose of this study was to evaluate changes in physiological and performance variables after 6 months of training (May to October) in well-trained cross-country skiers. We also wanted to compare baseline values and training induced changes in males and females, and young and older skiers, as well as in skiers with different genotypes. Therefore, the participants were instructed to train according to their own training programs worked out by themselves or their coaches prior to the research project, and report their daily training for the whole 6 months period. They were tested for a number of physiological, strength and performance variables over 2 days at three occasions; before (PRE), mid-way (POST1) and after (POST2) the study period. The test battery consisted of measurements of RUN-VO2max, VO2peak in double poling (DP-VO2peak), time to exhaustion (TTE), oxygen cost of double poling (CDP), lactate threshold in double poling (LT), jump height, 1RM and maximal power tests in half squat and pull-down and performance in a 5.64 km double poling time trial (TTDP). At baseline, blood samples were drawn to assess gene status in selected genes.
Subjects
A total of 46 well-trained cross-country skiers (30 males and 16 females), differing in age (16–48 years) and performance-level, were recruited for the whole study. The study’s medical doctor approved all participants for participation. However, 17 skiers were excluded because they were not able to fulfill the requirements of three testing sessions during the study period due to sickness or injuries or did not report their training habits sufficiently. Thus, 29 skiers were included in the statistical analyzes. To investigate age-related effects the included skiers were divided in two age groups (16–18 and ≥19 years). These groups were defined as either in, or above puberty, and also corresponding to in, or above high-school age. The ≥19 group included skiers from 19 to 48 years. All subjects were recruited by invitation to high-schools for skiers in Southeastern Norway or regional cross country ski teams. The included skiers differed substantially in performance level, from medium-junior level to top national level. The best male and female skiers had finished top 10 in numerous VISMA ski classics races (i.e., Vasaloppet and Marcialonga) and/or top 30 in the Norwegian national championship, and the slowest skiers finished in the lower part of national junior competitions. Subjects‘ characteristics are summarized in Table 1.
The study was conducted in accordance with the Declaration of Helsinki, and evaluated and approved by the regional ethics committee of Southeast Norway (REK 2017/2522) and the institutional research board at the University of South-Eastern Norway (former University College of South-Eastern Norway). After having received information about the study, all participants gave their written informed consent before participation. Parental written consent was collected for skiers below 18 years.
Test Procedures
In order to evaluate changes in physiological and performance variables related to the skiers training, all participants were tested at three separate occasions. PRE were performed in April/May, POST1 were performed in July/August, and POST2 were conducted in October/November. All testing procedures were the same at all testing sessions.
All tests were performed on two consecutive days. The participants were instructed to do only light training the last 24 h before testing, and no food or nutritious drinks were allowed 1 h before the first test. In between tests, the participants were allowed to eat a light meal of energy-rich food and drinks. The last meal before testing and food intake in-between tests were registered, and all participants were asked to consume the same food in the subsequent testing sessions (POST1 and POST2). All preparation procedures were the same at all three testing sessions. The tests were also conducted at approximately the same time of day (±2 h) at PRE, POST1 and POST2 to avoid circadian differences.
The first day of testing consisted of three maximal jump height tests, an incremental running test for determining RUN-VO2max, and a TTDP. Before the jump tests, the participants performed a self-conducted warm-up procedure of at least 10 min. This warm-up was registered and repeated at POST1 and POST2. Then they performed three separate jump tests in the following order: squat jump (SJ), counter-movement jump (CMJ) and counter-movement jump with arm swing (CMJas). For the SJ tests, the knee-angle were 90° and this was controlled by the same test leader at all tests. No counter-movements were allowed in this particular test, whereas no counter-movement restrictions were given for the CMJ and CMJas tests. All participants were given at least three consecutive attempts in each jump-test, and the best attempt was registered as the result. At least 3 min of rest were given between the separate jump tests to ensure sufficient restitution. All jump-tests were performed by use of a force platform (Ergotest Innovation, Porsgrunn, Norway) for jump height measurements. The force platform was calibrated in accordance with the manufacturers’ manual before each test. Jump height was calculated by the following equation,
where h is jump height, v is the velocity at take-off, which again is based on calculation of force multiplied with time divided by mass, and g is gravitation (Ergotest Innovation, Porsgrunn, Norway).
After at least 20 min of rest, the participants started a 10 min self-conducted warm-up procedure before an incremental VO2max test in running. This warm-up was registered and repeated at POST1 and POST2. The RUN-VO2max test was conducted by the same procedures as presented in Sunde et al. (2019). Briefly, the participants started at an intensity of 6% inclination and 7–8 km⋅h–1 and 9–10 km⋅h–1 for female and male, respectively. The test started with 1% increase in inclination every 30 s until 8% was reached, whereas only speed was increased by 0.5 km⋅h–1 every 30 s after that. All participants were instructed to run to voluntary fatigue, and the three highest subsequent VO2 measurements were used to calculate VO2max. Heart rate (HR) ≥ 98% of HRmax, respiratory exchange ratio (RER) ≥1.05, blood lactate concentration ([La–]b) ≥ 8.0 mmol⋅L–1, rate of perceived exertion (Borg scale 6–20) ≥17, and flattening of the VO2 curve was used to evaluate if VO2max was reached. The metabolic test system, MetaLyzer II Cortex (Biophysic GmbH, Leipzig, Germany) was used for all VO2 measurements, with measurements every 10 s. Before testing the O2-analyzer were calibrated with ambient air and certified calibration gases (16% O2/4% CO2), while the flow sensors were calibrated with a 3-L calibration syringe (Biophysic GmbH, Leipzig, Germany) before each test. The treadmill used was a Woodway PPS 55 sport (Waukesha, WI, United States), calibrated for speed and incline. HR were registered by the participants own heart rate monitors or by Polar s610 HR monitors (Kempele, Finland).
After at least 1 h of rest, a 5.64 km TTDP test was performed in a paved roller ski course track of 940 m. The TT procedures have been previously presented in Sunde et al. (2019). Only the DP technique was allowed throughout the test. The TT was organized with individual starts, and 30 s starting intervals. Drafting was not allowed. The subjects used their own roller-skis for classic skiing and poles and were instructed to use wheel type 2 for the time trial test. All subjects used the same pair of roller skis at PRE, POST1, and POST2. Differences in temperature and humidity may influence the rolling resistance of the roller skis, and thus the results of this test. Therefore, we used the same procedures for calculating a correction factor described previously in Sunde et al. (2019).
The second day of testing consisted first of sub-maximal VO2 and [La–]b measurements in DP, in order to determine CDP and LT. This was, after 5 min of active recovery, followed by a ramp protocol to exhaustion to determine DP-VO2peak. After 1 h of rest, the second day of testing ended with two maximal strength tests in half-squat and pull-down.
The DP tests were performed on a motorized treadmill specialized for cross-country skiing (Rodby RL 2700E, Rodby Innovation, Vänge, Sweden). Every participant performed one 30-min workout for familiarization to the DP treadmill before testing, as previously used in Sunde et al. (2019). All participants used the same pair of roller skis at all DP tests during the study period (Swenor Fiberglass, Sarpsborg, Norway) with the same binding system (NNN, Rottefella, Klokkarstua, Norway). The subjects were allowed to use their own poles and additional skiing equipment, which was the same in all three test sessions. During treadmill testing, the participants were attached to a safety harness, connected to the roof, to avoid falling. Three to six 4-min work periods, with registration of VO2 and HR measurements the last minute, were conducted for calculating CDP at LT intensity and LT. Work periods were only separated by 1-min for measurements of [La–]b. Whole blood lactate values were measured by a Lactate Scout+ (SensLab GmbH, Leipzig, ray Inc., Kyoto, Japan). The subjects started the first work period at a work intensity assumed to be 50–70% of their DP-VO2peak. This corresponded to 10–11.5 km⋅h–1 and 4% inclination for males and 6–8 km⋅h–1 and 4% inclination for females. In the following work periods, the speed increased by 1–3 km⋅h–1, and the test terminated after [La–]b levels exceeding the subjects’ LT. Warm up lactate value (i.e., the lowest measured lactate value) + 2.3 mmol⋅L–1 were used to define LT. This is in accordance with the protocol from Helgerud et al. (1990) and described and discussed in detail in Støren et al. (2014) and Sunde et al. (2019).
After 5-min of active rest, the subjects performed the RAMP protocol to exhaustion for determining DP-VO2peak. The starting intensity was set to 6% inclination and 7 km⋅h–1 for both genders. The inclination was constant through the whole test, while speed increased by 1 km⋅h–1 every 60 s. All participants received motivational feedback throughout the test. The test terminated when the skiers slowly moved backward, despite intense motivational feedback, and reached a pre-defined mark 1 m behind the subjects starting position on the treadmill. TTE was registered and the DP-VO2peak was defined as the mean of the two highest subsequent VO2-measurements. Maximal aerobic speed (MAS) in double poling were calculated in the same way as presented in Sunde et al. (2019) and Johansen et al. (2020), i.e., DP-VO2peak/CDP.
A 60-min rest period were given prior to the tests of 1RM and maximal power output in half-squat (Smith-machine, PreCore, Woodinville, WA, United States) and pull-down (Gym 2000, Vikersund, Norway). Pilot testing in Støren et al. (2008) showed no deterioration in 1RM half-squat 30 min after maximal aerobic tests, thus we considered 60-min to be more than sufficient to give valid maximal strength results. The strength tests protocol is identical to the protocol used in Sunde et al. (2019). Both strength tests started with 10 reps at approximately 50% of 1RM. After this, the following sets were performed at approximately 60% (5 reps), 70% (3 reps), and 80% (2 reps), only separated by 3 min rest periods. All repetitions were performed with a slow eccentric phase with a complete stop of movement in the lowest position (half-squat) or the highest position (pull-down) of approximately 1 s. This was followed by a maximal mobilization in the concentric phase. The MuscleLab system (Ergotest Innovation, Porsgrunn, Norway) calculated power output by measurements of lifting time and distance of work. After the sub-maximal series, the participants performed at least 1 rep at their estimated 1RM. From there on: 1 rep, and load increments of 2.5–10 kg from the subsequent lift, were conducted until 1RM was reached.
Training Registration
The participants were instructed to train according to their own training plans worked out by themselves or by their coaches throughout the study period, without any influence or interventional instructions from the research personnel. All participants recorded training data in digital training diaries, i.e., in an online diary from the Norwegian Olympic Federation, or in Polar Flow. The athletes had all used digital training diaries for at least 1 year prior to the study. Every training session and competition was recorded and controlled by the same research personnel throughout the study period, and 3-months prior to PRE. The two training periods between PRE to POST1 and POST1 to POST2 were defined as 1st training period (P1) and 2nd training period (P2). In order to investigate potential changes in training inside P1 and P2, the periods have been further divided into a total of four periods where appropriate (P1A, P1B, P2A, and P2B).
All training data were systemized based on training modality and training intensity. Training modality was either endurance, strength, speed/jump or other, and activity was running, roller-skiing, cross-country skiing or cycling. Roller-skiing and cross-country skiing on snow were defined as ski-specific training, while running and cycling was defined as unspecific training. Endurance training intensity were monitored as HR “time in zone,” and categorized into three intensity zones: (1) low-intensity training (LIT; ≤81% of HRmax), (2) moderate-intensity training (MIT; 82–87% of HRmax), and (3) high-intensity training (HIT; ≥88% of HRmax). All endurance training and competitions were performed with the skiers’ personal heart rate monitors. This is in accordance with the procedures used in Støren et al. (2008) and Sunde et al. (2010).
Strength training consisted mainly of maximal strength training and/or general strength training. Maximal strength training was targeting large muscle groups, i.e., 1–6 repetitions in, i.e., half squat, pull-down or deadlift. General strength training was performed with 10–30 repetitions and with a main purpose of increase stability and general strength in the upper-body and trunk. The duration of strength training sessions where quantified as the time between the first set of the first exercise and last set of the last exercise, including rest periods between sets and exercises. Additional warm-up and cool-down were registered as LIT, while stretching where included in “other training.” Jump training (i.e., 1–6 box-jumps or jump exercises in stairs) was quantified in the same manner as strength training. Speed training during LIT- or MIT-sessions was mainly performed during ski-specific training. The number of sprints were multiplied by 1.5 min since the period after each sprint was performed at a very low intensity. The monitoring of strength-, speed-, and jump training is in accordance with the quantification procedures used in Sandbakk et al. (2016).
DNA Sampling and Genotyping
Venous blood was drawn when the participants first attended to the laboratory before the physiological testing procedures at the first testing session (April/May). The EDTA tubes were stored at −20°C. Before the DNA extraction, the samples were thawed at room temperature. DNeasy Blood & Tissue Kit (Qiagen, MD, United States) was used to extract the DNA from 100 μl of blood following the manufacturer’s instructions.
ACE I/D polymorphism, rs4343 polymorphism in the ACE gene was genotyped as it might be the best proxy to I/D polymorphism (Abdollahi et al., 2008), than analyzed to determine the I/D genotype. Genotyping for all polymorphisms was performed using TaqMan® SNP Genotyping Assay. Assay IDs were as follows: C__11942562_20 for ACE rs4343; C____590093_1 for ACTN3 R577X; C__30469648_10 for ACSL1 rs6552828; C___1643192_20 for the PPARGC1A rs8192678; C___1839698_20 for IL6 rs1474347; C___1129864_10 for PPARG rs1801282 and C___2985251_20 for PPARA rs4253778 polymorphism (Thermo Fisher Scientific, MA, United States). StepOnePlusTM Real-Time PCR System (Applied Biosystems®, CA, United States) was used to carry out the qPCR. Genotype calling was performed by StepOne Software v2.0. 15 μl of final reaction volume contained 8.44 μl Genotyping Master Mix, 0.42 μl Assay mix (40×), 6.33 μl double distilled H2O and ∼100 ng of DNA template. Cycling conditions were as follows: 30 s at 60°C was followed by initial denaturation step for 10 min at 95°C; then, 40 cycles of denaturation at 95°C for 15 s were followed by annealing at 60°C for 1 min in cycling stage, finishing with the final post-read step for 30 s at 60°C.
Statistical Analyzes
Normality tests and Q-Q plots were used to evaluate normal distribution for main variables (TTDP, RUN-VO2max and MAS). In all cases, a normal distribution was observed, thus parametric statistics were used. Values were expressed as mean ± SD, and inter-individual variability in training and physiological variables were expressed as coefficient of variance (CV). To evaluate potential changes in physiological response and training characteristics for the total group, within sexes and within age groups, a Univariate General Linear Model (GLM) test with Tukey Post Hoc-tests was used. To examine potential differences between sexes and age groups in physiological response and training characteristics during the study period, GLM Univariate with pairwise comparisons and independent sample t-tests were conducted. For correlations between baseline values, and between differences between different test points (delta correlations), correlation coefficients r was used from Pearson’s bivariate tests. Correlation coefficients were evaluated in accordance with Hopkins (2000), which are presented in detail previously (Sunde et al., 2019). Since the participants represented both female and male skiers, also partial correlations were conducted corrected for sex and age.
One-way ANOVA with Tukey Post Hoc-tests was used to assess the associations between the genotypes and physiological and performance variables at baseline. To assess the effects of the alleles on these variables, a two-tailed independent sample t-test was applied. In order to test for the Hardy-Weinberg equilibrium (HWE) for all polymorphisms and to compare the genotype frequencies to those of other studies, Pearson’s Chi-square test (χ2) was used. When analyzing effects of different genotypes on physiological parameters, all female values from the physiological tests were multiplied according to the average gender difference between males and females in the present study. This was conducted to avoid bias effects of different gender representation for the different candidate genes and genotypes. In order to promote comparability between candidate gene studies, effect size (Cohen’s d) was calculated using Microsoft® Excel® (Redmond, WA, United States) for the gender corrected variables across the genotypes (Supplementary Table 6). The effect size was interpreted as follows: below 0.50 – small effect, 0.5 and above – moderate effect, 0.8 and above – large effect (Cohen, 1988). As the participants were following individual training programs, genetic analyzes of trainability were not performed. For all statistical analyzes performed, the statistical package for social science version 26 (SPSS, IBM, Chicago, IL, United States) was used. A p value < 0.05 was accepted as statistically significant in all tests (two-tailed).
Power calculations prior to the study revealed that with a between-group difference in the selected physiological variables of 5%, and with a common standard deviation of the same size, a sample size of 12 to 16 subjects were needed in each age- and gender group in order accomplish a significant level of 0.05 and a power of 80%. Regarding the genetic variables, the material is under-powered in order to accomplish full genetic analyses. Multivariate ANOVA analyzes between the different genotypes and the different physiological variables were thus not performed. However, the material was still interesting in order to see if there were substantial differences in physiological variables related to single genes. Also, the material was sufficient to investigate if the cohort of skiers differentiated from a general population from the same geographical area in genotype and allele frequencies.
Results
Training Characteristics
The skiers training was registered for 23.4 ± 2.2 weeks from PRE to POST2. From PRE to POST1 the skiers trained for 12.7 ± 1.7 weeks, and for 10.7 ± 1.4 weeks from POST1 to POST2. In total, 8460 training sessions were registered, with 5957 inside the 6-months study period. The remaining sessions registered were conducted in the 3 months before PRE. This corresponded to an average of 205 ± 48 sessions per skier during the study period, and 292 ± 72 sessions per skier when the training period before PRE were included.
Training characteristics for the whole group in P1 and P2 are presented in Table 2, while the sub-periods (P1A, P1B, P2A, and P2B) are presented in Supplementary Table 3. The mean total training volume in P1 was 701.5 ± 169.8 min⋅week–1 and increased significantly to 753.2 ± 137.6 min⋅week–1 in P2 (p < 0.05). Total endurance training accounted for 86.9 ± 6.6 and 84.4 ± 7.1% of total training volume in P1 and P2, respectively. The relative intensity distribution in the endurance training was 90.0 ± 4.3, 4.8 ± 2.2, and 5.2 ± 3.0% in LIT, MIT, and HIT, respectively, in P1. In P2, LIT, MIT, and HIT represented 89.6 ± 3.2, 4.8 ± 2.2, and 5.7 ± 2.4%, respectively. The relative intensity distribution did not change significantly throughout the 6-months training period. Ski-specific training accounted for 49.7 ± 13.6 and 55.7 ± 10.5% of total endurance training in P1 and P2, respectively. Total ski-specific training and ski-specific LIT increased significantly from P1 to P2 (p < 0.01), while ski-specific MIT and HIT remained unchanged. In total, 65.2 ± 18.0 and 62.4 ± 17.7% of ski-specific training was performed as classic skiing, while the remaining 34.8 ± 17.3 and 37.6 ± 17.7% was performed as freestyle-skiing in P1 and P2, respectively. Most of the remaining volume of total endurance training were performed either as running (40.1 ± 9.8% in P1, 38.6 ± 9.0% in P2) or as cycling (9.9 ± 14.7% in P1, 5.6 ± 7.0% in P2).
Strength training was performed regularly with 1–3 sessions per week throughout the study period. In P1, strength training accounted for 8.8 ± 4.0% of the total training volume while in P2, 10.3 ± 3.8% of total training volume was strength training. The amount of strength training increased significantly from P1 to P2 (p < 0.01). Speed/jump and other training stayed unchanged throughout the whole training period while accounting for 1.2 ± 1.3 and 3.1 ± 4.8% in P1 and 1.3 ± 1.4 and 4.0 ± 4.1% in P2, respectively.
Physiological Adaptations
Results in physiological and performance variables at the three testing sessions (PRE, POST1, and POST2) are presented in Table 3. No significant changes were observed in physiological and performance variables in the whole group from PRE to POST1, from POST1 to POST2, except for RERRUN (p < 0.05), or PRE to POST2.
Correlations between physiological and performance variables at baseline and between delta values in physiological, performance and training variables is presented in Tables 4–6. Strong correlations were observed between TTDP and DP-VO2peak (r = −0.79, p < 0.01), MAS (r = −0.79, p < 0.01), LTv (r = −0.82, p < 0.01), RUN-VO2max (r = −0.68, p < 0.01), and 1RM pull-down (r = −0.64, p < 0.01) at baseline for the whole group. Corrected for gender, strong significant correlations were still apparent between TTDP and DP-VO2peak (r = −0.63, p < 0.01), MAS (r = −0.58, p < 0.01), and LTv (r = −0.64, p < 0.01) at baseline. Corrected for age-groups, the similar strong correlations as seen for the whole group were almost at same level between TTDP and RUN-VO2max (r = −0.68, p < 0.01), LTv (r = −0.77, p < 0.01), DP-VO2peak (r = −0.76, p < 0.01), MAS (r = −0.75, p < 0.01), and 1RM pull-down (r = −0.52, p < 0.01). A strong correlation was also apparent between MAS and LTv, both independent (r = 0.93, p < 0.01) and dependent (r = 0.85, p < 0.01 and r = 0.89, p < 0.01) of gender and age, respectively.
No delta correlations were observed between ΔTTDP and any delta values of the physiological or training variables (Tables 5, 6). ΔMAS revealed strong significant correlations to ΔLTv (r = 0.57, p < 0.01) and ΔCDP (r = −0.85, p < 0.01). Δski specific training and ΔLITski showed low significant correlations to ΔLTv (r = 0.48, p < 0.01 and r = 0.45, p < 0.05, respectively), while ΔLITski showed a low significant correlation to ΔCDP (r = −0.41, p < 0.05).
Sex Differences
Male skiers trained significantly higher volumes than females 3 months before pre-tests (p < 0.05). Additionally, no statistical difference was observed in LIT, MIT, HIT, total endurance training, ski-specific training, running, strength training or other training between males and females in either P1 or P2. Males trained significantly more cycling in P2 (p < 0.05), and females trained significantly higher volumes of speed/jump training, both in P1 (p < 0.01) and P2 (p < 0.05). No statistical difference was observed in training progression throughout the 6-months period between males and females, except for other training (p < 0.05). Differences in training characteristics between males and females are shown in Figures 1A,B and Supplementary Table 4.
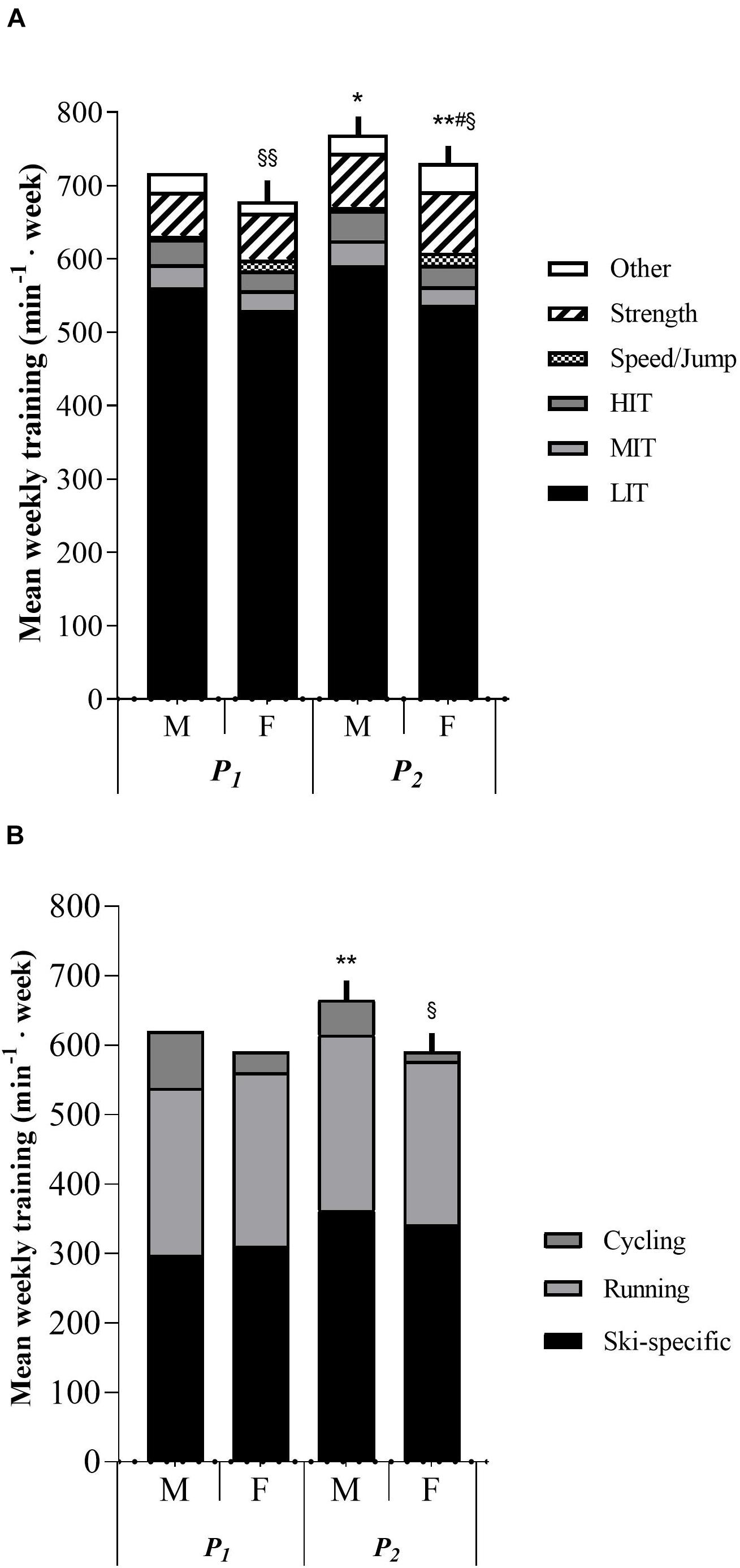
Figure 1. (A) Sex differences between males and females in training characteristics in minutes per week. M, males. F, females. P1, first training period from May to July. P2, second training period from August to October. LIT, low-intensity endurance training. MIT, moderate-intensity endurance training. HIT, high-intensity training. min– 1 week, minutes per week. *p < 0.05 significant difference from P1 in strength and other training. **p < 0.01 significant difference from P1 in strength training. #p < 0.05 significantly different in delta values in other training from male value. § p < 0.05 significantly different from male value in speed/jump training volume. §§ p < 0.01 significantly different from male value in speed/jump training volume. (B) Sex differences between males and females in endurance training mode in minutes per week. **p < 0.01 significantly different from P1 value in ski-specific training. § p < 0.05 significantly different from male value in cycling.
Significant sex differences in physiological and performance variables were observed at PRE-tests. Results from physiological and performance tests are presented in Supplementary Table 1. Males had on average 14.7% (p < 0.01, effect size = 2.28) better TTDP performance, 19.3% (p < 0.01, effect size = 1.91) higher RUN-VO2max (mL⋅kg–1⋅min–1), 19.3% (p < 0.01, effect size = 1.72) higher DP-VO2peak (mL⋅kg–1⋅min–1), 9.1% (p < 0.01, effect size = 0.97) better CDP (mL⋅kg–1⋅m), 30.2% (p < 0.01, effect size = 2.04) higher LTv and a 32.3% (p < 0.01, effect size = 2.16) higher MAS than females at baseline. In addition, males were 21.3% (p < 0.01, effect size = 1.22) and 30.5% (p < 0.01, effect size = 1.86) stronger than females in half squat and pull-down, respectively, and displayed 34.7% (p < 0.01, effect size = 1.37) and 45.9% (p < 0.01, effect size = 1.37) higher power values in half squat and pull-down, respectively. No significant gender differences were apparent in HR, RER, [La–]b or RPE in running or double-poling, %RUN-VO2max or LT%, at baseline (all effect sizes <0.7).
No sex differences were observed in physiological or performance adaptations from PRE to POST2, except for [La–]b in RUN-VO2max (p < 0.05, effect size = 0.93). From PRE to POST1, only RERRUN (p < 0.05, effect size = 0.88), CDP (p < 0.05, effect size = 0.77), and LT% (p < 0.05, effect size = 0.91) changed significantly different between males and females. However, no gender differences were observed in physiological or performance adaptations from POST1 to POST2. Training adaptations for males and females in key physiological variables are presented in Figure 2.
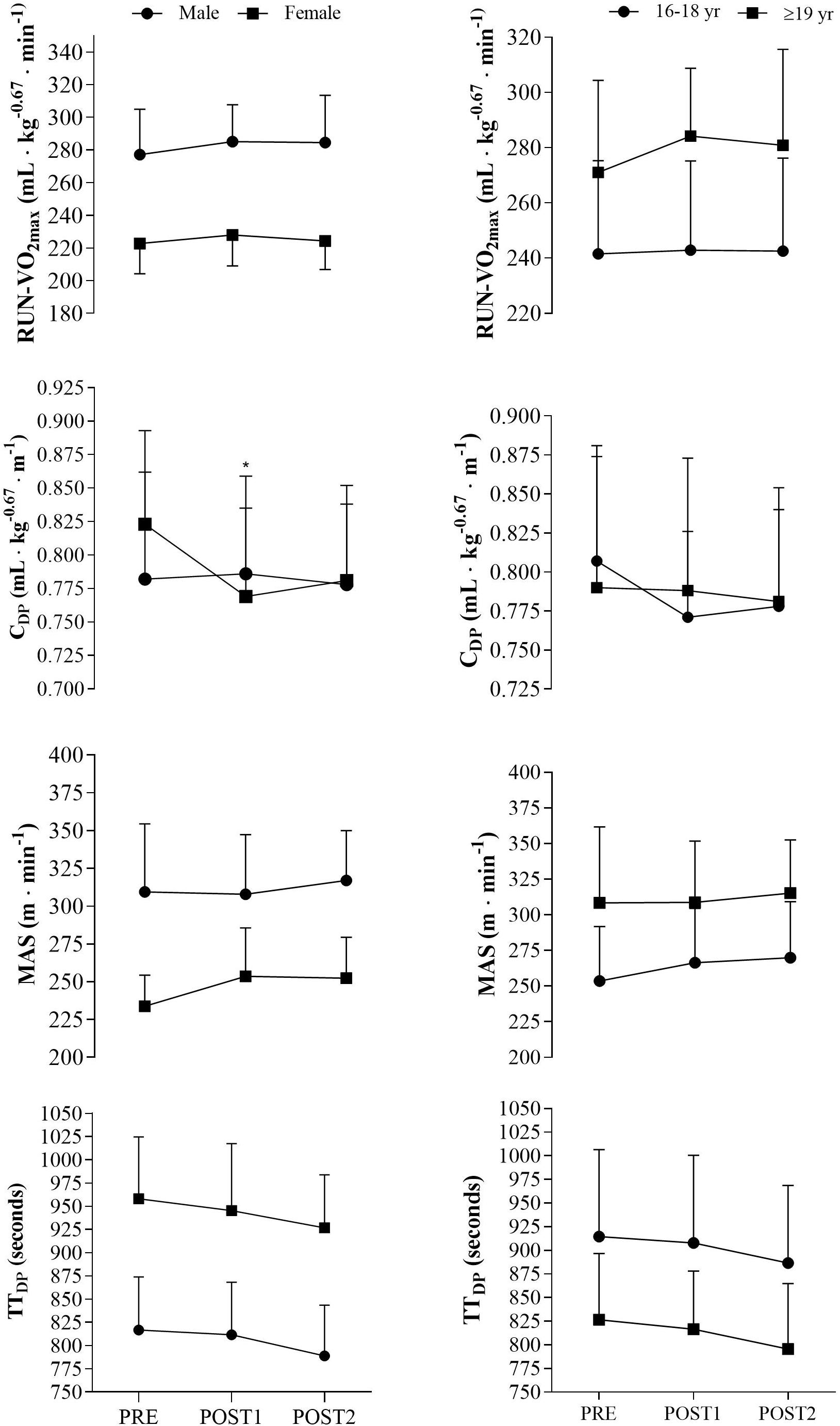
Figure 2. Sex and age differences in key physiological and performance variables. RUN-VO2max, maximal oxygen uptake in running. mL⋅kg– 0.67⋅min– 1, milliliters per kilogram raised to the power of –0.67 per minute. CDP, oxygen cost of double poling at lactate threshold. mL⋅kg– 0.67⋅m– 1, milliliters per kilogram raised to the power of –0.67 per meter. MAS, maximal aerobic speed. m⋅min– 1, meter per minute. TTDP, time trial performance in double poling. *p < 0.05 significant different from male delta value.
Age-Group Differences
No age differences were observed in total training volume 3-months before PRE. Total MIT volume was significantly higher in the ≥19 years group (p < 0.05) in both P1 and P2, while other training volume was significantly lower in the same group compared to the 16–18 years group. Speed/jump and strength training was significantly lower in the ≥19 years group in P2 (p < 0.05 and p < 0.01, respectively), while no difference was apparent in P1. No training differences between age groups were displayed in total training volume, LIT, HIT, ski-specific training, running, or cycling during the whole training period. No age group differences were observed in delta training values throughout the whole period. Training characteristics for the two age groups are presented in Figures 3A,B and Supplementary Table 5.
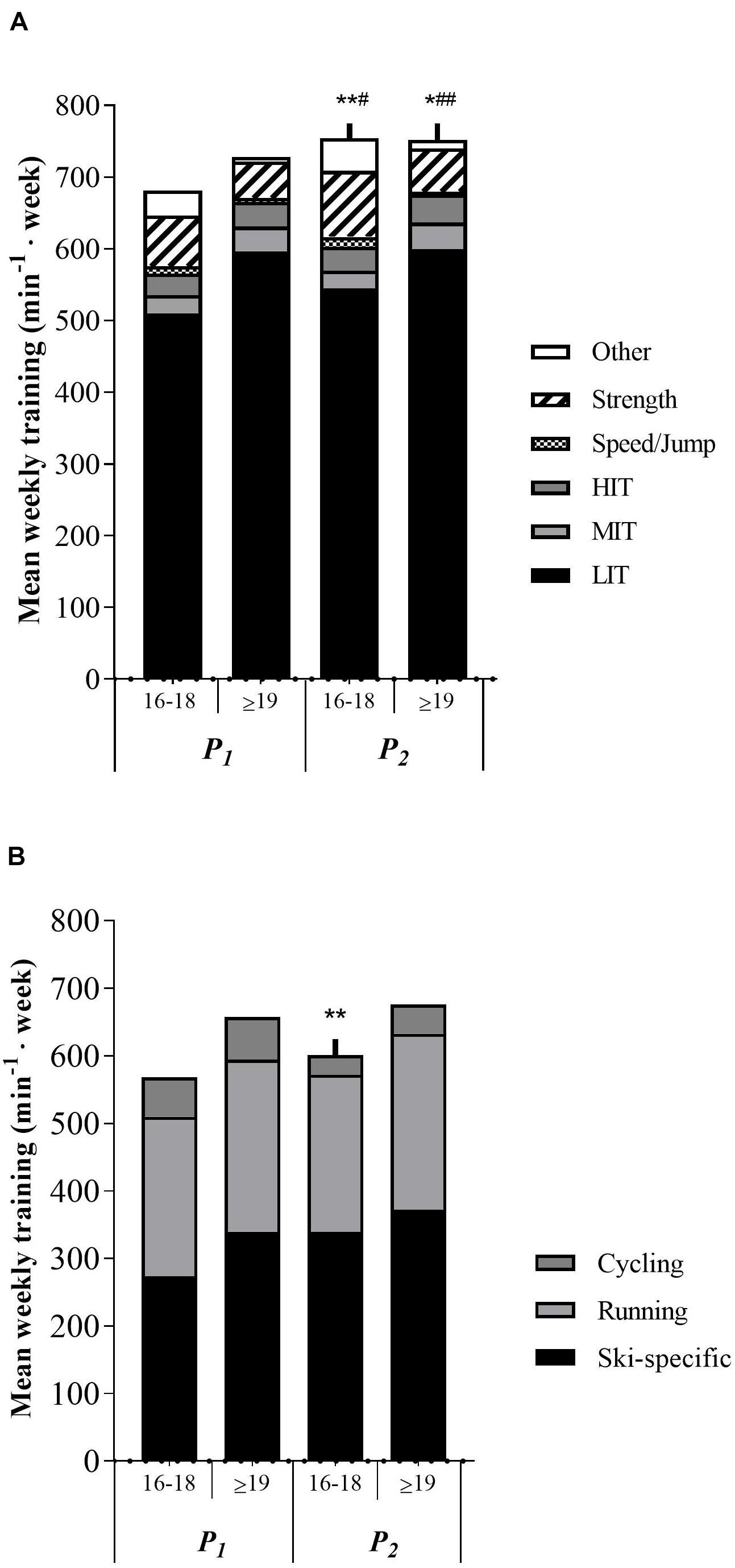
Figure 3. (A) Age differences in training characteristics in minutes per week. P1, first training period from May to July. P2, second training period from August to October. LIT, low-intensity endurance training. MIT, moderate-intensity endurance training. HIT, high-intensity training. min– 1 week, minutes per week. *p < 0.05 significantly different from P1 in strength training. **p < 0.01 significantly different from P1 in total training volume and strength training. #p < 0.05 significantly different from ≥19 years value in speed/jump training. ##p < 0.01 significantly different from 16 to 18 years value in strength and other training. (B) Age differences in endurance training mode in minutes per week. **p < 0.01 significantly different from P1 in ski-specific training.
Results from physiological and performance tests among age-groups are presented in Supplementary Table 2. At PRE the ≥19 years group had a 9.6% (p < 0.01) better TTDP performance, 12.0% (p < 0.05) higher DP-VO2peak (mL⋅kg–1⋅min–1), 7.3% (p < 0.05) better CDP, 23.4% (p < 0.01) higher LTv and 21.6% (p < 0.01) higher MAS than the younger skiers. In addition, the oldest skiers were significantly stronger in half squat (15.9%, p < 0.05) and pull-down (26.5%, p < 0.01), and had higher maximal power values both in half squat (35.1%, p < 0.01) and pull-down (41.5%, p < 0.01). No differences were observed between age groups in RUN-VO2max (mL⋅ kg–1 ⋅ min–1), RER, [La–]b, %RUN-VO2max or LT%.
No differences in delta values was observed between age groups from PRE to POST2, except for BW (p < 0.05, effect size = 0.94), [La–]b in running (p < 0.05, effect size = 0.92), LTv (p < 0.05, effect size = 0.87) and power in half squat (p < 0.05, effect size = 0.88). Additionally, differences in delta values were observed in RUN-VO2max (mL⋅kg–1⋅min–1, p < 0.05, effect size = 0.87) and power in half squat (p < 0.01, effect size = 1.22) from PRE to POST1. No differences were observed in physiological and performance adaptations from POST1 to POST2. Training adaptations in key variables for the two age-groups are presented in Figure 2.
Impact of the Selected Genes
All polymorphisms were successfully genotyped, and were at Hardy-Weinberg equilibrium (p > 0.05). Genotype frequencies are displayed in Table 7. Minor allele frequencies (MAF) for the genotyped polymorphisms were as follows: 53% for ACTN3, 41% for ACE and IL6, 40% for ACSL1, 38% for PPARGC1A, 19% for PPARA and 9% for PPARG polymorphism.
Key physiological and performance results among genotypes in ACTN3 and ACE at baseline is presented in Table 8. All genotype and allele data is presented in Supplementary Table 6. There were no differences in physiological and performance results between the three ACTN3 genotypes when analyzing the 29 included skiers. The same picture was shown when analyzing all successfully genotyped participants (n = 40), except for a significantly higher DP-VO2peak in the RX genotype compared to the RR genotype (Supplementary Table 8). When testing X allele carriers compared to the RR genotype, DP-VO2peak (mL⋅kg–1⋅min–1), both independent of- and corrected for gender, was, respectively, 12.4 and 8.8% higher (p < 0.05, effect sizes >0.80).
For the ACE I/D genotypes, individuals with the DD genotype displayed an 18.4% better CDP (mL⋅kg–0.67⋅m–1) compared to those with the II genotype (p < 0.01, effect size = 1.42). However, corrected for gender this difference was no longer significant. Individuals with DD genotype also displayed significantly higher values in 1RM pull-down from the II counterparts, both dependent (34%, p = 0.05, effect size = 1.67) and independent (23.4%, p < 0.05, effect size = 2.66) of gender (Table 8). Although the same picture was apparent, all significant associations disappeared when analyzing all 40 successfully genotyped participants (Supplementary Table 8). When comparing II genotypes to D carriers we detected a 9.2% higher RUN-VO2max (mL⋅kg–1⋅min–1), when corrected for gender (p < 0.05, effect size = 1.14). Overall, D allele carriers were 27.2% stronger than individuals with the II genotype (p < 0.05), and the association remained significant when corrected for gender (17.4%, p < 0.01, effect size = 1.27).
There were no significant associations between genotypes of the ACSL1 rs6552828 polymorphism and physiological variables. However, when corrected for gender, an 8.4% difference was observed in RUN-VO2max (mL⋅kg–1⋅min–1 and mL⋅kg–0.67⋅min–0.67; p < 0.05) between A allele carriers compared to the GG genotype. As to the PPARGC1A rs8192678, the highest RUN-VO2max (mL⋅kg–1⋅min–1, L⋅min–1 and mL⋅kg–0.67⋅min–1) was exhibited by the only individual with TT. Significant differences were observed in RUN-VO2max (p < 0.05), DP-VO2peak (p < 0.05) and 1RM pull-down (p < 0.05) between genotypes and allele carriers in PPARA rs4253778, IL6 rs1474347 and PPARG rs1801282 independent of gender. However, when corrected for gender, these differences disappeared.
Discussion
Main Findings
This is the first study to investigate effects of age, sex, selected genes and training on physiological and performance characteristics and adaptations in well-trained cross-country skiers. Throughout the 6 months period, the skiers displayed no differences in relative distribution of training intensity, although the total training volume and relative amount of ski-specific training increased. This led to no significant differences in physiological and performance variables. Neither was there observed any differences between groups in training adaptations throughout the 6 months training period. At baseline, the male skiers trained more than the female skiers did, but with approximately the same distribution regarding training modalities and intensity zones. We did not detect any major differences in physiological or performance variables based on genotypes.
Training Characteristics
The participants in the present study were all well-trained and competitive skiers, although not international elite athletes. All skiers competed at national events, and some skiers competed at Scandinavian or international long-distance events (i.e., Vasaloppet and Marcialonga). Thus, all athletes planned and performed their training with a goal of developing better performance capacity. Compared to training data from elite cross-country skiers (Losnegaard et al., 2013; Tønnessen et al., 2014; Sandbakk et al., 2016; Solli et al., 2017, 2018), most of the participants displayed lower training volumes. This may partly be explained by the fact that the skiers in the present study had to perform their daily training beside other work duties, studies or family obligations. The national level skiers in the study of Sandbakk et al. (2016) are thus more comparable to these participants. Secondly, approximately 50% of the skiers in the present study were still 16 to 18 years, and their total training volume was not statistically different from the older (≥19 years) skiers. This may be because the younger skiers were attending different skiing high schools, were training was an incorporated part of the school schedule. In previous studies, total training volume of younger skiers were lower than in older skiers (Sandbakk et al., 2010, 2013, 2016; Losnegaard et al., 2013; Solli et al., 2017).
The relative distribution of training intensity in the present study was in line with general recommendations for cross-country ski training (Sandbakk and Holmberg, 2017). Cross-country skiers are generally recommended to train with high amounts of LIT and low to moderate amounts of MIT and HIT during the preparation period (May to October). In the study of Sandbakk et al. (2016) national-class cross-country skiers performed on average 90% of their total endurance training at <81% of HRmax, 4% at 82–87% HRmax and 6% at 88% of HRmax or higher from May to October. The skiers in the present study displayed, respectively 90, 5, and 5% at the same intensity zones from May to July, showing almost exactly the same intensity distribution from August to October (88, 5, and 6%, respectively). However, different training quantification methods were used in the present study compared to Losnegaard et al. (2013) and Sandbakk et al. (2016). In the present study, training was registered as “time in zone,” meaning the exact time in each intensity zone regardless of how the training may have been planned. In Losnegaard et al. (2013) and Sandbakk et al. (2016), the session-goal approach was used, meaning that average HR during either continuous workouts or interval bouts is used to determine the intensity distribution during sessions. This makes the training data difficult to compare with the results in the present study, at least in the higher intensity zones. Sylta et al. (2014) has suggested a conversion factor of 1:3 for comparison of “time-in-zone” training data to session-goal training data for HIT training. By use of this factor on training data from Losnegaard et al. (2013) and Sandbakk et al. (2016), the HIT training should be only a third of what was reported. The study of Tønnessen et al. (2014) shows more comparable “time in zone” data for MIT and HIT in Olympic-medal winning skiers as the skiers in the present study. However, their study showed higher volumes of both HIT and total training volume compared to the present study.
In the present study, the skiers displayed almost no progression in training volume or relative distribution of training intensity during the 6 months period. These findings are in contrast to the study of Sandbakk et al. (2016), where the national level skiers showed a linear increase in training volume from May to September, generally due to higher volumes of LIT. However, the elite international skiers in the studies of Sandbakk et al. (2016) and Losnegaard et al. (2013) showed progression more similar to the skiers in the present study from May to October, but with an increase in HIT and a decrease in LIT during the competitive season, i.e., November to April. The latter may also be the case for the skiers in the present study, but training during the competitive season was not investigated here.
The skiers in the present study performed on average 50% of their total endurance training on ski-specific training, mainly roller skiing. The other half was devoted for the most part to running. These volumes and relative distribution of ski-specific vs. unspecific training are in close agreement with previous studies on cross-country skiers, both non-elite and elite (Losnegaard et al., 2013; Tønnessen et al., 2014; Sandbakk et al., 2016; Solli et al., 2017). Ski-specific training may target the ski-specific aerobic capacity, work economy and technical factors better than general endurance training (Johansen et al., 2020). The skiers added approximately 60 min per week of ski-specific training from P1 to P2, while running was held relatively constant throughout the whole training period. This may be a result of a desire to elevate ski-specific capacities (i.e., work economy or technical factors) closer to the competitive season, in line with training characteristics from Losnegaard et al. (2013) and Sandbakk et al. (2016).
The relative distribution of strength and speed/jump training was comparable to the training volumes observed previously (Losnegaard et al., 2013; Tønnessen et al., 2014). This is in line with the increased focus on the upper- and lower body strength and speed in modern cross-country skiing (Sandbakk and Holmberg, 2017; Sunde et al., 2019).
Training Adaptations
The 6 months of training from May to October led to no significant improvements in physiological and performance variables for the skiers in the present study. Since the training volume and intensity distribution was almost constant throughout the study period, this was no surprise. It is still noteworthy that junior- and sub-elite cross-country skiers that train a total of approximately 300 h from May to October show no improvements in physiological factors and only minor improvements in performance. On the other hand, the training performed was sufficient to maintain physiological and performance variables throughout the study period.
Strong correlations (p < 0.01) were found at baseline between TT performance and MAS (r = −0.79), DP-VO2peak (r = −0.83) and 1RM pulldown (r = −0.72). When corrected for gender all these correlations were still significant. This is in line with other studies examining performance determining factors in endurance sports, i.e., running, cycling and cross-country skiing (Pate and Kriska, 1984; Ingjer, 1991; di Prampero, 2003; Støren et al., 2013; Sunde et al., 2019; Johansen et al., 2020). Several studies have also observed better performance after improved MAS (Støren et al., 2008, 2012; Sunde et al., 2010; Johansen et al., 2020) or improved maximal strength (Hoff et al., 2002; Støren et al., 2008; Sunde et al., 2010). However, no significant relationships between changes in TTDP performance and changes in physiological variables were found in the present study.
The 3.3% non-significant improvement in TT performance in the present study is approximately half of that reported in Losnegaard et al. (2013). That study observed a 6% improvement from June to October in a 1000-meter TT in V2 skating in elite cross-country skiers, despite no improvements in VOpeak in V2 skating. However, C in V2 skating was significantly improved suggesting that the improvement in performance was due to an improvement in MAS in that study. Losnegaard et al. (2013) also explained the better performance by increased anaerobic capacity, measured as ΣO2-deficit. Anaerobic capacity was not measured directly in the present study. However, compared to the 1000 m TT used in Losnegaard et al. (2013) the anaerobic capacity should be of lesser importance in the 5.6 km TT used in the present study.
Overall aerobic capacity (RUN-VO2max) and specific (DP-VO2peak) aerobic capacity was not improved significantly from May to October in the present study. These findings are in line with studies investigating training patterns and development in VO2max in well-trained or elite cross-country skiers maintaining similar training routines (volume and intensity distribution) over longer periods (Rusko, 1987; Ingjer, 1992; Jones, 1998; Gaskill et al., 1999; Losnegaard et al., 2013; Solli et al., 2017). Further improvements of extremely high VO2max in elite endurance athletes have shown to be challenging. Compared to their elite counterparts, the skiers in the present study had approximately 20% lower aerobic capacities (Tønnessen et al., 2015; Sandbakk and Holmberg, 2017). This suggests that the potential for further improvements should be higher for the skiers in the present study, at least for the younger skiers. There is much evidence supporting that HIT may effectively improve VO2max, both in recreational and elite endurance athletes (Nilsson et al., 2004; Støren et al., 2012; Sandbakk et al., 2013; Rønnestad et al., 2014, 2016; Johansen et al., 2020). However, these interventional studies include longer or shorter periods of higher amounts of HIT, and lower total training volume. Stöggl and Sperlich (2014) reported superior adaptations in well-trained endurance athletes after 9 weeks of polarized training (56% LIT, 3% MIT, and 26% HIT) and HIT (43% LIT, 0% MIT, and 57% HIT) in VO2max, compared to training models with no training at HIT intensities and higher training volumes. This is well in line with the studies of Støren et al. (2012) and Gaskill et al. (1999), where endurance athletes experienced great improvements with a training program with higher amounts of HIT, with the same, or reduced total training volumes. Thus, we may speculate that more HIT training during pre-season may be crucial for further development of aerobic capacity in junior- and sub-elite cross-country skiers.
No statistically significant improvements in CDP were observed. However, like most of the other physiological variables, a slightly better average CDP was seen, although not significant. Losnegaard et al. (2013) reported improved C from June to October in elite cross-country skiers and this could be due to the increased ski-specific training. A significant correlation was also observed between change in total ski-specific training and ΔCDP in the present study, suggesting that adaptation is specifically to the movement patterns used in training (Scrimgeour et al., 1986; McMillan et al., 2005; Johansen et al., 2020). Previous studies have reported improved C after MST in both running (Støren et al., 2008), cycling (Sunde et al., 2010) and cross-country skiing (Hoff et al., 2002; Østerås et al., 2002). However, this relationship was not observed for the whole group in the present study, since MST and thus CDP did not change during the 6 month period.
Several previous studies have reported no training adaptations in LT in % of VO2max after shorter or longer periods of endurance or strength training (Helgerud et al., 2001, 2007; Støren et al., 2008, 2012; Sunde et al., 2010; Rønnestad et al., 2014). This is in line with results in the present study, since the skiers had almost exactly the same LT% at all test points. The present study also showed a strong correlation at baseline between MAS and LTv (r = 0.93, p < 0.01) indicating a close relationship, which have previously been reported (Støren et al., 2014; Sunde et al., 2019). Consequently, to elevate LTv skiers should aim to improve MAS (VO2max and C).
Sex Differences
Males had higher training volumes than females preceding the baseline tests in the present study. However, from May to October no significant sex differences were observed in total training volume, relative intensity distribution, endurance training, ski-specific training, strength training or other training. These training characteristics are in line with the findings in elite cross-country skiers from Solli et al. (2018), where males tended to train more in total than females throughout a whole year (∼ 90 h), although not significant. In Solli et al. (2018), strength and speed training was similar for males and females, as observed in the present study regarding strength training. However, in the present study the amount of speed and jump training was four times higher in females than males.
Males displayed significantly higher values than females in RUN-VO2max (19%), DP-VO2peak (19%), and MAS (32%), had better CDP (9%) and TTDP (15%) at baseline in the present study. These sex differences are in line with previous results (Sandbakk et al., 2014; Andersson et al., 2019; Sunde et al., 2019). Since MAS is the product of DP-VO2peak and CDP it was no surprise that the sum of sex differences in these two variables equalled almost exactly the difference seen in MAS. The gender difference in TTDP seemed to correspond to the 32% difference in MAS. This is further supported by the correlation between MAS and TTDP (r = −0.58, p < 0.01) at baseline corrected for gender.
Interestingly, the sex differences in DP-VO2peak was the same as in RUN-VO2max in the present study. Sandbakk et al. (2014) and Hegge et al. (2016), found the sex differences to be larger with increased contribution of upper-body musculature, i.e., larger in DP-VO2peak than in RUN-VO2max. Regarding 1RM strength variables, the gender differences were larger in 1RM pull-down (30%) compared to 1RM half-squat (21%) in the present study. These sex differences in 1RM strength are in line with previous results (Sandbakk et al., 2014; Sunde et al., 2019).
The sex differences at baseline in the present study were maintained in TTDP, RUN-VO2max, and DP-VO2peak from May to October due to no significant differences in training progression between males and females in this period. This may suggest that males and females do not differ in physiological and performance adaptations to a similar training pattern, which is in line with previous studies (Astorino et al., 2011; Støren et al., 2017; Varley-Campbell et al., 2018). However, sex difference in CDP declined significantly from PRE to POST1, due to a significantly improved CDP in females while the males maintained their pre-values. This may be explained by the lower training volumes in females 3-months prior to pre-test, resulting in a greater sex difference in CDP at PRE. From May to July, males and females trained more similar, at least in terms of ski-specific training, and this may have reduced the initial gap. Another explanation for the improved CDP observed in females, may be the relationship observed earlier between improved maximal strength and improved C in running, cycling and cross-country skiing (Hoff et al., 2002; Støren et al., 2008; Sunde et al., 2010). In the present study, a significant correlation was observed between Δ1RM pull-down and ΔCDP (r = −0.60, p < 0.05) in the female skiers, which supports this explanation. However, further improvements in CDP was not observed in females or males from August to October.
Age-Related Differences
To the best of our knowledge, no studies have investigated age-related differences in training characteristics and training adaptations between younger (junior athletes) and older skiers (senior athletes). In the present study, the age groups did not differ significantly in total training volume 3-months prior to pre-test. No differences were apparent in total training volume or in LIT, HIT, ski-specific training, running, cycling, strength or speed/jump training during the preparation period from May to October. The only difference between 16 and 18 years old compared to ≥19 years old was the amount of MIT, where the average difference were ∼ 20 min per week. Compared to other studies examining either junior- or adult skiers, the 16–18 years old skiers in the present study show similar training volumes as seen in previous studies on junior athletes, however, with a slightly lower amount of MIT and HIT (Sandbakk et al., 2011, 2013). However, the older skiers had lower training volumes compared to age-matched adult elite cross-country skiers (Losnegaard et al., 2013; Tønnessen et al., 2014; Sandbakk et al., 2016; Solli et al., 2017).
From May to October, the young and adult skiers did not differ significantly in training progression. The oldest skiers displayed almost no progression in all training variables, throughout the study period. This is in accordance to earlier observations of training progression in adult elite cross-country skiers (Losnegaard et al., 2013; Sandbakk et al., 2016).
At baseline, the adult skiers were 15% heavier than the younger skiers. A significant age-related difference was also apparent in TTDP (10.6%), which was followed by a 17.8% difference in MAS. Corrected for age, MAS showed a strong correlation to TTDP at baseline (r = −0.77, p < 0.01). The difference in MAS was almost exactly the same as in LTv, supporting that DP-VO2peak and CDP are the main predictors for LTv. The age difference in MAS is a consequence of the 10.7% difference in DP-VO2peak and the 7.9% difference in CDP. The age difference in RUN-VO2max and DP-VO2peak may be attributed to incomplete development of the cardiac system and muscle mass in the younger skiers still in puberty (Rusko, 1992). Additionally, the lower number of years of training in the young skiers may be an explanation for the observed difference. The difference in CDP may also be a result of less training years and experience in the younger skiers. In addition, the adult skiers had 21% higher 1RM pull-down than the younger skiers. Stronger skiers have shown to have higher peak forces in DP, lower DP frequency and shorter contact time (Sunde et al., 2019). However, all these age differences should be handled with great caution as they are most probably due to the sex differences in the two age groups. When analyzing age differences in males and females separately in the two groups, young and adult females differed in the same physiological and performance variables observed for the whole group, except for CDP and strength variables. For the males, almost every significant age-related difference disappeared, except for 1RM pull-down and DP-VO2peak in absolute values.
Effect of Selected Genes
We did not detect any major effects for the selected genes on physical and performance variables at baseline. Based on the low number of participants and the expected influence by single genes, this was not unexpected. However, we did find some minor effects.
In the present study the common ACTN3 R577X, X allele carriers demonstrated higher DP-VO2peak than participants with the RR genotype at PRE. This is in accordance to Pimenta et al. (2013) that observed that soccer players with the XX genotype had the highest VO2max. According to Yang et al. (2003) the X allele is overrepresented among endurance athletes, especially females. The importance of the advantageous allele is also likely dependent on the performance level (Eynon et al., 2012). However, others have not been able to confirm this (Papadimitriou et al., 2018). The X allele frequency in the present study was slightly higher (44 vs. 53%) among athletes than the general population from the same geographical area (Goleva-Fjellet et al., 2020). For the ACE gene, skiers with the II genotype exhibited higher RUN-VO2max compared with carriers of the D allele. However, participants with DD genotype demonstrated ∼15% better CDP and had ∼28% higher 1RM pull-down compared to the II genotype. The observed superior CDP among skiers with the DD genotype could be explained by gender differences in 1RM (Sunde et al., 2019). The I allele frequency among the cross-country skiers included in the present study was 14.6% higher than in a Norwegian cohort from the same geographic region (Goleva-Fjellet et al., 2020).
For the ACSL1 rs6552828, the A allele carriers had 8.4% higher RUN-VO2max (mL⋅kg–1⋅min–1 and mL⋅kg–0.67⋅min–1; p < 0.05) compared to the GG genotype. A relatively large effect of 6% on the training response of VO2max have been reported previously with the carriers and the common G allele exhibiting larger increase than the homozygotes of the less common A allele (Bouchard et al., 2011). The differences between the findings in the present study and the study of Bouchard et al. (2011) might be due to the different study population profiles, i.e., highly trained athletes vs. sedentary adults, respectively. The latter group have a larger potential of increasing their VO2max as a result of standardized exercise-training programs compared to athletes. The present study did not measure a significant increase in the VO2max throughout the testing period.
All associations between PPARGC1A rs8192678 and physiological and performance variables dissapeared when correcting for gender. The C allele (Gly) have been suggested to be an elite status endurance allele favorable to athletic ability (Tharabenjasin et al., 2019; Petr et al., 2020). Homozygotes of the C allele are generally more responding aerobic training compared to the T allele (Ser) (Petr et al., 2018). In the present study, only a single male participant possesed the least favorable genotype for endurance performance, i.e., TT. Despite possessing an unfavorable genotype to endurance performance, he demonstrated the highest RUN-VO2max of all participants. This points at carefullness when interpreting physiological performance based on single genes.
No significant associations were found for either of the following polymorphisms in the present study when corrected for gender: PPARA rs4253778, IL6 rs1474347, and PPARG rs1801282. For muscle function and jumping capacity, this is well in line with previous findings in other sports, at least for the PPARA rs4253778 polymorphism (Stastny et al., 2019). However, Stastny et al. (2019) found significant associations to other muscle parameters, such as reactive muscle index.
Despite previous findings on the effects of the ACE I/D and the ACTN3 R577X polymorphisms on athletic ability and trainability, the impact of these are not strong enough predictors to determine the athletic ability (Venezia and Roth, 2019). Results from the present study confirms that genotype frequencies for the two most investigated and replicated polymorphisms (i.e., ACE I/D and ACTN3 R577X) among the cross-country skiers were similar to those from a large general Scandinavian cohort (Goleva-Fjellet et al., 2020). Furthermore, there was the case of the one skier that possessed the least favorable endurance genotype for the PPARGC1A SNP, but still demonstrated the highest VO2max. These findings may indicate that possessing the optimal alleles of the different polymorphisms may be beneficial for endurance performance, but it is not critical for the athletic ability (Venezia and Roth, 2019; Petr et al., 2020). This may be especially true for athletes competing at a national level compared to world-class elite athletes (Eynon et al., 2012; Papadimitriou et al., 2016). However, the results from the present study should be treated with some caution due to the limited sample size. Some genotypes within the selected genes were either not present or only apparent in 1–2 participants, and may therefore influence our results. The material is thus prone to false negative results (type 2 errors), and we can only state that there were minor associations between some genotypes and physiological variables in our cohort of 29 skiers. This should be taken into account when interpreting the genetic results from the present study. Also, these athletes were already well trained and could be argued to not represent a good sample population to detect associations between genotype variants and physiological or performance characteristics.
Practical Implications
In the present study, maintaining the same training intensity distribution, and only increase total training volume was not sufficient to further improve aerobic capacity and cross-country skiing performance significantly throughout 6 months of training. This suggests that training programs with the same training intensity distribution, only differing in training volume, may not ensure optimal development of each individual skier independent of age and sex (Gaskill et al., 1999). For the individual well trained athlete, substantial changes in training volume and training intensity distribution could be necessary to facilitate further improvements, as observed in earlier studies (Gaskill et al., 1999; Støren et al., 2012; Bratland-Sanda et al., 2020). This is important knowledge for trainers of talented cross-country skiers that have faced stagnation.
An interesting finding in the present study is that our cohort of skiers did not differentiate genetically in two of the most investigated polymorphisms in association to athletic ability compared to a general Scandinavian cohort from the same geographical area. This may suggest that one might be able to reach a high national level in cross-country skiing without having the optimal genotypes in selected genes, with sufficient and individualized training.
Conclusion
Sex and age did influence physiological and performance variables at baseline, but did not influence training adaptations. Since the skiers in the present study did not display major changes in training, it was no surprise that no adaptations occurred in physiological or performance variables either. The genotype variants of selected genes were not critical determinants for physiological and performance variables in national and sub-elite cross-country skiers in the present study.
Data Availability Statement
Restrictions apply to the datasets: the datasets presented in this article are not readily available due to the Norwegian legislation regarding the publication of genetic data. Requests to access the datasets should be directed to the corresponding author.
Ethics Statement
The studies involving human participants were reviewed and approved by Regional Ethics Commitee of South-Eastern Norway, Telemark, Norway. Written informed consent to participate in this study was provided by the participants’ legal guardian/next of kin.
Author Contributions
J-MJ, SG-F, ØS, AS, MS, and JH all participated significantly in the planning and design of the study, as well as the data analyzing and the writing of the article. J-MJ, AS, ØS, SG-F, LG, LS, BF, and MS participated in the data collection. LG, LS, and BF also participated in the writing of the article. All authors read and approved the manuscript.
Conflict of Interest
The authors declare that the research was conducted in the absence of any commercial or financial relationships that could be construed as a potential conflict of interest.
Acknowledgments
We wish to thank all the participants in the study for great co-operation during the testing sessions and during the study period. We wish to thank Stina Stålberg, Leslie von der Voorde, and Trine Eidissen for all the help with taking blood samples.
Supplementary Material
The Supplementary Material for this article can be found online at: https://www.frontiersin.org/articles/10.3389/fphys.2020.581339/full#supplementary-material
References
Abdollahi, M. R., Huang, S., Rodriguez, S., Guthrie, P. A., Smith, G. D., Ebrahim, S., et al. (2008). Homogenous assay of rs4343, an ACE I/D proxy, and an analysis in the British Women’s Heart and Health study (BWHHS). Dis. Markers 24, 11–17. doi: 10.1155/2008/813679
Andersson, E. P., Govus, A., Shannon, O. M., and McGawley, K. (2019). Sex differences in performance and pacing strategies during sprint skiing. Front. Physiol. 10:295.
Astorino, T. A., Allen, R. P., Roberson, D. W., Jurancich, M., Lewis, R., McCarthy, K., et al. (2011). Adaptations to high-intensity training are independent of gender. Eur. J. Appl. Physiol. 111, 1279–1286. doi: 10.1007/s00421-010-1741-y
Austin, S., and St-Pierre, J. (2012). PGC1α and mitochondrial metabolism – emerging concepts and relevance in ageing and neurodegenerative disorders. J. Cell Sci. 125, 4963–4971. doi: 10.1242/jcs.113662
Bouchard, C., Sarzynski, M. A., Rice, T. K., Kraus, W. E., Church, T. S., Sung, Y. J., et al. (2011). Genomic predictors of the maximal O2 uptake response to standardized exercise training programs. J. Appl. Physiol. 110, 1160–1170. doi: 10.1152/japplphysiol.00973.2010
Bratland-Sanda, S., Pedersen, F. G., Haave, M. N., Helgerud, J., and Støren, Ø (2020). Large inter-individual differences in responses to a block of high intensity aerobic interval training: A case series in national-level cyclists and triathletes. Int. J. Exerc. Sci. 13, 480–487.
Cohen, J. (1988). Statistical Power Analysis for the Behavioral Sciences, 2 Edn. Hillsdale, NJ: Laurence Erlbaum Associates.
Conley, D. L., and Krahenbuhl, G. S. (1980). Running economy and distance running performance of highly trained athletes. Med. Sci. Sports Exerc. 12, 357–360. doi: 10.1249/00005768-198012050-00010
di Prampero, P. E. (2003). Factors limiting maximal performance in humans. Eur. J. Appl. Physiol. 90, 420–429. doi: 10.1007/s00421-003-0926-z
Eynon, N., Ruiz, J. R., Femia, P., Pushkarev, V. P., Cieszczyk, P., Maciejewska-Karlowska, A., et al. (2012). The ACTN3 R577X polymorphism across three groups of elite male European athletes. PLoS One 7:e43132. doi: 10.1371/journal.pone.0043132
Gaskill, S. E., Serfass, R. C., Bacharach, D. W., and Kelly, J. M. (1999). Responses to training in cross-country skiers. Med. Sci. Sports Exerc. 31, 1211–1217. doi: 10.1097/00005768-199908000-00020
Goleva-Fjellet, S., Bjurholt, A. M., Kure, E. H., Larsen, I. K., Støren, Ø, and Sæbø, M. (2020). Distribution of allele frequencies for genes associated with physical activity and/or physical capacity in a homogenous Norwegian cohort- a cross-sectional study. BMC Genetics 21:8.
Harvey, N. R., Voisin, S., Dunn, P. J., Sutherland, H., Yan, X., Jacques, M., et al. (2020). Genetic variants associated with exercise performance in both moderately trained and highly trained individuals. Mol. Genet. Genomics. 295, 515–523. doi: 10.1007/s00438-019-01639-8
Hegge, A. M., Bucher, E., Ettema, G., Faude, O., Holmberg, H. C., and Sandbakk, Ø (2016). Gender differences in power production, energetic capacity and efficiency of elite cross-country skiers during whole-body, upper-body, and arm poling. Eur. J. Appl. Physiol. 116, 291–300. doi: 10.1007/s00421-015-3281-y
Helgerud, J., Engen, L. C., Wisløff, U., and Hoff, J. (2001). Aerobic endurance training improves soccer performance. Med. Sci. Sports Exerc. 33, 1925–1931. doi: 10.1097/00005768-200111000-00019
Helgerud, J., Høydal, K. L., Wang, E., Karlsen, T., Berg, P. R., Bjerkaas, M., et al. (2007). Aerobic high-intensity intervals improve VO2max more than moderate training. Med. Sci. Sports Exerc. 39, 665–671. doi: 10.1249/mss.0b013e3180304570
Helgerud, J., Ingjer, F., and Strømme, S. B. (1990). Sex differences in performance-matched marathon runners. Eur. J. Appl. Physiol. 61, 433–439. doi: 10.1007/bf00236064
Hoff, J., Gran, A., and Helgerud, J. (2002). Maximal strength training improves aerobic endurance performance. Scand. J. Med. Sci. Sports. 12, 288–295. doi: 10.1034/j.1600-0838.2002.01140.x
Hopkins, W. G. (2000). A Scale of Min: A New View of Statistics. Available online at: http://www.sportsci.org/resource/stats/index.html (accessed May 28, 2020).
Ingjer, F. (1991). Maximal oxygen uptake as a predictor of performance ability in women and men elite cross-country skiers. Scand. J. Med. Sci. Sports 1, 25–30. doi: 10.1111/j.1600-0838.1991.tb00267.x
Ingjer, F. (1992). Development of maximal oxygen uptake in young elite male cross-country skiers: a longitudinal study. J. Sports Sci. 10, 49–63. doi: 10.1080/02640419208729906
Jacques, M., Landen, S., Voisin, S., and Eynon, N. (2019). “Summary findings on genetics and sport performance,” in Routledge Handbook of Sport and Exercise Systems Genetics, eds T. Lightfoot, M. J. Hubal, and S. M. Roth (Abingdon: Routledge), 347–356. doi: 10.4324/9781315146287-30
Johansen, J.-M., Eriksen, S., Sunde, A., Slettemeås, ØB., Helgerud, J., and Støren, Ø (2020). Improving utilization of maximal oxygen uptake and work economy in recreational cross-country skiers with high-intensity double-poling intervals. Int. J. Sports Physiol. Perform. doi: 10.1123/ijspp.2019-0689 [Epub ahead of print].
Jones, A. M. (1998). A five year physiological case study of an Olympic runner. Br. J. Sports Med. 32, 39–43. doi: 10.1136/bjsm.32.1.39
Losnegaard, T., Myklebust, H., Spencer, M., and Hallen, J. (2013). Seasonal variations in VO2max, O2-cost, O2-deficit, and performance in elite cross-country skiers. J. Strength Cond. Res. 27, 1780–1790. doi: 10.1519/jsc.0b013e31827368f6
Ma, F., Yang, Y., Li, X., Zhou, F., Gao, C., Li, M., et al. (2013). The association of sport performance with ACE and ACTN3 genetic polymorphisms: a systematic review and meta-analysis. PLoS One 8:e54685. doi: 10.1371/journal.pone.0054685
MacArthur, D. G., Seto, J. T., Chan, S., Quinlan, K. G., Raftery, J. M., Turner, N., et al. (2008). An Actn3 knockout mouse provides mechanistic insights into the association between alpha-actinin-3 deficiency and human athletic performance. Hum. Mol. Genet. 17, 1076–1086. doi: 10.1093/hmg/ddm380
Magi, A., Unt, E., Prans, E., Raus, L., Eha, J., Veraksits, A., et al. (2016). The association Analysis between ACE and ACTN3 genes polymorphisms and endurance capacity in young cross-country skiers: longitudinal study. J. Sports Sci. Med. 15, 287–294.
McMillan, K., Helgerud, J., MacDonald, R., and Hoff, J. (2005). Physiological adaptations to soccer specific endurance training in professional youth soccer players. Br. J. Sports Med. 39, 273–277. doi: 10.1136/bjsm.2004.012526
Nilsson, J. E., Holmberg, H. C., Tveit, P., and Hallén, J. (2004). Effects of 20-s and 180-s double poling interval training in cross-country skiers. Eur. J. Appl. Physiol. 92, 121–127. doi: 10.1007/s00421-004-1042-4
North, K. N., Yang, N., Wattanasirichaigoon, D., Mills, M., Easteal, S., and Beggs, A. H. (1999). A common nonsense mutation results in alpha-actinin-3 deficiency in the general population. Nat. Genet. 21, 353–354. doi: 10.1038/7675
Orysiak, J., Zmijewski, P., Klusiewicz, A., Kaliszewski, P., Malczewska-Lenczowska, J., Gajewski, J., et al. (2013). The association between ace gene variation and aerobic capacity in winter endurance disciplines. Biol. Sport 30, 249–253. doi: 10.5604/20831862.1077549
Østerås, H., Helgerud, J., and Hoff, J. (2002). Maximal strength-training effects on force-velocity and force-power relationships explain increases in aerobic performance in humans. Eur. J. Appl. Physiol. 88, 255–263. doi: 10.1007/s00421-002-0717-y
Papadimitriou, I. D., Lockey, S. J., Voisin, S., Herbert, A. J., Garton, F., Houweling, P. J., et al. (2018). No association between ACTN3 R577X and ACE I/D polymorphisms and endurance running times in 698 Caucasian athletes. BMC Genomics. 19:13.
Papadimitriou, I. D., Lucia, A., Pitsiladis, Y. P., Pushkarev, V. P., Dyatlov, D. A., Orekhov, E. F., et al. (2016). ACTN3 R577X and ACE I/D gene variants influence performance in elite sprinters: a multi-cohort study. BMC Genomics 17:285.
Pate, R. R., and Kriska, A. (1984). Physiological basis of the sex difference in cardiorespiratory endurance. Sports Med. 1, 87–98. doi: 10.2165/00007256-198401020-00001
Pescatello, L. S., Corso, L. M. L., Santos, L. P., Livingston, J., and Taylor, B. A. (2019). “Angiotensin-converting enzyme and the genomics of endurance performance,” in Routledge Handbook of Sport and Exercise Systems Genetics, eds T. Lightfoot, M. J. Hubal, and S. M. Roth (Abingdon: Routledge), 216–249. doi: 10.4324/9781315146287-21
Petr, M., Maciejewska-Skrendo, A., Zajac, A., Chycki, J., and Stastny, P. (2020). Association of elite sports status with gene variants of peroxisome proliferator activated receptors and their transcriptional coactivator. Int. J. Mol. Sci. 21:162. doi: 10.3390/ijms21010162
Petr, M., Stastny, P., Zajac, A., Tufano, J. J., and Maciejewska-Skrendo, A. (2018). The role of peroxisome proliferator-activated receptors and their transcriptional coactivators gene variations in human trainability: a systematic review. Int. J. Mol. Sci. 19:1472. doi: 10.3390/ijms19051472
Pimenta, E. M., Coelho, D. B., Veneroso, C. E., Barros Coelho, E. J., Cruz, I. R., Morandi, R. F., et al. (2013). Effect of ACTN3 gene on strength and endurance in soccer players. J. Strength Cond. Res. 27, 3286–3292. doi: 10.1519/jsc.0b013e3182915e66
Puthucheary, Z., Skipworth, J. R., Rawal, J., Loosemore, M., Van Someren, K., and Montgomery, H. E. (2011). The ACE gene and human performance: 12 years on. Sports Med. 41, 433–448. doi: 10.2165/11588720-000000000-00000
Rønnestad, B. R., Ellefsen, S., Nygaard, H., Zacharoff, E. E., Vikmoen, O., Hansen, J., et al. (2014). Effects of 12 weeks of block periodization on performance and performance indices in well-trained cyclists. Scand. J. Med. Sci. Sports. 24, 327–335. doi: 10.1111/sms.12016
Rønnestad, B. R., Hansen, J., Thyli, V., Bakken, T. A., and Sandbakk, Ø (2016). 5-week block periodization increases aerobic power in elite cross-country skiers. Scand. J. Med. Sci. Sports 26, 140–146. doi: 10.1111/sms.12418
Roth, S. M., Walsh, S., Liu, D., Metter, E. J., Ferrucci, L., and Hurley, B. F. (2008). The ACTN3 R577X nonsense allele is under-represented in elite-level strength athletes. Eur. J. Hum. Genet. 16, 391–394. doi: 10.1038/sj.ejhg.5201964
Rusko, H. K. (1987). Development of aerobic power in relation to age and training in cross-country skiers. Med. Sci. Sports Exerc. 24, 1040–1047.
Rusko, H. K. (1992). Development of aerobic power in relation to age and training in cross-country skiers. Med. Sci. Sports Exerc. 24, 1040–1047.
Sandbakk, Ø., Ettema, G., and Holmberg, H.-C. (2014). Gender differences in endurance performance by elite cross-country skiers are influenced by the contribution from poling. Scand. J. Med. Sci. Sports 24, 28–33. doi: 10.1111/j.1600-0838.2012.01482.x
Sandbakk, Ø, Hegge, A. M., Losnegaard, T., Skattebo, Ø, Tønnessen, E., and Holmberg, H. C. (2016). The physiological capacity of the world’s highest ranked female cross-country skiers. Med. Sci. Sports Exerc. 48, 1091–1100. doi: 10.1249/mss.0000000000000862
Sandbakk, Ø, and Holmberg, H. C. (2017). Physiological capacity and training routines of elite cross-country skiers: approaching the upper limits of human endurance. Int. J. Sports Physiol. Perform. 12, 1003–1011. doi: 10.1123/ijspp.2016-0749
Sandbakk, Ø, Holmberg, H. C., Leirdal, S., and Ettema, G. (2011). The physiology of world-class sprint skiers. Scand. J. Med. Sci. Sports 21, e9–e16.
Sandbakk, Ø, Sandbakk, S. B., Ettema, G., and Welde, B. (2013). Effects of intensity and duration in aerobic high-intensity interval training in highly training junior cross-country skiers. J. Strength Cond. Res. 27, 1974–1980. doi: 10.1519/jsc.0b013e3182752f08
Sandbakk, Ø, Welde, B., and Holmberg, H. C. (2010). Endurance training and sprint performance in elite junior cross-country skiers. J. Strength Cond. Res. 25, 1299–1305. doi: 10.1519/jsc.0b013e3181d82d11
Scrimgeour, A. G., Noakes, T. D., Adams, B., and Myburgh, K. (1986). The influence of weekly training distance on fractional utilization of maximum aerobic capacity in marathon and ultramarathon runners. Eur. J. Appl. Physiol. 55, 202–209. doi: 10.1007/bf00715006
Seiler, S., and Kjerland, G. Ø (2006). Quantifying trainng intensity distribution in elite endurance atheletes: is there evidence for an “optimal” distribution? Scand. J. Med. Sci. Sports 16, 49–56. doi: 10.1111/j.1600-0838.2004.00418.x
Solli, G. S., Kocbach, J., Seeberg, T. M., Tjønnås, J., Rindal, O. M. H., Haugnes, P., et al. (2018). Sex-based differences in speed, sub-technique selection, and kinematic patterns during low- and high-intensity training for classical cross-country skiing. PLoS ONE 13:e0207195. doi: 10.1371/journal.pone.0207195
Solli, G. S., Tønnessen, E., and Sandbakk, Ø (2017). The training characteristics of the world’s most successful female cross-country skier. Front. Physiol. 8:1069.
Stastny, P., Lehnert, M., De Ste Croix, M., Petr, M., Svoboda, Z., Maixnerova, E., et al. (2019). Effect of COL5A1, GDF5, and PPARA genes on a movement screen and neuromuscular performance in adolescent team sport athletes. J. Strength Cond. Res. 33, 2057–2065. doi: 10.1519/jsc.0000000000003142
Stöggl, T. L., and Sperlich, B. (2014). Polarized training has greater impact on key endurance variables than threshold, high intensity, or high volume training. Front. Physiol. 5:33.
Stöggl, T. L., and Sperlich, B. (2015). The training intensity distribution among well-trained and elite endurance athletes. Front. Physiol. 6:295.
Støren, Ø, Bratland-Sanda, S., Haave, M., and Helgerud, J. (2012). Improved VO2max and time trial performance with more high aerobic intensity interval training and reduced training volume: a case study on an elite national cyclist. J. Strength Cond. Res. 26, 2705–2711. doi: 10.1519/jsc.0b013e318241deec
Støren, Ø, Helgerud, J., Sæbø, M., Støa, E. M., Bratland-Sanda, S., Unhjem, R. J., et al. (2017). The effect of age on the VO2max response to high-intensity interval training. Med. Sci. Sports Exerc. 49, 78–85.
Støren, Ø, Helgerud, J., Støa, E. M., and Hoff, J. (2008). Maximal strength training improves running economy in distance runners. Med. Sci. Sports Exerc. 40, 1087–1092. doi: 10.1249/mss.0b013e318168da2f
Støren, Ø, Rønnestad, B. R., Sunde, A., Hansen, J., Ellefsen, S., and Helgerud, J. (2014). A time-saving method to assess power output at lactate threshold in well-trained and elite cyclists. J. Strength Cond. Res. 28, 622–629. doi: 10.1519/jsc.0b013e3182a73e70
Støren, Ø, Ulevåg, K., Larsen, M. H., Støa, E. M., and Helgerud, J. (2013). Physiological determinants of the cycling time trial. J. Strength Cond. Res. 27, 2366–2373. doi: 10.1519/jsc.0b013e31827f5427
Sunde, A., Johansen, J.-M., Gjøra, M., Paulsen, G., Bråten, M., Helgerud, J., et al. (2019). Stronger is better: The impact of upper-body strength in double poling performance. Front. Physiol. 10:1091.
Sunde, A., Støren, Ø, Bjerkaas, M., Larsen, M. H., Hoff, J., and Helgerud, J. (2010). Maximal strength training improves cycling economy in competitive cyclists. J. Strength Cond. Res. 24, 2157–2165. doi: 10.1519/jsc.0b013e3181aeb16a
Sylta, Ø, Tønnessen, E., and Seiler, S. (2014). From heart-rate data to training quantification: a comparison of 3 methods of training-intensity analysis. Int. J. Sports Physiol. Perform. 9, 100–107. doi: 10.1123/ijspp.2013-0298
Tharabenjasin, P., Pabalan, N., and Jarjanazi, H. (2019). Association of PPARGC1A Gly428Ser (rs8192678) polymorphism with potential for athletic ability and sports performance: a meta-analysis. PLoS One 14:e0200967.
Tønnessen, E., Haugen, T. A., Hem, E., Leirstein, S., and Seiler, S. (2015). Maximal aerobic capacity in the winter-olympics endurance disciplines: olympic-medal benchmarks for the time period 1990-2013. Int. J. Sports Physiol. Perform. 10, 835–839.
Tønnessen, E., Sylta, Ø, Haugen, T. A., Hem, E., Svendsen, I. S., and Seiler, S. (2014). The road to gold: training and peaking characteristics in the year prior to a gold medal endurance performance. PLoS One 9:e101796.
Varley-Campbell, J., Cooper, C., Wilkerson, D., Wardle, S., Greeves, J., and Lorenc, T. (2018). Sex-specific changes in physical performance following military training: a systematic review. Sports Med. 48, 2623–2640.
Venezia, A. C., and Roth, S. M. (2019). “The scientific and ethical challenges of using genetic information to predict sport performance,” in Routledge Handbook of Sport and Exercise Systems Genetics, eds T. Lightfoot, M. J. Hubal, and S. M. Roth (Abingdon: Routledge), 442–452.
Keywords: endurance training, skiing performance, training adaptations, double poling, maximal oxygen uptake, lactate threshold, work economy, genomics
Citation: Johansen J-M, Goleva-Fjellet S, Sunde A, Gjerløw LE, Skeimo LA, Freberg BI, Sæbø M, Helgerud J and Støren Ø (2020) No Change – No Gain; The Effect of Age, Sex, Selected Genes and Training on Physiological and Performance Adaptations in Cross-Country Skiing. Front. Physiol. 11:581339. doi: 10.3389/fphys.2020.581339
Received: 08 July 2020; Accepted: 02 October 2020;
Published: 26 October 2020.
Edited by:
Luca Paolo Ardigò, University of Verona, ItalyReviewed by:
Elisa Calabria, University of Verona, ItalyPetr Stastny, Charles University, Czechia
José Antonio De Paz, Universidad de León, Spain
Copyright © 2020 Johansen, Goleva-Fjellet, Sunde, Gjerløw, Skeimo, Freberg, Sæbø, Helgerud and Støren. This is an open-access article distributed under the terms of the Creative Commons Attribution License (CC BY). The use, distribution or reproduction in other forums is permitted, provided the original author(s) and the copyright owner(s) are credited and that the original publication in this journal is cited, in accordance with accepted academic practice. No use, distribution or reproduction is permitted which does not comply with these terms.
*Correspondence: Jan-Michael Johansen, amFuLW1pY2hhZWwuam9oYW5zZW5AdXNuLm5v