- 1Univ. Lille, CNRS, UMR 8518 - LOA - Laboratoire d’Optique Atmosphérique, Lille, France
- 2NASA Langley Research Center, Hampton, VA, United States
- 3School of Meteorology, The University of Oklahoma, Norman, OK, United States
- 4School of Physics and Astronomy, University of Leicester, Leicester, United Kingdom
- 5National Centre for Earth Observation, University of Leicester, Leicester, United Kingdom
- 6SRON Netherlands Institute for Space Research, Utrecht, Netherlands
- 7Aerospace Information Research Institute, Chinese Academy of Sciences, Beijing, China
Introduction
The past five decades have witnessed satellite remote sensing become one of most efficient tools for surveying the Earth at local, regional, and global spatial scales. These space-based observations have a non-destructive character that allows for rapid monitoring of the ambient atmosphere, its underlying surface, and the ocean mixed layer. Moreover, satellite instruments can observe toxic or hazardous environments without putting people or equipment at risk. Large-scale continuous satellite observations complement detailed (but sparse) field observations and provide measurements of unsurpassed volume and content for theoretical modeling and data assimilation.
There are a large number of highly important applications that currently rely upon data from satellites. Observations of the atmosphere are used for weather prediction, monitoring of environmental pollution, climatic change, etc. (Wielicki et al., 1996). Remote sensing of ocean surfaces is used for monitoring of coastline dynamics, sea surface temperature and salinity, ocean ecosystem and carbon biomass, sea level change, marine traffic and fisheries, mapping of water current and underlying topography in shallow waters, etc. (Fu et al., 2019). The remote sensing of the land from satellite greatly contributes to the exploration of mineral resources (Zhang et al., 2017), the monitoring of floods and droughts (Jeyaseelan, 2004), soil moisture (Lakshmi, 2013; Babaeian et al., 2019), vegetation (Xie et al., 2008), deforestation (Gao et al., 2020), forest fires (Lentile et al., 2006), agricultural monitoring (Atzberger, 2013), urban planning (Kadhim et al., 2016), etc. Finally, social science efforts to survey global crises (such as the COVID-19 pandemic) are benefitting from satellite remote sensing datasets that utilize diverse targeted visualization to classify human environments, and then relate these observations to various socioeconomic data sets, etc. (Diffenbaugh et al., 2020). Moreover, satellite remote sensing has provided an effective tool for gathering global information such as 1) planetary topography; 2) atmospheric profiles of the temperature, water vapor, carbon dioxide and other trace gases; 3) mineral and chemical compositions of surface and atmosphere, and 4) properties of cryosphere such as snow, sea ice, glacier, and melting ponds, and 5) particle and electromagnetic properties of the thermosphere, ionosphere and magnetosphere. Remote sensing of the Earth also advances the technological state of the art, and this assists the development of deep space remote sensing missions such as Voyager (Kohlhase and Penzo, 1977) and the Cassini-Huygens space-research mission (Matson et al., 2002).
In the early stages of observational satellite development, the design of satellite sensors was often highly target-specific. For example, a series of instruments were launched in the 1970s: Landsat and the Advanced Very High Resolution Radiometer(AVHRR) instruments targeted the monitoring of land surfaces and clouds, the Total Ozone Mapping Spectrometer (TOMS) instruments focused on observing total column ozone, and the High-resolution Infrared Radiation Sounder (HIRS) instruments supported weather forecasting and climate monitoring. The deployment of those missions provided unique data for each targeted subject, and the missions were praized by the corresponding scientific communities. Those missions were extended for many years to obtain climatologically significant data records. The renewed missions were constantly improved (both technically and logistically) based upon the accumulated experience.
The promising results of those missions encouraged the design and launch of increasingly advanced instruments with broader observational scopes in the two decade period from ∼1990 to ∼2010. For example, the Measurement of Pollution in the Troposphere (MOPITT), the Orbiting Carbon Observatory (OCO), and the Greenhouse gases Observing Satellite (GOSAT) missions were deployed for advanced monitoring of greenhouse gases such as carbon dioxide (CO2) and methane (CH4). Several thermal enhanced infrared sounders such as AIRS, TES, IASI, IMG, and CRIS were launched to monitor the atmospheric state for weather prediction and climate change (see list of instrument acronyms at the end of this article). Other satellite imagers were deployed for observing air, land, and ocean and to support inter-disciplinary studies, such as single-viewing MODIS, MERIS, and SGLI, bi-viewing ATSR and AATSR, multi-viewing MISR radiometers and POLDER polarimeters (King et al., 1999; Thies, and Bendix, 2011; Neukermans, et al., 2018). In addition to these more traditional passive observations, active measurements like the CloudSat radar and the CALIPSO lidar were deployed for monitoring the vertical structure of clouds and aerosols that are important for diverse atmospheric applications (Stephens, et al., 2002; Winker, et al., 2009).
All of these previous efforts provided valuable insight that helped to build the understanding regarding the real value, limitations, and potential of satellite remote sensing. Indeed, the resilient development of space instrument technologies and a boom in informatics has created an unprecedented situation where the limitations in hardware, data acquisition, and processing have drastically weakened, and significantly more advanced designs of satellite sensors can be developed and deployed. Additionally, the scientific community has already obtained very sizable amounts of satellite data, accumulated substantial experience in managing and analyzing the data, has a genuinely realistic vision of possible achievements with the existing satellite dataset, as well as knowledge of the steps that are necessary for improving the usefulness of satellite data in the future.
On the other hand, the community also realizes that the fundamental challenges of remote observations never end. For instance, separating signal from noise to retrieve a particular set of geophysical variables and accurate instrument calibration are ongoing challenges. Technological advances improve the information content of the observations, but the data is never fully sufficient to uniquely characterize all of the geophysical parameters of interest; the list of desired observables grows continuously with the advancement of science. Thus, remote sensing remains a fundamentally ill-posed problem that needs to be appropriately defined and constrained by theoretical models, a priori knowledge, and ancillary observations. These are important considerations when designing new scientific objectives.
Grand Challenges
The overall grand challenge in the advancement of satellite remote sensing is discovering innovative and affordable technologies and measurement concepts to solve new problems, and dealing with issues that have come to light after the satellite remote sensing experiments of the previous half century. Specifically, several complimentary aspects are expected to be addressed:
Increased Spatial and Temporal Coverage and Resolution of Satellite Observations
One of the major advantages of the satellite remote sensing is the capability to quickly observe large regions of the Earth. At the same time, coverage limitations of currently available satellite data are also evident (an overview of satellite coverage capabilities is discussed by Kim et al., 2020). For example, polar orbiting imagers in Low Earth Orbit (LEO) generally achieve global coverage in minimum of one day (but mostly two or more days), so many natural phenomena that have higher temporal and spatial variability are not fully captured. In this regard, high-orbit geostationary observations (GEO) address this limitation by providing frequent diurnal observation of the same object. However, there is a trade-off between spatial coverage and the resolution of satellite images (usually higher coverage results in lower spatial resolution). Achieving observations with both extensive spatial-temporal coverage and high spatial resolution is desirable for many applications, but also highly challenging. Hence, the design of satellite observations may need new innovations, ancillary data, and synergies of complimentary observations to address specific objects and relevant problems. This is discussed further in the next section.
Increased Information Content and Exploring Synergy of Observations
Although the high capabilities of satellite observations have been clearly documented, the current data provided by our satellite instruments have limited information content for many applications. Therefore, the deployment of new sensors with enhanced capabilities is desirable and planned.
For example, it has been clearly recognized that Multi-Angular Polarimeters (MAPs) provide the most appropriate data for characterizing detailed columnar properties of atmospheric aerosol and cloud (Mishchenko and Travis, 1997; Hasekamp and Landgraf, 2007; Lebsock et al., 2007; Dubovik et al., 2011; Wu et al., 2015; Xu et al., 2018; Stamnes et al., 2018, etc.), so the focus on polarimetric data in aerosol and cloud characterization is expected to significantly increase in next decade (Dubovik et al., 2019). Several advanced polarimetric missions are planned to be launched in coming few years by European and US space agencies including 3MI (Multi-View Multi-Channel Multi-Polarization Imaging mission) on MetOp-SG satellite (Fougnie, et al., 2018), Multi-Angle Imager for Aerosols (MAIA) instrument (Diner et al., 2018), Spex (Spectropolarimeter for Planetary Exploration) and Hyper-Angular Rainbow Polarimeter (HARP) as part of NASA PACE mission (Werdell et al., 2019), Multi Spectral Imaging Polarimeter (MSIP)/Aerosol-UA (Milinevsky, et al., 2019), MAP instruments as part of Copernicus CO2M Mission (European Space Agency, 2020; Janssens-Maenhout, et al., 2020; Dubovik et al., 2019), etc. Also, the China National Space Administration (CNSA) has significantly invested in polarimetric sensors. CNSA has recently launched several polarimetric remote sensing instruments including the MAI/TG-2, CAPI/TanSat, DPC/GF-5 and SMAC/GFDM and plans the launches of POSP, PCF, DPC-Lidar in coming years (Dubovik et al., 2019). The concepts of these instruments, their technical designs, and the algorithm development have been intensively discussed and tested using airborne prototypes as discussed in details by Duibovik et al. (2019).
Similarly, the number of satellite-based lidars and radars is expected to increase, since active remote sensing instruments provide detailed information about vertical variability of the atmosphere (Winker et al., 1996; Weitkamp, 2005; Chand et al., 2008). Indeed, most of the major space agencies are pursuing space-based lidar programs. For example, NASA launched the Geoscience Laser Altimeter System (GLAS) onboard the ICESat satellite in 2003 (Kwok et al., 2004), the Cloud-Aerosol Lidar with Orthogonal Polarization (CALIOP) onboard the CALIPSO satellite in 2006 (Winker et al., 2007), the Cloud-Aerosol Transport System (CATS) onboard the ISS in 2015 (Yorks et al., 2016), and the Advanced Topographic Laser Altimeter System (ATLAS) onboard ICESat-2 in 2018 (Neumann et al., 2019). Additionally, the ESA launched the Aladin wind lidar onboard the Aeolus satellite as part of the Living Planet Program (LPP) in 2018 (Källén, 2008), the CNSA will launch the DPC-Lidar onboard CM-1 satellite in 2021 (Dubovik et al., 2019), and the joint European/Japanese EarthCARE satellite (projected to launch in 2023) will include high-performance lidar and radar technology that has never been flown in space before (Illingsworth et al., 2015). The success and promise of these missions make lidars an essential component of future observing systems (National Academies of Sciences, Engineering, and Medicine, 2015).
At the same time, no single sensor provides comprehensive information about a targeted object in a complex environment, so there is a need to explore synergies of complementary observations. For example, even the most advanced multi-angular polarimeter cannot assure reliable 3D characterization of aerosols due to limited sensitivity to vertical variability of aerosol and clouds. The value of coordinated passive and active observations has been clearly recognized and accounted for in planning satellite missions as illustrated by Figure 1. For example, the A-Train satellite constellation provides polarimetric, radiometric, lidar and other complementary data (L’Ecuyer and Jiang, 2010). Similarly, the ongoing NASA Aerosol and Cloud, Convection and Precipitation (ACCP) study considers deployment of coordinated observations by passive (polarimeter, spectrometer, microwave radiometer) and active (lidar and radar) sensors (https://science.nasa.gov/earth-science/decadal-accp), National Academies of Sciences, Engineering, and Medicine, 2018.
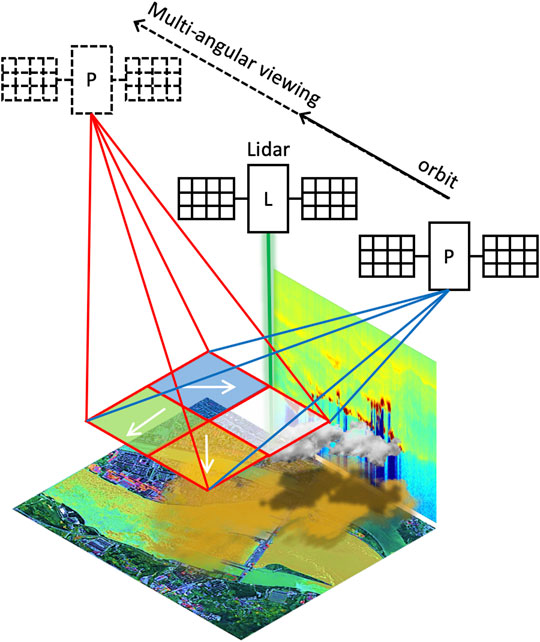
FIGURE 1. The lidar and MAP instrument combined observations to provide 3D characterization of the atmosphere.
Also, the next generation of retrievals (discussed further in the next section) intend to explore synergistic retrievals that rely upon observations from different instruments (e.g., Dubovik et al., 2011; Dubovik et al., 2014; Knobelspiesse, et al., 2011; Knobelspiesse and Nag, 2018). For example, the main challenge in retrieving properties of atmospheric aerosols is distinguishing light scattered by aerosol particles from light scattering by clouds, atmospheric gases, and the underlying surface. Satellite sensors such as the MODIS (radiometer) or POLDER (polarimeter) that are designed for aerosol monitoring may not have optimum capabilities for removing cloud, gas and surface contaminations (Sayer et al., 2020). Similarly, land reflectance observations usually need to remove scattering from atmospheric aerosols and gases; likewise, atmospheric gas monitoring may suffer from aerosol and cloud contamination. Thus, observations of multiple instruments with different sensitivities to clouds, aerosols and gases are always desirable. The analysis of aerosol measurements that are contaminated by clouds and trace gases over inhomogeneous surfaces may benefit from synergistic measurements from multiple instruments. IR imagery, lidar, and radar observations can be used to constrain cloud fractions, spectrometric data offers high sensitivity to gaseous concentrations, and high resolution imagery help reduce uncertainties associated with land surface heterogeneity.
For example, simultaneous multi-angular polarimetric observations by MAP/CO2M and DPC deployed in the framework of CO2M EU/Copernicus and GF-5 Chinese missions are expected to provide information for atmospheric correction and greenhouse gas monitoring (European Space Agency, 2020; Janssens-Maenhout, et al., 2020, Dubovik et al., 2019). Indeed, CO2 and other gases obtained by such instruments as OCO and GOSAT are provided only in conditions with very minor presence of atmospheric aerosol (e.g., Crisp et al., 2012). In this regard, the addition of MAP observations in the Copernicus CO2M mission is expected to improve the Greenhouse gases characterization in situations with moderate and possibly high presence of aerosols. As part of the NASA PACE mission, the SPEX and the HARP polarimetry instruments are expected to complement hyperspectral radiance data from the OCI and thereby provide more accurate aerosol information helpful for retrieving ocean surface and subsurface properties (Frouin et al., 2019; Remer et al., 2019a; Remer et al., 2019b; Werdell et al., 2019). Another example of a satellite combining polarization measurements with high-resolution spectroscopy is the high-resolution and multi-mode integrated imaging satellite (Gao Fen Duo Mo, or GFDM, Dubovik et al., 2019) successfully launched by the CNSA on July 3, 2020.
Finally, synergetic use of satellite data with surface and suborbital targeted measurements are also an important consideration. For example, the landscapes and surface properties in dense urban centers tend to be highly heterogeneous. Thus, the atmospheric processes and dynamics are affected by highly variable local activities, and observations of environmental air quality need to have high temporal frequency and high spatial resolution. Constellations of small and less expensive so called nano- or cube-satellites with various equator crossing times could improve coverage with increased numbers of orbiting instruments (Knobelspiesse and Nag, 2018). Additionally, surface measurements and routine sub-orbital measurements could also enhance satellite retrievals in populated areas (Kahn et al., 2017). For example, Liu et al. (2004) and Liu et al. (2007) suggested a PM monitoring technique that uses geostatistical regression of PM concentration from ground measurements and collocated satellite observations. The regression is assisted by use of a chemical transport model to fill temporal and/or spatial gaps when the measurements are unavailable or contaminated. This approach is being employed by the MAIA project to speciate aerosol components such as sulfate, nitrate, ammonium, black carbon, organic carbon, and dust (Diner et al., 2018). The spatial PM information are then further combined with health records to better understand the relationship between aerosol pollutants and adverse public health problems (Anderson et al., 2012).
Addressing the field-of-view difference in combining observations from different instruments is another challenge. In this regard, the accumulated experience in using the data from multiple instruments with different field-of-view deployed within A-Train constellation provided valuable insights for future missions (Chahine et al., 2006; Delanoë and Hogan 2010; Kato et al., 2010, Kato et al., 2011; Ceccaldi et al., 2013; Henderson et al., 2013; Mace and Zhang 2014).
Development of the State-of-Art Data Processing Approaches of the Next Generation
The quality of a remote-sensing retrieval algorithm is another critical aspect that affects the quality of the final product. In fact, once the instrument has been deployed, the quality of the resulting observational data cannot be radically improved, while retrieval algorithms remain under constant improvement. The final remote sensing product can be notably different not only due to ingesting data from different instruments, but also due to improvements of retrieval concepts. In this regard, the new generation of remote sensing retrieval algorithms have advanced significantly in the past decade. For example, new algorithms tend to rely upon fast and accurate atmospheric modeling (instead of using precomputed Look-Up-Tables, or LUT) and are capable of retrieving a large number of parameters. Additionally, simultaneous retrieval of aerosol properties together with land surface properties and/or cloud properties have been implemented (Hasekamp and Landgraf, 2005; Hasekamp and Landgraf, 2007; Hasekamp, 2010; Dubovik et al., 2011, 2014; Waquet et al., 2013; Xu et al., 2016; Xu et al., 2017; Li et al., 2018, etc.). Finally, within the framework of the CO2M EU/Copernicus discussed above, the joint retrieval of CO2 and aerosol properties is a promising approach for reducing the effect of aerosol contamination on the derived CO2 product.
We provided several examples of synergistic instruments in Grand Challenge #2 above. The preparation of these missions includes developments of adequate algorithms that are capable of analyzing multi-instrument measurements. At the same time the fundamental logic of the retrieval algorithm evolution itself suggests a high potential of using synergy of different observations for improving the accuracy of the retrievals (e.g., Knobelspiesse, et al., 2011; Dubovik et al., 2019). Moreover the idea of the development versatile instrument independent algorithm that can be applied to different observations or their combination becomes increasingly popular. Generalized Retrieval of Aerosol and Surface Properties (GRASP) (Dubovik et al., 2011; Dubovik et al., 2014) is an example of such algorithm. This algorithm can be used for diverse satellite and ground based passive and active measurement. It has also been successfully applied for simultaneous synergetic inversion of lidar profiles and columnar radiometric observations (e.g., Lopatin et al., 2013; Lopatin et al., 2020; Román et al., 2018).
Some algorithm challenges still remain for accurate satellite remote sensing of clouds. An accurate and efficient radiative transfer model is a prerequisite. While independent column approximation is widely adopted for retrieving cloud droplet size and optical depth, 3-D radiative transfer (RT) effects caused by cloud horizontal inhomogeneity (cloud top roughness) can be a reason for retrieval biases (e.g., Davis et al., 1997; Várnai and Marshak, 2001; Zhang et al., 2012; Liang and Di Girolama, 2013). The 3-D nature of clouds becomes more of a concern for investigating the interactions between clouds and aerosols (e.g., at cloud edges), starting from coupling their retrieval into a joint framework. Within this context, development of inversion-targeted fast yet accurate 3D RT model for geometrically and optically complex media, with incorporation of spectral signature of gas absorption and proper adoption of cloud particle scattering model is a pressing need. Development of reliable 3D radiative models is also needed for accounting horizontal heterogeneity of land surface for adequate interpretation of all satellite imagery (Evans, 1998; Evans and Stephens, 1991; Lyapustin, 2002; Lyapustin and Wang, 2005). Another relevant outstanding issue is constructing 3D cloud fields to model 3D radiation fields, which could potentially be addressed using a combination of active and passive sensors (Barker et al., 2011).
Many modeling and observational studies have demonstrated the significant role of cirrus clouds in contributing to weather and climate processes (e.g., Liou, 2002). Although optically thin (Sassen and Cho, 1992), cirrus clouds have global presence and regulate the Earth’s radiation and play an important role in the climate system. Cirrus particles have highly irregular shapes and their single-scattering properties such as single scattering albedo and scattering phase functions are significantly different from spherical particles (Yang et al., 2002; Yang et al., 2015). If an algorithm does not identify these irregular shapes, they can cause substantial biases in aerosol and cloud retrievals (Chew et al., 2011). Therefore, the identification of a representative cirrus particle model and including it in aerosol retrievals is a highly promising direction.
Also, the use of satellite data is strongly aligned with progress in global climate and Chemical Transport Models (CTM). For example, reliable aerosol retrievals can be assimilated into Chemical Transport Models (CTMs) to provide accurate aerosol loadings when observations are not available (e.g., Collins et al., 2001; Randles et al., 2017). At the same time, spectral and polarimetric information has substantial sensitivity to constrain aerosol type (Li et al., 2019) and satellite data may provide additional constraints for improving global emissions of the atmospheric components in chemical transport models (e.g., Chen et al., 2018; Chen et al., 2019; Elguindi et al., 2020). Therefore, synergizing satellite data processing with available modeled information is another highly promising research field for advancing satellite remote sensing.
Finally, at present, machine learning approaches are increasingly used to extract patterns and insights from the remote sensing and geospatial data (Lary et al., 2016). This branch of artificial intelligence is highly suitable and attractive for analysis and interpretation of Earth observation data because it proposes the methods that can “learn” from data, identify patterns, and make decisions with minimal human intervention. In particular, the emerging techniques of deep learning and deep neural networks have recently been used in remote sensing studies, especially for processing and analysis of huge amounts of data. Such techniques reveal the potential to extract spatio-temporal relations automatically and gain further knowledge helpful for improving forecasting and modeling of observed physical phenomena at multiple timescales. These methods are highly appealing for analysis of satellite data, especially if the versatility of data-driven machine learning is coupled with physical process models (e.g., Reichstein et al., 2019).
Achieving Continuity in Consistent Satellite Observations and Long-Term Data Record
A long-term and high-quality record of essential climate variables is critical for monitoring and studying the Earth’s Climate change. A necessary condition for achieving this goal is the continuity of the observations, which can only be assured when high quality data collection continues without breach. Otherwise, gaps in a multi-instrument data record cannot be properly interpreted, and the value of the satellite record nearly vanishes.
Thus, the absolute calibration of each instrument and the intercalibration of multiple pertinent sensors remains critical for success in nearly all objectives of satellite remote sensing (Wielicki et al., 2013). Calibrating many instruments is challenging, especially for constellations of small satellites. The requirements for maintaining long-term observations were highlighted by National Academies of Sciences, Engineering, and Medicine (2015); the CLARREO (Climate Absolute Radiance and Refractivity Observatory) mission is a first attempt to define a satellite mission dedicated toward this goal (Lukashin et al., 2017). Similar to the calibration of direct observations, the traceability and consistency of the next generation of satellite products to the present-day instrument suite is critical.
Conclusion
Thus, after more than a half of century past the launch of first satellite, the remote sensing of the Planet Earth from space has evolved into a highly elaborate tool that fuels fundamental science and supports daily activities vital for humanity. A very large number of satellite instruments have been developed and launched, and they have supplied a huge amount of data for diverse requirements. The number of satellite instruments and the quality and scope of the information collected by satellites is constantly improving. At the same time, the accumulated experience of the satellite remote sensing community reveals the challenges that need to be addressed for future development. Although each observation of the atmosphere, land, or ocean surface (and other fields of remote sensing) may have specific and quite different issues, several conceptual challenges are common for many satellite remote sensing disciplines. Specifically, an increase in value of the data and efficiency of satellite remote sensing approaches will likely be related to successes in:
• increasing both coverage and resolution in spatial and temporal records of observations;
• increasing information content of observations by deploying the satellite instruments with enhanced capabilities and exploring synergies of complimentary observations, e.g., synergy of passive imagery with active vertical profiling of the atmosphere and hyperspectral spectrometry, combining observations of different sensitivities obtained in different spectral ranges, or at different time or spatial scales, as well as, combining satellite observations with sub-orbital observations and chemical transport model results;
• development of the next generation state-of-art data processing approaches that rely on rigorous forward modeling and numerical inversion methodologies, considers extensive sets of state parameters (e.g., joint retrieval of parameters characterizing different atmospheric components such as gases, aerosols, clouds and underlying surface), and use new solution techniques such as deep learning and neural networks;
• achieving continuity in consistent satellite observations and long-term data sets by assuring sufficient compatibility and agreement of past, present and future data sets that are required for accumulating climate records.
Author Contributions
OD - concept of the paper, elaboration of strategy, contribution with overview of aerosol remote sensing and retrieval algorithms; GS - elaboration of strategy, contribution with overview of aerosol remote sensing, overall remote sensing overview, polishing of the paper style; FX - elaboration of strategy, contribution with overview of aerosol remote sensing and retrieval algorithms; YH - overall remote sensing overview, HB - elaboration of strategy, contribution with overview of transport modeling and trace gases remote sensing JL -elaboration of strategy, contribution with overview of trace gases remote sensing and trace gases retrieval algorithms; ZL - elaboration of strategy, contribution with overview of aerosol remote sensing.
Conflict of Interest
The authors declare that the research was conducted in the absence of any commercial or financial relationships that could be construed as a potential conflict of interest.
The handling editor declared a shared affiliation with two of the authors, GS and YH, at time of review.
Acknowledgments
The authors thank Anton Lopatin for the help with the preparation of the illustration. OD appreciates support from the Chemical and Physical Properties of the Atmosphere Project funded by the French National Research Agency through the Programme d’Investissement d’Avenir under contract ANR-11-LABX-0 0 05-01, the Regional Council “Hauts-de-France,” and the “European Funds for Regional Economic Development.”
Glossary
AATSR advanced along-track scanning radiometer
ACCP aerosol and cloud, convection and precipitation
AIRS atmospheric infrared sounder
ATSR along track scanning radiometer
AVHRR advanced very high resolution radiometer
CALIPSO cloud-aerosol lidar and infrared pathfinder satellite observations
CAPI cloud and aerosol polarization imager
CLARREO climate absolute radiance and refractivity observatory
CM-1 carbon monitoring satellite-1
CNSA China national space administration
CO2M copernicus anthropogenic carbon dioxide monitoring
CRIS cross-track infrared sounder
CTM chemical transport models
DPC directional polarimetric camera
ESA European space agency
EarthCARE Earth clouds, aerosols and radiation explorer
GEO geosynchronous equatorial orbit
GFDM gao Fen Duo Mo satellite
GOSAT greenhouse gases observing satellite
GRASP generalized retrieval of aerosol and surface properties
HARP hyper-angular rainbow polarimeter
HIRS high-resolution infrared radiation sounder
IASI infrared atmospheric sounding interferometer
IMG interferometric monitor for greenhouse gases
LEO low earth orbit
LUT look-up-tables
LPP living planet programme
MAI multi-angle polarization imager
MAIA multi-angle imager for aerosols
MAP multiple-angle-polarimeter
MERIS medium resolution imaging spectrometer
MSIP multi spectral imaging polarimeter
MISR multi-angle imaging spectroradiometer
MODIS moderate resolution imaging spectroradiometer
MOPITT measurement of pollution in the troposphere
NASA National aeronautics and space administration
OCI ocean color instrument
OCO orbiting carbon observatory
PACE plankton aerosol-cloud-ocean ecosystems
PCF polarization CrossFire suite
PM particulate matters
POLDER polarization and directionality of the Earth’s reflectances
POSP particulate observing scanning polarimeter
RT radiative transfer
SGLI second generation global imager
SMAC synchronization monitoring atmospheric corrector
SPEX spectropolarimeter for planetary exploration
TanSat carbon observing satellite
TES tropospheric emission spectrometer
TG tiangong spacecraft
TOMS total ozone mapping spectrometer
References
Anderson, J. O., Thundiyil, J. G., and Stolbach, A. (2012). Clearing the air: a review of the effects of particulate matter air pollution on human health. J. Med. Toxicol. 8, 166–175. doi:10.1007/s13181-011-0203-1
Atzberger, C. (2013). Advances in remote sensing of agriculture: context description, existing operational monitoring systems and major information needs. Rem. Sens. 5, 949–981. doi:10.3390/rs5020949
Babaeian, E., Sadeghi, M., Jones, S. B., Montzka, C., Vereecken, H., and Tuller, M. (2019). Ground, proximal, and satellite remote sensing of soil moisture. Rev. Geophys. 57, 530–616. doi:10.1029/2018RG000618
Barker, H. W., Jerg, M. P., Wehr, T., Kato, S., Donovan, D. P., and Hogan, R. J. (2011). A 3D cloud-construction algorithm for the EarthCARE satellite mission. Q. J. R. Meteorol. Soc. 137, 1042–1058. doi:10.1002/qj.824
Ceccaldi, M., Delanoë, J., Hogan, R. J., Pounder, N. L., Protat, A., and Pelon, J. (2013). From CloudSat-CALIPSO to EarthCare: evolution of the DARDAR cloud classification and its comparison to airborne radar-lidar observations. J. Geophys. Res. Atmos. 118, 7962–7981. doi:10.1002/jgrd.50579
Chahine, M. T., Pagano, T. S., Aumann, H. H., Atlas, R., Barnet, C., Blaisdell, J., et al. (2006). Airs. Bull. Am. Meteorol. Soc. 87 (7), 911–926. doi:10.1175/bams-87-7-911
Chand, D., Anderson, T., Wood, R., Charlson, R., Hu, Y., Liu, Z., et al. (2008). Quantifying above-cloud aerosol using spaceborne lidar for improved understanding of cloudy-sky direct climate forcing. J. Geophys. Res. 113, D13206. doi:10.1029/2007JD009433
Chen, C., Dubovik, O., Henze, D. K., Lapyonak, T., Chin, M., Ducos, F., et al. (2018). Retrieval of desert dust and Carbonaceous aerosol emissions over Africa from POLDER/PARASOL products generated by GRASP algorithm. Atmos. Chem. Phys. 18, 12551–12580. doi:10.5194/acp-18-12551-2018
Chen, C., Dubovik, O., Henze, D. K., Chin, M., Lapyonok, T., Schuster, G. L., et al. (2019). Constraining global aerosol emissions using POLDER/PARASOL satellite remote sensing observations. Atmos. Chem. Phys., 19, 14585–14606. doi:10.5194/acp-19-14585-2019
Chew, B. N., Campbell, J. R., Reid, J. S., Giles, D. M., Welton, E. J., Salinas, S. V., et al. (2011). Tropical cirrus cloud contamination in sun photometer data. Atmos. Environ. 45, 6724–6731. doi:10.1016/j.atmosenv.2011.08.017
Collins, W. D., Rasch, P. J., Eaton, B. E., Khattatov, B. V., Lamarque, J., and Zender, C. S. (2001). Simulating aerosols using a chemical transport model with assimilation of satellite aerosol retrievals: methodology for INDOEX. J. Geophys. Res. 106, 7313–7336. doi:10.1029/2000JD900507
Crisp, D., Fisher, B. M., O’Dell, C., Frankenberg, C., Basilio, R., Bösch, H., et al. (2012). The ACOS CO2 retrieval algorithm – Part II: global XCO2 data characterization. Atmos. Meas. Tech. 5, 687–707. doi:10.5194/amt-5-687-2012
Davis, A., Marshak, A., Cahalan, R., and Wiscombe, W. (1997). The Landsat scale–break in stratocumulus as a three–dimensional radiative transfer effect: implications for cloud remote sensing. J. Atmos. Sci. 54 (2), 241–260. doi:10.1175/1520-0469(1997)054<0241:TLSBIS>2.0.CO;2
Delanoë, J., and Hogan, R. J. (2010). Combined CloudSat-CALIPSO- MODIS retrievals of the properties of ice clouds. J. Geophys. Res. 115, D00H29. doi:10.1029/2009JD012346
Diffenbaugh, N. S., Field, C. B., and Wong-Parodi, E. A. (2020). The COVID-19 lockdowns: a window into the Earth System. Nat. Rev. Earth Environ. 1, 470–481. doi:10.1038/s43017-020-0079-1
Diner, D. J., Brauer, M., Bruegge, C., Burke, K. A., Chipman, R., Di Girolamo, L., et al. (2018). Advances in multiangle satellite remote sensing of speciated airborne particulate matter and association with adverse health effects: from MISR to MAIA. J. Appl. Remote Sens. 12, 042603. doi:10.1117/1.JRS.12.042603
Dubovik, O., Herman, M., Holdak, A., Lapyonok, T., Tanré, D., Deuzé, J. L., et al. (2011). Statistically optimized inversion algorithm for enhanced retrieval of aerosol properties from spectral multi-angle polarimetric satellite observations. Atmos. Meas. Tech. 4, 975–1018. doi:10.5194/amt-4-975-2011
Dubovik, O., Lapyonok, T., Litvinov, P., Herman, M., Fuertes, D., Ducos, F., et al. (2014). GRASP: a versatile algorithm for characterizing the atmosphere. SPIE: Newsroom. doi:10.1117/2.1201408.005558
Dubovik, O., Li, Z., Mishchenko, M. I., Tanre, D., Karol, Y., Bojkov, B., Cairns, B., et al. (2019). Polarimetric remote sensing of atmospheric aerosols: instruments, methodologies, results, and perspectives. J. Quant. Spectrosc. Radiait. Transfer 224, 474–511. doi:10.1016/j.jqsrt.2018.11.024
Elguindi, N., Granier, C., Stavrakou, T., Darras, S., Bauwens, M., Cao, H., et al. (2020). Intercomparison of magnitudes and trends in anthropogenic surfaceemissions from bottom-up inventories, top-down es-timates, and emission scenarios. Earth’s Future 8, e2020EF001520. doi:10.1029/2020EF001520
European Space Agency (2020). CO2M, MRD (Mission Requirements Document), Copernicus CO2 monitoring mission requirements document. Version 3.0, Earth and Mission Science Division. https://esamultimedia.esa.int/docs/EarthObservation/CO2M_MRD_v3.0_20201001_Issued.pdf.
Evans, K. F.(1998). The spherical harmonics discrete ordinate method for three-dimensional atmospheric radiative transfer. JAS55, 429–446.
Evans, K. F., and Stephens, G. L. (1991). A new polarized atmospheric radiative transfer model,. J. Quant. Spectrosc. Radiat. Transfer 46 (5), 413–423. doi:10.1175/1520-0469(1998)055<0429:TSHDOM>2.0.CO;2
Fougnie, B., Marbach, T., Lacan, A., Lang, R., Schlüssel, P., Poli, G., et al. (2018). The multi- viewing multi-channel multi-polarisation imager–overview of the 3MI polari- metric mission for aerosol and cloud characterization. J. Quant. Spectrosc. Radiat. Transf. 219, 23–32. doi:10.1016/j.jqsrt.2018.07.008
Frouin, R., Franz, B., Ibrahim, A., Knobelspiesse, K., Ahmad, Z., Cairns, B., et al. (2019). Atmospheric correction of satellite ocean-color imagery during the PACE Era. Front. Earth Sci. 7, 145. doi:10.3389/feart.2019.00145
Fu, L. L., Lee, T., Liu, W. T., and Kwok, R. (2019). 50 years of satellite remote sensing of the ocean. Meteorol. Monogr. 59, 5.1–5.46. doi:10.1175/AMSMONOGRAPHS-D-18-0010.1
Gao, Y., Skutsch, M., Paneque-Gálvez, J., and Ghilardi, A. (2020). Remote sensing of forest degradation: a review. Environ. Res. Lett. 11, 1020. doi:10.3390/f11091020
Hasekamp, O. P. (2010). Capability of multi-viewing-angle photo-polarimetric measurements for the simultaneous retrieval of aerosol and cloud properties. Atmos. Meas. Tech. 3, 839–851. doi:10.5194/amt-3-839-2010
Hasekamp, O. P., and Landgraf, J. (2007). Retrieval of aerosol properties over land surfaces: capabilities of multiple-viewing-angle intensity and polarization measurements. Appl. Optic. 46, 3332–3344. doi:10.1364/AO.46.003332
Hasekamp, O. P., and Landgraf, J. (2005). Retrieval of aerosol properties over the ocean from multispectral single-viewing-angle measurements of intensity and polarization: retrieval approach, information content, and sensitivity study. J. Geophys. Res. 110, D20207. doi:10.1029/2005JD006212
Henderson, D. S., L’Ecuyer, T., Stephens, G., Partain, P., and Sekiguchi, M. (2013). A multisensor perspective on the radiative impacts of clouds and aerosols. J. Appl. Meteorol. Climatol. 52 (4), 853–871. doi:10.1175/JAMC-D-12-025.1
Illingsworth, A., Barker, H., Beljaars, A., Ceccaldi, M., Chepfer, H., Clerbaux, N., et al. (2015). The earthcare satellite: the next step forward in global measurements of clouds, aerosols, precipitation, and radiation. Bull. Am. Meteorol. Soc. 96, 1311–1332. doi:10.1175/BAMS-D-12-00227.1
Janssens-Maenhout, G., Pinty, B., Dowell, M., Zunker, H., Andersson, E., Balsamo, G., et al. (2020). Towards an operational anthropogenic CO2 emissions monitoring and verification support capacity. Bull. Am. Meteorol. Soc. 101, E1439–E1451. doi:10.1175/BAMS-D-19-0017.1
Jeyaseelan, A. T. (2004). “Droughts and floods assessment and monitoring using remote sensing and GIS,” in Proceedings of satellite remote sensing and GIS applications in agricultural meteorology, 291–313.
Kadhim, N., Mourshed, M., and Bray, M. (2016). Advances in remote sensing applications for urban sustainability. Euromediterr J. Environ. Integr. 1, 7. doi:10.1007/s41207-016-0007-4
Kahn, R. A., Berkoff, T. A., Brock, C., Chen, G., Ferrare, R. A., Ghan, S., et al. (2017). SAM-CAAM: a concept for acquiring systematic aircraft measurements to characterize aerosol air masses. Bull. Am. Meteorol. Soc. 98, 2215–2228. doi:10.1175/BAMS-D-16-0003.1
Källén, E. (2008). Special issue with manuscripts related to ESA’s atmospheric dynamics mission/Aeolus. Tellus Dyn. Meteorol. Oceanogr. 60 (2), 189–190. doi:10.1111/j.1600-0870.2007.00296.x
Kato, S., Rose, F. G., Sun-Mack, S., Miller, W. F., Chen, Y., Rutan, D. A., et al. (2011). Improvements of top-of-atmosphere and surface irradiance computations with CALIPSO, CloudSat, and MODIS derived cloud and aerosol properties. J. Geophys. Res. 116, D19209. doi:10.1029/2011JD16050
Kato, S., Sun-Mack, S., Miller, W. F., Rose, F. G., Chen, Y., Minnis, P., et al. (2010). Relationships among cloud occurrence frequency, overlap, and effective thickness derived from CALIPSO and CloudSat merged cloud vertical profiles. J. Geophys. Res. 115, D00H28. doi:10.1029/2009JD012277
Kim, J., Jeong, U., Ahn, M.-H., Kim, J. H., Park, R. J., Lee, H., et al. (2020). New Era of air quality monitoring from space geostationary environment monitoring spectrometer (GEMS). Bull. Am. Meteorol. Soc. 101 (1), E1–E22. doi:10.1175/BAMS-D-18-0013
King, M. D., Kaufman, Y. J., Tanre´, D., and Nakajima, T. (1999). Remote sensing of tropospheric aerosols from space: past, present, and future. Bull. Am. Meteorol. Soc. 80, 2229–2259. doi:10.1175/1520-0477(1999)080<2229:RSOTAF>2.0.CO;2
Knobelspiesse, K., and Nag, S. (2018). Remote sensing of aerosols with small satellites in formation flight. Atmos. Meas. Tech. 11, 3935–3954. doi:10.5194/amt-11-3935-2018
Knobelspiesse, K., Cairns, B., Ottaviani, M., Ferrare, R., Hair, J., Hostetler, C., et al. (2011). Combined retrievals of boreal forest fire aerosol properties with a polarimeter and lidar. Atmos. Chem. Phys. 11, 7045–7067. doi:10.5194/acp-11-7045-2011
Kohlhase, C. E., and Penzo, P. A. (1977). Voyager mission description, Space Sci. Rev. 21, 77–101. doi:10.1007/BF00200846
Kwok, R., Zwally, H. J., and Yi, D. (2004). ICESat observations of Arctic sea ice: a first look. Geophys. Res. Lett. 31, L16401. doi:10.1029/2004GL020309
L’Ecuyer, T., and Jiang, J. (2010). Touring the atmosphere aboard the A-Train. Phys. Today 46, 36–41. doi:10.1063/1.3463626
Lakshmi, V. (2013). Remote sensing of soil moisture. ISRN Soil Sci. 33, 1–33. doi:10.1155/2013/424178
Lary, D. J., Alavi, A. H., Gandomi, A. H., and Walker, A. L. (2016). Machine learning in geosciences and remote sensing. Geosci. Front. 7, 3–10. doi:10.1016/j.gsf.2015.07.003
Lebsock, M., L’Ecuyer, T., and Stephens, G. (2007). Information content of near-infrared spaceborne multiangular polarization measurements for aerosol retrievals. J. Geophys. Res. 112, D14206. doi:10.1029/2007JD008535
Lentile, L. B., Holden, Z. A., Smith, A. M. S., Falkowski, M. J., Hudak, A. T., Morgan, P., et al. (2006). Remote sensing techniques to assess active fire characteristics and post-fire effects. Int. J. Wildland Fire 15, 319–345. doi:10.1071/WF05097
Li, L., Dubovik, O., Derimian, Y., Schuster, G. L., Lapyonok, T., Litvinov, P., et al. (2019). Retrieval of aerosol components directly from satellite and ground-based measurements. Atmos. Chem. Phys. 19, 13409–13443. doi:10.5194/acp-19-13409-2019
Li, Z., Hou, W., Hong, J., Zheng, F., Luo, D., Wang, J., et al. (2018). Directional Polarimetric Camera (DPC): monitoring aerosol spectral optical properties over land from satellite observation. J. Quant. Spectrosc. Radiait. Transfer 218, 21–37. doi:10.1016/j.jqsrt.2018.07.003
Liang, L., and Di Girolamo, L. (2013). A global analysis on the view-angle dependence of plane-parallel oceanic liquid water cloud optical thickness using data synergy from MISR and MODIS. J. Geophys. Res. Atmos. 118, 2389–2403. doi:10.1029/2012JD018201
Liu, Y., Park, R. J., Jacob, D. J., Li, Q. B., Kilaru, V., and Sarnat, J. A. (2004). Mapping annual mean ground-level PM2.5 concentrations using Multiangle Imaging Spectroradiometer aerosol optical thickness over the contiguous United States. J. Geophys. Res. Atmos. 109, D22206. doi:10.1029/2004JD005025
Liu, Y., Franklin, M., Kahn, R., and Koutrakis, P. (2007). Using aerosol optical thickness to predict ground-level PM2.5 concentrations in the St. Louis area: a comparison between MISR and MODIS. Remote Sens. Environ. 107, 33–44. doi:10.1016/j.rse.2006.05.022
Lopatin, A., Dubovik, O., Chaikovsky, A., Goloub, Ph., Lapyonok, T., Tanré, D., et al. (2013). Enhancement of aerosol characterization using synergy of lidar and sun – photometer coincident ob-servations: the GARRLiC algorithm. Atmos. Meas. Tech. 6, 2065–2088. doi:10.5194/amt-6-2065-2013
Lopatin, A., Dubovik, O., Fuertes, D., Stenchikov, G., Lapyonok, T., Veselovskii, I., et al. (2020). Synergy processing of diverse ground-based remote sensing and in situ data using GRASP algorithm: applications to radiometer, lidar and radiosonde observations. Atmos. Meas. Tech. Discuss. doi:10.5194/amt-2020-422
Lukashin, C., Goldin, D., and Hutchinson, C., Roithmayr Roithmayr, C. M., Sun, W., Thome, K., et al. (2017). CLARREO Pathfinder: on-orbit data matching and sensor inter-calibration,” in IEEE international geoscience and remote sensing symposium (IGARSS) (Fort Worth, TX: IEEE), 297–300.
Lyapustin, A. (2002). Radiative transfer code SHARM-3D for radiance simulations over a non-Lambertian nonhomogeneous surface: intercomparison study. Appl. Optics 41, 5607–5615. doi:10.1364/AO.41.005607
Lyapustin, A., and Wang, Y. (2005). Parameterized code SHARM-3D for radiative transfer over inhomogeneous surfaces. Appl. Opt. 44, 7602–7610. doi:10.1364/AO.44.007602
Mace, G. G., and Zhang, Q. (2014). The CloudSat radar-lidar geometrical profile product (RL-GeoProf): updates, improvements, and selected results. J. Geophys. Res. Atmos. 119, 9441–9462. doi:10.1002/2013JD021374
Matson, D. L., Spilker, L. J., and Lebreton, J.-P. (2002). The Cassini/Huygens mission to the saturnian system. Space Sci. Rev. 104, 1–58. doi:10.1023/A:1023609211620
Milinevsky, G., Oberemok, Y., Syniavskyi, I., Bovchaliuk, A., Kolomiets, I., Fesyanov, I., et al. (2019). Calibration model of polarimeters on board the Aerosol-UA space mission. J. Quant. Spectrosc. Radiait. Transf. 229, 92–105. doi:10.1016/j.jqsrt.2019.03.007
Mishchenko, M. I., and Travis, L. D. (1997). Satellite retrieval of aerosol properties over the ocean using polarization as well as intensity of reflected sunlight. J. Geophys. Res. 102, 16989–17013. doi:10.1029/96jd02425
National Academies of Sciences, Engineering, and Medicine (2015). Continuity of NASA Earth observations from space: a value framework. Washington, DC: The National Academies Press.
National Academies of Sciences, Engineering, and Medicine (2018). Thriving on our changing planet: a decadal strategy for Earth observation from space. Washington, DC: The National Academies Press.
Neukermans, G., Harmel, T., Galí, M., Rudorff, N., Chowdhary, J., Dubovik, O., et al. (2018). Harnessing remote sensing to address critical science questions on ocean-atmosphere interactions. Elem. Sci. Anth. 6, 71. doi:10.1525/elementa.331
Neumann, T., Martinoa, A. J., Markusa, T., Bae, S., Bock, M. R., Brenner, A. C., et al. (2019). The ice, cloud, and land elevation satellite – 2 mission: a global geolocated photon product derived from the advanced topographic laser altimeter system. Remote Sens. Environ. 233, 111325. doi:10.1016/j.rse.2019.111325
Randles, C. A., da Silva, A. M., Buchard, V., Colarco, P. R., Darmenov, A., Govindaraju, R., et al. (2017). The MERRA-2 aerosol reanalysis, 1980 onward. Part I: system description and data assimilation evaluation. J. Clim. 30 (17), 6823–6850. doi:10.1175/JCLI-D-16-0609.1
Reichstein, M., Camps-Valls, M. G., Stevens, B., Jung, M., Denzler, J., Carvalhais, N., et al. (2019). Deep learning and process understanding for data-driven Earth system science. Nature 566 (7743), 195–204. doi:10.1038/s41586-019-0912-1
Remer, L. A., Davis, A. B., Mattoo, S., Levy, R. C., Kalashnikova, O. V., Coddington, O., et al. (2019a). Retrieving aerosol characteristics from the PACE mission, Part 1: ocean color instrument. Front. Earth Sci. 7, 152. doi:10.3389/feart.2019.00152
Remer, L. A., Knobelspiesse, K., Zhai, P.-W., Xu, F., Kalashnikova, O., Chowdhary, J., et al. (2019b). Retrieving aerosol characteristics from the PACE mission, Part 2: multi-angle and polarimetry. Front. Environ. Sci. 2017, 7. doi:10.3389/fenvs.2019.00094
Román, R., Benavent-Oltra, J. A., Casquero-Vera, J. A., Lopatin, A., Cazorla, A., Lyamani, H., et al. (2018). Retrieval of aerosol profiles combining sunphotometer and ceilometer measurements in GRASP code. Atmos. Res. 204, 161–177. doi:10.1016/j.atmosres.2018.01.021
Sassen, K., and Cho, B. S. (1992). Subvisual-thin cirrus lidar dataset for satellite verification and climatological research. J. Appl. Meteorol. Climat. 31 (11), 1275–1285. doi:10.1175/1520-0450(1992)031<1275:STCLDF>2.0.CO;2
Sayer, A., Govaerts, Y., Kolmonen, P., Lipponen, A., Luffarelli, M., Mielonen, T., et al. (2020). A review and framework for the evaluation of pixel- level uncertainty estimates in satellite aerosol remote sensing. Atmos. Meas. Tech. 13, 373–404. doi:10.5194/amt-13-373-2020
Stamnes, S., Fan, Y., Chen, N., Li, W., Tanikawa, T., Lin, Z., et al. (2018). Advantages of measuring the Q Stokes parameter in addition to the total radiance I in the detection of absorbing aerosols. Front. Earth Sci. 6, 34. doi:10.3389/feart.2018.00034
Stephens, G. L., Vane, D. G., Boain, R. J., Mace, G. G., Sassen, K., Wang, Z., et al. (2002). The CloudSat mission and the A-Train. Bull. Am. Meteorol. Soc. 83, 1771–1790. doi:10.1175/BAMS-83-12-1771
Thies, B., and Bendix, J. (2011). Satellite based remote sensing of weather and climate: recent achievements and future perspectives. Meteorol. Appl. 18, 262–295. doi:10.1002/met.288
Várnai, T., and Marshak, A. (2001). Statistical analysis of the uncertainties in cloud optical depth retrievals caused by three-dimensional radiative effects. J. Atmos. Sci. 58 (12), 1540–1548. doi:10.1175/1520-0469(2001)058<1540:SAOTUI>2.0.CO;2
Waquet, F., Cornet, C., Deuzé, J.-L., Dubovik, O., Ducos, F., Goloub, P., et al. (2013). Retrieval of aerosol microphysical and optical properties above liquid clouds from POLDER/PARASOL polarization measurements. Atmos. Meas. Tech. 6, 991–1016. doi:10.5194/amt-6-991-2013
Weitkamp, C. (2005). LIDAR: range-resolved optical remote sensing of the atmosphere. New York: Springer.
Werdell, P. J., Behrenfeld, M. J., Bontempi, P. S., Boss, E. S., Cairns, B., Davis, G. T., et al. (2019). The plankton, aerosol, cloud, ocean ecosystem (PACE) mission: status, science, advances. Bull. Am. Meteorol. Soc. 100, 1775–1794. doi:10.1175/BAMS-D-18-0056.1
Wielicki, B. A., Barkstrom, B. R., Harrison, E. F., Lee, R. B., Smith, G. L., and Cooper, J. E. (1996). Clouds and the Earth’s Radiant Energy System (CERES): an Earth observing system experiment. Bull. Am. Meteorol. Soc. 77, 853–868. doi:10.1175/1520-0477(1996)077<0853:CATERE>2.0.CO;2
Wielicki, B., Young, D. F., Mlynczak, M. G., Thome, K. J., Leroy, S., Corliss, J., et al. (2013). Achieving climate change absolute accuracy in orbit, Bull. Am. Meteorol. Soc. 94, 1520–1539. doi:10.1175/BAMS-D-12-00149.1
Winker, D. M., Couch, R. H., and McCormick, P. (1996). An overview of LITE: NASA’s lidar in-space technology experiment, proc. IEEE 84, 164–180.
Winker, D. M., Hunt, W. H., and McGill, M. J. (2007). Initial performance assessment of CALIOP. Geophys. Res. Lett. 34, L19803. doi:10.1029/2007GL030135
Winker, D. M., Vaughan, M. A., Omar, A., Hu, Y., Powell, K. A., Liu, Z., et al. (2009). Overview of the CALIPSO mission and CALIOP data processing algorithms. J. Atmos. Ocean. Technol. 26, 2310–2323. doi:10.1175/2009JTECHA1281.1
Wu, L., Hasekamp, O., van Diedenhoven, B., and Cairns, B. (2015). Aerosol retrieval from multiangle, multispectral photopolarimetric measurements: importance of spectral range and angular resolution. Atmos. Meas. Tech. 8, 2625–2638. doi:10.5194/amt-8-2625-2015
Xie, Y., Sha, Z., and Yu, M. (2008). Remote sensing imagery in vegetation mapping: a review. J. Plant Ecol. 1, 9–23. doi:10.1093/jpe/rtm005
Xu, F., Dubovik, O., Zhai, P.-W., Diner, D. J., Kalashnikova, O. V., Seidel, F. C., et al. (2016). Joint retrieval of aerosol and water-leaving radiance from multi-spectral, multi-angular and polarimetric measurements over ocean. Atmos. Meas. Tech. 9, 2877–2907. doi:10.5194/amt-9-2877-2016
Xu, F., van Harten, G., Diner, D. J., Kalashnikova, O. V., Seidel, F. C., Bruegge, C. J., et al. (2017). Coupled retrieval of aerosol properties and land surface reflection using the Airborne Mul-tiangle SpectroPolarimetric Imager (AirMSPI). J. Geophys. Res. Atmos. 122, 7004–7026. doi:10.1002/2017JD026776
Xu, F., van Harten, G., Diner., D. J., Davis, A. B., Seidel, F., Rheingans, B., et al. (2018). Coupled retrieval of cloud and aerosol above cloud properties using AirMSPI. J. Geophys. Res. Atmos. 123, 3175–3204. doi:10.1002/2017JD027926
Yang, P., Liou, K. N., Bi, L., Liu, C., Yi, B., and Baum, B. A. (2015). On the radiative properties of ice clouds: light scattering, remote sensing, and radiation parameterization. Adv. Atmos. Sci. 3, 32–63. doi:10.1007/s00376-014-0011-z
Yang, P., Liou, K. N., Bi, L., Liu, C., Yi, B. Q., and Baum, B. A. (2002). On the radiative properties of ice clouds: light scattering, remote sensing, and radiation parameterization. Adv. Atmos. Sci. 32, 32–63. doi:10.1007/s00376-014-0011-z
Yorks, J. E., McGill, M. J., Palm, S. P., Hlavka, D. L., Selmer, P. A., Nowottnick, E. P., et al. (2016). An overview of the CATS level 1 processing algorithms and data products. Geophys. Res. Lett. 43, 4632–4639. doi:10.1002/2016GL068006
Keywords: satellite remote sensing, atmospheric aerosol, trace gases, land cover, clouds, synergy
Citation: Dubovik O, Schuster GL, Xu F, Hu Y, Bösch H, Landgraf J and Li Z (2021) Grand Challenges in Satellite Remote Sensing. Front. Remote Sens. 2:619818. doi: 10.3389/frsen.2021.619818
Received: 21 October 2020; Accepted: 07 January 2021;
Published: 24 February 2021.
Edited by:
Seiji Kato, National Aeronautics and Space Administration (NASA), United StatesReviewed by:
Oliver Lux, Helmholtz Association of German Research Centers (HZ), GermanyAlexei Lyapustin, National Aeronautics and Space Administration, United States
Copyright © 2021 Dubovik, Schuster, Xu, Hu, Bösch, Landgraf and Li. This is an open-access article distributed under the terms of the Creative Commons Attribution License (CC BY). The use, distribution or reproduction in other forums is permitted, provided the original author(s) and the copyright owner(s) are credited and that the original publication in this journal is cited, in accordance with accepted academic practice. No use, distribution or reproduction is permitted which does not comply with these terms.
*Correspondence: Oleg Dubovik, b2xlZy5kdWJvdmlrQHVuaXYtbGlsbGUuZnI=