- School of Medical Information Engineering, Guangzhou University of Chinese Medicine, Guangzhou, China
Introduction: Recognizing a tooth-marked tongue has important clinical diagnostic value in traditional Chinese medicine. Current deep learning methods for tooth mark detection require extensive manual labeling and tongue segmentation, which is labor-intensive. Therefore, we propose a weakly supervised multipleinstance active learning model for tooth-marked tongue recognition, aiming to eliminate preprocessing segmentation and reduce the annotation workload while maintaining diagnostic accuracy.
Method: We propose a one-stage method tongenerate tooth mark instances that eliminates the need for pre-segmentation of the tongue. To make full use of unlabeled data, we introduce a semisupervised learning paradigm to pseudo-label unlabeled tongue images with high model confidence in active learning and incorporate them into the training set to improve the training efficiency of the active learning model. In addition, we propose an instance-level hybrid query method considering the diversity of tooth marks.
Result: Experimental results on clinical tongue images verify the effectiveness of the proposed method, which achieves an accuracy of 93.88% for tooth-marked tongue recognition, outperforming the recently introduced weakly supervised approaches.
Conclusion: The proposed method is effective with only a small amount of image-level annotation, and its performance is comparable to that of image-level annotation, instance-level annotation and pixel-level annotation, which require a large number of tooth markers. Our method significantly reduces the annotation cost of the binary classification task of traditional Chinese medicine tooth mark recognition.
1 Introduction
The tongue is the main organ of human internal organs and can reflect disorder and even pathological changes in human internal organs. Tongue diagnosis is a non-invasive diagnostic method that provides important signs for early diagnosis of disease and human health. The tooth mark, one of the most important features of the tongue, is usually formed when the tongue body is squeezed by adjacent teeth. The clinical manifestations of patients with a tooth-marked tongue are anorexia, abdominal distention, gastric distention, and constipation (David Zhang and Zhang, 2017). Therefore, identifying tooth-marked tongue is of great value for clinical diagnosis. In the clinic, tooth-marked tongue recognition depends on doctors’ subjective observation of the morphological information of the tooth marks on the tongue. Researchers have attempted to use digital image processing and feature analysis for objective tooth mark recognition, especially based on tooth mark shape and color features (Hsu et al., 2011; Lo et al., 2012; Shao et al., 2014). However, due to the great differences in the shape and color of tooth marks, it is difficult to ensure the reliability and accuracy of tooth-marked tongue recognition according to the color or shape characteristics.
Deep neural networks (DNNs), with their significant feature representation advantages, have been applied to tooth-marked tongue recognition. There are two kinds of tooth-marked tongue recognition methods: one is tooth-marked tongue classification based on supervised image-level annotation, and the other is object detection based on tooth-marked areas. For the first category of tooth-marked tongue image classification, Sun et al. (2019) proposed to classify tooth-marked tongue images by deep convolution networks, and the concerned area of tooth marks is visualized by the Grad-CAM (Selvaraju et al., 2017) model. Wang et al. (2020) used a deeper convolutional neural network (CNN), Resnet34 (He et al., 2016), and demonstrated that their method achieved better efficiency and scene generalization ability. Lu et al. (2023) proposed a prior regularization tooth-marked tongue recognition method utilizing the prior knowledge of the location and width of tooth marks. Tan et al. (2023) used a non-subsampled wavelet transform for multi-scale decomposition and applied the autoregressive local linear model encoding algorithm to retain key texture information and remove redundant data in the decomposed sub-images. This enabled the model to more comprehensively extract the texture features of tooth-marked tongues, thereby improving the accuracy of identification.
However, tooth-marked tongue recognition is a fine-grained classification problem, which is not suitable for classification using the above image-level supervision information (Fu et al., 2017). For the other category of tooth-marked tongue recognition with respect to object detection, Weng et al. (2021) proposed that the tooth mark on the tongue be selected by frame, introduced the YOLOv3 (Redmon and Farhadi, 2018) model in object detection to detect the tooth mark, and obtained promising results. Li et al. (2018) considered tooth-marked tongue recognition as a multiple instance learning (MIL) framework and first used the prior knowledge that the tooth mark is concave–convex to generate candidate regions. They then trained an instance feature extractor followed by multi-instance classification Via support vector machine (MISVM) (Andrews et al., 2002) for classification to obtain good performance. The above methods based on object detection can yield promising performance, but they require a large number of tooth mark instances with strong annotation, which carries a huge labor cost. Recently, Zhou et al. (2022) proposed a weakly supervised target detection model (WSDDN) (Bilen and Vedaldi, 2016) for tooth-marked tongue recognition to avoid the labor cost of toothmark instances. However, this method requires tongue segmentation in advance, which has a very large clinical workload. More importantly, the above tooth-marked tongue recognition methods do not consider large numbers of unlabeled data. When clinical labeling is very cumbersome, this unlabeled tongue information should be included to improve the performance of tooth-marked tongue recognition.
Tooth-marked tongue detection with few image-level annotations is a very challenging problem. First, if the tongue body is not pre-segmented, the boundary information of the tongue cannot be obtained. Consequently, it becomes difficult to extract samples based on the prior knowledge that tooth marks are distributed on the edge of the tongue body. Then, the simple instance generation method (Van de Sande et al., 2011; Girshick, 2015; Zitnick and Dollár, 2014) in object detection often generates many instances from the background so that the image-level information learned by the model is seriously disturbed by background noise, resulting in inconsistent uncertainty between image-level and target instances. Subsequently, a large amount of unlabeled tongue image data with tooth mark information is not utilized by deep learning networks, and whether to use active learning or semi-supervised learning for fine-grained detection tasks without instance-level labeling is still an unexplored problem. Finally, due to the diversity of the instances of tooth marks produced by the tooth-marked tongue, it is difficult to accurately represent image-level information only by the uncertainty of the instance.
In this work, we propose a weakly supervised multiple-instance active learning (WSMIAL) model for tooth-marked tongue recognition that can significantly reduce the labeling cost from three aspects: instance-level tooth mark region selection, pixel-level tongue segmentation, and image-level category labeling. Specifically, we propose a one-stage method to generate toothmark instances without pre-tongue segmentation. Then, we introduce a semi-supervised learning paradigm to pseudo-label images with high model confidence and incorporate them into the training set to improve the training efficiency of the active learning model. In addition, we align the uncertainty consistency between the tooth mark instances and the tongue image and propose an instance-level hybrid query method considering the diversity of tooth marks. Through comparative experiments of clinical tongue images with related work, the proposed model has a competitive performance in tooth-marked tongue recognition, and its annotation cost is much lower than the existing tooth-marked tongue recognition models.
2 Materials and methods
2.1 Study population and tooth mark labels
The study was approved by the local ethics committee, and the patients signed the informed consent form. We used standard equipment designed by Daoshi Medical Technology Co., Ltd. (DS01-B) to obtain tongue images from patients in the local institute. We obtained 1,108 tongue images from the local medical research institution. Figure 1 shows the representative tongue images with or without tooth marks. The clinical criteria for the diagnosis of tooth-marked tongue in traditional Chinese medicine are as follows: first, observe whether there are teeth squeezing on both sides of the tongue body, resulting in tooth marks; Second, for parts of the tongue where the tooth mark is not obvious, observe the color depth of the suspicious area. The extruded tooth mark area usually has a darker color (Weng et al., 2021), as shown in Figures 1a,c. Four traditional Chinese medicine doctors with 2–5 years of clinical experience divided the tongue image into tooth-marked or non-tooth-marked areas and framed the mark area judged as a tooth-marked tongue to construct the tongue image data set. Although the proposed method in this work does not need to segment the tongue in advance and does not need instances of tooth marks to realize other relevant tooth-marked tongue recognition methods and compare their performance, we also arranged for doctors to segment the tongue and label the tooth marks.
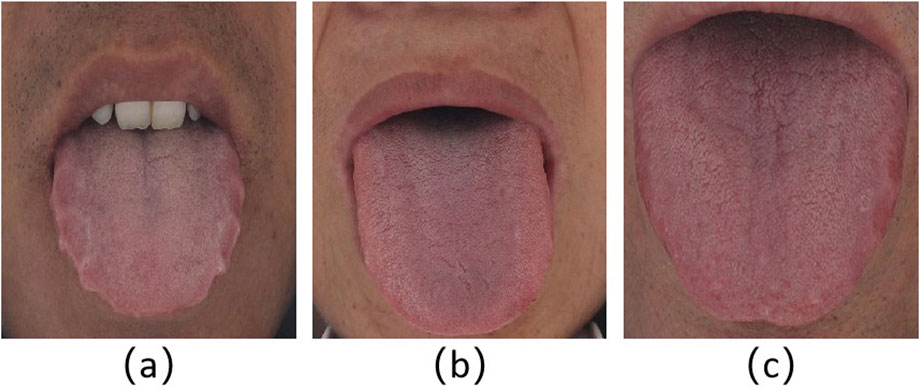
Figure 1. Representative tongue images. (a) Tooth-marked tongue. The outline of both sides of the tongue is obviously distorted, and the color of the tongue extrusion area changes; (b) tongue without tooth marks. The tongue is flat, with regular peripheral contour, without contour deformation and discoloration area; (c) suspicious tooth-marked tongue. Because the tongue body is flat and the surrounding contour is not distorted, its label is finally determined as a tooth-marked tongue by the color change of the extrusion area of the tongue edge.
The flowchart of the work in this article is presented in Figure 2. The blue arrows represent the workflow of labeled data, the light pink arrows represent the workflow of unlabeled data, and the gradient arrows represent the workflow of sharing labeled and unlabeled data. Specifically, during the pre-training of the feature extractor and the weakly supervised multi-instance learning stage, only the data with labels is used, and the output is a binary classification label of whether an image represents a toothed tongue or not. In the instance uncertainty learning stage, unlabeled data are added to the training, and then they enter the instance representation learning stage together. The output is a tongue image with a bounding box of the predicted tooth mark area. Finally, the unlabeled data are subjected to efficient active learning. The pseudo-labeled standard samples are finally placed in the label data pool to start a new round of training. Only samples that are particularly difficult to identify will be given to the experts for annotation.
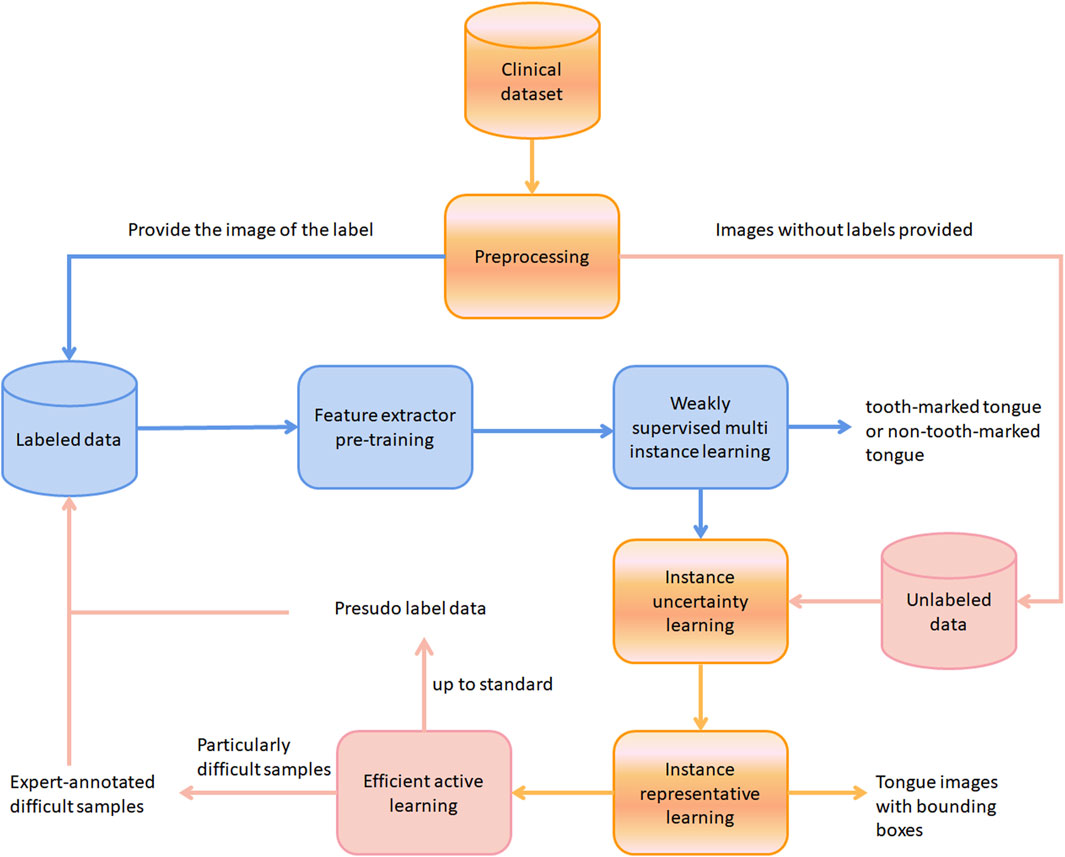
Figure 2. The workflow of this work. The blue, light pink, and black arrows represent the processes of labeled data, unlabeled data, and combined data processing, respectively.
2.2 Proposed method
In order to reduce the interference of the background on tooth mark recognition, previous studies (Li et al., 2018; Sun et al., 2019; Wang et al., 2020; Weng et al., 2021; Zhou et al., 2022) generally needed to preliminarily segment the tongue, which has high labor costs. By comparison, we use a one-stage detection method to directly extract the candidate tooth mark area to avoid the tedious work of tongue pre-segmentation. Then, considering that tooth-marked tongue recognition is a fine-grained classification problem, we adopt the multi-instance learning paradigm (Li et al., 2018). In addition, obtaining a large number of labeled tooth-marked tongues requires high labor costs, while unlabeled data are relatively easy to obtain and have not been used in tooth-marked tongue recognition. Typically, there are two ways to use unlabeled data: active learning and semi-supervised learning. The former requires expert participation, and the latter does not. Inspired by the works by Wang et al. (2017) and Zhang et al. (2022), we use the combination of the two to obtain better performance. The main difference is that we acquire image information based on instance information and build a multi-instance efficient active learning framework.
Previous studies have shown that weakly supervised target detection can assist in locating the location of tooth marks, and its advantage is that it does not require instance-level annotation (Zhou et al., 2022). If the active learning model is introduced based on the weakly supervised network, a significant reduction of instance-level and image-level annotations can be achieved. However, there are two problems in simply combining weakly supervised target detection and active learning. The first is weak supervision. There is no instance box labeling, so it is difficult to filter instances. If the image information is simply obtained by averaging the instance information with high uncertainty, it will lead to the problem of background instance interference. Second, due to the diversity of tooth marks, the selected examples cannot fully represent the image. In order to solve the above problems, we start with the uncertainty consistency between instances and images and the representative learning of instances and get the best image-level information through multi-instance learning.
Figure 3 shows the overall framework of the proposed WSMIAL model. The framework is composed of an instance generation module, a weakly supervised learning module, and a multi-instance active detection module.
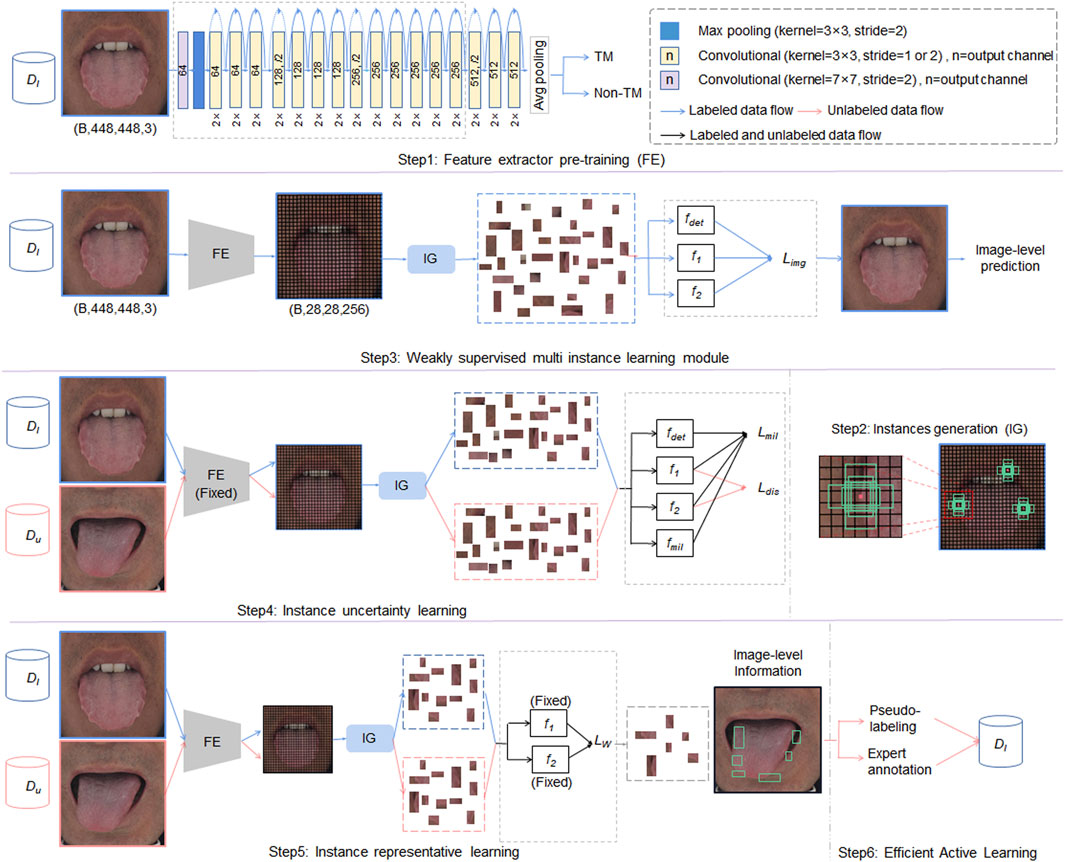
Figure 3. The proposed weakly supervised multiple-instance active learning (WSMIAL) network. FE means feature extractor,
As shown in Figure 3, the blue, red, and black arrows represent the processes of labeled data, unlabeled data, and combined data processing, respectively. Initially, in step 1, the feature extractor is pre-trained using labeled data. Subsequently, step 2 involves generating instance proposals, which are then employed in step 3 for image-level predictions aimed at identifying tooth-marked tongues. Step 4 performs instance uncertainty learning, fixes the feature extractor’s parameters, and fine-tunes the instance classifiers (
2.3 Instances generation module
Similar to Weng et al. (2021), we use a one-stage method to generate instances of tooth marks. The preset boundary boxes are the length and width obtained by K-means clustering (Redmon and Farhadi, 2018), while the backbone of feature extraction is replaced by Resnet34, which has been demonstrated to be the best tooth mark feature extraction model (Wang et al., 2020). For the predefined bounding boxes, we randomly select nine clusters and three scales, evenly distributing the clusters across the scales. The nine clusters are (37, 19), (20, 41), (25, 36), (37, 35), (27, 52), (21, 69), (34, 47), (37, 64), and (32, 90). We divide images into
2.4 Weakly supervised learning module
In the instance generation module, each lattice is responsible for predicting the bounding box and the confidence of the bounding box, but it may not completely contain objects. The generated bounding box may not be able to frame the target well, and there is no real bounding box, so the confidence cannot be calculated according to the definition (Redmon et al., 2016):
Specifically, the detection branch is defined as Equation 1:
where
The classification branch is defined as Equations 2, 3:
where
In the two branches of our model, the final score of each region is obtained by taking the Hadamard product of two module scores:
Finally, we calculate the image classification loss function as Equation 5:
2.5 Multi-instance active learning
The key to active learning lies in sample selection. If we simply use the image score in Equation 4 as the information degree of the image to select the sample, we will inevitably introduce many background noise instances. Because we do not use the pre-segmentation method to generate candidate instances, the image score will be calculated using many background noises. In addition, there is diversity in tooth mark instances, and such an image score cannot represent the information degree of the image. Therefore, we build the instance uncertainty learning module and the instance representative learning module.
2.5.1 Instance uncertainty learning
To learn the uncertainty at the instance level, we use two antagonistic instance classifiers in the detection network to obtain the prediction of the instance (Lakshminarayanan et al., 2017). The prediction difference between these two classifiers is maximized to predict the uncertainty of the instance. The relevant equations are shown in Equations 6, 7:
where
In addition, we add multiple instance learning classifiers
The classification score Equation 4 at the image level is updated to Equation 9
We reweight the uncertainty score of instances by minimizing the loss of image classification to reweight the uncertainty of instances while filtering noise instances. This actually defines an expectation maximization procedure (Andrews et al., 2002; Bilen and Vedaldi, 2016). Considering that the MIL score of instances from backgrounds is very small, this can be achieved by optimizing the following loss function:
This makes instances with larger classification scores but smaller MIL scores be suppressed as the background. First, the initial detector is obtained by applying MIL loss in the labeled pool training process, and then the instance uncertainty in the unlabeled set is reweighted. We combine the image classification scores of all categories into a score vector, and then reweight the example uncertainty, as represented in Equation 11:
Finally, we update the optimization loss in Equation 6 to Equation 12:
According to Equation 8 and Equation 10, MIL loss ensures that the highlighted instance uncertainty can represent the image uncertainty, that is, minimizing the classification loss of the image and minimizing the gap between the instance uncertainty and the image uncertainty. Through the iterative optimization of Equation 12, the gap between instance-level observation and image-level evaluation can be narrowed statistically, and this is helpful in suppressing instances with high noise.
2.5.2 Instance representative learning
A well-known problem of uncertainty-based sampling in active learning is the so-called sampling bias (Li et al., 2021), indicating that the current instance cannot represent the image’s potential distribution (Yuan et al., 2021). In particular, during the generation of tooth marks, there are many instances with similar characteristics to tooth marks that are not distributed on the tongue. As shown in Figure 3, teeth, lips, cracks, and ecchymosis are distributed on the tongue, which makes the instances based on uncertainty alone unable to fully represent the information of the image. Therefore, we introduce Wasserstein distance to select the most representative instances.
The advantage of Wasserstein distance (Villani, 2009) is that even if the two distributions do not overlap, Wasserstein distance can still reflect their distance. This has been applied to the field of image generation and has been demonstrated to be a good measure of diversity (Gulrajani et al., 2017; Zhang et al., 2020). The Wasserstein distance is defined as
where
Because
When the Lipschitz constant
Due to the strong fitting ability of a deep neural network, a series of
The smaller the value of
2.5.3 Efficient active learning
Because the data with image-level annotation need annotation are expensive, the labeled samples in active learning are not sufficient to train a deep neural network because most unlabeled samples are usually ignored in active learning. It is difficult to obtain proper feature representation by fine-tuning the deep neural network using these few information samples. Therefore, we introduce a more efficient active learning method, namely, semi-supervised active learning (Wang et al., 2017; Li et al., 2021). Specifically, a few images with rich information help train more powerful classifiers, while most high-confidence images help learn more distinctive feature representations. On the one hand, although the number of labeled data is small, the most uncertain unlabeled samples usually have a great potential impact on the classifier. Selecting and labeling them in training can help to develop a better decision boundary for the classifier. On the other hand, although the performance of the classifier cannot be significantly improved, the unlabeled samples with high confidence are close to the labeled samples in the feature space of a deep neural network. The practice of pseudo-labeling and incorporating them into the training set can improve the generalization performance of modularity and is conducive to the low-density separation between categories (Zou et al., 2019; Wei et al., 2021).
Because the uncertainty between the image and the instance has been aligned, we select the top
It is worth noting that the image will be pseudo-marked only when the uncertainty of the image is less than
where
2.6 The implementation
The proposed model is implemented using PyTorch (pytorch.org), and the objective function is minimized by using the AdmaW algorithm. We used an NVIDIA RTX3090 graphics card with 24 G memory. Parameters are set as follows:
3 Experimental results
3.1 Experimental setup and evaluation metric
In order to assess the performance of the proposed method and conduct an objective comparison with other related methods, we annotate all tooth marks in tongue images at the image and instance levels in advance. Meanwhile, different proportions of labels were assigned in specific experiments to simulate the active learning process of interacting with doctors and objectively compared with other methods. Specifically, because only a few studies only used CNN as a feature extractor (Li et al., 2018; Wang et al., 2020; Zhou et al., 2022; Weng et al., 2021), we use Resnet34 (Wang et al., 2020) as the backbone for fair comparison in all the methods. The clinical screening data set contains 401 images of tooth-marked tongues and 707 images of non-tooth-marked tongues. We randomly used 108 of 1,108 images as an independent test set
Accuracy reflects the percentage of correct predictions made by the model over the entire dataset. It is calculated by the Equation 20:
Precision represents the proportion of all samples predicted by the model to be in the positive category that are actually in the positive category. It is calculated by Equation 21:
Recall, also known as the sensitivity or true-positive rate, measures the proportion of samples that the model correctly identifies as being in the positive category of all samples that truly belong in the positive category. It is calculated as Equation 22:
F1 score (F1-Score) is the reconciled average of precision and recall and is often used to balance the trade-off between the two. It is particularly adaptable to the problem of category imbalance. It is calculated by Equation 23:
3.2 Performance comparison with different methods
Current tooth-marked tongue recognition methods (Li et al., 2018; Wang et al., 2020; Zhou et al., 2022; Weng et al., 2021) all require 100% image-level annotation, while the object detection-based methods (Li et al., 2018; Zhou et al., 2022; Weng et al., 2021) require further instance-level annotation. In order to obtain better detection performance (Li et al., 2018; Wang et al., 2020; Zhou et al., 2022), the tongue body must be segmented at the pixel level in advance in order to eliminate the interference of the background area outside the tongue. From the performance comparison in Table 1 and Figure 4, it can be seen that Li et al. (2018) have the top performance of tooth-marked tongue recognition among the above methods but also require the highest annotation cost. By comparison, our method can match the performance of Li et al. (2018) with only 50% image-level annotation without instance-level and pixel-level annotation. Moreover, after active learning completes 100% annotation of the image, the tooth-marked tongue recognition performance can slightly exceed the results of Li et al. (2018). This is because our active learning strategy based on instance uncertainty and diversity can make the model obtain a better decision boundary for tooth-marked tongue recognition. In addition, the pseudo-annotation method can make the active learning model with small data more robust.
3.3 Ablation study of multi-instance active learning
In terms of query strategy in active learning, we compare the proposed query strategy with baseline random sampling (random) and common active learning strategies based on instance filtering, such as sampling based on least confidence (Guo et al., 2017), sampling based on uncertainty (entropy) (Lakshminarayanan et al., 2017), and unsupervised clustering sampling based on diversity (K-means) (Ash et al., 2019). As shown in Figure 5, the sampling based on uncertainty outperforms random, minimum confidence (least confidence), and clustering (K-means). Under the same detection model (Section 2.4 WSL module), the entropy method has the best performance among the common active learning strategies, but they all fail to surpass the performance of WSL’s backbone Resnet34 under 100% annotation. The methods of deterministic learning and representative learning of examples are targeted to solve the problems of weakly supervised detection of background noise and lack of representativeness of examples and make full use of unlabeled data, thus surpassing other common active learning strategies.
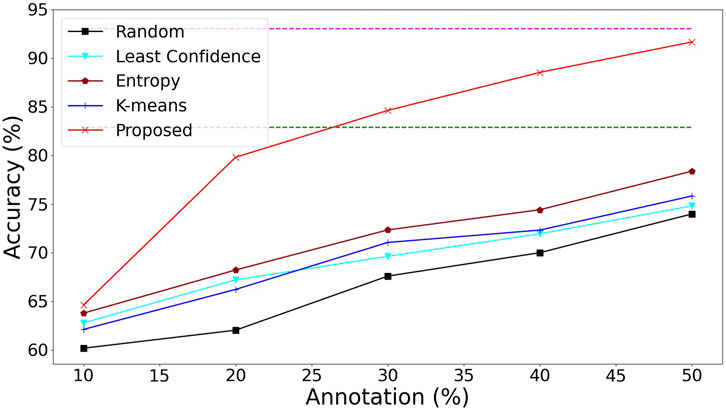
Figure 5. Accuracy of tooth-marked tongue recognition with different query strategies under active learning. The green dashed line represents the performance of ResNet34 (Wang et al., 2020), and the purple dashed line represents the performance of MISVM Li et al. (2018).
As shown in Figure 6, IUL represents the uncertainty of our alignment instances and images, IRL represents that we use the Wasserstein distance to learn the diversity of instances, and EAL represents the introduction of the pseudo-labeling process. Because we have excluded noisy instances by aligning instances to image uncertainty (IUL), the performance of the model is further improved, outperforming direct classification with 100% image-level annotations while requiring only 40% of image-level annotations way of ResNet34 Wang et al. (2020). Our approach (Proposed) outperforms ResNet34’s approach when only 30% image-level annotations are required. In the case where only 50% image-level annotations are required, our method can achieve almost the same performance as the fully annotated MISVM method Li et al. (2018). Combined with the results in Table 1, our model outperforms the MISVM method when it is finally trained to 100% image-level annotation (Table 1), which demonstrates that our method can greatly reduce the annotation cost while maintaining a high level of performance.
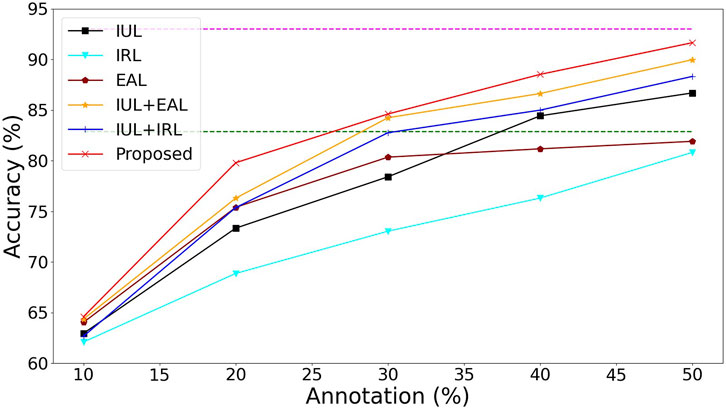
Figure 6. Ablation performance of the proposed model with the accuracy of tooth-marked tongue recognition. The green dashed line represents the performance of ResNet34 (Wang et al., 2020), and the purple dashed line represents the performance of MISVM Li et al. (2018).
Finally, we visualize the selected instances to confirm that our method can improve the accuracy of image recognition through instance information and the effect of weakly supervised localization to assist clinical diagnosis. As shown in Figure 7, our method can reduce the background noise of the instances shown in Figure 8 and highlight the most informative instances by aligning the uncertainty of instances and images and learning from the diversity of instances. A–B and C–D are the images selected by the model in the second and fifth rounds of active learning to request expert annotation, respectively. In particular, as shown in A, the model is not sensitive to large tooth marks, which may be caused by the fact that we do not use multi-scale detection methods like the common one-stage method. In B and D, the model fails to detect the tongue tip. This may be due to the fact that most of the teeth marks on the tongue are located on both sides of the tongue (Zhou et al., 2022). The model identifies the instances in the upper left corner of C and D as informative regions, and these regions were also identified by later experts as earlier annotation errors (as we introduced in Section “Experiment setup and evaluation metric,” we first label all and then simulate the process of requesting experts to label), the difficult areas where these experts will make mistakes are the areas that need the most attention of the model. E and F are the samples selected in the fifth round of the pseudo-labeling process of the model. It can be clearly seen that the model has made more accurate discrimination on the tooth mark area of these images, so the final information of these images is the lowest, and the pseudo-labels are added to enrich the training data.
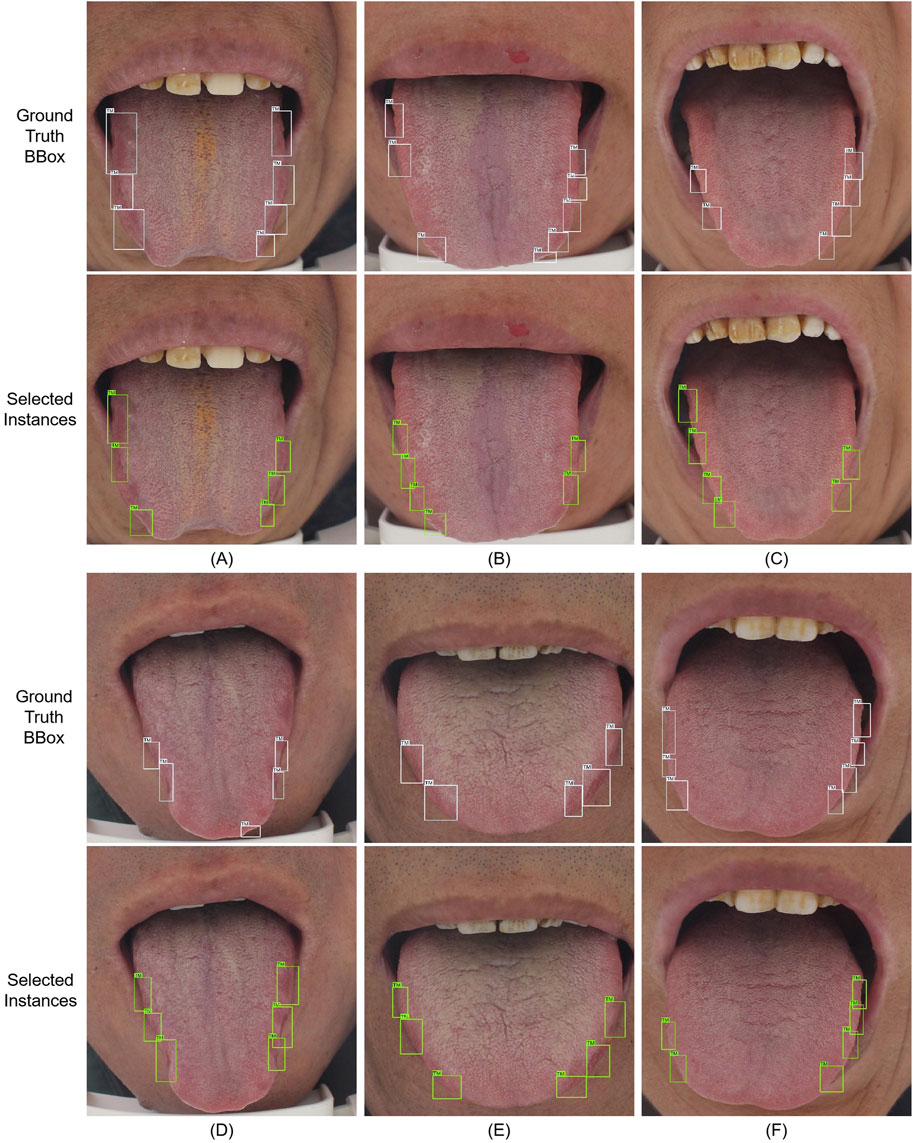
Figure 7. Ground-truth labels of the tooth mark location boxes and examples selected by our method. A–D are the images selected by the model in the second and fifth rounds of active learning to request expert annotation respectively. E, F are the samples selected in the fifth round of pseudo-labeling process of the model.
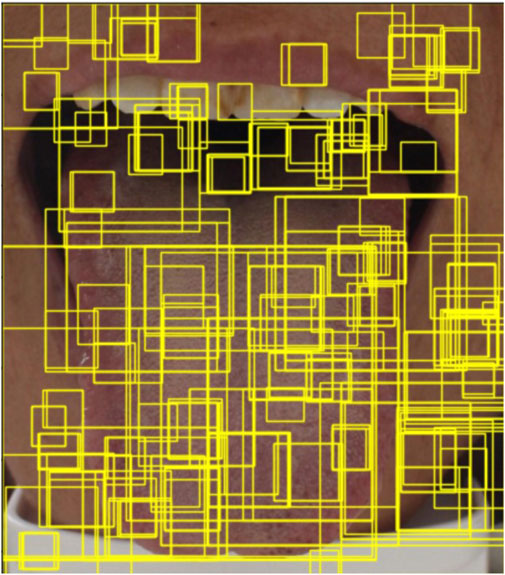
Figure 8. An example generated by the selective search method Van de Sande et al. (2011) based on the common method of object detection. The yellow bounding boxes shown in the figure represent the object proposals generated by the selective search algorithm.
4 Discussion
In this work, we propose an efficient multi-instance active learning model to recognize tooth-marked tongues. This model is based on incomplete and inaccurate supervision within the weakly supervised learning framework. Compared with the previous tooth-marked tongue recognition methods (Li et al., 2018; Wang et al., 2020; Zhou et al., 2022; Weng et al., 2021), the proposed method solves the problem of inaccurate supervision based on multi-instance learning and does not need a large number of instance-level annotation. The proposed method adopts the one-stage detection method and does not need the pixel-level annotation of tongue pre-segmentation. In addition, we build an active learning framework, which is not available in the previous tooth-marked recognition methods. We use the most informative data to request expert annotation so that the model can better represent the tooth mark distribution and reduce the amount of image-level annotation. Specifically, we construct the consistency of instance uncertainty and image uncertainty to reduce the noise instances in tooth mark detection. We further screened the toothmark instances based on the diversity of instances, and were able to extract more representative instances.
For active learning, previous studies have used the uncertainty and diversity of images to select image samples (Wang et al., 2018; Ash et al., 2019), combined the pseudo-tagging technology of semi-supervised learning with active learning (Wang et al., 2017; Zhang et al., 2022), and combined multi-instance target detection with active learning (Yuan et al., 2021). However, the proposed method is different. We used only image-level annotation based on weakly supervised multi-instance learning and the uncertainty and diversity of samples to screen the information of image samples and construct an efficient framework of active learning by using pseudo-labeling technology. Compared with the training model with 100% all-labeled data, the accuracy results of 30%–50% samples are also competitive. Note that this is under the premise of active learning of traditional Chinese medicine data with small samples; 50% of the data of 1,108 cases is actually not much and is difficult to compare with 20% of the data of 7,295 cases of active learning of mainstream medical data (Zhang et al., 2022). Our method of constructing image information based on instance information captures fine-grained information, enabling active learning models to be effective with small datasets.
Although our proposed method is designed to solve the problem of excessive annotation of instances in tooth-marked tongue recognition, it can be applied to many other applications in the field of active detection that require much instance-level annotation, such as active detection of video streams (Zhu et al., 2020) or the field of instance-level feature extraction based on image-level annotation only, such as whole slide image (WSI) classification (Shao et al., 2021). The study reported that one of the challenges of video detection is the inconsistent cognition of labels and the time-consuming and laborious labeling (Zhu et al., 2020). In the future, video detection technology can be based on weak supervision or self-supervision. In addition, a WSI image is generally large and difficult to obtain and, therefore, very difficult to label accurately (Shao et al., 2021). In the case of small instances, our method can reduce the instance-level and image-level annotation and may not significantly decrease the detection and classification performance. In addition, the query strategy based on the uncertainty and diversity of examples can better solve the problem of instance diversity.
There are some studies that need to be improved in this work. First, there is some correlation information between the instance tooth marks of the same tongue. Previous studies (Shao et al., 2021; Schölkopf et al., 2007) have shown that the mining of multi-instance correlation information can further improve detection performance. Therefore, it is possible to improve the accuracy of the model by using the correlation between tooth marks. Then, our method is based on the model of object detection, but most object detection models are based on multi-scale to obtain joint accuracy. In this work, we select the appropriate (shallow) scale to obtain the detection accuracy. Because the tooth mark is generally small on the tongue and accounts for a small proportion of the whole picture, a shallow scale can have good detection performance for small targets. However, the multi-scale method has been demonstrated to have better detection performance in the field of object detection, so we will consider how to further improve the performance based on multi-scale object detection. In addition, we can strengthen the original feature representation through feature reconstruction (Chen et al., 2024). This will enable the model to better capture the key features of tooth-marked tongues. After feature extraction, a voting integration method (Bao et al., 2024) can be employed. By combining the predictions of multiple weakly supervised classifiers through weighted voting, the influence of noisy labels can be mitigated. This process enhances the model’s robustness and accuracy. Furthermore, there are not only tooth marks on the tongue but also cracks, ecchymosis, congestion, and other diseases, and we have not considered these additional symptoms in this work. In future research, we will focus on conducting specialized multi-class, multi-label, and multi-center studies on tongue images.
5 Conclusion
In this study, we proposed a weakly supervised active learning tooth-marked tongue detection model with only a few image-level labels. Experimental results showed that the proposed method is effective with only a small amount of image-level annotation, and its performance is comparable to that of image-level annotation, instance-level annotation, and pixel-level annotation, which require many tooth markers. Our method significantly reduces the annotation cost of the binary classification task of traditional Chinese medicine tooth mark recognition.
Data availability statement
The original contributions presented in the study are included in the article/supplementary material; further inquiries can be directed to the corresponding author.
Ethics statement
The studies involving humans were approved by the Ethics Committee of Dongzhimen Hospital, which is affiliated with the Beijing University of Chinese Medicine. The studies were conducted in accordance with the local legislation and institutional requirements. The participants provided their written informed consent to participate in this study.
Author contributions
FD: formal analysis, methodology, and writing – original draft. SL: writing – review and editing and methodology. ZY: writing – review and editing and data curation. WZ: formal analysis, writing – review and editing, project administration, and conceptualization.
Funding
The author(s) declare that financial support was received for the research and/or publication of this article. This project has received funding from the Platform Project of the Big Data Research Center for Traditional Chinese Medicine at Guangzhou University of Chinese Medicine (No. A1-2601-25-439-127Z102). The second project (without a project number): The “Open Competition” Project of Chinese Medicine Basic Theory Studies (Guangzhou University of Chinese Medicine).
Acknowledgments
The authors highly thank the Dongzhimen Hospital, which is affiliated to Beijing University of Chinese Medicine, for providing tongue images for this research.
Conflict of interest
The authors declare that the research was conducted in the absence of any commercial or financial relationships that could be construed as a potential conflict of interest.
Generative AI statement
The authors declare that no Generative AI was used in the creation of this manuscript.
Publisher’s note
All claims expressed in this article are solely those of the authors and do not necessarily represent those of their affiliated organizations, or those of the publisher, the editors and the reviewers. Any product that may be evaluated in this article, or claim that may be made by its manufacturer, is not guaranteed or endorsed by the publisher.
References
Andrews S., Tsochantaridis I., Hofmann T. (2002). “Support vector machines for multiple-instance learning,” in Advances in Neural Information Processing Systems. NIPS.
Ash J. T., Zhang C., Krishnamurthy A., Langford J., Agarwal A. (2019). Deep batch active learning by diverse, uncertain gradient lower bounds. CoRR abs/1906, 03671. doi:10.48550/arXiv.1906.03671
Bao W., Liu Y., Chen B. (2024). Oral_voting_transfer: classification of oral microorganisms’ function proteins with voting transfer model. Front. Microbiol. 14, 1277121. doi:10.3389/fmicb.2023.1277121
Bilen H., Vedaldi A. (2016). “Weakly supervised deep detection networks,” in 2016 IEEE Conference on Computer Vision and Pattern Recognition (CVPR) (IEEE), 2846–2854. doi:10.1109/CVPR.2016.311
Chen B., Li N., Bao W. (2024). Clpr_in_ml: cleft lip and palate reconstructed features with machine learning. Curr. Bioinforma. 20, 179–193. doi:10.2174/0115748936330499240909082529
Fu J., Zheng H., Mei T. (2017). “Look closer to see better: recurrent attention convolutional neural network for fine-grained image recognition,” in 2017 IEEE Conference on Computer Vision and Pattern Recognition (CVPR) (IEEE), 4476–4484. doi:10.1109/CVPR.2017.476
Girshick R. (2015). Fast r-cnn. IEEE International Conference on Computer Vision ICCV, 1440–1448. doi:10.1109/ICCV.2015.169
Gulrajani I., Ahmed F., Arjovsky M., Dumoulin V., Courville A. C. (2017). Improved training of wasserstein gans. CoRR abs/1704, 00028. doi:10.48550/arXiv.1704.00028
Guo C., Pleiss G., Sun Y., Weinberger K. Q. (2017). “On calibration of modern neural networks,” in Proceedings of the 34th international conference on machine learning. (PMLR), vol. 70 of Proceedings of machine learning research. Editors D. Precup, and Y. W. Teh, 1321–1330.
He K., Zhang X., Ren S., Sun J. (2016). “Deep residual learning for image recognition,” in 2016 IEEE Conference on Computer Vision and Pattern Recognition (CVPR), 770–778. doi:10.1109/CVPR.2016.90
Hsu Y. C., Chen Y. C., Lo L. C., Chiang J. Y. (2011). “Automatic tongue feature extraction,” in 2010 international computer symposium (ICS2010). doi:10.1109/COMPSYM.2010.5685377
Lakshminarayanan B., Pritzel A., Blundell C. (2017). Simple and scalable predictive uncertainty estimation using deep ensembles.
Li X., Zhang Y., Cui Q., Yi X., Zhang Y. (2018). Tooth-marked tongue recognition using multiple instance learning and cnn features. IEEE Trans. Cybern. 49, 380–387. doi:10.1109/TCYB.2017.2772289
Li Y., Fan B., Zhang W., Ding W., Yin J. (2021). Deep active learning for object detection. Inf. Sci. 579, 418–433. doi:10.1016/j.ins.2021.08.019
Lo L. C., Chen Y. F., Chen W. J., Cheng T. L., Chiang J. Y. (2012). The study on the agreement between automatic tongue diagnosis system and traditional Chinese medicine practitioners. Evidence-Based Complementray Altern. Med. 2012, 505063. doi:10.1155/2012/505063
Lu J., Liu M., Chen H. (2023). “Prtmtm: a priori regularization method for tooth-marked tongue classification,” in 2023 IEEE International Symposium on Circuits and Systems (ISCAS), Monterey, CA, USA (IEEE), 1–5. doi:10.1109/ISCAS46773.2023.10181870
Redmon J., Divvala S., Girshick R., Farhadi A. (2016). “You only look once: unified, real-time object detection,” in 2016 IEEE conference on computer vision and pattern recognition (CVPR), 779–788. doi:10.1109/CVPR.2016.91
Redmon J., Farhadi A. (2018). Yolov3: an incremental improvement. CoRR abs/1804.02767. doi:10.48550/arXiv.1804.02767
Schölkopf B., Platt J., Hofmann T. (2007). Multi-instance multi-label learning with application to scene classification, 1609–1616.
Selvaraju R. R., Cogswell M., Das A., Vedantam R., Parikh D., Batra D. (2017). “Grad-cam: visual explanations from deep networks via gradient-based localization,” in 2017 IEEE International Conference on Computer Vision (ICCV), Venice, Italy (IEEE), 618–626. doi:10.1109/ICCV.2017.74
Shao Q., Li X., Fu Z. (2014). “Recognition of teeth-marked tongue based on gradient of concave region,” in 2014 International Conference on Audio, Language and Image Processing, Shanghai, China (IEEE), 968–972. doi:10.1109/ICALIP.2014.7009938
Shao Z., Bian H., Chen Y., Wang Y., Zhang J., Ji X., et al. (2021). Transmil: transformer based correlated multiple instance learning for whole slide image classication. CoRR abs/2106, 00908. doi:10.48550/arXiv.2106.00908
Sun Y., Dai S., Li J., Zhang Y., Li X. (2019). Tooth-marked tongue recognition using gradient-weighted class activation maps. Future Internet 11, 45. doi:10.3390/fi11020045
Tan W., Chang D., Li J., He D. (2023). “Tooth-marked tongue recognition based on wavelet transform and feature fusion,” in 2023 IEEE 6th Information Technology,Networking,Electronic and Automation Control Conference, Chongqing, China (IEEE), 6, 1274–1280. doi:10.1109/ITNEC56291.2023.10082166
Van de Sande K. E., Uijlings J. R., Gevers T., Smeulders A. W. (2011). “Segmentation as selective search for object recognition,” in 2011 international conference on computer vision (IEEE), Barcelona, Spain (IEEE), 1879–1886. doi:10.1007/s11263-013-0620-5
Villani C. (2009). Optimal transport: Old and new. grundlehren der mathema-tischen wissenschaften. Springer.
Wang K., Zhang D., Li Y., Zhang R., Lin L. (2017). Cost-effective active learning for deep image classification. IEEE Trans. Circuits Syst. Video Technol. 27, 2591–2600. doi:10.1109/TCSVT.2016.2589879
Wang M., Fu K., Min F. (2018). “Active learning through two-stage clustering,” in 2018 IEEE International Conference on Fuzzy Systems, Rio de Janeiro, Brazil (FUZZ-IEEE), 1–7. doi:10.1109/FUZZ-IEEE.2018.8491674
Wang X., Liu J., Wu C., Liu J., Chen J., Chen Y., et al. (2020). Artificial intelligence in tongue diagnosis: using deep convolutional neural network for recognizing unhealthy tongue with tooth-mark. Comput. Struct. Biotechnol. J. 18, 973–980. doi:10.1016/j.csbj.2020.04.002
Wei C., Sohn K., Mellina C., Yuille A., Yang F. (2021). “Crest: a class-rebalancing self-training framework for imbalanced semi-supervised learning,” in 2021 IEEE/CVF conference on computer vision and pattern recognition (CVPR), 10852–10861. doi:10.1109/CVPR46437.2021.01071
Weng H., Li L., Lei H., Luo Z., Li C., Li S. (2021). A weakly supervised tooth-mark and crack detection method in tongue image. Concurrency Comput. Pract. Exp. 33. doi:10.1002/cpe.6262
Xiao H., Herman M., Wagner J., Ziesche S., Etesami J., Linh T. H. (2019). Wasserstein adversarial imitation learning. CoRR abs/1906.08113. doi:10.48550/arXiv.1906.08113
Yuan T., Wan F., Fu M., Liu J., Xu S., Ji X., et al. (2021). “Multiple instance active learning for object detection,” in 2021 IEEE/CVF conference on computer vision and pattern recognition (CVPR), 5326–5335. doi:10.1109/CVPR46437.2021.00529
Zhang M., Wang Y., Ma X., Xia L., Yang J., Li Z., et al. (2020). “Wasserstein distance guided adversarial imitation learning with reward shape exploration,” in 2020 IEEE 9th Data Driven Control and Learning Systems Conference (DDCLS), Liuzhou, China (IEEE), 1165–1170. doi:10.1109/DDCLS49620.2020.9275169
Zhang W., Zhu L., Hallinan J., Zhang S., Makmur A., Cai Q., et al. (2022). “Boostmis: boosting medical image semi-supervised learning with adaptive pseudo labeling and informative active annotation,” in Proceedings of the IEEE/CVF conference on computer vision and pattern recognition.
Zhou J., Li S., Wang X., Yang Z., Hou X., Lai W., et al. (2022). Weakly supervised deep learning for tooth-marked tongue recognition. Front. Physiology 13, 847267. doi:10.3389/fphys.2022.847267
Zhou Z.-H. (2017). A brief introduction to weakly supervised learning. Natl. Sci. Rev. 5, 44–53. doi:10.1093/nsr/nwx106
Zhu Y., Li X., Liu C., Zolfaghari M., Xiong Y., Wu C., et al. (2020). A comprehensive study of deep video action recognition. CoRR abs/2012, 06567. doi:10.48550/arXiv.2012.06567
Zitnick C. L., Dollár P. (2014). “Edge boxes: locating object proposals from edges,” in Computer vision – eccv 2014. Editors D. Fleet, T. Pajdla, B. Schiele, and T. Tuytelaars (Cham: Springer International Publishing), 391–405.
Keywords: tooth-marked tongue, weakly supervised learning, multiple instance learning, active learning, pseudo label
Citation: Deng F, Li S, Yang Z and Zhou W (2025) Weakly supervised multiple-instance active learning for tooth-marked tongue recognition. Front. Physiol. 16:1598850. doi: 10.3389/fphys.2025.1598850
Received: 24 March 2025; Accepted: 05 May 2025;
Published: 11 June 2025.
Edited by:
Xi Wang, The Chinese University of Hong Kong, ChinaReviewed by:
Wenzheng Bao, Xuzhou University of Technology, ChinaHao Liang, Hunan University of Chinese Medicine, China
Copyright © 2025 Deng, Li, Yang and Zhou. This is an open-access article distributed under the terms of the Creative Commons Attribution License (CC BY). The use, distribution or reproduction in other forums is permitted, provided the original author(s) and the copyright owner(s) are credited and that the original publication in this journal is cited, in accordance with accepted academic practice. No use, distribution or reproduction is permitted which does not comply with these terms.
*Correspondence: Wu Zhou, emhvdXd1QGd6dWNtLmVkdS5jbg==
† These authors have contributed equally to this work and share first authorship